- 1Boston Children’s Hospital, Boston, MA, United States
- 2Harvard Medical School, Boston, MA, United States
- 3University of Massachusetts Memorial Medical Center, Worcester, MA, United States
- 4University of Massachusetts Medical School, Worcester, MA, United States
- 5Lurie Center for Autism, Massachusetts General Hospital for Children, Lexington, MA, United States
- 6Child Study Center, Yale University School of Medicine, New Haven, CT, United States
- 7Boston University Medical Center, Boston, MA, United States
- 8Boston University School of Medicine, Boston, MA, United States
- 9Center for Children with Special Needs, Floating Children’s Hospital at Tufts Medical Center, Boston, MA, United States
- 10Tufts University School of Medicine, Boston, MA, United States
- 11Autism Consortium at Harvard Medical School, Boston, MA, United States
- 12Eunice Kennedy Shriver Center, University of Massachusetts Medical School, Worcester, MA, United States
Background Early identification and treatment of individuals with autism spectrum disorder (ASD) improves outcomes, but specific evidence needed to individualize treatment recommendations is lacking. Biomarkers that could be routinely measured within the clinical setting could potentially transform clinical care for patients with ASD. This demonstration project employed collection of biomarker data during regular autism specialty clinical visits and explored the relationship of biomarkers with clinical ASD symptoms.
Methods Eighty-three children with ASD, aged 5–10 years, completed a multi-site feasibility study integrating the collection of biochemical (blood serotonin, urine melatonin sulfate excretion) and clinical (head circumference, dysmorphology exam, digit ratio, cognitive and behavioral function) biomarkers during routine ASD clinic visits. Parents completed a demographic survey and the Aberrant Behavior Checklist-Community. Cognitive function was determined by record review. Data analysis utilized Wilcoxon two-sample tests and Spearman correlations.
Results Participants were 82% male, 63% White, 19% Hispanic, with a broad range of functioning. Group means indicated hyperserotonemia. In a single regression analysis adjusting for race and median household income, higher income was associated with higher levels of blood serotonin and urine melatonin sulfate excretion levels (p = 0.004 and p = 0.04, respectively). Melatonin correlated negatively with age (p = 0.048) and reported neurologic problems (p = 0.02). Dysmorphic status correlated with higher reported stereotyped behavior (p = 0.02) and inappropriate speech (p = 0.04).
Conclusion This demonstration project employed collection of multiple biomarkers, allowed for examination of associations between biochemical and clinical measures, and identified several findings that suggest direction for future studies. This clinical research model has promise for integrative biomarker research in individuals with complex, heterogeneous neurodevelopmental disorders such as ASD.
Introduction
Autism spectrum disorder (ASD) is a highly heritable, heterogeneous neurodevelopmental disorder characterized by impaired social interaction and communication as well as restricted, repetitive behavior presenting in early childhood (American Psychiatric Association and DSM-5 Task Force, 2013). There is no curative treatment for ASD, but early intensive behavioral treatment can significantly improve long-term developmental outcomes (Lord and Mcgee, 2001; Estes et al., 2015; Reichow et al., 2018). However, specific measures needed to individualize treatment recommendations are lacking. Biomarkers could potentially identify clinically meaningful subgroups within highly heterogeneous populations and thus allow for more precise, individualized medical care by identifying risk, confirming diagnosis or guiding response to treatments (National Research Council, 2011; Veenstra-VanderWeele and Blakely, 2012; Insel, 2014; Interagency Autism CoordinatingCommittee [IACC], 2014; Ruggeri et al., 2014; De Los Reyes and Aldao, 2015; Varcin and Nelson, 2016). Recent authors have advocated for simultaneous measurement of multiple biomarkers to inform the understanding of the numerous systems and complex interactions that are likely to be involved in heterogeneous conditions (Hammock et al., 2012; Schendel et al., 2012; Ruggeri et al., 2014).
An extensive body of research is emerging on potential biomarkers in ASD including genetic, biochemical, proteomic, metabolomic, immune and redox markers as well as neuroimaging, electrophysiologic, physical and behavioral characteristics (Wang et al., 2011; Frustaci et al., 2012; Tordjman et al., 2013; Gabriele et al., 2014; Ruggeri et al., 2014; Varcin and Nelson, 2016).
Biochemical markers include neurotransmitters, hormones and markers of immune function and inflammation. Studies have consistently shown higher mean levels of platelet serotonin in individuals with ASD compared to controls (Schain and Freedman, 1961; Anderson et al., 1987; Cook and Leventhal, 1996; Mulder et al., 2004; Hammock et al., 2012; Gabriele et al., 2014; Pagan et al., 2014). Prior studies have also shown lower plasma levels of the pineal hormone melatonin and overnight urinary excretion of its major metabolite, melatonin sulfate, in individuals with ASD (Tordjman et al., 2005; Pagan et al., 2014). These biochemical markers can also vary with race, age, and gender (McBride et al., 1998; Duffy et al., 2011; Hammock et al., 2012). For example, elevations in platelet serotonin are particularly notable in prepubertal children with ASD (McBride et al., 1998).
A small number of studies have examined relationships between the chosen biomarkers and clinical characteristics in children with ASD. Elevated platelet serotonin levels have been associated in ASD with poorer speech development (Hranilovic et al., 2007), impaired social communication and play skills (Mulder et al., 2010), disruptive behavior (Kuperman et al., 1987), self-injury (Kolevzon et al., 2010), and higher autism severity (Abdulamir et al., 2018). Reduced urinary melatonin was associated with impaired social communication and play skills in ASD (Tordjman et al., 2012), and with higher serotonin levels (Mulder et al., 2010). Physical features such as macrocephaly, lower 2D:4D ratio and dysmorphic features are more common in individuals with ASD compared to controls and may correlate with symptom severity including lower IQ, language deficits and comorbid seizures (Courchesne et al., 1999, 2011; Manning et al., 2001; Miles et al., 2005; Sacco et al., 2007; Schaefer and Mendelsohn, 2008; Miller et al., 2010; Honekopp, 2012). Given the high rates of co-occurring conditions such as GI problems, sleep problems and seizures in ASD, studies examining the relationships of these conditions to biomarkers of interest could further inform understanding of subpopulations within the autism spectrum (Aldinger et al., 2015; Kohane, 2015).
Recently, studies have examined correlations between biomarkers or assessed more than one biomarker simultaneously (Sacco et al., 2010; Hammock et al., 2012; Schendel et al., 2012; Pagan et al., 2014; Ruggeri et al., 2014). For example, Hammock and colleagues found that oxytocin and serotonin were inversely related in individuals with ASD (Hammock et al., 2012). Pagan and colleagues examined associations of biomarkers with autism severity and clinical symptoms (e.g., melatonin with sleep disruption) and found that combined analysis of serotonin, N-acetylserotonin and melatonin levels in individuals differentiated individuals with autism from controls with 80% sensitivity and 85% specificity (Pagan et al., 2014). Sacco and colleagues collected information on a number of clinical traits in a study population and identified clusters of phenotypes (Sacco et al., 2010). The SEED study, a large national epidemiologic study of individuals with ASD and controls, is gathering data on multiple biomarkers and clinical characteristics (Schendel et al., 2012).
These types of studies can help elucidate potential etiologic pathways, distinguish cases from controls and identify subgroups of patients who may be phenotypically similar. One limitation of prior research, however, has been small sample sizes and participation bias. Identification of biomarkers that could be measured within the clinical setting would target large numbers of participants and potentially transform clinical care for patients with ASD (Hammock et al., 2012). Relatively few biomarkers, however, have been studied systematically in children with ASD, and the cost and feasibility of biomarker measurement varies. For example, neurophysiological biomarkers such as EEG require specialized equipment and staff training and cannot be easily completed during a regular follow-up clinic visit (Varcin and Nelson, 2016).
The clinical setting remains a relatively untapped resource for investigation of neurodevelopmental disorders including ASD for several reasons, among them differing priorities between clinical care and research, practical challenges including limited research infrastructure and cultural and attitudinal barriers in many clinical settings. Additionally, the specific core features of ASD such as sensory sensitivity and behavioral rigidity present barriers for individuals to participate in research.
To address these challenges, we conducted a multi-site demonstration project that evaluated the feasibility of integrating collection of biomarker and clinical data during ASD specialty clinic visits (Sices et al., 2017). Our primary hypotheses related to feasibility of the research model and we found that individuals with a range of developmental and behavioral functioning were able to participate and that the study activities did not interfere with clinical care. As part of the study, we collected multiple biomarkers on each participant to maximize the information collected in conjunction with a scheduled clinical visit (as opposed to a separate research visit). Our approach to assessment of correlations between biomarkers was exploratory. The chosen biomarkers, platelet serotonin and urinary melatonin sulfate, head circumference, dysmorphic status, and ratio of second and fourth digits (2D:4D), had prior evidence of association with ASD and could be measured with relative ease, efficiency and economy across multiple sites (Honekopp, 2012; Schendel et al., 2012; Teatero and Netley, 2013; Ruggeri et al., 2014; Mackus et al., 2017; Paynter et al., 2018).
This paper describes the range for platelet serotonin, urinary melatonin sulfate, head circumference, dysmorphic status and 2D:4D ratio in our clinical study population. In addition, this paper explores potential relationships among individual biomarkers, demographic features including sex, socioeconomic status (SES), and clinical features including cognitive level, behavioral function and co-morbid medical symptoms.
Materials and Methods
Participants
We recruited participants from five academic medical centers in Massachusetts that specialize in ASD assessment and treatment. Recruitment and classification procedures have been described previously (Sices et al., 2017). This study was approved by the Institutional Review Board at the lead site (Boston Children’s Hospital).
Children between 5 and 10 years old with a prior clinical diagnosis of ASD and scheduled for a regular clinic follow-up visit between April 2014–May 2015 at one of the five research sites were eligible to participate in the study. ASD diagnoses were verified by the children’s clinicians, all of whom were pediatric specialists with expertise in the diagnosis and treatment of ASD (developmental behavioral pediatricians, pediatric neurologists, nurse practitioners, and child psychiatrists). Participants’ ages were limited to between 5 and 10 years to improve stability of the ASD diagnosis and to increase the likelihood of pre-pubertal status, as some biomarkers vary with puberty.
Only one child per family was enrolled in the study. Exclusion criteria included having a non-English speaking caregiver or taking medication that could affect serotonin or melatonin metabolism within 2–6 weeks of the study visit (Supplementary Table 1). There were no exclusions for epilepsy, language impairment, level of intellectual functioning, or known genetic syndrome. Prior to conducting study activities, written informed consent was obtained from the participant’s legal guardian and informed assent was elicited from children age 7 years and older, who were able.
Data/Sample Collection Measures
Parents/guardians completed a demographic and medical history form including birth history, medications, co-morbid medical symptoms, and family medical history. Parents/guardians also completed the Aberrant Behavior Checklist-Community (ABC-C), a 58-item behavioral functioning measure for children and adults with developmental disabilities that includes five subscales: irritability and agitation; lethargy and social withdrawal; stereotypic behavior; hyperactivity and non-compliance; and inappropriate speech (Aman et al., 1985). Scoring was completed using the method validated for children with ASD (Kaat et al., 2014).
Research staff reviewed medical records for each participant to identify results of the most recent cognitive testing (developmental or IQ test results). To allow for comparison amongst the various developmental and intellectual assessment measures documented across all participants, only non-verbal cognitive scores (Bayley cognitive score or non-verbal IQ) were analyzed. Study data were collected and managed using REDCap (Research Electronic Data Capture), a secure, web-based application for electronic data capture hosted at the lead site.
We estimated household income based on participant zip code as a proxy for SES. We assigned the median income reported in the 2012 U.S. Census for each participant’s zip code. Zip code regions were assigned to a quintile based on the national breakdown of income distribution (Geronimus and Bound, 1998; U.S. Department of Commerce, 2013).
As a part of each participant’s clinic visit, clinicians or their clinical staff obtained measurements of head circumference, height and weight. Clinicians then recorded results of a standardized dysmorphology examination (Miles et al., 2008; Sices et al., 2017). Photocopies of each participant’s hands were obtained by study staff following the clinic visit to provide 2D:4D ratios using established methodology (Manning et al., 1998). Photocopies that were not clear or did not demonstrate clear markings of the digits were omitted from analysis.
A 3 mL EDTA-anticoagulated whole blood sample was obtained from each participant for platelet count and serotonin analysis. Automated platelet count measurements were performed locally at each respective study site within 24 h of collection. Aliquots of whole blood were stored at −80°C until shipment on dry ice to the Anderson research laboratory at Yale University for platelet (whole blood) serotonin measurement, using previously published methodology (Anderson et al., 1987; Epperson et al., 2001). Overnight urine sample was collected for melatonin sulfate and creatinine analyses either from a first morning void, or an overnight diaper, thus representing nighttime production of melatonin for all samples. Urine samples were similarly stored at −80°C until shipment on dry ice to the Anderson laboratory. Melatonin sulfate-like immunoreactivity was measured by ELISA kit provided by IBL International (Toronto, ON, Canada) and creatinine levels were determined by HPLC using UV absorbance detection (240 nm) following 10-fold dilution (Hausen et al., 1981).
Statistical Analysis and Considerations
Wilcoxon two-sample tests were used throughout to test for differences between groups of participants with and without a given characteristic. Spearman correlations were computed to estimate correlation between two continuous variables. Income was analyzed as a continuous variable. Race was challenging to analyze due to the small number of participants endorsing some racial categories, the number of participants selecting more than one category and the number selecting only the ‘Other’ category, which was not further specified. Linear regression models were used to test for effects of race and median household income on the two primary biomarker outcomes (platelet serotonin and urinary melatonin sulfate). A p-value < 0.05 was used as a cut-off for statistical significance. All tests were two-sided. Due to the exploratory nature of the study, no adjustments were made for multiple comparisons (Rothman, 1990).
Results
A total of 88 participants were enrolled in the study; five were subsequently excluded from data analysis resulting in an analyzable sample of 83. Of the five excluded individuals, two did not provide at least one sample for biochemical measurement (blood for platelet serotonin or urine for melatonin sulfate excretion), two were discovered to be taking medications not disclosed at time of enrollment [fluoxetine (n = 1) and melatonin (n = 1)], and one voluntarily withdrew from the study.
Group Descriptive Statistics
Demographics
Participant demographics are summarized in Table 1. The mean age of participants was 7.4 ± 1.6 years and 82% were male. Participants identified race and ethnicity by checking as many categories as applied. Overall, 63% endorsed White only, 7% Black only, 5% Asian only, 13% endorsed more than one racial category and 14% endorsed an “Other” category that was not further specified. Nineteen percent endorsed Hispanic ethnicity. Mean average income (estimated from zip code of residence) was $57,700 (SD 19,800), with a range from $26,944 to $121,693 across all participants (Table 1).
Developmental Function
ABC-C score distributions for the study group were comparable with recently published norms for children with ASD and represented a range of behavioral function (Kaat et al., 2014). Cognitive test results were available for 65 participants (78%). Mean age at cognitive testing was 5.4 years (SD = 2.2 years). Mean time elapsed from date of cognitive testing to date of biomarker sample collection was 2.3 years (SD = 1.8 years). Reported non-verbal standard scores ranged from 43 to 121 (mean = 88, SD = 19) with 14% having a non-verbal cognitive score below 70. Genetic testing results were reported for 58 patients. Of those, 1 had a genetic syndrome and 7 had a variant of unknown significance (Table 1).
Biomarkers
Discussion of study activity completion rates and feasibility has been previously described (Sices et al., 2017). Mean values and standard deviations as well as completion/collection rates for each of the biomarkers are shown in Table 2.
Biochemical Markers
The serotonin and urinary melatonin sulfate excretion measures were non-normally distributed (Shapiro–Wilk tests, all p-values < 0.001). Mean levels for platelet serotonin in our participant group were elevated from previously published norms (McBride et al., 1998) consistent with the presence of hyperserotonemia. Urinary melatonin sulfate excretion rates in our ASD participant group, however, were not lower than prior reported rates in control populations (Tordjman et al., 2005) (Table 2).
Age was negatively correlated with melatonin sulfate excretion (r = −0.26, p = 0.048). We did not find an age association for serotonin. Contrary to previously published results, we did not identify a negative correlation between platelet serotonin and melatonin sulfate excretion levels (r = −0.008, p = 0.95). In addition, there were no correlations between platelet serotonin and melatonin sulfate excretion levels and sex, ethnicity, non-verbal cognitive level, or ABC-C subscale scores (Table 3).
Due to the limited numbers of participants endorsing certain race categories we were not able to conduct comparative analyses. We examined platelet serotonin level and melatonin excretion rate by household income. The bivariate correlation of platelet serotonin and median household income was positive but did not reach statistical significance (r = 0.20, p = 0.09). In a single linear regression model testing for the effects both of race and of median household income on platelet serotonin, however, race and income were each independently associated with platelet serotonin. Household income was significantly associated with higher platelet serotonin levels in this regression with mean platelet serotonin increasing an estimated 9.8 points per $10K increase in household income (p = 0.004).
Melatonin sulfate and median household income were positively correlated (r = 0.25, p = 0.03) in bivariate analysis. In the linear regression model, higher median household income was associated with higher melatonin sulfate excretion levels with an estimated increase of 13.8 ng/mg creatinine per $10K increase in household income (p = 0.04).
Physical Markers
2D:4D values were consistent with prior reports showing ratios for ASD participants below published norms (Manning et al., 2001). Head circumference values had a mean z-score 0.77 with range from −1.72 to 3.60. Twelve of the 72 participants (17%) with head circumference measurements were macrocephalic (z-score > 2SD). Dysmorphology examination was performed on 75 participants: 6 (8%) were identified as dysmorphic. Additionally, 42 of these participants (55%) had an abnormal finding in one or more body regions with the most frequently identified regions being the ear (20%), hair pattern (18%) and philtrum (16%). Dysmorphic status did not correlate with report of abnormal genetic test results. Only 3 of the 6 dysmorphic participants had cognitive testing results, scores ranged from 70 to 100 (Table 2).
Co-occuring Conditions
Participants had high rates of caregiver reported co-occuring medical and psychiatric conditions including: 52% with gastrointestinal (GI) conditions, 40% with sleep problems, 41% with psychiatric conditions (including 25% with ADHD and 5% with anxiety disorder), 31% with history of regression in developmental skills, and 20% with neurologic conditions (including 7% with seizures) (Table 4).
Correlations of Biochemical Measures With Participant Characteristics
Biochemical Measures, Physical Characteristics, and Behavioral Functioning
Platelet serotonin levels and melatonin sulfate excretion were not significantly correlated with head circumference z-score, 2D:4D ratio, or dysmorphology status. There were also no associations among the physical measures (head circumference, 2D:4D, and dysmorphology status). Patients with dysmorphic status scored significantly higher than other patients on two of the ABC subscales [stereotypic behaviors: 10.0 (SD: 4.0) vs. 5.1 (SD: 4.5), p = 0.019; inappropriate speech 7.0 (SD: 2.9) vs. 4.5 (SD: 3.5), p = 0.043].
Biochemical Markers and Co-occurring Conditions
We examined correlations between both urinary melatonin sulfate excretion and platelet serotonin with co-occurring conditions including GI and neurologic problems, seizures, and sleep conditions reported by caregivers (Table 5). Patients with neurologic conditions (n = 15) had lower melatonin sulfate excretion than patients without these conditions [respectively: mean (SD): 91.4 (42.5) vs. 145.7 (81.3), p = 0.02]. There was no difference in mean age of participants with and without neurologic conditions (p = 0.69). No association was found between melatonin sulfate excretion and reported sleep problems (Table 5). We identified a trend toward an association between higher platelet serotonin levels and reported GI conditions (medians: 778 vs. 702, p = 0.08) Three participants had platelet serotonin values above 2000 ng/billion platelets (one was > 2 SD and the other two were > 3 SD above the mean); all three participants endorsed GI symptoms.
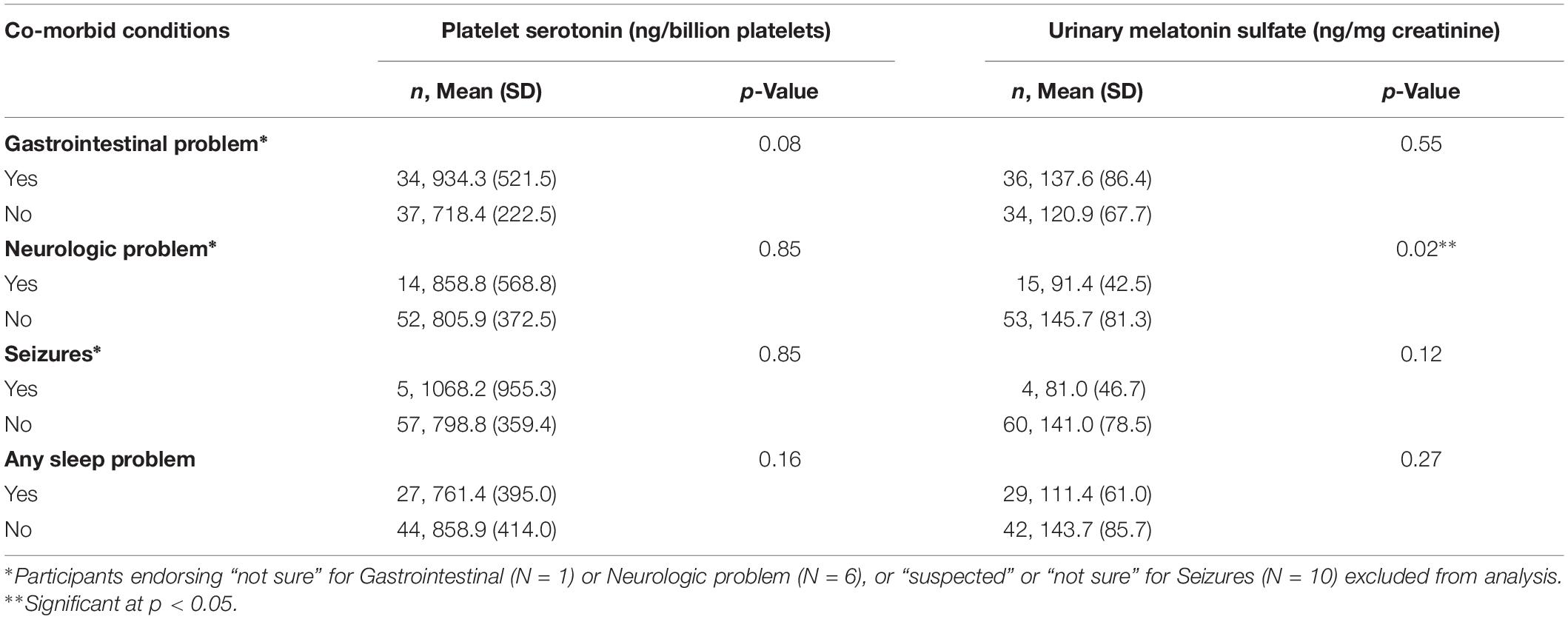
Table 5. Mean and standard deviation (SD) for biochemical markers in patients with co-morbid medical conditions, and comparisons between participants endorsing and not endorsing these conditions.
Discussion
In this pilot demonstration feasibility project, collection of multiple biomarkers during a regularly scheduled ASD specialty clinical visit allowed for the examination of associations between biochemical and clinical measures, and identified several findings that suggest direction for future studies. While our findings for individual biochemical and clinical biomarkers should not be viewed as definitive, we found associations between platelet serotonin and melatonin sulfate excretion with patient demographic and clinical characteristics that illustrate the potential of this approach to generate important information about multiple biomarkers and functional domains within a single heterogeneous clinical patient population.
Consistent with prior research, we identified elevated platelet serotonin levels in our sample, with means and ranges similar to previously published data for children with ASD (McBride et al., 1998; Mulder et al., 2004; Hammock et al., 2012). In contrast to previous studies, urinary melatonin sulfate excretion rates in our ASD participant group were not lower than prior reported rates in control populations (Tordjman et al., 2005). However, the limited available data on a similar age range and using a similar analytical methodology limit comparison of the melatonin sulfate results to prior reports. The overall racial distribution and the high proportion of participants endorsing more than one race precluded analysis of biomarker levels by race. Unexpectedly, however, we found that higher income, independent of race, was associated with higher platelet serotonin and higher melatonin sulfate excretion. The reasons for this are likely complex, as income estimated by zip code was used as a proxy for SES and may reflect multiple social and environmental factors including diet. Lower melatonin sulfate excretion was associated with increasing age during childhood in our sample as has been previously reported (Tordjman et al., 2005). In contrast, we did not find an age-based variation in platelet serotonin whereas prior studies found that serotonin levels correlate negatively with age in children with ASD (McBride et al., 1998; Hammock et al., 2012). This may be explained by the narrow age range of our study. A more recent study did not find an age-based difference in serotonin when comparing individuals with ASD who were below 16 years with those at or above 16 years (Pagan et al., 2014). Additionally, although a prior study showed a negative correlation between platelet serotonin and urinary melatonin (Mulder et al., 2010), the Pagan study did not find this correlation in a multi-aged (children and adults) sample of 230 patients with ASD (Pagan et al., 2014). Further studies with detailed sociodemographic variables will be needed to clarify these findings.
Frequencies of dysmorphic physical features in the study sample were similar to prior published findings for children with ASD. Dysmorphology examination identified six participants (8%) as dysmorphic using the scoring algorithm described by Miles et al. (2008). This compares to a rate of 12% reported as dysmorphic by Miles for a population of patients with autistic disorder. Of note, we did not preferentially select patients from settings that would typically have higher levels of dysmorphic features such as genetic specialty clinics. Although we did not have sufficient power to examine the relationship of dysmorphic status and cognitive functioning, dysmorphic status was correlated with higher ratings on the ABC stereotypy and inappropriate speech domains, suggesting higher symptom severity. This is consistent with prior studies documenting that patients with “syndromic autism” have more severe phenotypes (Miles et al., 2005; Schaefer and Mendelsohn, 2008).
Caregiver reported frequencies of comorbid medical and psychiatric conditions including developmental regression, sleep problems, ADHD, GI symptoms, and seizures were similar to those reported in other ASD cohorts (Richdale, 1999; Goldberg et al., 2003; Lord et al., 2004; Polimeni et al., 2005; Leyfer et al., 2006; Ibrahim et al., 2009; Buie et al., 2010; Murray, 2010; Aldinger et al., 2015). Study participants had lower levels of anxiety (5%) than previously reported (Filipek et al., 2000; Myers and Johnson, 2007; Volkmar et al., 2014), most likely explained by the study exclusion criteria that restricted participants on medications known to influence serotonin levels.
Serotonin [5-hydroxytryptamine (5-HT)] and melatonin are important in neurogenesis, synaptogenesis, mood, sleep, and GI function (Gabriele et al., 2014). Gastrointestinal symptoms were reported by 51% of participants, consistent with prior studies showing rates of GI symptoms ranging from 9 to 91% in children with ASD (Molloy and Manning-Courtney, 2003; Valicenti-McDermott et al., 2006; Ibrahim et al., 2009; Buie et al., 2010). A recent meta-analysis reported an odds ratio of 4.42 for GI problems in children with ASD (McElhanon et al., 2014). We did not identify significant associations between GI symptoms and platelet serotonin or melatonin sulfate excretion. However, we did observe a trend-level association between higher platelet serotonin and reported GI symptoms. In addition, three participants who were outliers with very high platelet serotonin (above 2000 ng/billion platelets) all reported GI symptoms. This is consistent with a recent study examining whole blood serotonin levels and co-occurring GI symptoms in 82 children age 6–15 years with ASD that identified a moderate positive correlation (r = 0.23, p = 0.048) between GI symptom score and serotonin levels in Caucasian participants (Marler et al., 2016). In addition, specific serotonin-related gene variants in individuals with ASD may contribute to gastrointestinal disturbance (Abdelrahman et al., 2014). Future studies examining serotonin levels in patients with ASD and co-occurring GI problems appear warranted.
Melatonin sulfate excretion was negatively associated with reported neurologic problems (p = 0.02), with much of the association due to lower levels in participants reporting diagnosed or suspected seizures. The rate of seizures (7%) was lower than that reported for other populations of children and adolescents with ASD. However, the age range of our study participants was between the two peak onset periods for seizures in ASD, early childhood and adolescence (Filipek et al., 2000; Myers and Johnson, 2007). An analysis of medical conditions in the AGRE and Simons Simplex Cohort (SSC) found similar rates of seizures (12.2 and 5.3% respectively) in two cohorts with mean age 9.2 and 9.0 years (Aldinger et al., 2015). Prior studies document lower melatonin secretion in individuals with refractory epilepsy compared to controls, and elevated melatonin levels following seizures; importantly, these studies did not examine associations with ASD (Bazil et al., 2000; Yalyn et al., 2006; Paprocka et al., 2010). Future studies examining urinary melatonin sulfate excretion levels in individuals with ASD and epilepsy would be of interest.
Variants in melatonin pathway genes have been associated with sleep onset delay (Veatch et al., 2015) and melatonin administration has been associated with improvements in sleep in children with ASD (Doyen et al., 2011; Guenole et al., 2011; Rossignol and Frye, 2011; Malow et al., 2012). Lower melatonin sulfate excretion levels were associated with reported insomnia in a population of 145 children and adults with ASD (Pagan et al., 2014). However, despite a high rate of reported sleep problems in our cohort (40%), there was no association found with melatonin sulfate excretion rates. This might be due to exclusion from study participation of individuals who were currently taking melatonin. Our rates of reported sleep problems were similar to those in unrestricted ASD populations (Sikora et al., 2012) but lower than those reported in the AGRE cohort (55.5%) and SSC (72.5%) (Aldinger et al., 2015).
Our study had several limitations. As this was a pilot demonstration study evaluating the feasibility of collecting data during specialty clinical visits, we did not have a control group. However, our primary intent was to examine whether and how individual biomarkers were inter-related within this clinical ASD population. Recruitment was solely from subspecialty tertiary care clinics. Our sample, however, was representative of the general population of individuals with ASD with regard to biomarker values and symptom severity. In addition, we view the direct integration of the research study within clinical visits as a strength that demonstrates the feasibility of the model.
The average income of our participants, as estimated by their home zip codes, is above the national average, and none of our participants were in the lowest national income quintile. However, the income ranges for participants are in line with the region surrounding all five clinical sites (U.S. Department of Commerce, 2013). We note that 11% of our participants endorsed more than one race. Given changing national demographics, it will become ever more important to consider how to best categorize and analyze data with respect to race and ethnicity.
We relied on expert clinician determination of ASD diagnosis, and parent report of behavioral function and comorbid medical and psychiatric symptoms. Cognitive data were historical, utilized a variety of measures, and were not concurrent with biomarker collection. In addition, availability of cognitive evaluation data, age at evaluation, and timing relative to this study varied across subjects (Sices et al., 2017).
Diet can affect both platelet serotonin and melatonin levels; however, dietary histories were not obtained. We also did not evaluate complete Tanner Staging or blood work to assess pubertal stage. Exclusion of individuals taking medications/supplements that could influence serotonin or melatonin levels likely reduced participation from some individuals with disruptive behavior, anxiety, and sleep problems.
The sample size, in combination with missing data for some variables, limited our power to identify smaller correlations. In the future, the use of clinical registries across multiple sites would facilitate systematic and consistent collection of data and tracking of outcomes for larger samples. Due to the exploratory nature of our study we did not correct for multiple comparisons, which increased the risk of Type I errors. Power was also impacted by the heterogeneity of the population sample. Future studies may need to look at subgroups of patients with particular symptom presentations or enriched samples to improve the ability to identify relationships.
This study examined potential correlations among biomarkers gathered during or in association with ASD specialty clinic visits. We found interesting potential correlations between biochemical markers, SES, and neurologic and GI symptoms that may be further explored in future studies. This translational research model has promise for defining subgroups of patients based on biomarker profiles and can help guide future studies on the use of biomarkers for individualized prognosis and treatment planning in individuals with complex, heterogeneous neurodevelopmental disorders such as ASD.
Members of the Autism Consortium Biomarkers Study Clinicians Group
Boston University Medical Center: Marilyn Augustyn, Stephanie Blenner, Lynn Hironaka, Jennifer Radesky, Arathi Reddy, Jayna Schumacher, Laura Sices, Robert Keder Boston Children’s Hospital: Carolyn Bridgemohan, Elizabeth Harstad, April Levin, Leonard Rappaport, Alison Schonwald, Sarah Spence, Laura Weissman Center for Children With Special Needs, Floating Children’s Hospital at Tufts Medical Center: A. Stacie Colwell, Carmina Erdei, Eric Goepfert, Karen J. Miller, Christina Sakai, Monica Ultmann, L. Erik Von Hahn Lurie Center for Autism, Massachusetts General Hospital for Children: Jessica Helt, Yamini J. Howe, Ann M. Neumeyer, Christine Stine University of Massachusetts Memorial Medical Center: Roula Choueiri, David M. Cochran, Jean A. Frazier, Andrew W. Zimmerman.
Data Availability
The datasets generated and/or analyzed during the current study are not publicly available due to inclusion of protected health information but are available from the corresponding author on reasonable request.
Ethics Statement
This study was approved by the Institutional Review Board at the lead site (Boston Children’s Hospital): Assurance Identification No. FWA00002071 IRB Registration No. IRB00000352.
Author Contributions
CB conceived of the study, participated in the design and coordination, analysis and interpretation of results and drafted the manuscript. DC, YH, LS, AZ, RC, SB, JF, and AN participated in the study design, coordination, analysis and interpretation of results and helped draft the manuscript. KM, MU, KP, LF, and JH participated in the coordination, analysis and interpretation of results and helped draft the manuscript. PF completed the statistical analysis, interpreted the results and helped draft the manuscript. GA participated in the design of the study, performance of biochemical measurements, interpretation of results and helped draft the manuscript. All authors read and approved the final manuscript.
Conflict of Interest Statement
MA, RC, JF, AN, and KM were members of the Autism Consortium Steering Committee. The Autism Consortium was responsible for distributing the initial SFARI grant to the participating sites. SS receives other research funding from Simons Foundation Autism Research Initiative. SS received honoraria and travel support from Simons Foundation Autism Research Initiative in the past. GA has served as a consultant to Eli Lilly and Company and to Novartis Pharmaceuticals. JF has received research support from Fulcrum Therapeutics, Janssen Research and Development, and Roche as well as NICHD, NINDS, and NIMH. AZ has received fees for expert legal testimony and research support from the U.S. Department of Defense.
The remaining authors declare that the research was conducted in the absence of any commercial or financial relationships that could be construed as a potential conflict of interest.
Funding
This work was supported by grants from the Simons Foundation Autism Research Initiative (SFARI) award # 290933 to The Autism Consortium and award # 412328 to CB.
This work was conducted with support from The Harvard Catalyst Clinical and Translational Science Center (National Center for Research Resources and the National Center for Advancing Translational Sciences, National Institutes of Health Award UL1 TR001102) (NCATS grant #8UL1TR000170) and financial contributions from Harvard University and its affiliated academic healthcare centers.
Acknowledgments
Simons Foundation Autism Research Initiative (SFARI); The Autism Consortium, Dierdre Phillips Executive Director; Leonard Rappaport, Sarah Spence, Marilyn Augustyn, and Mustafa Sahin for their contributions to the concept and development of the study, and for their subsequent guidance and support including critical review of the manuscript draft; Walter Kaufmann for his contribution to the concept and development of the project; William Barbaresi and the Boston Children’s Hospital DMC Writer’s Group for critical review of manuscript drafts; the Boston Children’s Hospital Translational Neuroscience Center; and all of the families who participated in this project.
Supplementary Material
The Supplementary Material for this article can be found online at: https://www.frontiersin.org/articles/10.3389/fnint.2019.00031/full#supplementary-material
References
Abdelrahman, H. M., Sherief, L. M., Alghobashy, A. A., Abdel Salam, S. M., Hashim, H. M., Abdel Fattah, N. R., et al. (2014). Association of 5-HT2A receptor gene polymorphisms with gastrointestinal disorders in Egyptian children with autistic disorder. Res. Dev. Disabil. 36C, 485–490. doi: 10.1016/j.ridd.2014.10.023
Abdulamir, H. A., Abdul-Rasheed, O. F., and Abdulghani, E. A. (2018). Serotonin and serotonin transporter levels in autistic children. Saudi Med. J. 39, 487–494. doi: 10.15537/smj.2018.5.21751
Aldinger, K. A., Lane, C. J., Veenstra-VanderWeele, J., and Levitt, P. (2015). Patterns of risk for multiple co-occurring medical conditions replicate across distinct cohorts of children with autism spectrum disorder. Autism Res. 8, 771–781. doi: 10.1002/aur.1492
Aman, M. G., Singh, N. N., Stewart, A. W., and Field, C. J. (1985). The aberrant behavior checklist: a behavior rating scale for the assessment of treatment effects. Am. J. Ment. Defic. 89, 485–491.
American Psychiatric Association, and DSM-5 Task Force (2013). Diagnostic and Statistical Manual of Mental Disorders: DSM-5. Washington, DC: American Psychiatric Association.
Anderson, G. M., Feibel, F. C., and Cohen, D. J. (1987). Determination of serotonin in whole blood, platelet-rich plasma, platelet-poor plasma and plasma ultrafiltrate. Life Sci. 40, 1063–1070. doi: 10.1016/0024-3205(87)90568-6
Bazil, C. W., Short, D., Crispin, D., and Zheng, W. (2000). Patients with intractable epilepsy have low melatonin, which increases following seizures. Neurology 55, 1746–1748. doi: 10.1212/wnl.55.11.1746
Buie, T., Fuchs, G. J. III, Furuta, G. T., Kooros, K., Levy, J., Lewis, J. D., et al. (2010). Recommendations for evaluation and treatment of common gastrointestinal problems in children with ASDs. Pediatrics 125(Suppl.), S19–S29. doi: 10.1542/peds.2009-1878D
Cook, E. H., and Leventhal, B. L. (1996). The serotonin system in autism. Curr. Opin. Pediatr. 8, 348–354. doi: 10.1097/00008480-199608000-00008
Courchesne, E., Campbell, K., and Solso, S. (2011). Brain growth across the life span in autism: age-specific changes in anatomical pathology. Brain Res. 1380, 138–145. doi: 10.1016/j.brainres.2010.09.101
Courchesne, E., Muller, R. A., and Saitoh, O. (1999). Brain weight in autism: normal in the majority of cases, megalencephalic in rare cases. Neurology 52, 1057–1059.
De Los Reyes, A., and Aldao, A. (2015). Introduction to the special issue: toward implementing physiological measures in clinical child and adolescent assessments. J. Clin. Child Adolesc. Psychol. 44, 221–237. doi: 10.1080/15374416.2014.891227
Doyen, C., Mighiu, D., Kaye, K., Colineaux, C., Beaumanoir, C., Mouraeff, Y., et al. (2011). Melatonin in children with autistic spectrum disorders: recent and practical data. Eur. Child Adolesc. Psychiatry 20, 231–239. doi: 10.1007/s00787-011-0162-168
Duffy, J. F., Cain, S. W., Chang, A.-M., Phillips, A. J. K., Munch, M. Y., Gronfier, C., et al. (2011). Sex difference in the near-24-hour intrinsic period of the human circadian timing system. Proc. Natl. Acad. Sci. U.S.A. 108(Suppl.), 15602–15608. doi: 10.1073/pnas.1010666108
Epperson, N., Czarkowski, K. A., Ward-O’Brien, D., Weiss, E., Gueorguieva, R., Jatlow, P., et al. (2001). Maternal sertraline treatment and serotonin transport in breast-feeding mother-infant pairs. Am. J. Psychiatry 158, 1631–1637. doi: 10.1176/appi.ajp.158.10.1631
Estes, A., Munson, J., Rogers, S. J., Greenson, J., Winter, J., and Dawson, G. (2015). Long-term outcomes of early intervention in 6-year-old children with autism spectrum disorder. J. Am. Acad. Child Adolesc. Psychiatry 54, 580–587. doi: 10.1016/j.jaac.2015.04.005
Filipek, P. A., Accardo, P. J., Ashwal, S., Baranek, G. T., Cook, E. H. J., Dawson, G., et al. (2000). Practice parameter: screening and diagnosis of autism: report of the quality standards subcommittee of the american academy of neurology and the child neurology society. Neurology 55, 468–479.
Frustaci, A., Neri, M., Cesario, A., Adams, J. B., Domenici, E., Dalla Bernardina, B., et al. (2012). Oxidative stress-related biomarkers in autism: systematic review and meta-analyses. Free Radic. Biol. Med. 52, 2128–2141. doi: 10.1016/j.freeradbiomed.2012.03.011
Gabriele, S., Sacco, R., and Persico, A. M. (2014). Blood serotonin levels in autism spectrum disorder: a systematic review and meta-analysis. Eur. Neuropsychopharmacol. 24, 919–929. doi: 10.1016/j.euroneuro.2014.02.004
Geronimus, A. T., and Bound, J. (1998). Use of census-based aggregate variables to proxy for socioeconomic group: evidence from national samples. Am. J. Epidemiol. 148, 475–486. doi: 10.1093/oxfordjournals.aje.a009673
Goldberg, W. A., Osann, K., Filipek, P. A., Laulhere, T., Jarvis, K., Modahl, C., et al. (2003). Language and other regression: assessment and timing. J. Autism Dev. Disord. 33, 607–616. doi: 10.1023/b:jadd.0000005998.47370.ef
Guenole, F., Godbout, R., Nicolas, A., Franco, P., Claustrat, B., and Baleyte, J.-M. (2011). Melatonin for disordered sleep in individuals with autism spectrum disorders: systematic review and discussion. Sleep Med. Rev. 15, 379–387. doi: 10.1016/j.smrv.2011.02.001
Hammock, E., Veenstra-VanderWeele, J., Yan, Z., Kerr, T. M., Morris, M., Anderson, G. M., et al. (2012). Examining autism spectrum disorders by biomarkers: example from the oxytocin and serotonin systems. J. Am. Acad. Child Adolesc. Psychiatry 51, 712–721.e1. doi: 10.1016/j.jaac.2012.04.010
Hausen, A., Fuchs, D., and Wachter, H. (1981). Determination of creatinine in urine by high performance liquid chromatography (HPLC) (author’s transl). J. Clin. Chem. Clin. Biochem. 19, 375–378.
Honekopp, J. (2012). Digit ratio 2D:4D in relation to autism spectrum disorders, empathizing, and systemizing: a quantitative review. Autism Res. 5, 221–230. doi: 10.1002/aur.1230
Hranilovic, D., Bujas-Petkovic, Z., Vragovic, R., Vuk, T., Hock, K., and Jernej, B. (2007). Hyperserotonemia in adults with autistic disorder. J. Autism Dev. Disord. 37, 1934–1940. doi: 10.1007/s10803-006-0324-326
Ibrahim, S. H., Voigt, R. G., Katusic, S. K., Weaver, A. L., and Barbaresi, W. J. (2009). Incidence of gastrointestinal symptoms in children with autism: a population-based study. Pediatrics 124, 680–686. doi: 10.1542/peds.2008-2933
Insel, T. R. (2014). The NIMH research domain criteria (RDoC) project: precision medicine for psychiatry. Am. J. Psychiatry 171, 395–397. doi: 10.1176/appi.ajp.2014.14020138
Interagency Autism CoordinatingCommittee [IACC] (2014). IACC Strategic Plan for Autism Spectrum Disorder Research – 2013 Update. doi: 10.1176/appi.ajp.2014.14020138
Kaat, A. J., Lecavalier, L., and Aman, M. G. (2014). Validity of the aberrant behavior checklist in children with autism spectrum disorder. J. Autism Dev. Disord. 44, 1103–1116. doi: 10.1007/s10803-013-1970-1970
Kohane, I. S. (2015). An autism case history to review the systematic analysis of large-scale data to refine the diagnosis and treatment of neuropsychiatric disorders. Biol. Psychiatry 77, 59–65. doi: 10.1016/j.biopsych.2014.05.024
Kolevzon, A., Newcorn, J. H., Kryzak, L., Chaplin, W., Watner, D., Hollander, E., et al. (2010). Relationship between whole blood serotonin and repetitive behaviors in autism. Psychiatry Res. 175, 274–276. doi: 10.1016/j.psychres.2009.02.008
Kuperman, S., Beeghly, J., Burns, T., and Tsai, L. (1987). Association of serotonin concentration to behavior and IQ in autistic children. J. Autism Dev. Disord. 17, 133–140. doi: 10.1007/bf01487265
Leyfer, O. T., Folstein, S. E., Bacalman, S., Davis, N. O., Dinh, E., Morgan, J., et al. (2006). Comorbid psychiatric disorders in children with autism: interview development and rates of disorders. J. Autism Dev. Disord. 36, 849–861. doi: 10.1007/s10803-006-0123-120
Lord, A. C., and Mcgee, J. P. (2001). “National academy of sciences committee on educational interventions for children with autism,” in Educating Children with Autism, eds J. P. McGee and C. Lord (Washington, DC: National Academy Press), 211–229.
Lord, C., Shulman, C., and DiLavore, P. (2004). Regression and word loss in autistic spectrum disorders. J. Child Psychol. Psychiatry 45, 936–955. doi: 10.1111/j.1469-7610.2004.t01-1-00287.x
Mackus, M., de Kruijff, D., Otten, L. S., Kraneveld, A. D., Garssen, J., and Verster, J. C. (2017). The 2D: 4D digit ratio as a biomarker for autism spectrum disorder. Autism Res. Treat. 2017:1048302. doi: 10.1155/2017/1048302
Malow, B., Adkins, K. W., McGrew, S. G., Wang, L., Goldman, S. E., Fawkes, D., et al. (2012). Melatonin for sleep in children with autism: a controlled trial examining dose, tolerability, and outcomes. J. Autism Dev. Disord. 42, 1729–1737. doi: 10.1007/s10803-011-1418-1413
Manning, J. T., Baron-Cohen, S., Wheelwright, S., and Sanders, G. (2001). The 2nd to 4th digit ratio and autism. Dev. Med. Child Neurol. 43, 160–164. doi: 10.1111/j.1469-8749.2001.tb00181.x
Manning, J. T., Scutt, D., Wilson, J., and Lewis-Jones, D. I. (1998). The ratio of 2nd to 4th digit length: a predictor of sperm numbers and concentrations of testosterone, luteinizing hormone and oestrogen. Hum. Reprod. 13, 3000–3004. doi: 10.1093/humrep/13.11.3000
Marler, S., Ferguson, B. J., Lee, E. B., Peters, B., Williams, K. C., McDonnell, E., et al. (2016). Brief report: whole blood serotonin levels and gastrointestinal symptoms in autism spectrum disorder. J. Autism Dev. Disord. 46, 1124–1130. doi: 10.1007/s10803-015-2646-2648
McBride, P. A., Anderson, G. M., Hertzig, M. E., Snow, M. E., Thompson, S. M., Khait, V. D., et al. (1998). Effects of diagnosis, race, and puberty on platelet serotonin levels in autism and mental retardation. J. Am. Acad. Child Adolesc. Psychiatry 37, 767–776. doi: 10.1097/00004583-199807000-199807017
McElhanon, B. O., McCracken, C., Karpen, S., and Sharp, W. G. (2014). Gastrointestinal symptoms in autism spectrum disorder: a meta-analysis. Pediatrics 133, 872–883. doi: 10.1542/peds.2013-3995
Miles, J. H., Takahashi, T. N., Bagby, S., Sahota, P. K., Vaslow, D. F., Wang, C. H., et al. (2005). Essential versus complex autism: definition of fundamental prognostic subtypes. Am. J. Med. Genet. A 135, 171–180. doi: 10.1002/ajmg.a.30590
Miles, J. H., Takahashi, T. N., Hong, J., Munden, N., Flournoy, N., Braddock, S. R., et al. (2008). Development and validation of a measure of dysmorphology: useful for autism subgroup classification. Am. J. Med. Genet. A 146A, 1101–1116. doi: 10.1002/ajmg.a.32244
Miller, D. T., Adam, M. P., Aradhya, S., Biesecker, L. G., Brothman, A. R., Carter, N. P., et al. (2010). Consensus statement: chromosomal microarray is a first-tier clinical diagnostic test for individuals with developmental disabilities or congenital anomalies. Am. J. Hum. Genet. 86, 749–764. doi: 10.1016/j.ajhg.2010.04.006
Molloy, C. A., and Manning-Courtney, P. (2003). Prevalence of chronic gastrointestinal symptoms in children with autism and autistic spectrum disorders. Autism 7, 165–171. doi: 10.1177/1362361303007002004
Mulder, E. J., Anderson, G. M., Kema, I. P., de Bildt, A., van Lang, N. D. J., den Boer, J. A., et al. (2004). Platelet serotonin levels in pervasive developmental disorders and mental retardation: diagnostic group differences, within-group distribution, and behavioral correlates. J. Am. Acad. Child Adolesc. Psychiatry 43, 491–499. doi: 10.1097/00004583-200404000-200404016
Mulder, E. J., Anderson, G. M., Kemperman, R. F. J., Oosterloo-Duinkerken, A., Minderaa, R. B., and Kema, I. P. (2010). Urinary excretion of 5-hydroxyindoleacetic acid, serotonin and 6-sulphatoxymelatonin in normoserotonemic and hyperserotonemic autistic individuals. Neuropsychobiology 61, 27–32. doi: 10.1159/000258640
Murray, M. J. (2010). Attention-deficit/hyperactivity disorder in the context of autism spectrum disorders. Curr. Psychiatry Rep. 12, 382–388. doi: 10.1007/s11920-010-0145-143
Myers, S. M., and Johnson, C. P. (2007). Management of children with autism spectrum disorders. Pediatrics 120, 1162–1182. doi: 10.1542/peds.2007-2362
National Research Council (2011). Toward Precision Medicine: Building a Knowledge Network for Biomedical Research and a New Taxonomy of Disease. Washington, DC: The National Academies Press, doi: 10.17226/13284
Pagan, C., Delorme, R., Callebert, J., Goubran-Botros, H., Amsellem, F., Drouot, X., et al. (2014). The serotonin-N-acetylserotonin-melatonin pathway as a biomarker for autism spectrum disorders. Transl. Psychiatry 4:e479. doi: 10.1038/tp.2014.120
Paprocka, J., Dec, R., Jamroz, E., and Marszal, E. (2010). Melatonin and childhood refractory epilepsy–a pilot study. Med. Sci. Monit. 16, CR389–CR396.
Paynter, J., Sulek, R., Luskin-Saxby, S., Trembath, D., and Keen, D. (2018). Allied health professionals’ knowledge and use of ASD intervention practices. J. Autism Dev. Disord. 48, 2335–2349. doi: 10.1007/s10803-018-3505-3501
Polimeni, M. A., Richdale, A. L., and Francis, A. J. P. (2005). A survey of sleep problems in autism, Asperger’s disorder and typically developing children. J. Intellect. Disabil. Res. 49, 260–268. doi: 10.1111/j.1365-2788.2005.00642.x
Reichow, B., Hume, K., Barton, E. E., and Boyd, B. A. (2018). Early intensive behavioral intervention (EIBI) for young children with autism spectrum disorders (ASD). Cochrane Database Syst. Rev. 5:CD009260. doi: 10.1002/14651858.CD009260.pub3
Richdale, A. L. (1999). Sleep problems in autism: prevalence, cause, and intervention. Dev. Med. Child Neurol. 41, 60–66. doi: 10.1111/j.1469-8749.1999.tb00012.x
Rossignol, D. A., and Frye, R. E. (2011). Melatonin in autism spectrum disorders: a systematic review and meta-analysis. Dev. Med. Child Neurol. 53, 783–792. doi: 10.1111/j.1469-8749.2011.03980.x
Rothman, K. J. (1990). No adjustments are needed for multiple comparisons. Epidemiology 1, 43–46. doi: 10.1097/00001648-199001000-00010
Ruggeri, B., Sarkans, U., Schumann, G., and Persico, A. M. (2014). Biomarkers in autism spectrum disorder: the old and the new. Psychopharmacology 231, 1201–1216. doi: 10.1007/s00213-013-3290-3297
Sacco, R., Curatolo, P., Manzi, B., Militerni, R., Bravaccio, C., Frolli, A., et al. (2010). Principal pathogenetic components and biological endophenotypes in autism spectrum disorders. Autism Res. 3, 237–252. doi: 10.1002/aur.151
Sacco, R., Militerni, R., Frolli, A., Bravaccio, C., Gritti, A., Elia, M., et al. (2007). Clinical, morphological, and biochemical correlates of head circumference in autism. Biol. Psychiatry 62, 1038–1047. doi: 10.1016/j.biopsych.2007.04.039
Schaefer, G. B., and Mendelsohn, N. J. (2008). Clinical genetics evaluation in identifying the etiology of autism spectrum disorders. Genet. Med. 10, 301–305. doi: 10.1097/GIM.0b013e31816b5cc9
Schain, R. J., and Freedman, D. X. (1961). Studies on 5-hydroxyindole metabolism in autistic and other mentally retarded children. J. Pediatr. 58, 315–320. doi: 10.1016/s0022-3476(61)80261-8
Schendel, D. E., Diguiseppi, C., Croen, L. A., Fallin, M. D., Reed, P. L., Schieve, L. A., et al. (2012). The study to explore early development (SEED): a multisite epidemiologic study of autism by the centers for autism and developmental disabilities research and epidemiology (CADDRE) network. J. Autism Dev. Disord. 42, 2121–2140. doi: 10.1007/s10803-012-1461-1468
Sices, L., Pawlowski, K., Farfel, L., Phillips, D., Howe, Y., Cochran, D. M., et al. (2017). Feasibility of conducting autism biomarker research in the clinical setting. J. Dev. Behav. Pediatr. 38, 483–492. doi: 10.1097/DBP.0000000000000470
Sikora, D. M., Johnson, K., Clemons, T., and Katz, T. (2012). The relationship between sleep problems and daytime behavior in children of different ages with autism spectrum disorders. Pediatrics 130(Suppl.), S83–S90. doi: 10.1542/peds.2012-0900F
Teatero, M. L., and Netley, C. (2013). A critical review of the research on the extreme male brain theory and digit ratio (2D:4D). J. Autism Dev. Disord. 43, 2664–2676. doi: 10.1007/s10803-013-1819-1816
Tordjman, S., Anderson, G. M., Bellissant, E., Botbol, M., Charbuy, H., Camus, F., et al. (2012). Day and nighttime excretion of 6-sulphatoxymelatonin in adolescents and young adults with autistic disorder. Psychoneuroendocrinology 37, 1990–1997. doi: 10.1016/j.psyneuen.2012.04.013
Tordjman, S., Anderson, G. M., Pichard, N., Charbuy, H., and Touitou, Y. (2005). Nocturnal excretion of 6-sulphatoxymelatonin in children and adolescents with autistic disorder. Biol. Psychiatry 57, 134–138. doi: 10.1016/j.biopsych.2004.11.003
Tordjman, S., Najjar, I., Bellissant, E., Anderson, G. M., Barburoth, M., Cohen, D., et al. (2013). Advances in the research of melatonin in autism spectrum disorders: literature review and new perspectives. Int. J. Mol. Sci. 14, 20508–20542. doi: 10.3390/ijms141020508
U.S. Department of Commerce (2013). US Census Bureau Income Current Population Survey. Available at: http://www.census.gov/hhes/www/cpstables/032013/hhinc/toc.htm (accessed March 31, 2016)
Valicenti-McDermott, M., McVicar, K., Rapin, I., Wershil, B. K., Cohen, H., and Shinnar, S. (2006). Frequency of gastrointestinal symptoms in children with autistic spectrum disorders and association with family history of autoimmune disease. J. Dev. Behav. Pediatr. 27, S128–S136.
Varcin, K. J., and Nelson, C. A. III (2016). A developmental neuroscience approach to the search for biomarkers in autism spectrum disorder. Curr. Opin. Neurol. 29, 123–129. doi: 10.1097/WCO.0000000000000298
Veatch, O. J., Pendergast, J. S., Allen, M. J., Leu, R. M., Johnson, C. H., Elsea, S. H., et al. (2015). Genetic variation in melatonin pathway enzymes in children with autism spectrum disorder and comorbid sleep onset delay. J. Autism Dev. Disord. 45, 100–110. doi: 10.1007/s10803-014-2197-2194
Veenstra-VanderWeele, J., and Blakely, R. D. (2012). Networking in autism: leveraging genetic, biomarker and model system findings in the search for new treatments. Neuropsychopharmacology 37, 196–212. doi: 10.1038/npp.2011.185
Volkmar, F., Siegel, M., Woodbury-Smith, M., King, B., McCracken, J., and State, M. (2014). Practice parameter for the assessment and treatment of children and adolescents with autism spectrum disorder. J. Am. Acad. Child Adolesc. Psychiatry 53, 237–257. doi: 10.1016/j.jaac.2013.10.013
Wang, L., Angley, M. T., Gerber, J. P., and Sorich, M. J. (2011). A review of candidate urinary biomarkers for autism spectrum disorder. Biomarkers 16, 537–552. doi: 10.3109/1354750X.2011.598564
Keywords: autism, ASD, biomarkers, serotonin, melatonin, dysmorphology, clinical research
Citation: Bridgemohan C, Cochran DM, Howe YJ, Pawlowski K, Zimmerman AW, Anderson GM, Choueiri R, Sices L, Miller KJ, Ultmann M, Helt J, Forbes PW, Farfel L, Brewster SJ, Frazier JA and Neumeyer AM on behalf of the Autism Consortium Biomarkers Study Clinicians (2019) Investigating Potential Biomarkers in Autism Spectrum Disorder. Front. Integr. Neurosci. 13:31. doi: 10.3389/fnint.2019.00031
Received: 19 March 2019; Accepted: 03 July 2019;
Published: 02 August 2019.
Edited by:
John A. Sweeney, University of Cincinnati, United StatesReviewed by:
Felix Scholkmann, University Hospital Zürich, SwitzerlandZheng Wang, University of Chinese Academy of Sciences, China
Copyright © 2019 Bridgemohan, Cochran, Howe, Pawlowski, Zimmerman, Anderson, Choueiri, Sices, Miller, Ultmann, Helt, Forbes, Farfel, Brewster, Frazier and Neumeyer on behalf of the Autism Consortium Biomarkers Study Clinicians. This is an open-access article distributed under the terms of the Creative Commons Attribution License (CC BY). The use, distribution or reproduction in other forums is permitted, provided the original author(s) and the copyright owner(s) are credited and that the original publication in this journal is cited, in accordance with accepted academic practice. No use, distribution or reproduction is permitted which does not comply with these terms.
*Correspondence: Carolyn Bridgemohan, Carolyn.bridgemohan@childrens.harvard.edu
†These authors have contributed equally to this work