- 1School of Pharmacy, Shanghai University of Medicine and Health Sciences, Shanghai, China
- 2Shanghai Key Laboratory of Molecular Imaging, Shanghai University of Medicine and Health Sciences, Shanghai, China
- 3School of Health Science and Engineering, University of Shanghai for Science and Technology, Shanghai, China
- 4Department of Ultrasound Medicine, Huashan Hospital Affiliated to Fudan University, Shanghai, China
- 5Department of Neurology, Zhongshan Hospital, Fudan University, Shanghai, China
Introduction: Alzheimer’s disease (AD) is a progressive and debilitating neurodegenerative disorder prevalent among older adults. Although AD symptoms can be managed through certain treatments, advancing the understanding of underlying disease mechanisms and developing effective therapies is critical.
Methods: In this study, we systematically analyzed transcriptome data from temporal lobes of healthy individuals and patients with AD to investigate the relationship between AD and mitochondrial autophagy. Machine learning algorithms were used to identify six genes—FUNDC1, MAP1LC3A, CSNK2A1, VDAC1, CSNK2B, and ATG5—for the construction of an AD prediction model. Furthermore, AD was categorized into three subtypes through consensus clustering analysis.
Results: The identified genes are closely linked to the onset and progression of AD and can serve as reliable biomarkers. The differences in gene expression, clinical features, immune infiltration, and pathway enrichment were examined among the three AD subtypes. Potential drugs for the treatment of each subtype were also identified.
Discussion: The findings observed in the present study can help to deepen the understanding of the underlying disease mechanisms of AD and enable the development of precision medicine and personalized treatment approaches.
1. Introduction
Alzheimer’s disease (AD) is a debilitating neurodegenerative disorder characterized by a progressive decline in memory and cognitive function. The underlying mechanisms of AD require further elucidation in spite of considerable research on the subject, and current therapeutic options are limited (Lane et al., 2018). Recently, alterations in cellular energy metabolism, including defects in mitochondrial function, were reported to influence AD pathogenesis (Moreira et al., 2010; Wang et al., 2020).
Mitochondrial autophagy, also known as mitophagy, is a crucial cellular process involved in maintaining cellular homeostasis and energy metabolism. Mitophagy is a process of selective degradation through which damaged or surplus mitochondria are targeted for removal, thereby promoting mitochondrial turnover and preventing the accumulation of damaged mitochondria (Kim et al., 2007). Reportedly, AD is characterized by the accumulation of damaged neuronal mitochondria and increased oxidative stress caused as a result of the impairment of mitophagy (Smith et al., 2000; Manczak et al., 2006; Kerr et al., 2017; De Gaetano et al., 2021). In addition, mitophagy dysfunction inhibits ATP production and activates AMPK. Excessive activation of AMPK further reduces ATP production and induces tau protein phosphorylation, which is crucial for Aβ synaptic toxicity. Additionally, impaired mitophagy has a negative impact on microglia. Microglia play a key role in clearing neurotoxic protein components; however, when mitophagy is impaired, microglia cannot effectively phagocytize and remove Aβ plaques, resulting in the accumulation of Aβ (Leuner et al., 2012; Fang et al., 2019; Song et al., 2021); these are typically considered characteristic features of AD. Furthermore, mitophagy is known to be affected by AD-associated genetic mutations, including those in the presenilin 1 gene (Martin-Maestro et al., 2017), highlighting a direct connection between AD and mitophagy. Promoting mitophagy can improve cognitive function in AD animal models, and drugs targeting the mitophagy pathway may have therapeutic potential for the treatment of AD (Cen et al., 2020, 2021; Chen et al., 2021; Liang et al., 2021; Wang et al., 2021).
These findings highlight the fact that the relationship between AD and mitophagy has progressively garnered attention as an important field of research with immense potential to improve the current understanding of the underlying mechanisms of AD and to develop novel therapeutic strategies.
The purpose of the present study was to gain a deeper understanding of the relationship between AD and mitophagy through a systematic analysis of transcriptomic data from the middle temporal gyrus (MTG) in healthy individuals and patients with AD. Machine learning algorithms were utilized to construct prediction models for AD based on 27 mitophagy-related genes (MRGs). These models were evaluated in terms of their performance through multiple validation techniques, including receiver operating characteristic (ROC) curves, calibration curves, nomograms, decision curve analyses (DCA), and external datasets. Additionally, three subtypes of AD were developed through consistent cluster analysis, the biological functions, and pathways specific to each subtype were compared, and interpatient differences were analyzed in terms of age and sex in each subtype. Weighted gene co-expression network analysis (WGCNA) was used to obtain hubgenes, and the Connectivity Map (CMap) database was utilized to identify potential small molecule drugs that may target each subtype.
2. Materials
2.1. Data acquisition and processing
The NCBI Gene Expression Omnibus (GEO) public database1 was used to search for gene expression data, and these data were obtained using the “GEOquery” R package. The data comprised two datasets, GSE109887 (GPL10904 platform) and GSE132903 (GPL10558 platform), which were merged to form a total of 130 and 143 samples from healthy individuals and patients with AD, respectively. The samples were collected from the MTG, a site of early AD pathology (Ray and Zhang, 2010; Chen et al., 2022). Merged data were processed to eliminate batch effects from different platforms and to normalize the data using the “sva” package. A principal component analysis (PCA) was subsequently performed to assess data combinations. The performance of the prediction model was validated using the GSE5281 dataset (GPL570 platform), which contained 74 and 87 samples from healthy individuals and patients with AD, respectively, and the samples were obtained from several brain regions including the entorhinal cortex, hippocampus, medial temporal gyrus, posterior cingulate, superior frontal gyrus, and primary visual cortex.
The mitophagy-associated gene set “REACTOME_MITOPHAGY.v7.5.1.gmt” was procured from the Reactome database. Meanwhile, the gene set “BIOCARTA_INFLAM_PATHWAY.v7.5.1.gmt,” which is associated with inflammation factors, was acquired from the Biocarta database.
2.2. Gene set variation analysis
The gene set variation analysis (GSVA) was performed using the “GSVA” R package to investigate the differences in the expression of MRGs between patients with AD and healthy individuals and between subtypes of AD. The results were visualized using the “ggpubr” package to clearly demonstrate the variations in gene expression.
2.3. Identification of differentially expressed MRGs
Differential gene expression between samples was determined using the “limma” package, with a stringent criterion of p < 0.05. The MRGs with differential expression in patients with AD were determined by taking into consideration the intersection of the differentially expressed genes (DEGs) and MRGs. The DEGs were visualized as a volcano plot and heatmap generated using the “ggplot2” and “pheatmap” packages, respectively.
2.4. Construction and validation of prediction models
The 27 MRGs were screened using the random forest method, and the characteristic genes were selected according to the minimum cross-validation error achieved from the ntree = 1,000 iteration. The importance score of the selected characteristic genes was subsequently evaluated. The selection process was made more specific through the application of a stepwise regression algorithm (Gu et al., 2022) to the top 10 genes. The prediction models were established through multifactor logistic regression and the graphical representation of the model was depicted using the forest plot obtained from the R package “forestplotter.” Screening and modeling processes were implemented using the “randomForest” and “caret” R packages, respectively. ROC curves were constructed using the R package “pROC,” and the area under the ROC curve (AUC) of the prediction model was determined. Furthermore, a nomogram model was established using the “rms” R package. The predictive power of each characteristic gene was quantified by assigning a predictive power score, and the total score represented the sum of the predictive power scores for all characteristics in the prediction model. The predictive power of the line graph model was evaluated by using calibration curves and DCA (Lai et al., 2021). The validity of these results was verified with an external dataset.
2.5. Analysis of immune infiltration and expression levels of inflammatory factor genes
The relative proportion of infiltrated immune cells was quantified using the MCPcounter algorithm. MCPcounter is a transcriptomic-based quantitative method that measures the absolute abundance of eight immune cell populations (T cells, CD8 T cells, Cytotoxic lymphocytes, B lineage, NK cells, Monocytic lineage, Myeloid dendritic cells, and Neutrophils) and two stromal cell populations (Endothelial cells, Fibroblasts) in heterogeneous tissues. The method relies on cell type-specific gene expression values to derive an abundance score for each individual cell type and sample, allowing for direct comparison of cell type abundance across different experimental conditions. Additionally, the expression levels of inflammatory factor genes were analyzed in the samples from healthy individuals and patients with AD, as well as in subtypes, to detect differential expression. The correlation between MRGs and infiltrated immune cells and inflammatory factors was explored using Spearman’s correlation coefficient.
2.6. Consensus clustering analysis
Unsupervised clustering analysis was performed on the 143 samples from patients with AD using the “ConsensusClusterPlus” R package according to the expression profiles of 27 MRGs. The K-means algorithm was utilized, with a maximum subtype number set at 10 (k = 10), a sampling ratio of 0.8, and 100 re-sampling times. The optimal number of clusters was determined through the evaluation of the cumulative distribution function (CDF) curve, tracking plots, consensus matrix, delta area, and consistent cluster score (>0.9) based on the results of consensus clustering.
2.7. Analysis of enrichment
The gene ontology (GO) and Kyoto Encyclopedia of Genes and Genomes (KEGG) pathway analysis of DEGs among subtypes was performed using the “clusterProfiler” R software package.
2.8. Weighted gene co-expression network analysis
Weighted gene co-expression network analysis was performed using the “WGCNA” R package for the identification of the hub genes for each subtype. The genes in the top 5,000 of median absolute deviation (MAD) were initially selected, and a similarity matrix was constructed by computing the correlation coefficients between each pair of genes. The soft threshold of 12 was subsequently used for the conversion of a similarity matrix into an adjacency matrix, and further into a topological overlap matrix (TOM) to evaluate the average network connectivity of each gene. The “blockwiseModules” functions (minModuleSize = 30, mergeCutHeight = 0.25) were used to categorize genes with similar expression profiles into modules, and each module was identifiable with a unique color. The module signature genes (ME) represented the gene expression profile of each module and facilitated the authors in accurately assessing the relationship with the phenotype. Module membership (MM) represents the relevance of the gene to the module through the correlation coefficient with the gene expression values and ME. ME and MM were used to identify crucial subtype-specific modules.
2.9. Identification of potential small molecule drugs
Potential subtype-specific small molecule drugs for the treatment of AD were identified by obtaining the intersection of core genes and DEGs for each subtype. The top 150 upregulated and downregulated genes with the highest fold change were subsequently inputted into the Connectivity Map (CMAP) database.2 The purpose of this search was the identification of drugs with potentially beneficial effects on the treatment of AD according to subtype. The drug scores ranged from −100 to 100; a lower score indicated a greater potential for the drug in terms of its applications in the treatment of the corresponding AD subtype.
3. Results
3.1. Dysregulation of mitochondrial autophagy and activation of the immune system in AD
The expression profiles of 27 MRGs were compared between patients with AD and normal controls using the GSE109887 and GSE132903 datasets (Figures 1A,B). Typically, MRGs appeared to be downregulated in patients with AD (Figures 1C,D), and this result was validated by the GSE5281 dataset (Supplementary Figure 1A). Analysis of differential expression revealed 21 DEGs, of which 18 were downregulated (TOMM20, VDAC1, TOMM70A, CSNK2A1, MAP1LC3A, FUNDC1, UBB, MAP1LC3B, UBC, TOMM5, CSNK2B, SRC, ATG5, PARK2, TOMM6, PGAM5, TOMM22, and TOMM7) and three were upregulated (UBA52, MFN2, and ATG12; Figure 1E, Supplementary Figure 1B). Correlation analysis revealed that TOMM20, PARK2, SRC, and CSNK2A1 were positively correlated with multiple genes from the 21 differentially expressed mitophagy-related genes, while UBA52 and ATG12 were negatively correlated with multiple genes from the same gene set (Figure 1F). Inflammation factor expression analysis showed that CD4, CSF1, CSF3, IFNA1, and IL1A were downregulated in AD, whereas HLA-DRB3, HLA-DRB4, IL10, IL15, IL5, IL6, PDGFA, and TGFB3 were upregulated (Supplementary Figure 1C). Analysis of immune cell infiltration using the MCPcounter algorithm showed that the proportions of T cells, cytotoxic lymphocytes, and monocytes increased in patients with AD, whereas that of NK cells decreased (Figure 1G).
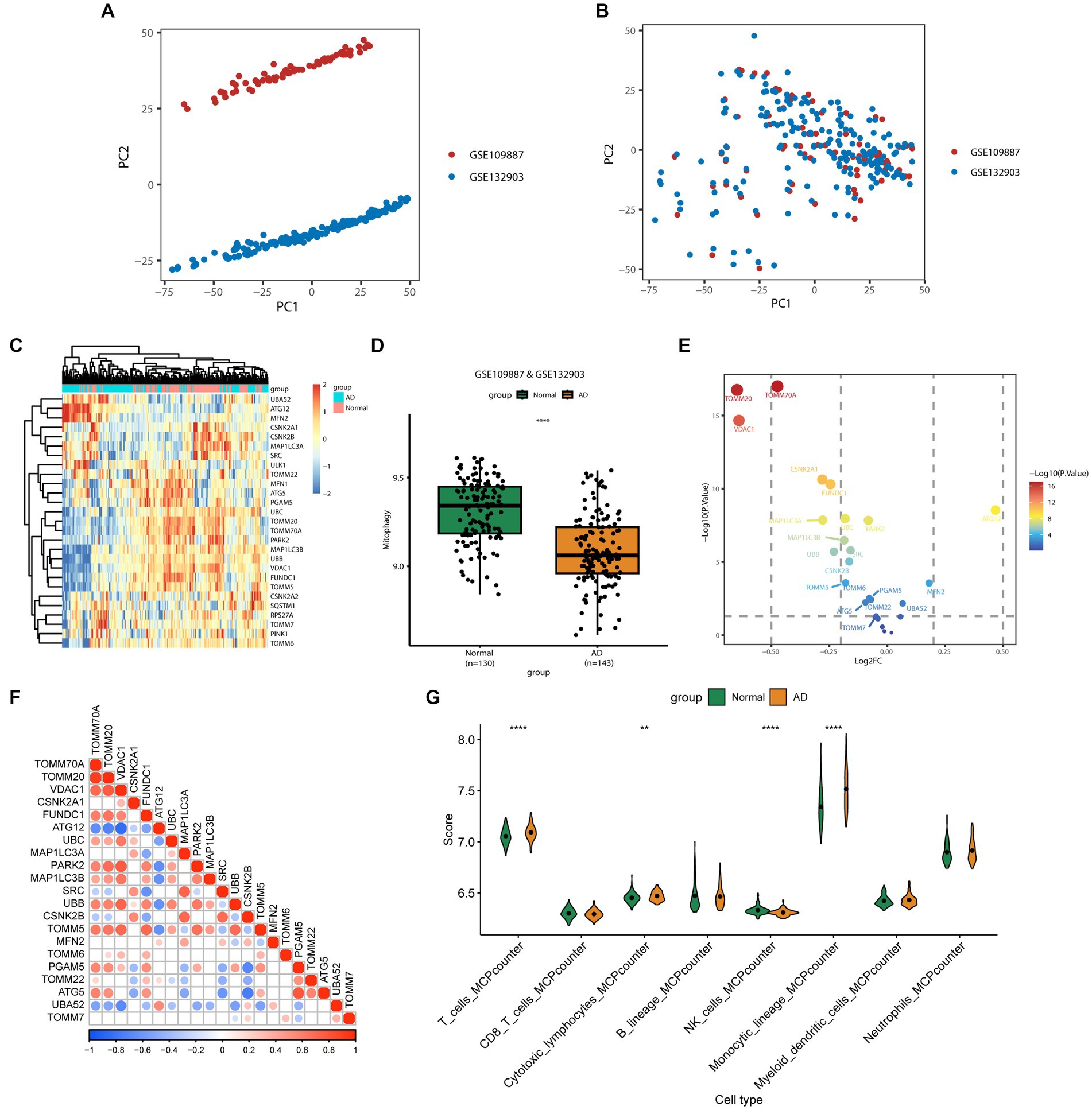
Figure 1. Differentially expressed mitophagy-related genes (MRGs) in Alzheimer’s disease (AD). (A) Principal component analysis (PCA) of GSE109887 and GSE132903 datasets before batch effect removal. (B) PCA of GSE109887 and GSE132903 datasets after batch effect removal. (C) The expression patterns of 27 MRGs are presented in the heatmap. Each row represents a specific MRG and each column represents a sample. The color gradient ranging from blue to red indicates low to high expression levels, respectively. (D) The box plot displays the GSVA scores of the MRG gene set across samples to observe the overall expression differences of MRG between healthy individuals and patients with AD. p values were estimated by Student’s t-test. ****p < 0.0001. (E) The volcano map illustrates 21 differentially expressed MRGs in AD, where a negative log2FC indicates downregulation and a positive log2FC indicates upregulation of the gene expression. (F) Correlation analysis between the 21 differentially expressed genes. Red and blue represent positive and negative correlations, respectively. The depth of color represents different correlation coefficients. (G) The violin diagram shows the difference in infiltrated immune cells between patients with AD and healthy subjects. The horizontal axis represents the immune cell types, while the vertical axis denotes the scores calculated by the mcpcounter algorithm. p values were estimated by Student’s t-test. **p < 0.01, ****p < 0.0001.
3.2. Construction and evaluation of predictive models
Multiple machine learning algorithms (Random Forest, Stepwise Regression, and Multivariate Logistic Regression) were used to screen the 27 MRGs genes and construct a predictive model for AD. First, the 27 genes were inputted into the Random Forest classifier, and the correlation between the model error and the number of random forest trees revealed that the error rate was the most stable when using 698 trees (Figure 2A). The top 10 genes, determined on the basis of importance ranking, were selected for further analysis using Stepwise Regression and Multivariate Logistic Regression (Figure 2B). The results of these algorithms showed that FUNDC1, MAP1LC3A, CSNK2A1, VDAC1, CSNK2B, and ATG5 were the six remaining genes (Figure 2C). Furthermore, their odds ratios were < 1, which suggested that they could be considered protective factors for AD. The predictive performance of these six genes was verified through ROC analysis (AUC = 0.887; Figure 2D), and a validation set ROC analysis (AUC = 0.843; Supplementary Figure 2A), which highlighted the model’s excellent performance in terms of predicting AD. A scoring model was consequently established to evaluate the probability of AD based on the expression of the abovementioned six genes (Figure 2E). Calibration and DCA curves were constructed and analyzed using the validation set, and the Apparent line and Bias-Corrected line in the calibration curve appeared to have a good fit with the Ideal line (Figure 2F, Supplementary Figure 2B). The DCA curve showed that the model had higher net profit when all the selected feature genes were used (Figure 2G, Supplementary Figure 2C), indicating that the model is reliable for predicting AD. Correlation analyses were subsequently performed between these six feature genes and the coding genes of amyloid precursor protein (APP) and tau protein (MAPT); these genes are closely associated with AD development and progression. The results showed that the expression of these six genes was positively correlated with both APP and MAPT (Supplementary Figures 3A,B), suggesting that these genes have exceptional predictive value for AD.
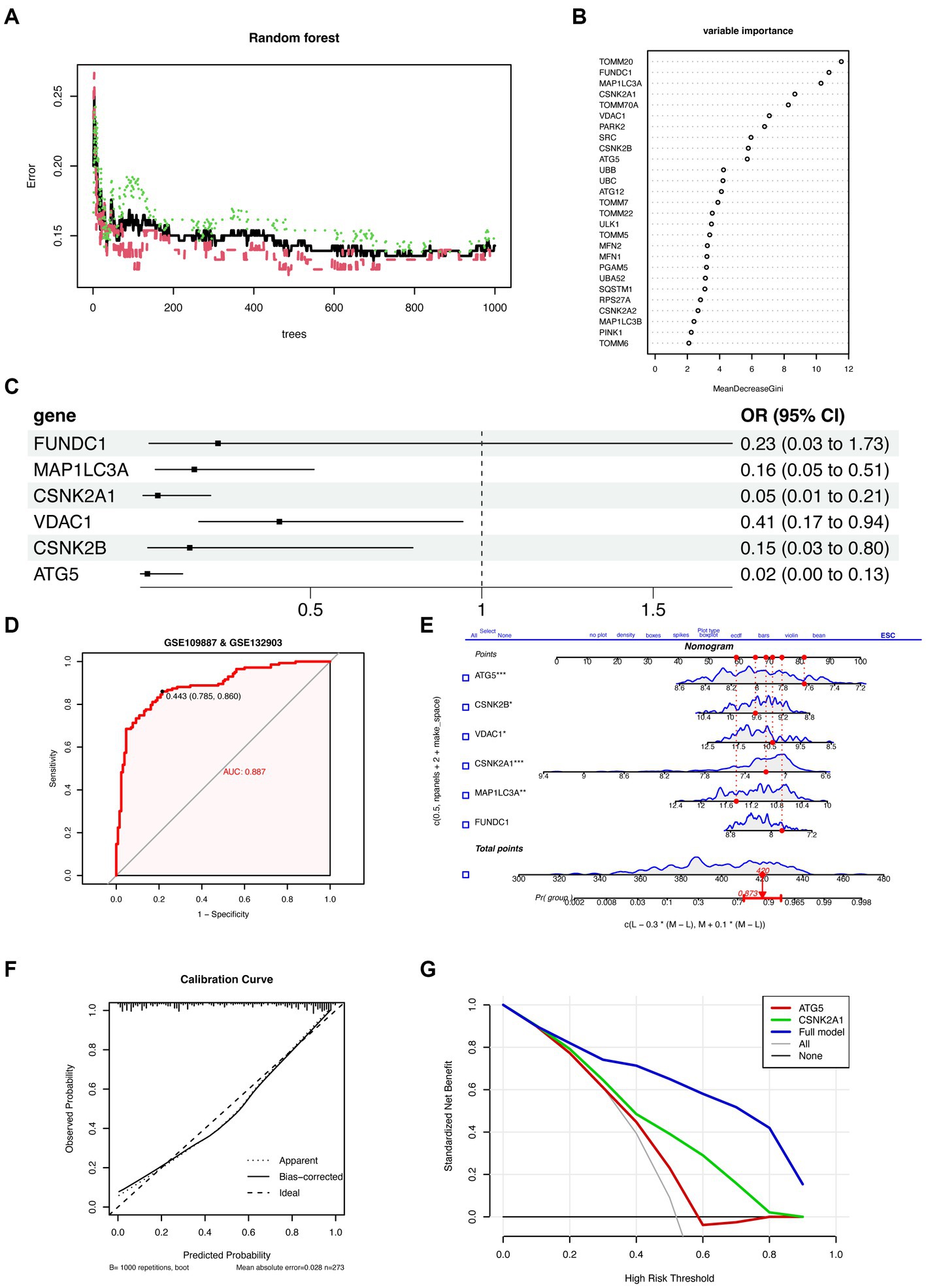
Figure 2. The establishment and evaluation of the predictive model. (A) Cumulative residual distribution of random forest learning model. The horizontal axis represents the number of trees (decision tree classifiers), while the vertical axis represents the corresponding prediction error under that number of trees. The plot demonstrates the variation of prediction error of the Random Forest learning model under different quantities of trees. (B) Ranking plot depicting the variable importance in random forest. The MeanDecreaseGini index is used to measure the importance of feature genes in the model, where a higher value indicates a higher level of importance of that variable in the model. (C) Forest plot demonstrating the six variables selected through stepwise logistic regression screening. (D) Receiver operating characteristic curve evaluating the diagnostic performance of feature genes. The horizontal axis represents the false positive rate, while the vertical axis represents the true positive rate. A higher AUC indicates a higher accuracy of the model in diagnosing the disease. (E) Nomogram for predicting the risk of AD based on feature genes. The feature weight of six important feature genes is used as input variables, where the “Points” refer to the corresponding scores of each input variable, and the “Total points” denote the total score obtained by adding up the scores of all input variables. “Pr (group)” represents the risk score corresponding to the “Total points,” which indicates the likelihood of developing AD. (F) Calibration curve illustrating the calibration performance of a predictive model. The horizontal axis represents the predicted value, while the vertical axis represents the actual observed value. The closer the bias-corrected curve is to the Ideal dashed line, the higher the calibration performance of the model. (G) DCA estimates the clinical benefit of the nomogram. The plot shows a comparison of the net clinical benefits for different prediction models at different decision thresholds. The blue model, which is constructed using six feature genes, performs the best, with the highest net clinical benefit relative to the optimal treatment reference line.
3.3. Identification of mitochondrial autophagy subtypes in AD
The consensus clustering method was applied to samples from patients with AD based on the expression profiles of 27 MRGs. The best clustering number was K = 3 (Figure 3A), based on a comprehensive evaluation of the CDF curve (Figure 3B), delta area (Figure 3C), tracking plot (Figure 3D), and consistent cluster score (Figure 3E). Differences in the expression of mitochondrial autophagy genes, clinical features (age and sex), and diagnostic model efficacy among the three subtypes were displayed using a heatmap (Figure 3F). The GSVA indicated that the overall expression of MRGs for the clusters was as follows in descending order of magnitude: clusters 1, 2, and 3 (Figure 3G).
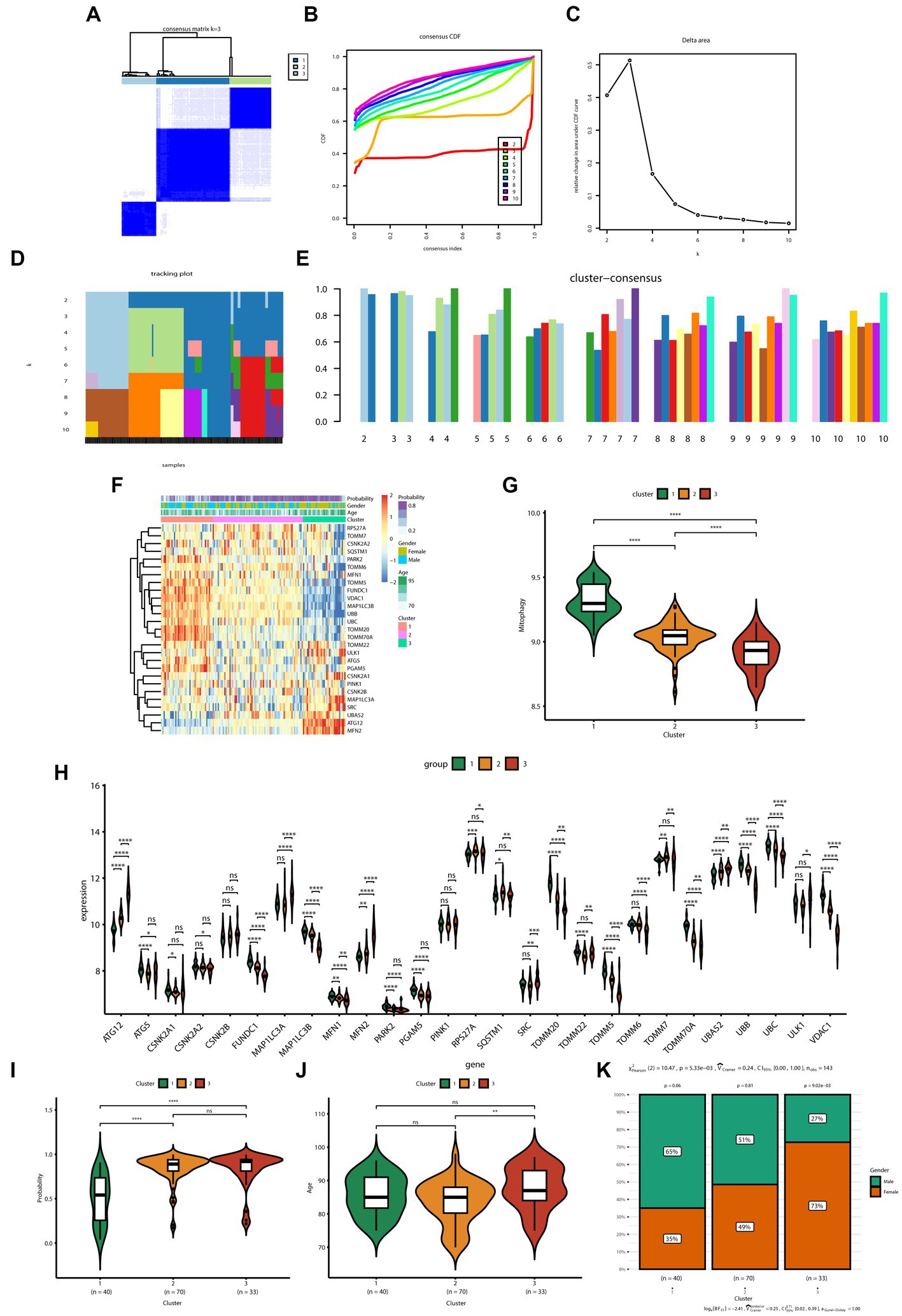
Figure 3. Identification of the subtypes of Alzheimer’s disease and differences between subtypes. (A) Consensus clustering matrix showing the clustering agreement between samples when k (number of clusters) = 3. (B) Representative cumulative distribution function (CDF) curve showing the clustering results for k (number of clusters) ranging from 2 to 10. (C) Relative changes in CDF delta area curves, which measure the stability of clustering across different values of k (number of clusters). (D) Tracking plot illustrating the classification of samples into different subtypes based on the clustering results obtained using different values of k (number of clusters). Each sample is assigned a different color based on its membership in different clusters. (E) Consensus scores for each subtype when k (number of clusters) ranges from 2 to 10. The x-axis represents the number of clusters, while the y-axis represents the consensus score for each cluster. A higher consensus score indicates a more robust clustering result. (F) Heatmap depicts the expression of 27 mitochondrial autophagy-related genes in three subtypes, with the addition of clinical information. The “Probability” shown in the color scale represents the likelihood that the patient be diagnosed with AD based on a diagnostic model constructed using six genes. (G) The boxplot displays the overall expression of MRGs among the three subtypes (cluster 1, cluster 2, and cluster 3). p values were estimated by Student’s t-test. ****p < 0.0001. (H) The expression levels of 27 MRGs were compared between three clusters (cluster 1: n = 40, cluster 2: n = 70, cluster 3: n = 33) using Student’s t-test. *p < 0.05, **p < 0.01, ***p < 0.001, ****p < 0.0001; ns, no significance. (I) Comparison of the diagnostic performance of the prediction models for the three subtypes (cluster 1: n = 40, cluster 2: n = 70, cluster 3: n = 33). p values were estimated by Student’s t-test. ****p < 0.0001; ns, no significance. (J) Comparison of the age of patients with AD in the three subtypes (cluster 1: n = 40, cluster 2: n = 70, cluster 3: n = 33). p values were estimated by Student’s t-test. **p < 0.01; ns, no significance. (K) Gender distribution of patients with AD in three subtypes.
The expression of specific genes—including PARK2, ATG5, FUNDC1, TOMM20, and VDAC1—was significantly lower in clusters 2 and 3 than in cluster 1 (Figure 3H). Furthermore, the AD prediction model constructed in the present study showed a higher predictive ability for clusters 2 and 3 than that for cluster 1 (Figure 3I). Moreover, patients with AD in cluster 3 were significantly older than those in cluster 2 (Figure 3J). In terms of sex, the number of female patients progressively increased from clusters 1 to 3; the number of female patients in cluster 3 was significantly higher than the number of male patients (Figure 3K).
3.4. Differences in immune infiltration of the subtypes of mitochondrial autophagy
The MCPcounter algorithm was used to analyze immune infiltration across three subtypes. The heatmap displayed the overall differences in immune infiltration among three subtypes (Figure 4A). Specifically, the infiltration levels of B lineage, myeloid dendritic cells, neutrophils, and T cells gradually increased from cluster 1 to 3. Additionally, the infiltration level of monocytic lineage in clusters 2 and 3 was significantly higher than those in cluster 1. Conversely, the infiltration levels of NK cells in clusters 2 and 3 were significantly lower than those in cluster 1 (Figure 4B). In terms of inflammatory factors, cluster 1 exhibited significantly lower expression levels of CD4, HLA-DRB3, IL10, PDGFA, and TGFB3 in contrast to clusters 2 and 3; however, IFNA1 and IL1A were markedly upregulated in cluster 1. Furthermore, Cluster 3 displayed the lowest expression levels of HLA-DRA, HLA-DRB4, L11, IL1A, IL6, and TGFB2, whereas L10, IL5, and PDGFA were significantly upregulated in cluster 3 (Supplementary Figure 4A).
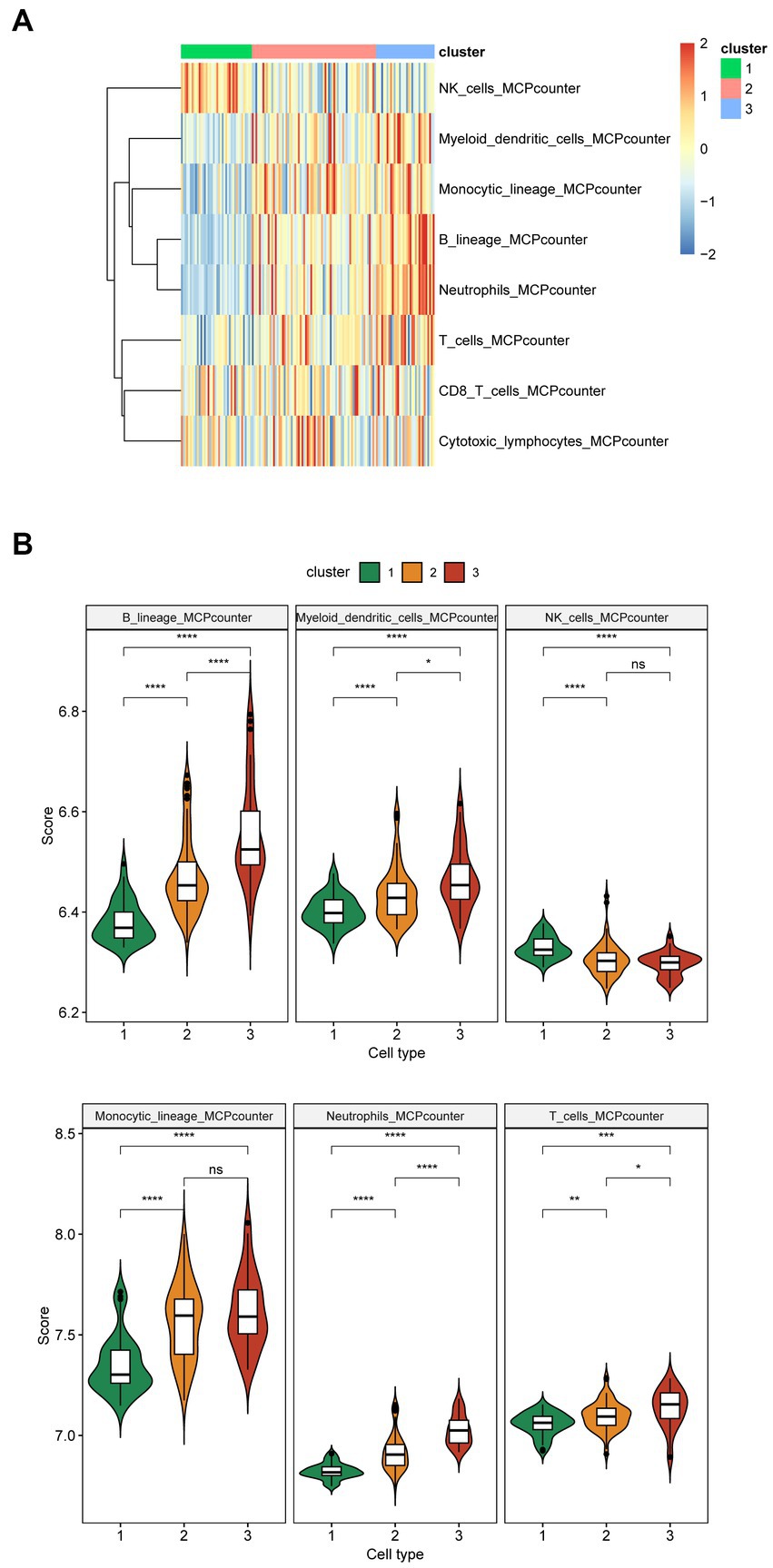
Figure 4. Immune infiltration of the three subtypes of Alzheimer’s disease. (A) The heatmap shows overall immune infiltration in the three subtypes. Blue represents relatively low levels of immune cell infiltration, while red represents relatively high levels of infiltration. (B) The violin plot shows immune infiltration in the three subtypes (cluster 1: n = 40, cluster 2: n = 70, cluster 3: n = 33). The x-axis represents different subtypes, while the y-axis represents the scores calculated by the MCPcounter algorithm. Immune cells with no difference in infiltration levels across the three cluster are not displayed. p values were estimated by Student’s t-test. *p < 0.05, **p < 0.01, ***p < 0.001, ****p < 0.0001; ns, no significance.
3.5. GO and KEGG enrichment analysis of three subtypes
Gene ontology and KEGG enrichment analyses were performed on DEGs of one subtype relative to the other two subtypes. The findings of GO enrichment analysis demonstrated that the subtypes examined herein exhibited distinct functional characteristics in terms of cellular growth, synaptic organization, and neuronal projection. Although all three subtypes shared certain similarities in the regulating processes related to neuronal projection extension and chemosynaptic transmission, the subtypes were different in terms of their specific biological processes. Cluster 1 was associated with regulation of binding, regulation of synapse structure or activity, and regulation of axonogenesis (Figure 5A), whereas cluster 2 was associated with regulation of nervous system development, developmental cell growth, and vesicle−mediated transport in synapse (Figure 5B). Cluster 3 was involved in positive regulation of cellular catabolic process, regulation of cell growth, and regulation of apoptotic signaling pathway (Figure 5C). Although clusters 1 and 2 shared certain functional characteristics, including protein complex oligomerization and protein localization to the plasma membrane, cluster 3 was predominantly different with regard to its involvement in the organic acid catabolic process and regulation of DNA-binding transcription factor activity.
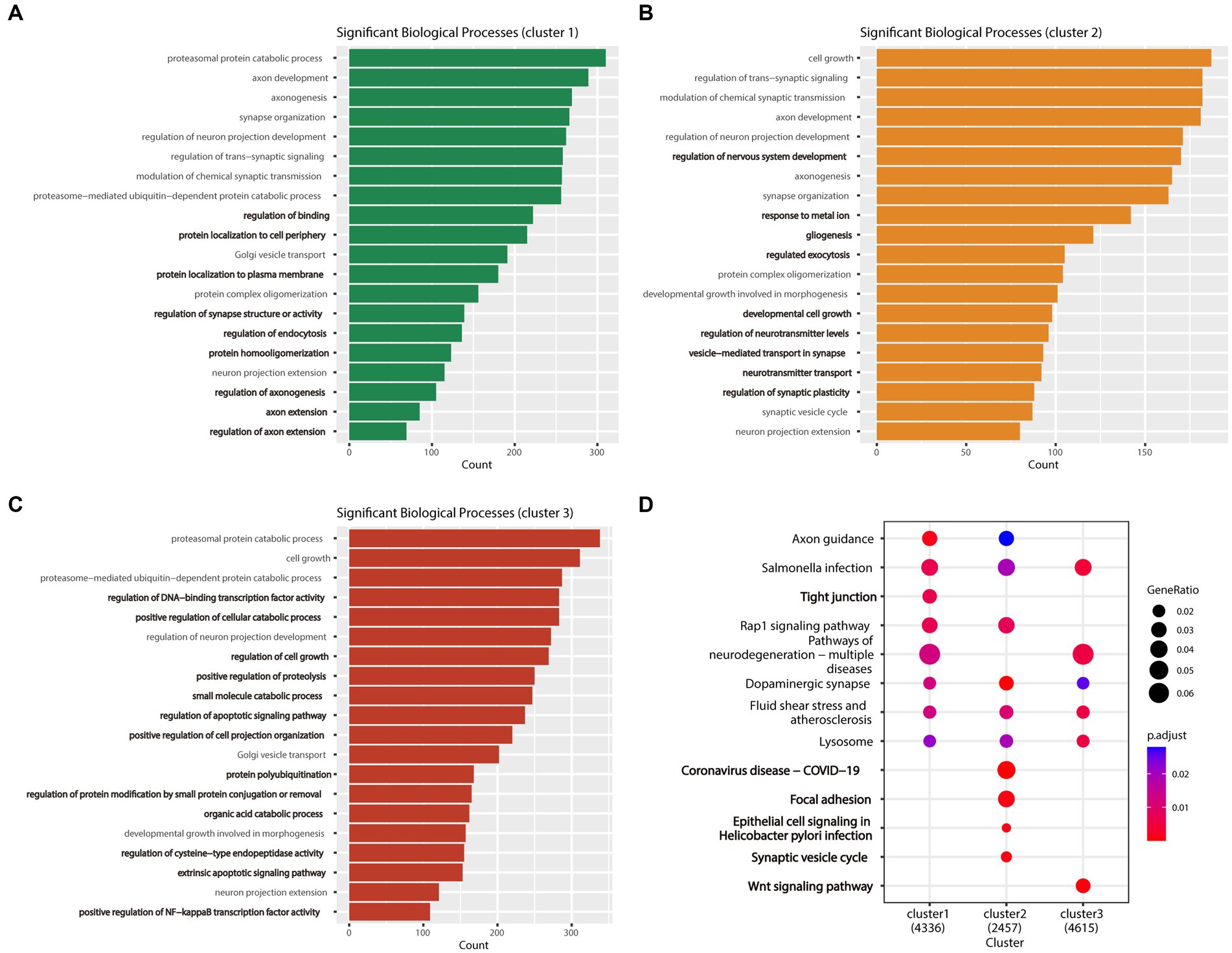
Figure 5. Enrichment analysis results of the three AD subtypes. (A) Bar plot of the biological process GO analysis results for Cluster 1, with the biological processes unique to this cluster highlighted in bold. (B) Bar plot of the biological process GO analysis results for Cluster 2, with the biological processes unique to this cluster highlighted in bold. (C) Bar plot of the biological process GO analysis results for Cluster 3, with the biological processes unique to this cluster highlighted in bold. (D) The bubble plot shows the KEGG pathway analysis results for the three subtypes (clusters 1, 2, and 3) combined, with pathways unique to each subtype highlighted in bold.
Kyoto Encyclopedia of Genes and Genomes pathway enrichment analysis of the three subtypes enabled the identification of commonly enriched pathways across all three subtypes, including the dopaminergic synapse, lysosome, and salmonella infection pathways. Notably, each subtype exhibited its unique pathways. Cluster 2 was primarily enriched in pathways related to coronavirus disease infectious disease (COVID-19), epithelial cell signaling in Helicobacter pylori infections, focal adhesion, and synaptic vesicle cycle. In contrast, clusters 1 and 3 were associated with the tight junction and the wnt signaling pathways. Furthermore, we found that the rap1 signaling pathway was commonly enriched in clusters 1 and 2, whereas the pathways of neurodegeneration—multiple diseases were commonly enriched in clusters 1 and 3 (Figure 5D).
3.6. Screening of hub genes and prediction of small molecule drugs
Weighted gene co-expression network analysis was performed to identify key gene modules associated with each subtype of AD. By combining the R2 values and the Mean Connectivity values, a soft threshold of 12 was selected to construct a scale-free network (Figure 6A), and 12 modules were identified using hierarchical clustering. Each of these modules was represented through different colors, while the gray module represented unassigned genes (Figure 6B). Based on the correlation analysis between each module (excluding the gray module) and clinical features (clusters 1, 2, and 3), we selected the “brown” module as the core module for cluster 1, the “yellow” module for cluster 2, and the “green” module for cluster 3 (Figure 6C). In order to identify hub genes in the core modules associated with each subtype, we used the KME (module eigengene-based connectivity measure) value as a screening criteria. KME measures the inter-gene correlation within a module, and high KME values indicate that a gene is highly connected with other genes in the module and may act as a hub gene. By intersecting the hub genes identified by KME with the DEGs in each subtype, we determined the upregulated and downregulated genes that were most closely associated with each subtype. Because of the input limit of 150 genes for cMap, DEGs exceeding 150 were sorted using fold change, and only the top 150 genes were selected. By combining the findings from cMap analysis and relevant literature, we determined that the molecule with the lowest score was the most promising predicted drug for each subtype. Therefore, LY-278584, cetraxate, and embelin were considered predicted drugs for subtypes 1, 2, and 3, respectively (Figure 6D; Table 1).
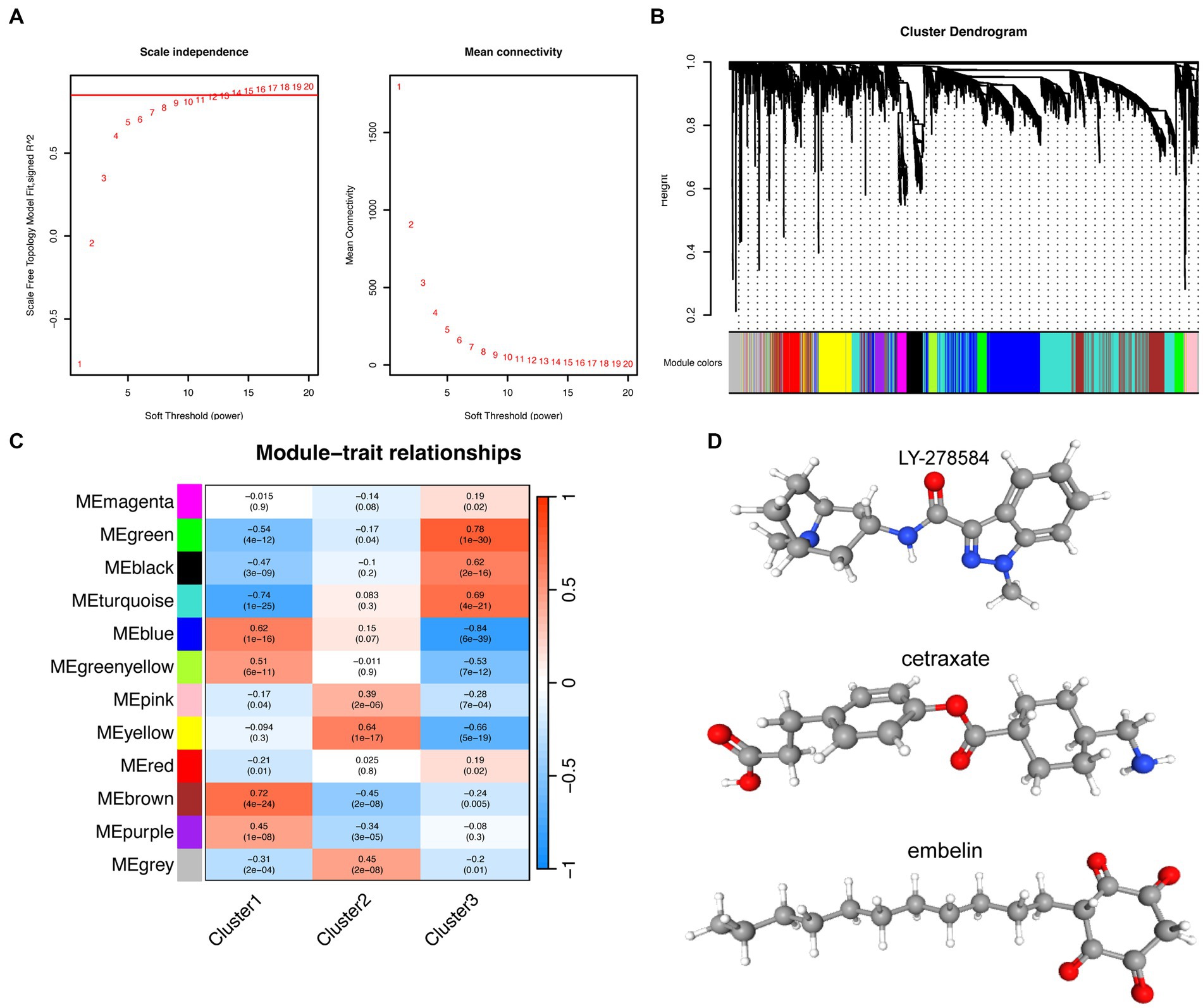
Figure 6. Prediction of small molecule drugs for the three subtypes of Alzheimer’s disease. (A) The left panel shows the indices of the scale-free topological model fit for different soft threshold powers, while the right panel displays the corresponding mean connectivity values for each soft threshold power. (B) Cluster dendrogram of genes. The leaf nodes (i.e., the bottom-most nodes) of the cluster dendrogram represent individual genes or small clusters, while the top of the tree represents the overall expression pattern of all genes in the gene set. (C) Correlations between different modules and clusters. Each row represents a different gene co-expression module, and each column represents a different cluster. The values in the boxes represent the correlation coefficients, with red and blue distinguishing positive and negative correlations, respectively. The values in parentheses represent the significance value of p. (D) Molecular structures of predicted small molecule drugs: LY-278584, cetraxate, and embelin.
4. Discussion
Alzheimer’s disease, a progressive and debilitating neurodegenerative condition, represents the most common cause of dementia among older adults (Hou et al., 2017). It is a complex disorder and involves multiple etiological factors including genetic and environmental influences (Armstrong, 2013); additionally, the underlying mechanisms of AD remain unclear. Mitophagy in neurons is believed to critically influence AD pathogenesis (Chu, 2019); therefore, the potential of targeting MRGs for the treatment of AD should be explored owing to its significant importance in clinical settings. To this end, MRGs were used to construct a predictive model of AD and refine AD subtypes to further clarify the mechanism underlying the pathogenesis of AD, reveal the heterogeneity of AD, and provide new ideas and methods for understanding and treating AD. Transcriptome data from the MTG of healthy individuals and patients with AD were systematically analyzed to investigate the relationship between AD and mitochondrial autophagy. GSVA revealed that the overall expression of MRGs was downregulated in patients with AD. Specifically, 18 MRGs, including those involved in the TOM complex (TOMM20, TOMM70A, TOMM5, TOMM6, TOMM22, and TOMM7), were significantly downregulated, whereas only three genes (UBA52, MFN2, and ATG12) were upregulated. The TOM complex was reported to play a crucial role in mitochondrial protein transport and localization, as well as in the synthesis and maintenance of mitochondrial homeostasis (Wang et al., 2021). Therefore, downregulation of the expression of this complex may result in mitochondrial dysfunction (Chai et al., 2018). In light of these findings, it is evident that changes in the expression levels of genes related to mitochondrial autophagy may play a critical role in AD development and progression.
Developing a predictive model for AD based on MRGs is a promising approach to elucidate the underlying mechanisms of AD and to develop new treatment strategies. Defects in mitochondrial autophagy were reported to critically influence AD pathogenesis. However, to the best of our knowledge, few studies have attempted to systematically investigate the potential utility of MRGs in predicting AD. The purpose of the present study was to address this paucity of information through the use of using machine learning algorithms to identify six genes—FUNDC1, MAP1LC3A, CSNK2A1, VDAC1, CSNK2B, and ATG5—out of 27 MRGs, to construct a prediction model for AD. FUNDC1 is a mitochondrial outer membrane protein that critically affects the regulation of mitochondrial quality control and metabolism. FUNDC1 is reported to play an important role in several diseases, such as cancer, cardiovascular disease, and neurological disorders, including AD (Chen et al., 2016). In an in vitro model of hippocampal neurons obtained from acquired epilepsy, low FUNDC1 expression significantly increased neuronal apoptosis (Zhang et al., 2023). The downregulation of FUNDC1 levels in cases of AD in particular may lead to mitochondrial dysfunction, accelerating the AD progression (Jetto et al., 2022). MAP1LC3A, also known as LC3A, is a protein associated with mitophagy and autophagy. This protein binds to autophagosomes formed during the autophagy process and participates in waste degradation after the fusion of autophagosomes and lysosomes (Cherra et al., 2010; Iriondo et al., 2022). VDAC1—an ion channel on the mitochondrial membrane—is closely related to mitochondrial function and stability (Hiller et al., 2008). VDAC1 can interact with Aβ and phosphorylated tau, which are associated with the deposition and toxicity of Aβ, leading to mitochondrial dysfunction and cell apoptosis. In addition, VDAC1 has a critical role in neuronal development and synapse formation, which implies that it is potentially involved in AD onset and progression (Marin et al., 2007; Cuadrado-Tejedor et al., 2011; Manczak and Reddy, 2012; Reddy, 2013; Smilansky et al., 2015). CSNK2A1 and CSNK2B encode the α and β subunits, respectively, of the protein kinase CK2, which plays a vital role in regulating Aβ deposition and MAPT phosphorylation in AD (Borgo et al., 2021). ATG5 is an important gene in the autophagy pathway; it participates in the formation and regulation of intracellular membrane structures. During the process of autophagy, ATG5 forms a complex with ATG12 and participates in the formation and degradation of autophagic vesicles (Codogno and Meijer, 2006; Walczak and Martens, 2013). The autophagy pathway was reported to play an important role in AD onset and progression (Li et al., 2010), and ATG5 is thought to be closely associated with the occurrence and development of AD. In light of this information, multiple validation techniques were used in the present study to evaluate the performance of these models. Across different datasets, the AUC values were 0.887 and 0.843, respectively. Our models demonstrated high predictive capability for AD. Furthermore, we found a positive correlation between these six genes and the genes encoding APP and MAPT, suggesting that FUNDC1, MAP1LC3A, CSNK2A1, VDAC1, CSNK2B, and ATG5 have the potential to be used as reliable biomarkers to successfully establish AD diagnosis.
Consistent cluster analysis was used to classify AD into three subtypes based on the expression profiles of MRGs, and the differences between these subtypes were analyzed in terms of gene expression, clinical features, immune infiltration, and pathway enrichment. Notably, MRGs exhibited significant differences among the three subtypes. Among the six diagnostic genes, FUNDC1 and VDAC1 showed significant differences in terms of their expression in each subtype, both genes showed the highest expression levels in cluster 1, intermediate expression levels in cluster 2, and low expression in cluster 3. Considering that these diagnostic genes are protective factors for AD, a higher expression level is associated with a lower risk of developing AD. Moreover, high levels of FUNDC1 and VDAC1 may promote cellular autophagy (Xie et al., 2021; Liu et al., 2022), which could help eliminate the deposition of harmful proteins, including Aβ and MAPT. Furthermore, the predictive model presented in the current study appeared to be significantly better in terms of diagnostic performance for the subtypes with relatively low MRGs expression levels, i.e., clusters 2 and 3, than that for the subtype with higher MRGs expression levels, i.e., cluster 1.
The activation of the immune system is believed to alter central nervous system inflammatory mechanisms and increases amyloid protein load, which may lead to AD onset and progression (Heppner et al., 2015). In the current study, the analysis of differences in immune cells and inflammatory factors between patients with AD and healthy individuals showed that the proportions of T cells, cytotoxic lymphocytes, and monocytic lineage were increased in cases of AD, whereas NK cells were decreased. Regarding inflammatory factors, upregulated expression of HLA-DRB3, HLA-DRB4, IL10, IL15, IL5, IL6, PDGFA, and TGFB3 and downregulated expression of CD4, CSF1, CSF3, IFNA1, and IL1A was observed. Therefore, the immune system of patients with AD is dysregulated and may contribute to the pathological process of AD. Similar to the expression of MRGs, it is imperative to highlight that several immune cells—including B lineage, monocytic lineage, neutrophils, and T cells—exhibited significant intersubtype differences. The infiltration level was highest in cluster 3, followed by cluster 2, and lowest in cluster 1. Furthermore, the proportion of myeloid dendritic cells was significantly lower in cluster 1 than in cluster 2 and cluster 3. The exact role of B cells in AD requires further elucidation. Although B cells can produce immunoglobulins that slow the progression of AD, B cells in the brain may produce pro-inflammatory cytokines that promote AD-associated neuroinflammation and disease progression. Additionally, animal models have shown that B cell activation and infiltration in the brain are associated with AD, and therapeutic depletion of B cells can reverse AD progression (Kim et al., 2021). Neutrophils have been reported to influence AD progression by promoting Aβ pathology and cognitive impairment. The removal or inhibition of neutrophils in AD mouse models can significantly improve cognitive function, reduce Aβ plaque burden and neuronal damage, inhibit neuroinflammation, and restore cerebral blood flow and blood–brain barrier integrity. These results suggest that neutrophils are involved in the promotion of AD and are positively correlated with disease severity (Zenaro et al., 2015; Dong et al., 2018; Stock et al., 2018). The role of T cells in AD remains unclear; however, animal experiments have revealed that cerebral amyloidosis promotes T cell infiltration (Ferretti et al., 2016). These findings are consistent with our research results, which indicated that infiltration levels of these cells are higher in patients with AD with lower levels of mitochondrial autophagy.
According to the results of GO and KEGG enrichment analysis, the three subtypes of AD exhibit differences in specific biological processes and pathways. In terms of biological processes, the three subtypes show distinct functional characteristics in terms of cell growth, synaptic tissue, and neuron projection. KEGG analysis revealed that cluster 2 is enriched in COVID-19, epithelial cell signaling in H. pylori infection, focal adhesion, and synaptic vesicle cycle. SARS-CoV-2—the causative agent of COVID-19—has the ability to attack the central nervous system (Desforges et al., 2019) and may accelerate brain aging and promote the development of neurodegenerative diseases (Ciaccio et al., 2021). Although a definitive correlation between SARS-CoV-2 infection and susceptibility to AD does not exist, the risk of AD was reported to be significantly increased in older adults with COVID-19 (Wang et al., 2022). Our results further support the potential link between AD and COVID-19. “Epithelial cell signaling in H. pylori infection” denotes the cellular signal transduction pathway activated by H. pylori infection, which is associated with chronic gastritis and gastric cancer (Naumann and Crabtree, 2004). Notably, a link between H. pylori infection and AD has been reported, with some studies suggesting that H. pylori infection may be implicated in the pathophysiology of AD (Doulberis et al., 2018). Accordingly, the enrichment of signaling pathways related to H. pylori-infected epithelial cells in Cluster 2 suggests their potential involvement in AD pathogenesis. Focal adhesions are a type of cell–extracellular matrix adhesion structure that plays a key role in biological processes such as cell movement, proliferation, differentiation, and signal transduction (Stupack and Cheresh, 2002; Mitra et al., 2005). Additionally, focal adhesions can regulate Aβ signal transduction and cell death in cases of AD (Caltagarone et al., 2007). The synaptic vesicle cycle refers to the process by which presynaptic cells store, release, and reuptake neurotransmitters in synaptic vesicles. This process is essential to ensure the release of neurotransmitters and to maintain synaptic plasticity (Sudhof, 1995); furthermore, it has been a popular area of research related to neurodegenerative diseases (Wang et al., 2017). The tight junction is a pathway enriched in Cluster 1 that critically influences the maintenance of the integrity of the blood–brain barrier (Liu et al., 2012). AD can reportedly affect the blood–brain barrier function in in vitro experiments by altering the expression and localization of tight junction proteins (Desai et al., 2007). Impaired blood–brain barrier function in patients with AD may affect the clearance of Aβ, thereby accelerating AD progression (Algotsson and Winblad, 2007). The Wnt signaling pathway is a unique pathway enriched in cluster 3 that plays a crucial role in regulating adult brain structure and function. Downregulation of Aβ-induced Wnt signaling is associated with disease progression in AD, and activation of Wnt signaling can mitigate Aβ neurotoxicity and protect neurons. Therefore, the Wnt signaling pathway may be an important target for designing therapeutic strategies for AD in the future (Inestrosa and Varela-Nallar, 2014; Wan et al., 2014; Tapia-Rojas and Inestrosa, 2018).
Weighted gene co-expression network analysis helped in the identification of hub genes associated with each subtype and inputted the differentially expressed hub genes into the cMap database to screen for small-molecule drugs. LY-278584 may be a potential small molecule drug for cluster 1, Cetraxate for cluster 2, and Embelin for cluster 3. LY-278584 is a type of 5-HT3 receptor antagonist, and the 5-HT3 receptor is a neurotransmitter-gated ion channel located on the cell membrane that is highly expressed in the entorhinal cortex, hippocampus CA1 area, amygdala, substantia nigra, and brainstem. Antagonists of the 5-HT3 receptor are commonly used to treat nausea and vomiting and have also been found to have therapeutic effects on psychiatric disorders such as epilepsy, schizophrenia, and anxiety (Thompson and Lummis, 2007; Zhao et al., 2018). Importantly, 5-HT3 receptor antagonists may also delay memory impairment and enhance cognitive function, making them potential drugs for improving memory impairment in patients with AD (Fakhfouri et al., 2019). Cetraxate is a mucosal protective agent that can inhibit the activity of H. pylori and is commonly used as an anti-ulcer and anti-H. pylori drug (Kamada et al., 2000; Wu et al., 2004). Although there are currently no reports of cetraxate being used to treat AD, H. pylori may play a negative role in the development of AD, as it may enter the brain by disrupting the blood–brain barrier through circulating mononuclear cells and cause neurodegeneration (Doulberis et al., 2018). Further research is needed to determine whether cetraxate can be used to treat AD. Embelin is a natural product that can inhibit the aggregation of amyloid proteins and reduce inflammation. In addition, it can cross the blood–brain barrier and has antioxidant properties, which can prevent neuronal oxidative damage by reducing lipid peroxidation, thereby highlighting its potential as a therapeutic drug for AD (Nuthakki et al., 2019; Arora et al., 2023). Furthermore, embelin has been reported to be a potential drug for treating COVID-19 (Singh et al., 2021).
5. Conclusion
This study provides new evidence linking changes in MRGs expression levels to AD development and progression. The outcomes of the present study demonstrated that the overall expression of MRGs is downregulated in patients with AD, and six genes—i.e., FUNDC1, MAP1LC3A, CSNK2A1, VDAC1, CSNK2B, and ATG5—were identified that can be used to construct an AD prediction model with high predictive ability across different datasets. Furthermore, AD was classified into three subtypes based on MRGs expression profiles and the differences in gene expression, clinical features, immune infiltration, and pathway enrichment were analyzed among these subtypes. Despite our study is subject to limitations including relatively small sample sizes and a lack of experimental validation, the high heterogeneity of AD may lead to mitochondrial autophagy not occurring in all patients. Nonetheless, the results of the current study provide novel insights into the mechanisms underlying AD and emphasize the potential utility of MRGs in the diagnosis of and personalized treatment for AD.
Data availability statement
The original contributions presented in the study are included in the article/Supplementary material; further inquiries can be directed to the corresponding authors.
Author contributions
XG and CL conceived and designed the study. WM, YS, and PZ were responsible for the collection and assembly of data, data analysis, and interpretation. WM and YS were involved in the writing of the manuscript. GW, XC, and XG provided help in revising the manuscript. All authors contributed to the article and approved the submitted version.
Funding
This work was supported by the National Natural Science Foundation of China (81772829 and 81830052), the Special Program for Collaborative Innovation, the Construction project of Shanghai Key Laboratory of Molecular Imaging (18DZ2260400) and “Top-100 Talent Cultivation Plan” of Shanghai University of Medicine and Health Sciences, and Funding Scheme for Training Young Teachers in Shanghai Colleges.
Conflict of interest
The authors declare that the research was conducted in the absence of any commercial or financial relationships that could be construed as a potential conflict of interest.
Publisher’s note
All claims expressed in this article are solely those of the authors and do not necessarily represent those of their affiliated organizations, or those of the publisher, the editors and the reviewers. Any product that may be evaluated in this article, or claim that may be made by its manufacturer, is not guaranteed or endorsed by the publisher.
Supplementary material
The Supplementary material for this article can be found online at: https://www.frontiersin.org/articles/10.3389/fnmol.2023.1205541/full#supplementary-material
Footnotes
References
Algotsson, A., and Winblad, B. (2007). The integrity of the blood-brain barrier in Alzheimer's disease. Acta Neurol. Scand. 115, 403–408. doi: 10.1111/j.1600-0404.2007.00823.x
Arora, R. A., Virendra, S. A., and Chawla, P. A. (2023). Mechanistic study on the possible role of embelin in treating neurodegenerative disorders. CNS Neurol. Disord. Drug Targets
Armstrong, R. A. (2013). What causes alzheimer's disease? Folia Neuropathol. 51, 169–188. doi: 10.5114/fn.2013.37702
Borgo, C., D'Amore, C., Sarno, S., Salvi, M., and Ruzzene, M. (2021). Protein kinase CK2: a potential therapeutic target for diverse human diseases. Signal Transduct. Target. Ther. 6:183. doi: 10.1038/s41392-021-00567-7
Caltagarone, J., Jing, Z., and Bowser, R. (2007). Focal adhesions regulate Abeta signaling and cell death in Alzheimer's disease. Biochim. Biophys. Acta 1772, 438–445. doi: 10.1016/j.bbadis.2006.11.007
Cen, X., Chen, Y., Xu, X., Wu, R., He, F., Zhao, Q., et al. (2020). Pharmacological targeting of MCL-1 promotes mitophagy and improves disease pathologies in an Alzheimer's disease mouse model. Nat. Commun. 11:5731. doi: 10.1038/s41467-020-19547-6
Cen, X., Xu, X., and Xia, H. (2021). Targeting MCL1 to induce mitophagy is a potential therapeutic strategy for Alzheimer disease. Autophagy 17, 818–819. doi: 10.1080/15548627.2020.1860542
Chai, Y. L., Xing, H., Chong, J. R., Francis, P. T., Ballard, C. G., Chen, C. P., et al. (2018). Mitochondrial translocase of the outer membrane alterations may underlie dysfunctional oxidative phosphorylation in Alzheimer's disease. J. Alzheimers Dis. 61, 793–801. doi: 10.3233/JAD-170613
Chen, S., Chang, Y., Li, L., Acosta, D., Li, Y., Guo, Q., et al. (2022). Spatially resolved transcriptomics reveals genes associated with the vulnerability of middle temporal gyrus in Alzheimer's disease. Acta Neuropathol. Commun. 10:188. doi: 10.1186/s40478-022-01494-6
Chen, M., Chen, Z., Wang, Y., Tan, Z., Zhu, C., Li, Y., et al. (2016). Mitophagy receptor FUNDC1 regulates mitochondrial dynamics and mitophagy. Autophagy 12, 689–702. doi: 10.1080/15548627.2016.1151580
Chen, C., Yang, C., Wang, J., Huang, X., Yu, H., Li, S., et al. (2021). Melatonin ameliorates cognitive deficits through improving mitophagy in a mouse model of Alzheimer's disease. J. Pineal Res. 71:e12774. doi: 10.1111/jpi.12774
Cherra, S. J. 3rd, Kulich, S. M., Uechi, G., Balasubramani, M., Mountzouris, J., Day, B. W., et al. (2010). Regulation of the autophagy protein LC3 by phosphorylation. J. Cell Biol. 190, 533–539. doi: 10.1083/jcb.201002108
Chu, C. T. (2019). Mechanisms of selective autophagy and mitophagy: implications for neurodegenerative diseases. Neurobiol. Dis. 122, 23–34. doi: 10.1016/j.nbd.2018.07.015
Ciaccio, M., Lo Sasso, B., Scazzone, C., Gambino, C. M., Ciaccio, A. M., Bivona, G., et al. (2021). COVID-19 and Alzheimer's disease. Brain Sci. 11:305. doi: 10.3390/brainsci11030305
Codogno, P., and Meijer, A. J. (2006). Atg 5: more than an autophagy factor. Nat. Cell Biol. 8, 1045–1047. doi: 10.1038/ncb1006-1045
Cuadrado-Tejedor, M., Vilarino, M., Cabodevilla, F., Del Rio, J., Frechilla, D., and Perez-Mediavilla, A. (2011). Enhanced expression of the voltage-dependent anion channel 1 (VDAC1) in Alzheimer's disease transgenic mice: an insight into the pathogenic effects of amyloid-beta. J. Alzheimers Dis. 23, 195–206. doi: 10.3233/JAD-2010-100966
De Gaetano, A., Gibellini, L., Zanini, G., Nasi, M., Cossarizza, A., and Pinti, M. (2021). Mitophagy and oxidative stress: the role of aging. Antioxidants 10:794. doi: 10.3390/antiox10050794
Desai, B. S., Monahan, A. J., Carvey, P. M., and Hendey, B. (2007). Blood-brain barrier pathology in Alzheimer's and Parkinson's disease: implications for drug therapy. Cell Transplant. 16, 285–299. doi: 10.3727/000000007783464731
Desforges, M., Le Coupanec, A., Dubeau, P., Bourgouin, A., Lajoie, L., Dube, M., et al. (2019). Human coronaviruses and other respiratory viruses: underestimated opportunistic pathogens of the central nervous system? Viruses 12:14. doi: 10.3390/v12010014
Dong, Y., Lagarde, J., Xicota, L., Corne, H., Chantran, Y., Chaigneau, T., et al. (2018). Neutrophil hyperactivation correlates with Alzheimer's disease progression. Ann. Neurol. 83, 387–405. doi: 10.1002/ana.25159
Doulberis, M., Kotronis, G., Thomann, R., Polyzos, S. A., Boziki, M., Gialamprinou, D., et al. (2018). Review: impact of Helicobacter pylori on Alzheimer's disease: what do we know so far? Helicobacter 23:12454. doi: 10.1111/hel.12454
Fakhfouri, G., Rahimian, R., Dyhrfjeld-Johnsen, J., Zirak, M. R., and Beaulieu, J. M. (2019). 5-HT (3) receptor antagonists in neurologic and neuropsychiatric disorders: the iceberg still lies beneath the surface. Pharmacol. Rev. 71, 383–412. doi: 10.1124/pr.118.015487
Fang, E. F., Hou, Y., Palikaras, K., Adriaanse, B. A., Kerr, J. S., Yang, B., et al. (2019). Mitophagy inhibits amyloid-beta and tau pathology and reverses cognitive deficits in models of Alzheimer's disease. Nat. Neurosci. 22, 401–412. doi: 10.1038/s41593-018-0332-9
Ferretti, M. T., Merlini, M., Spani, C., Gericke, C., Schweizer, N., Enzmann, G., et al. (2016). T-cell brain infiltration and immature antigen-presenting cells in transgenic models of Alzheimer's disease-like cerebral amyloidosis. Brain Behav. Immun. 54, 211–225. doi: 10.1016/j.bbi.2016.02.009
Gu, X., Lai, D., Liu, S., Chen, K., Zhang, P., Chen, B., et al. (2022). Hub genes, diagnostic model, and predicted drugs related to Iron metabolism in Alzheimer's disease. Front. Aging Neurosci. 14:949083. doi: 10.3389/fnagi.2022.949083
Heppner, F. L., Ransohoff, R. M., and Becher, B. (2015). Immune attack: the role of inflammation in Alzheimer disease. Nat. Rev. Neurosci. 16, 358–372. doi: 10.1038/nrn3880
Hiller, S., Garces, R. G., Malia, T. J., Orekhov, V. Y., Colombini, M., and Wagner, G. (2008). Solution structure of the integral human membrane protein VDAC-1 in detergent micelles. Science 321, 1206–1210. doi: 10.1126/science.1161302
Hou, Y., Song, H., Croteau, D. L., Akbari, M., and Bohr, V. A. (2017). Genome instability in Alzheimer disease. Mech. Ageing Dev. 161, 83–94. doi: 10.1016/j.mad.2016.04.005
Inestrosa, N. C., and Varela-Nallar, L. (2014). Wnt signaling in the nervous system and in Alzheimer's disease. J. Mol. Cell Biol. 6, 64–74. doi: 10.1093/jmcb/mjt051
Iriondo, M. N., Etxaniz, A., Varela, Y. R., Ballesteros, U., Hervas, J. H., Montes, L. R., et al. (2022). LC3 subfamily in cardiolipin-mediated mitophagy: a comparison of the LC3A, LC3B and LC3C homologs. Autophagy 18, 2985–3003. doi: 10.1080/15548627.2022.2062111
Jetto, C. T., Nambiar, A., and Manjithaya, R. (2022). Mitophagy and neurodegeneration: between the knowns and the unknowns. Front. Cell Dev. Biol. 10:837337. doi: 10.3389/fcell.2022.837337
Kamada, T., Haruma, K., Miyoshi, E., Mihara, M., Kitadai, Y., Yoshihara, M., et al. (2000). Cetraxate, a mucosal protective agent, combined with omeprazole, amoxycillin, and clarithromycin increases the eradication rate of helicobacter pylori in smokers. Aliment. Pharmacol. Ther. 14, 1089–1094. doi: 10.1046/j.1365-2036.2000.00807.x
Kerr, J. S., Adriaanse, B. A., Greig, N. H., Mattson, M. P., Cader, M. Z., Bohr, V. A., et al. (2017). Mitophagy and Alzheimer's disease: cellular and molecular mechanisms. Trends Neurosci. 40, 151–166. doi: 10.1016/j.tins.2017.01.002
Kim, I., Rodriguez-Enriquez, S., and Lemasters, J. J. (2007). Selective degradation of mitochondria by mitophagy. Arch. Biochem. Biophys. 462, 245–253. doi: 10.1016/j.abb.2007.03.034
Kim, K., Wang, X., Ragonnaud, E., Bodogai, M., Illouz, T., DeLuca, M., et al. (2021). Therapeutic B-cell depletion reverses progression of Alzheimer's disease. Nat. Commun. 12:2185. doi: 10.1038/s41467-021-22479-4
Lai, D., Tan, L., Zuo, X., Liu, D., Jiao, D., Wan, G., et al. (2021). Prognostic Ferroptosis-related lnc RNA signatures associated with immunotherapy and chemotherapy responses in patients with stomach Cancer. Front. Genet. 12:798612. doi: 10.3389/fgene.2021.798612
Lane, C. A., Hardy, J., and Schott, J. M. (2018). Alzheimer's disease. Eur. J. Neurol. 25, 59–70. doi: 10.1111/ene.13439
Leuner, K., Schutt, T., Kurz, C., Eckert, S. H., Schiller, C., Occhipinti, A., et al. (2012). Mitochondrion-derived reactive oxygen species lead to enhanced amyloid beta formation. Antioxid. Redox Signal. 16, 1421–1433. doi: 10.1089/ars.2011.4173
Li, L., Zhang, X., and Le, W. (2010). Autophagy dysfunction in Alzheimer's disease. Neurodegener. Dis. 7, 265–271. doi: 10.1159/000276710
Liang, J., Wang, C., Zhang, H., Huang, J., Xie, J., and Chen, N. (2021). Exercise-induced benefits for Alzheimer's disease by stimulating Mitophagy and improving mitochondrial function. Front. Aging Neurosci. 13:755665. doi: 10.3389/fnagi.2021.755665
Liu, W. Y., Wang, Z. B., Zhang, L. C., Wei, X., and Li, L. (2012). Tight junction in blood-brain barrier: an overview of structure, regulation, and regulator substances. CNS Neurosci. Ther. 18, 609–615. doi: 10.1111/j.1755-5949.2012.00340.x
Liu, H., Zang, C., Yuan, F., Ju, C., Shang, M., Ning, J., et al. (2022). The role of FUNDC1 in mitophagy, mitochondrial dynamics and human diseases. Biochem. Pharmacol. 197:114891. doi: 10.1016/j.bcp.2021.114891
Manczak, M., Anekonda, T. S., Henson, E., Park, B. S., Quinn, J., and Reddy, P. H. (2006). Mitochondria are a direct site of a beta accumulation in Alzheimer's disease neurons: implications for free radical generation and oxidative damage in disease progression. Hum. Mol. Genet. 15, 1437–1449. doi: 10.1093/hmg/ddl066
Manczak, M., and Reddy, P. H. (2012). Abnormal interaction of VDAC1 with amyloid beta and phosphorylated tau causes mitochondrial dysfunction in Alzheimer's disease. Hum. Mol. Genet. 21, 5131–5146. doi: 10.1093/hmg/dds360
Marin, R., Ramirez, C. M., Gonzalez, M., Gonzalez-Munoz, E., Zorzano, A., Camps, M., et al. (2007). Voltage-dependent anion channel (VDAC) participates in amyloid beta-induced toxicity and interacts with plasma membrane estrogen receptor alpha in septal and hippocampal neurons. Mol. Membr. Biol. 24, 148–160. doi: 10.1080/09687860601055559
Martin-Maestro, P., Gargini, R., Sproul, A. A., Garcia, E., Anton, L. C., Noggle, S., et al. (2017). Mitophagy failure in fibroblasts and iPSC-derived neurons of Alzheimer's disease-associated Presenilin 1 mutation. Front. Mol. Neurosci. 10:291. doi: 10.3389/fnmol.2017.00291
Mitra, S. K., Hanson, D. A., and Schlaepfer, D. D. (2005). Focal adhesion kinase: in command and control of cell motility. Nat. Rev. Mol. Cell Biol. 6, 56–68. doi: 10.1038/nrm1549
Moreira, P. I., Carvalho, C., Zhu, X., Smith, M. A., and Perry, G. (2010). Mitochondrial dysfunction is a trigger of Alzheimer's disease pathophysiology. Biochim. Biophys. Acta 1802, 2–10. doi: 10.1016/j.bbadis.2009.10.006
Naumann, M., and Crabtree, J. E. (2004). Helicobacter pylori-induced epithelial cell signalling in gastric carcinogenesis. Trends Microbiol. 12, 29–36. doi: 10.1016/j.tim.2003.11.005
Nuthakki, V. K., Sharma, A., Kumar, A., and Bharate, S. B. (2019). Identification of embelin, a 3-undecyl-1,4-benzoquinone from Embelia ribes as a multitargeted anti-Alzheimer agent. Drug Dev. Res. 80, 655–665. doi: 10.1002/ddr.21544
Ray, M., and Zhang, W. (2010). Analysis of Alzheimer's disease severity across brain regions by topological analysis of gene co-expression networks. BMC Syst. Biol. 4:136. doi: 10.1186/1752-0509-4-136
Reddy, P. H. (2013). Amyloid beta-induced glycogen synthase kinase 3beta phosphorylated VDAC1 in Alzheimer's disease: implications for synaptic dysfunction and neuronal damage. Biochim. Biophys. Acta 1832, 1913–1921. doi: 10.1016/j.bbadis.2013.06.012
Singh, R. S., Singh, A., Kaur, H., Batra, G., Sarma, P., Kaur, H., et al. (2021). Promising traditional Indian medicinal plants for the management of novel coronavirus disease: a systematic review. Phytother. Res. 35, 4456–4484. doi: 10.1002/ptr.7150
Smilansky, A., Dangoor, L., Nakdimon, I., Ben-Hail, D., Mizrachi, D., and Shoshan-Barmatz, V. (2015). The voltage-dependent Anion Channel 1 mediates amyloid beta toxicity and represents a potential target for Alzheimer disease therapy. J. Biol. Chem. 290, 30670–30683. doi: 10.1074/jbc.M115.691493
Smith, M. A., Rottkamp, C. A., Nunomura, A., Raina, A. K., and Perry, G. (2000). Oxidative stress in Alzheimer's disease. Biochim. Biophys. Acta 1502, 139–144. doi: 10.1016/S0925-4439(00)00040-5
Song, M., Zhao, X., and Song, F. (2021). Aging-dependent Mitophagy dysfunction in Alzheimer's disease. Mol. Neurobiol. 58, 2362–2378. doi: 10.1007/s12035-020-02248-y
Stock, A. J., Kasus-Jacobi, A., and Pereira, H. A. (2018). The role of neutrophil granule proteins in neuroinflammation and Alzheimer's disease. J. Neuroinflammation 15:240. doi: 10.1186/s12974-018-1284-4
Stupack, D. G., and Cheresh, D. A. (2002). Get a ligand, get a life: integrins, signaling and cell survival. J. Cell Sci. 115, 3729–3738. doi: 10.1242/jcs.00071
Sudhof, T. C. (1995). The synaptic vesicle cycle: a cascade of protein-protein interactions. Nature 375, 645–653. doi: 10.1038/375645a0
Tapia-Rojas, C., and Inestrosa, N. C. (2018). Loss of canonical Wnt signaling is involved in the pathogenesis of Alzheimer's disease. Neural Regen. Res. 13, 1705–1710. doi: 10.4103/1673-5374.238606
Thompson, A. J., and Lummis, S. C. (2007). The 5-HT3 receptor as a therapeutic target. Expert Opin. Ther. Targets 11, 527–540. doi: 10.1517/14728222.11.4.527
Walczak, M., and Martens, S. (2013). Dissecting the role of the Atg 12-Atg5-Atg16 complex during autophagosome formation. Autophagy 9, 424–425. doi: 10.4161/auto.22931
Wan, W., Xia, S., Kalionis, B., Liu, L., and Li, Y. (2014). The role of Wnt signaling in the development of Alzheimer's disease: a potential therapeutic target? Biomed. Res. Int. 2014:301575. doi: 10.1155/2014/301575
Wang, L., Davis, P. B., Volkow, N. D., Berger, N. A., Kaelber, D. C., and Xu, R. (2022). Association of COVID-19 with new-onset Alzheimer's disease. J. Alzheimers Dis. 89, 411–414. doi: 10.3233/JAD-220717
Wang, H., Fu, J., Xu, X., Yang, Z., and Zhang, T. (2021). Rapamycin activates Mitophagy and alleviates cognitive and synaptic plasticity deficits in a mouse model of Alzheimer's disease. J. Gerontol. A Biol. Sci. Med. Sci. 76, 1707–1713. doi: 10.1093/gerona/glab142
Wang, Q., Guan, Z., Qi, L., Zhuang, J., Wang, C., Hong, S., et al. (2021). Structural insight into the SAM-mediated assembly of the mitochondrial TOM core complex. Science 373, 1377–1381. doi: 10.1126/science.abh0704
Wang, W., Zhao, F., Ma, X., Perry, G., and Zhu, X. (2020). Mitochondria dysfunction in the pathogenesis of Alzheimer's disease: recent advances. Mol. Neurodegener. 15:30. doi: 10.1186/s13024-020-00376-6
Wang, C., Zhao, D., Shah, S. Z. A., Yang, W., Li, C., and Yang, L. (2017). Proteome analysis of potential synaptic vesicle cycle biomarkers in the cerebrospinal fluid of patients with sporadic Creutzfeldt-Jakob disease. Mol. Neurobiol. 54, 5177–5191. doi: 10.1007/s12035-016-0029-6
Wu, C. J., Hsu, P. I., Lo, G. H., Lo, C. C., Lin, C. K., Shie, C. B., et al. (2004). Comparison of cetraxate-based and pantoprazole-based triple therapies in the treatment of Helicobacter pylori infection. J. Chin. Med. Assoc. 67, 161–167.
Xie, J., Cui, Y., Chen, X., Yu, H., Chen, J., Huang, T., et al. (2021). VDAC1 regulates mitophagy in NLRP3 inflammasome activation in retinal capillary endothelial cells under high-glucose conditions. Exp. Eye Res. 209:108640. doi: 10.1016/j.exer.2021.108640
Zenaro, E., Pietronigro, E., Della Bianca, V., Piacentino, G., Marongiu, L., Budui, S., et al. (2015). Neutrophils promote Alzheimer's disease-like pathology and cognitive decline via LFA-1 integrin. Nat. Med. 21, 880–886. doi: 10.1038/nm.3913
Zhang, Y., Lian, Y., Lian, X., Zhang, H., Chen, Y., Sheng, H., et al. (2023). FUNDC1 mediated Mitophagy in epileptic hippocampal neuronal injury induced by magnesium-free fluid. Neurochem. Res. 48, 284–294. doi: 10.1007/s11064-022-03749-z
Keywords: Alzheimer’s disease, mitochondrial autophagy, mitochondrial-related genes, biomarkers, prediction model, subtyping
Citation: Ma W, Su Y, Zhang P, Wan G, Cheng X, Lu C and Gu X (2023) Identification of mitochondrial-related genes as potential biomarkers for the subtyping and prediction of Alzheimer’s disease. Front. Mol. Neurosci. 16:1205541. doi: 10.3389/fnmol.2023.1205541
Edited by:
Guiyou Liu, Chinese Academy of Sciences (CAS), ChinaReviewed by:
Homira Behbahani, Karolinska Institutet (KI), SwedenChi Kwan Tsang, First Affiliated Hospital of Jinan University, China
Zhijie Han, Chongqing Medical University, China
Copyright © 2023 Ma, Su, Zhang, Wan, Cheng, Lu and Gu. This is an open-access article distributed under the terms of the Creative Commons Attribution License (CC BY). The use, distribution or reproduction in other forums is permitted, provided the original author(s) and the copyright owner(s) are credited and that the original publication in this journal is cited, in accordance with accepted academic practice. No use, distribution or reproduction is permitted which does not comply with these terms.
*Correspondence: Xuefeng Gu, Z3V4ZkBzdW1ocy5lZHUuY24=; Changlian Lu, bHZjbEBzdW1ocy5lZHUuY24=
†These authors share first authorship