- 1Institute of Clinical Pharmacology, Goethe-University, Frankfurt am Main, Germany
- 2Fraunhofer Institute of Molecular Biology and Applied Ecology-Project Group, Translational Medicine and Pharmacology (IME-TMP), Frankfurt am Main, Germany
- 3DataBionics Research Group, University of Marburg, Marburg, Germany
Genes causally involved in human insensitivity to pain provide a unique molecular source of studying the pathophysiology of pain and the development of novel analgesic drugs. The increasing availability of “big data” enables novel research approaches to chronic pain while also requiring novel techniques for data mining and knowledge discovery. We used machine learning to combine the knowledge about n = 20 genes causally involved in human hereditary insensitivity to pain with the knowledge about the functions of thousands of genes. An integrated computational analysis proposed that among the functions of this set of genes, the processes related to nervous system development and to ceramide and sphingosine signaling pathways are particularly important. This is in line with earlier suggestions to use these pathways as therapeutic target in pain. Following identification of the biological processes characterizing hereditary insensitivity to pain, the biological processes were used for a similarity analysis with the functions of n = 4,834 database-queried drugs. Using emergent self-organizing maps, a cluster of n = 22 drugs was identified sharing important functional features with hereditary insensitivity to pain. Several members of this cluster had been implicated in pain in preclinical experiments. Thus, the present concept of machine-learned knowledge discovery for pain research provides biologically plausible results and seems to be suitable for drug discovery by identifying a narrow choice of repurposing candidates, demonstrating that contemporary machine-learned methods offer innovative approaches to knowledge discovery from available evidence.
Introduction
Persistent pain is a major healthcare issue, as defined by WHO, affecting about a fifth of the European population increasing to a third in the over 70-year old (Elliott et al., 1999; Breivik et al., 2006). It has a highly complex pathophysiology (Julius and Basbaum, 2001; Schaible, 2007) and it is triggered by several different causes, such as cancer (Portenoy, 1992) and surgery (Kehlet et al., 2006). Therefore, the search for novel analgesic strategies is an active research topic receiving public funding (Kringel and Lötsch, 2015). In addition to the identification of novel drug targets from molecular research as the main line of research, scanning the existing pharmacopeia for repurposing candidates becomes increasingly successful (Ashburn and Thor, 2004). This is facilitated by developments in computational data science (President's Information Technology Advisory Committee, 2005).
An accepted source of novel options for the pharmacological treatment of (persistent) pain is the study of genes causally involved in hereditary syndromes with insensitivity to pain (Goldberg et al., 2012; Table 1). This set of genes has provided the targets of novel analgesics (Table 2). However, this unique set of genotypes also enables the study of key biological processes of pain from the perspective of the functions of the involved genes. As shown recently, similarities between the profiles of biological processes in which the genes coding for the targets of available drugs with the profile of the biological processes in which a given set of genes is involved can be employed for drug classification or repurposing (Lötsch and Ultsch, 2016a,b). Hence, picturing the genetic background of human insensitivity to pain could be explored for drug repurposing based on functional similarity in addition to the development of novel substances targeting some of the respective gene products (Table 2). The present analysis made extensive use of computational biology, knowledge discovery methods, publicly available databases and data mining tools (Table 3) to merge results from pain, genetics and pharmacological research.
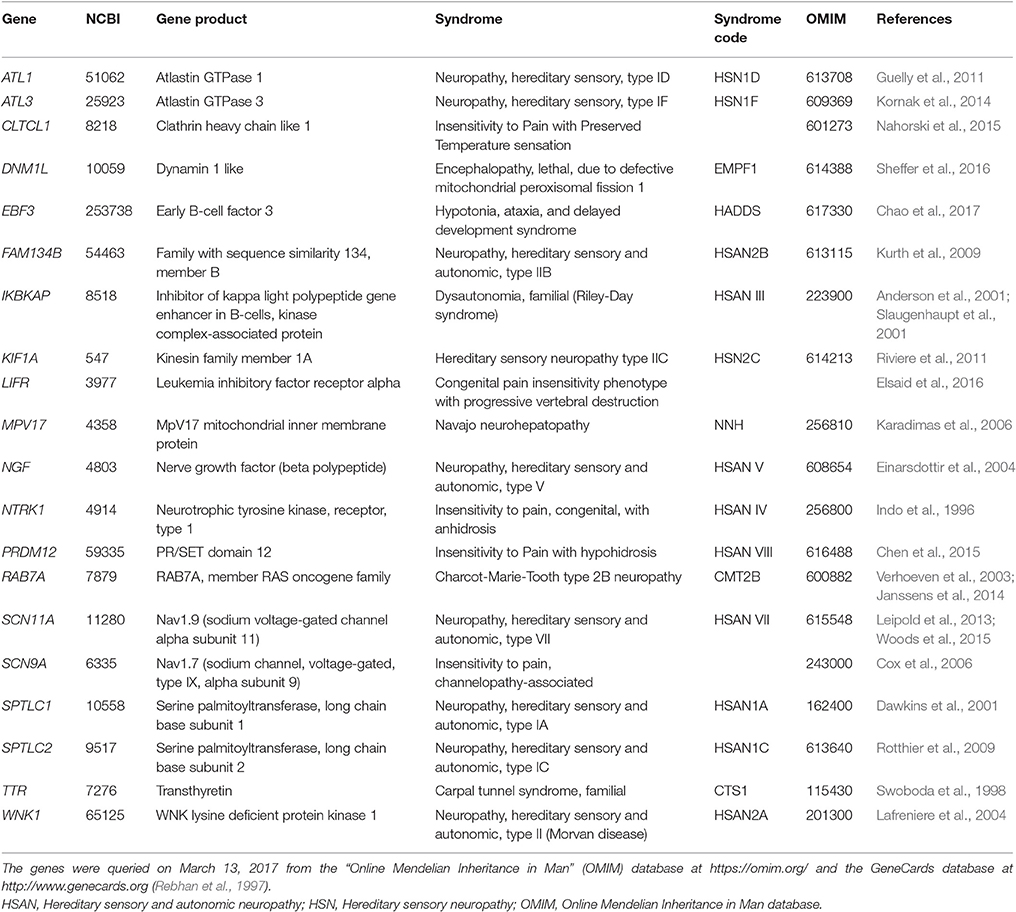
Table 1. A set of n = 20 genes, in alphabetical order, that were reported to be causally associated with the hereditary human phenotype of insensitivity to pain.
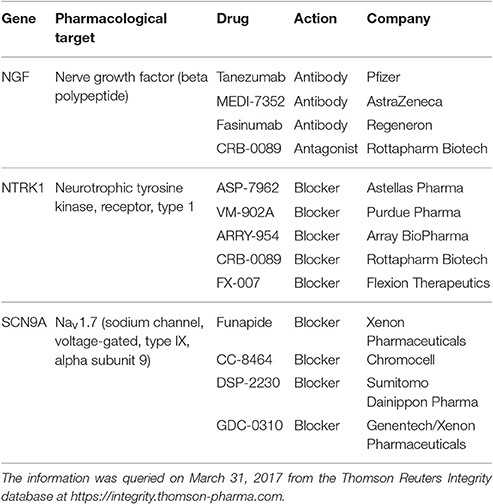
Table 2. Novel analgesic drugs developed with the purpose to antagonistically target genes associated with human hereditary insensitivity to pain, i.e., to mimic the pain-insensitivity phenotype observed in carriers of loss-of-function mutations in these genes, being currently in a clinical phase of development according to publicly available sources of information (Table 3).
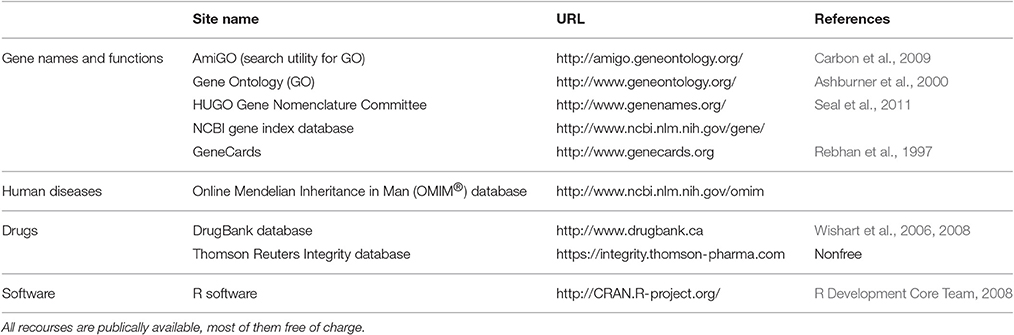
Table 3. Overview on data sources and computational tools used for the present data science approach to drug repurposing from knowledge about the functions of genes related to insensitivity to pain in humans.
Methods
Data were analyzed using the R software package (version 3.3.3 for Linux; http://CRAN.R-project.org/; R Development Core Team, 2008). Following querying the relevant sets of genes by mining publicly available databases, the analyses aimed at (i) identifying the systems biology of hereditary insensitivity to pain as conferred by the functions of the causally involved genes and (ii) to explore whether this knowledge can be employed for drug repurposing approaches aimed at identifying potential candidates for the treatment of pain. The analytical steps are summarized in Figure 1 and described in full detail in the following paragraphs.
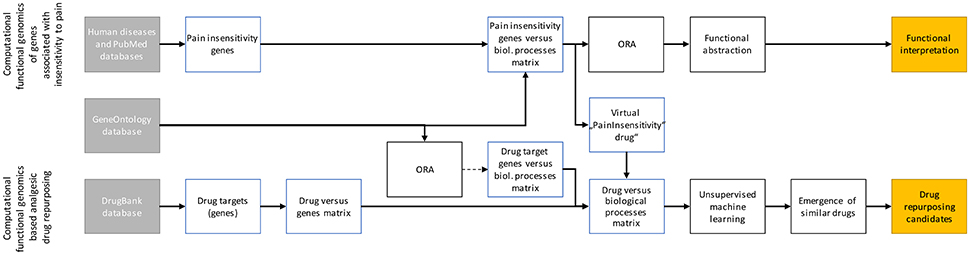
Figure 1. Scheme of the data analysis workflow. The analyses had two major aims, i.e., (i) assessing the biological functions of genes reportedly associated with insensitivity to pain (upper line) and (ii) using the biological processes in which these genes are involved to find repurposing candidates among DrugBank listed drugs (lower line). To this end, genes were identified in databases and their biological functions were associated based on the annotations in the Gene Ontology database; for the drug target coding genes (>4,000) with a filter for too many irrelevant terms implemented as an overrepresentation analysis. Such filter was not necessary for the only 20 genes associated with insensitivity to pain. However, for the latter, overrepresentation analysis (ORA) followed by functional abstraction was performed to obtain a comprehensible set of >10 biological functions which summarize the biological roles of these genes in an interpretable manner. The information obtained in the ORA of the 20 pain insensitivity genes was used to generate a virtual “pain drug” that was introduced into the “drug vs. biological processes” matrix of all drugs. Subsequently, unsupervised machine learning was used to find data structures among all drugs. Those drugs that, in the high dimensional vector space of associations with GO terms (biological processes), laid near the virtual “PainInsensitivity drug” were the repurposing candidates.
Data Mining
Genes involved in several different syndromes sharing the common phenotype of insensitivity to pain were queried on March 27, 2017 from the “Online Mendelian Inheritance in Man” (OMIM) database at (Online Mendelian Inheritance in Man, OMIM®, McKusick-Nathans Institute of Genetic Medicine, Johns Hopkins University, Baltimore, MD, USA) at https://omim.org/ and the GeneCards database at http://www.genecards.org (Rebhan et al., 1997) for “insensitivity to pain.” In addition, the medical literature was searched in the PubMed database at https://www.ncbi.nlm.nih.gov/pubmed. This provided a set of n = 20 genes (Table 1) that included genes causing human hereditary sensory and autonomic neuropathies (HSAN) and further genes for which published evidence is available for a causal implication with the common phenotype of insensitivity to pain.
To assess whether the known functions of the genes associated with human hereditary insensitivity to pain could be used for drug repurposing, genes coding for known molecular targets of known drugs were taken from the DrugBank database (version 5.0; http://www.drugbank.ca; Wishart et al., 2006, 2008). Specifically, a query of the DrugBank database on March 13, 2017, identified 4,834 drug entries, including 4,630 FDA-approved small molecule drugs, interacting with 2,215 unique targets. This provided the 4,834 × 2,215 “drug vs. gene” matrix.
Assessment of the Functions of Genes Associated with Insensitivity to Pain
The biological functions in which the products of genes associated with insensitivity to pain are involved were queried from the Gene Ontology knowledge base (GO; http://www.geneontology.org/; Ashburner et al., 2000). In this database, knowledge about the functions of genes is stored using a controlled and clearly defined vocabulary of GO terms (Camon et al., 2003) allocated to each gene (Camon et al., 2004). The GO database is searchable for three major categories, consisting of biological process, cellular component and molecular function. As the best representation of processes affected in hereditary insensitivity to pain as a potential source of therapeutic approaches, the GO category biological process was selected. According to the GO database, this category contains one or more ordered collections of molecular functions involving chemical or physical transformations such as cell growth and maintenance or signal transduction (Ashburner et al., 2000).
To capture biological processes that are particularly relevant to the present gene set, while eliminating data noise from common processes, the set of pain insensitivity genes was submitted to over-representation analysis (ORA) (Backes et al., 2007). This compared the occurrence (as defined by its annotation term) of the particular set of genes covered by a GO term with the number of genes expected to be defined by this term. The significance of the association of a GO term with the expected list of genes was determined by means of a Fisher's exact test (Fisher, 1922). The ORA attributed p-values to the resulting GO terms. A p-value threshold, tp, of 0.05 was applied and corrected for multiple testing using the false discovery rate (Benjamini and Hochberg, 1995).
The result was a representation of the complete knowledge about the biological roles of genes associated with insensitivity to pain in the form of a directed acyclic graph (DAG; Thulasiraman and Swamy, 1992). This depicted the biological processes as a higher level of organization of genes and signaling pathways described in a polyhierarchical structure where the processes are connected to each other by “is-a,” “part-of,” and “regulates” relationships (Ashburner et al., 2000). This result was used as the basis for a description of the functions of genes associated with insensitivity to pain. However, the complete DAG usually contained 136 GO terms that eluded intuitive interpretation (Miller, 1956). Therefore, the obtained results were transformed into a more intelligible form using the method of “functional abstraction” (Ultsch and Lötsch, 2014). This aims to reduce the numbers of GO terms using a heuristic search algorithm that identifies so-called “functional areas” (Ultsch and Lötsch, 2014), which are GO terms that qualify by their informational importance as headlines representing specific aspects (taxonomies) of the complete DAG with maximal coverage, precision, informational value and conciseness (Ultsch and Lötsch, 2014).
Assessment of Drug Repurposing Based on Computational Analysis
Following analysis of the biological processes in which the genes associated with human hereditary insensitivity to pain, the possibility was explored whether the discovered knowledge could be employed for a drug repurposing approach that uses functional similarity between drugs and key functions of a trait-relevant gene set (Lötsch and Ultsch, 2016a,b). This was based on (i) the biological processes identified as characterizing the genes associated with insensitivity to pain obtained in above analysis, and required in addition (ii) associating drugs, via the genes coding for their targets, with biological processes followed by (iii) the identification of those drugs that are associated with similar biological processes that characterize the genes associated with insensitivity to pain phenotypes. These analytical steps will be described in as follows.
As described previously (Lötsch and Ultsch, 2016a,b), the association of drugs with biological processes was obtained by combining drugs which are associated with molecular targets available from the DrugBank database with genes associated with biological processes as queried from the Gene Ontology database (Table 3). Specifically, a set of n = 2,215 genes queried as drug targets from the DrugBank database was submitted to overrepresentation analysis to identify relevant biological processes, expressed as GO terms, which can be addressed by the available drugs. ORA, performed as described above, however, with the parameters tp = 1·10−15 and α correction according to Bonferroni (1936), provided 830 GO terms that can be considered as specifically describing the biological processes in which 2,215 targets of the drugs known to the DrugBank database are involved. This resulted in a 2,215 × 830 “gene vs. biological process” matrix. Here, in contrast to its use for functional interpretation of a gene set applied onto the pain insensitivity genes as described above, the ORA served merely as a filter for relevant GO terms, which was set at a conservative p-value threshold to eliminate too many generic terms from the matrix; as a functional interpretation of the biological roles of all drug targets was not intended, functional abstraction was not applied. From the matrix product of the “drug vs. genes” matrix and the “genes vs. processes” matrix, a 4,834 × 830 “drug vs. biological process” matrix was provided.
Subsequently, a virtual drug “PainInsensitivity” was added to the “drug vs. biological process” matrix. This “drug” carried a single vector composed of the numbers that indicate how often particular biological processes have been associated with a member of the n = 20 sized gene set causally involved in hereditary insensitivity to pain. Using the biological processes that were addressed by both, DrugBank queried drugs and the “PainInsensitivity” drug, a 4,512 × 38 sized “drug vs. biological process” matrix was obtained. Within this matrix, the Euclidian distances of each of the 4,511 DrugBank annotated drugs to the “PainInsensitivity” drug were calculated.
To eliminate drugs dissimilar to the “PainInsensitivity” drug, the reciprocals of the Euclidian distances were submitted to calculated ABC analysis (Ultsch and Lötsch, 2015). This is a categorization technique for the selection of a most important subset among a larger set of items. It divides a set of positive data into three disjoint subsets “A,” “B,” and “C.” Subset “A” comprises the profitable values, i.e., “the important few” (Pareto, 1909; Juran, 1975) and is found using the x-value where the slope of an ABC curve (Gastwirth and Glauberman, 1976), i.e., a plot of cumulative distribution of items sorted in decreasing order of magnitude, takes a value of 1. These calculations were done using our R package “ABCanalysis” (http://CRAN.R-project.org/web/packages/ABCanalysis/index.html; Ultsch and Lötsch, 2015). To exclude drugs at large distances from the “PainInsensitivity” drug, ABC analysis was performed in a nested manner, i.e., ABC set “A” of a first analysis was re-submitted to a second ABC analysis. This provided a 414 × 38 sized “drug vs. biological process” matrix.
In this 414 × 38 sized “drug vs. biological process” matrix, data structures were analyzed to identify a cluster of DrugBank annotated compounds located in the vicinity to the “PainInsensitivity” drug. This was obtained using unsupervised machine learning (Murphy, 2012). Specifically, each drug is represented as a vector in a d = 38-dimensional feature space of positive associations with biological processes. A projection and visualization method was used that projects the d-dimensional feature space onto a two-dimensional plane and depicts the structures of the feature space in form of a landscape. A self-organizing artificial neuronal network of Kohonen (1982) type emergent SOM, (ESOM) (Ultsch and Sieman, 1990; Lötsch and Ultsch, 2014) was used. The neural network consisted of a two-dimensional toroid grid (Ultsch, 2003) of 50 × 80 neurons (n = 4,000 units). Each neuron holds, in addition to the input vector from the high-dimensional space of processes associated to each drug, a further vector carrying “weights,” which were initially randomly drawn from the range of the data variables and subsequently adapted to the data during the learning phase that used 50 epochs.
The trained emergent self-organizing map (ESOM) represents the drugs on a two-dimensional toroid map as the localizations of the “best matching units” (BMU). On top of this grid the distance structures in the high-dimensional feature space of biological processes is depicted in form of a so-called U-Matrix (Ultsch and Sieman, 1990; Lötsch and Ultsch, 2014). The machine learning was performed using the R library “UMatrix” (M. Thrun et al., Marburg, Germany, publicly available at http://www.uni-marburg.de/fb12/datenbionik/softwareweb/packages/ABCanalysis/index.html. Only the cluster was further regarded that included the “PainInsensitivity” drug. For the DrugBank annotated members of this cluster, evidence was queried from the literature supporting, or discouraging, a possible involvement in pain.
Results
Computational Analysis of the Genes Involved in Hereditary Insensitivity to Pain
To identify the systems biology of hereditary insensitivity to pain, a set of n = 20 unique genes (Table 1) for which published evidence supported an association with the human phenotype of inherited insensitivity to pain was queried form publicly available databases (Table 3). The biological functions associated with the expression of these genes and their respective products were queried from the Gene Ontology knowledge base (GO; http://www.geneontology.org/; Ashburner et al., 2000). Over-representation analysis (ORA) against all human genes (n = 18,750 in the used GO version) and using an FDR corrected p-value threshold of tp = 0.05 resulted in 136 significant GO terms (Supplementary Figure 1).
Subsequent functional abstraction, which is a method developed to reduce a large set of GO terms to a comprehensible small subset of “headline terms” or “functional areas” that represent specific aspects (taxonomies) of the complete polyhierarchy with maximal coverage, precision, informational value and conciseness (Ultsch and Lötsch, 2014), identified 8 GO terms qualifying as headlines to summarize the biological functions that are particularly important addressed by the 20 genes associated with insensitivity to pain, among all human genes (Table 4). A GO annotation with pain was found in two particular taxonomies of the polyhierarchy, i.e., under the headline “multicellular organismal response to stress” (GO:0033555) that exclusively included response to pain (GO:0048265) and its descendants, and again under the headline “Neurological system process” (GO:0050877), which at the chosen p-value threshold ended downstream with “sensory perception of pain” (GO:0019233) and “neuronal action potential” (GO:0019228).
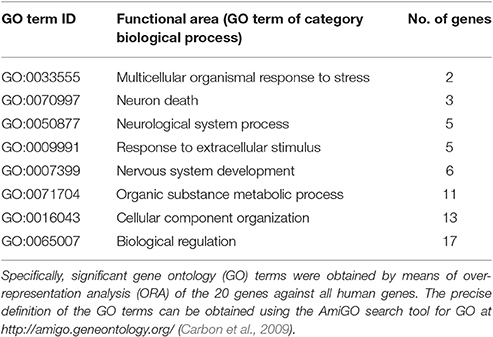
Table 4. Functional areas representing the genetic background of hereditary insensitivity to pain presented in a polyhierarchy of GO terms with a maximum of certainty, information value, coverage and conciseness (Ultsch and Lötsch, 2014).
A second larger group of biological processes typically annotated with the genes associated with insensitivity to pain comprised developmental and structural aspects of the nervous system, i.e., “nervous system development” (GO:0007399) and “neuron death” (GO:0070997) including “negative regulation of apoptotic process” (GO:0043524) as a downstream terminal of the taxonomy, and also processes of “cellular component organization” (GO:0016043). A third larger group of biological processes typically annotated with the genes associated with insensitivity to pain comprised metabolic processes (“organic substance metabolic process,” GO:0071704), which included biosynthetic processes related to sphingolipids (“sphingosine biosynthetic process,” GO:0046512) and ceramides (“ceramide biosynthetic process,” GO:0046513), “nerve growth factor processing” (GO:0032455), “positive regulation of lipophagy” (GO:1904504), and “positive regulation of histone H3-K9 dimethylation” (GO:1900111). The remaining processes could only be summarized in large sets of more heterogeneous biological functions such as “biological regulation.”
Candidate Drugs for Repurposing
To explore whether the results of the computational analysis of genes associated with human insensitivity to pain could be employed for drug repurposing, a virtual drug “PainInsensitivity” was created that carried a single vector composed of the numbers of how often particular biological processes have been associated with a member of the n = 20 genes causally involved in hereditary insensitivity to pain. Only the n = 38 biological processes were included that were also associated with any drug queried from the DrugBank database. Similarly, vectors coding for the associations with biological processes were assigned to each drug. The data space was reduced by eliminating drugs at large Euclidian distances from the “PainInsensitivity” drug, using nested ABC analysis.
Following projection of the resulting 414 × 38 sized “drug vs. biological process” data space onto a toroid grid of 50 × 80 neurons and training of a self-organizing map, a U-matrix visualization was displayed on top of this SOM (Figure 2). Large U-heights in this visualization indicated a large gap in the data space whereas low U-heights indicated that the points are close to each other in the data space. The distance-based data structure indicated a cluster that comprised the virtual drug “PainInsensitivity” (red dot in Figure 2) together with further n = 22 DrugBank listed substances (Table 5). These substances can be considered as repurposing candidates for pain, based on their vicinity (yellow dots in Figure 2) in the high-dimensional data space to the biological processes associated with the genes causally involved in insensitivity to pain as represented on the SOM by the “PainInsensitivity” drug.
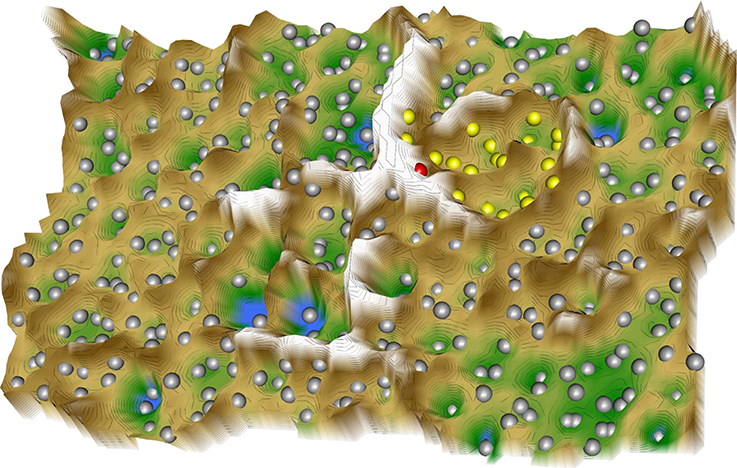
Figure 2. 3D-view of the U-matrix visualization of distance based structures of the 414 × 38 sized “drug vs. biological process” matrix, comprising the 413 drugs annotated with one or more of the n = 38 biological processes assigned to both, the set of 20 genes causally implicated in insensitivity to pain and the drug targets queried form the DrugBank database, which following ABC analysis based item selection were found in at closer Euclidian distances form the virtual “PainInsensitivity” drug (red dot) that carried all of the n = 38 processes. The U-matrix has been obtained using a projection of the data points onto a toroid grid of 4,000 neurons where opposite edges are connected. The U-Matrix was colored as a geographical map with brown (up to snow-covered) heights and green valleys with blue lakes. Valleys indicate clusters and watersheds indicate borderlines between different clusters. The dots indicate the so-called “best matching units” (BMUs) of the self-organizing map (SOM), which are those neurons whose weight vector is most similar to the input. A single neuron can be the BMU for more than one data point; hence, the number of BMUs may not be equal to the number of drugs. In the vicinity of the red dot, i.e., the virtual “PainInsensitivity” drug, a mount ridge surrounded valley was observed that represented a cluster of drugs (yellow dots) most similar to the virtual “PainInsensitivity” drug. However, the latter was located eccentrically in the cluster indicating that the similarity to any of the DrugBank queried repurposing candidates for pain therapy is incomplete. The other drugs (gray dots) lay outside the cluster of the “PainInsensitivity” drug and could therefore be rejected as repurposing candidates based on the present approach to search for drugs that with respect to the addressed biological processes are most similar to the pattern of biological processes in which the genes associated with insensitivity to pain are involved. The figure has been created using the R software package (version 3.3.3 for Linux; http://CRAN.R-project.org/; R Development Core Team, 2008) using our R library “Umatrix” (M. Thrun, F. Lerch, Marburg, Germany, http://www.uni-marburg.de/fb12/datenbionik/software; file http://www.uni-marburg.de/fb12/datenbionik/umatrix.tar.gz).
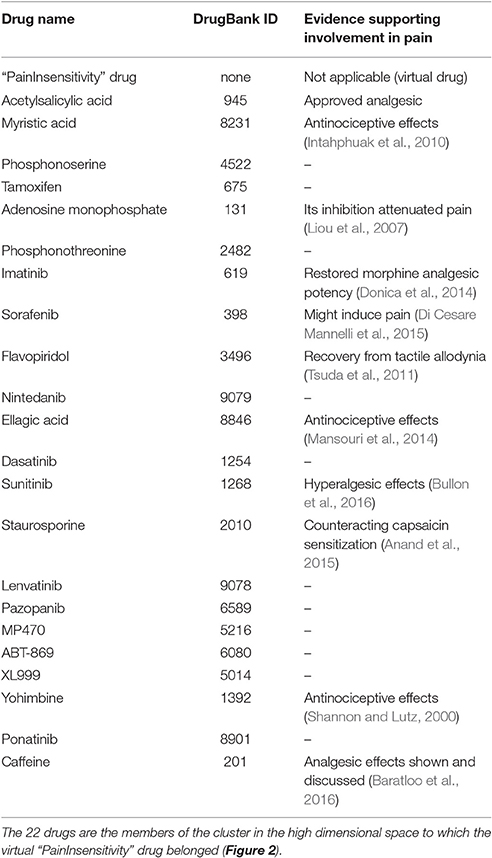
Table 5. Candidate DrugBank listed substances that qualify for repurposing as treatments of pain, according to the similarity between the biological processes associated with the n = 20 genes causally involved in human insensitivity to pain and captured in the virtual “PainInsensitivity” drug, and the biological processes in which the 413 drugs queried from the DrugBank database (Wishart et al., 2006, 2008) are involved.
Discussion
The present analysis used empirical evidence for human genes that when nonfunctional may cause the phenotype of insensitivity to pain. The set of n = 20 genes included those causally involved in hereditary sensory and autonomic neuropathies and for long in the center of pain research, and in addition further genes involved in several different neurological syndromes or, such as SCN9A, genes that when nonfunctional are mainly associated with insensitivity to pain. Indeed, the only additional phenotype in subjects carrying loss-of-function mutations in SCN9A was anosmia (Cox et al., 2010). In the present analysis, the common phenotype of insensitivity to pain associated with the complete gene set was used to address the functional background of pain insensitivity and to explore a computational attempt at using the information for drug repurposing. The computational approach allowed using data on the biological functions of genes acquired in any context, without restriction to pain research (Lötsch et al., 2013).
The computational analysis of genes associated with human insensitivity to pain included, in addition to basic functions as neuronal signaling or the perception of pain, as major components (i) nervous system development and structural component assembly and (ii) lipid-mediator based signaling including sphingosines and ceramides. Nervous system development, while presently probably also associated with the hereditary developmental disorders of the nervous system, appear to be a consistent part of the genetic background of pain since they also emerged as particularly prominent systemic functions of larger sets of genes related to all aspects of pain (Ultsch et al., 2016). As discussed there, as possible explanation of the consistent appearance of nervous system development among key biological processes exerted by pain-relevant sets of genes involves the concept of chronic pain as a dysregulation in biological processes that describe its systemic features of learning and neuronal plasticity (Mansour et al., 2014). Thus, the structural aspect of nervous system development would be compatible with both, hereditary developmental neuropathies and pain.
A role of lipid signaling is compatible with prior knowledge of the pathophysiology of pain. Indeed, the ceramide-to-sphingosine pathway has been proposed already as a therapeutic target in pain (Salvemini et al., 2013). Similarly, sphingosine-1-phosphate induced nociceptor excitation and ongoing pain behavior in mice and humans (Camprubi-Robles et al., 2013) and therefore, sphingosine-1-phosphate receptors have been proposed as novel targets for the treatment of pain (Welch et al., 2012). While these findings have emerged from basic research, the present prominent role of this pathway highlights the suitability of the computational approach analyzing available information about a particular subset of pain-related genes. Again, the biological plausibility applies to both, developmental neuropathies and pain.
The idea behind using the knowledge about the biological processes characterizing insensitivity to pain for drug repurposing is to use the similarity measure in the high-dimensional vector space of the drug's interactions with biological processes for the identification of substances qualifying for the treatment of traits defined on the basis of biological processes (Lötsch and Ultsch, 2016b). A disease-relevant gene set is functionally analyzed and compared with the biological processes in which the targets of available drugs are involved. This approach presently resulted in the selection of a subset of n = 22 substances, which is the size of the cluster that surrounded the functional information of the present trait of interest in the high-dimensional data space (Figure 2). Results obtained with the present computational approach require basic research verification. However, the set included substances for which such evidence could be found. For example, myristic acid is contained at 18.64% in coconut oil, which when orally administered to rats produced moderate anti-inflammatory and anti-nociceptive effects (Intahphuak et al., 2010). Imatinib, a tyrosine kinase inhibitor, had no antinociceptive effects in a nerve injury model in rats when administered alone; however, when combined with a previously ineffective dose of morphine, complete pain relief was obtained (Donica et al., 2014), which was attributed to its platelet-derived growth factor inhibiting actions (Mcgary et al., 2004).
Similarly, flavopiridol or alvocidib, an inhibitor of cyclin-dependent kinases, was shown to facilitate the recovery from tactile allodynia when in a rat nerve injury model, which was attributed to its Janus kinase pathway inhibiting actions (Tsuda et al., 2011). Ellagic acid, a polyphenolic compound from plants such as raspberries, eucalyptus, and nuts (Clifford and Scalbert, 2000), showed antinociceptive effects and potentiated the effects of morphine in different rat models of pain (Mansouri et al., 2014). Furthermore, staurosporine, an alkaloid initially isolated from the bacterium Streptomyces staurosporeus (Omura et al., 1977) and found to inhibit protein kinases, inhibited Angiotensin II mediated sensitization of post mortem analyzed human nerves (Anand et al., 2015), which is in line with the targeting of angiotensin 2 type II receptors as a novel treatment for neuropathic pain (Rice et al., 2014).
However, the list of candidate drugs for repurposing also included the multitargeted receptor tyrosine kinase inhibitors sorafenib and sunitinib, for which evidence suggests hyperalgesic actions. This emphasizes that, as discussed previously (Lötsch and Ultsch, 2016b), a limitation of the present implementation of this computational approach to drug repurposing consist of the unsigned inclusion of drug vs. target interactions, i.e., without distinction of agonistic from antagonistic actions. Therefore, topical experts' knowledge is required to amend this limitation. Of further note, the present computational approach at drug repurposing based on computational analysis of gene functions and similarity measures in the high-dimensional data space still depends on the accuracy and completeness of the information in the queried databases. This makes it vulnerable to research bias and erroneous information in the databases, however, the increasing trend toward “big data” supports the expectation of a continuously broadening knowledge base.
The present computational analysis of the genes involved in human hereditary insensitivity to pain in a drug repurposing context extends previous applications of the recently proposed concept of “process pharmacology” (Lötsch and Ultsch, 2016b) by (i) the inclusion of a topically selected set of genes in addition to a previously used set of genes derived from microarray analysis, and (ii) the introduction of the concept of a “virtual drug,” i.e., a vector of biological processes representing the key functions of a gene set that can be entered as a further drug into the drug vs. biological process matrix, thereby facilitating the search for repurposing candidates by using similarity measures in the high dimensional vector space. As described previously (Lötsch and Ultsch, 2016a,b), in the present approach diseases are regarded as resulting from the activity of pathophysiological processes captured in the GO database via the category “biological processes,” i.e., series of events or molecular functions with a defined beginning and end (Ashburner et al., 2000). Such a focus on disease relevant biological processes (Lötsch and Ultsch, 2016b) has been shown to provide a robust basis for drug classification agreeing with classical approaches (Lötsch and Ultsch, 2016a) and may provide a phenotypic approach to drug discovery and repurposing.
The present ORAs were performed with different p-value thresholds. The conservative p-value threshold of tp = 1·10−15 used for the analysis of the drug targets was heuristically chosen to accommodate the intention to only include highly relevant terms and to obtain a set size of 500–1,000 terms that had proven suitable in previous similar analyses (Lötsch and Ultsch, 2016a,b). By contrast, the p-value threshold of 0.05 chosen for the ORA of the n = 20 genes associated with human insensitivity to pain was chosen for practical reasons. That is, it was the strictest criterion providing the generally accepted significance level of p = 0.05 and a correction for multiple testing, a stricter criterion or the use of more conservative α-correction according to Bonferroni (1936) led to a nearly empty ORA result for the present set of n = 20 genes involved inhuman insensitivity to pain and could therefore not applied. However, a systematic test of the optimum p-value threshold for the present computational drug repurposing approach remains an active research topic to be addressed in future work.
By placing the modulation of biological processes into the focus with molecular targets merely serving as intermediates, the present concept may exceed the particular molecular mechanism by which a drug acts making it particularly suitable for drug repurposing by eliminating the restriction to a specific molecular target. In this respect, the present concept complements non-redundantly alternative implementations of computational science in drug research and development. For example, the analysis of molecular interaction networks (e.g., protein-protein interaction networks or gene-gene co-expression networks) may be employed to study the possible consequences of target removals, considering a target as effective when its removal modifies the network in an essential way (Penrod et al., 2011). Other approaches proposed to use supervised machine learning, i.e., support vector machines, to analyze druggable protein-protein interaction on the basis of the number of shared GO terms indicating similarities in biological function between two interacting proteins (Sugaya et al., 2007; Sugaya and Ikeda, 2009). Further approaches include pathway based analysis where pathway annotations, such as provided by the Kyoto Encyclopedia of Genes and Genomes (KEGG) pathway database are “translated into a two-dimensional statistical test problem that involves Wilcoxon's signed rank sum test in order to compute a Z-score for each pathway that quantifies the degree of alteration across the different experimental conditions” (Herwig and Lehrach, 2006). These approaches have also shown to provide clinically useful approaches to drug development such as the identification of anticancer drug combinations (Azmi et al., 2010), which complements a similar success of the present method (Lötsch and Ultsch, 2016b). This emphasizes the increasing utility of a variety of computational approaches to gene functions in drug research, for which the present analysis adds further support.
Most contemporary machine learning techniques for the analysis of genomic data are of the supervised learning type (for a recent review, see Libbrecht and Noble, 2015). These methods aim at the diagnosis (classification) of cases. By contrast, in the present work unsupervised methods were used, which mainly aim at structure detection in high dimensional (genetic) data. Typical methods for structure detection in high dimensional data are (i) nonlinear projections such as multidimensional scaling (MDS) (Tzeng et al., 2008), t-SNE (van der Maaten and Hinton, 2008; Bushati et al., 2011) or curvilinear component analysis (Alanis-Lobato et al., 2015) and (ii) clustering methods summarized in (Pirim et al., 2012). The ESOM/Umatrix method used here can be regarded as a combination of a disentangling and neighborhood preserving projection method combined with a clustering algorithm. This method has been demonstrated to be superior to other approaches in the sense, that complex and intertwined clusters can be identified, however, no spurious structures are artificially introduced by the clustering method itself (Ultsch and Lötsch, 2017) for which a superiority to many other projection methods has been shown (Tasdemir and Merényi, 2012).
Conclusions
Genes causally involved in human insensitivity to pain provide a unique source of studying the pathophysiology of pain and the development of novel analgesic drugs. In keeping with the contemporary trend toward “big data” analysis in biomedical research, an integrated computational analysis was performed to study the set of genes for emergent, principal pathophysiological processes that characterize insensitivity to pain. As a result, a particular importance for pain perception was observed for processes related to structural changes in the nervous system and to ceramide and sphingosine signaling pathways, which is compatible with suggestions of using these pathways as therapeutic target in pain. Using the biological processes characterizing hereditary insensitivity to pain for drug repurposing, a clear cluster of n = 22 substances emerged that comprised several drugs for which implications in pain have been shown occasionally in preclinical experiments. Thus, the present concept provides biologically plausible results and seems to be suitable for drug discovery by identifying a narrow choice of repurposing candidates, demonstrating that contemporary machine-learned methods offer innovative approaches to knowledge discovery from previous evidence.
Author Contributions
Wrote the paper, conception or design of the work: JL. Analyzed the data: JL and CL. Data collection: DK and JL. Provided advice for data analysis: AU.
Funding
This work has been funded by the Landesoffensive zur Entwicklung wissenschaftlich-ökonomischer Exzellenz (LOEWE), LOEWE-Zentrum für Translationale Medizin und Pharmakologie (JL) with the specific project funding under the name “Process pharmacology: A data science based approach to drug repurposing” and by the European Union Seventh Framework Programme (FP7/2007–2013) under grant agreement no. 602919 (JL, GLORIA). The funders had no role in study design, data collection and analysis, decision to publish, or preparation of the manuscript.
Conflict of Interest Statement
The authors declare that the research was conducted in the absence of any commercial or financial relationships that could be construed as a potential conflict of interest.
Supplementary Material
The Supplementary Material for this article can be found online at: http://journal.frontiersin.org/article/10.3389/fnmol.2017.00252/full#supplementary-material
Supplementary Figure 1. This figure shows the top-down representation of the GO terms representing the biological processes in which the set of n = 20 genes causally involved in hereditary absence of pain perception (Table 1) is involved (for an enlarged version, see the Supplementary Figure). The graphical representation follows the standard of the GO knowledgebase, where GO terms are related to each other by “is-a,” “part-of,” and “regulates” relationships forming a polyhierarchy organized in a directed acyclic graph (DAG, Thulasiraman and Swamy, 1992). The figure represents the results of an over-representation analysis with parameters for the p-value threshold, tp = 0.05 and FDR α-correction. (Top) Significant terms are shown as colored circles with the number of member genes, the number of expected genes by chance and the significance of the deviation in the observed from the expected number of genes indicated (yellow = headline, red = significant term located in the polyhierarchy below a functional area). Blue vertices or blue labels, are the most specific terms (leaves of the DAG) at the end of a taxonomy in the polyhierarchy. The biological processes in which these genes are involved can be summarized by seven primary “functional areas” representing the most remarkable nodes with respect to their localization in the polyhierarchy. (Bottom) Zoomed parts of particular functional areas, recreated in a slightly different arrangement to enhance visibility.
References
Alanis-Lobato, G., Cannistraci, C. V., Eriksson, A., Manica, A., and Ravasi, T. (2015). Highlighting nonlinear patterns in population genetics datasets. Sci. Rep. 5:8140. doi: 10.1038/srep08140
Anand, U., Yiangou, Y., Sinisi, M., Fox, M., Macquillan, A., Quick, T., et al. (2015). Mechanisms underlying clinical efficacy of Angiotensin II type 2 receptor (AT2R) antagonist EMA401 in neuropathic pain: clinical tissue and in vitro studies. Mol. Pain 11:38. doi: 10.1186/s12990-015-0038-x
Anderson, S. L., Coli, R., Daly, I. W., Kichula, E. A., Rork, M. J., Volpi, S. A., et al. (2001). Familial dysautonomia is caused by mutations of the IKAP gene. Am. J. Hum. Genet. 68, 753–758. doi: 10.1086/318808
Ashburn, T. T., and Thor, K. B. (2004). Drug repositioning: identifying and developing new uses for existing drugs. Nat. Rev. Drug Discov. 3, 673–683. doi: 10.1038/nrd1468
Ashburner, M., Ball, C. A., Blake, J. A., Botstein, D., Butler, H., Cherry, J. M., et al. (2000). Gene ontology: tool for the unification of biology. Gene Ontol. Consort. Nat. Genet. 25, 25–29. doi: 10.1038/75556
Azmi, A. S., Wang, Z., Philip, P. A., Mohammad, R. M., and Sarkar, F. H. (2010). Proof of concept: network and systems biology approaches aid in the discovery of potent anticancer drug combinations. Mol. Cancer Ther. 9, 3137–3144. doi: 10.1158/1535-7163.MCT-10-0642
Backes, C., Keller, A., Kuentzer, J., Kneissl, B., Comtesse, N., Elnakady, Y. A., et al. (2007). Genetrail–advanced gene set enrichment analysis. Nucleic Acids Res. 35, W186–W192. doi: 10.1093/nar/gkm323
Baratloo, A., Rouhipour, A., Forouzanfar, M. M., Safari, S., Amiri, M., and Negida, A. (2016). The role of Caffeine in pain management: a brief literature review. Anesth. Pain Med. 6:e33193. doi: 10.5812/aapm.33193
Benjamini, Y., and Hochberg, Y. (1995). Controlling the false discovery rate - a practical and powerful approach to multiple testing. J. R. Stat. Soc. B. 57, 289–300.
Bonferroni, C. E. (1936). Teoria statistica delle classi e calcolo delle probabilita. Pubblicazioni del R Ist. Super. di Sci. Economiche e Commerciali di Firenze 8, 3–62.
Breivik, H., Collett, B., Ventafridda, V., Cohen, R., and Gallacher, D. (2006). Survey of chronic pain in Europe: prevalence, impact on daily life, and treatment. Eur. J. Pain 10, 287–333. doi: 10.1016/j.ejpain.2005.06.009
Bullon, P., Alcocer-Gomez, E., Carrion, A. M., Marin-Aguilar, F., Garrido-Maraver, J., Roman-Malo, L., et al. (2016). AMPK phosphorylation modulates pain by activation of NLRP3 Inflammasome. Antioxid. Redox Signal. 24, 157–170. doi: 10.1089/ars.2014.6120
Bushati, N., Smith, J., Briscoe, J., and Watkins, C. (2011). An intuitive graphical visualization technique for the interrogation of transcriptome data. Nucleic Acids Res. 39, 7380–7389. doi: 10.1093/nar/gkr462
Camon, E., Magrane, M., Barrell, D., Binns, D., Fleischmann, W., Kersey, P., et al. (2003). The gene ontology annotation (GOA) project: implementation of GO in SWISS-PROT, TrEMBL, and InterPro. Genome Res. 13, 662–672. doi: 10.1101/gr.461403
Camon, E., Magrane, M., Barrell, D., Lee, V., Dimmer, E., Maslen, J., et al. (2004). The gene ontology annotation (GOA) database: sharing knowledge in uniprot with gene ontology. Nucleic Acids Res. 32, D262–D266. doi: 10.1093/nar/gkh021
Camprubi-Robles, M., Mair, N., Andratsch, M., Benetti, C., Beroukas, D., Rukwied, R., et al. (2013). Sphingosine-1-phosphate-induced nociceptor excitation and ongoing pain behavior in mice and humans is largely mediated by S1P3 receptor. J. Neurosci. 33, 2582–2592. doi: 10.1523/JNEUROSCI.4479-12.2013
Carbon, S., Ireland, A., Mungall, C. J., Shu, S., Marshall, B., Lewis, S., et al. (2009). AmiGO: online access to ontology and annotation data. Bioinformatics 25, 288–289. doi: 10.1093/bioinformatics/btn615
Chao, H.-T., Davids, M., Burke, E., Pappas, J. G., Rosenfeld, J. A., Mccarty, A. J., et al. (2017). A syndromic neurodevelopmental disorder caused by De Novo variants in EBF3. Am. J. Hum. Genet. 100, 128–137. doi: 10.1016/j.ajhg.2016.11.018
Chen, Y. C., Auer-Grumbach, M., Matsukawa, S., Zitzelsberger, M., Themistocleous, A. C., Strom, T. M., et al. (2015). Transcriptional regulator PRDM12 is essential for human pain perception. Nat. Genet. 47, 803–808. doi: 10.1038/ng.3308
Clifford, M. N., and Scalbert, A. (2000). Ellagitannins – nature, occurrence and dietary burden. J. Sci. Food Agric. 80, 1118–1125. doi: 10.1002/(SICI)1097-0010(20000515)80:7<1118::AID-JSFA570>3.0.CO;2-9
Cox, J. J., Reimann, F., Nicholas, A. K., Thornton, G., Roberts, E., Springell, K., et al. (2006). An SCN9A channelopathy causes congenital inability to experience pain. Nature 444, 894–898. doi: 10.1038/nature05413
Cox, J. J., Sheynin, J., Shorer, Z., Reimann, F., Nicholas, A. K., Zubovic, L., et al. (2010). Congenital insensitivity to pain: novel SCN9A missense and in-frame deletion mutations. Hum. Mutat. 31, E1670–E186. doi: 10.1002/humu.21325
Dawkins, J. L., Hulme, D. J., Brahmbhatt, S. B., Auer-Grumbach, M., and Nicholson, G. A. (2001). Mutations in SPTLC1, encoding serine palmitoyltransferase, long chain base subunit-1, cause hereditary sensory neuropathy type I. Nat. Genet. 27, 309–312. doi: 10.1038/85879
Di Cesare Mannelli, L., Maresca, M., Farina, C., Scherz, M. W., and Ghelardini, C. (2015). A model of neuropathic pain induced by sorafenib in the rat: effect of dimiracetam. Neurotoxicology 50, 101–107. doi: 10.1016/j.neuro.2015.08.002
Donica, C. L., Cui, Y., Shi, S., and Gutstein, H. B. (2014). Platelet-derived growth factor receptor-beta antagonism restores morphine analgesic potency against neuropathic pain. PLoS ONE 9:e97105. doi: 10.1371/journal.pone.0097105
Einarsdottir, E., Carlsson, A., Minde, J., Toolanen, G., Svensson, O., Solders, G., et al. (2004). A mutation in the nerve growth factor beta gene (NGFB) causes loss of pain perception. Hum. Mol. Genet. 13, 799–805. doi: 10.1093/hmg/ddh096
Elliott, A. M., Smith, B. H., Penny, K. I., Smith, W. C., and Chambers, W. A. (1999). The epidemiology of chronic pain in the community. Lancet 354, 1248–1252. doi: 10.1016/S0140-6736(99)03057-3
Elsaid, M. F., Chalhoub, N., Kamel, H., Ehlayel, M., Ibrahim, N., Elsaid, A., et al. (2016). Non-truncating LIFR mutation: causal for prominent congenital pain insensitivity phenotype with progressive vertebral destruction? Clin. Genet. 89, 210–216. doi: 10.1111/cge.12657
Fisher, R. A. (1922). On the interpretation of Chi square from contingency tables, and the calculation of P. J. R. Stat. Soc. 85, 87–94. doi: 10.2307/2340521
Gastwirth, J. L., and Glauberman, M. (1976). The interpolation of the lorenz curve and gini index from grouped data. Econometrica 44, 479–483. doi: 10.2307/1913977
Goldberg, Y. P., Pimstone, S. N., Namdari, R., Price, N., Cohen, C., Sherrington, R. P., et al. (2012). Human mendelian pain disorders: a key to discovery and validation of novel analgesics. Clin. Genet. 82, 367–373. doi: 10.1111/j.1399-0004.2012.01942.x
Guelly, C., Zhu, P. P., Leonardis, L., Papic, L., Zidar, J., Schabhuttl, M., et al. (2011). Targeted high-throughput sequencing identifies mutations in atlastin-1 as a cause of hereditary sensory neuropathy type I. Am. J. Hum. Genet. 88, 99–105. doi: 10.1016/j.ajhg.2010.12.003
Herwig, R., and Lehrach, H. (2006). Expression profiling of drug response-from genes to pathways. Dialogues Clin. Neurosci. 8, 283–293.
Indo, Y., Tsuruta, M., Hayashida, Y., Karim, M. A., Ohta, K., Kawano, T., et al. (1996). Mutations in the TRKA/NGF receptor gene in patients with congenital insensitivity to pain with anhidrosis. Nat. Genet. 13, 485–488. doi: 10.1038/ng0896-485
Intahphuak, S., Khonsung, P., and Panthong, A. (2010). Anti-inflammatory, analgesic, and antipyretic activities of virgin coconut oil. Pharm. Biol. 48, 151–157. doi: 10.3109/13880200903062614
Janssens, K., Goethals, S., Atkinson, D., Ermanoska, B., Fransen, E., Jordanova, A., et al. (2014). Human Rab7 mutation mimics features of charcot-marie-tooth neuropathy type 2B in Drosophila. Neurobiol. Dis. 65, 211–219. doi: 10.1016/j.nbd.2014.01.021
Julius, D., and Basbaum, A. I. (2001). Molecular mechanisms of nociception. Nature 413, 203–210. doi: 10.1038/35093019
Karadimas, C. L., Vu, T. H., Holve, S. A., Chronopoulou, P., Quinzii, C., Johnsen, S. D., et al. (2006). Navajo neurohepatopathy is caused by a mutation in the MPV17 gene. Am. J. Hum. Genet. 79, 544–548. doi: 10.1086/506913
Kehlet, H., Jensen, T. S., and Woolf, C. J. (2006). Persistent postsurgical pain: risk factors and prevention. Lancet 367, 1618–1625. doi: 10.1016/S0140-6736(06)68700-X
Kohonen, T. (1982). Self-organized formation of topologically correct feature maps. Biol. Cybern. 43, 59–69. doi: 10.1007/BF00337288
Kornak, U., Mademan, I., Schinke, M., Voigt, M., Krawitz, P., Hecht, J., et al. (2014). Sensory neuropathy with bone destruction due to a mutation in the membrane-shaping atlastin GTPase 3. Brain 137, 683–692. doi: 10.1093/brain/awt357
Kringel, D., and Lötsch, J. (2015). Pain research funding by the European union seventh framework programme. Eur. J. Pain 19, 595–600. doi: 10.1002/ejp.690
Kurth, I., Pamminger, T., Hennings, J. C., Soehendra, D., Huebner, A. K., Rotthier, A., et al. (2009). Mutations in FAM134B, encoding a newly identified Golgi protein, cause severe sensory and autonomic neuropathy. Nat. Genet. 41, 1179–1181. doi: 10.1038/ng.464
Lafreniere, R. G., Macdonald, M. L., Dube, M. P., Macfarlane, J., O'driscoll, M., Brais, B., et al. (2004). Identification of a novel gene (HSN2) causing hereditary sensory and autonomic neuropathy type II through the study of Canadian genetic isolates. Am. J. Hum. Genet. 74, 1064–1073. doi: 10.1086/420795
Leipold, E., Liebmann, L., Korenke, G. C., Heinrich, T., Giesselmann, S., Baets, J., et al. (2013). A de novo gain-of-function mutation in SCN11A causes loss of pain perception. Nat. Genet. 45, 1399–1404. doi: 10.1038/ng.2767
Libbrecht, M. W., and Noble, W. S. (2015). Machine learning applications in genetics and genomics. Nat. Rev. Genet. 16, 321–332. doi: 10.1038/nrg3920
Liou, J. T., Liu, F. C., Hsin, S. T., Yang, C. Y., and Lui, P. W. (2007). Inhibition of the cyclic adenosine monophosphate pathway attenuates neuropathic pain and reduces phosphorylation of cyclic adenosine monophosphate response element-binding in the spinal cord after partial sciatic nerve ligation in rats. Anesth. Analg. 105, 1830–1837, doi: 10.1213/01.ane.0000287652.42309.5c
Lötsch, J., Doehring, A., Mogil, J. S., Arndt, T., Geisslinger, G., and Ultsch, A. (2013). Functional genomics of pain in analgesic drug development and therapy. Pharmacol. Ther. 139, 60–70. doi: 10.1016/j.pharmthera.2013.04.004
Lötsch, J., and Ultsch, A. (2014). “Exploiting the structures of the U-matrix,” in Advances in Intelligent Systems and Computing, eds T. Villmann, F. M. Schleif, M. Kaden and M. Lange. (Heidelberg: Springer), 248–257.
Lötsch, J., and Ultsch, A. (2016a). A machine-learned computational functional genomics-based approach to drug classification. Eur. J. Clin. Pharmacol. 72, 1449–1461. doi: 10.1007/s00228-016-2134-x
Lötsch, J., and Ultsch, A. (2016b). Process pharmacology: a pharmacological data science approach to drug development and therapy. CPT Pharmacometrics Syst. Pharmacol. 5, 192–200 doi: 10.1002/psp4.12072
Mansour, A. R., Farmer, M. A., Baliki, M. N., and Apkarian, A. V. (2014). Chronic pain: the role of learning and brain plasticity. Restor. Neurol. Neurosci. 32, 129–139. doi: 10.3233/RNN-139003
Mansouri, M. T., Naghizadeh, B., and Ghorbanzadeh, B. (2014). Ellagic acid enhances morphine analgesia and attenuates the development of morphine tolerance and dependence in mice. Eur. J. Pharmacol. 741, 272–280. doi: 10.1016/j.ejphar.2014.08.024
Mcgary, E. C., Onn, A., Mills, L., Heimberger, A., Eton, O., Thomas, G. W., et al. (2004). Imatinib mesylate inhibits platelet-derived growth factor receptor phosphorylation of melanoma cells but does not affect tumorigenicity in vivo. J. Invest. Dermatol. 122, 400–405. doi: 10.1046/j.0022-202X.2004.22231.x
Miller, G. A. (1956). The magical number seven plus or minus two: some limits on our capacity for processing information. Psychol. Rev. 63, 81–97. doi: 10.1037/h0043158
Nahorski, M. S., Al-Gazali, L., Hertecant, J., Owen, D. J., Borner, G. H., Chen, Y. C., et al. (2015). A novel disorder reveals clathrin heavy chain-22 is essential for human pain and touch development. Brain 138, 2147–2160. doi: 10.1093/brain/awv149
Omura, S., Iwai, Y., Hirano, A., Nakagawa, A., Awaya, J., Tsuchya, H., et al. (1977). A new alkaloid AM-2282 OF Streptomyces origin. Taxonomy, fermentation, isolation and preliminary characterization. J. Antibiot. 30, 275–282. doi: 10.7164/antibiotics.30.275
Pareto, V. (1909). Manuale Di Economia Politica. Milan: Società Editrice Libraria, Revised and Translated into French as Manuel D'économie Politique. Paris: Giard et Briére.
Penrod, N. M., Cowper-Sal-Lari, R., and Moore, J. H. (2011). Systems genetics for drug target discovery. Trends Pharmacol. Sci. 32, 623–630. doi: 10.1016/j.tips.2011.07.002
Pirim, H., Eksioglu, B., Perkins, A., and Yuceer, C. (2012). Clustering of high throughput gene expression data. Comput. Oper. Res. 39, 3046–3061. doi: 10.1016/j.cor.2012.03.008
Portenoy, R. K. (1992). Cancer pain: pathophysiology and syndromes. Lancet 339, 1026–1031. doi: 10.1016/0140-6736(92)90545-E
President's Information Technology Advisory Committee (2005). Report to the President: Computational Science: Ensuring America's Competitiveness. Arlington: National Coordination Office for Information Technology Research and Development.
Rebhan, M., Chalifa-Caspi, V., Prilusky, J., and Lancet, D. (1997). Genecards: integrating information about genes, proteins and diseases. Trends Genet. 13:163. doi: 10.1016/S0168-9525(97)01103-7
Rice, A. S., Dworkin, R. H., Mccarthy, T. D., Anand, P., Bountra, C., Mccloud, P. I., et al. (2014). EMA401, an orally administered highly selective angiotensin II type 2 receptor antagonist, as a novel treatment for postherpetic neuralgia: a randomised, double-blind, placebo-controlled phase 2 clinical trial. Lancet 383, 1637–1647. doi: 10.1016/S0140-6736(13)62337-5
Riviere, J. B., Ramalingam, S., Lavastre, V., Shekarabi, M., Holbert, S., Lafontaine, J., et al. (2011). KIF1A, an axonal transporter of synaptic vesicles, is mutated in hereditary sensory and autonomic neuropathy type 2. Am. J. Hum. Genet. 89, 219–230. doi: 10.1016/j.ajhg.2011.06.013
Rotthier, A., Baets, J., De Vriendt, E., Jacobs, A., Auer-Grumbach, M., Levy, N., et al. (2009). Genes for hereditary sensory and autonomic neuropathies: a genotype-phenotype correlation. Brain 132, 2699–2711. doi: 10.1093/brain/awp198
Salvemini, D., Doyle, T., Kress, M., and Nicol, G. (2013). Therapeutic targeting of the ceramide-to-sphingosine 1-phosphate pathway in pain. Trends Pharmacol. Sci. 34, 110–118. doi: 10.1016/j.tips.2012.12.001
Schaible, H. G. (2007). Peripheral and central mechanisms of pain generation. Handb. Exp. Pharmacol. 177, 3–28.
Seal, R. L., Gordon, S. M., Lush, M. J., Wright, M. W., and Bruford, E. A. (2011). Genenames.org: the HGNC resources in 2011. Nucleic Acids Res. 39, D514–D519. doi: 10.1093/nar/gkq892
Shannon, H. E., and Lutz, E. A. (2000). Yohimbine produces antinociception in the formalin test in rats: involvement of serotonin(1A) receptors. Psychopharmacology 149, 93–97. doi: 10.1007/s002139900343
Sheffer, R., Douiev, L., Edvardson, S., Shaag, A., Tamimi, K., Soiferman, D., et al. (2016). Postnatal microcephaly and pain insensitivity due to a de novo heterozygous DNM1L mutation causing impaired mitochondrial fission and function. Am. J. Med. Genet. A 170, 1603–1607. doi: 10.1002/ajmg.a.37624
Slaugenhaupt, S. A., Blumenfeld, A., Gill, S. P., Leyne, M., Mull, J., Cuajungco, M. P., et al. (2001). Tissue-specific expression of a splicing mutation in the IKBKAP gene causes familial dysautonomia. Am. J. Hum. Genet. 68, 598–605. doi: 10.1086/318810
Sugaya, N., and Ikeda, K. (2009). Assessing the druggability of protein-protein interactions by a supervised machine-learning method. BMC Bioinform. 10:263. doi: 10.1186/1471-2105-10-263
Sugaya, N., Ikeda, K., Tashiro, T., Takeda, S., Otomo, J., Ishida, Y., et al. (2007). An integrative in silico approach for discovering candidates for drug-targetable protein-protein interactions in interactome data. BMC Pharmacol. 7:10. doi: 10.1186/1471-2210-7-10
Swoboda, K. J., Engle, E. C., Scheindlin, B., Anthony, D. C., and Jones, H. R. (1998). Mutilating hand syndrome in an infant with familial carpal tunnel syndrome. Muscle Nerve 21, 104–111.
Tasdemir, K., and Merényi, E. (2012). Som-Based Topology Visualization for Interactive Analysis of High-Dimensional Large Datasets. Machine Learning Reports, University of Bielefeld.
Tsuda, M., Kohro, Y., Yano, T., Tsujikawa, T., Kitano, J., Tozaki-Saitoh, H., et al. (2011). JAK-STAT3 pathway regulates spinal astrocyte proliferation and neuropathic pain maintenance in rats. Brain 134, 1127–1139. doi: 10.1093/brain/awr025
Tzeng, J., Lu, H. H., and Li, W. H. (2008). Multidimensional scaling for large genomic data sets. BMC Bioinform. 9:179. doi: 10.1186/1471-2105-9-179
Ultsch, A. (2003). “Maps for visualization of high-dimensional data spaces,” in Proceeding Workshop on Self-Organizing Maps (WSOM) (Kyushu), 225–230.
Ultsch, A., Kringel, D., Kalso, E., Mogil, J. S., and Lötsch, J. (2016). A data science approach to candidate gene selection of pain regarded as a process of learning and neural plasticity. Pain 157, 2747–2757. doi: 10.1097/j.pain.0000000000000694
Ultsch, A., and Lötsch, J. (2014). Functional abstraction as a method to discover knowledge in gene ontologies. PLoS ONE 9:e90191. doi: 10.1371/journal.pone.0090191
Ultsch, A., and Lötsch, J. (2015). Computed ABC analysis for rational selection of most informative variables in multivariate data. PLoS ONE 10:e0129767. doi: 10.1371/journal.pone.0129767
Ultsch, A., and Lötsch, J. (2017). Machine-learned cluster identification in high-dimensional data. J. Biomed. Inform. 66, 95–104. doi: 10.1016/j.jbi.2016.12.011
Ultsch, A., and Sieman, H. P. (1990). “Kohonen's self organizing feature maps for exploratory data analysis”, in INNC'90, International Neural Network Conference (Alphen aan den Rijn: Wolters-Kluwer), 305–308.
van der Maaten, L. J. P., and Hinton, G. E. (2008). Visualizing high-dimensional data using t-SNE. J. Mach. Learn. Res. 9, 2579–2605.
Verhoeven, K., De Jonghe, P., Coen, K., Verpoorten, N., Auer-Grumbach, M., Kwon, J. M., et al. (2003). Mutations in the small GTP-ase late endosomal protein RAB7 cause Charcot-Marie-Tooth type 2B neuropathy. Am. J. Hum. Genet. 72, 722–727. doi: 10.1086/367847
Welch, S. P., Sim-Selley, L. J., and Selley, D. E. (2012). Sphingosine-1-phosphate receptors as emerging targets for treatment of pain. Biochem. Pharmacol. 84, 1551–1562. doi: 10.1016/j.bcp.2012.08.010
Wishart, D. S., Knox, C., Guo, A. C., Cheng, D., Shrivastava, S., Tzur, D., et al. (2008). DrugBank: a knowledgebase for drugs, drug actions and drug targets. Nucleic Acids Res. 36, D901–D906. doi: 10.1093/nar/gkm958
Wishart, D. S., Knox, C., Guo, A. C., Shrivastava, S., Hassanali, M., Stothard, P., et al. (2006). DrugBank: a comprehensive resource for in silico drug discovery and exploration. Nucleic Acids Res. 34, D668–D672. doi: 10.1093/nar/gkj067
Keywords: data science, computational biology, pain, humans, genetic variation, machine learning, perception, big data
Citation: Lötsch J, Lippmann C, Kringel D and Ultsch A (2017) Integrated Computational Analysis of Genes Associated with Human Hereditary Insensitivity to Pain. A Drug Repurposing Perspective. Front. Mol. Neurosci. 10:252. doi: 10.3389/fnmol.2017.00252
Received: 02 April 2017; Accepted: 26 July 2017;
Published: 08 August 2017.
Edited by:
Michaela Kress, Innsbruck Medical University, AustriaReviewed by:
Eleftherios Pilalis, National Hellenic Research Foundation, GreecePatrick May, University of Luxembourg, Luxembourg
Copyright © 2017 Lötsch, Lippmann, Kringel and Ultsch. This is an open-access article distributed under the terms of the Creative Commons Attribution License (CC BY). The use, distribution or reproduction in other forums is permitted, provided the original author(s) or licensor are credited and that the original publication in this journal is cited, in accordance with accepted academic practice. No use, distribution or reproduction is permitted which does not comply with these terms.
*Correspondence: Jörn Lötsch, j.loetsch@em.uni-frankfurt.de