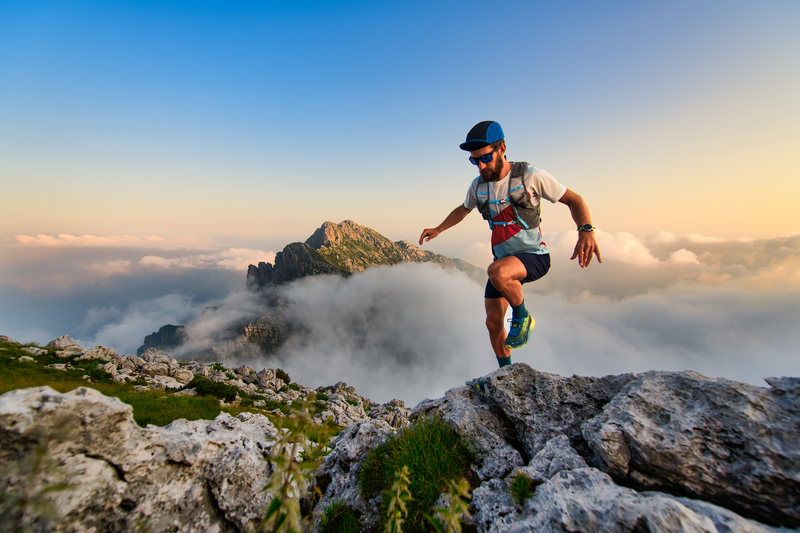
95% of researchers rate our articles as excellent or good
Learn more about the work of our research integrity team to safeguard the quality of each article we publish.
Find out more
MINI REVIEW article
Front. Mol. Neurosci. , 05 July 2021
Sec. Brain Disease Mechanisms
Volume 14 - 2021 | https://doi.org/10.3389/fnmol.2021.695873
This article is part of the Research Topic Autism Spectrum Disorders and Metal Dyshomeostasis View all 8 articles
Metal dyshomeostasis plays a significant role in various neurological diseases such as Alzheimer’s disease, Parkinson’s disease, Autism Spectrum Disorders (ASD), and many more. Like studies investigating the proteome, transcriptome, epigenome, microbiome, etc., for years, metallomics studies have focused on data from their domain, i.e., trace metal composition, only. Still, few have considered the links between other “omes,” which may together result in an individual’s specific pathologies. In particular, ASD have been reported to have multitudes of possible causal effects. Metallomics data focusing on metal deficiencies and dyshomeostasis can be linked to functions of metalloenzymes, metal transporters, and transcription factors, thus affecting the proteome and transcriptome. Furthermore, recent studies in ASD have emphasized the gut-brain axis, with alterations in the microbiome being linked to changes in the metabolome and inflammatory processes. However, the microbiome and other “omes” are heavily influenced by the metallome. Thus, here, we will summarize the known implications of a changed metallome for other “omes” in the body in the context of “omics” studies in ASD. We will highlight possible connections and propose a model that may explain the so far independently reported pathologies in ASD.
Many metals are found in the human body. Although the essentiality is evident for K, Na, Ca, Mg, Fe, Zn, Cu, Mn, Mo, and Co, research is ongoing to decipher which other metals can be considered toxic, neutral, or beneficial to humans. The body’s metal composition varies between organs, tissue, and even on the subcellular level. Metals such as K, Na, Mg, and Ca are required in higher concentrations than trace metals such as Fe, Zn, Cu, and Mn. However, although trace metals may only be needed in small quantities, they are essential in metabolism, growth, and development (Zoroddu et al., 2019). Each metal may function in various processes as a cofactor for proteins such as enzymes and signaling ions. Some may also play a role in redox reactions accepting and donating electrons. Others play a structural role in biological molecules, while again others aid ligand binding to specific receptors (Mertz, 1998). The presence and concentration of each of the metals within a cellular compartment, cell, tissue, organ, etc., establishes the metallome. The metallome consists of “free” metal ions and metals in metal-containing molecules and proteins such as metalloproteins and metalloenzymes. Metal localization and levels are highly controlled by processes regulating metal homeostasis.
Metals with biological functions are also referred to as biometals. Each biometal has a network of transporters and buffering proteins involved in the uptake and transport that facilitate the process carried out by them. Notably, an imbalance of one particular trace metal is unlikely in disease states. Typically, the concentrations and ratios of multiple trace metals are impacted if one metal concentration is altered. An example of such an event is apparent in individuals with irritable bowel disease (IBD). Zinc concentrations are typically decreased from 15 to 40% in affected individuals. Conversely, copper concentrations are increased in these individuals (Schneider et al., 2020). This observation is in line with many studies, including research on ASD, that show that zinc and copper interact at the mucosal level and in the blood, where the ratios are inversely related (Li et al., 2014).
Metal homeostasis can be influenced by multiple factors such as genetic mutations but also by environmental factors such as nutritional intake, stress, and immune-modulatory events (Greenough et al., 2013; Grabrucker, 2016). Alterations in an individual’s metallome have been shown for the major neurodegenerative diseases such as Alzheimer’s and Parkinson’s disease, and Amyotrophic lateral sclerosis (ALS) (Scholefield et al., 2020). In the future, specific metal profiles may even be utilized as biomarkers of particular illnesses. They may be targeted to alleviate symptoms, and preventative measures may be put in place to counteract dyshomeostasis. In particular, biometals have gained attention in relation to ASD (Hagmeyer et al., 2018; Yang et al., 2019a; Nakagawa and Yamada, 2021).
Studies have consistently implicated the putative role of trace metals in neurobehavioral disorders and behaviors such as attention deficit hyperactivity (Fe, Zn), cognitive impairments (Zn, Fe, Mn, Co), depression (Zn, Cr, Fe, Co), and ASD (Zn, Cu, Co, Mn) (Cortese et al., 2012; Graham et al., 2014; Alghadir et al., 2016; Saghazadeh et al., 2017; Yang et al., 2019b). Specific behavioral features characterize ASD. Individuals with ASD display impaired social abilities, problems with language and communication, repetitive behaviors, and often experience sensory alterations and co-morbidities such as seizures, hyperactivity, sleep disorders, increased risk of allergies, gastrointestinal problems, and metabolic plus mitochondrial diseases, which commence early in life (Lord et al., 2020). ASD are a group of heterogeneous disorders that are likely caused and influenced by environmental and genetic factors or a combination of both. Thus, the complexity of ASD is difficult to address using animal models (Betancur, 2011). ASD may be characterized by elevated levels of mitochondrial dysfunction, lipid peroxidation, and decreased levels of antioxidants such as transferrin, ceruloplasmin, metallothionein, and glutathione which is evident through blood serum analysis (Chauhan et al., 2004). Accordingly, the mechanisms commonly studied in ASD in relation to metal dyshomeostasis include various factors such as oxidative stress, the competition of toxic metals with essential metals such as Cu and Zn, inflammation, neurotransmitter synthesis, and neuronal dysfunction, such as impaired synaptic function and neurogenesis (Vela et al., 2015; Ha et al., 2018; Pangrazzi et al., 2020). Metal dyshomeostasis in ASD has been consistently under review in recent years. Most frequently, an imbalance in the Zn:Cu ratio has been reported in ASD, and mechanistic studies hint at a causative role (Bjorklund, 2013).
For example, a decrease in Zn and an increase in Cu results in higher levels of oxidative stress. Oxidative stress is caused by the oxidation of biomolecules, leading to damage of cells and tissues and is contributing to inflammation in many diseases (Singh et al., 2019a). Reactive oxygen species (ROS), including H2O2 and OH–, are continuously present under aerobic conditions. Particularly in the case of increased Cu and decreased Zn concentrations, the positive regulation of apometallothionein is affected. Apomethallothionein binds with a high affinity to toxic metals and, as such, plays a pivotal role alongside Zn in the detoxification of toxic metals (Kang, 2006; Macedoni-Lukšič et al., 2015; Mostafa et al., 2016). Metals such as Cd, Pb, and Hg have been implicated in promoting intracellular ROS production. However, not all metals can act as electron donors or acceptors. For example, Zn is redox inert and considered an antioxidant through indirect interactions, acting as an inhibitor of NADPH oxidases which catalyzes the production of O2 using NADPH as an electron donor (Marreiro et al., 2017). In oxidative stress conditions, superoxide dismutase (SOD), which contains both Cu, Mn, and Zn, converts O2 to H2O2 (Cortese et al., 2008). A deficiency in either Zn, Cu, or Mn have been shown to impact oxidative stress by decreasing the levels of SOD (Meguid et al., 2011). Zn, Cu, and Mn also increase glutathione production, which maintains the cellular redox state acting as a further protective function in oxidative stress. Evidence suggests that toxic metals such as Cd contribute to oxidative stress, thereby promoting inflammation by mediating the activity of the transcription factors NF-κB and AP-1, which are redox-sensitive (Yang et al., 2007). ROS influence the pathophysiology of various neurobehavioral disorders, but an imbalance of these antioxidant enzymes has been particularly shown to correlate with regressive ASD (Chauhan et al., 2004).
ROS production is closely linked to pro-inflammatory processes. Zinc can downregulate the production of inflammatory cytokines through NF-κB signaling (Foster and Samman, 2012). Throughout fetal development, zinc-deficient conditions result in increased inflammation and altered central nervous system (CNS) development (Sauer et al., 2016). Increased inflammation has been reported in many human and animal studies on ASD (Theoharides et al., 2016; Freitas et al., 2018).
Free Zn in the CNS is contained within various brain regions, with the highest concentration found in the hippocampus. It is then released into the synaptic cleft to regulate the activity of multiple postsynaptic receptors such as N-methyl-D-aspartate receptors (Chowanadisai et al., 2005; Stoll et al., 2007). Zinc may enter the postsynaptic compartment and is also released into the postsynapse from intracellular zinc stores in a synaptic-activity-dependent manner. Postsynaptic scaffold formation is a pivotal function of Zn mediated by proteins such as SHANK2 and SHANK3. These proteins are at the center of an ASD-linked postsynaptic signaling network (Grabrucker et al., 2014).
Also, neurogenesis is controlled by metal ion concentrations such as Fe, Cu, and Zn. In ASD, it is typically hindered due to effects in various pathways (Wang et al., 2019; Kumar et al., 2021). Zn deficiency has been shown to reduce the proliferative development of neuronal stem cells, leading to decreased cell survival (Pfaender et al., 2016). Even marginal Zn deficiency affects neurogenesis by altering the number of neurons and reducing neuronal specification (Kumar et al., 2021). Altered neurogenesis has also been implicated in ASD. Taken together, due to the various roles of metals in human physiology, the altered metal composition may have significant knock-on effects on many other processes that control proteostasis, gene expression, cell signaling, organ development, and metabolism.
Since metals occur in the human body as charged elements and ions cannot diffuse passively through biological membranes, their transport and storage are mediated by many proteins. Indeed, it has been estimated that 10% of the human genome encodes for zinc-binding proteins (Andreini et al., 2006). Therefore, if genetic mutations develop that alter the protein function of metal-binding proteins, they may influence the metallome and lead to an imbalance of metals in an individual (Zhang et al., 2019). Through this mechanism, the transcriptome (the set of all coding and non-coding RNA transcripts at a particular time in a specific location) and proteome (the set of proteins present at a specific time in a certain location) are tightly linked to the metallome. However, metal-binding proteins are found among the class of DNA transcription factors, DNA repair pathways, and proteins and enzymes regulating cellular signaling pathways resulting in the production or degradation of selected transcripts/proteins (Lin et al., 2006). Thus, the metallome also affects the genome, transcriptome, and proteome through various mechanisms.
A large proportion of studies have turned to research on the gut-brain axis in various neurobehavioral disorders. Through this, insights into how the gut microbiome influences the brain and neural signaling have been emerging (Mayer et al., 2014). Microbiomics comprises the investigation of microbial compositions within an individual at a certain time, specifically within different organs/tissues within individuals, such as the gut (Gilbert et al., 2018). The gut microbiome can influence gastrointestinal (GI) physiology along with inflammation and immune function (Vuong and Hsiao, 2017). Additionally, the microbiome has been thought to play a role in the inflammasome simultaneously. The inflammasome comprises an assembly cascade of immune cell inflammatory response cytokines such as NLRP’s (Nucleotide-binding oligomerization domain, Leucine rich Repeat and Pyrin domain containing), caspases, and interleukins (ILs) (Zheng et al., 2020). For example, the regulation of IL-18-mediated antimicrobial peptide production by the gut microbiome has been shown (Chen, 2017). The inflammasome may also be controlled by epigenomic effects, whereby post-translational modifications such as phosphorylation and histone ubiquitination affect many functions carried out by the inflammasome (Man and Kanneganti, 2015).
However, the microbiome, inflammasome, and epigenome (the chemical changes to an organism’s DNA and histone proteins) are also tightly linked to the metallome. Microbiota have needs for biometals, and the availability of these will influence the microbiota composition. For example, Zn deficiency promotes dysbiosis of the microbiota by favoring Enterobacterial colonization (Byndloss et al., 2018). On the other hand, microbiota composition will also affect the amount of trace metals available for the host, thereby affecting the metallome. The epigenome is also influenced by metal availability. The accumulation of metals such as Cd, Zn, and Cu have been shown to play a role in altering epigenetic outcomes, including DNA methylation, histone modification, and miRNAs (Mordaunt et al., 2019; Brito et al., 2020). Examples include the methylation of Metallothionein-1 (MT-1), whereby Cd changes the methylation patterns of the Hpa II sites within the MT-1 gene, which expression decreases in return (Compere and Palmiter, 1981; Wang et al., 2012), and alterations in the promoter region of metallothionein-2 (MT2) through changing histone modification in mice in response to Zn deficiency (Kurita et al., 2013). As previously mentioned, reactive oxygen species and oxidative stress are influenced by metal availability, which has been shown to play a role in the promotion and inhibition of inflammasome activation, particularly the NLRP3 inflammasome (Martinon, 2010; Chen et al., 2016; Fan et al., 2017). In addition, a close link between inflammatory processes and microbiota composition exists. In line with this, zinc deficiency resulted in altered microbiota composition and increased inflammation in a mouse model (Sauer et al., 2019).
The proteome, metallome, and microbiome are also key determinants of the metabolome (the complete set of small-molecule chemicals found within a biological sample at a certain time). Metabolomic research in ASD primarily focuses on small-molecule metabolites in the brain, plasma, and urine (Kurochkin et al., 2019; Needham et al., 2021). So far, it has been reported that the metabolome of an individual corresponds to the microbiome of the individual. However, the impacts of metallome dyshomeostasis have rarely been monitored in parallel with both the gut microbiome and fecal metabolome. Through its interaction with the proteome and microbiome, major effects of the metallome on the metabolome are expected that may play a role in neurodevelopmental disorders (Mounicou et al., 2009; Grabrucker, 2020a). For example, exposure to chronic lead (Pb) has been shown to alter gut microbiota composition (microbiome), along with changes in the expression of genes (transcriptome/proteome) related to lipid metabolism and significantly altered metabolite concentrations (metabolome) in mice (Xia et al., 2018).
The lipidome (the totality of lipids present at a certain time in a specific location) and glycome (the entire complement of sugars present at a certain time in a specific location) are subsets of the metabolome (Santoru et al., 2017; Zierer et al., 2018; Kurochkin et al., 2019). Previous studies have focused on the effects of an altered metal availability on the lipidomic profiles and subsequent impact on inflammation. Such an example includes a recent study demonstrating a high-fat diet in mice and a Zn sufficient diet, leading to a highly protective profile in both liver and plasma lipoproteins and a greater antiatherogenic response in the liver when compared to Zn deficient mice (Kostara et al., 2018). Further to this, another study using rats focused on the effects of excess Cd. Upon exposure to excess Cd, an increase was observed in hepatic cholesterol, phospholipids, and triglycerides (Sarmiento-Ortega et al., 2017). These studies suggest a close connection between the metallome and lipidome.
The vast majority of ASD-related studies based on “omics” approaches focus on a single “ome” that is evaluated and used to make predictions about possible pathomechanisms (Supplementary Table 1). However, as described above, the “omes” of the body are interlinked, whereby alterations in one field may affect numerous others.
Studies of cellular metal compositions and their associated proteins have been of increasing interest throughout recent years. In these studies, various factors have been considered, such as age, the severity of ASD, impacts of toxic metals on essential metals, and the presence of specific metalloproteins such as metallothioneins (Vergani et al., 2011; Al-Farsi et al., 2013; Macedoni-Lukšič et al., 2015; Hawari et al., 2020). However, since the direct measurement of metal concentrations in brain tissue of human participants with ASD is currently not achievable, most metallomics studies were performed using blood, hair, nail, or tooth samples. Unfortunately, the focus on the ASD population is mostly neglecting the crucial developmental window where trace metal abnormalities have most impact, which may be in utero, with only a few studies being able to measure the metallome during pregnancy. Despite the importance of metal homeostasis in development and neurological functions, few metallomics studies have been performed in animal models for ASD, which would allow a direct assessment of metals in the brain tissue. For example, while hypozincemia was detected in the maternal immune activation (MIA) rat model (Galvão et al., 2015), to our knowledge, no metallomics data is available from other rodent models for ASD despite zinc supplementation being able to rescue some ASD behaviors in MIA mice, valproic acid-treated rodent models, and Shank3 knockout mice (Grabrucker, 2020b).
However, some insights can be gained from epidemiological metallomics studies in ASD. For example, a metallomics study with 77 pairs of mothers and babies confirmed the relationship between the presence of toxic metals and levels of essential metals. The results demonstrated an accumulation of three specific toxic metals, lead, cadmium, and aluminum which decrease with age while showing a correlation between a deficiency in Zn and Mg and toxic metal accumulation in the offspring (Yasuda et al., 2020). This pattern, an increase in toxic metals, and a decrease in essential metals has been shown in a plethora of metallomics data. Many studies have shown that children with ASD display a higher body content of toxic metals such as Pb, Hg, and Cd compared to neurotypical controls (Cohen et al., 1982; Eppright et al., 1996; Filipek et al., 1999; Al-Ayadhi, 2005; Fido and Al-Saad, 2005; Nataf et al., 2006; Adams et al., 2007; Geier and Geier, 2007; Clark et al., 2010; Geier et al., 2010, 2012; Blaurock-Busch et al., 2011; Elsheshtawy et al., 2011; Lakshmi Priya and Geetha, 2011; Al-Farsi et al., 2013; Yasuda et al., 2013; Saghazadeh and Rezaei, 2017), and altered levels of essential metals, such as a decrease in Zn (Wecker et al., 1985; Blaurock-Busch et al., 2011; Elsheshtawy et al., 2011; Yasuda et al., 2011; Li et al., 2014; Tabatadze et al., 2015) and increase in Cu (Blaurock-Busch et al., 2011; Elsheshtawy et al., 2011; Lakshmi Priya and Geetha, 2011; Russo and deVito, 2011; Li et al., 2014; Tabatadze et al., 2015). In most studies, both a deficiency in Zn and an increase in Cu occur in the same samples. However, the results are sometimes inconsistent, and the quality of studies differs due to sample size, a study population that is limited to a specific region, differences in protocols such as measuring fasting vs. non-fasting blood samples, and methods such as using inductively coupled plasma mass spectrometry (ICP-MS) or atomic absorption spectroscopy (AAS). In addition, participants with ASD may be selected based on an independently obtained diagnosis, or more carefully assessed within a study, for example, regarding their autism severity. Nevertheless, three meta-analyses investigating a total of 19 different case control studies with ca. 1,516 participants (831 ASD, 685 controls) confirm that low Zn levels are associated with ASD (Sayehmiri et al., 2015; Babaknejad et al., 2016; Saghazadeh et al., 2017).
The probably most extensive study to date that investigated 1,967 hair samples of individuals with ASD found a clear correlation between age and abnormal metal levels (Yasuda et al., 2011, 2013). Intriguingly, in several studies, the severity of trace metal abnormalities was directly correlated to the severity of ASD symptoms (Elsheshtawy et al., 2011; Lakshmi Priya and Geetha, 2011; Li et al., 2014).
Although a recent meta-analysis investigating iron levels in individuals with and without ASD found no significant differences between the two groups (Tseng et al., 2018), a Chinese cohort study with 254 children that investigated whole blood trace metal levels demonstrated a significant difference between individuals with ASD and without, with a 5% higher Fe concentration in boys with ASD when compared to girls with ASD (Wu et al., 2019). A previous study had found similar differences using hair samples where a significant increase in Fe concentration was found in boys but not girls. Future studies should focus on the mechanism of this gender difference and evaluate whether the Fe level may be a suitable predictor of ASD in boys (Bener et al., 2017; Skalny et al., 2017). In addition, future studies will benefit from the correlation of metal abnormalities to specific features and severities of ASD symptoms in male and female participants (Fiore et al., 2020). Overall, to date, several high-quality studies that use ICP-MS, more than 100 participants, and correlate metallomics data with ASD severity (Russo and deVito, 2011; Arora et al., 2017) could link abnormal metal levels to ASD, despite some variability due to the various factors influencing the results of metallomics studies such as the age of the participants, diet, method of assessment, biosample used, and heterogeneity of the ASD cohort. From the studies and meta-analyses performed, abnormal Zn/Cu ratios consistently emerge as being linked to ASD. The detection of altered Cu/Zn ratios has been proposed as a biomarker for ASD (Faber et al., 2009). It may be complemented in the future by detection of other dysregulated metals defining an ASD-specific metal profile. Evaluating metal profiles has the advantage that early detection, and therefore, early intervention, may be possible as studies have shown that metal alterations are most prominent in children with ASD under the age of three (Yasuda et al., 2011) thus before most ASD diagnoses are established. In addition, detection of these metal profiles during pregnancy may not only allow intervention but even prevention.
Proteomic/transcriptomic studies in ASD investigate specific changes for the discovery of biomarkers or the identification of pathways that may contribute to the pathological features of ASD, such as oxidative stress, inflammation, and altered synaptic development and plasticity (Abraham et al., 2019b). Biomarker discovery is mostly made in human studies using easily accessible samples such as saliva, blood, and urine. In contrast, research on the pathomechanisms of ASD is mostly done with the help of cell culture and animal models, with few human studies using post-mortem cerebral tissue (Gandal et al., 2018; Schwede et al., 2018). These studies identified several significant differences in the proteome of individuals with ASD and model systems. For example, numerous genes/proteins were identified changed in these studies that have been implicated in metabolic functions, vesicular biology, mitochondria, and intracellular signaling. Pathway analysis using proteomics/transcriptomics data has also pointed to synaptic processes with significant alterations in glutamate (NMDA) receptor signaling identified in various brain regions. In addition, pathways involved in redox mechanisms and inflammatory processes were in the focus (Voineagu et al., 2011; Feng et al., 2017; Gandal et al., 2018; Schwede et al., 2018; Abraham et al., 2019a; Gordon et al., 2021; Hewitson et al., 2021). However, there is a lack of overlap between the genes/proteins identified due to the heterogeneity of ASD and different cohorts of varying ages and severity of symptoms used in human proteomic/transcriptomics studies (Wetie et al., 2015b; Cortelazzo et al., 2016). Major gene/protein alterations identified throughout the studies mentioned in Supplementary Table 1 include interleukins and inflammatory cytokines, myelination, mitochondrial, and synaptic proteins (Broek et al., 2014; Wetie et al., 2015a; Feng et al., 2017).
Transcriptomic analysis of both ASD mouse models and human postmortem brain tissue has additionally revealed the dysregulation of genes primarily involved in cellular stress responses, apoptosis, chromatin modification, and DNA repair. One such example includes 8-oxodeoxyguanosine, a heavily studied oxidative stress marker (Dizdaroglu et al., 2002). The increased expression is due to the inhibition of the Ogg1 protein through various possible mechanisms such as epigenetic histone modification or hypomethylation of the promoter sequence (Shpyleva et al., 2014). As previously mentioned, Zn and Cd dyshomeostasis are implicated in oxidative stress, contributing to the epigenetic mechanisms disrupting the Ogg1 expression (Karoui-Kharrat et al., 2017; Elwej et al., 2018). Several animal studies have been performed using Shank3 knockout mice, BTBR mice, NMDA receptor subunit NR1 knockdown mice, or FMR1 knockout mice. These studies connect the synaptic disruptions with an altered cortical expression of proteins that function in mRNA transport, neurotransmission, and synaptic plasticity (Liao et al., 2008). Another study used the MeCP2-deficient mouse model to mimic Rett syndrome, revealed alterations in cholesterol synthesis and metabolism (Pacheco et al., 2017). Data analysis of the transcriptome, in particular, found varying mRNA co-expression modules along with distinct miRNA expression patterns among different cohorts of individuals with ASD when compared to neurotypically developing individuals (Ramaswami et al., 2020). Network approaches used in the transcriptomic analysis can deduce functional roles of genes based on the accompanying network. For example, module M12 included the overrepresentation of various ASD susceptibility genes such as AHI1 and SLC25A12 (Voineagu et al., 2011). In general, proteomics and transcriptomics studies in ASD hint at abnormalities of synaptic proteins, proteins regulating mitochondrial function, and proteins mediating immune processes, as well as lipid metabolism (Abraham et al., 2019b).
Epigenomic studies in ASD have a focus on fetal development. Findings suggest an important role for epigenetic alterations in regulating the transcriptional level of gene modules (co-expressed genes) (Amiri et al., 2018). Epigenomic studies in ASD primarily focus on methylation and acetylation patterns commencing in utero. Various ASD-associated genes and receptors have been identified as the target of epigenetic alterations, such as SHANK3, MECP2, FMR1, etc. (Edamura and Pearson, 2005; Zhu et al., 2014; Yuksel et al., 2016; Lu et al., 2020). A histone acetylome-wide association study published in 2016 carried out ChIP-sequencing on 257 postmortem samples from individuals with ASD along with matched controls indicated that approximately 68% of ASD cases contained a mutual acetylome signature. Despite the heterogeneity of ASD etiology, acetylation patterns across cortical regions display similar alterations leaning toward collective downstream effects to the acetylome (Sun et al., 2016). Again, synaptic processes are targeted by many epigenetic modifications. For example, GRB10 contributes to a negative feedback loop that decreases mTORC1 signaling, contributing to ASD pathology through synaptic excitation and regulation. Thus, the deacetylation of GRB10 may influence a common synaptic pathway, thereby contributing to idiopathic ASD (Sun et al., 2016). A meta-analysis using blood samples carried out on 800 individuals with ASD suggested 55 significant CpG sites (Andrews et al., 2018). The major findings concluded from epigenomic studies show major hypomethylation and hypermethylation at specific loci in neuronal signaling and developmental genomic regions. Such an example includes the hypermethylation of the GAD1 promoter leading to an increase in promoter binding of MeCP2, which has been found in both human and relevant animal models in neurobehavioral disorders (Zhubi et al., 2014). This hypermethylation, in turn, has been linked further to prenatal immune activation (Labouesse et al., 2015).
Further research has indicated that dysregulation of microRNAs in the brain contributes to ASD pathogenesis. A study has found both hypomethylated and overexpressed microRNAs and the respective genes in ASD brain samples such as miR-142, miR-451a, and miR-144-3p which have been shown to play a role in synaptic function and also target the oxytocin receptor gene (Mor et al., 2015).
In humans, alterations in the metabolome of individuals with ASD have been assessed mainly through blood and urine samples. A study focused on gray matter in the prefrontal cortex and its metabolite composition has identified 16 altered metabolic pathways, including cysteine and methionine metabolism, glutathione metabolism, citric acid cycle, and various others, whereas urine and blood sampling identified 10 alterations such as purine and pyruvate metabolism, nicotinate and nicotinamide metabolism and galactose metabolism (Kurochkin et al., 2019). A recent study aimed to characterize metabolomic features in hyperpurinergic conditions using a maternal immune activation mouse model. Fifty percent of plasma metabolites (202/401) from 37 pathways were altered significantly, with xanthosine, dopamine, and L-isoleucine ranked as the most altered metabolites in the maternal immune activation mice (Zolkipli-Cunningham et al., 2021). A further study has focused on valproic acid-induced ASD rodent models. Particularly in the hippocampus, significant alterations were observed as prematurely as neonatal development, whereby 16 metabolites differed significantly, including glutamate, uracil, and N-acetylaspartate (Abreu et al., 2021). Another quantitative metabolomics study was carried out on mice. A total of 999 lipids were identified, where 13.7% showed a significant difference between ASD and typically developing controls. The most prominent lipids included glycerolipids, cholesterol, poly-unsaturated fatty acids, and phospholipids, where short-chain fatty acids were found in a lesser quantity in ASD (Needham et al., 2021). The overall findings reported in multiple studies are conflicted in terms of metabolomic profiles and gender. Further research will be required due to the inconsistency of the results (Ruoppolo et al., 2015; Diaz et al., 2016; Abreu et al., 2021; Courraud et al., 2021). Interestingly, alterations observed in infants from day 1 to 10 post-birth have been hypothesized to be linked to the microbiome and inflammasome (Courraud et al., 2021).
Microbiome analysis using human samples and rodent models has consistently revealed significant differences between individuals with ASD and the control group. However, a lack of consistency is observed regarding the specific microbiota profile of the ASD group. Many changes are found on a bacterium phylum level rather than a species level. However, microbiome analysis may play a significant role in the characterization of subgroups within the ASD population (Finegold et al., 2010; Golubeva et al., 2017; Sauer et al., 2019; Sgritta et al., 2019). Gut dysbiosis plays a substantial role in the pathology of ASD. A particular emphasis has been put on the microbiome in the early development of individuals and the factors which may influence the gut flora, including antibiotic use, nutrition intake, infections, stress, etc. (Sivamaruthi et al., 2020). A focus on the treatment and reintroduction of bacterial strains has shown promising results in animal studies, particularly in mice. Such an example includes the treatment of L. reuteri, whereby ASD-like symptoms were diminished and reversed (Buffington et al., 2016). A similar approach was used in germ-free mice, which decreased abnormal behavior once they administered human “infant type” Bifidobacterium species (Luk et al., 2018). Further studies and meta-analyses have focused on bacterial composition and ratios, finding an increased ratio of Firmicutes to Bacteroidetes in ASD cohorts compared to typically developing cohorts (Borre et al., 2014). Candida has been found in a significantly higher abundance, while Streptococcus, Prevotella, and Veillonella are significantly decreased compared to individuals without ASD (Kushak et al., 2017; Strati et al., 2017). However, most meta-analyses in human and mouse models failed to reveal ASD-specific signatures despite most studies showing significant differences in microbiota composition of individuals with ASD.
Immune responses in ASD are often altered and may play a prominent role with regard to GI issues and other symptoms experienced by individuals with ASD. Recent studies show that inflammation is highly linked to the symptoms and severity of ASD in the individual (Patel et al., 2018). NLRP3 and AIM2 inflammasomes have been implicated in this process (Saresella et al., 2016). The dysregulation of several cytokines is further involved in the pathology of ASD (Zhu et al., 2006; Raison et al., 2010). Alterations in inflammatory levels result from multiple systemic and external factors that affect immune activation, such as maternal immune activation and allergies, autoimmunity, genetics, and maternal exposure to toxic metals (Crespi and Thiselton, 2011; Wei et al., 2011; Garay et al., 2013; Frye, 2015). Recent reviews have focused on cytokine expression measured from neonatal blood samples and cerebral spinal fluid, whereby alternative cytokine levels have been identified across different studies. A consistent finding includes the increased expression of IL-6 and tumor necrosis factors (TNF) (Ashwood et al., 2011; Abdallah et al., 2013; Tsilioni et al., 2015; Abruzzo et al., 2019). Conversely, various other studies have found conflicting data on other cytokines using different sample types, potentially indicating an impact of age, gender, and severity of ASD in the individuals (Abdallah et al., 2012; Masi et al., 2017; Siniscalco et al., 2018; Saghazadeh et al., 2019).
Given that most “omes” are interlinked (Figure 1), it may be possible that the independently reported alterations indeed are part of one specific process that affects all “omes” of the body and that forms the biological core of the ASD pathology and reveals an ASD signature.
Figure 1. The metallome’s role in ASD can be expanded through its interactions with various other “omes.” Each arrow indicates a significant relationship whereby dyshomeostasis and further alterations in one impact other “omes” functionality.
In ASD, metal dyshomeostasis will affect the proteome, epigenome, microbiome, metabolome, and inflammasome. Together, directly or indirectly, the changes in these “omes” will affect biological targets resulting in the pathologies underpinning ASD. For example, zinc deficiency during pregnancy alters microbiota composition and increases inflammatory marker expression in the periphery but also in the brain (Sauer and Grabrucker, 2019). Thus, alterations in the metal profile will have indirect effects on brain functions by causing extracerebral changes. However, systemic zinc deficiency will also affect brain zinc levels. Therefore, zinc-dependent proteins within the brain will directly react to differences in metal concentrations, as shown in in vitro experiments (Grabrucker et al., 2014).
Among individuals with ASD, synaptopathies have been identified as a prominent feature (Keller et al., 2017). Synaptic pathologies include impaired synapse formation and maturation as well as plasticity, probably mediated by the dysregulation of glutamatergic ion channels, alterations in various pre-and postsynaptic scaffolding and cell adhesion proteins (e.g., Neurexin, Neurologin, SHANK3), and proteins that function in intracellular signaling (e.g., PTEN, mTOR) (van Spronsen and Hoogenraad, 2010; Yoo et al., 2014; Xing et al., 2019). In line with this, transcriptomics, proteomics, and epigenomic studies have identified various synaptic proteins altered in ASD.
Metals will also affect synaptic processes through their role as cofactors in enzymatic functions, causing and reducing oxidative stress and functioning as intracellular signaling ions and neurotransmitters. Zn deficiency, for example, reduces synaptic protein scaffold formation through direct binding to SHANK3 and modulates NMDA and AMPA receptor function (Grabrucker, 2014; Grabrucker et al., 2014; Li et al., 2018a). Furthermore, Zn may also modify epigenomic alterations carried out in fetal development, particularly in the generation of critical metabolites such as methionine and SAM, which carry out roles in DNA methylation. The generation of these metabolites relies on methyltransferases in which Zn acts as a cofactor in transferring methyl groups to homocysteine (Uriu-Adams and Keen, 2010; Sanusi et al., 2021). The epigenetic modifications may, in turn, affect the expression and function of synaptic proteins.
The stimulation of proinflammatory cytokine production, including IL-1β through lipopolysaccharide exposure during development, has been shown to promote metallothionein to sequester Zn leading to further hypozincemia in the fetus (Kirsten et al., 2015). Thus, inflammation may affect synapses indirectly by restricting Zn availability. However, Zn itself plays a role in inflammation, particularly in deficient conditions such as malnutrition (Gammoh and Rink, 2017). A prominent pathology identified in ASD is the increase in pro-inflammatory signaling. Studies have found a significant increase in inflammatory markers such as IL-6, IL-8, and TNF-α in ASD (Alzghoul et al., 2019). Through a regulatory role in pro-inflammatory signaling pathways, Zn levels influence cytokine expression. In addition, Zn deficiency was shown to increase gut permeability in mice provoking inflammation in the brain, identified by an increase in IL-6 and GFAP (Sauer and Grabrucker, 2019). Decreased bioavailability of Zn and may lead to NLRP3-driven inflammation. This is also evident in Alzheimer’s diseases and leads to cognitive decline in animal models, which may translate to ASD models (Pellegrini et al., 2020; Rivers-Auty et al., 2021). Increased cytokine expression and the activation of glial cells have been linked to synapse loss and modification of synaptic function (Kim et al., 2020).
With approximately 20% of dietary Zn absorbed by intestinal bacteria, alterations in gut microbial composition occur in response to Zn availability (Reed et al., 2015; Koren and Tako, 2020). Excess dietary Zn has been positively implicated in resistance to Clostridium difficile (Zackular et al., 2016). In addition, altered microbial compositions were observed in Zn deficient conditions whereby the levels of Veruccomicrobia were decreased, and Firmicutes and Actinobacteria levels were increased. Actinobacteria are classed as pathobionts and have implications in various inflammatory diseases such as IBD. Thus, also through effects on microbiota, the metallome may alter the inflammatory state.
Lipid profiles in ASD are altered significantly through oxidative stress, gut microbiome disruption, nutrient intake, and so on (Tamiji and Crawford, 2010; Mazahery et al., 2017). Studies have indicated an imbalance in polyunsaturated fatty acids, which may contribute to ASD-like behaviors (Tamiji and Crawford, 2010). A recent study focused on the reduction of metal-containing proteins such as ceruloplasmin (Cu) and Transferrin (Fe) observed in individuals with ASD, which increased lipid peroxidation. However, further research is required to conclude whether this reduction is region-specific in the brain similar to Cu and Fe levels (Chauhan et al., 2004). Another study has used serum analysis to identify alterations in lipoproteins linking essential metal ratios in the process. Results concluded a significant correlation between Zn/Cu ratios with high-density lipoproteins and total cholesterol levels (Al-Bazzaz et al., 2020). Lipid metabolism and the metabolome play a critical role in cellular structures and remodeling in disease states. Disruption in the lipidome, such as increased cholesterol, also leads to the activation of the NLRP3 inflammasome giving rise to inflammatory pathologies (Anand, 2020). In turn, the gut microbiome, which has been shown to alter the inflammasome, can also contribute to the lipidome and vice versa (Fu et al., 2015; Wang et al., 2016).
The interlinked alterations across each “ome” can be traced back to metal dysregulation while also connecting and affecting further profiles resulting in the heterogeneity of ASD pathogenesis. However, to find an ASD-specific signature and possibly biomarkers for ASD, more research needs to investigate multiple “omes” in the same model or individual with ASD to allow correlation studies. Until then, studies investigating at least two “omes” may be used to generate a map of overlapping alterations.
ASD is a disorder with a multifactorial etiology and highly variable clinical phenotype, where thus far, 1,231 genes have been implicated in ASD (Simons foundation autism research initiative—SFARI). To fully uncover the causes of ASD data, scientists can utilize mixed multi-omics data integration approaches to discover a biological process that would be affected by alterations across the various “omes.” So far, this method has been successfully implemented in a variety of complex diseases, such as cancer (Liu et al., 2013, 2016; Muqaku et al., 2017). Mixed multi-omics is a systematic biological data analysis approach, where ≥ 2 data sets or “omes” derived from various analytical techniques, such as proteomics, genomics, transcriptomics, epigenomics, metabolomics, and microbiomics, are integrated and studied together (Figure 2). By fusing and integrating the multiple “omes,” scientists can view and analyze complex, extensive biological data, uncover novel associations between biological entities, and identify biomarkers that can be utilized for disease or developmental condition early diagnosis and treatment development.
Figure 2. (A) Ever-growing popularity of multi-omics data analysis approach, shown as PubMed citation count from 2005 to 2020. (B) Mixed multi-omics data integration and analysis can pave the way for personalized medicine and biomarker discovery. This figure was created using Biorender.com.
Extracting meaningful information from each omics experiment with an extremely large number of data points is a computationally challenging task. This is further complicated by the vastly heterogeneous nature of biological data with non-linear interactions and shared effects on a multitude of factors, making it difficult to separate real biological signals from experimental noise. Noise may arise from the biological system being analyzed, the analytical platform used, and omics-specific analysis workflows. This challenge intensifies when mixed omics approaches are integrated together. The large-scale mixed multi-omics data integration requires careful data normalization, further statistical standardization, and even machine-learning tool application (Libbrecht and Noble, 2015; Min et al., 2017). Machine-learning tools are advantageous for analyzing amalgamated omics data and can carry out dimensionality reduction, clustering, the association of clinical measures, and disease prognosis (Li et al., 2018b).
Simple, graphical, and exploratory approaches such as principal component analysis (PCA) are often used to dimensionally reduce large data sets. In contrast, canonical correlation analysis (CCA) is utilized to study the overall correlation between two data sets. Additionally, other integrative frameworks for omics data utilize sparse CCA (Parkhomenko et al., 2009), multivariate partial least square analysis (Palermo et al., 2009), or multiple factor analysis (de Tayrac et al., 2009). Currently available tools for mixed multi-omics data integration can be partitioned into local and web-based applications, where local tools require R, Python, or Galaxy programming knowledge and are challenging to implement, these include mixOmics DIABLO/MINT, omicade4 or iOmicsPASS, and web-based applications such as, 3Omics, Paintomics, and Galaxy P/M which have user-friendly interfaces (Table 1). However, user-friendly web-based tools should only be used when the underlying methods are fully understood, as blind application often adversely affects progress in the field. At present, there is no single application or approach which can be applied for processing, analysis, and interpretation of data arising from integrated mixed multi-omics studies. Therefore, as computational biology is rapidly emerging, there is a need to develop amalgamated strategies and tools to aid in reproducible, high throughput, user-friendly, and effective platforms to study integrated mixed multi-omics data.
Taken together, combining results obtained from a multitude of individual “omics” studies may identify processes that lead to various disease states, but with one overlapping motif that may be the biological correlate of the shared features of ASD. With this knowledge, novel therapies could be developed targeting alternatives to the proteome, but with secondary effects on those protein networks currently in the focus of drug development in ASD (Park, 2020). An optimal starting point could include the metallome to counteract metal dyshomeostasis and, through its interaction with other “omes,” address symptoms emerging through effects of the microbiome, metabolome, lipidome, etc. Targeted metal supplementation or delivery of compounds targeting metal regulatory proteins may provide a critical instrument in developing therapeutic interventions due to the variety of roles of trace metals, for example, in histone modification, protein degradation, and inflammation (Chen et al., 2019). Given that metal dyshomeostasis has been identified in various diseases such as Alzheimer’s disease and Parkinson’s disease, those treatments may not be single-asset drugs. In addition, metal concentrations could provide valuable insight throughout pre-and early post-natal development as potential biomarkers of ASD since the metallome may serve as an indirect readout for alterations in the other, less accessible “omes.”
JS, SM, and AG drafted the manuscript. KM edited the manuscript. JS, SM, and AG prepared the figures. AG prepared the final version. All authors contributed to the article and approved the submitted version.
AG and JS were supported by the International Zinc Association (IZA) in collaboration with Zinpro Corp.
The authors declare that this study received funding from Zinpro Corp. The funder was not involved in the study design, collection, analysis, interpretation of data, the writing of this article, or the decision to submit it for publication.
The Supplementary Material for this article can be found online at: https://www.frontiersin.org/articles/10.3389/fnmol.2021.695873/full#supplementary-material
Abdallah, M. W., Larsen, N., Grove, J., Nørgaard-Pedersen, B., Thorsen, P., Mortensen, E. L., et al. (2013). Amniotic fluid inflammatory cytokines: Potential markers of immunologic dysfunction in autism spectrum disorders. World J. Biol. Psychiatry 14, 528–538. doi: 10.3109/15622975.2011.639803
Abdallah, M. W., Larsen, N., Mortensen, E. L., Atladóttir, H. Ó, Nørgaard-Pedersen, B., Bonefeld-Jørgensen, E. C., et al. (2012). Neonatal levels of cytokines and risk of autism spectrum disorders: An exploratory register-based historic birth cohort study utilizing the Danish Newborn Screening Biobank. J. Neuroimmunol. 252, 75–82. doi: 10.1016/j.jneuroim.2012.07.013
Abraham, J. R., Szoko, N., Barnard, J., Rubin, R. A., Schlatzer, D., Lundberg, K., et al. (2019a). Proteomic investigations of autism brain identify known and novel pathogenetic processes. Sci. Rep. 9:13118. doi: 10.1038/s41598-019-49533-y
Abraham, J., Szoko, N., and Natowicz, M. R. (2019b). “Proteomic Investigations of Autism Spectrum Disorder: Past Findings, Current Challenges, and Future Prospects,” in Reviews on Biomarker Studies in Psychiatric and Neurodegenerative Disorders Advances in Experimental Medicine and Biology, ed. P. C. Guest (Cham: Springer International Publishing), 235–252.
Abreu, A. C., Navas, M. M., Fernández, C. P., Sánchez-Santed, F., and Fernández, I. (2021). NMR-based metabolomics approach to explore brain metabolic changes induced by prenatal exposure to autism-inducing chemicals. ACS Chem. Biol. 16, 753–765. doi: 10.1021/acschembio.1c00053
Abruzzo, P. M., Matté, A., Bolotta, A., Federti, E., Ghezzo, A., Guarnieri, T., et al. (2019). Plasma peroxiredoxin changes and inflammatory cytokines support the involvement of neuro-inflammation and oxidative stress in Autism Spectrum Disorder. J. Transl. Med. 17:332. doi: 10.1186/s12967-019-2076-z
Adams, J. B., Romdalvik, J., Ramanujam, V. M. S., and Legator, M. S. (2007). Mercury, lead, and zinc in baby teeth of children with autism versus controls. J. Toxicol. Environ. Health A 70, 1046–1051. doi: 10.1080/15287390601172080
Al-Ayadhi, L. Y. (2005). Heavy metals and trace elements in hair samples of autistic children in central Saudi Arabia. Neurosci. Riyadh Saudi Arab. 10, 213–218.
Al-Bazzaz, A., A-Dahir, K., Almashhadani, A., and Al-Ani, I. (2020). Estimation of fasting serum levels of glucose, zinc, copper, zinc/copper ratio and their relation to the measured lipid profile in autistic patients and non-autistic controls in Jordan. Biomed. Pharmacol. J. 13, 481–488.
Al-Farsi, Y. M., Waly, M. I., Al-Sharbati, M. M., Al-Shafaee, M. A., Al-Farsi, O. A., Al-Khaduri, M. M., et al. (2013). Levels of heavy metals and essential minerals in hair samples of children with autism in oman: a case–control study. Biol. Trace Elem. Res. 151, 181–186. doi: 10.1007/s12011-012-9553-z
Alghadir, A. H., Gabr, S. A., and Al-Eisa, E. (2016). Effects of physical activity on trace elements and depression related biomarkers in children and adolescents. Biol. Trace Elem. Res. 172, 299–306. doi: 10.1007/s12011-015-0601-3
Alzghoul, L., Abdelhamid, S. S., Yanis, A. H., Qwaider, Y. Z., Aldahabi, M., Albdour, S. A., et al. (2019). The association between levels of inflammatory markers in autistic children compared to their unaffected siblings and unrelated healthy controls. Turk. J. Med. Sci. 49, 1047–1053. doi: 10.3906/sag-1812-167
Amiri, A., Coppola, G., Scuderi, S., Wu, F., Roychowdhury, T., Liu, F., et al. (2018). Transcriptome and epigenome landscape of human cortical development modeled in brain organoids. Science 362:eaat6720. doi: 10.1126/science.aat6720
Anand, P. K. (2020). Lipids, inflammasomes, metabolism, and disease. Immunol. Rev. 297, 108–122. doi: 10.1111/imr.12891
Andreini, C., Banci, L., Bertini, I., and Rosato, A. (2006). Counting the zinc-proteins encoded in the human genome. J. Proteome Res. 5, 196–201. doi: 10.1021/pr050361j
Andrews, S. V., Sheppard, B., Windham, G. C., Schieve, L. A., Schendel, D. E., Croen, L. A., et al. (2018). Case-control meta-analysis of blood DNA methylation and autism spectrum disorder. Mol. Autism 9:40. doi: 10.1186/s13229-018-0224-6
Arora, M., Reichenberg, A., Willfors, C., Austin, C., Gennings, C., Berggren, S., et al. (2017). Fetal and postnatal metal dysregulation in autism. Nat. Commun. 8:15493. doi: 10.1038/ncomms15493
Ashwood, P., Krakowiak, P., Hertz-Picciotto, I., Hansen, R., Pessah, I., and de Water, J. V. (2011). Elevated plasma cytokines in autism spectrum disorders provide evidence of immune dysfunction and are associated with impaired behavioral outcome. Brain. Behav. Immun. 25:40. doi: 10.1016/j.bbi.2010.08.003
Auffray, C., Balling, R., Barroso, I., Bencze, L., Benson, M., Bergeron, J., et al. (2016). Making sense of big data in health research: towards an EU action plan. Genome Med. 8:71. doi: 10.1186/s13073-016-0323-y
Babaknejad, N., Sayehmiri, F., Sayehmiri, K., Mohamadkhani, A., and Bahrami, S. (2016). The relationship between zinc levels and autism: a systematic review and meta-analysis. Iran. J. Child Neurol. 10, 1–9.
Bener, A., Khattab, A. O., Bhugra, D., and Hoffmann, G. F. (2017). Iron and vitamin D levels among autism spectrum disorders children. Ann. Afr. Med. 16, 186–191. doi: 10.4103/aam.aam_17_17
Betancur, C. (2011). Etiological heterogeneity in autism spectrum disorders: more than 100 genetic and genomic disorders and still counting. Brain Res. 1380, 42–77. doi: 10.1016/j.brainres.2010.11.078
Bjorklund, G. (2013). The role of zinc and copper in autism spectrum disorders. Acta Neurobiol. Exp. 73, 225–236.
Blaurock-Busch, E., Amin, O. R., and Rabah, T. (2011). Heavy metals and trace elements in hair and urine of a sample of arab children with autistic spectrum disorder. Mćdica 6, 247–257.
Borre, Y. E., O’Keeffe, G. W., Clarke, G., Stanton, C., Dinan, T. G., and Cryan, J. F. (2014). Microbiota and neurodevelopmental windows: implications for brain disorders. Trends Mol. Med. 20, 509–518. doi: 10.1016/j.molmed.2014.05.002
Brito, S., Lee, M.-G., Bin, B.-H., and Lee, J.-S. (2020). Zinc and Its transporters in epigenetics. Mol. Cells 43, 323–330. doi: 10.14348/molcells.2020.0026
Broek, J. A., Guest, P. C., Rahmoune, H., and Bahn, S. (2014). Proteomic analysis of post mortem brain tissue from autism patients: evidence for opposite changes in prefrontal cortex and cerebellum in synaptic connectivity-related proteins. Mol. Autism 5:41. doi: 10.1186/2040-2392-5-41
Buffington, S. A., Di Prisco, G. V., Auchtung, T. A., Ajami, N. J., Petrosino, J. F., and Costa-Mattioli, M. (2016). Microbial reconstitution reverses maternal diet-induced social and synaptic deficits in offspring. Cell 165, 1762–1775. doi: 10.1016/j.cell.2016.06.001
Byndloss, M. X., Pernitzsch, S. R., and Bäumler, A. J. (2018). Healthy hosts rule within: ecological forces shaping the gut microbiota. Mucosal. Immunol. 11, 1299–1305. doi: 10.1038/s41385-018-0010-y
Chauhan, A., Chauhan, V., Brown, W. T., and Cohen, I. (2004). Oxidative stress in autism: increased lipid peroxidation and reduced serum levels of ceruloplasmin and transferrin - the antioxidant proteins. Life Sci. 75, 2539–2549. doi: 10.1016/j.lfs.2004.04.038
Chen, A. Y., Adamek, R. N., Dick, B. L., Credille, C. V., Morrison, C. N., and Cohen, S. M. (2019). Targeting metalloenzymes for therapeutic intervention. Chem. Rev. 119, 1323–1455. doi: 10.1021/acs.chemrev.8b00201
Chen, G. Y. (2017). Regulation of the gut microbiome by inflammasomes. Free Radic. Biol. Med. 105, 35–40. doi: 10.1016/j.freeradbiomed.2016.11.011
Chen, H., Lu, Y., Cao, Z., Ma, Q., Pi, H., Fang, Y., et al. (2016). Cadmium induces NLRP3 inflammasome-dependent pyroptosis in vascular endothelial cells. Toxicol. Lett. 246, 7–16. doi: 10.1016/j.toxlet.2016.01.014
Chowanadisai, W., Kelleher, S. L., and Lönnerdal, B. (2005). Maternal zinc deficiency reduces NMDA receptor expression in neonatal rat brain, which persists into early adulthood. J. Neurochem. 94, 510–519. doi: 10.1111/j.1471-4159.2005.03246.x
Clark, B., Vandermeer, B., Simonetti, A., and Buka, I. (2010). Is lead a concern in Canadian autistic children? Paediatr. Child Health 15, 17–22.
Cohen, D. J., Paul, R., Anderson, G. M., and Harcherik, D. F. (1982). Blood lead in autistic children. Lancet 2, 94–95. doi: 10.1016/s0140-6736(82)91707-x
Compere, S. J., and Palmiter, R. D. (1981). DNA methylation controls the inducibility of the mouse metallothionein-I gene in lymphoid cells. Cell 25, 233–240. doi: 10.1016/0092-8674(81)90248-8
Cortelazzo, A., Felice, C. D., Guerranti, R., Signorini, C., Leoncini, S., Zollo, G., et al. (2016). Expression and oxidative modifications of plasma proteins in autism spectrum disorders: Interplay between inflammatory response and lipid peroxidation. Proteomics Clin. Appl. 10, 1103–1112. doi: 10.1002/prca.201500076
Cortese, M. M., Suschek, C. V., Wetzel, W., Kröncke, K.-D., and Kolb-Bachofen, V. (2008). Zinc protects endothelial cells from hydrogen peroxide via Nrf2-dependent stimulation of glutathione biosynthesis. Free Radic. Biol. Med. 44, 2002–2012. doi: 10.1016/j.freeradbiomed.2008.02.013
Cortese, S., Angriman, M., Lecendreux, M., and Konofal, E. (2012). Iron and attention deficit/hyperactivity disorder: what is the empirical evidence so far? A systematic review of the literature. Expert Rev. Neurother. 12, 1227–1240. doi: 10.1586/ern.12.116
Courraud, J., Ernst, M., Svane Laursen, S., Hougaard, D. M., and Cohen, A. S. (2021). Studying autism using untargeted metabolomics in newborn screening samples. J. Mol. Neurosci. 2021:2. doi: 10.1007/s12031-020-01787-2
Crespi, B. J., and Thiselton, D. L. (2011). Comparative immunogenetics of autism and schizophrenia. Genes Brain Behav. 10, 689–701. doi: 10.1111/j.1601-183X.2011.00710.x
de Tayrac, M., Lê, S., Aubry, M., Mosser, J., and Husson, F. (2009). Simultaneous analysis of distinct Omics data sets with integration of biological knowledge: multiple factor analysis approach. BMC Genom. 10:32. doi: 10.1186/1471-2164-10-32
Diaz, S. O., Pinto, J., Barros, A. S., Morais, E., Duarte, D., Negrão, F., et al. (2016). Newborn urinary metabolic signatures of prematurity and other disorders: a case control study. J. Proteome Res. 15, 311–325. doi: 10.1021/acs.jproteome.5b00977
Dizdaroglu, M., Jaruga, P., Birincioglu, M., and Rodriguez, H. (2002). Free radical-induced damage to DNA: mechanisms and measurement1, 2 1This article is part of a series of reviews on “Oxidative DNA Damage and Repair.” The full list of papers may be found on the homepage of the journal. 2Guest Editor: Miral Dizdaroglu. Free Radic. Biol. Med. 32, 1102–1115. doi: 10.1016/S0891-5849(02)00826-2
Edamura, K. N., and Pearson, C. E. (2005). DNA Methylation and replication: implications for the “Deletion Hotspot” region of FMR1. Hum. Genet. 2, 301–304. doi: 10.1007/s00439-005-0037-5
Elsheshtawy, E., Tobar, S., Sherra, K., Atallah, S., and Elkasaby, R. (2011). Study of some biomarkers in hair of children with autism. Middle East Curr. Psychiatry 18, 6–10. doi: 10.1097/01.XME.0000392842.64112.64
Elwej, A., Ghorbel, I., Chaabane, M., Soudani, N., Mnif, H., Boudawara, T., et al. (2018). Zinc and selenium modulate barium-induced oxidative stress, cellular injury and membrane-bound ATPase in the cerebellum of adult rats and their offspring during late pregnancy and early postnatal periods. Arch. Physiol. Biochem. 124, 237–246. doi: 10.1080/13813455.2017.1392579
Eppright, T. D., Sanfacon, J. A., and Horwitz, E. A. (1996). Attention deficit hyperactivity disorder, infantile autism, and elevated blood-lead: a possible relationship. Mo. Med. 93, 136–138.
Faber, S., Zinn, G. M., Kern, J. C., and Kingston, H. M. (2009). The plasma zinc/serum copper ratio as a biomarker in children with autism spectrum disorders. Biomarkers 14, 171–180. doi: 10.1080/13547500902783747
Fan, Y., Zhang, X., Yang, L., Wang, J., Hu, Y., Bian, A., et al. (2017). Zinc inhibits high glucose-induced NLRP3 inflammasome activation in human peritoneal mesothelial cells. Mol. Med. Rep. 16, 5195–5202. doi: 10.3892/mmr.2017.7236
Feng, C., Chen, Y., Pan, J., Yang, A., Niu, L., Min, J., et al. (2017). Redox proteomic identification of carbonylated proteins in autism plasma: insight into oxidative stress and its related biomarkers in autism. Clin. Proteomics 14:2. doi: 10.1186/s12014-017-9138-0
Fido, A., and Al-Saad, S. (2005). Toxic trace elements in the hair of children with autism. Autism Int. J. Res. Pract. 9, 290–298. doi: 10.1177/1362361305053255
Filipek, P. A., Accardo, P. J., Baranek, G. T., Cook, E. H., Dawson, G., Gordon, B., et al. (1999). The screening and diagnosis of autistic spectrum disorders. J. Autism Dev. Disord. 29, 439–484. doi: 10.1023/A:1021943802493
Finegold, S. M., Dowd, S. E., Gontcharova, V., Liu, C., Henley, K. E., Wolcott, R. D., et al. (2010). Pyrosequencing study of fecal microflora of autistic and control children. Anaerobe 16, 444–453. doi: 10.1016/j.anaerobe.2010.06.008
Fiore, M., Barone, R., Copat, C., Grasso, A., Cristaldi, A., Rizzo, R., et al. (2020). Metal and essential element levels in hair and association with autism severity. J. Trace Elem. Med. Biol. 57:126409. doi: 10.1016/j.jtemb.2019.126409
Foster, M., and Samman, S. (2012). Zinc and regulation of inflammatory cytokines: implications for cardiometabolic disease. Nutrients 4, 676–694. doi: 10.3390/nu4070676
Freitas, B. C., Mei, A., Mendes, A. P. D., Beltrão-Braga, P. C. B., and Marchetto, M. C. (2018). Modeling inflammation in autism spectrum disorders using stem cells. Front. Pediatr. 6:394. doi: 10.3389/fped.2018.00394
Frye, R. E. (2015). Metabolic and mitochondrial disorders associated with epilepsy in children with autism spectrum disorder. Epilepsy Behav. 47, 147–157. doi: 10.1016/j.yebeh.2014.08.134
Fu, J., Bonder, M. J., Cenit, M. C., Tigchelaar, E. F., Maatman, A., Dekens, J. A. M., et al. (2015). The gut microbiome contributes to a substantial proportion of the variation in blood lipids. Circ. Res. 117, 817–824. doi: 10.1161/CIRCRESAHA.115.306807
Galvão, M. C., Chaves-Kirsten, G. P., Queiroz-Hazarbassanov, N., Carvalho, V. M., Bernardi, M. M., and Kirsten, T. B. (2015). Prenatal zinc reduces stress response in adult rat offspring exposed to lipopolysaccharide during gestation. Life Sci. 120, 54–60. doi: 10.1016/j.lfs.2014.10.019
Gammoh, N. Z., and Rink, L. (2017). Zinc in infection and inflammation. Nutrients 9:624. doi: 10.3390/nu9060624
Gandal, M. J., Zhang, P., Hadjimichael, E., Walker, R. L., Chen, C., Liu, S., et al. (2018). Transcriptome-wide isoform-level dysregulation in ASD, schizophrenia, and bipolar disorder. Science 362:aat8127. doi: 10.1126/science.aat8127
Garay, P. A., Hsiao, E. Y., Patterson, P. H., and McAllister, A. K. (2013). Maternal immune activation causes age- and region-specific changes in brain cytokines in offspring throughout development. Brain. Behav. Immun. 31, 54–68. doi: 10.1016/j.bbi.2012.07.008
Geier, D. A., Audhya, T., Kern, J. K., and Geier, M. R. (2010). Blood mercury levels in autism spectrum disorder: Is there a threshold level? Acta Neurobiol. Exp. 70, 177–186.
Geier, D. A., and Geier, M. R. (2007). A prospective study of mercury toxicity biomarkers in autistic spectrum disorders. J. Toxicol. Environ. Health A 70, 1723–1730. doi: 10.1080/15287390701457712
Geier, D. A., Kern, J. K., King, P. G., Sykes, L. K., and Geier, M. R. (2012). Hair toxic metal concentrations and autism spectrum disorder severity in young children. Int. J. Environ. Res. Public. Health 9, 4486–4497. doi: 10.3390/ijerph9124486
Gilbert, J. A., Blaser, M. J., Caporaso, J. G., Jansson, J. K., Lynch, S. V., and Knight, R. (2018). Current understanding of the human microbiome. Nat. Med. 24, 392–400. doi: 10.1038/nm.4517
Golubeva, A. V., Joyce, S. A., Moloney, G., Burokas, A., Sherwin, E., Arboleya, S., et al. (2017). Microbiota-related changes in bile acid & tryptophan metabolism are associated with gastrointestinal dysfunction in a mouse model of autism. EBioMedicine 24, 166–178. doi: 10.1016/j.ebiom.2017.09.020
Gordon, A., Forsingdal, A., Klewe, I. V., Nielsen, J., Didriksen, M., Werge, T., et al. (2021). Transcriptomic networks implicate neuronal energetic abnormalities in three mouse models harboring autism and schizophrenia-associated mutations. Mol. Psychiatry 26, 1520–1534. doi: 10.1038/s41380-019-0576-0
Grabrucker, A. M. (2014). A role for synaptic zinc in ProSAP/Shank PSD scaffold malformation in autism spectrum disorders. Dev. Neurobiol. 74, 136–146. doi: 10.1002/dneu.22089
Grabrucker, A. M. (2016). “Zinc in the developing brain,” in Nutrition and the developing brain, eds V. H. Moran and N. M. Lowe (Boca Raton, FL: CRC Press), 143–168.
Grabrucker, A. M. (2020b). “Chapter 10: Animal models for autism—links to biometal abnormalities,” in Biometals in Autism Spectrum Disorders, ed. A. M. Grabrucker (Cambridge, MA: Academic Press), 149–157.
Grabrucker, S., Jannetti, L., Eckert, M., Gaub, S., Chhabra, R., Pfaender, S., et al. (2014). Zinc deficiency dysregulates the synaptic ProSAP/Shank scaffold and might contribute to autism spectrum disorders. Brain J. Neurol. 137, 137–152. doi: 10.1093/brain/awt303
Graham, S. F., Nasaruddin, M. B., Carey, M., Holscher, C., McGuinness, B., Kehoe, P. G., et al. (2014). Age-Associated changes of brain copper, iron, and zinc in Alzheimer’s disease and dementia with lewy bodies. J. Alzheimers Dis. 42, 1407–1413. doi: 10.3233/JAD-140684
Greenough, M. A., Camakaris, J., and Bush, A. I. (2013). Metal dyshomeostasis and oxidative stress in Alzheimer’s disease. Neurochem. Int. 62, 540–555. doi: 10.1016/j.neuint.2012.08.014
Ha, H. T. T., Leal-Ortiz, S., Lalwani, K., Kiyonaka, S., Hamachi, I., Mysore, S. P., et al. (2018). Shank and zinc mediate an AMPA receptor subunit switch in developing neurons. Front. Mol. Neurosci. 11:405. doi: 10.3389/fnmol.2018.00405
Hagmeyer, S., Sauer, A. K., and Grabrucker, A. M. (2018). Prospects of zinc supplementation in autism spectrum disorders and shankopathies such as phelan mcdermid syndrome. Front. Synaptic Neurosci. 10:11. doi: 10.3389/fnsyn.2018.00011
Hawari, I., Eskandar, M. B., and Alzeer, S. (2020). The role of lead, manganese, and zinc in Autism Spectrum Disorders (ASDs) and Attention-Deficient Hyperactivity Disorder (ADHD): a case-control study on syrian children affected by the syrian crisis. Biol. Trace Elem. Res. 197, 107–114. doi: 10.1007/s12011-020-02146-3
Hewitson, L., Mathews, J. A., Devlin, M., Schutte, C., Lee, J., and German, D. C. (2021). Blood biomarker discovery for autism spectrum disorder: A proteomic analysis. PLoS One 16:e0246581. doi: 10.1371/journal.pone.0246581
Kang, Y. J. (2006). Metallothionein redox cycle and function. Exp. Biol. Med. 231, 1459–1467. doi: 10.1177/153537020623100903
Karoui-Kharrat, D., Kaddour, H., Hamdi, Y., Mokni, M., Amri, M., and Mezghani, S. (2017). Response of antioxidant enzymes to cadmium-induced cytotoxicity in rat cerebellar granule neurons. Open Life Sci. 12, 113–119. doi: 10.1515/biol-2017-0013
Keller, R., Basta, R., Salerno, L., and Elia, M. (2017). Autism, epilepsy, and synaptopathies: a not rare association. Neurol. Sci. 38, 1353–1361. doi: 10.1007/s10072-017-2974-x
Kim, Y. S., Choi, J., and Yoon, B.-E. (2020). Neuron-Glia interactions in neurodevelopmental disorders. Cells 9:2176. doi: 10.3390/cells9102176
Kirsten, T. B., Chaves-Kirsten, G. P., Bernardes, S., Scavone, C., Sarkis, J. E., Bernardi, M. M., et al. (2015). Lipopolysaccharide exposure induces maternal hypozincemia, and prenatal zinc treatment prevents autistic-like behaviors and disturbances in the striatal dopaminergic and mTOR systems of offspring. PLoS One 10:e0134565. doi: 10.1371/journal.pone.0134565
Koh, H. W. L., Fermin, D., Vogel, C., Choi, K. P., Ewing, R. M., and Choi, H. (2019). iOmicsPASS: network-based integration of multiomics data for predictive subnetwork discovery. NPJ Syst. Biol. Appl. 5:22. doi: 10.1038/s41540-019-0099-y
Koren, O., and Tako, E. (2020). Chronic dietary zinc deficiency alters gut microbiota composition and function. Proceedings 61:16. doi: 10.3390/IECN2020-06993
Kostara, C. E., Lekkas, P., Vezyraki, P., Angelidis, C., Deligiannis, I.-K., Bairaktari, E. T., et al. (2018). Lipidome of plasma lipoproteins and liver is zinc- modulated in high fat diet treated mice. J. Trace Elem. Med. Biol. 50, 268–275. doi: 10.1016/j.jtemb.2018.07.007
Krämer, A., Green, J., Pollard, J., and Tugendreich, S. (2014). Causal analysis approaches in ingenuity pathway analysis. Bioinforma. Oxf. Engl. 30, 523–530. doi: 10.1093/bioinformatics/btt703
Kumar, V., Kumar, A., Singh, K., Avasthi, K., and Kim, J.-J. (2021). Neurobiology of zinc and its role in neurogenesis. Eur. J. Nutr. 60, 55–64. doi: 10.1007/s00394-020-02454-3
Kuo, T.-C., Tian, T.-F., and Tseng, Y. J. (2013). 3Omics: a web-based systems biology tool for analysis, integration and visualization of human transcriptomic, proteomic and metabolomic data. BMC Syst. Biol. 7:64. doi: 10.1186/1752-0509-7-64
Kurita, H., Ohsako, S., Hashimoto, S., Yoshinaga, J., and Tohyama, C. (2013). Prenatal zinc deficiency-dependent epigenetic alterations of mouse metallothionein-2 gene. J. Nutr. Biochem. 24, 256–266. doi: 10.1016/j.jnutbio.2012.05.013
Kurochkin, I., Khrameeva, E., Tkachev, A., Stepanova, V., Vanyushkina, A., Stekolshchikova, E., et al. (2019). Metabolome signature of autism in the human prefrontal cortex. Commun. Biol. 2, 1–10. doi: 10.1038/s42003-019-0485-4
Kushak, R. I., Winter, H. S., Buie, T. M., Cox, S. B., Phillips, C. D., and Ward, N. L. (2017). Analysis of the duodenal microbiome in autistic individuals: association with carbohydrate digestion. J. Pediatr. Gastroenterol. Nutr. 64, e110–e116. doi: 10.1097/MPG.0000000000001458
Labouesse, M. A., Dong, E., Grayson, D. R., Guidotti, A., and Meyer, U. (2015). Maternal immune activation induces GAD1 and GAD2 promoter remodeling in the offspring prefrontal cortex. Epigenetics 10, 1143–1155. doi: 10.1080/15592294.2015.1114202
Lakshmi Priya, M. D., and Geetha, A. (2011). Level of trace elements (Copper, Zinc, Magnesium and Selenium) and toxic elements (lead and mercury) in the hair and nail of children with autism. Biol. Trace Elem. Res. 142, 148–158. doi: 10.1007/s12011-010-8766-2
Li, H., Zhang, J., and Niswander, L. (2018a). Zinc deficiency causes neural tube defects through attenuation of p53 ubiquitylation. Development 145:dev169797. doi: 10.1242/dev.169797
Li, Y., Wu, F.-X., and Ngom, A. (2018b). A review on machine learning principles for multi-view biological data integration. Brief. Bioinform. 19, 325–340. doi: 10.1093/bib/bbw113
Li, S., Wang, J., Bjørklund, G., Zhao, W., and Yin, C. (2014). Serum copper and zinc levels in individuals with autism spectrum disorders. Neuroreport 25, 1216–1220. doi: 10.1097/WNR.0000000000000251
Liao, L., Park, S. K., Xu, T., Vanderklish, P., and Yates, J. R. (2008). Quantitative proteomic analysis of primary neurons reveals diverse changes in synaptic protein content in fmr1 knockout mice. Proc. Natl. Acad. Sci. U. S. A. 105, 15281–15286. doi: 10.1073/pnas.0804678105
Libbrecht, M. W., and Noble, W. S. (2015). Machine learning applications in genetics and genomics. Nat. Rev. Genet. 16, 321–332. doi: 10.1038/nrg3920
Lin, H., Han, L., Zhang, H., Zheng, C., Xie, B., Cao, Z., et al. (2006). Prediction of the functional class of metal-binding proteins from sequence derived physicochemical properties by support vector machine approach. BMC Bioinform. 7:S13. doi: 10.1186/1471-2105-7-S5-S13
Liu, G., Dong, C., and Liu, L. (2016). Integrated Multiple “-omics” data reveal subtypes of hepatocellular carcinoma. PloS One 11:e0165457. doi: 10.1371/journal.pone.0165457
Liu, Y., Devescovi, V., Chen, S., and Nardini, C. (2013). Multilevel omic data integration in cancer cell lines: advanced annotation and emergent properties. BMC Syst. Biol. 7:14. doi: 10.1186/1752-0509-7-14
Lord, C., Brugha, T. S., Charman, T., Cusack, J., Dumas, G., Frazier, T., et al. (2020). Autism spectrum disorder. Nat. Rev. Dis. Primer 6, 1–23. doi: 10.1038/s41572-019-0138-4
Lu, Z., Liu, Z., Mao, W., Wang, X., Zheng, X., Chen, S., et al. (2020). Locus-specific DNA methylation of Mecp2 promoter leads to autism-like phenotypes in mice. Cell Death Dis. 11, 1–11. doi: 10.1038/s41419-020-2290-x
Luk, B., Veeraragavan, S., Engevik, M., Balderas, M., Major, A., Runge, J., et al. (2018). Postnatal colonization with human “infant-type” Bifidobacterium species alters behavior of adult gnotobiotic mice. PLoS One 13:e0196510. doi: 10.1371/journal.pone.0196510
Macedoni-Lukšič, M., Gosar, D., Bjørklund, G., Oražem, J., Kodrič, J., Lešnik-Musek, P., et al. (2015). Levels of metals in the blood and specific porphyrins in the urine in children with autism spectrum disorders. Biol. Trace Elem. Res. 163, 2–10. doi: 10.1007/s12011-014-0121-6
Man, S. M., and Kanneganti, T.-D. (2015). Regulation of inflammasome activation. Immunol. Rev. 265, 6–21. doi: 10.1111/imr.12296
Marreiro, D., do, N., Cruz, K. J. C., Morais, J. B. S., Beserra, J. B., Severo, J. S., et al. (2017). Zinc and oxidative stress: current mechanisms. Antioxid. Basel Switz 6:24. doi: 10.3390/antiox6020024
Martinon, F. (2010). Signaling by ROS drives inflammasome activation. Eur. J. Immunol. 40, 616–619. doi: 10.1002/eji.200940168
Masi, A., Breen, E. J., Alvares, G. A., Glozier, N., Hickie, I. B., Hunt, A., et al. (2017). Cytokine levels and associations with symptom severity in male and female children with autism spectrum disorder. Mol. Autism 8:63. doi: 10.1186/s13229-017-0176-2
Mayer, E. A., Knight, R., Mazmanian, S. K., Cryan, J. F., and Tillisch, K. (2014). Gut microbes and the brain: paradigm shift in neuroscience. J. Neurosci. 34, 15490–15496. doi: 10.1523/JNEUROSCI.3299-14.2014
Mazahery, H., Stonehouse, W., Delshad, M., Kruger, M. C., Conlon, C. A., Beck, K. L., et al. (2017). Relationship between long chain n-3 Polyunsaturated fatty acids and autism spectrum disorder: systematic review and meta-analysis of case-control and randomised controlled trials. Nutrients 9:155. doi: 10.3390/nu9020155
Meguid, N. A., Dardir, A. A., Abdel-Raouf, E. R., and Hashish, A. (2011). Evaluation of oxidative stress in autism: defective antioxidant enzymes and increased lipid peroxidation. Biol. Trace Elem. Res. 143, 58–65. doi: 10.1007/s12011-010-8840-9
Meng, C., Helm, D., Frejno, M., and Kuster, B. (2016). moCluster: identifying joint patterns across multiple omics data sets. J. Proteome Res. 15, 755–765. doi: 10.1021/acs.jproteome.5b00824
Meng, C., Kuster, B., Culhane, A. C., and Gholami, A. M. (2014). A multivariate approach to the integration of multi-omics datasets. BMC Bioinform. 15:162. doi: 10.1186/1471-2105-15-162
Mertz, W. (1998). Review of the scientific basis for establishing the essentiality of trace elements. Biol. Trace Elem. Res. 66, 185–191. doi: 10.1007/BF02783137
Min, S., Lee, B., and Yoon, S. (2017). Deep learning in bioinformatics. Brief. Bioinform. 18, 851–869. doi: 10.1093/bib/bbw068
Mor, M., Nardone, S., Sams, D. S., and Elliott, E. (2015). Hypomethylation of miR-142 promoter and upregulation of microRNAs that target the oxytocin receptor gene in the autism prefrontal cortex. Mol. Autism 6:46. doi: 10.1186/s13229-015-0040-1
Mordaunt, C. E., Kieffer, D. A., Shibata, N. M., Członkowska, A., Litwin, T., Weiss, K.-H., et al. (2019). Epigenomic signatures in liver and blood of Wilson disease patients include hypermethylation of liver-specific enhancers. Epigenetics Chromatin 12:10. doi: 10.1186/s13072-019-0255-z
Mostafa, G. A., Bjørklund, G., Urbina, M. A., and Al-Ayadhi, L. Y. (2016). The levels of blood mercury and inflammatory-related neuropeptides in the serum are correlated in children with autism spectrum disorder. Metab. Brain Dis. 31, 593–599. doi: 10.1007/s11011-015-9784-8
Mounicou, S., Szpunar, J., and Lobinski, R. (2009). Metallomics: the concept and methodology. Chem. Soc. Rev. 38, 1119–1138. doi: 10.1039/B713633C
Muqaku, B., Eisinger, M., Meier, S. M., Tahir, A., Pukrop, T., Haferkamp, S., et al. (2017). Multi-omics analysis of serum samples demonstrates reprogramming of organ functions via systemic calcium mobilization and platelet activation in metastatic melanoma. Mol. Cell. Proteomics 16, 86–99. doi: 10.1074/mcp.M116.063313
Nakagawa, Y., and Yamada, S. (2021). A novel hypothesis on metal dyshomeostasis and mitochondrial dysfunction in amyotrophic lateral sclerosis: Potential pathogenetic mechanism and therapeutic implications. Eur. J. Pharmacol. 892:173737. doi: 10.1016/j.ejphar.2020.173737
Nataf, R., Skorupka, C., Amet, L., Lam, A., Springbett, A., and Lathe, R. (2006). Porphyrinuria in childhood autistic disorder: Implications for environmental toxicity. Toxicol. Appl. Pharmacol. 214, 99–108. doi: 10.1016/j.taap.2006.04.008
Needham, B. D., Adame, M. D., Serena, G., Rose, D. R., Preston, G. M., Conrad, M. C., et al. (2021). Plasma and fecal metabolite profiles in autism spectrum disorder. Biol. Psychiatry 89, 451–462. doi: 10.1016/j.biopsych.2020.09.025
Pacheco, N. L., Heaven, M. R., Holt, L. M., Crossman, D. K., Boggio, K. J., Shaffer, S. A., et al. (2017). RNA sequencing and proteomics approaches reveal novel deficits in the cortex of Mecp2-deficient mice, a model for Rett syndrome. Mol. Autism 8:56. doi: 10.1186/s13229-017-0174-4
Palermo, G., Piraino, P., and Zucht, H.-D. (2009). Performance of PLS regression coefficients in selecting variables for each response of a multivariate PLS for omics-type data. Adv. Appl. Bioinforma. Chem. 2, 57–70. doi: 10.2147/aabc.s3619
Pangrazzi, L., Balasco, L., and Bozzi, Y. (2020). Oxidative stress and immune system dysfunction in autism spectrum disorders. Int. J. Mol. Sci. 21:3293. doi: 10.3390/ijms21093293
Park, D. I. (2020). Genomics, transcriptomics, proteomics and big data analysis in the discovery of new diagnostic markers and targets for therapy development. Prog. Mol. Biol. Transl. Sci. 173, 61–90. doi: 10.1016/bs.pmbts.2020.04.017
Parkhomenko, E., Tritchler, D., and Beyene, J. (2009). Sparse canonical correlation analysis with application to genomic data integration. Stat. Appl. Genet. Mol. Biol. 8:Article1. doi: 10.2202/1544-6115.1406
Patel, S., Masi, A., Dale, R. C., Whitehouse, A. J. O., Pokorski, I., Alvares, G. A., et al. (2018). Social impairments in autism spectrum disorder are related to maternal immune history profile. Mol. Psychiatry 23, 1794–1797. doi: 10.1038/mp.2017.201
Pellegrini, C., Antonioli, L., Calderone, V., Colucci, R., Fornai, M., and Blandizzi, C. (2020). Microbiota-gut-brain axis in health and disease: Is NLRP3 inflammasome at the crossroads of microbiota-gut-brain communications? Prog. Neurobiol. 191:101806. doi: 10.1016/j.pneurobio.2020.101806
Pfaender, S., Föhr, K., Lutz, A.-K., Putz, S., Achberger, K., Linta, L., et al. (2016). Cellular zinc homeostasis contributes to neuronal differentiation in human induced pluripotent stem cells. Neural Plast. 2016:3760702. doi: 10.1155/2016/3760702
Raison, C. L., Borisov, A. S., Woolwine, B. J., Massung, B., Vogt, G., and Miller, A. H. (2010). Interferon-α effects on diurnal hypothalamic–pituitary–adrenal axis activity: relationship with proinflammatory cytokines and behavior. Mol. Psychiatry 15, 535–547. doi: 10.1038/mp.2008.58
Ramaswami, G., Won, H., Gandal, M. J., Haney, J., Wang, J. C., Wong, C. C. Y., et al. (2020). Integrative genomics identifies a convergent molecular subtype that links epigenomic with transcriptomic differences in autism. Nat. Commun. 11:4873. doi: 10.1038/s41467-020-18526-1
Reed, S., Neuman, H., Moscovich, S., Glahn, R. P., Koren, O., and Tako, E. (2015). Chronic zinc deficiency alters chick gut microbiota composition and function. Nutrients 7, 9768–9784. doi: 10.3390/nu7125497
Rivers-Auty, J., Tapia, V. S., White, C. S., Daniels, M. J., Drinkall, S., Kennedy, P. T., et al. (2021). Zinc status alters Alzheimer’s disease progression through NLRP3-dependent inflammation. J. Neurosci. Off. J. Soc. Neurosci. 41, 3025–3038. doi: 10.1523/JNEUROSCI.1980-20.2020
Rohart, F., Eslami, A., Matigian, N., Bougeard, S., and Lê Cao, K.-A. (2017). MINT: a multivariate integrative method to identify reproducible molecular signatures across independent experiments and platforms. BMC Bioinformatics 18:128. doi: 10.1186/s12859-017-1553-8
Ruoppolo, M., Scolamiero, E., Caterino, M., Mirisola, V., Franconi, F., and Campesi, I. (2015). Female and male human babies have distinct blood metabolomic patterns. Mol. Biosyst. 11, 2483–2492. doi: 10.1039/c5mb00297d
Russo, A. J., and deVito, R. (2011). Analysis of copper and zinc plasma concentration and the efficacy of zinc therapy in individuals with Asperger’s syndrome, pervasive developmental disorder not otherwise specified (PDD-NOS) and Autism. Biomark. Insights 6:S7286. doi: 10.4137/BMI.S7286
Saghazadeh, A., Ahangari, N., Hendi, K., Saleh, F., and Rezaei, N. (2017). Status of essential elements in autism spectrum disorder: systematic review and meta-analysis. Rev. Neurosci. 28, 783–809. doi: 10.1515/revneuro-2017-0015
Saghazadeh, A., Ataeinia, B., Keynejad, K., Abdolalizadeh, A., Hirbod-Mobarakeh, A., and Rezaei, N. (2019). Anti-inflammatory cytokines in autism spectrum disorders: A systematic review and meta-analysis. Cytokine 123:154740. doi: 10.1016/j.cyto.2019.154740
Saghazadeh, A., and Rezaei, N. (2017). Systematic review and meta-analysis links autism and toxic metals and highlights the impact of country development status: Higher blood and erythrocyte levels for mercury and lead, and higher hair antimony, cadmium, lead, and mercury. Prog. Neuropsychopharmacol. Biol. Psychiatry 79, 340–368. doi: 10.1016/j.pnpbp.2017.07.011
Santoru, M. L., Piras, C., Murgia, A., Palmas, V., Camboni, T., Liggi, S., et al. (2017). Cross sectional evaluation of the gut-microbiome metabolome axis in an Italian cohort of IBD patients. Sci. Rep. 7:9523. doi: 10.1038/s41598-017-10034-5
Sanusi, K. O., Ibrahim, K. G., Abubakar, B., Malami, I., Bello, M. B., Imam, M. U., et al. (2021). Effect of maternal zinc deficiency on offspring health: The epigenetic impact. J. Trace Elem. Med. Biol. 65:126731. doi: 10.1016/j.jtemb.2021.126731
Saresella, M., Piancone, F., Marventano, I., Zoppis, M., Hernis, A., Zanette, M., et al. (2016). Multiple inflammasome complexes are activated in autistic spectrum disorders. Brain. Behav. Immun. 57, 125–133. doi: 10.1016/j.bbi.2016.03.009
Sarmiento-Ortega, V. E., Treviño, S., Flores-Hernández, J. Á, Aguilar-Alonso, P., Moroni-González, D., Aburto-Luna, V., et al. (2017). Changes on serum and hepatic lipidome after a chronic cadmium exposure in Wistar rats. Arch. Biochem. Biophys. 635, 52–59. doi: 10.1016/j.abb.2017.10.003
Sauer, A. K., Bockmann, J., Steinestel, K., Boeckers, T. M., and Grabrucker, A. M. (2019). Altered intestinal morphology and microbiota composition in the autism spectrum disorders associated SHANK3 mouse model. Int. J. Mol. Sci. 20:2134. doi: 10.3390/ijms20092134
Sauer, A. K., and Grabrucker, A. M. (2019). Zinc deficiency during pregnancy leads to altered microbiome and elevated inflammatory markers in mice. Front. Neurosci. 13:1295. doi: 10.3389/fnins.2019.01295
Sayehmiri, F., Babaknejad, N., Bahrami, S., Sayehmiri, K., Darabi, M., and Rezaei-Tavirani, M. (2015). Zn/Cu levels in the field of autism disorders: a systematic review and meta-analysis. Iran. J. Child Neurol. 9, 1–9.
Schneider, T., Caviezel, D., Ayata, C. K., Kiss, C., Niess, J. H., and Hruz, P. (2020). The Copper/Zinc ratio correlates with markers of disease activity in patients with inflammatory bowel disease. Crohns Colitis 360 2:otaa001. doi: 10.1093/crocol/otaa001
Scholefield, M., Unwin, R. D., and Cooper, G. J. S. (2020). Shared perturbations in the metallome and metabolome of Alzheimer’s, Parkinson’s, Huntington’s, and dementia with Lewy bodies: A systematic review. Ageing Res. Rev. 63:101152. doi: 10.1016/j.arr.2020.101152
Schwede, M., Nagpal, S., Gandal, M. J., Parikshak, N. N., Mirnics, K., Geschwind, D. H., et al. (2018). Strong correlation of downregulated genes related to synaptic transmission and mitochondria in post-mortem autism cerebral cortex. J. Neurodev. Disord. 10:18. doi: 10.1186/s11689-018-9237-x
Sgritta, M., Dooling, S. W., Buffington, S. A., Momin, E. N., Francis, M. B., Britton, R. A., et al. (2019). Mechanisms underlying microbial-mediated changes in social behavior in mouse models of autism spectrum disorder. Neuron 101, 246–259. doi: 10.1016/j.neuron.2018.11.018
Shpyleva, S., Ivanovsky, S., Conti, A., de Melnyk, S., Tryndyak, V., Beland, F. A., et al. (2014). Cerebellar oxidative DNA damage and altered DNA methylation in the BTBR T+tf/J mouse model of autism and similarities with human post mortem cerebellum. PLoS One 9:e113712. doi: 10.1371/journal.pone.0113712
Singh, A., Kukreti, R., Saso, L., and Kukreti, S. (2019a). Oxidative stress: A Key modulator in neurodegenerative diseases. Molecules 24:1583. doi: 10.3390/molecules24081583
Singh, A., Shannon, C. P., Gautier, B., Rohart, F., Vacher, M., Tebbutt, S. J., et al. (2019b). DIABLO: an integrative approach for identifying key molecular drivers from multi-omics assays. Bioinforma. Oxf. Engl. 35, 3055–3062. doi: 10.1093/bioinformatics/bty1054
Siniscalco, D., Schultz, S., Brigida, A. L., and Antonucci, N. (2018). Inflammation and neuro-immune dysregulations in autism spectrum disorders. Pharmaceuticals 11:56. doi: 10.3390/ph11020056
Sivamaruthi, B. S., Suganthy, N., Kesika, P., and Chaiyasut, C. (2020). The role of microbiome, dietary supplements, and probiotics in autism spectrum disorder. Int. J. Environ. Res. Public. Health 17:2647. doi: 10.3390/ijerph17082647
Skalny, A. V., Simashkova, N. V., Skalnaya, A. A., Klyushnik, T. P., Bjørklund, G., Skalnaya, M. G., et al. (2017). Assessment of gender and age effects on serum and hair trace element levels in children with autism spectrum disorder. Metab. Brain Dis. 32, 1675–1684. doi: 10.1007/s11011-017-0056-7
Stoll, L., Hall, J., Van Buren, N., Hall, A., Knight, L., Morgan, A., et al. (2007). Differential regulation of ionotropic glutamate receptors. Biophys. J. 92, 1343–1349. doi: 10.1529/biophysj.106.089896
Strati, F., Cavalieri, D., Albanese, D., De Felice, C., Donati, C., Hayek, J., et al. (2017). New evidences on the altered gut microbiota in autism spectrum disorders. Microbiome 5:24. doi: 10.1186/s40168-017-0242-1
Sun, W., Poschmann, J., Cruz-Herrera Del Rosario, R., Parikshak, N. N., Hajan, H. S., Kumar, V., et al. (2016). Histone acetylome-wide association study of autism spectrum disorder. Cell 167, 1385–1397. doi: 10.1016/j.cell.2016.10.031
Tabatadze, T., Zhorzholiani, L., Kherkheulidze, M., Kandelaki, E., and Ivanashvili, T. (2015). Hair heavy metal and essential trace element concentration in children with austism spectrum disorder. Georgian Med. News 248, 77–82.
Tamiji, J., and Crawford, D. A. (2010). The neurobiology of lipid metabolism in autism spectrum disorders. Neurosignals 18, 98–112. doi: 10.1159/000323189
Theoharides, T. C., Stewart, J. M., Panagiotidou, S., and Melamed, I. (2016). Mast cells, brain inflammation and autism. Eur. J. Pharmacol. 778, 96–102. doi: 10.1016/j.ejphar.2015.03.086
Tseng, P.-T., Cheng, Y.-S., Yen, C.-F., Chen, Y.-W., Stubbs, B., Whiteley, P., et al. (2018). Peripheral iron levels in children with attention-deficit hyperactivity disorder: a systematic review and meta-analysis. Sci. Rep. 8:788. doi: 10.1038/s41598-017-19096-x
Tsilioni, I., Taliou, A., Francis, K., and Theoharides, T. C. (2015). Children with autism spectrum disorders, who improved with a luteolin-containing dietary formulation, show reduced serum levels of TNF and IL-6. Transl. Psychiatry 5:e647. doi: 10.1038/tp.2015.142
Uriu-Adams, J. Y., and Keen, C. L. (2010). Zinc and reproduction: effects of zinc deficiency on prenatal and early postnatal development. Birth Defects Res. B. Dev. Reprod. Toxicol. 89, 313–325. doi: 10.1002/bdrb.20264
van Spronsen, M., and Hoogenraad, C. C. (2010). Synapse pathology in psychiatric and neurologic disease. Curr. Neurol. Neurosci. Rep. 10, 207–214. doi: 10.1007/s11910-010-0104-8
Vela, G., Stark, P., Socha, M., Sauer, A. K., Hagmeyer, S., and Grabrucker, A. M. (2015). Zinc in gut-brain interaction in autism and neurological disorders. Neural. Plast. 2015:e972791. doi: 10.1155/2015/972791
Vergani, L., Cristina, L., Paola, R., Luisa, A. M., Shyti, G., Edvige, V., et al. (2011). Metals, metallothioneins and oxidative stress in blood of autistic children. Res. Autism Spectr. Disord. 5, 286–293. doi: 10.1016/j.rasd.2010.04.010
Voineagu, I., Wang, X., Johnston, P., Lowe, J. K., Tian, Y., Horvath, S., et al. (2011). Transcriptomic analysis of autistic brain reveals convergent molecular pathology. Nature 474, 380–384. doi: 10.1038/nature10110
Vuong, H. E., and Hsiao, E. Y. (2017). Emerging roles for the gut microbiome in autism spectrum disorder. Biol. Psychiatry 81, 411–423. doi: 10.1016/j.biopsych.2016.08.024
Wang, B., Li, Y., Shao, C., Tan, Y., and Cai, L. (2012). Cadmium and its epigenetic effects. Curr. Med. Chem. 19, 2611–2620. doi: 10.2174/092986712800492913
Wang, H., Abel, G. M., Storm, D. R., and Xia, Z. (2019). Cadmium exposure impairs adult hippocampal neurogenesis. Toxicol. Sci. 171, 501–514. doi: 10.1093/toxsci/kfz152
Wang, Z., Koonen, D., Hofker, M., and Fu, J. (2016). Gut microbiome and lipid metabolism: from associations to mechanisms. Curr. Opin. Lipidol. 27, 216–224. doi: 10.1097/MOL.0000000000000308
Wecker, L., Miller, S. B., Cochran, S. R., Dugger, D. L., and Johnson, W. D. (1985). Trace element concentrations in hair from autistic children. J. Ment. Defic. Res. 29, 15–22. doi: 10.1111/j.1365-2788.1985.tb00303.x
Wei, H., Zou, H., Sheikh, A. M., Malik, M., Dobkin, C., Brown, W. T., et al. (2011). IL-6 is increased in the cerebellum of autistic brain and alters neural cell adhesion, migration and synaptic formation. J. Neuroinflam. 8:52. doi: 10.1186/1742-2094-8-52
Wetie, A. G. N., Wormwood, K. L., Charette, L., Ryan, J. P., Woods, A. G., and Darie, C. C. (2015a). Comparative two-dimensional polyacrylamide gel electrophoresis of the salivary proteome of children with autism spectrum disorder. J. Cell. Mol. Med. 19, 2664–2678. doi: 10.1111/jcmm.12658
Wetie, A. G. N., Wormwood, K. L., Russell, S., Ryan, J. P., Darie, C. C., and Woods, A. G. (2015b). A pilot proteomic analysis of salivary biomarkers in autism spectrum disorder. Autism Res. 8, 338–350. doi: 10.1002/aur.1450
Wu, L., Mao, S., Lin, X., Yang, R., and Zhu, Z. (2019). Evaluation of whole blood trace element levels in chinese children with autism spectrum disorder. Biol. Trace Elem. Res. 191, 269–275. doi: 10.1007/s12011-018-1615-4
Xia, J., Jin, C., Pan, Z., Sun, L., Fu, Z., and Jin, Y. (2018). Chronic exposure to low concentrations of lead induces metabolic disorder and dysbiosis of the gut microbiota in mice. Sci. Total Environ. 631-632, 439–448. doi: 10.1016/j.scitotenv.2018.03.053
Xing, X., Zhang, J., Wu, K., Cao, B., Li, X., Jiang, F., et al. (2019). Suppression of Akt-mTOR pathway rescued the social behavior in Cntnap2 -deficient mice. Sci. Rep. 9:3041. doi: 10.1038/s41598-019-39434-5
Yang, G.-J., Liu, H., Ma, D.-L., and Leung, C.-H. (2019a). Rebalancing metal dyshomeostasis for Alzheimer’s disease therapy. J. Biol. Inorg. Chem. 24, 1159–1170. doi: 10.1007/s00775-019-01712-y
Yang, R., Zhang, Y., Gao, W., Lin, N., Li, R., and Zhao, Z. (2019b). Blood levels of trace elements in children with attention-deficit hyperactivity disorder: results from a case-control study. Biol. Trace Elem. Res. 187, 376–382. doi: 10.1007/s12011-018-1408-9
Yang, Z., Yang, S., Qian, S. Y., Hong, J.-S., Kadiiska, M. B., Tennant, R. W., et al. (2007). Cadmium-induced toxicity in rat primary mid-brain neuroglia cultures: role of oxidative stress from microglia. Toxicol. Sci. 98, 488–494. doi: 10.1093/toxsci/kfm106
Yasuda, H., Tsutsui, T., and Suzuki, K. (2020). Metallomics analysis for assessment of toxic metal burdens in Infants/Children and their mothers: early assessment and intervention are essential. Biomolecules 11:6. doi: 10.3390/biom11010006
Yasuda, H., Yasuda, Y., and Tsutsui, T. (2013). Estimation of autistic children by metallomics analysis. Sci. Rep. 3:1199. doi: 10.1038/srep01199
Yasuda, H., Yoshida, K., Yasuda, Y., and Tsutsui, T. (2011). Infantile zinc deficiency: Association with autism spectrum disorders. Sci. Rep. 1:129. doi: 10.1038/srep00129
Yoo, J., Bakes, J., Bradley, C., Collingridge, G. L., and Kaang, B.-K. (2014). Shank mutant mice as an animal model of autism. Philos. Trans. R. Soc. B Biol. Sci. 369:20130143. doi: 10.1098/rstb.2013.0143
Yuksel, M. E., Yuceturk, B., Karatas, O. F., Ozen, M., and Dogangun, B. (2016). The altered promoter methylation of oxytocin receptor gene in autism. J. Neurogenet. 30, 280–284. doi: 10.1080/01677063.2016.1202951
Zackular, J. P., Moore, J. L., Jordan, A. T., Juttukonda, L. J., Noto, M. J., Nicholson, M. R., et al. (2016). Dietary zinc alters the microbiota and decreases resistance to Clostridium difficile infection. Nat. Med. 22, 1330–1334. doi: 10.1038/nm.4174
Zhang, Y., Ying, H., and Xu, Y. (2019). Comparative genomics and metagenomics of the metallomes. Metallomics 11, 1026–1043. doi: 10.1039/c9mt00023b
Zheng, D., Liwinski, T., and Elinav, E. (2020). Inflammasome activation and regulation: toward a better understanding of complex mechanisms. Cell Discov. 6, 1–22. doi: 10.1038/s41421-020-0167-x
Zhu, C.-B., Blakely, R. D., and Hewlett, W. A. (2006). The Proinflammatory Cytokines Interleukin-1beta and tumor necrosis factor-alpha activate serotonin transporters. Neuropsychopharmacology 31, 2121–2131. doi: 10.1038/sj.npp.1301029
Zhu, L., Wang, X., Li, X.-L., Towers, A., Cao, X., Wang, P., et al. (2014). Epigenetic dysregulation of SHANK3 in brain tissues from individuals with autism spectrum disorders. Hum. Mol. Genet. 23, 1563–1578. doi: 10.1093/hmg/ddt547
Zhubi, A., Cook, E. H., Guidotti, A., and Grayson, D. R. (2014). Epigenetic mechanisms in autism spectrum disorder. Int. Rev. Neurobiol. 115, 203–244. doi: 10.1016/B978-0-12-801311-3.00006-8
Zierer, J., Jackson, M. A., Kastenmüller, G., Mangino, M., Long, T., Telenti, A., et al. (2018). The fecal metabolome as a functional readout of the gut microbiome. Nat. Genet. 50, 790–795. doi: 10.1038/s41588-018-0135-7
Zolkipli-Cunningham, Z., Naviaux, J. C., Nakayama, T., Hirsch, C. M., Monk, J. M., Li, K., et al. (2021). Metabolic and behavioral features of acute hyperpurinergia and the maternal immune activation mouse model of autism spectrum disorder. PLoS One 16:e0248771. doi: 10.1371/journal.pone.0248771
Keywords: autism, metallome, zinc deficiency, inflammation, microbiome, proteome, lipidome, transcriptome
Citation: Stanton JE, Malijauskaite S, McGourty K and Grabrucker AM (2021) The Metallome as a Link Between the “Omes” in Autism Spectrum Disorders. Front. Mol. Neurosci. 14:695873. doi: 10.3389/fnmol.2021.695873
Received: 22 April 2021; Accepted: 14 June 2021;
Published: 05 July 2021.
Edited by:
David Blum, INSERM U1172 Centre de Recherche Jean Pierre Aubert, FranceReviewed by:
Arnaud B. Nicot, INSERM U1064 Centre de Recherche en Transplantation et Immunologie, FranceCopyright © 2021 Stanton, Malijauskaite, McGourty and Grabrucker. This is an open-access article distributed under the terms of the Creative Commons Attribution License (CC BY). The use, distribution or reproduction in other forums is permitted, provided the original author(s) and the copyright owner(s) are credited and that the original publication in this journal is cited, in accordance with accepted academic practice. No use, distribution or reproduction is permitted which does not comply with these terms.
*Correspondence: Andreas M. Grabrucker, YW5kcmVhcy5ncmFicnVja2VyQHVsLmll
Disclaimer: All claims expressed in this article are solely those of the authors and do not necessarily represent those of their affiliated organizations, or those of the publisher, the editors and the reviewers. Any product that may be evaluated in this article or claim that may be made by its manufacturer is not guaranteed or endorsed by the publisher.
Research integrity at Frontiers
Learn more about the work of our research integrity team to safeguard the quality of each article we publish.