- Department of Neurology, Cognitive Disorders Center, Huadong Hospital, Fudan University, Shanghai, China
Vascular dementia (VaD) is considered to be the second most common form of dementia after Alzheimer’s disease, and no specific drugs have been approved for VaD treatment. We aimed to identify shared transcriptomic signatures between the frontal cortex and temporal cortex in VaD by bioinformatics analyses. Gene ontology and pathway enrichment analyses, protein–protein interaction (PPI) and hub gene identification, hub gene–transcription factor interaction, hub gene–microRNA interaction, and hub gene–drug interaction analyses were performed. We identified 159 overlapping differentially expressed genes (DEGs) between the frontal cortex and temporal cortex that were enriched mainly in inflammation and innate immunity, synapse pruning, regeneration, positive regulation of angiogenesis, response to nutrient levels, and positive regulation of the digestive system process. We identified 10 hub genes in the PPI network (GNG13, CD163, C1QA, TLR2, SST, C1QB, ITGB2, CCR5, CRH, and TAC1), four central regulatory transcription factors (FOXC1, CREB1, GATA2, and HINFP), and four microRNAs (miR-27a-3p, miR-146a-5p, miR-335-5p, and miR-129-2-3p). Hub gene–drug interaction analysis found four drugs (maraviroc, cenicriviroc, PF-04634817, and efalizumab) that could be potential drugs for VaD treatment. Together, our results may contribute to understanding the underlying mechanisms in VaD and provide potential targets and drugs for therapeutic intervention.
Introduction
Vascular dementia (VaD) is a neurocognitive disorder that also has other characteristics such as behavioral symptoms, locomotor abnormalities, and autonomic dysfunction (O’Brien and Thomas, 2015). Various clinical and population studies have indicated that the prevalence of early-onset VaD (<65 years old) ranges from 3.1 to 44% (Vieira et al., 2013). VaD is considered to be the second most common form of dementia after Alzheimer’s disease (AD), posing a heavy burden on families and societies (Khan et al., 2016). However, no specific drugs have been approved for VaD treatment. Growing evidence indicates that stroke, hypertension, diabetes, and atherosclerosis are risk factors for VaD (Kalaria, 2018). A recent study found that the incidence of post-event dementia at 1 year was 34⋅4% in patients with severe stroke, 8⋅2% in patients with minor stroke, and 5⋅2% in patients with transient ischemic attack (Pendlebury and Rothwell, 2019). The underlying mechanism of VaD is still largely unknown but may be associated with pathological processes including chronic hypoperfusion and hypoxia (Tukacs et al., 2020), vascular endothelial dysfunction and damage (Wang et al., 2018), increased blood–brain barrier permeability (Ueno et al., 2019), and oxidative stress and inflammation (Guo et al., 2020; Wang et al., 2020). Therefore, there is a need to elucidate the detailed molecular mechanisms of VaD and explore potential drugs for VaD treatment.
Bioinformatics analysis of transcriptomic data is used widely to identify biomarkers, therapeutic targets, and the mechanisms of various diseases, including dementia (Ma et al., 2019). Key modules and hub genes related to AD have been identified (Rahman M. R. et al., 2020; Lee and Lee, 2021), yet VaD has received little attention. A vascular basis for neuronal atrophy in both the temporal and frontal lobes in VaD that is entirely independent of any Alzheimer’s pathology has been reported (Kalaria, 2016). Therefore, in this study, we analyzed transcriptome signatures of the temporal cortex and frontal cortex samples from a microarray dataset of VaD to identify overlapping differentially expressed genes (DEGs) between the two brain regions. Gene ontology and pathway enrichment analyses were conducted to predict the biological activities of the overlapping DEGs. Protein–protein interaction (PPI) analysis was performed to detect potential relationships among the proteins encoded by the overlapping DEGs. Hub genes and key modules were identified using Cytoscape software. We also performed hub gene–transcription factor (TF) and hub gene–microRNA (miRNA) interaction analyses to detect potential transcriptional and posttranscriptional regulatory factors, and hub gene–drug interaction analysis to identify candidate drugs. These inclusive systems biology processes allowed us to identify potential biomolecular signatures of VaD, which will give new insights into the underlying mechanisms and provide potential targets for therapeutic intervention.
Materials and Methods
A schematic diagram of the workflow of the bioinformatics analyses is shown in Figure 1.
Identification of DEGs From Microarray Datasets of the Frontal Cortex and Temporal Cortex Samples From Patients With VaD
We used the Gene Expression Omnibus (GEO) dataset, GSE122063, which contains gene expression profiles of the frontal cortex and temporal cortex samples from patients with AD, VaD, and matched controls (McKay et al., 2019). In the current study, we analyzed only the gene expression data of the frontal cortex and temporal cortex from VaD and matched control patients. The dataset is available from the National Center for Biotechnology Information (NCBI) GEO database1 (Barrett et al., 2013). To identify overlapping DEGs, we analyzed a subset comprising 18 temporal cortex and 18 frontal cortex samples of VaD and 22 matched control samples using the Limma package in the NCBI GEO2R online tool. The threshold values for significant DEGs were adjusted P-value < 0.05 and | log fold change (FC)| > 1. Volcano plots were used to visualize the DEGs in frontal cortex or temporal cortex versus the matched controls. A Venn diagram was used to identify the overlapping DEGs between the two brain regions (Bardou et al., 2014).
Gene Ontology and Pathway Enrichment Analyses
Gene ontology (GO) enrichment analysis under the three main GO categories, biological process, molecular function, and cellular component, and pathway enrichment analysis of the overlapping DEGs between the two brain regions were performed on the web-based portal Metascape2 (Zhou et al., 2019). The Kyoto Encyclopedia of Genes and Genomes (KEGG) (Kanehisa et al., 2017), Reactome (Jassal et al., 2020), and WikiPathways databases (Martens et al., 2021) were used for pathway annotation. The PANTHER database was applied to detect protein class over-representation of the proteins encoded by the overlapping DEGs (Mi et al., 2013). P-value < 0.01 was selected as the threshold for significant enrichment.
Protein–Protein Interaction Network Construction and Module Analysis
To detect the potential relationships among the proteins encoded by the overlapping DEGs, we used the Search Tool for the Retrieval of Interacting Genes (STRING) database, version 11.03 to construct a PPI network; a combined score of >0.7 was set as the cut-off criterion of statistical significance (Szklarczyk et al., 2017). Cytoscape 3.7.14 was used to visualize the PPI network (Shannon et al., 2003). Ten genes with the highest degree of connectivity were identified as hub genes using the cytoHubba plugin, and key modules were identified using the molecular complex detection (MCODE) plugin.
Hub Gene–Transcription Factor Interaction and Hub Gene–miRNA Interaction Analyses
Hub genes are considered to be key genes that play vital roles in the biological process of interest. Therefore, we performed hub gene–TF and hub gene–miRNA interaction analyses to detect TFs and miRNAs that may regulate the hub genes at transcriptional and posttranscriptional levels, respectively. The JASPAR (Khan et al., 2018) and TarBase v8 (Karagkouni et al., 2018) databases were searched to identify TFs and miRNAs based on topological parameters (i.e., degree and betweenness centrality), respectively, using the NetworkAnalyst software (Xia et al., 2015). In addition, motif analysis of DEGs was performed to discover these genes promoter sequence motifs via the MEME algorithm in the MEME Suite5 (Bailey et al., 2015). E-value < 0.01 was selected as the threshold for significant motifs. In order to see if any transcription factor identified above has enriched motifs on these genes, those discovered significant motifs were compared with motifs in JASPAR (NON-REDUNDANT) DNA (21 Databases) motif databases using the motif database scanning algorithm Tomtom (Gupta et al., 2007). The StarBase datasets6 (Li et al., 2014) presented the miRNA–target interactions by intersecting the predicting target sites of miRNAs with binding sites of Ago protein, which were derived from CLIP-seq data and users can search the interactions of miRNA-target by selecting one/multiple target-predicting programs (PITA, RNA22, miRmap, DIANA-microT, miRanda, PicTar, and TargetScan). Therefore we used it to predict microRNA binding sites of hub genes.
Drug–Gene Interaction Analysis
The Drug--Gene Interaction Database (DGIdb)7 was searched to identify potential drugs that could be used in the treatment of VaD. DGIdb is an online database that provides drug-gene interaction and gene druggability information from various sources, including the literature (PubMed and clinical trial databases) and several drug databases (DrugBank, PharmGKB, and ChEMBL) (Cotto et al., 2018). The 10 identified hub genes were imported into DGIdb as potential targets to search for existing drugs that could interact with them. Drugs that showed specific types of interactions with the hub genes were selected. Cytoscape software was applied to visualize the interactions between the selected drugs and the corresponding target genes. To identify related clinical trials, the drugs that showed specific types of interactions with the hub genes were input into the ClinicalTrials.gov registry,8 the largest clinical trials database which contains over 329,000 trials worldwide.
Results
Identification of DEGs
The differential expression analysis detected 128 upregulated and 183 downregulated genes in the frontal cortex (Figure 2A and Supplementary Table 1), and 131 upregulated and 165 downregulated genes in the temporal lobe cortex (Figure 2B and Supplementary Table 1) of VaD compared with the matched controls. A total of 159 overlapping DEGs, including 62 upregulated and 97 downregulated genes, were detected between the two brain regions (Figure 2C, Table 1, and Supplementary Table 1).
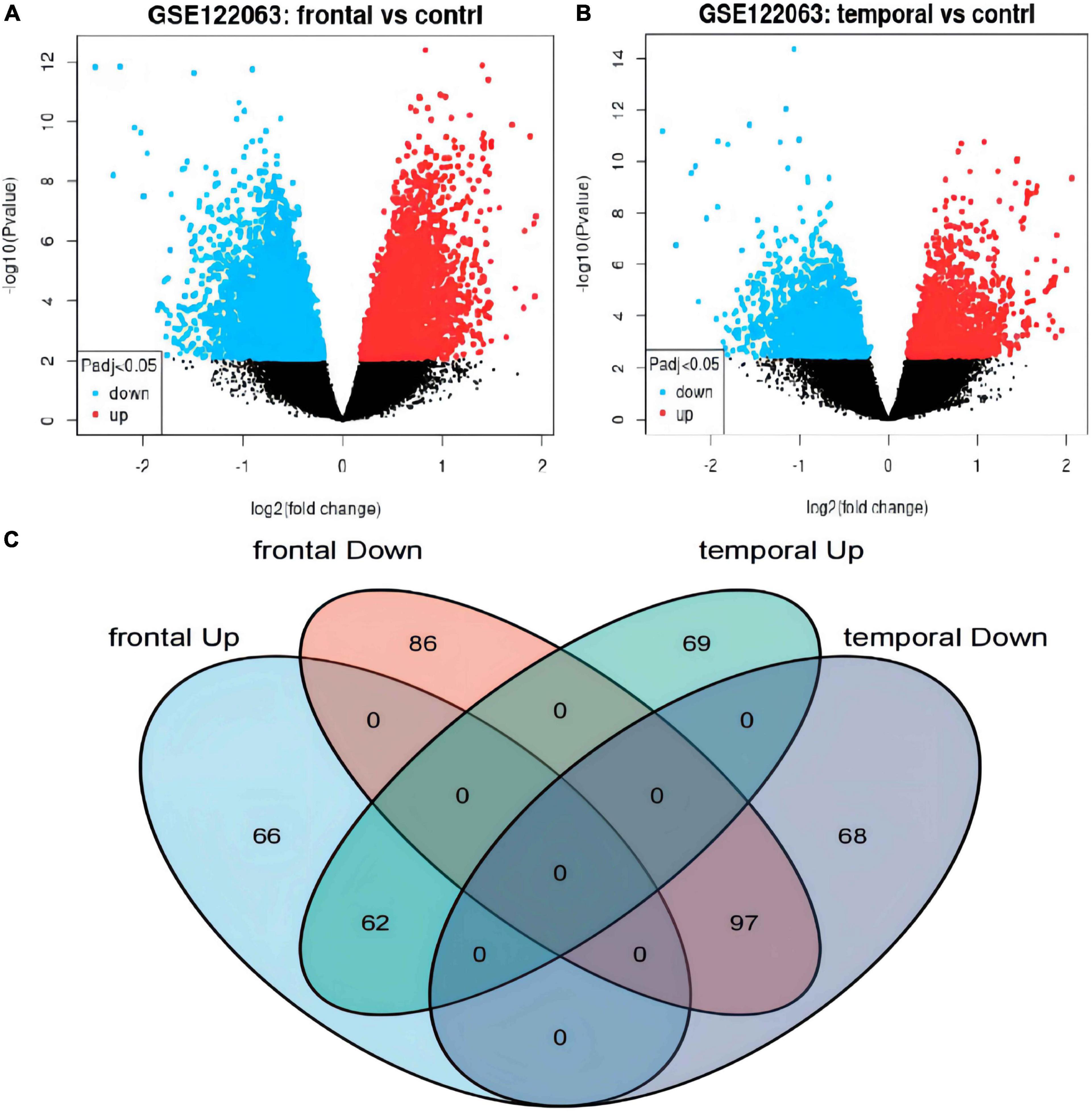
Figure 2. Identification of differentially expressed genes (DEGs) in frontal cortex and temporal cortex in the vascular dementia microarray dataset GSE122063. (A,B) Volcano plots of DEGs in frontal cortex or temporal cortex of vascular dementia versus the controls. Blue indicates downregulated genes; red indicates upregulated genes; gray indicates genes that were not significantly differentially expressed. (C) Venn diagram showing the numbers of overlapping DEGs between the two brain regions.
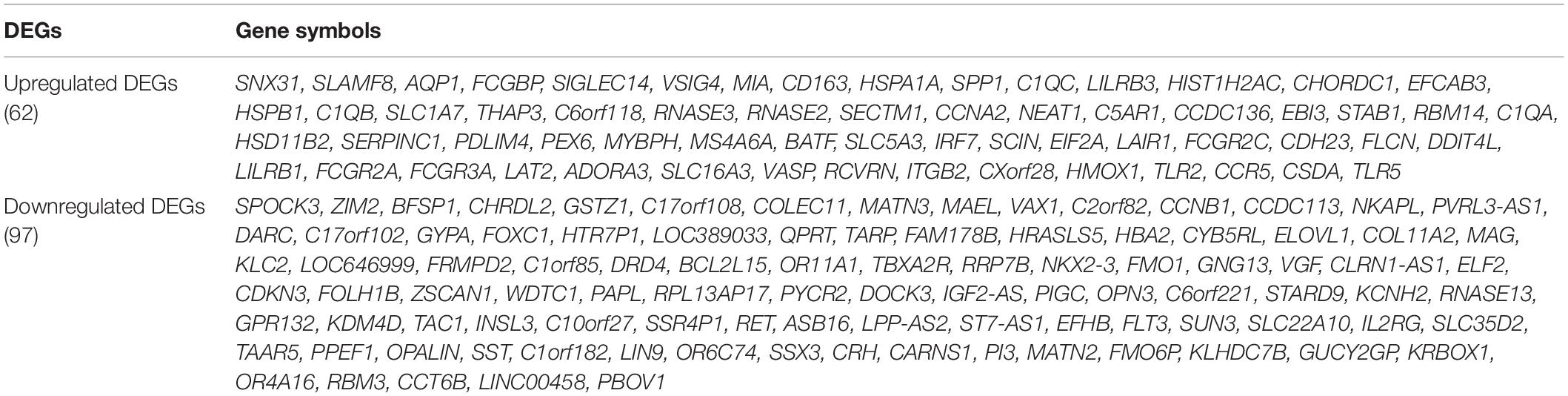
Table 1. Differentially expressed genes (DEGs) that overlap in the frontal cortex and temporal cortex of vascular dementia.
Functional Enrichment Analysis of the Overlapping DEGs
The protein class over-representation analysis of proteins encoded by the overlapping DEGs classified them into different groups according to their functions and activities (Figure 3). The results of the GO and signal pathways enrichment analyses of the overlapping DEGs are given in Tables 2, 3, respectively. The most significantly enriched terms were inflammation and innate immunity, synapse pruning, regeneration, positive regulation of angiogenesis, response to nutrient levels, and positive regulation of the digestive system process, which may help in understanding the DEGs involved in the pathogenesis of VaD. We also performed the gene ontology enrichment and pathway enrichment analysis of up-regulated genes and down-regulated genes separately, and the results were presented in the Supplementary Materials “GO analysis” sheet and “pathway analysis” sheet of Supplementary Table 4 respectively.
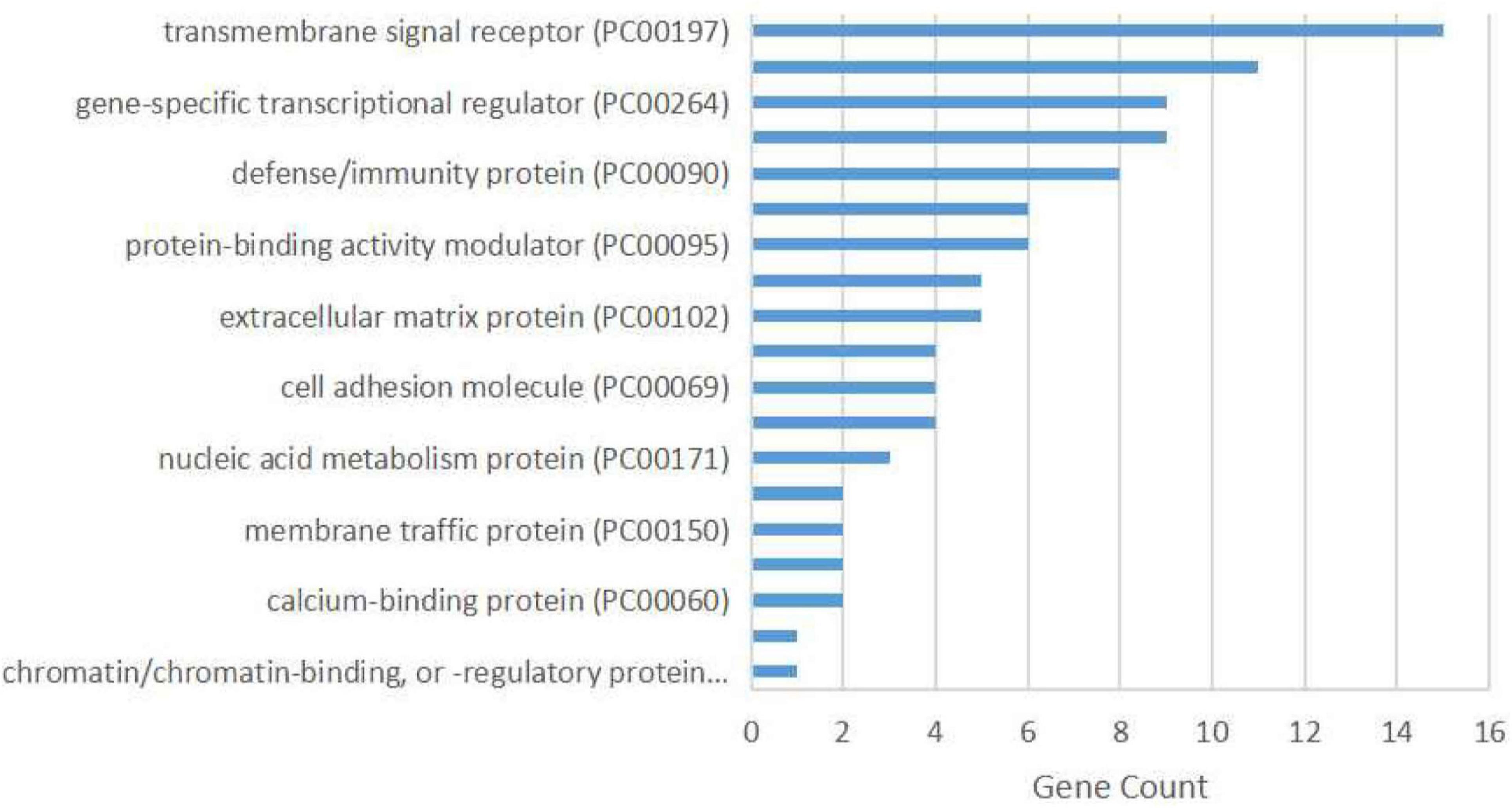
Figure 3. Protein class over-representation of proteins encoded by the overlapping differentially expressed genes identified using the PANTHER database.
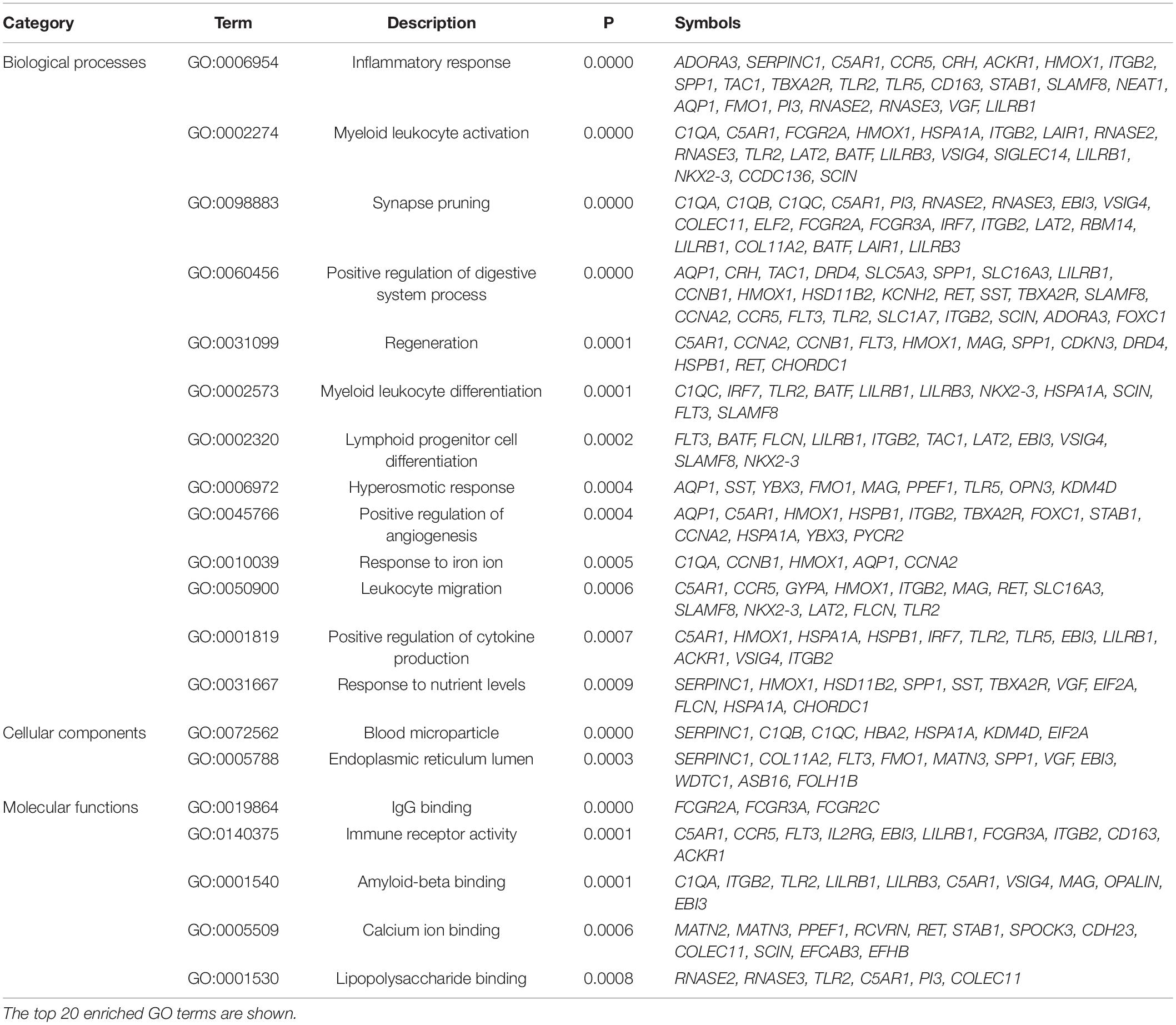
Table 2. Gene ontology (GO) enrichment analysis of the overlapping differentially expressed genes in the frontal cortex and temporal cortex in vascular dementia.
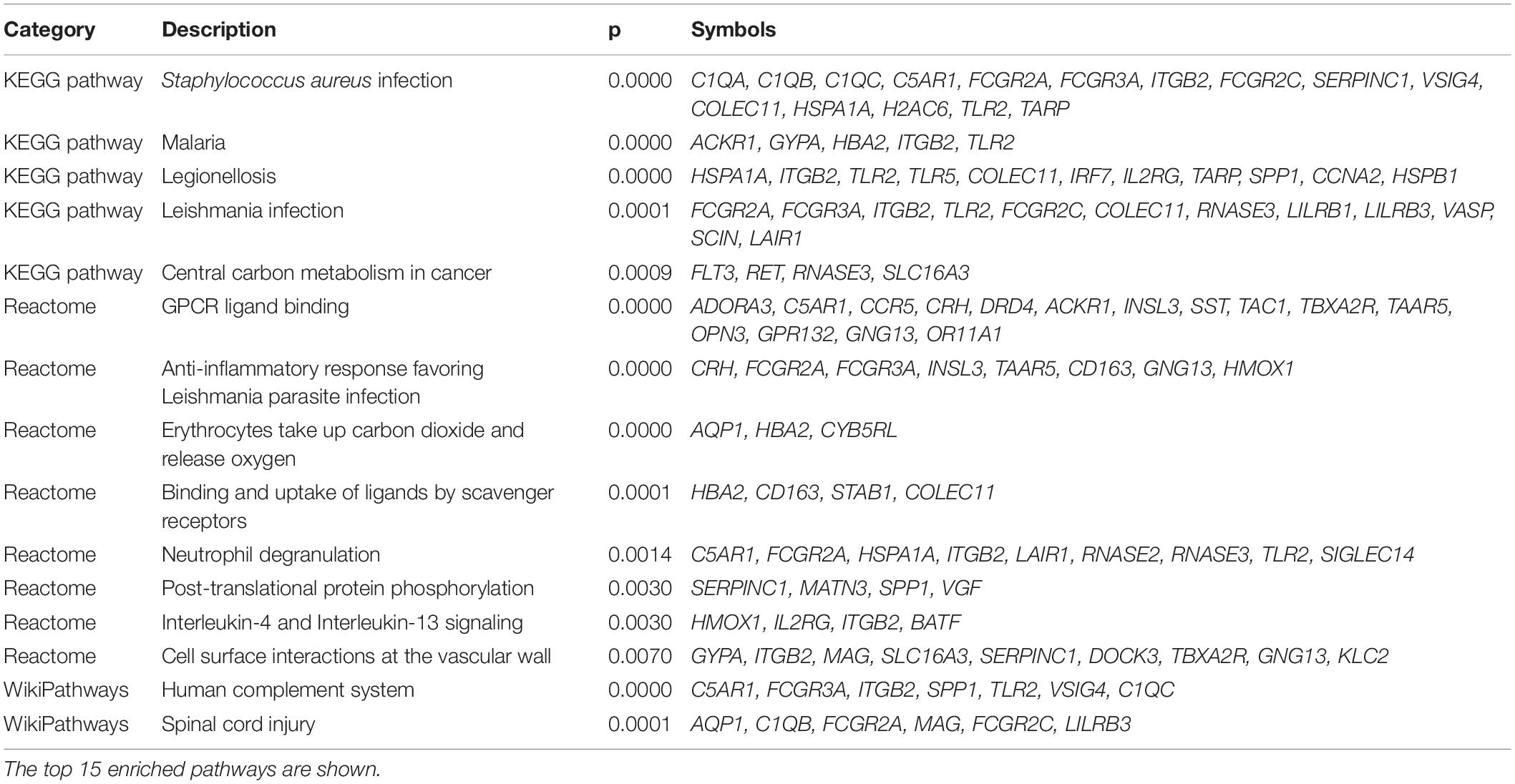
Table 3. Molecular pathway enrichment analysis of the overlapping differentially expressed genes in frontal cortex and temporal cortex in vascular dementia.
Protein–Protein Interaction Network Construction and Module Analysis
The PPI network of the 159 overlapping DEGs has 142 nodes and 88 edges (Figure 4 and Supplementary Table 2); disconnected nodes in the network are hidden. The average node degree of the PPI network was 1.16, and average local clustering coefficient was 0.268, and the PPI enrichment p-value was 1.82e-13. The topological analysis of the PPI network identified ten hub genes, GNG13, CD163, C1QA, TLR2, SST, C1QB, ITGB2, CCR5, CRH, and TAC1 (Figure 5A and Table 4). The MCODE analysis identified three sub-networks, implying these overlapping genes may be involved in different biological processes. The sub-network with the highest MCODE score (score = 5.2) is shown in Figure 5B.
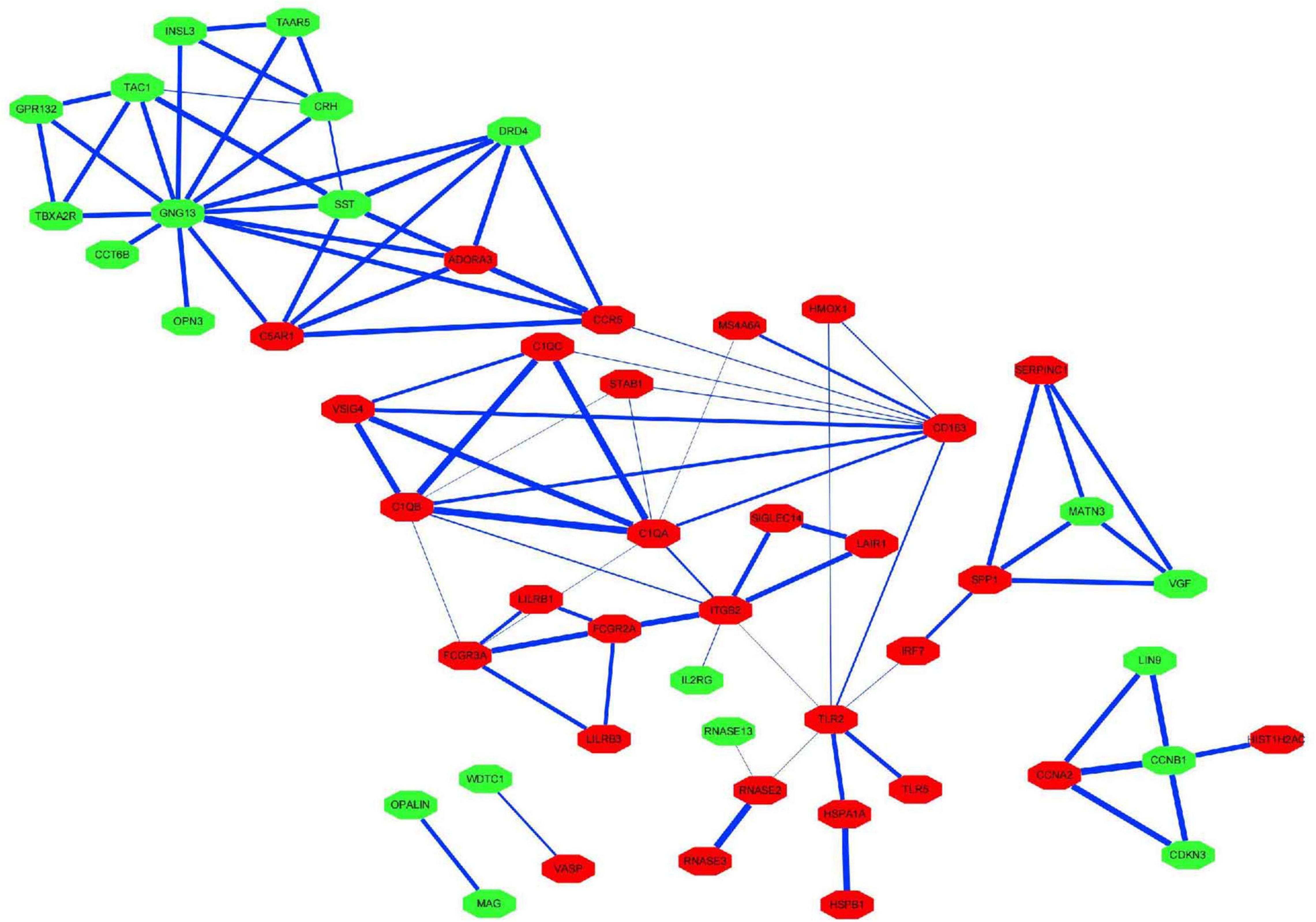
Figure 4. Protein–protein interaction network for the proteins encoded by the overlapping differentially expressed genes. The nodes represent the encoded proteins and the edges represent their interactions. Green indicates downregulated genes; red indicates upregulated genes. The width of the blue line indicates the combined score obtained using the STRING database.
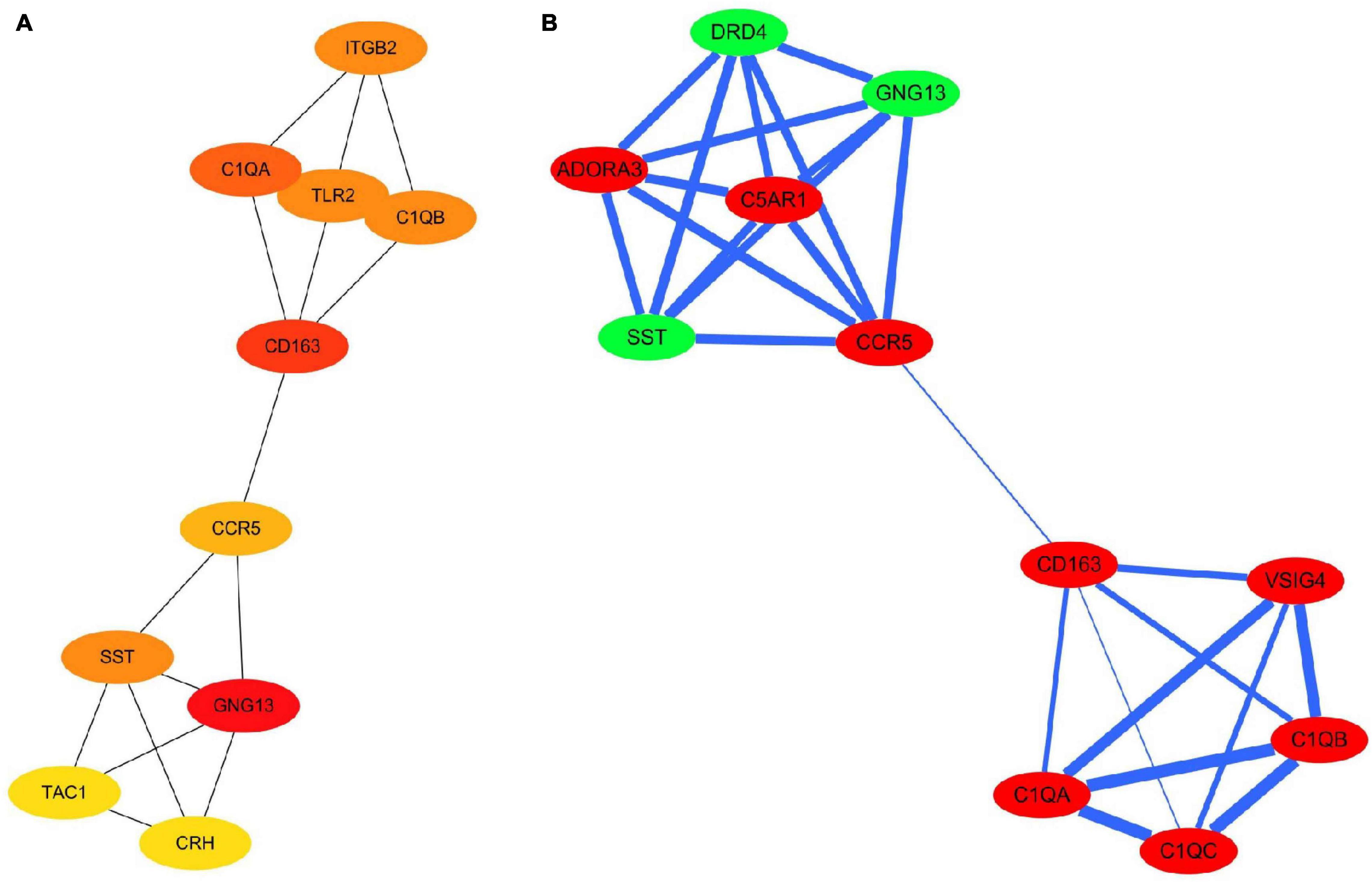
Figure 5. Hub genes and modules identified in the protein–protein interaction network visualized using Cytoscape software. (A) Top 10 hub genes with a higher degree of connectivity. (B) Sub-network with the highest MCODE score is shown. Green indicates downregulated genes; red indicates upregulated genes. The width of the blue line indicates the combined score obtained using the STRING database.
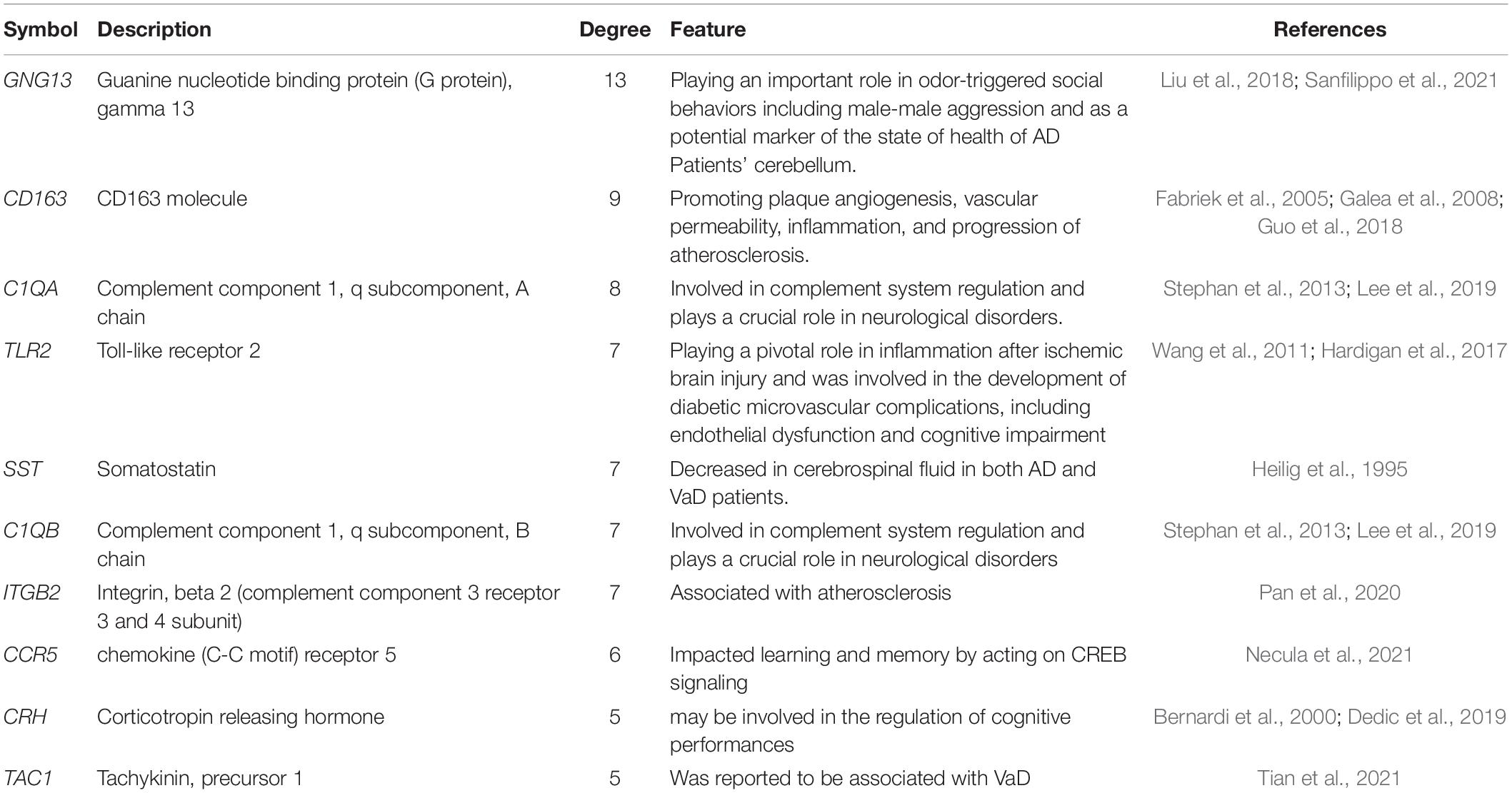
Table 4. Ten hub genes identified in the protein–protein interaction network of proteins encoded by the overlapping differentially expressed genes in vascular dementia.
Regulatory Signatures of VaD
We analyzed the hub gene–TF interactions (Figure 6) and hub gene–miRNA interactions (Figure 7) and identified central regulatory TFs and miRNAs using the topological parameters. Four TFs, FOXC1, CREB1, GATA2, and HINFP, and four miRNAs, miR-27a-3p, miR-146a-5p, miR-335-5p, and miR-129-2-3p were detected from the two interaction networks, respectively (Table 5). The results of common DEGs-TF and DEGs-miRNA analysis were provided in the Supplementary Figures 1, 2, respectively, and Supplementary Table 2. We performed motif analysis of DEGs and discovered 28 significant motifs (Figure 8). And these motifs were compared with motifs in JASPAR (NON-REDUNDANT) DNA (21 Databases) motif databases and several transcription factors identified above (GATA2, TFAP2A, GATA3, MEF2A, KLF5, ELK4, SP1, JUND, EGR1, E2F6, NR2F1, NR2C2, SOX10, and E2F4) were matched to these motifs (Supplementary Table 5). The results of the microRNA binding sites of these hub genes were provided in the Supplementary Table 6.”
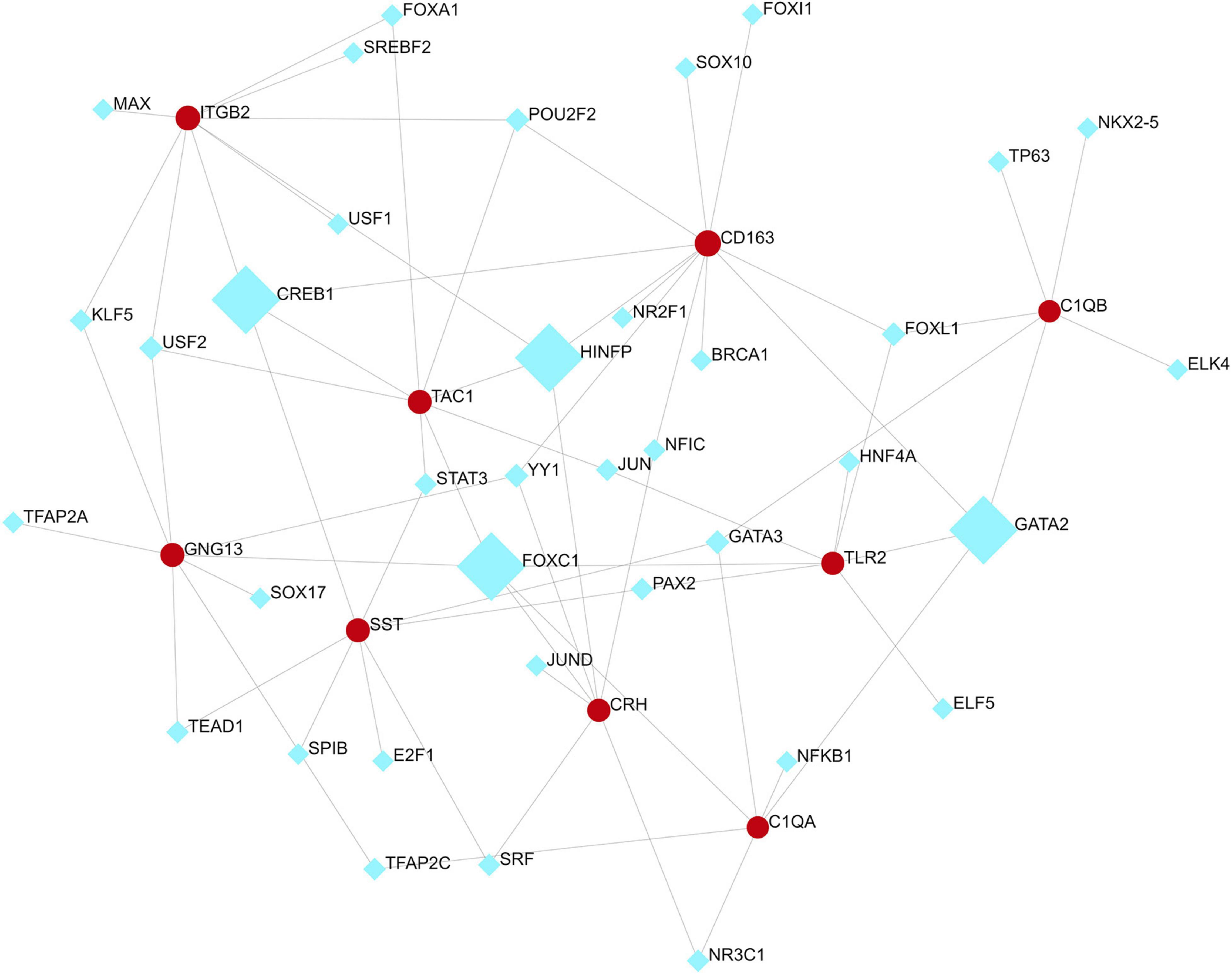
Figure 6. Hub gene–transcription factor interaction network. Medium confidence score was used to construct the network.
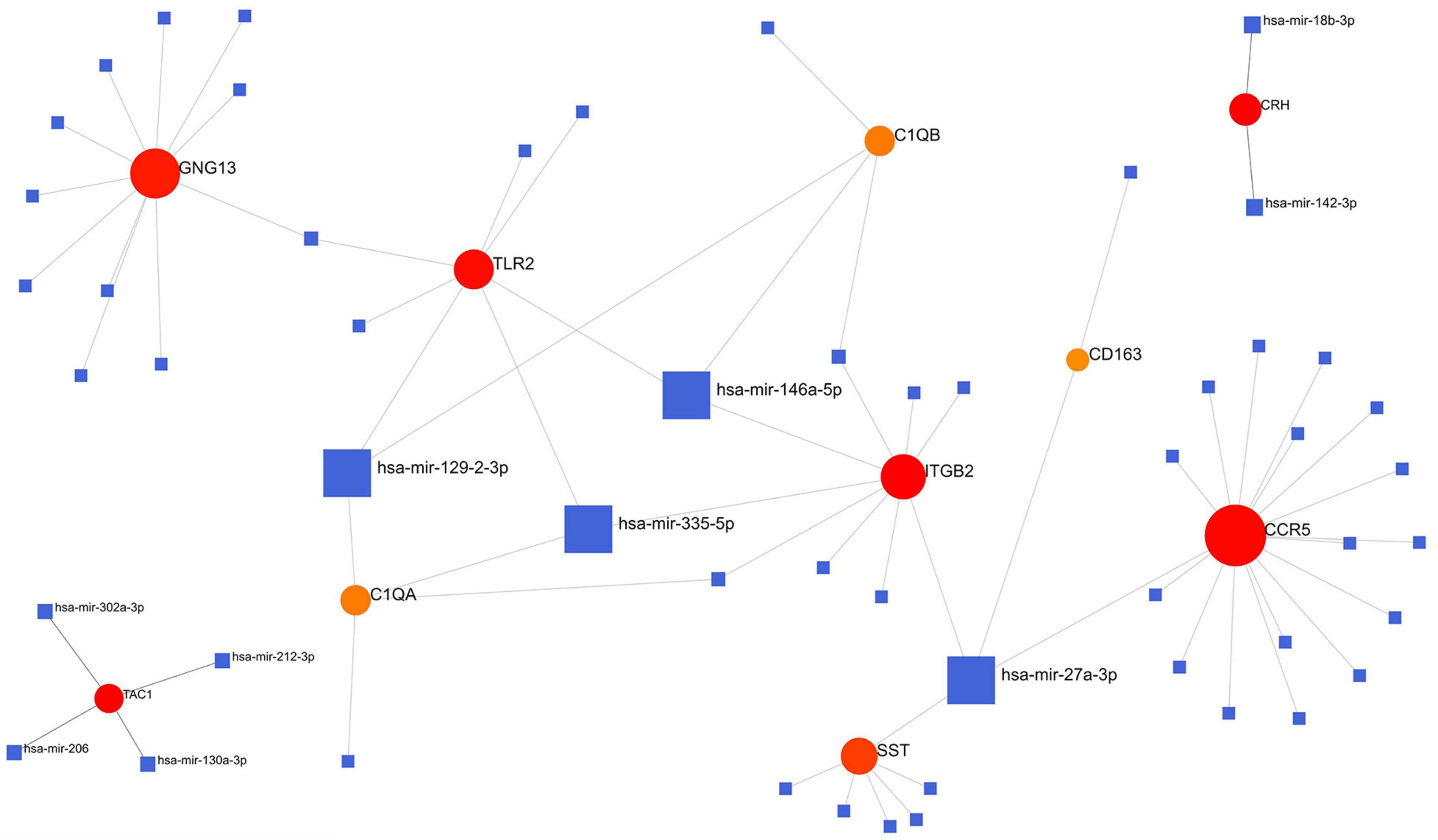
Figure 7. Hub gene–miRNA interaction network. Medium confidence score was used to construct the network.
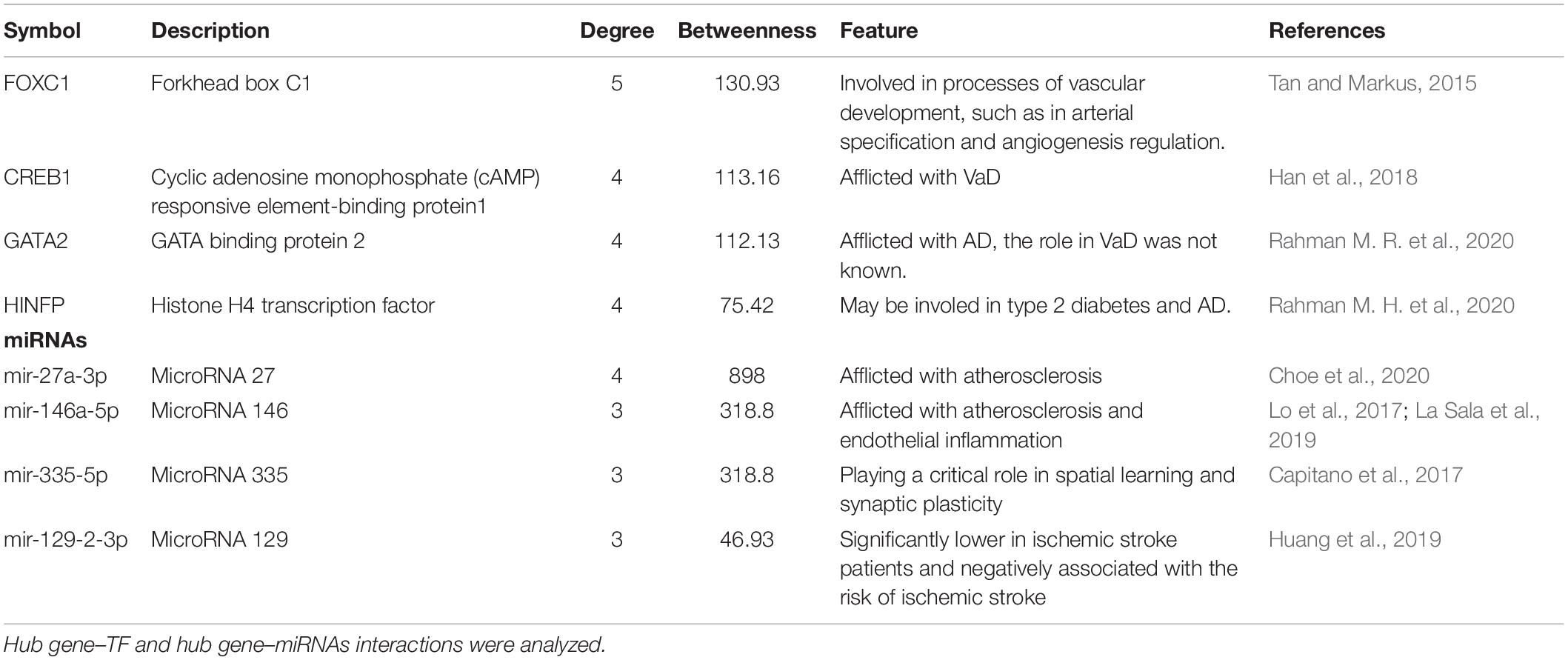
Table 5. Transcription factors (TFs) and miRNAs identified as transcriptional and/or posttranscriptional regulators of the hub genes in the protein–protein interaction network of VaD.
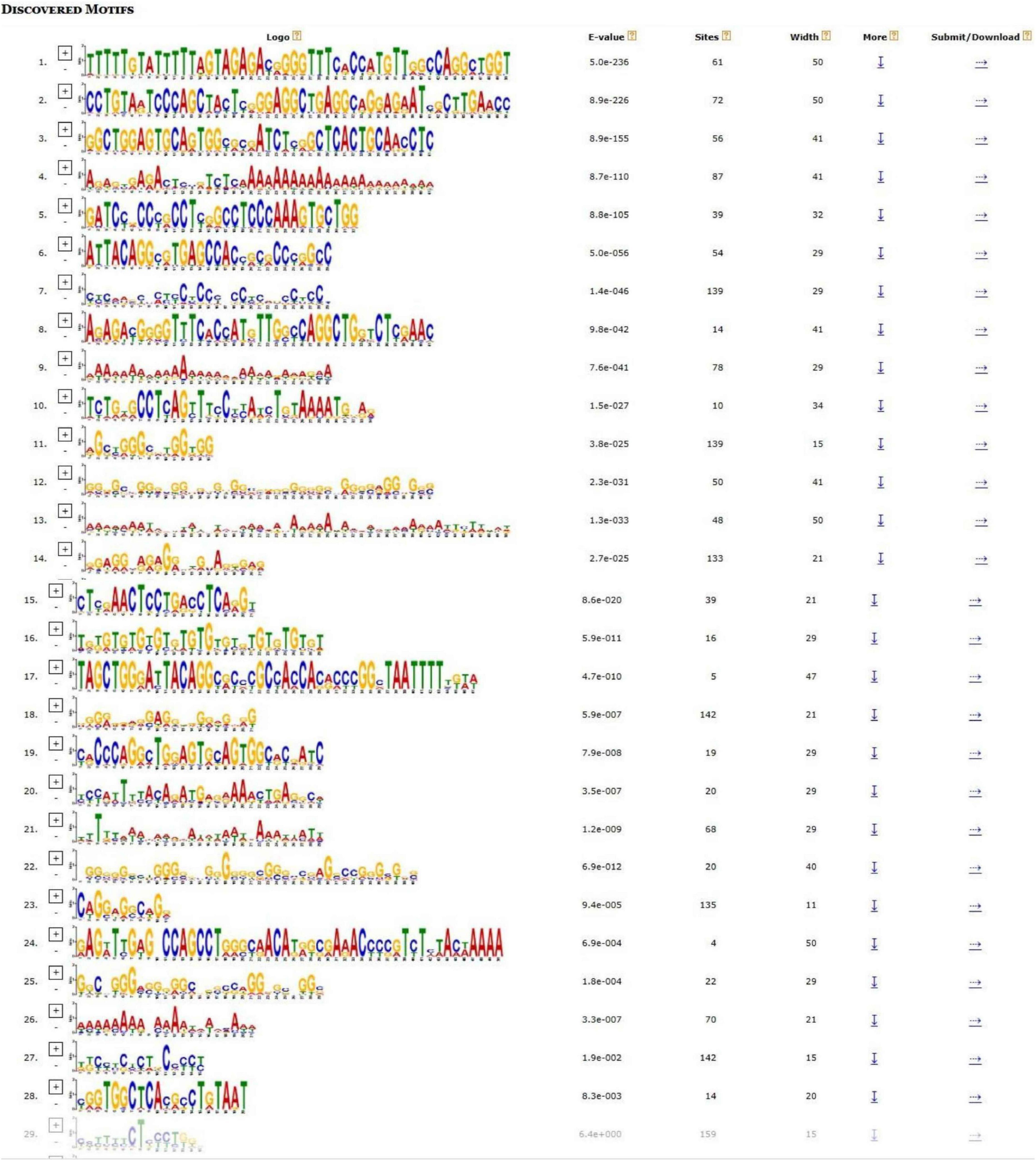
Figure 8. Motif analysis of differentially expressed genes (DEGs). Significant motifs were presented (E value < 0.05).
Drug–Gene Interaction Analysis
The 10 hub genes were considered as potential druggable targets for VaD treatment. The drug-gene interaction analysis found 69 candidate target drugs/compounds for VaD treatment. Among them, 22 targeted TAC1, 19 targeted ITGB2, 15 targeted CCR5, 7 targeted SST, 4 targeted TLR2, and 2 targeted CRH. For 47 of the candidate drugs, no specific types of interactions with the hub genes have been reported, and therefore these drugs need further investigation. For the other 22 candidate drugs, specific types of interactions with hub genes have been reported and were visualized using Cytoscape software (Figure 9 and Supplementary Table 3). No candidate drugs were identified for GNG13, CD163, C1QA, or TLR2. Until now, none of the 22 drugs have been used directly to treat VaD, as was shown by searching the ClinicalTrials.gov registry.
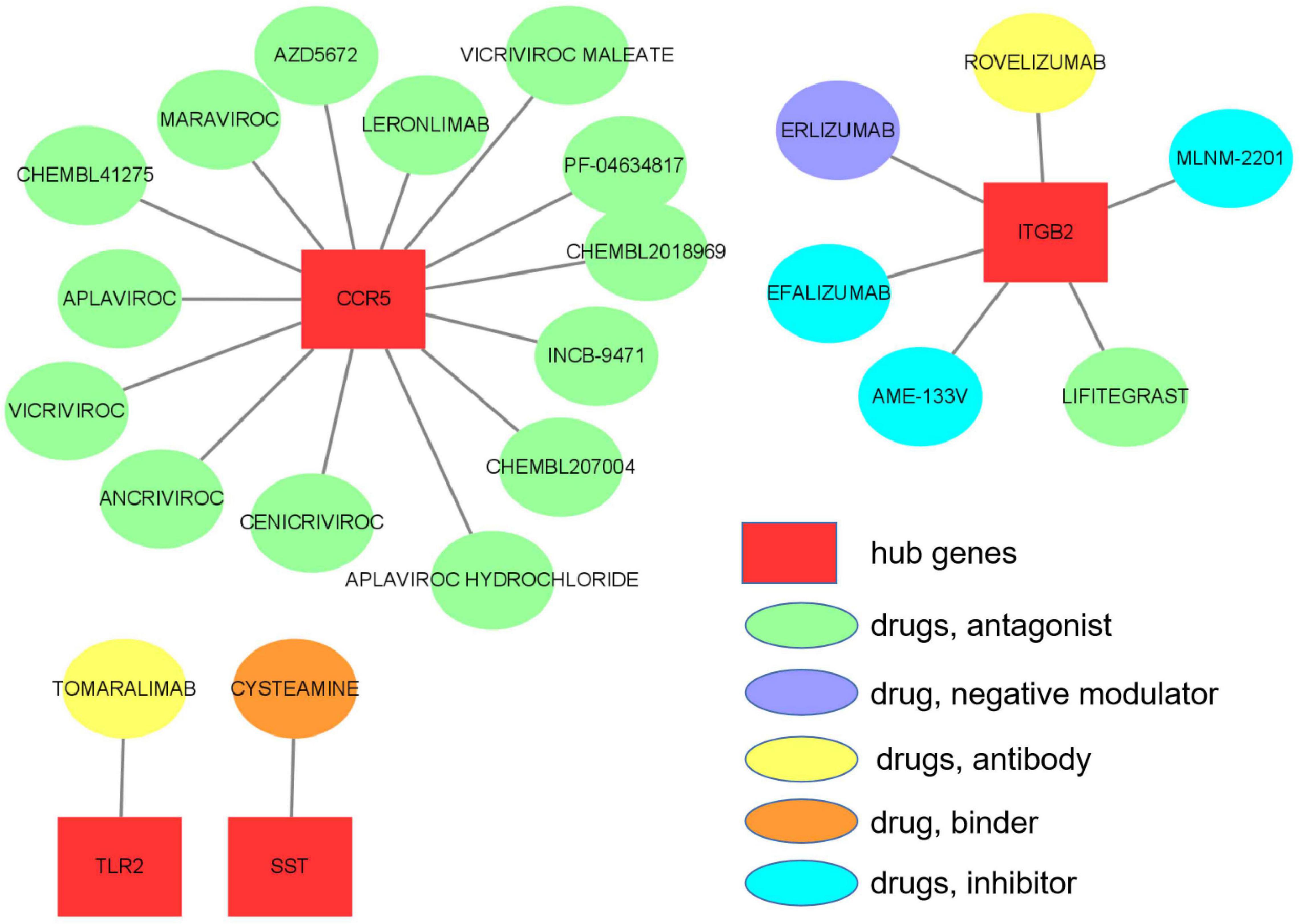
Figure 9. Hub gene–drug interactions that were found in the Drug–Gene Interaction Database (DGIdb) for four hub genes, TLR2, SST, ITGB2, and CCR5, are visualized using Cytoscape software.
Discussion
Vascular dementia is a progressive disease that affects cognitive abilities, especially executive functioning. The heterogeneity of causes of VaD makes it challenging to elucidate the neuropathological substrates and mechanisms of VaD. In this study, we used multi-stage bioinformatics analyses to identify biomolecular signatures that underlie the pathophysiological mechanisms of VaD by analyzing the gene expression patterns in the temporal cortex and frontal cortex samples from patients with VaD.
A total of 159 overlapping DEGs between these brain regions were identified. We performed GO and pathway enrichment analysis to obtain further insights into the functions and signaling pathways of the overlapping DEGs. We found that these DEGs were enriched mainly in inflammation and innate immunity, synapse pruning, regeneration, positive regulation of angiogenesis, response to nutrient levels, and positive regulation of the digestive system process. Accumulating evidence indicates that inflammation and innate immunity play crucial roles in the progression of VaD. Synaptic pruning is essential for the development and maintenance of healthy brain circuitry by removing less active or “weak” synapses to allow the strengthening and maturation of more active connections in a neural activity-dependent process. Disruption of synaptic pruning has been associated with several neural disorders, such as schizophrenia and AD (Presumey et al., 2017). The synaptic pruning pathway was shown to be activated early in the brain of patients with AD where it mediates synapse loss (Hong et al., 2016), yet its role in VaD is largely unknown and needs future research. Increasing evidence has suggested that aberrant angiogenesis may play a role in the development of VaD, but its precise role remains controversial. A significant association between angiogenesis activity and cerebrovascular disease, which is a significant contributor to VaD, has been reported (Callahan et al., 2020), whereas other studies have indicated that promoting angiogenesis, especially in functional blood vessels, can reduce the extent of ischemia and improve cognition in VaD (Yang et al., 2017; Zhao et al., 2019). Clearly, more studies are needed to better understand the potential of angiogenesis as an intervention target.
We performed PPI analysis to discover the potential relationships among the proteins encoded by the overlapping DEGs and identified 10 hub genes (GNG13, CD163, C1QA, TLR2, SST, C1QB, ITGB2, CCR5, CRH, and TAC). Heterotrimeric G proteins, which consist of alpha, beta, and gamma subunits, function as signal transducers for the seven-transmembrane, G protein-coupled receptors. GNG13, which encodes a gamma subunit, is expressed in taste, retinal, and neuronal tissues, and the GNG13 protein is involved in the gustatory signal transduction pathway by interacting with proteins containing PDZ domains (Li et al., 2006; Liu et al., 2012). Liu et al. (2018) suggested that the GNG13 subunit was a critical signaling component in both the main olfactory epithelium and apical vomeronasal epithelium, by playing an important role in odor-triggered social behaviors including male–male aggression. A recent study found the expression level of GNG13 was significantly reduced in patients with AD compared with its expression in healthy control subjects and GNG13 may act as a potential biomarker in Purkinje cells, indicating the state of health of the cerebellum (Sanfilippo et al., 2021). However, insufficient information is available on its role in VaD. Future experiments are needed for verification.
The protein encoded by CD163 is a cell-surface glycoprotein in the scavenger receptor cysteine-rich superfamily, and it is expressed exclusively in monocytes and macrophages (Ritter et al., 1999). A recent study found that CD163+ macrophages promoted plaque angiogenesis, vascular permeability, inflammation, and progression of atherosclerosis, and deletion of CD163 in mice reduced intraplaque neovascularization and plaque progression (Guo et al., 2018). Besides, CD163+ macrophages and microglia were in the central and peripheral nervous system and may play a role in the inflammatory process (Fabriek et al., 2005; Galea et al., 2008). Atherosclerosis and inflammatory responses are known to be related to VaD (Kalaria, 2018), which is consistent with our results. The proteins encoded by C1QA and C1QB belong to the C1q family, whose members are the first components of the complement pathway and are involved in complement system regulation and play crucial roles in neurological disorders (Lee et al., 2019). A previous study indicated that aged C1qa-knockout mice showed reduced levels of cognitive and memory decline (Stephan et al., 2013), which is in accordance with the results of our study.
The protein encoded by TLR2 is a member of the toll-like receptor (TLR) family, whose members are significant pattern recognition receptors of the innate immune system, initiating inflammatory cascades by recognizing pathogen- and damaged-associated molecular patterns. TLR2 was found to play a pivotal role in inflammation after ischemic brain injury (Wang et al., 2011) and was involved in the development of diabetic microvascular complications, including endothelial dysfunction and cognitive impairment (Hardigan et al., 2017). In this study, we found that TLR2 was upregulated in the VaD samples compared with the controls samples, which supports the previous results.
Somatostatin, which is encoded by SST, is a widely distributed peptide in the central nervous system where it affects the rates of neurotransmission, and it was reported to be decreased in cerebrospinal fluid in patients with AD and VaD (Heilig et al., 1995). CCR5 encodes a seven-membrane, G protein-coupled receptor that may impact learning and memory by acting on CREB signaling, a pathway that is critical for learning and memory (Necula et al., 2021). CRH encodes a member of the corticotropin-releasing factor family that functions as a significant regulator of homeostasis, mediating the autonomic, behavioral, and neuroendocrine responses to stress (Dedic et al., 2019). Previous studies indicated that CRH might be involved in the regulation of cognitive performances (Bernardi et al., 2000).
Hub genes are considered to be key genes that play vital roles in biological processes and can affect the regulation of other genes in related pathways; thus, hub genes are often important targets and research hotspots. We studied hub gene–TF and hub gene–miRNA interactions to identify potential transcriptional and posttranscriptional regulators of the 10 identified hub genes. We identified four TFs (FOXC1, CREB1, GATA2, and HINFP) and four miRNAs (miR-27a-3p, miR-146a-5p, miR-335-5p, and miR-129-2-3p) as regulators of the hub genes in VaD. FOXC1 is involved in vascular development processes, including arterial specification and angiogenesis regulation, and may play a role in small vessel disease, which is the primary pathology underlying vascular cognitive impairment (Tan and Markus, 2015). Cyclic adenosine monophosphate responsive element-binding protein 1 (CREB1) is a leucine-zipper transcription factor that plays an essential role in long-term memory formation (Sadamoto et al., 2010). Silencing of CREB1 exasperated cognitive dysfunction in vascular dementia (VD) mouse model by inhibiting activation of the PKA-CREB signaling pathway (Han et al., 2018). GATA2, which encodes GATA-binding protein 2, was found to be differentially expressed in AD (Rahman M. R. et al., 2020), but its role in VaD has not been reported so far.
The reduced level of miR-27a-3p found in the cerebrospinal fluid of patients with AD indicated it as a candidate biomarker for AD (Sala et al., 2013). A recent study reported that reduction of miR-27a-3p in vascular smooth muscle cells may result in the development of vascular calcification that develops in association with atherosclerosis, one of the critical risk factors of VaD (Choe et al., 2020). miR-146a-5p was also reported to be associated with dysfunction of the vascular endothelium and may be involved in regulating high glucose-induced endothelial inflammation and atherosclerosis (Lo et al., 2017; La Sala et al., 2019). Capitano et al. (2017) found that overexpression of miR-335-5p impaired spatial memory and long-term potentiation maintenance in mice, indicating that miR-335-5p may play a critical role in spatial learning and synaptic plasticity. The blood level of miR-129-2-3p was significantly lower in ischemic stroke patients and negatively associated with the risk of ischemic stroke (Huang et al., 2019; Lo et al., 2017). These miRNAs can be considered as candidate biomarkers for VaD.
To the best of our knowledge, there are no definitive drugs available for VaD treatment. We performed a drug-hub gene interaction analysis to detect potential target drugs/compounds for VaD treatment and identified a total of 22 drugs that were predicted to have specific types of interactions with the hub genes. We checked the 22 candidate drugs in the ClinicalTrials.gov registry (see footnote 8). Although none of the 22 drugs have been used directly to treat VaD, four of them (maraviroc, cenicriviroc, PF-04634817, and efalizumab) could be potential drugs for VaD treatment. Maraviroc, cenicriviroc, and PF-04634817 are all CCR5 antagonists and efalizumab is an IGTB2 antagonist. Maraviroc and cenicriviroc were reported to improve cognition in HIV-infected individuals with cognitive impairment through their antiretroviral and anti-inflammatory effects (Mora-Peris et al., 2018; Alagaratnam et al., 2019). Moreover, maraviroc was found to reduce cardiovascular risk by modulation of atherosclerotic progression in vivo and in vitro (Afonso et al., 2017; Francisci et al., 2019). PF-04634817 and efalizumab are being developed for the treatment of diabetic nephropathy (Gale et al., 2018) and type 1 diabetes mellitus (Posselt et al., 2010), respectively. Diabetes mellitus and its complications are known risk factors for VaD. These four drugs need to be evaluated as potential drugs in VaD treatment in future studies.
Our study has some limitations. First, our results are based on publicly available microarray data, and no clinical or experimental confirmation of the roles of the proteins encoded by the identified genes of interest was attempted in the present study. Second, the expression profiles from only one GEO dataset were analyzed because of the limited number of available microarray datasets of VaD. In future studies, if available, multiple datasets should be analyzed simultaneously to increase the reliability of the results. Notwithstanding these limitations, this study offers some insights into the underlying mechanisms involved in the progression of VaD.
Conclusion
We identified overlapping DEGs between the frontal cortex and temporal cortex of VaD. These overlapping DEGs were enriched mainly in GO terms and pathways associated with inflammation and innate immunity, synapse pruning, regeneration, positive regulation of angiogenesis, and response to nutrient levels, all of which play crucial roles in the progression of VaD. We also identified hub genes, TFs, and miRNAs that were predicted to regulate the expression of the hub genes, as well as candidate drugs that target the hub genes. Our results may contribute to understanding the underlying mechanisms in VaD and provide potential targets and drugs for therapeutic intervention.
Data Availability Statement
The datasets presented in this study can be found in online repositories. The names of the repository/repositories and accession number(s) can be found in the article/Supplementary Material.
Author Contributions
JS, WW, and LZ conceived and designed the study, analyzed the data, wrote the manuscript, and contributed to the designs of the methods used in this study. All authors have read and agreed to the publication of this version of the manuscript.
Funding
This work was funded by Shanghai Municipal Key Clinical Specialty, grant number shslczdzk02801, the Shanghai Municipal Health Commission, grant number 2020YJZX0109, the Shanghai Pujiang Program, grant number 2019PJD013, and National Natural Science Foundation of China (NSFC), grant number 81471103. The funders had no role in the design of the study; in the collection, analyses, or interpretation of data; in the writing of the manuscript, or in the decision to publish the results.
Conflict of Interest
The authors declare that the research was conducted in the absence of any commercial or financial relationships that could be construed as a potential conflict of interest.
The reviewer YZ has declared a shared parent affiliation with the authors at the time of review.
Publisher’s Note
All claims expressed in this article are solely those of the authors and do not necessarily represent those of their affiliated organizations, or those of the publisher, the editors and the reviewers. Any product that may be evaluated in this article, or claim that may be made by its manufacturer, is not guaranteed or endorsed by the publisher.
Acknowledgments
We thank Margaret Biswas, from Liwen Bianji (Edanz) (www.liwenbianji.cn/) for editing the English text of a draft of this manuscript.
Supplementary Material
The Supplementary Material for this article can be found online at: https://www.frontiersin.org/articles/10.3389/fnmol.2022.751044/full#supplementary-material
Footnotes
- ^ https://www.ncbi.nlm.nih.gov/geo/
- ^ http://metascape.org/
- ^ www.string-db.org
- ^ www.cytoscape.org
- ^ http://meme-suite.org
- ^ http://starbase.sysu.edu.cn/
- ^ www.dgidb.org
- ^ https://clinicaltrials.gov/
References
Afonso, P., Auclair, M., Caron-Debarle, M., and Capeau, J. (2017). Impact of CCR5, integrase and protease inhibitors on human endothelial cell function, stress, inflammation and senescence. Antivir. Ther. 22, 645–657. doi: 10.3851/IMP3160
Alagaratnam, J., Dilly-Penchala, S., Challenger, E., Else, L., Legg, K., Petersen, C., et al. (2019). Cerebrospinal fluid exposure of cenicriviroc in HIV-positive individuals with cognitive impairment. Br. J. Clin. Pharmacol. 85, 1039–1040. doi: 10.1111/bcp.13878
Bailey, T. L., Johnson, J., Grant, C. E., and Noble, W. S. (2015). The MEME suite. Nucleic Acids Res. 43, W39–W49. doi: 10.1093/nar/gkv416
Bardou, P., Mariette, J., Escudie, F., Djemiel, C., and Klopp, C. (2014). Jvenn: an interactive Venn diagram viewer. BMC Bioinformatics 15:293. doi: 10.1186/1471-2105-15-293
Barrett, T., Wilhite, S. E., Ledoux, P., Evangelista, C., Kim, I. F., Tomashevsky, M., et al. (2013). NCBI GEO: archive for functional genomics data sets–update. Nucl. Acids Res. 41, D991–D995. doi: 10.1093/nar/gks1193
Bernardi, F., Lanzone, A., Cento, R. M., Spada, R. S., Pezzani, I., Genazzani, A. D., et al. (2000). Allopregnanolone and dehydroepiandrosterone response to corticotropin-releasing factor in patients suffering from Alzheimer’s disease and vascular dementia. Eur. J. Endocrinol. 142, 466–471. doi: 10.1530/eje.0.1420466
Callahan, C. M., Apostolova, L. G., Gao, S., Risacher, S. L., Case, J., Saykin, A. J., et al. (2020). Novel markers of angiogenesis in the setting of cognitive impairment and dementia. J. Alzheimers Dis. 75, 959–969. doi: 10.3233/JAD-191293
Capitano, F., Camon, J., Licursi, V., Ferretti, V., Maggi, L., Scianni, M., et al. (2017). MicroRNA-335-5p modulates spatial memory and hippocampal synaptic plasticity. Neurobiol. Learn. Mem. 139, 63–68. doi: 10.1016/j.nlm.2016.12.019
Choe, N., Kwon, D. H., Ryu, J., Shin, S., Cho, H. J., Joung, H., et al. (2020). MiR-27a-3p targets ATF3 to reduce calcium deposition in vascular smooth muscle cells. Mol. Ther. Nucleic Acids 22, 627–639. doi: 10.1016/j.omtn.2020.09.030
Cotto, K. C., Wagner, A. H., Feng, Y. Y., Kiwala, S., Coffman, A. C., Spies, G., et al. (2018). DGIdb 3.0: a redesign and expansion of the drug-gene interaction database. Nucleic Acids Res. 46, D1068–D1073. doi: 10.1093/nar/gkx1143
Dedic, N., Kühne, C., Gomes, K. S., Hartmann, J., Ressler, K. J., Schmidt, M. V., et al. (2019). Deletion of CRH from GABAergic forebrain neurons promotes stress resilience and dampens Stress-Induced changes in neuronal activity. Front. Neurosci. Switz. 13:986. doi: 10.3389/fnins.2019.00986
Fabriek, B. O., Van Haastert, E. S., Galea, I., Polfliet, M. M., Dopp Van Den Heuvel, M. M., et al. (2005). CD163-positive perivascular macrophages in the human CNS express molecules for antigen recognition and presentation. Glia 51, 297–305. doi: 10.1002/glia.20208
Francisci, D., Pirro, M., Schiaroli, E., Mannarino, M. R., Cipriani, S., Bianconi, V., et al. (2019). Maraviroc intensification modulates atherosclerotic progression in HIV-Suppressed patients at high cardiovascular risk. a randomized, crossover pilot study. Open Forum Infect. Dis. 6:z112. doi: 10.1093/ofid/ofz112
Gale, J. D., Gilbert, S., Blumenthal, S., Elliott, T., Pergola, P. E., Goteti, K., et al. (2018). Effect of PF-04634817, an oral CCR2/5 chemokine receptor antagonist, on albuminuria in adults with overt diabetic nephropathy. Kidney Int. Rep. 3, 1316–1327. doi: 10.1016/j.ekir.2018.07.010
Galea, I., Felton, L. M., Waters, S., van Rooijen, N., Perry, V. H., and Newman, T. A. (2008). Immune-to-brain signalling: the role of cerebral CD163-positive macrophages. Neurosci. Lett. 448, 41–46. doi: 10.1016/j.neulet.2008.09.081
Guo, L., Akahori, H., Harari, E., Smith, S. L., Polavarapu, R., Karmali, V., et al. (2018). CD163+ macrophages promote angiogenesis and vascular permeability accompanied by inflammation in atherosclerosis. J. Clin. Invest. 128, 1106–1124. doi: 10.1172/JCI93025
Guo, T., Fang, J., Tong, Z. Y., He, S., and Luo, Y. (2020). Transcranial direct current stimulation ameliorates cognitive impairment via modulating oxidative stress, inflammation, and autophagy in a rat model of vascular dementia. Front. Neurosci. 14:28. doi: 10.3389/fnins.2020.00028
Gupta, S., Stamatoyannopoulos, J. A., Bailey, T. L., and Noble, W. S. (2007). Quantifying similarity between motifs. Genome Biol. 8:R24. doi: 10.1186/gb-2007-8-2-r24
Han, X. R., Wen, X., Wang, Y. J., Wang, S., Shen, M., Zhang, Z. F., et al. (2018). Effects of CREB1 gene silencing on cognitive dysfunction by mediating PKA-CREB signaling pathway in mice with vascular dementia. Mol. Med. 24:18. doi: 10.1186/s10020-018-0020-y
Hardigan, T., Hernandez, C., Ward, R., Hoda, M. N., and Ergul, A. (2017). TLR2 knockout protects against diabetes-mediated changes in cerebral perfusion and cognitive deficits. Am. J. Physiol. Regul. Integr. Comp. Physiol. 312, R927–R937. doi: 10.1152/ajpregu.00482.2016
Heilig, M., Sjogren, M., Blennow, K., Ekman, R., and Wallin, A. (1995). Cerebrospinal fluid neuropeptides in Alzheimer’s disease and vascular dementia. Biol. Psychiatry 38, 210–216. doi: 10.1016/0006-3223(94)00239-Y
Hong, S., Beja-Glasser, V. F., Nfonoyim, B. M., Frouin, A., Li, S., Ramakrishnan, S., et al. (2016). Complement and microglia mediate early synapse loss in Alzheimer mouse models. Science 352, 712–716. doi: 10.1126/science.aad8373
Huang, S., Lv, Z., Wen, Y., Wei, Y., Zhou, L., Ke, Y., et al. (2019). MiR-129-2-3p directly targets SYK gene and associates with the risk of ischaemic stroke in a Chinese population. J. Cell. Mol. Med. 23, 167–176. doi: 10.1111/jcmm.13901
Jassal, B., Matthews, L., Viteri, G., Gong, C., Lorente, P., Fabregat, A., et al. (2020). The reactome pathway knowledgebase. Nucleic Acids Res. 48, D498–D503. doi: 10.1093/nar/gkz1031
Kalaria, R. N. (2016). Neuropathological diagnosis of vascular cognitive impairment and vascular dementia with implications for Alzheimer’s disease. Acta Neuropathol. 131, 659–685. doi: 10.1007/s00401-016-1571-z
Kalaria, R. N. (2018). The pathology and pathophysiology of vascular dementia. Neuropharmacology 134, 226–239. doi: 10.1016/j.neuropharm.2017.12.030
Kanehisa, M., Furumichi, M., Tanabe, M., Sato, Y., and Morishima, K. (2017). New perspectives on genomes, pathways, diseases and drugs. Nucleic Acids Res. 45, D353–D361. doi: 10.1093/nar/gkw1092
Karagkouni, D., Paraskevopoulou, M. D., Chatzopoulos, S., Vlachos, I. S., Tastsoglou, S., Kanellos, I., et al. (2018). DIANA-TarBase v8: a decade-long collection of experimentally supported miRNA-gene interactions. Nucleic Acids Res. 46, D239–D245. doi: 10.1093/nar/gkx1141
Khan, A., Fornes, O., Stigliani, A., Gheorghe, M., Castro-Mondragon, J. A., van der Lee, R., et al. (2018). JASPAR 2018: update of the open-access database of transcription factor binding profiles and its web framework. Nucleic Acids Res. 46, D260–D266. doi: 10.1093/nar/gkx1126
Khan, A., Kalaria, R. N., Corbett, A., and Ballard, C. (2016). Update on Vascular Dementia. J. Geriatr. Psychiatry Neurol. 29, 281–301. doi: 10.1177/0891988716654987
La Sala, L., Prattichizzo, F., and Ceriello, A. (2019). The link between diabetes and atherosclerosis. Eur. J. Prev. Cardiol. 26, 15–24. doi: 10.1177/2047487319878373
Lee, J. D., Coulthard, L. G., and Woodruff, T. M. (2019). Complement dysregulation in the central nervous system during development and disease. Semin. Immunol. 45:101340. doi: 10.1016/j.smim.2019.101340
Lee, T., and Lee, H. (2021). Shared Blood Transcriptomic Signatures between Alzheimer’s Disease and Diabetes Mellitus. Biomedicines 9:34. doi: 10.3390/biomedicines9010034
Li, J. H., Liu, S., Zhou, H., Qu, L. H., and Yang, J. H. (2014). StarBase v2.0: decoding miRNA-ceRNA, miRNA-ncRNA and protein-RNA interaction networks from large-scale CLIP-Seq data. Nucleic Acids Res. 42, D92–D97. doi: 10.1093/nar/gkt1248
Li, Z., Benard, O., and Margolskee, R. F. (2006). Ggamma13 interacts with PDZ domain-containing proteins. J. Biol. Chem. 281, 11066–11073. doi: 10.1074/jbc.M600113200
Liu, Q., Li, S., Lu, C., Yu, C. R., and Huang, L. G. (2018). protein gamma subunit Ggamma13 is essential for olfactory function and aggressive behavior in mice. Neuroreport 29, 1333–1339. doi: 10.1097/WNR.0000000000001122
Liu, Z., Fenech, C., Cadiou, H., Grall, S., Tili, E., Laugerette, F., et al. (2012). Identification of new binding partners of the chemosensory signaling protein Ggamma13 expressed in taste and olfactory sensory cells. Front. Cell. Neurosci. 6:26. doi: 10.3389/fncel.2012.00026
Lo, W. Y., Peng, C. T., and Wang, H. J. (2017). MicroRNA-146a-5p Mediates High Glucose-Induced Endothelial Inflammation via Targeting Interleukin-1 Receptor-Associated Kinase 1 Expression. Front. Physiol. 8:551. doi: 10.3389/fphys.2017.00551
Ma, G., Liu, M., Du, K., Zhong, X., Gong, S., Jiao, L., et al. (2019). Differential expression of mRNAs in the brain tissues of patients with alzheimer’s disease based on GEO expression profile and its clinical significance. Biomed. Res. Int. 2019:8179145. doi: 10.1155/2019/8179145
Martens, M., Ammar, A., Riutta, A., Waagmeester, A., Slenter, D. N., Hanspers, K., et al. (2021). WikiPathways: connecting communities. Nucleic Acids Res. 49, D613–D621. doi: 10.1093/nar/gkaa1024
McKay, E. C., Beck, J. S., Khoo, S. K., Dykema, K. J., Cottingham, S. L., Winn, M. E., et al. (2019). Peri-Infarct upregulation of the oxytocin receptor in vascular dementia. J. Neuropathol. Exp. Neurol. 78, 436–452. doi: 10.1093/jnen/nlz023
Mi, H., Muruganujan, A., and Thomas, P. D. (2013). PANTHER in 2013: modeling the evolution of gene function, and other gene attributes, in the context of phylogenetic trees. Nucleic Acids Res. 41, D377–D386. doi: 10.1093/nar/gks1118
Mora-Peris, B., Bouliotis, G., Ranjababu, K., Clarke, A., Post, F. A., Nelson, M., et al. (2018). Changes in cerebral function parameters with maraviroc-intensified antiretroviral therapy in treatment naive HIV-positive individuals. Aids 32, 1007–1015. doi: 10.1097/QAD.0000000000001786
Necula, D., Riviere-Cazaux, C., Shen, Y., and Zhou, M. (2021). Insight into the roles of CCR5 in learning and memory in normal and disordered states. Brain Behav. Immun. 92, 1–9. doi: 10.1016/j.bbi.2020.11.037
O’Brien, J. T., and Thomas, A. (2015). Vascular dementia. Lancet 386, 1698–1706. doi: 10.1016/S0140-6736(15)00463-8
Pan, Y., Yu, C., Huang, J., Rong, Y., Chen, J., and Chen, M. (2020). Bioinformatics analysis of vascular RNA-seq data revealed hub genes and pathways in a novel Tibetan minipig atherosclerosis model induced by a high fat/cholesterol diet. Lipids Health Dis. 19:54. doi: 10.1186/s12944-020-01222-w
Pendlebury, S. T., and Rothwell, P. M. (2019). Incidence and prevalence of dementia associated with transient ischaemic attack and stroke: analysis of the population-based Oxford Vascular Study. Lancet Neurol. 18, 248–258. doi: 10.1016/S1474-4422(18)30442-3
Posselt, A. M., Bellin, M. D., Tavakol, M., Szot, G. L., Frassetto, L. A., Masharani, U., et al. (2010). Islet transplantation in type 1 diabetics using an immunosuppressive protocol based on the anti-LFA-1 antibody efalizumab. Am. J. Transplant. 10, 1870–1880. doi: 10.1111/j.1600-6143.2010.03073.x
Presumey, J., Bialas, A. R., and Carroll, M. C. (2017). Complement system in neural synapse elimination in development and disease. Adv. Immunol. 135, 53–79. doi: 10.1016/bs.ai.2017.06.004
Rahman, M. H., Peng, S., Hu, X., Chen, C., Rahman, M. R., Uddin, S., et al. (2020). A Network-Based bioinformatics approach to identify molecular biomarkers for type 2 diabetes that are linked to the progression of neurological diseases. Int. J. Environ. Res. Public Health 17:1035. doi: 10.3390/ijerph17031035
Rahman, M. R., Islam, T., Zaman, T., Shahjaman, M., Karim, M. R., Huq, F., et al. (2020). Identification of molecular signatures and pathways to identify novel therapeutic targets in Alzheimer’s disease: insights from a systems biomedicine perspective. Genomics 112, 1290–1299. doi: 10.1016/j.ygeno.2019.07.018
Ritter, M., Buechler, C., Langmann, T., and Schmitz, G. (1999). Genomic organization and chromosomal localization of the human CD163 (M130) gene: a member of the scavenger receptor cysteine-rich superfamily. Biochem. Biophys. Res. Commun. 260, 466–474. doi: 10.1006/bbrc.1999.0866
Sadamoto, H., Kitahashi, T., Fujito, Y., and Ito, E. (2010). Learning-Dependent gene expression of CREB1 isoforms in the molluscan brain. Front. Behav. Neurosci. 4:25. doi: 10.3389/fnbeh.2010.00025
Sala, F. C., Lau, P., Salta, E., Tournoy, J., Bossers, K., Vandenberghe, R., et al. (2013). Reduced expression of hsa-miR-27a-3p in CSF of patients with Alzheimer disease. Neurology 81, 2103–2106. doi: 10.1212/01.wnl.0000437306.37850.22
Sanfilippo, C., Musumeci, G., Kazakova, M., Mazzone, V., Castrogiovanni, P., Imbesi, R., et al. (2021). GNG13 is a potential marker of the state of health of alzheimer’s disease patients’ cerebellum. J. Mol. Neurosci. 71, 1046–1060. doi: 10.1007/s12031-020-01726-1
Shannon, P., Markiel, A., Ozier, O., Baliga, N. S., Wang, J. T., Ramage, D., et al. (2003). Cytoscape: a software environment for integrated models of biomolecular interaction networks. Genome Res. 13, 2498–2504. doi: 10.1101/gr.1239303
Stephan, A. H., Madison, D. V., Mateos, J. M., Fraser, D. A., Lovelett, E. A., Coutellier, L., et al. (2013). A dramatic increase of C1q protein in the CNS during normal aging. J. Neurosci. 33, 13460–13474. doi: 10.1523/JNEUROSCI.1333-13.2013
Szklarczyk, D., Morris, J. H., Cook, H., Kuhn, M., Wyder, S., Simonovic, M., et al. (2017). The STRING database in 2017: quality-controlled protein-protein association networks, made broadly accessible. Nucleic Acids Res. 45, D362–D368. doi: 10.1093/nar/gkw937
Tan, R. Y., and Markus, H. S. (2015). Monogenic causes of stroke: now and the future. J. Neurol. 262, 2601–2616. doi: 10.1007/s00415-015-7794-4
Tian, X., Qin, Y., Tian, Y., Ge, X., Cui, J., Han, H., et al. (2021). Identification of vascular dementia and Alzheimer’s disease hub genes expressed in the frontal lobe and temporal cortex by weighted co-expression network analysis and construction of a protein-protein interaction. Int. J. Neurosci. 18, 1–12. doi: 10.1080/00207454.2020.1860966
Tukacs, V., Mittli, D., Gyorffy, B. A., Hunyady-Gulyas, E., Hlatky, D., Toth, V., et al. (2020). Chronic stepwise cerebral hypoperfusion differentially induces synaptic proteome changes in the frontal cortex, occipital cortex, and hippocampus in rats. Sci. Rep. 10:15999. doi: 10.1038/s41598-020-72868-w
Ueno, M., Chiba, Y., Murakami, R., Matsumoto, K., Fujihara, R., Uemura, N., et al. (2019). Disturbance of intracerebral fluid clearance and Blood-Brain barrier in vascular cognitive impairment. Int. J. Mol. Sci. 20:2600. doi: 10.3390/ijms20102600
Vieira, R. T., Caixeta, L., Machado, S., Silva, A. C., Nardi, A. E., Arias-Carrion, O., et al. (2013). Epidemiology of early-onset dementia: a review of the literature. Clin. Pract. Epidemiol. Ment. Health. 9, 88–95. doi: 10.2174/1745017901309010088
Wang, F., Cao, Y., Ma, L., Pei, H., Rausch, W. D., and Li, H. (2018). Dysfunction of cerebrovascular endothelial cells: prelude to vascular dementia. Front. Aging Neurosci. 10:376. doi: 10.3389/fnagi.2018.00376
Wang, X. X., Zhang, B., Xia, R., and Jia, Q. Y. (2020). Inflammation, apoptosis and autophagy as critical players in vascular dementia. Eur. Rev. Med. Pharmacol. Sci. 24, 9601–9614. doi: 10.26355/eurrev_202009_23048
Wang, Y. C., Lin, S., and Yang, Q. W. (2011). Toll-like receptors in cerebral ischemic inflammatory injury. J. Neuroinflamm. 8:134. doi: 10.1186/1742-2094-8-134
Xia, J., Gill, E. E., and Hancock, R. E. (2015). NetworkAnalyst for statistical, visual and network-based meta-analysis of gene expression data. Nat. Protoc. 10, 823–844. doi: 10.1038/nprot.2015.052
Yang, X., Li, C., Chen, C., Tang, X., Cheng, G., and Li, X. (2017). Protective effect of Shouwu Yizhi decoction against vascular dementia by promoting angiogenesis. Chin. J. Nat. Med. 15, 740–750. doi: 10.1016/S1875-5364(17)30105-X
Zhao, H., Mao, J., Yuan, Y., Feng, J., Cheng, H., Fan, G., et al. (2019). Sodium dichloroacetate stimulates angiogenesis by improving endothelial precursor cell function in an AKT/GSK-3beta/Nrf2 dependent pathway in vascular dementia rats. Front. Pharmacol. 10:523. doi: 10.3389/fphar.2019.00523
Keywords: systems biology, gene–drug interaction, hub gene, vascular dementia, protein–protein interaction (PPI)
Citation: Shu J, Wei W and Zhang L (2022) Identification of Molecular Signatures and Candidate Drugs in Vascular Dementia by Bioinformatics Analyses. Front. Mol. Neurosci. 15:751044. doi: 10.3389/fnmol.2022.751044
Received: 02 August 2021; Accepted: 17 January 2022;
Published: 11 February 2022.
Edited by:
Eva Zerovnik, Institut Jožef Stefan (IJS), SloveniaCopyright © 2022 Shu, Wei and Zhang. This is an open-access article distributed under the terms of the Creative Commons Attribution License (CC BY). The use, distribution or reproduction in other forums is permitted, provided the original author(s) and the copyright owner(s) are credited and that the original publication in this journal is cited, in accordance with accepted academic practice. No use, distribution or reproduction is permitted which does not comply with these terms.
*Correspondence: Wenshi Wei, d2Vuc2hpd2VpMTk5OUAxNjMuY29t; Li Zhang, bGl6aGFuZ19odWFkb25nQDE2My5jb20=