- 1School of Graduates Studies, Program in Neural and Behavioral Sciences, State University of New York, Downstate Health Sciences University, Brooklyn, NY, United States
- 2Institute for Genomics in Health, State University of New York, Downstate Health Sciences University, Brooklyn, NY, United States
- 3School of Graduate Studies, Program in Molecular and Cell Biology, State University of New York, Downstate Health Sciences University, Brooklyn, NY, United States
- 4Department of Pathology, State University of New York, Downstate Health Sciences University, Brooklyn, NY, United States
- 5The Robert F. Furchgott Center for Neural and Behavioral Science, State University of New York, Downstate Health Sciences University, Brooklyn, NY, United States
- 6Department of Cell Biology, State University of New York, Downstate Health Sciences University, Brooklyn, NY, United States
- 7Department of Genetics, Human Genetics Institute of New Jersey, Rutgers University, Piscataway, NJ, United States
The rodent hippocampus is a spatially organized neuronal network that supports the formation of spatial and episodic memories. We conducted bulk RNA sequencing and spatial transcriptomics experiments to measure gene expression changes in the dorsal hippocampus following the recall of active place avoidance (APA) memory. Through bulk RNA sequencing, we examined the gene expression changes following memory recall across the functionally distinct subregions of the dorsal hippocampus. We found that recall induced differentially expressed genes (DEGs) in the CA1 and CA3 hippocampal subregions were enriched with genes involved in synaptic transmission and synaptic plasticity, while DEGs in the dentate gyrus (DG) were enriched with genes involved in energy balance and ribosomal function. Through spatial transcriptomics, we examined gene expression changes following memory recall across an array of spots encompassing putative memory-associated neuronal ensembles marked by the expression of the IEGs Arc, Egr1, and c-Jun. Within samples from both trained and untrained mice, the subpopulations of spatial transcriptomic spots marked by these IEGs were transcriptomically and spatially distinct from one another. DEGs detected between Arc + and Arc− spots exclusively in the trained mouse were enriched in several memory-related gene ontology terms, including “regulation of synaptic plasticity” and “memory.” Our results suggest that APA memory recall is supported by regionalized transcriptomic profiles separating the CA1 and CA3 from the DG, transcriptionally and spatially distinct IEG expressing spatial transcriptomic spots, and biological processes related to synaptic plasticity as a defining the difference between Arc + and Arc− spatial transcriptomic spots.
1 Introduction
The neural operations supporting memory require the involvement of various brain systems interconnected through neural networks (Kitamura et al., 2017). The activity of these networks is fine-tuned by experience through the selective recruitment of ensembles of neurons ascribed to contain the bits of information associated with memory (Josselyn and Tonegawa, 2020). Neuronal ensemble activity is shaped by synaptic plasticity mechanisms that modulate the weight and efficacy of the ensemble’s synaptic connections (Takeuchi et al., 2014; Malenka and Bear, 2004). Changes in gene expression are a key underlying mechanism in synaptic plasticity (Mayford et al., 2012), and recent studies have identified multiple profiles of gene expression associated with memory (Marco et al., 2020; Rao-Ruiz et al., 2019; Reshetnikov et al., 2020).
In the rodent hippocampus, encoding of spatial memory information is supported by the diversity of synaptic computations within and across its sub-fields (Poo et al., 2016; Mayford, 2014). Each hippocampal subregion (i.e., Dentate Gyrus, CA1, CA2, CA3) shows distinct patterns of activity to process spatial information and to form or retrieve memories (Middleton and McHugh, 2020; Chevaleyre and Siegelbaum, 2010; Senzai, 2019; Basu and Siegelbaum, 2015; Amaral and Witter, 1989; Sloviter and Lomo, 2012). At the regional level, patterns of activity are conferred through the combination of cyto-architecture, local synaptic circuitry, neuronal cell types and synaptic inputs unique to each region (van Strien et al., 2009; Senzai, 2019; Kesner et al., 2004; Lisman, 1999; Ryan et al., 2015; Hunsaker and Kesner, 2013; Molitor et al., 2021; Rao-Ruiz et al., 2019; Shpokayte et al., 2022; Sullivan et al., 2021; Shah et al., 2016; Cho et al., 2016). At the single-cell level, the functional state of hippocampal neurons is defined by connectivity, hippocampal subregional location, and experience-dependent recruitment into a memory ensemble (Josselyn and Tonegawa, 2020; Tonegawa et al., 2018). The investigation of memory across molecular and functional levels necessitates an approach that connects the scales of hippocampal organization between cellular mechanisms and networks of cells. Though hundreds of individual genes supporting synaptic plasticity at the cellular level have been identified (Sanes and Lichtman, 1999; Alberini and Kandel, 2014; Asok et al., 2019; Magee and Grienberger, 2020; Malenka and Bear, 2004), the transcriptomic profiles which characterize memory across hippocampal subregions and memory-associated neuronal ensembles are understudied.
In this study, we present an exploratory approach to expand our understanding of the spatial organization of transcriptomic profiles by providing evidence that hippocampal subregions undergo distinct differential gene expression of biological mechanisms to support memory. We characterized changes in gene expression across the major subregions of the hippocampus using bulk RNA sequencing of micro-dissected dorsal hippocampal subregions from mice that learned an active place avoidance memory task. The regionalized changes in gene expression with memory in the hippocampus is a developing area of investigation often using behavioral paradigms like fear conditioning or the Morris Water Maze (Cho et al., 2016; Sardoo et al., 2022; Reshetnikov et al., 2020; Harris et al., 2020). Our approach involved training mice in the active place avoidance paradigm (Cimadevilla et al., 2000; Stuchlik et al., 2013), a spatial memory task that distinguishes itself from these other aversive paradigms because it requires proper utilization of cognitive control to solve the spatial task (Chung et al., 2021; Kelemen and Fenton, 2016). Results from our bulk RNA sequencing data suggest that the consolidation of an active place avoidance memory is supported by regionalized transcriptomic profiles separating the CA1 and CA3 from the DG.
Following the establishment of the memory-associated transcriptomic profiles of bulk hippocampal subregions we deepened our exploration of these transcriptomic changes using an innovative RNA sequencing methodology known as spatial transcriptomics. Spatial transcriptomics provides localized whole transcriptome sequencing across tissue sections at near single cell resolution (a.k.a. spatial transcriptomic spots) (Stahl et al., 2016). With this approach, we investigated the putative memory-associated neuronal ensemble by characterizing the spatial distribution of immediate early gene (IEG)-associated gene expression across coronal dorsal hippocampal sections. Memory-associated neuronal ensembles are a sparsely distributed population of neurons which are strongly activated together during the acquisition and recall of a memory, and are thought to encode the traces of information relevant to that memory (Ryan et al., 2015; Guan et al., 2016; Chen et al., 2019). Numerous studies have identified IEGs such as Arc, c-Fos, Egr1, c-Jun, Npas4, Bdnf, and Fmrp to characterize memory-associated neuronal ensembles (Sauvage et al., 2019; Josselyn and Tonegawa, 2020; Meenakshi et al., 2021; Erwin et al., 2020). Moreover, the expression of Arc mRNA has been used to tag and characterize the properties of memory-associated neuronal ensembles (Guzowski et al., 1999; Ramirez-Amaya et al., 2005; Lee et al., 2022).
Through our combined understanding of IEG activation and memory-associated neuronal ensembles, we used the detection of IEG expression in spatial transcriptomic spots to infer the location of these ensembles and explore the transcriptomic changes supporting spatial memory in the hippocampus. Consistent with our bulk RNA sequencing findings, spatial transcriptomics also showed differential gene expression between the hippocampal regions from an active place avoidance memory trained compared to an untrained mouse. We then investigated the difference between memory-associated neuronal populations through the analysis of spatial transcriptomic spots expressing the IEGs Arc, Egr1, and c-Jun. Comparison of the gene expression profiles from these IEG-expressing spatial transcriptomic spots between the memory trained and the untrained mouse revealed differential expression of genes involved in energy production and cytoplasmic translation. Novel analyses comparing IEG-expressing spatial transcriptomic spots within each mouse hippocampal sample (i.e., within the memory trained mouse or within the untrained mouse) revealed that IEG-expressing spatial spots were transcriptomically and spatially distinct from one another. With Arc, only the comparison between Arc-expressing and non-Arc-expressing spatial transcriptomic spots in the memory trained mouse –but not the untrained mouse– detected gene expression profiles linked with synaptic plasticity and memory. Additionally, similar comparisons amongst Egr1 and c-Jun expressing versus non-expressing spots revealed collections of differentially expressed distinguishing each of the investigated populations of IEG-expressing spots within the trained sample.
While still at an exploratory level, our spatial transcriptomics results highlight the role of Arc in shaping memory-associated ensembles and of the detection of gene expression profiles unique to the comparison Arc-expressing versus non-Arc-expressing spatial transcriptomic spots in an animal trained in the active place avoidance memory task. We speculate that a neuronal network being actively recruited in the consolidation of memory (such as the hippocampal network in our study) manifests transcriptomically divergent cellular microenvironments (such as the Arc-expressing and non-Arc-expressing spatial transcriptomic spots in our study) that could facilitate the processing of memory information.
2 Methods
2.1 Animals
A total of 18 adult ArcCreERT2::eYFPflx mice (Denny et al., 2014) with C57BL/6 genetic background aged 3–4 months were utilized across all experimental cohorts. Bulk RNA sequencing experiments utilized 3 male and 3 female mice, 2 females and 1 male were assigned to the trained behavioral condition, and 1 female and 2 males were assigned to the untrained behavioral condition (see section “Behavior” for details). Spatial transcriptomics experiments utilized 2 male mice, 1 mouse was assigned to each behavioral condition. RT-qPCR experiments utilized 12 male mice, with 6 mice assigned to each behavioral condition. Prior to experimental onset mice were bred in-house at the SUNY Downstate Health Sciences University vivarium (Brooklyn, NY, USA). Mice were housed in groups of two to five per cage. Beginning on the first day of training and testing, mice were single housed in shoebox cages in a sound attenuation cubicle (Med Associates). For every experimental run, a minimum of two mice —each undergoing the same behavioral conditioning— were simultaneously housed in the sound attenuation cubicle. Ad libitum food and water was provided. Mice were randomly assigned to behavioral cohorts before the start of the experiment. Mice were handled daily for 3 days prior to the start of the experiment to reduce anxiety and improve voluntary approach. All animal procedures proposed are approved by, and will be performed following, the Institutional Animal Care and Use Committee guidelines at SUNY Downstate Health Sciences University.
2.2 Behavior
All procedures were performed in compliance with the Institutional Animal Care and Use Committee of the State University of New York, Downstate Health Sciences University. ArcCreERT2::eYFPflx male mice were trained in a hippocampus-dependent two-frame active place avoidance task. The place avoidance system consisted of a 40-cm diameter arena with a parallel rod floor that could rotate at 1 rpm. The position of the animal was tracked using PC-based software (Tracker, Bio-Signal Group Corp., Brooklyn, NY) that analyzed 30-Hz digital video images from an overhead camera. Mice in the trained condition learned the active place avoidance task. Place avoidance of a 60° zone was reinforced by a constant current foot shock (60 Hz, 500 ms, 0.2 mA) that was scrambled (5-poles) across pairs of the floor rods. Rotation of the arena would carry the mouse into the shock zone unless the animal actively avoided the zone. Entering the shock zone for more than 500 ms triggered shock. Additional shocks occurred every 0.5 s until the animal left the shock zone. Measures of place avoidance were computed by TrackAnalysis software (Bio-Signal Group Corp., Brooklyn, NY, USA). The number of detections of the animal in the shock zone was tracked as the performance metric for the training trials.
On day one, mice assigned to the trained behavioral condition (trained mice) received a 30-min trial with the shock off to habituate to the rotating arena. Across the next 2 days the animals experienced four training trials, with two 30-min trials a day with the activated shock with a 40 min inter-trial-interval. Control (untrained mice) experienced identical training conditions, except for the shock always being off. The number of detections of the animal in the shock zone was tracked as the training performance metric for the training trials. Memory retention performance was assessed 24 h after the final training session in a 10-min retention test with the shock off. Latency to the animal’s second entrance into the shock zone was tracked as the memory retention performance metric for the retention test. Previous research indicates the latency to second entrance as a valid behavioral metric for retention test performance because it represents place avoidance memory recall that is less affected by to the animal’s potential errors in self-localization once placed in the rotating arena (Cimadevilla et al., 2000; Stuchlik et al., 2013; Cimadevilla et al., 2001).
2.3 Microdissected bulk RNA sequencing
60 min post retention test, mice were euthanized (5% isoflurane vaporized in 100% oxygen), and brains were extracted, washed in ice cold artificial cerebrospinal fluid, blocked, and mounted on a vibratome stage. Hippocampal subregions (DG, CA3, CA1) were microdissected from 400 μm thick coronal live tissue sections. Subregions from the dorsal hippocampus were extracted and microdissected using microsurgical tools. Microdissected pieces of tissue from each animal were pooled by subregion in 500 μL of prechilled TRIzol in a 1.5 mL microcentrifuge tubes and stored at −80°C.
Total RNA was extracted using the Direct-zol RNA miniprep kit (Zymo Research, Irvine, CA, United States) according to the manufacturer’s protocol. RNA quality was assessed on an Agilent 2200 TapeStation (Agilent Technologies, Palo Alto, CA, United States). Samples with a RIN greater than 7 were processed for library preparation with NEBnext rRNA depletion kit and ULTRAII FS RNA-seq Library Preparation Kit for Illumina (New England Biolabs, Ipswich, MA, United States). This procedure involved steps for mRNA enrichment, fragmentation, random primed cDNA synthesis, second strand synthesis, end repair, A-tailing, adaptor ligation, and PCR amplification. Size selection and cleanup was performed with SPRI select beads (Beckman Coulter, Indianapolis, IN, United States). Sequencing was performed on the NovaSeq 6000 (Illumina, Inc., San Diego, CA, United States) to obtain paired end sequencing reads.
2.4 Bulk RNA sequencing data preprocessing
RNA sequencing reads obtained from NovaSeq 6000 were systemically processed to ensure high-quality outputs for downstream analyses. To evaluate the quality of the reads, quality control analysis was performed using FASTQC v0.11.9. RNA sequencing reads in Binary Base Call (BCL) format were subsequently converted using bcl2fastq2 v2.20 to FASTQ format, and simultaneously demultiplexing to assign sequences to their respective samples based on index sequences. Demultiplexed samples that did not pass quality control were excluded from future analyses in the pipeline. Next, FASTQ files were then mapped to mouse MM10 (GRCm39) reference genome using STAR 2.7.10a aligner. Lastly, the transcript quantification of the reads was done using Salmon v1.2.0 to create a count matrix for downstream analyses.
2.5 Bulk RNA sequencing analyses
We conducted several differential expression analyses using DESeq2 v1.1.0 in the Illumina BaseSpace, comparing RNA counts between trained and untrained sample groups. Genes with a false discovery rate (FDR) below 0.05 were deemed differentially expressed. To identify the variances in our count data, we performed principal component analysis (PCA) on the normalized count data, encompassing all hippocampal regions and within each specific region.
To visualize our data, we generated a heatmap in ComplexHeatmap v2.18.0 using Z-scores, which standardized the expression levels of genes across samples (Gu et al., 2016). The heatmap, organized with dendrogram and sorted using Euclidean distance, allowed us to identify patterns of similarity in gene expression across samples.
To explore deeper into biological significance, we conducted Gene Ontology (GO) enrichment analysis using clusterProfiler v4.10.0 in R (Wu et al., 2021), focusing on biological processes, cellular components, and molecular functions. Only enriched GO terms with an FDR less than 0.01 were identified as significant, and the top 10 GO terms were displayed in a ranked dot plot. Redundant terms were filtered out using simplify with a cutoff of 0.7. Biological processes were ordered along the y-axis based on their ordered significance within each column from left to right. Additionally, if a biological process in one analysis is amongst the top 10 of another analysis, the dot signifying its enrichment will be shown at the corresponding row in the plot.
We also utilized Vennplex v1.0.0.2 software to compare and visualize the overlaps among differentially expressed genes (DEGs) from our differential expression analyses (Cai et al., 2013). The gene lists were categorized into upregulated and downregulated, allowing the visualizing of the gene overlaps and identification of counter-regulated genes (genes exhibiting opposite expression trends in different regions). We employed the SuperExactTest v1.1.0 in R to statistically assess whether the observed overlapping genes across different hippocampal regions significantly exceeded the expected overlap by random coincidence (Wang et al., 2015). The assessment was performed using hypergeometric distribution and Fisher’s Exact test, and the statistical significance of these gene overlaps was shown in upset-style plots.
2.6 Tissue preparation for spatial transcriptomics
60 min after retention test, mice were euthanized and brains were extracted and immediately prepared for snap freezing in cuvettes of Tissue-Tek Optimal Cutting Temperature compound (Sakura Finetek USA, Torrance, CA, United States) floating in a bath of methyl-butane chilled by liquid nitrogen. Brain blocks were cryosectioned at −20°C (10X Genomics, 2023) and 10 μm thick coronal sections containing the dorsal portion of the hippocampus were obtained (bregma −1.7 and − 2.2 mm, Paxinos and Franklin, 2019). Collected tissue sections are trimmed to fit within the 5 mm × 5 mm capture area on the Visium gene expression slide. Sections were mounted on a prechilled Visium gene expression slide or a Tissue Optimization slide.
For Optimization, all tissue sections were collected from a single tissue. For Visium gene expression, slide capture areas contained one section per biological sample. Slides were fixed in prechilled methanol before staining using the Visium spatial tissue optimization protocol (Genomics, 2019) or the Visium spatial gene expression protocol (Genomics, 2023).
Tissues permeabilization time on the gene expression slide was set to 18 min based on our tissue optimization results. Images were taken according to the Visium Spatial Gene Expression Imaging Guidelines (Genomics, 2021). Brightfield histology images for the Visium Tissue Optimization and Gene Expression slides were taken using the Leica Aperio CS2 Slide Scanner at 20x magnification. Tissue optimization fluorescent images were taken on a Zeiss LSM 800 (555 nm LED, 75% intensity, and 200 ms exposure).
mRNA was extracted and libraries were prepared following the Visium Spatial Gene Expression User Guide (Genomics, 2023). Libraries were indexed using the Dual Index Kit TT Set A (PN-1000215). Indexed libraries were loaded at 300 pM and sequenced on a NovaSeq 6000 System (Illumina) using a NovaSeq S4 Reagent Kit (200 cycles, catalog no. 20027466, Illumina), targeting a sequencing depth of approximately 2.0 × 108 read-pairs per sample. Sequencing depth was determined through a calculation of a suggestion 50,000 read pairs per spatial capture spot (Genomics, 2023). Our tissue samples covered roughly 80% of the 5,000 spatial capture spots within the Visium fiducial frame [(0.8 × 5,000) × 50,000 = 2.0 × 108]. Sequencing was performed using the following read protocol: read 1: 28 cycles; i7 index read: 10 cycles; i5 index read: 10 cycles; and read 2: 91 cycles.
2.7 Spatial transcriptomics preprocessing
Raw RNA sequencing data obtained from NovaSeq 6000 were systemically processed to ensure high-quality outputs for downstream analyses. Raw sequencing data in BCL format were converted using bcl2fastq2 v2.20 to FASTQ format, and simultaneously demultiplexing to assign sequences to their respective samples based on both i5 and i7 index sequences. Following the conversion, these FASTQ files were subsequently processed with TrimGalore v.0.6.5 to automate quality, adapter trimming and perform quality control.
For the alignment and the quantification of gene expression, Space Ranger v2.0.0 (10x Genomics) was used to generate and quantify raw gene-barcode matrices from RNA sequencing data. Alignment and quantification of Visium spatial gene expression data utilizes the same tools implemented in the analysis of single cell RNA-seq data sets. Data were aligned to refdata-gex-mm10-2020-A, a mouse genome index provided by 10x Genomics, which is an annotated version of mm10 genome assembly. The gene-barcode matrices identified the number of unique molecular identifiers (UMIs) associated with each gene for each cellular barcode, allowing the quantification of transcript abundance.
After the alignment and quantification, Space Ranger toolset was utilized to normalize the gene expression data within the raw gene-barcode matrices to account for any technical variations. Finally, we also utilized Space Ranger to align gene expression data with spatial coordinates derived from histological images of each sample, facilitating the visualization of transcriptional activity to specific tissue location.
2.8 Spatial transcriptomics analysis
Gene expression outputs from Space Ranger were analyzed using the software package Seurat v5 in the R coding environment (Hao et al., 2023; Butler et al., 2018). Spatial gene expression data were cropped to only include the capture spots within the dorsal hippocampus. Using default Seurat commands, data from each sample were normalized, transformed (using SCTransform v2) and integrated. The integrated data set were further normalized and clustered (resolution = 0.8) in PCA space. Clustered data can be visualized through UMAP projections of the capture spots or in the 2D space of the tissue section. Cell type annotation was performed by integrating our data with a random subset of 10,000 hippocampal cells from the Allen Brain Atlas Cortex and Hippocampus Single Cell Taxonomy (Yao et al., 2021). Predicted cell-type identities were calculated and optimized based on gene expression similarity and anatomical location.
Capture spots were grouped along two different criteria to calculate differential gene expression in our samples. First, capture spots belonging to the whole hippocampus and each anatomical cell layer in the DG, CA3 and CA1 regions were compared across training conditions. Next, within each training condition, hippocampal capture spots were separated into two groups based on the detectable (>0) expression of the immediate early gene (e.g., Arc + and Arc− groups). IEG expressing and not expressing groups of spots were compared both within training conditions and across training conditions. Differential gene expression was calculated using the Wilcoxon rank sum test (the default method for Seurat). Genes with an of FDR below 0.05 were considered differentially expressed genes/transcripts. GO term enrichment and overlap analyses on detected DEGs were performed as described above for bulk RNA sequencing DEGs.
Expression data for 23 IEGs from all hippocampal spots evaluated through pairwise Pearson’s Correlations using the R-language base stats package. Pearson’s correlation coefficients between all IEG pairs were calculated within samples, alongside p values to assess significance. Heatmaps of the correlation matrix were plotted using the R-language package, ComplexHeatmap.
2.9 RT-qPCR
Dorsal hippocampal subregions (CA1, CA3, and DG) were microdissected as described for bulk sequencing. Tissue was homogenized using TRIzol (Thermo Fisher Scientific, Waltham, MA, United States) before RNA extraction by guanidinium-phenol-chloroform extraction with ethanol precipitation was performed and quantified using spectrophotometry. cDNA was reverse transcribed from sample bulk RNA using Thermo Fisher’s SuperScript IV Kit (Thermo Fisher Scientific, Waltham, MA, United States). Relative gene expression was quantified using the SYBR green master mix system (Ponchel et al., 2003). 10 μL reactions were prepared in the wells of a 384 well plate, hippocampal regions were each prepared on separated plates. Each well contained 2.5 ng of template cDNA and 0.5 μM each of forward and reverse primers for one gene per well for a total reaction volume of 10 μL (sequences and melting temperatures can be found in Table 1). Each gene was loaded in triplicate in each plate to account for pipetting errors. Plates were incubated in the Bio-Rad CFX384 Real-Time PCR Detection System (Bio-Rad Life Sciences, Hercules, CA, United States) using the following schedule: 50°C for 5 min (1 cycle), 95°C for 10 min (1 cycle), 60°C for 1 min (1 cycle), and 95°C for 15 s followed by 60°C for 1 min (40 cycles).
Primers were selected to validate the most significant genes with the highest fold change of expression for each regional comparison of trained and untrained animals. The genes Crym and Kit were selected from the DEGs detected in the DG comparison. Rasal1 and Pcsk1n were selected from the DEGs detected in the CA3 comparison. And Bok, Homer3, Serpina3n, and Shank3 were selected from the DEGs detected in the CA1 comparison.
Ct values for each reaction are automatically determined (roughly 20% of the plateau value). Data were analyzed for relative gene expression quantification utilizing the ∆∆Ct method, using the expression of Gapdh as the internal control. Relative expression values were normalized to the expression value of the untrained group. The Levene’s test was uses to assess the homogeneity of variances for Log2 transformed relative expression. Upon confirmation of homogeneity of variances, Student’s t-tests were performed on each primer target across training conditions using log2 transformed relative expression value for each comparison.
3 Results
3.1 APA trained mice exhibit place avoidance memory
To investigate gene expression changes induced by active place avoidance (APA) training in the hippocampal network, we conducted bulk RNA-sequencing and spatial transcriptomics (see Figure 1A for experimental timeline). In the APA, mice learn to avoid a 60° shock zone on a rotating circular arena (Cimadevilla et al., 2000; Stuchlik et al., 2013). Mice trained in the APA task with the shock zone on (n = 4, 2 females, 2 male) exhibited a decrease in the number of detections in the shock zone during the acquisition trials (Figure 1B) and longer re-entry times to the shock zone during the memory retention test trials (Figure 1C) relative to the mice in the untrained cohort (n = 4, 1 female, 3 males). Re-entry times to the shock zone (i.e., time to second entrance) was used as the retention test performance metric because it represents performance that is more resistant to the animal’s potential errors in self-localization once placed in the rotating arena (Cimadevilla et al., 2000; Stuchlik et al., 2013; Cimadevilla et al., 2001). From this pool of animals 3 trained (2 females, 1 male) and 3 untrained (1 female, 2 male) were utilized in Bulk RNA-sequencing experiments and 1 trained and 1 untrained animal (2 males) were utilized in spatial transcriptomic experiments.
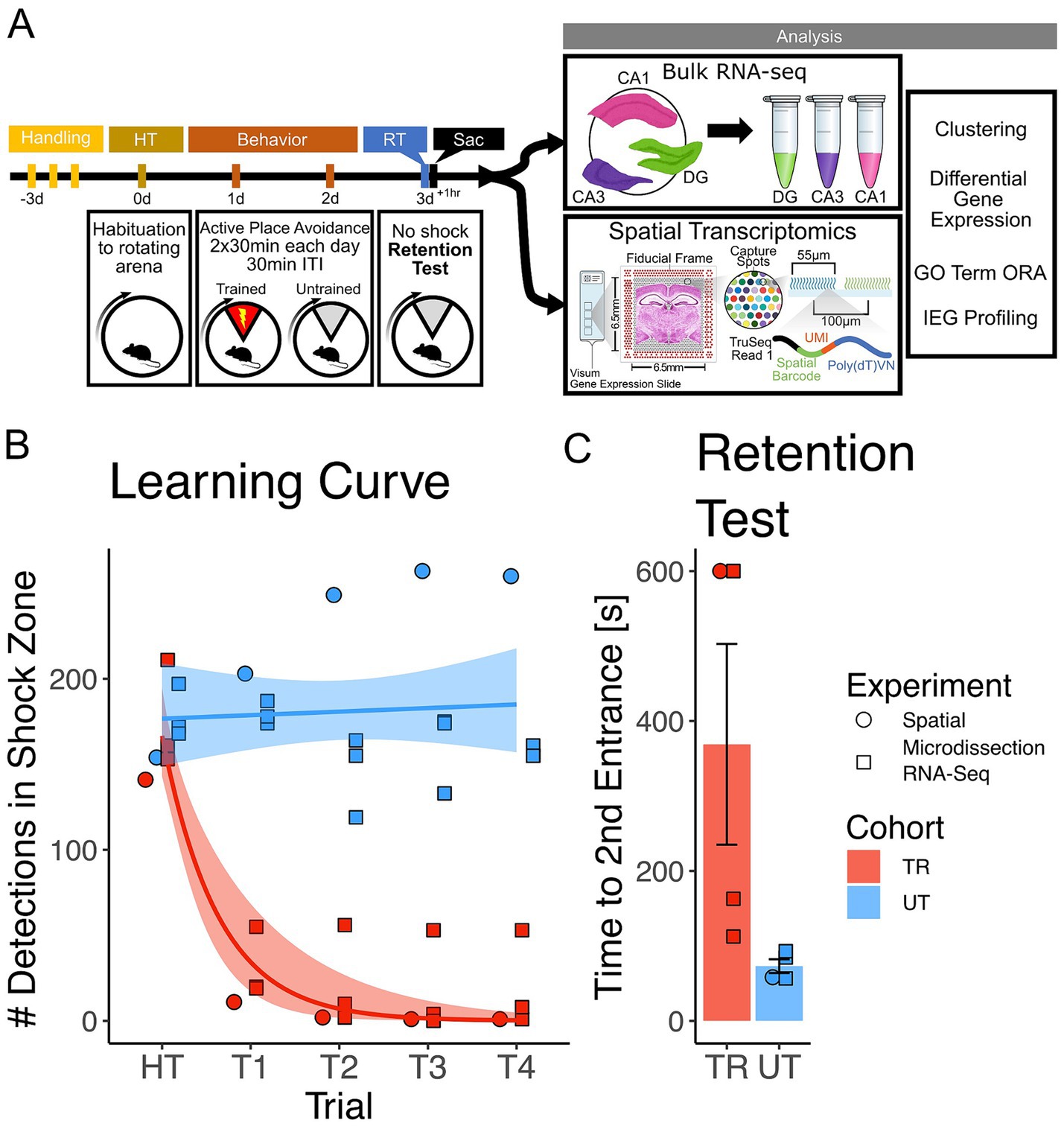
Figure 1. Mice trained in the APA learned to avoid the location of an unmarked shock zone. (A) Experimental timeline. Mice were trained in the active place avoidance paradigm over the course of 4 days following 3 days of handling. On day 0 mice were habituated (HT) to a rotating arena with no active shock zone. On day 1 and 2 mice assigned to the trained (TR) behavioral condition (n = 4) received two 30-min trials with an active shock zone. Mice assigned to the untrained (UT) behavioral condition (n = 4) were not exposed to an active shock zone. On day 3 all mice received a 10-min retention test trial (exposed to the rotating arena with no active shock zone). Mice were sacrificed 60 min following the end of the retention test, brains were collected for further processing. Samples from three of the four trained mice (2 females, 1 male) and untrained mice (1 female, 2 males) were processed with bulk RNA sequencing, while the remaining mice (1 trained male, 1 untrained male) had their samples processed with spatial transcriptomics. (B) Training performance measured as number detections in the shock zone. Mice assigned to the trained behavioral condition learned to avoid the location of the shock zone indicated by the decreased number of shocked triggered. Exponential fit learning curves included with shaded error bars (SE). (C) Memory performance measured as time to second entrance in the retention test trial. Trained mice demonstrated higher time to second entrance during the retention test. Trained mean 375 s ± 70.4 s (SE), Untrained mean 58.7 s ± 8.51 s (SE). Line and point color reflect the assigned behavioral condition (untrained = blue, trained = red). Point shape reflects the experimental pipeline a given animal was assigned to (square = bulk RNA-seq, circle = spatial transcriptomics).
3.2 Hippocampal subregional transcriptomes are stratified by training condition
To explore the molecular mechanisms following memory retention test across the dorsal hippocampus, bulk RNA-sequencing was performed on the Dentate Gyrus (DG), CA3, and CA1 hippocampal subregions collected from trained and untrained mice (trained: 2 females, 1 male; untrained: 1 female, 2 male). Principal component analysis (PCA) from all 3 subregions combined reveals a separation of samples by behavioral cohort (Figure 2A), indicating that differences in training contribute substantially to the overall variability of gene expression in our samples. Separation between samples is further accentuated when PCA is performed separately on data from each subregion (Figures 2B–D). Consistently, hierarchical clustering of gene expression profiles reliably grouped samples by their behavioral cohort (Figure 2E).
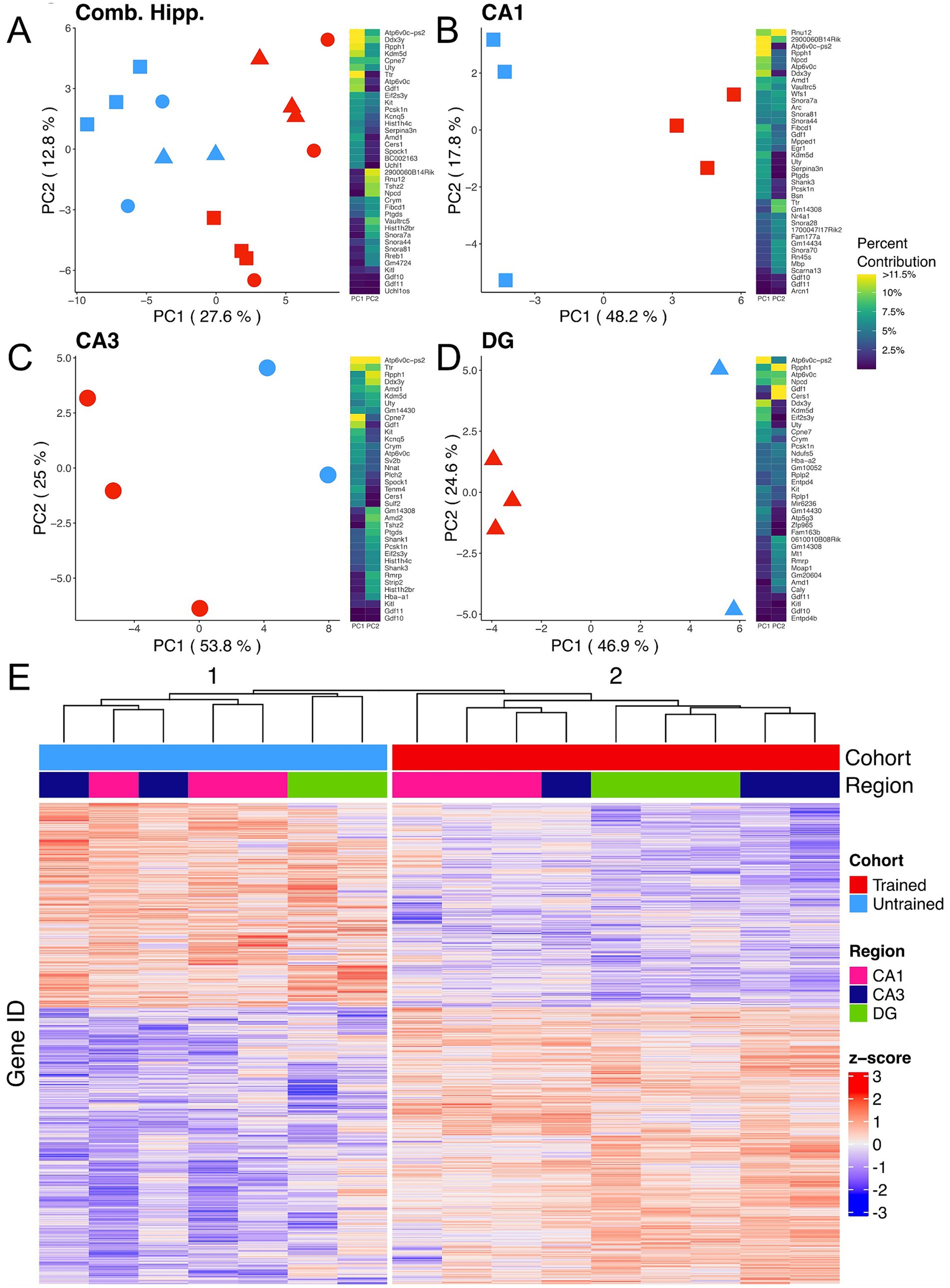
Figure 2. APA training results in substantial changes in hippocampal subregion gene expression profiles. (A–D) Left, principal component analyses of all hippocampal subregions (A), CA1 (B), CA3 (C), DG (D) illustrate a clear separation between samples collected from trained (red) and untrained (blue) mice along the axis that explains the most variance. Hippocampal regions are also indicated by the point shape, squares refer to the CA1, circles to the CA3, and triangles to the DG. Right, heatmap with top 20 contributing genes to the first two principal components of each are reported in the heat map to the right of each plot. Sample size for the CA1 was 3 trained and 3 untrained. Sample size for the CA3 was 3 trained and 2 untrained. And Sample size for the DG was 3 trained and 2 untrained. Two samples from separate untrained animals (1 DG, 1 CA3) did not pass sequencing quality control and were excluded from PCA analyses. (E) Normalized gene expression of the top 2000 most significant DEGs. Hierarchical clustering of gene expression profiles plotted as a dendrogram clusters samples by training condition.
3.3 Hippocampal subregional enrichment of learning and memory-related biological processes
We carried out differential gene expression analysis comparing APA-trained to untrained mice. We detected 1,663, 606, 315, and 350 differentially expressed genes (DEGs) (adjusted p-value <0.05) in the combined hippocampal subfields, CA1, CA3 and DG respectively, when contrasting APA-trained and untrained mice (Figures 3A–D and Supplementary Figures 1A–D). Despite high statistical significance (FDR < 0.005), most DEG in each trained versus untrained comparison exhibited modest fold change (|log2 fold| < 1.0) difference. Next, we investigated the overlap of regionally detected DEGs (FDR < 0.05) to elucidate the distribution of DEGs across hippocampal subregions. We found the largest overlap of upregulated DEGs between the CA3 and CA1 subregions with a total of 127 intersecting genes (Figure 3E and Supplementary Figure 1E).
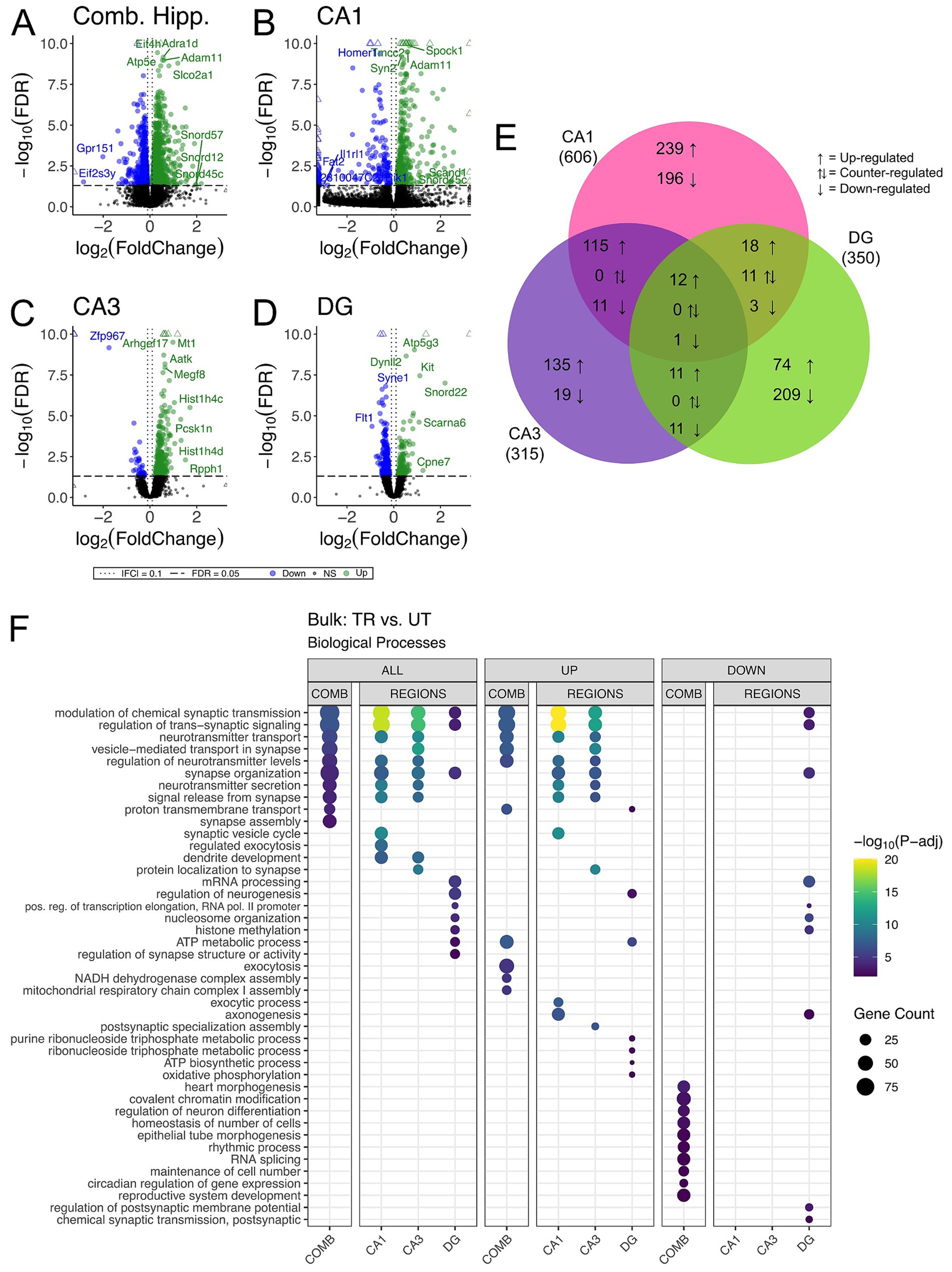
Figure 3. Bulk RNA-seq of hippocampal subregions reveals similar expression profiles in CA1 and CA3 regions in trained samples. (A–D) Volcano plot of DEGs between trained and untrained animals in all hippocampal subregions combined (A), and in the CA1 (B), CA3 (C), and DG (D) subregions individually. Sample size for the CA1 was 3 trained and 3 untrained. Sample size for the CA3 was 3 trained and 2 untrained. And Sample size for the DG was 3 trained and 2 untrained. Two samples from separate untrained animals (one DG, one CA3) did not pass sequencing quality control and were excluded from differential gene expression analyses. In all hippocampal subregions combined, 966 genes were upregulated and 697 were downregulated. 393 genes were upregulated and 213 were downregulated in the CA1, 273 genes were upregulated and 42 were downregulated in the CA3, and 117 genes were upregulated and 223 were downregulated in the DG. See Supplementary Figures 1A–D for full sized plots. (E) Overlaps in regional DEGs demonstrate greatest similarity between CA1 and CA3 subregions. DEGs from each regional analysis were stratified by direction of fold change. Overlaps in DEGs between the CA3 and CA1 subregions were significant (Fisher’s Exact Test). See Supplementary Figure 1E for further details. (F) Regional enrichment of biological processes detected amongst all DEGs (left) and stratified by up- (middle) and down-regulated (right) DEGs. The top 10 biological processes detected in each analysis are shown on the y-axis. Biological processes are ordered based on their statistical significance in the left-most column in which they are detected. Dot color reflects the statistical significance [−log10(FDR)] of the biological process enrichment. Dot size reflects the number of detected DEGs mapped to the genes involved in a given biological process.
GO enrichment analysis was conducted to identify overrepresented biological process among DEGs from the trained versus untrained comparison and identified within each hippocampal subregion (The Gene Ontology Consortium, 2019). Among the DEGs detected in the combined hippocampal subregions, we found overrepresentation of genes involved in the modulation of neurotransmitter signaling (Figure 3F). These DEGs included Ywhag, Bsn, and Nup153, which have been previously implicated in these cellular functions (Wachi et al., 2017; Annamneedi et al., 2021; Leone et al., 2019). DEGs detected in both the CA3 and CA1 were also enriched with genes involved in the modulation of neurotransmitter signaling. The genes Snap25, Shank1, Shank 3, Bsn and Ywhag were identified in the overlap of upregulated DEGs between these regions and have been studied for their involvement in synaptic transmission (Irfan et al., 2019; Mao et al., 2015; Shi et al., 2017; Kouser et al., 2013). DEGs detected in the DG were enriched with genes involved in neurogenesis and transcriptional regulation. Among these DEGs were Kit, H2afz, and Kmt2e, which have been studied for their relationship to neurogenesis and transcriptional regulation (Katafuchi et al., 2000; Ferreira et al., 2016; Barbieri et al., 2018; Zovkic et al., 2014; Collins et al., 2019). When enrichment analyses were separated by up- and down-regulated DEGs, the differences separating the CA1 and CA3 from the DG became more striking with no significant enrichment of biological processes among the downregulated DEGs of the CA1 and CA3. These data reveal a spatial distribution of gene expression across the dorsal hippocampus of trained animals, defined by a greater degree of similarity between the CA1 and CA3 than between either of these subregions and the DG.
3.4 RT-qPCR validation of regionally detected DEGs
We performed targeted gene expression analyses using RT-qPCR on DG, CA3, and CA1 subregion samples collected from similarly behaviorally conditioned (i.e., trained and untrained) animals (Supplementary Figures 3A,B). We probed a total of 8 genes (with Gapdh as an internal control), each identified for their high statistical significance and relatively large positive fold change of expression in one of the region-specific comparisons between trained and untrained samples. In the DG, we identified significant differential expression of the gene Kit between trained and untrained animals, which replicates our results from bulk RNA sequencing comparisons of in this region (Supplementary Figure 3C). In the CA3 we identified significant differential expression of the gene Rasal1 between trained and untrained animals, replicating our results from bulk RNA sequencing (Supplementary Figure 3D). In the CA1, Homer3 and Crym were significantly differentially expressed (Supplementary Figure 3E). Differential expression of Homer3 in the CA1 was an expected validation of our bulk RNA sequencing findings, but the detection of Crym differential expression was unexpected as it was selected as a candidate DEG from the DG. In sum, RT-qPCR allowed us to validate the differential expression of select target genes with the largest expression fold changes in our bulk RNA-seq dataset. Consistent with prior reports, some of our target genes which exhibited comparatively lower fold changes of expression escaped detection because of the limitation in RT-qPCR relative to RNA-sequencing approaches (Coenye, 2021; Everaert et al., 2017; Reshetnikov et al., 2020).
3.5 Differences in the spatial distribution of hippocampal gene expression between trained and untrained animals
To further investigate the spatial distribution of DEGs associated with APA memory training across the hippocampus, we performed spatial transcriptomics on coronal sections containing the dorsal hippocampus from one trained (male) and one untrained (male) mouse. Computational analyses of integrated capture spots in the hippocampal slices from trained and untrained animals revealed distinct clusters (seen in a Uniform Manifold Approximation and Projection (UMAP) plot) which map along anatomical boundaries in a spatial transcriptomic plot (Figures 4A,B). Spatial transcriptomics data were integrated with the Allen Brain Atlas (Yao et al., 2021) to computationally annotate each spot with the cell-type most prominently detected (Figures 4C,D).
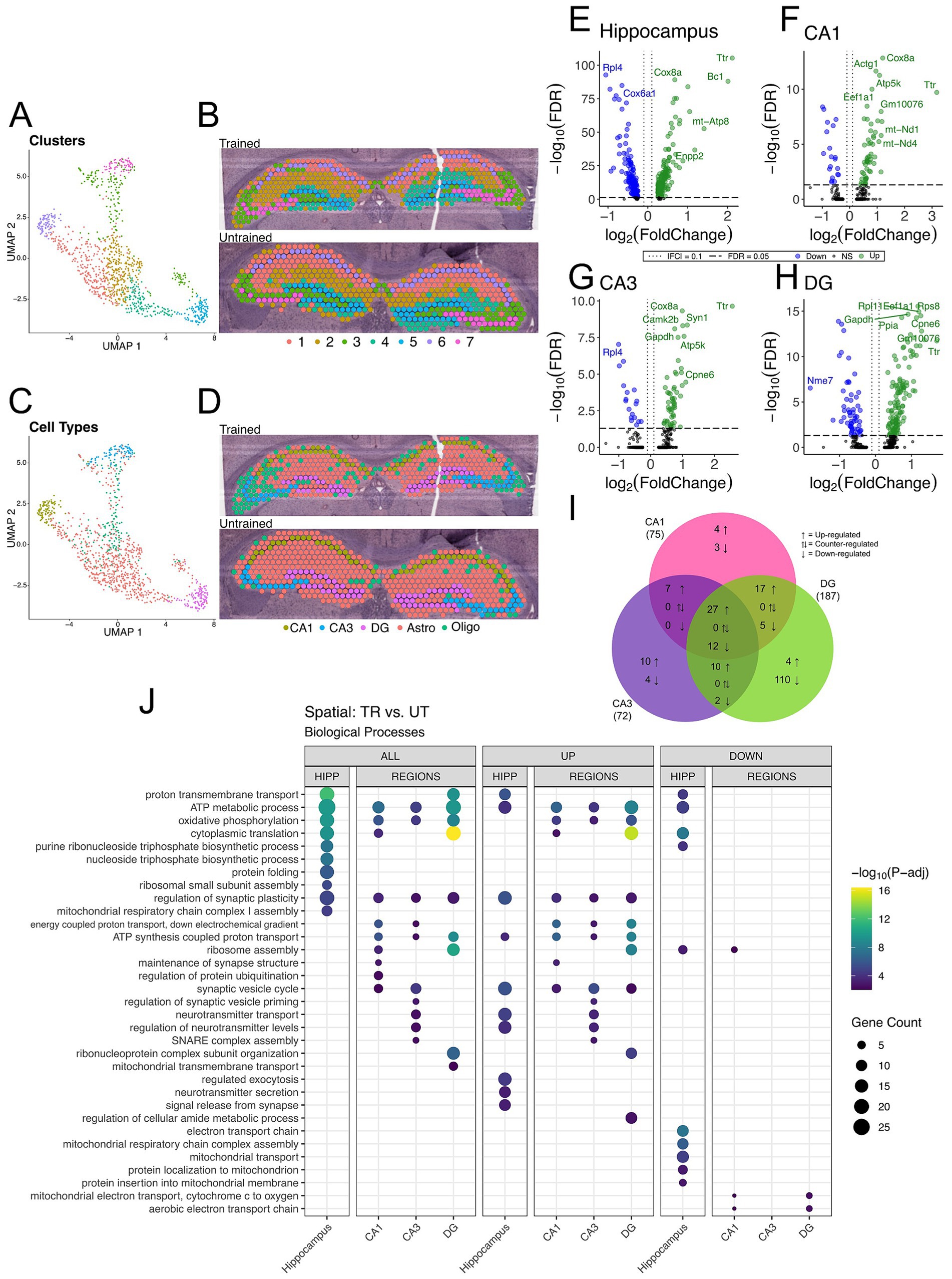
Figure 4. DEGs detected between trained and untrained animals within hippocampal subregions using spatial transcriptomics are enriched with genes involved in similar biological processes to those seen in bulk RNA-seq. (A,B) UMAP plot (A) and spatial transcriptomic maps of hippocampal spots (B) from trained and untrained mouse samples (n = 1 per group). Discrete color scale displays unsupervised graph-based clustering of hippocampal spots. Clusters are labeled 1 through 7 from greatest (1) to least (7) number of spots belonging to each unbiased cluster. Unbiased clustering groups spots along anatomical boundaries reflecting natural transcriptomic differences between morphologically distinct regions in the hippocampus. (C,D) UMAP plot (C) and cell-type annotated spatial transcriptomic maps of hippocampal spots (D) in samples from the trained and untrained mouse. Discrete color scale reflects the predominant cell type surveyed by each capture spot which was determined by cell-type annotation of hippocampal capture spots through automated integration of our data with the single cell Allen Brain Atlas for mice. (E–H) Volcano plot of DEGs between all hippocampal (E), CA1 (F), CA3 (G), and DG (H) cell-layer spots from trained and untrained mice (n = 1 per group). In the analysis of all hippocampal combined, 205 genes were upregulated and 147 were downregulated. 55 genes were upregulated and 20 were downregulated in the CA1 cell layer, 54 genes were upregulated and 18 were downregulated in the CA3 cell layer, and 129 genes were upregulated and 58 were downregulated in the DG cell layer. (I) Overlap of regionally detected DEGs stratified by direction of fold change. Overlaps were tested with Fisher’s exact test. See Supplementary Figure 2 for further details. (J) Regional enrichment of biological processes detected amongst all DEGs (left) and stratified by up- (middle) and down-regulated (right) DEGs. The top 10 biological processes detected in each analysis are shown on the y-axis. Biological processes are ordered based on their statistical significance in the left-most column in which they are detected. Dot color reflects the statistical significance [−log10(FDR)] of the biological process enrichment. Dot size reflects the number of detected DEGs mapped to the genes involved in a given biological process. See Supplementary Figure 2 for enrichment of Cellular Component and Molecular Function GO terms.
Differential gene expression analysis between trained and untrained mice identified a total of 352 DEGs in all hippocampal spots, with further stratification by subregional cell layers detecting 75 DEGs in the CA1, 72 DEGs in the CA3, and 187 DEGs in the DG (Figures 4E–I and Supplementary Figure 2). GO enrichment analyses of the DEGs detected between all hippocampal spots identified biological processes related to energy production and protein expression (Figure 4J). DEGs detected in the analysis of the CA1 cell layer were enriched with biological processes related to energy production and synaptic function. In the CA3, DEGs were enriched with genes involved in neurotransmitter release and energy production. And in the DG, DEGs were enriched with genes involved in energy production and translational machinery. Notably, the biological process “regulation of synaptic plasticity” (GO: 0048167) was enriched among the DEGs detected between all subregional comparisons between trained and untrained animals.
These spatial transcriptomics data are concordant with our bulk RNA sequencing data. The overlap of DEGs detected in each hippocampal region between the two techniques was lower than expected (10, 10, 22, in the CA1, CA3, and DG, respectively). Notwithstanding, there is stronger concordance between the biological process enrichment detected with the two techniques. We found upregulation of genes involved in the regulation of synaptic plasticity across all three hippocampal subregions in both experiments. These findings reinforce a subregion-specific model of memory-associated transcriptional activation following memory recall.
3.6 Arc-expressing hippocampal spots exhibit changes in the expression of memory-associated genes and biological processes
Our data suggest that differences in behavioral conditioning contributed to variations in the spatial distribution of synaptic plasticity related gene expression across the hippocampal network. It is well established that a surge of Arc expression accompanies memory associated processes such as consolidation and retrieval, and differences in behavioral conditioning affect the recruitment of Arc-expressing hippocampal neurons which form the memory-associated neuronal ensemble (Gouty-Colomer et al., 2016; Gallo et al., 2018; Carrillo-Reid, 2022; Gouty-Colomer et al., 2016; Hsieh et al., 2021; Chia and Otto, 2013). As such, we studied the Arc-expressing spatial transcriptomics spots to assess changes in biological processes within the memory associated neuronal ensemble following APA memory recall. Spatial transcriptomic spots with detectable expression of Arc mRNA (>0 copies) were determined to be Arc + in this experiment. Given the resolution and capture efficiency of spatial transcriptomics (Asp et al., 2020), these spots represent regions of space with high expression of Arc mRNA across one or more cells/cellular compartments. Hence, we utilized these Arc + spots as a proxy for the location of the putative memory-associated neuronal populations with induced expression of Arc following the retrieval of the active place avoidance memory.
We observed a sparse distribution of Arc + spots in our samples consistent with the characteristic expression of Arc protein in the hippocampus from studies investigating memory associated ensembles (Mayford, 2014; Chia and Otto, 2013; Gouty-Colomer et al., 2016). Out of the 542 spots in the hippocampus from the trained animal, 195 spots had detectable expression of Arc mRNA (Arc+). The untrained animal, 169 Arc + spots out of 568 hippocampal spots (Supplementary Figure 4C). In both samples, spots with the highest level of Arc expression concentrated primarily in the CA1 cell layer (Figures 5A,B).
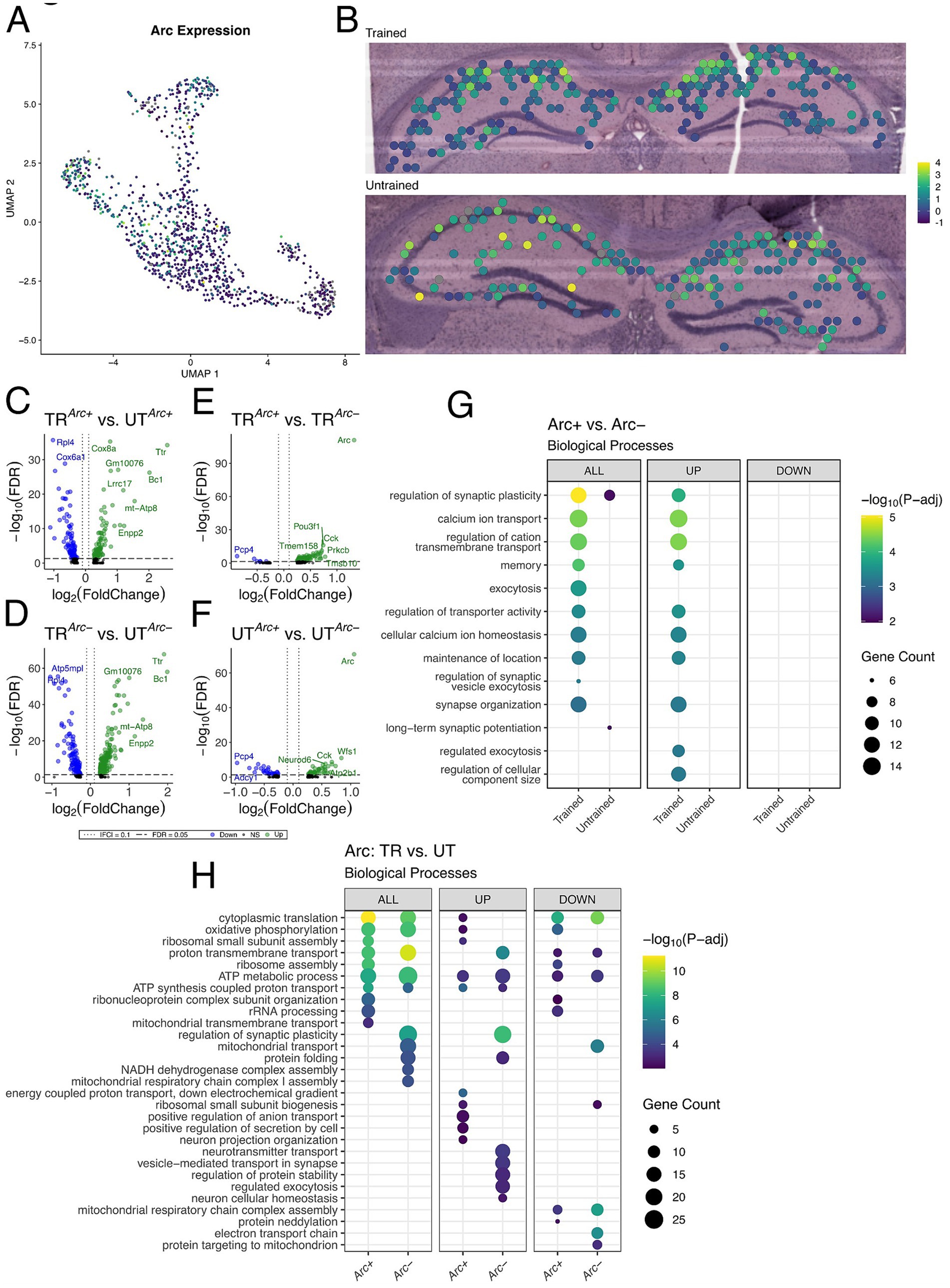
Figure 5. Arc-positive spots in the trained sample are enriched with genes involved in synaptic plasticity. (A,B) UMAP plot (A) and spatial transcriptomic maps of hippocampal spots (B) in the trained and untrained samples colored to reflect normalized expression of Arc. Arc positivity is determined by detectable expression (>0 copies) of Arc mRNA in a spatial transcriptomic spot. (C,D) Volcano plots of differential gene expression of Arc positive (C) and negative (D) spots across behavioral conditions. In the analysis of Arc + spots across behavioral conditions 85 upregulated and 89 downregulated genes were detected. In the analysis of Arc- spots across behavioral conditions 113 upregulated and 240 downregulated genes were detected. (E,F) Volcano plots of differential gene expression between Arc positive and negative spots within trained (E) and untrained (F) samples. In the analysis of Arc spots within the trained animal 95 upregulated and 6 downregulated genes were detected. In the analysis of Arc spots within the untrained animal 47 upregulated and 31 downregulated genes were detected. (G) Biological processes overrepresented in the analysis of Arc-expressing spots within trained and untrained samples amongst all DEGs (left) and stratified by up- (middle) and down-regulated (right) DEGs. The top 10 biological processes detected in each analysis are shown on the y-axis. Biological processes are ordered based on their statistical significance in the left-most column in which they are detected. Dot color reflects the statistical significance [−log10(FDR)] of the biological process enrichment. Dot size reflects the number of detected DEGs mapped to the genes involved in a given biological process. (H) Biological processes overrepresented in the analysis of Arc-expressing spots across trained and untrained samples amongst all DEGs (left) and stratified by up- (middle) and down-regulated (right) DEGs. The top 10 biological processes detected in each analysis are shown on the y-axis. Biological processes are ordered based on their statistical significance in the left-most column in which they are detected.
To assess synaptic plasticity-related transcriptional changes in these Arc + spots, we conducted multiple differential expression comparisons: (1) across sets of Arc + or Arc− spots between the trained and untrained animal (Figures 5C,D), and (2) within the trained or untrained animal between sets of Arc + and Arc− (Figures 5E,F). The comparison of Arc + spots across trained and untrained animals detected 174 significant DEGs, and comparison of Arc− spots across trained and untrained animals detected 353 significant DEGs. GO term enrichment analysis of DEGs detected across Arc + spots in the trained and untrained animal revealed enrichment with biological processes related to ribosomal function and energy production (Figure 5H). A finding which is consistent with the regionalized differences observed between the trained and untrained animals through spatial transcriptomics (Figure 4J). Rpl4 and Rpl5 were differentially expressed in the analysis across Arc + spots in the trained and untrained, and are known for their roles in ribosomal biogenesis (Robledo et al., 2008). Enrichment analyses of DEGs detected in Arc− spots across training conditions revealed a similar set biological processes related to energy production as those detected in the analysis of Arc + spots. Differences between the two comparisons are accentuated when enrichment analyses are performed separately on up- and down-regulated DEGs.
101 DEGs were detected within Arc + and Arc− spots in the trained animal, and 78 DEGs were detected within Arc + and Arc− spots in the untrained animal. Notably, substantially more biological processes related to synaptic plasticity and neuronal excitability were enriched in the DEGs Arc + spots of the trained animal as compared to Arc + spots of the untrained animal. This difference is enhanced when the enrichment analyses are separated by up- and down-regulated DEGs, where only the upregulated DEGs within the Arc + spots of the trained animal demonstrate enrichment of any biological processes (Figure 5G). Prkcb and Dkk3, known for their role in synaptic plasticity (Weeber et al., 2000; Martin-Flores et al., 2024), were detected amongst upregulated genes in the comparison of Arc + and Arc− spots in the trained animal. In summary, enrichment analyses in Arc + spots across training conditions detected biological processes related to energy metabolism and ribosomal biogenesis, while Arc + spots within the trained sample revealed biological processes related to synaptic plasticity and transmission.
3.7 IEG-expressing hippocampal spots comprise distinct gene expression profiles
Our findings demonstrate that behavioral training induces discrete transcriptional changes in the population of spots marked by Arc expression. However, memory traces are likely comprise overlapping yet molecularly distinct neuronal populations tagged by IEGs (Sun et al., 2020; Nambu et al., 2022; Okuno, 2011; Gallo et al., 2018). We sought to examine spots marked by the expression of the IEG most and least correlated with the expression of Arc to evaluate the functional relationship between these subsets of the memory-associated ensemble. Pairwise correlations identified Egr1 and c-Jun as the IEGs most and least correlated with Arc, respectively (Supplementary Figures 4A,B, 5A–F).
Unlike Arc, which codes for a cytoskeleton regulating protein, both Egr1 and c-Jun code for transcription factors. IEGs which function as transcription factors are thought to promote the expression of activity related genes which execute the cellular level changes necessary for memory storage (Yap and Greenberg, 2018; Sun et al., 2020; Kim et al., 2018; Yelhekar et al., 2024). Genes downstream of Egr1-driven transcription are involved in vesicular release, neurotransmitter metabolism, receptor expression, and synaptic plasticity (Duclot and Kabbaj, 2017; Sekiya et al., 2019). Genes downstream of c-Jun-driven transcription in the brain are involved in neurite growth, axonal regeneration, and synaptic long term depression (Raivich and Behrens, 2006; Haeusgen et al., 2009).
We performed differential gene expression analyses on Egr1 and c-Jun expressing spots across and within behavioral conditions. As described in the previous section, spatial transcriptomic spots were determined as positive if they had detectable expression of the IEG (either Egr1 or c-Jun). In total, 380 out of 542 spots in the hippocampus of the trained animal and 256 out of 568 spots in the untrained animal were found to express detectable levels of Egr1 (Supplementary Figure 3G). In both samples, spots with the highest level of Egr1 expression were located predominantly in the CA1 cell layer, following the spatial distribution of Arc expression (Figures 6A,B). Detectable levels of c-Jun were seen in 308 out of 542 spots in the hippocampal sample from the trained animal and 175 out of 568 spots in the untrained animal (Supplementary Figure 3G). In both samples, spots with the highest level of c-Jun expression were located predominantly in the DG granule cell layer (Figures 6C,D).
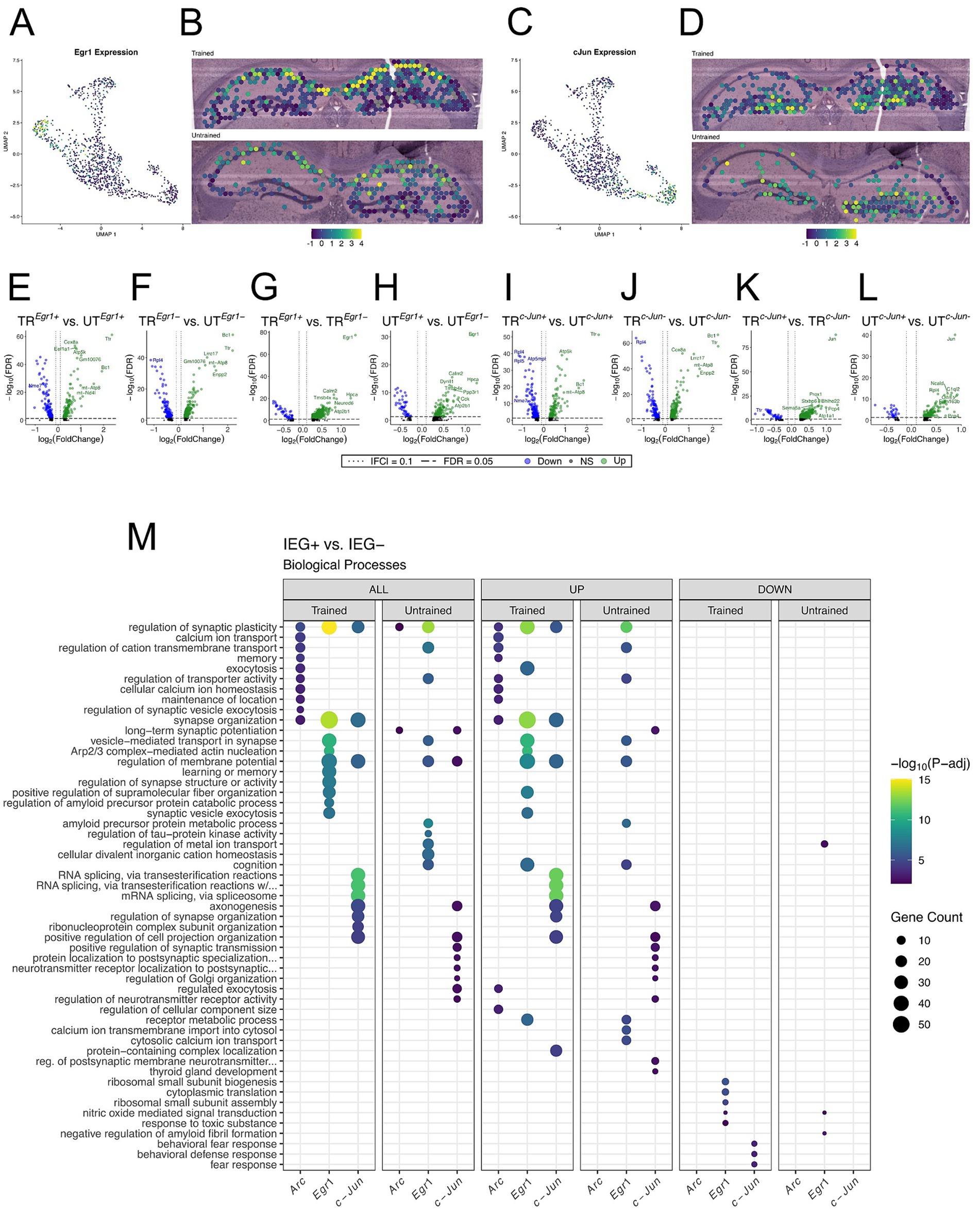
Figure 6. Spatial transcriptomics reveals distinct collections of biological processes enriched among Arc, Egr1, and c-Jun-expressing spots. (A,B) UMAP plot (A) and spatial transcriptomic maps (B) of hippocampal spots in the trained and untrained sample colored to reflect the normalized expression of Egr1. (C,D) UMAP plot (C) and spatial transcriptomic maps (D) of hippocampal spots in the trained and untrained samples colored to reflect normalized expression of c-Jun. Egr1 and c-Jun positivity is determined by detectable expression (>0 copies) of Egr1 or c-Jun mRNA in a spatial transcriptomic spot. (E–H) Volcano plots of differential gene expression of Egr1 positive (E) and negative (F) spots across behavioral conditions and within trained (G) and untrained (H) samples. In the analysis of Egr1+ spots across behavioral conditions 135 upregulated and 160 downregulated genes were detected. In the analysis of Egr1- spots across behavioral conditions 216 upregulated and 111 downregulated genes were detected. In the analysis of Egr1 spots within the trained animal 509 upregulated and 46 downregulated genes were detected. In the analysis of Egr1 spots within the untrained animal 150 upregulated and 46 downregulated genes were detected. (I–L) Volcano plots of differential gene expression of c-Jun positive (I) and negative (J) spots across behavioral conditions and within trained (K) and untrained (L) samples. In the analysis of c-Jun + spots across behavioral conditions 123 upregulated and 175 downregulated genes were detected. In the analysis of c-Jun- spots across behavioral conditions 193 upregulated and 121 downregulated genes were detected. In the analysis of c-Jun spots within the trained animal 440 upregulated and 40 downregulated genes were detected. In the analysis of c-Jun spots within the untrained animal 138 upregulated and 28 downregulated genes were detected. (M) Biological processes overrepresented between the IEG+ and IEG- spots within each sample. Analysis of GO term overrepresentation is stratified by all DEGs (left), up- (middle), and down-regulated (right) DEGs. The top 10 biological processes detected in each analysis are shown on the y-axis. Biological processes are ordered based on their statistical significance in the left-most column in which they are detected. Dot color reflects statistical significance [−log10(FDR)] of biological process enrichment. Dot size reflects number of detected DEGs mapped to the genes involved in a given biological process.
In the analysis of Egr1+ or Egr1- spots across training conditions, 295 DEGs were detected in the Egr1+ spots, and 327 DEGs in the Egr- spots (Figures 6E,F). In the analysis of c-Jun + or c-Jun- spots across training conditions, 298 DEGs were detected in the c-Jun + spots, and 314 DEGs in the c-Jun- spots (Figures 6I,J). GO enrichment analyses of all three IEG expressing spot populations across trained and untrained conditions revealed similar sets of enriched biological processes (Supplementary Figure 3D), namely those involved in ribosomal function and energy production. A finding which is consistent with the regionalized differences observed between the trained and untrained animals through spatial transcriptomics (Figure 4J).
In the analysis between Egr1+ and Egr- spots, 555 DEGs were identified within the trained animal, and 196 DEGs within the untrained animal (Figures 6G,H). In the analysis between c-Jun + and c-Jun- spots, 480 DEGs were identified within the trained animal, and 166 DEGs within the untrained animal (Figures 6K,L).
Due to the high degree of correlation between the expression of Arc and Egr1, and the low correlation between the expression of Arc and c-Jun, we expected that Arc and Egr1-expressing spots would appear more functionally similar to one another than they would to c-Jun-expressing spots, within the same sample. Counterintuitively, comparisons of IEG+ and IEG- spots within each sample revealed distinct collections of biological processes overrepresented in the Arc, Egr1, and c-Jun expressing populations. Additionally, in contrast to the within sample analyses of Arc spots (Figure 5G), within sample analyses of Egr1 and c-Jun spots were enriched with numerous biological processes in both trained and untrained mice. This effect is enhanced when enrichment analyses are stratified by up- and down-regulation. Biological processes enriched among DEGs in the Egr1 spots within the trained and untrained mice were both involved in synaptic organization and plasticity (Figure 6M). Biological processes involved in RNA splicing, synaptic plasticity, and axonal growth were overrepresented in c-Jun spots in both trained and untrained mice. Together, these data suggest that IEG-expression following the memory retention test marks subsets of neurons which are enriched with distinct sets of biological processes.
4 Discussion
In this study, we used a combination of bulk RNA sequencing and spatial transcriptomics to identify changes in gene expression between APA-trained and untrained conditions, across each major subregion of the dorsal hippocampus and within IEG-expressing spots following memory recall elicited during retention test. A recent study utilizing spatial transcriptomics found distinct transcriptomic signatures in the hippocampal subregions following training in a spatial object recognition task (Vanrobaeys et al., 2023). This finding illustrates the power of using novel transcriptomic analyses to explore the spatial distribution of gene expression profiles when investigating memory-associated neuronal networks.
4.1 Regionalization of molecular mechanisms following memory recall
Different brain regions are recruited depending on the type of memory training an animal is exposed to Rolls (2000) and Tonegawa et al. (2018). We found evidence supporting the regionalization of gene expression in trained compared to untrained animals resulting in overrepresentation of GO terms across the dorsal hippocampus following spatial memory recall. A finding consistent with prior reports demonstrating enrichment of biological processes related to transcription and synaptic differentiation in the DG following APA recall (Harris et al., 2020). In our study, comparative bulk RNA sequencing analyses between trained and untrained animals revealed abundant overlap of detected DEGs and enriched biological processes between the CA1 and CA3 subregions. However, the transcriptional landscape observed in the DG exhibited divergence from both CA subregions, suggesting recruitment of specialized molecular pathways in the trained animals in a subfield specific manner following memory recall.
Cells in the CA1 and CA3 subregions share similar morphologies and ontology as compared to the dentate gyrus (Stanfield and Cowan, 1979; Khalaf-Nazzal and Francis, 2013; Cembrowski and Spruston, 2019), which could account for our regionalized findings in the bulk RNA sequencing analysis. During memory recall, the functional coupling of the CA3 and CA1 subregions (Montgomery and Buzsaki, 2007; Carr et al., 2012) is also reflected by the gene expression changes observed in this analysis. Our findings support the role of neurogenesis and protein synthesis in the DG (Alam et al., 2018; Kitamura et al., 2009; Kitamura and Inokuchi, 2014; Deng et al., 2010), as well as the memory-associated increases in efficacy in CA3-CA1 synaptic connections (Aksoy-Aksel and Manahan-Vaughan, 2013; Pavlowsky and Alarcon, 2012) associated with the APA training experience. Furthermore, our data also implicate enhanced energetic requirements in the DG during periods of high cognitive demand that enable accurate spatial navigation and memory recall (Mendez-Lopez et al., 2010; Christie and Schrater, 2015), evidenced by the enrichment of ATP metabolic pathways with the trained condition.
4.2 Technical considerations of using spatial transcriptomics
We employed an integrative approach combining bulk RNA sequencing and spatial transcriptomics to determine the transcriptomic profile elicited in the hippocampus following the recall of a consolidated spatial memory. Both methodologies provided unique utility and insight into the transcriptomic changes in the brain following memory and have proven to be highly compatible (Li and Wang, 2021; Vanrobaeys et al., 2023). Our bulk RNA sequencing uncovered robust hippocampal subregion-specific distinctions in biological process enrichment between trained and untrained conditions. Meanwhile, spatial transcriptomic analyses revealed anatomical stratification of DEGs but convergence on overlapping sets of enriched biological processes across the CA1, CA3, and DG cell layers. The apparent differences between the results obtained from these two results were unexpected. Between the two techniques, few of the regionalized DEGs were overlapping yet, we found that DEGs detected in each region were involved in the regulation of synaptic plasticity. This finding indicates that both techniques are detecting similar biologically significant changes supporting APA memory recall.
The distinct results noted by the bulk and spatial transcriptomic experiments potentially arise from key chemical and technical variances between the two methodologies. First, the 55 μm capture spots of the 10x Visium spatial transcriptomics platform permits a more granular analysis of gene expression differences between specifically annotated populations of cells, as opposed to the entirety of a bulk section (Stahl et al., 2016). Second, relative to bulk sequencing, 10x’s Visium spatial transcriptomics requires more tissue processing before RNA extraction and has lower RNA detection sensitivity (Asp et al., 2020), which might skew the genes detected between the two studies. Nevertheless, we surmise that our dual omics approach leverages the combined strength and resolution of these emerging technologies to explore the gene expression changes following spatial memory recall simultaneously within individual cell populations and across a spatially distributed neuronal network.
4.3 Arc-expressing spots are enriched with genes involved in synaptic plasticity
Using spatial transcriptomics, we examined the spatial distribution of IEG expression in the dorsal hippocampus to infer the location of neurons involved in memory formation. We hypothesized that spatially restricted subsets of IEG-expressing memory-associated neurons would be detected amongst these spots and enriched with genes related to synaptic plasticity. To test this, mice were sacrificed following retention test during the temporal window comprising the peak expression of IEGs as well as the initial upregulation phase of late response genes (Yap and Greenberg, 2018; Sheng and Greenberg, 1990). This approach enabled concurrent transcriptional profiling of both the rapid and delayed genomic responses linked to memory formation. The spatial patterning of IEG induction and overlap with plasticity-related genes could reveal the locations of neurons recruited to the memory trace with the dorsal hippocampus.
Comparisons of Arc + spots across the trained and untrained animal revealed enrichment of biological processes related to energy metabolism and ribosomal function. Critically, comparisons of Arc + versus Arc− spots within the trained animal were substantially different from those in the untrained animal. Specifically, Arc + spots in the trained animal displayed upregulation of biological processes related to synaptic plasticity, several of which were also enriched in the bulk analyses. These data imply that the significant functional modulation occurring selectively in the Arc-expressing neuronal population following learning may largely drive the transcriptional differences observed between training conditions in the analysis of bulk hippocampal subregions.
We found that the expression of Arc mRNA was only detectable in a subset of spatial transcriptomic spots in the hippocampus. This spatial distribution of Arc expression differs from reference atlases measuring the expression of Arc in the hippocampus through in situ hybridization (Kasukawa et al., 2011; Lein et al., 2007). This discrepancy can be accounted for by the lower RNA detection sensitivity of spatial transcriptomics, which would bias detectable Arc mRNA to the regions of space with the highest expression (Asp et al., 2020). As discussed previously, Arc expression is induced following robust memory-related neuronal activity (Ryan et al., 2015; Guan et al., 2016; Chen et al., 2019; Guzowski et al., 1999; Ramirez-Amaya et al., 2005; Lee et al., 2022). In our data we find the regions of space with the highest expression of Arc are mainly along the principal cell layer of the CA1 subregion. This finding indicates that this hippocampal subregion has the highest density of strongly activated Arc + neurons belonging to the memory associated neuronal ensemble.
10x Visium spatial transcriptomics permits a resolution of 55 μm, given the size of an individual capture spot. The density of cell bodies varies across regions of the hippocampus meaning that a spot of this size covers approximately 10–50 cell bodies and countless packed nerve fibers. Neuronal activity and IEG expression also vary by region and layer. In the densely packed granule cell layer of the DG, a small fraction of cells are active and strongly expressing IEGs (including Arc) after learning (Ramirez-Amaya et al., 2006). In contrast, the large pyramidal cells of the CA1 and CA3 principal cell layers are recruited in greater relative proportions following memory and have higher basal level of Arc expression (Guzowski et al., 2001). Therefore, the number of neighboring Arc-expressing cells required to reach a detectable level with spatial transcriptomics correspondingly varies by region. For example, the number of small granule cells required per capture spot might be greater than the number of required pyramidal cells. As such, we cannot determine the cellular composition of the spots (e.g., spots containing many cells expressing Arc versus spots containing one or few cells highly expressing Arc) across the regions of the hippocampus. Although the approach is not single-cell resolution, and multiple steps of the protocol decrease the sensitivity of the mRNA detection, we do reason that this spatial transcriptomic approach is suitable for the detection of highly expressed mRNAs, like the IEG mRNAs elicited by the reactivation of a memory. With discretion, we speculate that the training experience tunes molecular processes in cell populations (i.e., spatial spots), which we can define as microenvironments, to encode learned information.
We put forward the notion that the organization of the sparsely recruited Arc-expressing spots that we investigate in this paper may be constituted by subsets of memory engram cells neighbored by a network of complementary cells that creates a mesh of sinks and sources in neural activity (i.e., microenvironments) with the purpose of information coding. It is not yet known if the functional changes that distinguish cells in a memory-associated neuronal ensemble from surrounding cells are the same functional changes that distinguish these cells across training conditions. Our data suggest that there are two defining features of the Arc + spots: the exclusive upregulation of synaptic plasticity mechanisms relative to Arc- spots in the trained animal, and the upregulation of energy production and ribosomal function in the trained versus the untrained animal. In support of these findings, a recent study identified synaptic plasticity as a defining feature of a neuron in a memory-associated neuronal ensemble (Jeong et al., 2021). Additionally, increases in energy production and ribosomal biogenesis have been found during periods of high cognitive demand (Magistretti and Allaman, 2015; Hernández et al., 2015), which corresponds to the trained avoidance behavior of the APA paradigm (Cimadevilla et al., 2000; Wesierska et al., 2005).
4.4 Egr1- and c-Jun-expressing spots exhibit distinct transcriptomic profiles
Extensively studied for its role in memory storage, the Arc-tagged neuronal ensemble represents only a fraction of neurons activated during a memory event (Lacagnina et al., 2019; Sun et al., 2020; Sweis et al., 2021). Distinct subgroups of the neurons activated during a memory event are marked by several Immediate Early Genes (IEGs), which are associated with different patterns of intense neuronal activity (Okuno, 2011; Sun and Lin, 2016; Sheng et al., 1993; Guzowski et al., 2001; Tonegawa et al., 2018). This IEG expressing neuronal ensembles could encode multiple memory traces for a specific behavioral experience (Kitamura et al., 2017; Tonegawa et al., 2018; Tonegawa et al., 2015; Terranova et al., 2023). Using spatial transcriptomics, we assessed the gene expression profiles of spots expressing the IEGs Egr1 and c-Jun, which were the IEGs most and least correlated with Arc expression, respectively.
Arc+, Egr1+, and c-Jun + spots displayed enrichment for largely distinct sets of biological processes within both trained and untrained animals. In contrast to the within sample comparisons of Arc spots, numerous biological processes were overrepresented within the trained and untrained animal for both Egr1 and c-Jun spots. Specifically, Egr1 spots displayed enrichment of biological processes related to synaptic organization and synaptic plasticity, whereas c-Jun spots displayed enrichment of biological processes related to RNA splicing, synaptic plasticity, and axonal projection. Additionally, the spatial distribution of Arc and Egr1 shows high levels of expression in the CA1 cell layer of trained and untrained samples, while the expression of c-Jun is high in the DG. In one study, Egr1 protein was shown to have higher expression in the CA1 following 1 day old spatial memory retrieval, relative to later time points (Barry et al., 2016). In the same study, Arc protein was difficult to detect in the CA1 due to low staining contrast in trained and control animals. While our data replicated the finding for Egr1 mRNA, they also point toward the utility of spatial transcriptomic studies to investigate the memory trace as it evolves over time.
Differing IEG induction likely relates to unique patterns of neuronal activation (Lyons and West, 2011; Madabhushi and Kim, 2018; Tyssowski et al., 2018; Flavell and Greenberg, 2008) and downstream circuit engagement (Roy et al., 2017), highlighting the functional and spatial diversity amongst recruited cells within memory associated neuronal ensembles. Both Egr1 and c-Jun code for transcription factors whose downstream genes have been linked with synaptic plasticity and memory processes (Yelhekar et al., 2024). Genes downstream of Egr1-driven transcription are involved in vesicular release, neurotransmitter metabolism, receptor expression, and synaptic plasticity (Duclot and Kabbaj, 2017; Sekiya et al., 2019). Genes downstream of c-Jun-driven transcription in the brain are involved in neurite growth, axonal regeneration, and synaptic long term depression (Raivich and Behrens, 2006; Haeusgen et al., 2009). At the behavioral level, Egr1 and c-Jun expression has been reported in response to fear memory and acute stress, respectively, while Arc expression has been linked to the encoding of contextual information (including location), in spatial memory tasks (Cheval et al., 2012; Maddox et al., 2011; Sherrin et al., 2010; Kubik et al., 2007; Gouty-Colomer et al., 2016; Cleland et al., 2017; Ramirez-Amaya et al., 2005).
It is well described that different patterns of neuronal activity can differentially trigger the expression of distinct IEGs (Okuno, 2011, Sun and Lin, 2016, Sheng et al., 1993, Guzowski et al., 2001, Tonegawa et al., 2018). And that different patterns of neuronal activity impinging on the same or distinct subsets of a neuronal population are also seen during the acquisition and retrieval of a memory experience (Lyons and West, 2011; He et al., 2019; Kim et al., 2018). While the direct link between types neuronal activity and IEG expression has yet to be established, it is plausible to model their connection as a continuous feature space that could describe cellular mechanisms across neuronal populations encoding of the various components of a memory experience (Kitamura et al., 2017; Tonegawa et al., 2018; Tonegawa et al., 2015; Terranova et al., 2023). In our study, the differences observed amongst the Egr1, c-Jun, and Arc expressing spots could be related to emotional, temporal, and spatial components inherent to both memory experiences of trained and untrained animals in the APA apparatus (Sun et al., 2020).
Presently, we cannot make specific connections between particular IEG-driven mechanisms and specific behavioral components of a memory experience. This remains a core gap in our knowledge of how the brain stores memory. Addressing this gap in knowledge would necessitate an approach linking molecular mechanisms and neuronal networks to simultaneously identify biological pathways associated with behavioral features (e.g., aversive or rewarding, early-term or long-term), the subset of neurons enhancing/repressing those pathways and, importantly, the connectivity of these neurons (inputs and outputs) within brain circuits.
4.5 Investigating memory across multiple scales in the brain
In summary, we performed an integrated investigation of hippocampal transcriptional dynamics following spatial memory recall leveraging the high sensitivity bulk RNA sequencing to complement the high spatial resolution of spatial transcriptomics. Regionally restricted expression patterns were observed with the CA1 and CA3 subregion exhibiting enrichment for synaptic plasticity and transmission pathways, while the DG was more prominently categorized by enrichment for protein synthesis and energy metabolism pathways. We identified a specialized signature of Arc expressing spots in trained mice categorized by upregulation of genes involved synaptic plasticity and transmission. Additionally, functionally distinct IEG expressing populations were revealed with Arc, Egr1, and c-Jun expressing spots exhibiting differential pathway enrichment and anatomical distribution.
The hippocampal transcriptional landscape captured in this study of APA memory recall represents a single transitionary state over the life of a memory. Networks in the hippocampus undergo complex spatio-temporal tuning in flow of information depending on memory phase, valence, and cognitive load (Terranova et al., 2022; Roy et al., 2017; Marks et al., 2022). As a memory evolves through systems consolidation, the neural ensembles, cellular properties, and molecular profiles supporting the memory trace are thought to transform correspondingly (Tonegawa et al., 2018; Alberini and Kandel, 2014). However, capturing this gradual reshaping of memory representations across hippocampal subregions has remained challenging. Emerging spatial molecular profiling techniques offer unmatched resolution for mapping distributed neuronal populations while preserving native tissue context. Looking forward, studies exploring the molecular memory trace would benefit from employing multiple transcripomic technologies on the same neuronal system to gather vital information across spatial scales, as we have done here. By applying transcriptomic methods to concurrently inspect spatial loci and neuronal populations, the topological features of the molecular signatures supporting memory could be elucidated.
Data availability statement
The data for this project can be found using accession numbers GSE279272 and GSE279273. GSE....72 has the microdissection experiments data. GSE...73 has the data for the spatial transcriptomic experiments. They can be found published and released at the following links: https://www.ncbi.nlm.nih.gov/geo/query/acc.cgi?acc=GSE279273, https://www.ncbi.nlm.nih.gov/geo/query/acc.cgi?acc=GSE279272.
Ethics statement
The animal study was approved by the State University of New York Downstate Health Sciences University Institutional Animal Care and Use Committee (IACUC) #15-10461. The study was conducted in accordance with the local legislation and institutional requirements.
Author contributions
IV: Data curation, Formal analysis, Investigation, Methodology, Validation, Visualization, Writing – original draft, Writing – review & editing, Conceptualization. SP: Data curation, Methodology, Writing – original draft, Writing – review & editing, Investigation. VT: Formal analysis, Software, Visualization, Writing – original draft, Writing – review & editing, Methodology. AH: Supervision, Writing – original draft, Writing – review & editing. OE: Conceptualization, Funding acquisition, Methodology, Supervision, Validation, Writing – original draft, Writing – review & editing. JA: Conceptualization, Funding acquisition, Project administration, Resources, Supervision, Writing – original draft, Writing – review & editing.
Funding
The author(s) declare financial support was received for the research, authorship, and/or publication of this article. This work was supported by Senior Vice President of Research Seed Grant (State University of New York, Downstate Health Sciences University) and partial support from NINDS grant R21NS091830.
Conflict of interest
The authors declare that the research was conducted in the absence of any commercial or financial relationships that could be construed as a potential conflict of interest.
Publisher’s note
All claims expressed in this article are solely those of the authors and do not necessarily represent those of their affiliated organizations, or those of the publisher, the editors and the reviewers. Any product that may be evaluated in this article, or claim that may be made by its manufacturer, is not guaranteed or endorsed by the publisher.
Supplementary material
The Supplementary material for this article can be found online at: https://www.frontiersin.org/articles/10.3389/fnmol.2024.1386239/full#supplementary-material
References
10X Genomics (2023). Visium spatial protocols – tissue preparation guide. Available at: https://www.10xgenomics.com/support/spatial-gene-expression-fresh-frozen/documentation/steps/tissue-prep/visium-spatial-protocols-tissue-preparation-guide
Aksoy-Aksel, A., and Manahan-Vaughan, D. (2013). The temporoammonic input to the hippocampal Ca1 region displays distinctly different synaptic plasticity compared to the Schaffer collateral input in vivo: significance for synaptic information processing. Front. Synaptic Neurosci. 5:5. doi: 10.3389/fnsyn.2013.00005
Alam, M. J., Kitamura, T., Saitoh, Y., Ohkawa, N., Kondo, T., and Inokuchi, K. (2018). Adult neurogenesis conserves hippocampal memory capacity. J. Neurosci. 38, 6854–6863. doi: 10.1523/JNEUROSCI.2976-17.2018
Alberini, C. M., and Kandel, E. R. (2014). The regulation of transcription in memory consolidation. Cold spring Harb. Perspect. Biol. 7:a021741. doi: 10.1101/cshperspect.a021741
Amaral, D. G., and Witter, M. P. (1989). The three-dimensional organization of the hippocampal formation: a review of anatomical data. Neuroscience 31, 571–591. doi: 10.1016/0306-4522(89)90424-7
Annamneedi, A., Del Angel, M., Gundelfinger, E. D., Stork, O., and Caliskan, G. (2021). The presynaptic scaffold protein bassoon in forebrain excitatory neurons mediates hippocampal circuit maturation: potential involvement of TrkB signalling. Int. J. Mol. Sci. 22:7944. doi: 10.3390/ijms22157944
Asok, A., Leroy, F., Rayman, J. B., and Kandel, E. R. (2019). Molecular mechanisms of the memory trace. Trends Neurosci. 42, 14–22. doi: 10.1016/j.tins.2018.10.005
Asp, M., Bergenstrahle, J., and Lundeberg, J. (2020). Spatially resolved transcriptomes-next generation tools for tissue exploration. BioEssays 42:e1900221. doi: 10.1002/bies.201900221
Barbieri, R., Contestabile, A., Ciardo, M. G., Forte, N., Marte, A., Baldelli, P., et al. (2018). Synapsin I and Synapsin ii regulate neurogenesis in the dentate gyrus of adult mice. Oncotarget 9, 18760–18774. doi: 10.18632/oncotarget.24655
Barry, D. N., Coogan, A. N., and Commins, S. (2016). The time course of systems consolidation of spatial memory from recent to remote retention: a comparison of the immediate early genes Zif268, c-Fos and arc. Neurobiol. Learn. Mem. 128, 46–55. doi: 10.1016/j.nlm.2015.12.010
Basu, J., and Siegelbaum, S. A. (2015). The Corticohippocampal circuit, synaptic plasticity, and memory. Cold Spring Harb. Perspect. Biol. 7:a021733. doi: 10.1101/cshperspect.a021733
Butler, A., Hoffman, P., Smibert, P., Papalexi, E., and Satija, R. (2018). Integrating single-cell transcriptomic data across different conditions, technologies, and species. Nat. Biotechnol. 36, 411–420. doi: 10.1038/nbt.4096
Cai, H., Chen, H., Yi, T., Daimon, C. M., Boyle, J. P., Peers, C., et al. (2013). VennPlex–A novel Venn diagram program for comparing and visualizing datasets with differentially regulated Datapoints. PLoS One 8:e53388. doi: 10.1371/journal.pone.0053388
Carr, M. F., Karlsson, M. P., and Frank, L. M. (2012). Transient slow gamma synchrony underlies hippocampal memory replay. Neuron 75, 700–713. doi: 10.1016/j.neuron.2012.06.014
Carrillo-Reid, L. (2022). Neuronal ensembles in memory processes. Semin. Cell Dev. Biol. 125, 136–143. doi: 10.1016/j.semcdb.2021.04.004
Cembrowski, M. S., and Spruston, N. (2019). Heterogeneity within classical cell types is the rule: lessons from hippocampal pyramidal neurons. Nat. Rev. Neurosci. 20, 193–204. doi: 10.1038/s41583-019-0125-5
Chen, B. K., Murawski, N. J., Cincotta, C., Mckissick, O., Finkelstein, A., Hamidi, A. B., et al. (2019). Artificially enhancing and suppressing Hippocampus-mediated memories. Curr. Biol. 29, 1885–1894.e4. doi: 10.1016/j.cub.2019.04.065
Cheval, H., Chagneau, C., Levasseur, G., Veyrac, A., Faucon-Biguet, N., Laroche, S., et al. (2012). Distinctive features of Egr transcription factor regulation and DNA binding activity in CA1 of the hippocampus in synaptic plasticity and consolidation and reconsolidation of fear memory. Hippocampus. 22, 631–42.
Chevaleyre, V., and Siegelbaum, S. A. (2010). Strong Ca2 pyramidal neuron synapses define a powerful disynaptic cortico-hippocampal loop. Neuron 66, 560–572. doi: 10.1016/j.neuron.2010.04.013
Chia, C., and Otto, T. (2013). Hippocampal arc (Arg3.1) expression is induced by memory recall and required for memory reconsolidation in trace fear conditioning. Neurobiol. Learn. Mem. 106, 48–55. doi: 10.1016/j.nlm.2013.06.021
Cho, J. H., Huang, B. S., and Gray, J. M. (2016). Rna sequencing from neural ensembles activated during fear conditioning in the mouse temporal association cortex. Sci. Rep. 6:31753. doi: 10.1038/srep31753
Christie, S. T., and Schrater, P. (2015). Cognitive cost as dynamic allocation of energetic resources. Front. Neurosci. 9:289. doi: 10.3389/fnins.2015.00289
Chung, A., Jou, C., Grau-Perales, A., Levy, E. R. J., Dvorak, D., Hussain, N., et al. (2021). Cognitive control persistently enhances hippocampal information processing. Nature 600, 484–488. doi: 10.1038/s41586-021-04070-5
Cimadevilla, J. M., Fenton, A. A., and Bures, J. (2001). New spatial cognition tests for mice: passive place avoidance on stable and active place avoidance on rotating arenas. Brain Res. Bull. 54, 559–563. doi: 10.1016/S0361-9230(01)00448-8
Cimadevilla, J. M., Kaminsky, Y., Fenton, A., and Bures, J. (2000). Passive and active place avoidance as a tool of spatial memory research in rats. J. Neurosci. Methods 102, 155–164. doi: 10.1016/S0165-0270(00)00288-0
Cleland, J. P., Willis, E. F., Bartlett, P. F., and Vukovic, J. (2017). Somatic arc protein expression in hippocampal granule cells is increased in response to environmental change but independent of task-specific learning. Sci. Rep. 7:12477. doi: 10.1038/s41598-017-12583-1
Collins, B. E., Greer, C. B., Coleman, B. C., and Sweatt, J. D. (2019). Histone H3 lysine K4 methylation and its role in learning and memory. Epigenetics Chromatin 12:7. doi: 10.1186/s13072-018-0251-8
Coenye, T. (2021). Do results obtained with RNA-sequencing require independent verification?. Biofilm. 3, 100043.
Deng, W., Aimone, J. B., and Gage, F. H. (2010). New neurons and new memories: how does adult hippocampal neurogenesis affect learning and memory? Nat. Rev. Neurosci. 11, 339–350. doi: 10.1038/nrn2822
Denny, C. A., Kheirbek, M. A., Alba, E. L., Tanaka, K. F., Brachman, R. A., Laughman, K. B., et al. (2014). Hippocampal memory traces are differentially modulated by experience, time, and adult neurogenesis. Neuron 83, 189–201. doi: 10.1016/j.neuron.2014.05.018
Duclot, F., and Kabbaj, M. (2017). The role of early growth response 1 (Egr1) in brain plasticity and neuropsychiatric disorders. Front. Behav. Neurosci. 11:35. doi: 10.3389/fnbeh.2017.00035
Erwin, S. R., Sun, W., Copeland, M., Lindo, S., Spruston, N., and Cembrowski, M. S. (2020). A sparse, spatially biased subtype of mature granule cell dominates recruitment in hippocampal-associated behaviors. Cell Rep. 31:107551. doi: 10.1016/j.celrep.2020.107551
Everaert, C., Luypaert, M., Maag, J. L. V., Cheng, Q. X., Dinger, M. E., Hellemans, J., et al. (2017). Benchmarking of RNA-sequencing analysis workflows using whole-transcriptome RT-qPCR expression data. Sci Rep. 7:1559.
Ferreira, E., Shaw, D. M., and Oddo, S. (2016). Identification of learning-induced changes in protein networks in the hippocampi of a mouse model of Alzheimer’s disease. Transl. Psychiatry 6:e849. doi: 10.1038/tp.2016.114
Flavell, S. W., and Greenberg, M. E. (2008). Signaling mechanisms linking neuronal activity to gene expression and plasticity of the nervous system. Annu. Rev. Neurosci. 31, 563–590. doi: 10.1146/annurev.neuro.31.060407.125631
Gallo, F. T., Katche, C., Morici, J. F., Medina, J. H., and Weisstaub, N. V. (2018). Immediate early genes, memory and psychiatric disorders: focus on c-Fos, Egr1 and arc. Front. Behav. Neurosci. 12:79. doi: 10.3389/fnbeh.2018.00079
Genomics, X. (2019). Visium spatial gene expression reagent kits - tissue optimization. Available at: https://www.10xgenomics.com/support/spatial-gene-expression-fresh-frozen/documentation/steps/tissue-optimization/visium-spatial-tissue-optimization-reagents-kits-user-guide
Genomics, X. (2021). Visium Spatial Gene Expression Imaging Guidelines. Available at: https://www.10xgenomics.com/support/spatial-gene-expression-fresh-frozen/documentation/steps/imaging/visium-spatial-gene-expression-imaging-guidelines
Genomics, X. (2023). Visium spatial gene expression reagent kits user guide. Available at: https://www.10xgenomics.com/support/spatial-gene-expression-fresh-frozen/documentation/steps/library-construction/visium-spatial-gene-expression-reagent-kits-user-guide
Gouty-Colomer, L. A., Hosseini, B., Marcelo, I. M., Schreiber, J., Slump, D. E., Yamaguchi, S., et al. (2016). Arc expression identifies the lateral amygdala fear memory trace. Mol. Psychiatry 21, 364–375. doi: 10.1038/mp.2015.18
Gu, Z., Eils, R., and Schlesner, M. (2016). Complex heatmaps reveal patterns and correlations in multidimensional genomic data. Bioinformatics 32, 2847–2849. doi: 10.1093/bioinformatics/btw313
Guan, J. S., Jiang, J., Xie, H., and Liu, K. Y. (2016). How does the sparse memory "engram" neurons encode the memory of a spatial-temporal event? Front. Neural Circ. 10:61. doi: 10.3389/fncir.2016.00061
Guzowski, J. F., Mcnaughton, B. L., Barnes, C. A., and Worley, P. F. (1999). Environment-specific expression of the immediate-early gene arc in hippocampal neuronal ensembles. Nat. Neurosci. 2, 1120–1124. doi: 10.1038/16046
Guzowski, J. F., Setlow, B., Wagner, E. K., and Mcgaugh, J. L. (2001). Experience-dependent gene expression in the rat hippocampus after spatial learning: a comparison of the immediate-early genes arc, c-fos, and zif268. J. Neurosci. 21, 5089–5098. doi: 10.1523/JNEUROSCI.21-14-05089.2001
Haeusgen, W., Boehm, R., Zhao, Y., Herdegen, T., and Waetzig, V. (2009). Specific activities of individual c-Jun N-terminal kinases in the brain. Neuroscience 161, 951–959. doi: 10.1016/j.neuroscience.2009.04.014
Hao, Y., Stuart, T., Kowalski, M. H., Choudhary, S., Hoffman, P., Hartman, A., et al. (2023). Dictionary learning for integrative, multimodal and scalable single-cell analysis. Nat. Biotechnol. 42, 293–304. doi: 10.1038/s41587-023-01767-y
Harris, R. M., Kao, H.-Y., Alarcón, J. M., Fenton, A. A., and Hofmann, H. A. (2020). Transcriptome analysis of hippocampal subfields identifies gene expression profiles associated with long-term active place avoidance memory. bioRxiv :2020.02.05.935759. doi: 10.1101/2020.02.05.935759
He, Q., Wang, J., and Hu, H. (2019). Illuminating the activated brain: emerging activity-dependent tools to capture and control functional neural circuits. Neurosci. Bull. 35, 369–377. doi: 10.1007/s12264-018-0291-x
Hernández, A. I., Alarcon, J. M., and Allen, K. D. (2015). New ribosomes for new memories? Commun. Integr. Biol. 8:e1017163. doi: 10.1080/19420889.2015.1017163
Hsieh, C., Tsokas, P., Grau-Perales, A., Lesburgueres, E., Bukai, J., Khanna, K., et al. (2021). Persistent increases of Pkmzeta in memory-activated neurons trace Ltp maintenance during spatial long-term memory storage. Eur. J. Neurosci. 54, 6795–6814. doi: 10.1111/ejn.15137
Hunsaker, M. R., and Kesner, R. P. (2013). The operation of pattern separation and pattern completion processes associated with different attributes or domains of memory. Neurosci. Biobehav. Rev. 37, 36–58. doi: 10.1016/j.neubiorev.2012.09.014
Irfan, M., Gopaul, K. R., Miry, O., Hökfelt, T., Stanton, P. K., and Bark, C. (2019). Snap-25 isoforms differentially regulate synaptic transmission and long-term synaptic plasticity at central synapses. Sci. Rep. 9:6403. doi: 10.1038/s41598-019-42833-3
Jeong, Y., Cho, H. Y., Kim, M., Oh, J. P., Kang, M. S., Yoo, M., et al. (2021). Synaptic plasticity-dependent competition rule influences memory formation. Nat. Commun. 12:3915. doi: 10.1038/s41467-021-24269-4
Josselyn, S. A., and Tonegawa, S. (2020). Memory engrams: recalling the past and imagining the future. Science 367:eaaw4325. doi: 10.1126/science.aaw4325
Kasukawa, T., Masumoto, K. H., Nikaido, I., Nagano, M., Uno, K. D., Tsujino, K., et al. (2011). Quantitative expression profile of distinct functional regions in the adult mouse brain. PLoS One 6:e23228. doi: 10.1371/journal.pone.0023228
Katafuchi, T., Li, A. J., Hirota, S., Kitamura, Y., and Hori, T. (2000). Impairment of spatial learning and hippocampal synaptic potentiation in c-kit mutant rats. Learn. Mem. 7, 383–392. doi: 10.1101/lm.33900
Kelemen, E., and Fenton, A. A. (2016). Coordinating different representations in the hippocampus. Neurobiol. Learn. Mem. 129, 50–59. doi: 10.1016/j.nlm.2015.12.011
Kesner, R. P., Lee, I., and Gilbert, P. (2004). A behavioral assessment of hippocampal function based on a subregional analysis. Rev. Neurosci. 15, 333–351. doi: 10.1515/revneuro.2004.15.5.333
Khalaf-Nazzal, R., and Francis, F. (2013). Hippocampal development - old and new findings. Neuroscience 248, 225–242. doi: 10.1016/j.neuroscience.2013.05.061
Kim, S., Kim, H., and Um, J. W. (2018). Synapse development organized by neuronal activity-regulated immediate-early genes. Exp. Mol. Med. 50, 1–7. doi: 10.1038/s12276-018-0061-x
Kitamura, T., and Inokuchi, K. (2014). Role of adult neurogenesis in hippocampal-cortical memory consolidation. Mol. Brain 7:13. doi: 10.1186/1756-6606-7-13
Kitamura, T., Ogawa, S. K., Roy, D. S., Okuyama, T., Morrissey, M. D., Smith, L. M., et al. (2017). Engrams and circuits crucial for systems consolidation of a memory. Science 356, 73–78. doi: 10.1126/science.aam6808
Kitamura, T., Saitoh, Y., Takashima, N., Murayama, A., Niibori, Y., Ageta, H., et al. (2009). Adult neurogenesis modulates the hippocampus-dependent period of associative fear memory. Cell 139, 814–827. doi: 10.1016/j.cell.2009.10.020
Kouser, M., Speed, H. E., Dewey, C. M., Reimers, J. M., Widman, A. J., Gupta, N., et al. (2013). Loss of predominant Shank3 isoforms results in hippocampus-dependent impairments in behavior and synaptic transmission. J. Neurosci. 33, 18448–18468. doi: 10.1523/JNEUROSCI.3017-13.2013
Kubik, S., Miyashita, T., and Guzowski, J. F. (2007). Using immediate-early genes to map hippocampal subregional functions. Learn. Mem. 14, 758–770. doi: 10.1101/lm.698107
Lacagnina, A. F., Brockway, E. T., Crovetti, C. R., Shue, F., Mccarty, M. J., Sattler, K. P., et al. (2019). Distinct hippocampal engrams control extinction and relapse of fear memory. Nat. Neurosci. 22, 753–761. doi: 10.1038/s41593-019-0361-z
Lee, B. H., Shim, J. Y., Moon, H. C., Kim, D. W., Kim, J., Yook, J. S., et al. (2022). Real-time visualization of mrna synthesis during memory formation in live mice. Proc. Natl. Acad. Sci. U. S. A. 119:e2117076119. doi: 10.1073/pnas.2117076119
Lein, E. S., Hawrylycz, M. J., Ao, N., Ayres, M., Bensinger, A., Bernard, A., et al. (2007). Genome-wide atlas of gene expression in the adult mouse brain. Nature 445, 168–176. doi: 10.1038/nature05453
Leone, L., Colussi, C., Gironi, K., Longo, V., Fusco, S., Li Puma, D. D., et al. (2019). Altered Nup153 expression impairs the function of cultured hippocampal neural stem cells isolated from a mouse model of Alzheimer’s disease. Mol. Neurobiol. 56, 5934–5949. doi: 10.1007/s12035-018-1466-1
Li, X., and Wang, C. Y. (2021). From bulk, single-cell to spatial RNA sequencing. Int. J. Oral Sci. 13:36. doi: 10.1038/s41368-021-00146-0
Lisman, J. E. (1999). Relating hippocampal circuitry to function: recall of memory sequences by reciprocal dentate-Ca3 interactions. Neuron 22, 233–242. doi: 10.1016/S0896-6273(00)81085-5
Lyons, M. R., and West, A. E. (2011). Mechanisms of specificity in neuronal activity-regulated gene transcription. Prog. Neurobiol. 94, 259–295. doi: 10.1016/j.pneurobio.2011.05.003
Madabhushi, R., and Kim, T. K. (2018). Emerging themes in neuronal activity-dependent gene expression. Mol. Cell. Neurosci. 87, 27–34. doi: 10.1016/j.mcn.2017.11.009
Maddox, S. A., Monsey, M. S., and Schafe, G. E. (2011). Early growth response gene 1 (Egr-1) is required for new and reactivated fear memories in the lateral amygdala. Learn Mem. 18, 24–38.
Magee, J. C., and Grienberger, C. (2020). Synaptic plasticity forms and functions. Annu. Rev. Neurosci. 43, 95–117. doi: 10.1146/annurev-neuro-090919-022842
Magistretti, P. J., and Allaman, I. (2015). A cellular perspective on brain energy metabolism and functional imaging. Neuron 86, 883–901. doi: 10.1016/j.neuron.2015.03.035
Malenka, R. C., and Bear, M. F. (2004). Ltp and ltd: an embarrassment of riches. Neuron 44, 5–21. doi: 10.1016/j.neuron.2004.09.012
Mao, W., Watanabe, T., Cho, S., Frost, J. L., Truong, T., Zhao, X., et al. (2015). Shank1 regulates excitatory synaptic transmission in mouse hippocampal parvalbumin-expressing inhibitory interneurons. Eur. J. Neurosci. 41, 1025–1035. doi: 10.1111/ejn.12877
Marco, A., Meharena, H. S., Dileep, V., Raju, R. M., Davila-Velderrain, J., Zhang, A. L., et al. (2020). Mapping the epigenomic and transcriptomic interplay during memory formation and recall in the hippocampal engram ensemble. Nat. Neurosci. 23, 1606–1617. doi: 10.1038/s41593-020-00717-0
Marks, W. D., Yokose, J., Kitamura, T., and Ogawa, S. K. (2022). Neuronal ensembles organize activity to generate contextual memory. Front. Behav. Neurosci. 16:805132. doi: 10.3389/fnbeh.2022.805132
Martin-Flores, N., Podpolny, M., Mcleod, F., Workman, I., Crawford, K., Ivanov, D., et al. (2024). Downregulation of Dickkopf-3, a Wnt antagonist elevated in Alzheimer’s disease, restores synapse integrity and memory in a disease mouse model. eLife. 12:RP89453. doi: 10.7554/eLife.89453
Mayford, M. (2014). The search for a hippocampal engram. Philos. Trans. R. Soc. Lond. Ser. B Biol. Sci. 369:20130161. doi: 10.1098/rstb.2013.0161
Mayford, M., Siegelbaum, S. A., and Kandel, E. R. (2012). Synapses and memory storage. Cold Spring Harb. Perspect. Biol. 4:a005751. doi: 10.1101/cshperspect.a005751
Meenakshi, P., Kumar, S., and Balaji, J. (2021). In vivo imaging of immediate early gene expression dynamics segregates neuronal ensemble of memories of dual events. Mol. Brain 14:102. doi: 10.1186/s13041-021-00798-3
Mendez-Lopez, M., Mendez, M., Begega, A., and Arias, J. L. (2010). Spatial short-term memory in rats: effects of learning trials on metabolic activity of limbic structures. Neurosci. Lett. 483, 32–35. doi: 10.1016/j.neulet.2010.07.054
Middleton, S. J., and Mchugh, T. J. (2020). Ca2: a highly connected intrahippocampal relay. Annu. Rev. Neurosci. 43, 55–72. doi: 10.1146/annurev-neuro-080719-100343
Molitor, R. J., Sherrill, K. R., Morton, N. W., Miller, A. A., and Preston, A. R. (2021). Memory reactivation during learning simultaneously promotes dentate gyrus/ca<sub>2,3</sub> pattern differentiation and ca<sub>1</sub> memory integration. J. Neurosci. 41, 726–738. doi: 10.1523/JNEUROSCI.0394-20.2020
Montgomery, S. M., and Buzsaki, G. (2007). Gamma oscillations dynamically couple hippocampal Ca3 and Ca1 regions during memory task performance. Proc. Natl. Acad. Sci. U. S. A. 104, 14495–14500. doi: 10.1073/pnas.0701826104
Nambu, M. F., Lin, Y. J., Reuschenbach, J., and Tanaka, K. Z. (2022). What does engram encode?: heterogeneous memory engrams for different aspects of experience. Curr. Opin. Neurobiol. 75:102568. doi: 10.1016/j.conb.2022.102568
Okuno, H. (2011). Regulation and function of immediate-early genes in the brain: beyond neuronal activity markers. Neurosci. Res. 69, 175–186. doi: 10.1016/j.neures.2010.12.007
Pavlowsky, A., and Alarcon, J. M. (2012). Interaction between long-term potentiation and depression in Ca1 synapses: temporal constrains, functional compartmentalization and protein synthesis. PLoS One 7:e29865. doi: 10.1371/journal.pone.0029865
Paxinos, G., and Franklin, K. B. J. (2019). Paxinos and Franklin’s the mouse brain in stereotaxic coordinates. San Diego, CA: Academic Press.
Ponchel, F., Toomes, C., Bransfield, K., Leong, F. T., Douglas, S. H., Field, S. L., et al. (2003). Real-time Pcr based on Sybr-green I fluorescence: an alternative to the TaqMan assay for a relative quantification of gene rearrangements, gene amplifications and micro gene deletions. BMC Biotechnol. 3:18. doi: 10.1186/1472-6750-3-18
Poo, M. M., Pignatelli, M., Ryan, T. J., Tonegawa, S., Bonhoeffer, T., Martin, K. C., et al. (2016). What is memory? The present state of the engram. BMC Biol. 14:40. doi: 10.1186/s12915-016-0261-6
Raivich, G., and Behrens, A. (2006). Role of the Ap-1 transcription factor c-Jun in developing, adult and injured brain. Prog. Neurobiol. 78, 347–363. doi: 10.1016/j.pneurobio.2006.03.006
Ramirez-Amaya, V., Marrone, D. F., Gage, F. H., Worley, P. F., and Barnes, C. A. (2006). Integration of new neurons into functional neural networks. J. Neurosci. 26, 12237–12241. doi: 10.1523/JNEUROSCI.2195-06.2006
Ramirez-Amaya, V., Vazdarjanova, A., Mikhael, D., Rosi, S., Worley, P. F., and Barnes, C. A. (2005). Spatial exploration-induced arc mrna and protein expression: evidence for selective, network-specific reactivation. J. Neurosci. 25, 1761–1768. doi: 10.1523/JNEUROSCI.4342-04.2005
Rao-Ruiz, P., Couey, J. J., Marcelo, I. M., Bouwkamp, C. G., Slump, D. E., Matos, M. R., et al. (2019). Engram-specific transcriptome profiling of contextual memory consolidation. Nat. Commun. 10:2232. doi: 10.1038/s41467-019-09960-x
Reshetnikov, V. V., Kisaretova, P. E., Ershov, N. I., Shulyupova, A. S., Oshchepkov, D. Y., Klimova, N. V., et al. (2020). Genes associated with cognitive performance in the Morris water maze: an Rna-seq study. Sci. Rep. 10:22078. doi: 10.1038/s41598-020-78997-6
Robledo, S., Idol, R. A., Crimmins, D. L., Ladenson, J. H., Mason, P. J., and Bessler, M. (2008). The role of human ribosomal proteins in the maturation of rrna and ribosome production. RNA 14, 1918–1929. doi: 10.1261/rna.1132008
Rolls, E. T. (2000). Memory systems in the brain. Annu. Rev. Psychol. 51, 599–630. doi: 10.1146/annurev.psych.51.1.599
Roy, D. S., Kitamura, T., Okuyama, T., Ogawa, S. K., Sun, C., Obata, Y., et al. (2017). Distinct neural circuits for the formation and retrieval of episodic memories. Cell 170, 1000–1012.e19. doi: 10.1016/j.cell.2017.07.013
Ryan, T. J., Roy, D. S., Pignatelli, M., Arons, A., and Tonegawa, S. (2015). Memory. Engram cells retain memory under retrograde amnesia. Science 348, 1007–1013. doi: 10.1126/science.aaa5542
Sanes, J. R., and Lichtman, J. W. (1999). Can molecules explain long-term potentiation? Nat. Neurosci. 2, 597–604. doi: 10.1038/10154
Sardoo, A. M., Zhang, S., Ferraro, T. N., Keck, T. M., and Chen, Y. (2022). Decoding brain memory formation by single-cell Rna sequencing. Brief. Bioinform. 23:bbac412. doi: 10.1093/bib/bbac412
Sauvage, M., Kitsukawa, T., and Atucha, E. (2019). Single-cell memory trace imaging with immediate-early genes. J. Neurosci. Methods 326:108368. doi: 10.1016/j.jneumeth.2019.108368
Sekiya, T., Kato, K., Kawaguchi, A., and Nagata, K. (2019). Involvement of Ctcf in transcription regulation of Egr1 at early G1 phase as an architecture factor. Sci. Rep. 9:329. doi: 10.1038/s41598-018-36753-x
Senzai, Y. (2019). Function of local circuits in the hippocampal dentate gyrus-Ca3 system. Neurosci. Res. 140, 43–52. doi: 10.1016/j.neures.2018.11.003
Shah, S., Lubeck, E., Zhou, W., and Cai, L. (2016). In situ transcription profiling of single cells reveals spatial organization of cells in the mouse hippocampus. Neuron 92, 342–357. doi: 10.1016/j.neuron.2016.10.001
Sheng, H. Z., Fields, R. D., and Nelson, P. G. (1993). Specific regulation of immediate early genes by patterned neuronal activity. J. Neurosci. Res. 35, 459–467
Sheng, M., and Greenberg, M. E. (1990). The regulation and function of c-fos and other immediate early genes in the nervous system. Neuron 4, 477–485. doi: 10.1016/0896-6273(90)90106-P
Sherrin, T., Blank, T., Hippel, C., Rayner, M., Davis, R. J., and Todorovic, C. (2010). Hippocampal c-Jun-N-terminal kinases serve as negative regulators of associative learning. J Neurosci. 30, 3976–61.
Shi, R., Redman, P., Ghose, D., Hwang, H., Liu, Y., Ren, X., et al. (2017). Shank proteins differentially regulate synaptic transmission. eNeuro 4:ENEURO.0163-15.2017. doi: 10.1523/ENEURO.0163-15.2017
Shpokayte, M., Mckissick, O., Guan, X., Yuan, B., Rahsepar, B., Fernandez, F. R., et al. (2022). Hippocampal cells segregate positive and negative engrams. Commun. Biol. 5:1009. doi: 10.1038/s42003-022-03906-8
Sloviter, R. S., and Lomo, T. (2012). Updating the lamellar hypothesis of hippocampal organization. Front. Neural Circ. 6:102. doi: 10.3389/fncir.2012.00102
Stahl, P. L., Salmen, F., Vickovic, S., Lundmark, A., Navarro, J. F., Magnusson, J., et al. (2016). Visualization and analysis of gene expression in tissue sections by spatial transcriptomics. Science 353, 78–82. doi: 10.1126/science.aaf2403
Stanfield, B. B., and Cowan, W. M. (1979). The morphology of the hippocampus and dentate gyrus in normal and reeler mice. J. Comp. Neurol. 185, 393–422. doi: 10.1002/cne.901850302
Stuchlik, A., Petrasek, T., Prokopova, I., Holubova, K., Hatalova, H., Vales, K., et al. (2013). Place avoidance tasks as tools in the behavioral neuroscience of learning and memory. Physiol. Res. 62, S1–S19. doi: 10.33549/physiolres.932635
Sullivan, K. E., Kendrick, R. M., and Cembrowski, M. S. (2021). Elucidating memory in the brain via single-cell transcriptomics. J. Neurochem. 157, 982–992. doi: 10.1111/jnc.15250
Sun, X., Bernstein, M. J., Meng, M., Rao, S., Sorensen, A. T., Yao, L., et al. (2020). Functionally distinct neuronal ensembles within the memory engram. Cell 181, 410–423.e17. doi: 10.1016/j.cell.2020.02.05
Sun, X., and Lin, Y. (2016). Npas4: linking neuronal activity to memory. Trends Neurosci. 39, 264–275. doi: 10.1016/j.tins.2016.02.003
Sweis, B. M., Mau, W., Rabinowitz, S., and Cai, D. J. (2021). Dynamic and heterogeneous neural ensembles contribute to a memory engram. Curr. Opin. Neurobiol. 67, 199–206. doi: 10.1016/j.conb.2020.11.017
Takeuchi, T., Duszkiewicz, A. J., and Morris, R. G. (2014). The synaptic plasticity and memory hypothesis: encoding, storage and persistence. Philos. Trans. R. Soc. Lond. Ser. B Biol. Sci. 369:20130288. doi: 10.1098/rstb.2013.0288
Terranova, J. I., Yokose, J., Osanai, H., Marks, W. D., Yamamoto, J., Ogawa, S. K., et al. (2022). Hippocampal-amygdala memory circuits govern experience-dependent observational fear. Neuron 110:e13. doi: 10.1016/j.neuron.2022.01.019
Terranova, J. I., Yokose, J., Osanai, H., Ogawa, S. K., and Kitamura, T. (2023). Systems consolidation induces multiple memory engrams for a flexible recall strategy in observational fear memory in male mice. Nat. Commun. 14:3976. doi: 10.1038/s41467-023-39718-5
The Gene Ontology Consortium (2019). The gene ontology resource: 20 years and still going strong. Nucleic Acids Res. 47, D330–D338. doi: 10.1093/nar/gky1055
Tonegawa, S., Morrissey, M. D., and Kitamura, T. (2018). The role of engram cells in the systems consolidation of memory. Nat. Rev. Neurosci. 19, 485–498. doi: 10.1038/s41583-018-0031-2
Tonegawa, S., Pignatelli, M., Roy, D. S., and Ryan, T. J. (2015). Memory engram storage and retrieval. Curr. Opin. Neurobiol. 35, 101–109. doi: 10.1016/j.conb.2015.07.009
Tyssowski, K. M., Destefino, N. R., Cho, J. H., Dunn, C. J., Poston, R. G., Carty, C. E., et al. (2018). Different neuronal activity patterns induce different gene expression programs. Neuron 98, 530–546. e11. doi: 10.1016/j.neuron.2018.04.001
Van Strien, N. M., Cappaert, N. L., and Witter, M. P. (2009). The anatomy of memory: an interactive overview of the parahippocampal-hippocampal network. Nat. Rev. Neurosci. 10, 272–282. doi: 10.1038/nrn2614
Vanrobaeys, Y., Mukherjee, U., Langmack, L., Beyer, S. E., Bahl, E., Lin, L. C., et al. (2023). Mapping the spatial transcriptomic signature of the hippocampus during memory consolidation. Nat. Commun. 14:6100. doi: 10.1038/s41467-023-41715-7
Wachi, T., Cornell, B., and Toyo-Oka, K. (2017). Complete ablation of the 14-3-3epsilon protein results in multiple defects in neuropsychiatric behaviors. Behav. Brain Res. 319, 31–36. doi: 10.1016/j.bbr.2016.11.016
Wang, M., Zhao, Y., and Zhang, B. (2015). Efficient test and visualization of multi-set intersections. Sci. Rep. 5:16923. doi: 10.1038/srep16923
Weeber, E. J., Atkins, C. M., Selcher, J. C., Varga, A. W., Mirnikjoo, B., Paylor, R., et al. (2000). A role for the β isoform of protein kinase C in fear conditioning. J. Neurosci. 20, 5906–5914. doi: 10.1523/JNEUROSCI.20-16-05906.2000
Wesierska, M., Dockery, C., and Fenton, A. A. (2005). Beyond memory, navigation, and inhibition: behavioral evidence for hippocampus-dependent cognitive coordination in the rat. J. Neurosci. 25, 2413–2419. doi: 10.1523/JNEUROSCI.3962-04.2005
Wu, T., Hu, E., Xu, S., Chen, M., Guo, P., Dai, Z., et al. (2021). clusterProfiler 4.0: a universal enrichment tool for interpreting omics data. Innovation 2:100141. doi: 10.1016/j.xinn.2021.100141
Yao, Z., Van Velthoven, C. T. J., Nguyen, T. N., Goldy, J., Sedeno-Cortes, A. E., Baftizadeh, F., et al. (2021). A taxonomy of transcriptomic cell types across the isocortex and hippocampal formation. Cell 184, 3222–3241. e26. doi: 10.1016/j.cell.2021.04.021
Yap, E. L., and Greenberg, M. E. (2018). Activity-regulated transcription: bridging the gap between neural activity and behavior. Neuron 100, 330–348. doi: 10.1016/j.neuron.2018.10.013
Yelhekar, T. D., Meng, M., Doupe, J., and Lin, Y. (2024). All Iegs are not created equal-molecular sorting within the memory engram. Adv. Neurobiol. 38, 81–109. doi: 10.1007/978-3-031-62983-9_6
Keywords: spatial transcriptomics, gene expression, memory, hippocampus, immediate early gene, IEG-expressing ensemble, memory-associated neuronal ensemble
Citation: Vingan I, Phatarpekar S, Tung VSK, Hernández AI, Evgrafov OV and Alarcon JM (2024) Spatially resolved transcriptomic signatures of hippocampal subregions and Arc-expressing ensembles in active place avoidance memory. Front. Mol. Neurosci. 17:1386239. doi: 10.3389/fnmol.2024.1386239
Edited by:
Victor Ramírez-Amaya, Medical Research Institute Mercedes and Martín Ferreyra (INIMEC), ArgentinaReviewed by:
Peer Wulff, University of Kiel, GermanyVarun B. Chokshi, Johns Hopkins University, United States
Kira Balueva, Christian Albrechts University, Germany
Copyright © 2024 Vingan, Phatarpekar, Tung, Hernández, Evgrafov and Alarcon. This is an open-access article distributed under the terms of the Creative Commons Attribution License (CC BY). The use, distribution or reproduction in other forums is permitted, provided the original author(s) and the copyright owner(s) are credited and that the original publication in this journal is cited, in accordance with accepted academic practice. No use, distribution or reproduction is permitted which does not comply with these terms.
*Correspondence: Juan Marcos Alarcon, anVhbm1hcmNvcy5hbGFyY29uQGRvd25zdGF0ZS5lZHU=