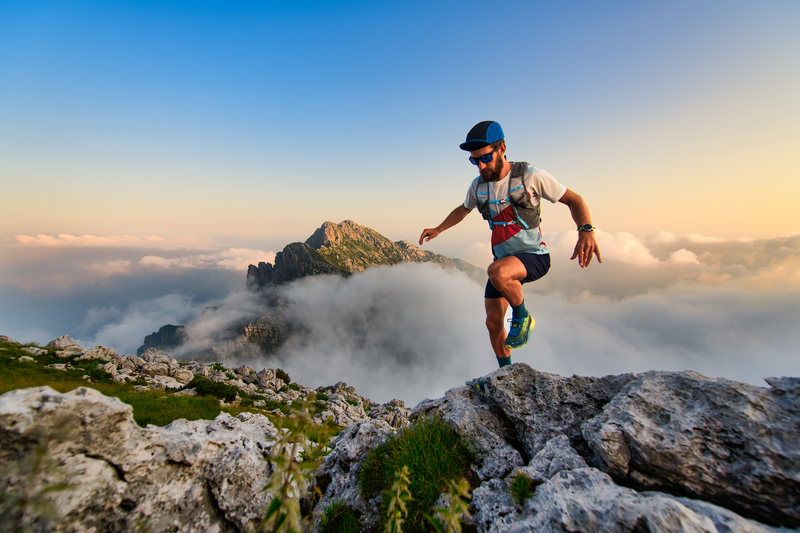
94% of researchers rate our articles as excellent or good
Learn more about the work of our research integrity team to safeguard the quality of each article we publish.
Find out more
HYPOTHESIS AND THEORY article
Front. Integr. Neurosci. , 13 February 2018
Volume 12 - 2018 | https://doi.org/10.3389/fnint.2018.00006
This article is part of the Research Topic 10 Years of Impactful, Open Neuroscience View all 47 articles
Five years ago, we introduced the thrift hypothesis of dopamine (DA), suggesting that the primary role of DA in adaptive behavior is regulating behavioral energy expenditure to match the prevailing economic conditions of the environment. Here we elaborate that hypothesis with several new ideas. First, we introduce the concept of affordability, suggesting that costs must necessarily be evaluated with respect to the availability of resources to the organism, which computes a value not only for the potential reward opportunity, but also the value of resources expended. Placing both costs and benefits within the context of the larger economy in which the animal is functioning requires consideration of the different timescales against which to compute resource availability, or average reward rate. Appropriate windows of computation for tracking resources requires corresponding neural substrates that operate on these different timescales. In discussing temporal patterns of DA signaling, we focus on a neglected form of DA plasticity and adaptation, changes in the physical substrate of the DA system itself, such as up- and down-regulation of receptors or release probability. We argue that changes in the DA substrate itself fundamentally alter its computational function, which we propose mediates adaptations to longer temporal horizons and economic conditions. In developing our hypothesis, we focus on DA D2 receptors (D2R), arguing that D2R implements a form of “cost control” in response to the environmental economy, serving as the “brain’s comptroller”. We propose that the balance between the direct and indirect pathway, regulated by relative expression of D1 and D2 DA receptors, implements affordability. Finally, as we review data, we discuss limitations in current approaches that impede fully investigating the proposed hypothesis and highlight alternative, more semi-naturalistic strategies more conducive to neuroeconomic investigations on the role of DA in adaptive behavior.
Though studied for over half a century, the dopamine (DA) system continues to pose unanswered questions and inspire controversy. Newer methods have yielded advances in our understanding but at the same time opened up new questions. For many years, debate focused on whether DA modulated appetitive behavior through effects on motivation or learning (Wise, 2004; Salamone et al., 2005; Berridge, 2007), both anchored in the history of psychological theory. Recent years have seen growing interest in a neuroeconomic perspective on DA (Glimcher et al., 2005; Phillips et al., 2007; Kable and Glimcher, 2009; Sharp et al., 2012; Schultz et al., 2015). Traditional approaches draw upon psychological and physiological concepts about motivation and regulation, such as drive, reinforcement, and homeostasis as underlying determinants of behavior—asking how DA mediates these mechanisms. In keeping with Marr’s levels of analysis (Marr, 1982), a neuroeconomic perspective begins by defining the computational problem; broadly, adaptation and survival poses an essentially economic question: how to optimally deploy organismal resources to obtain maximal benefit in a given environment, yielding the greatest probability of survival (Glimcher, 2003). Within neuroeconomics, DA has figured prominently as a key neural substrate for tracking the value of stimuli and actions and modulating decision-making accordingly.
This shift in conceptual framework is illustrated in a recent study by Berke and colleagues (Hamid et al., 2016) where using both microdialysis and fast-scan cyclic voltammetry, they carefully measured DA signaling in rats during a probabilistic selection task. Their results effectively integrate previous competing views on DA. With regards to the debate on whether DA acts primarily by: (i) enhancing motivation and energizing behavior towards appetitive goals (Robbins and Everitt, 1992; Berridge et al., 2009; Salamone and Correa, 2012); or (ii) providing a teaching signal, modifying behavior through reinforcement learning (Schultz et al., 1997; Montague et al., 2004; Wise, 2006), they observe DA signals consistent with both. That is, increases in DA preceded increased motivated behavior, but prior DA activity affected subsequent behavior as well. What is crucial is the way in which they formulate the integration of these previously competing conceptualizations of DA function: DA signals the value of work. This three word integration represents a neuroeconomic conceptualization where the integration of various aspects of DA subserve a crucial organismal computational (and economic) challenge: deciding how to allocate resources to maximize return.
DA, often referred to as the “reward neurotransmitter”, is widely associated with regulating appetitive motivation. However, DA also regulates behavioral activation in a more generalized way, increasing or decreasing behavioral energy expenditure—activity—independent of pursuit of appetitive goals (for review, Beeler et al., 2012c). Salamone et al. (2007) have long argued that DA regulates an animal’s willingness to work, effectively energizing behavior to allow animals to overcome costs associated with pursuing goals. Following a set of studies conducted in homecage operant boxes with hyperdopaminergic mice (Beeler et al., 2010, 2012b,d), in which the results did not fit neatly into any reward-oriented theory of DA, and building on Salamone’s work, we proposed the thrift hypothesis of DA (Beeler, 2012; Beeler et al., 2012c). In brief, we argued that the primary function of DA was to regulate behavioral energy expenditure, which was accomplished along two dimensions or axes: (1) regulating a generalized willingness to expend energy along a continuum from energy conservation/storage to liberal energy expenditure/utilization; and (2) an additional dimension in which DA regulates how “careful” an organism is in its energy expenditure, which we conceptualized as regulating how tightly behavioral choice is coupled to prior learning about value (Beeler et al., 2010; Kayser et al., 2015), commonly known as the explore-exploit balance (Sutton and Barto, 1998; Daw et al., 2006). This latter captures the “reward” aspect of DA by determining the extent to which energy would be directed toward previously rewarding activities. Our premise was that DA adapted to a rich or poor environmental economy by either up- or down-regulating, respectively, which in turn favored energy expenditure and exploration (maximal utilization of resources and opportunities) or energy conservation and maximal exploitation of prior learning (effectively also maximal utilization of scarce resources).
In this review and hypothesis article, we elaborate the rudimentary thrift hypothesis focusing on the neglected question of how the availability of resources—both internal and external—are factored in to DA-mediated computations and signaling about value, and corresponding decisions to expend energy or not: to do or not to do. Our aim is to develop a basic evolutionary perspective on DA: as animals cannot control resource availability, all adaptation and survival necessarily devolves to the problem of optimally expending energy within the constraints of the economic environment in which the animal finds itself. That is, the animal cannot control its environment, but it can control its choices within that environment, specifically how it utilizes the energy it has available. A central concern here is the last part: how does an organism, and putatively the DA system, assess resource availability and incorporate that into computations signaling “the value of work” that regulate behavioral choice and energy expenditure.
Within the “motivational perspective” of DA, debate has continued as to whether, in cost-benefit computations, DA is modulating cost or benefit. Berridge and Robinson (1998), in their incentive-salience theory, suggest that DA enhances the incentive associated with stimuli, thus propelling expenditure of energy toward those stimuli, effectively driving behavior by increasing expected “benefit”. Salamone et al. (2007), in contrast, have argued that DA enhances motivation by increasing an animal’s willingness to work toward some goal, driving behavior by decreasing cost sensitivity. It is practically difficult to discern the difference between these: if expected benefit is increased, this will increase the costs an animal is willing to incur, leading to increased effort. Conversely, if sensitivity to costs is reduced, this effectively increases benefit relative to costs—and again, the animal works harder. While the recent work of Hamid et al. (2016) offers an elegant integration of these two ideas in their formulation that DA signals “the value of work”, this formulation provokes further questions.
Hamid et al. (2016) suggest that DA signals instantaneous value, which at target regions could both increase the incentive associated with relevant stimuli (increase expected benefit) and energize responses (decrease cost sensitivity). That is, DA instructs the animal both how valuable the stimuli/reward are and how much effort should be expended. In a sense, this begs the question: how are costs and benefits weighed and factored in to generate an instantaneous value signal that both increases incentive and decreases cost-sensitivity. Simplistically, acceptable costs and benefit should mirror each other such that the greater the benefit, the greater the willingness to incur costs. However, the factors that determine cost and benefit are different. Benefit is computed based on utility: need or desire. Cost, however, is contingent upon available resources, i.e., ability to pay. Consequently, the willingness to incur costs does not necessarily scale with perceived benefit. Intuitively, in our daily lives what primarily constrains expenditures is not the perceived value of goods, but the resources we have to exchange for those goods; that is, rather than scaling acceptable costs to benefit, we typically have to scale perceived benefits to affordable costs. Affordability weighs the value (utility, benefit) of the potential reward against the value of the resources that must be given up to obtain that reward (costs), the latter value determined by the animal’s available resources or wealth. For example, for an animal with energetic wealth (plentiful internal stores of energy, rich energy environment), the value of energy expended in lever pressing might be very low as energy is in plentiful supply, and thus lever pressing costs may factor very little compared to the expected benefit of the reward. In contrast, for an animal in energy deficit in a scarce environment, energy expended in lever pressing may be very valuable, and thus weigh against expected benefit of reward much more greatly, depressing the net value in cost-benefit calculations. Put another way, before an animal can determine how many lever presses a given reward may be worth, the animal has to place a value on the lever presses. By analogy, before I can determine if a purchase is worth $10.00, I have to determine the worth of $10.00, which will differ greatly depending upon my general wealth, whether I am a millionaire or a poor graduate student. If DA is providing an instantaneous value signal, does this signal incorporate affordability?
The extent to which a putative DA value signal is modulated by resource availability, i.e., affordability determined by the animal’s “economic circumstances”, has been little investigated. Both Salamone’s work and our own suggest that DA mediated changes in behavioral energy expenditure are not limited to appetitive pursuit; generalized, non-appetitive behavior is altered (e.g., open field, wheel-running) and even in appetitive activities, increased energy expenditure does not necessarily lead to increased consumption; for example, hyperdopaminergic animals will work harder for the same amount of food (Beeler et al., 2010, 2012b). If energy is taken as a form of currency, these data suggest that increased DA signals energy wealth and promotes profligate rather than penurious expenditure (Beeler et al., 2012c). Returning to Hamid et al. (2016) notion of an instantaneous value signal, we suggest that affordability (i.e., value of resources expended) can be implemented in two ways: (1) by directly modulating the DA value signal itself; and/or (2) by altering the response to the DA value signal at targets regions, potentially modulating incentive and cost-sensitivity independently. We will argue for both and suggest that DA D2 receptor (D2R) plays a central role in incorporating affordability into DA mediated value signaling.
By “timescale” we mean both the period of time against which computations of available resources are calculated, both internal (organismal) and external (environmental), as well as the temporal scale(s) in which DA signaling is modulated. In foraging theory, a cardinal challenge facing animals foraging for food is known as the patch-leaving problem (Stephens and Krebs, 1986). In brief, if an animal is in a patch of food that is being depleted, such that obtaining additional food requires more time and/or effort, at what point should the animal leave the patch and seek a new food source? This pits the increasing costs associated with the current patch against the costs (time, effort, risk) of finding a new patch. The classical solution to this, known as the marginal value theorem, suggest that the optimal strategy is to leave the patch when the local rate of return drops below the average rate of return over time in the environment (Charnov, 1976; Constantino and Daw, 2015). This leaves open the question, though, of what period of time should be used to compute the average rate of return? Average rate of return for that day of foraging? The last few days? During this season (months)? Some lifetime cumulative average? A case could be made for all of these: rates of return might vary daily based on factors such as weather (windy day blowing acorns off trees or a rainy day bringing worms out of the ground, for example), shorter periods of days/weeks may vary based on growth cycles of particular plants. Clearly seasonal variation in availability of resources should be taken into account—winter foraging using a summer average rate of return could be catastrophic. Longer periods, such as might be associated with extended droughts or other fluctuations would be appropriate to take into consideration, and finally, lifetime rate of return may, importantly, reflect the general harshness of the organism’s environment, such as the difference between a desert and a farm mouse. These different possible timescales for evaluation of resource availability generate both a theoretical question—what would be the optimal choice of timescale(s)—as well as a practical, empirical question: do animals, including humans, track average value on different timescales, and if so, how is this computed and signaled and how does it contribute to decision-making?
The question of the appropriate temporal horizon against which to calculate averages has a corollary question: what is the optimal learning rate. That is, how readily should new information update prior knowledge? Mathematically, learning rate effectively determines the period of information taken into account. A high learning rate means that new information quickly outweighs prior information, favoring recency and a shorter window of averaging. Conversely, a low learning rate gives little weight to new information, favoring cumulative information over a longer period of time. Thus, in addition to the question of what are appropriate periods against which to evaluate resource availability, there is the intimately related question of what are appropriate, or optimal, learning rates by which new information should be incorporated and weighed against prior information. Optimal learning rates are contingent upon environmental conditions. In a highly variable, “noisy” environment, responding to rapid fluctuations rather than broader trends might result in suboptimal decision-making. Conversely, in a more stable environment, failure to respond to a significant change rapidly may result in lost opportunity and reduced adaptation.
Though the question of what time scales are relevant and appropriate for consideration is more obvious in the case of external, environmental resources, the same question also applies to internal, organismal resources. For brevity, we highlight the temporal difference in insulin and leptin, signaling immediate energy resources and long-term energy stores, respectively. The same issues pertain: the degree to which a transient decrease in energy (i.e., reduced blood glucose) may increase the cost an animal is willing to expend to obtain food may vary depending upon long-term stores, or reserves, of energy.
An important aspect of tracking resource availability is that, to an extent, experienced resource availability is dependent upon the animal’s choices. For example, an animal that persists longer than optimal in a depleting patch will, over time, depress their average rate of return (e.g., Wikenheiser et al., 2013). Insofar as this average rate of return contributes to decision-making, the possibility exists of entering a self-imposed, vicious cycle of diminishing returns. This mismatch between behavior and actual conditions can be observed with regards to internal resources as well, as observed in obesity where an animal with “excess” reserves may, nonetheless, favor persistent conservation and storage. In effect, an organism’s computations and subsequent choices can create a perceived or experienced environment inconsistent with the actual environment in which the animal is functioning.
In asking how resource availability might be tracked, computed and contribute to decision-making—incorporating “affordability”—the question of time scale, or temporal windows for tracking and computation, plays a critical role in defining the context of decision-making. As we hypothesize that DA signaling is modulated by resource availability, signaling affordability as well as value, we turn next to the question of temporal patterning of DA signals.
Based on early electrophysiological observations, DA cell activity has long been characterized as having two modes of firing (Grace and Bunney, 1984a,b; Hyland et al., 2002). Tonic refers to on-going, low frequency (~4 Hz) irregular firing intermittently interrupted with short, high frequency bursts of actions potentials, or phasic activity. Tonic activity is believed to maintain extracellular DA at relatively stable concentrations as the primary mode of clearance at low release rates is diffusion (Venton et al., 2003; Cragg and Rice, 2004; Arbuthnott and Wickens, 2007). Phasic activity, in contrast, generates transient elevations of DA at a subsecond timescale that can be correlated to events and stimuli occurring in the animal’s environment, suggesting phasic signaling is responsive to on-going sensory experience (Schultz et al., 1993; Carelli and Wightman, 2004; Roitman et al., 2004; Stuber et al., 2005; Tobler et al., 2005; Cheer et al., 2007; Day et al., 2007; Schultz, 2007a; Bromberg-Martin and Hikosaka, 2009; Bromberg-Martin et al., 2010; Cameron et al., 2014; Hart et al., 2014, 2015; Kishida et al., 2016). Schultz (2007b) proposed that tonic DA modulates motivational processes while phasic DA, temporally synchronized with sensory events, mediates reinforcement learning. Niv et al. (2007) proposed that tonic DA tracks average reward rate over time, such that greater average reward increases tonic DA. Increased tonic DA, in turn, energizes behavior in response to overall greater reward availability, minimizing the opportunity costs associated with failure to harvest available reward, memorably expressed as “the cost of sloth”.
The notion that DA signals through two distinct modes, tonic and phasic, is not without critics. Recent studies have observed a generalized increase in DA—not associated with temporally discrete stimuli such as cues—within the context of a task where a hungry animal has an opportunity to earn food, an effect that involves learning about the potential value associated with the task-environment (context) as this effect increases across training trials (Howe et al., 2013; Hamid et al., 2016). While it might be tempting to think of this generalized, task-related increase in DA as “tonic”, this only opens the door to the sort of questions asked above about the timescales on which “tonic” DA operates, as well as how tonic activity is regulated in association with on-going sensory experience. The generalized increase in DA signaling observed in the task context by both Howe et al. (2013) and Hamid et al. (2016) presumably reflects learning about contextual stimuli that predict reward availability similar to discrete cues but with less temporal specificity. Hamid et al. (2016) argue against the idea of distinct tonic and phasic DA signals and propose instead that at any given moment DA provides an instantaneous value signal. We agree and would argue that the moment-to-moment readout of DA activity arises as a compound signal integrating value information on multiple timescales. That is, the presence of contextual stimuli associated with increased probability of reward (e.g., task environment) is compounded with more temporally resolved stimuli (e.g., discrete cues) to generate fluctuations in instantaneous probability of reward.
Consequently, we would modify the hypothesis of Niv et al. (2007), retaining the notion that average rate of reward factors into DA signaling, but, like Hamid et al. (2016), rejecting the separation of DA activity into distinct tonic and phasic activity. Instead, opportunity for value/reward can be computed on multiple timescales—from seconds, such as the appearance of a cue-light indicating reward availability imminently, to minutes to hours, such as a constellation of stimuli indicating a context (task environment) associated with greater reward availability. This reformulation leaves open the question of how other timescales for computing the average reward rate may contribute to the observed patterns of DA signaling. That is, to what extent is task-associated increase observed in Hamid and Howe contingent on even broader timescales, such as the condition of extreme scarcity (i.e., 85% body weight food restriction) during the other 23 h of the day?
We propose that different timescales operate in a nested fashion to produce a compound instantaneous DA signal: expected value (opportunity) at a given timescale is contingent upon the average rate of reward in the broader, enclosing timescale (Figure 1). Thus, we would argue that the context-associated increase in DA across a task is predicated on the larger timescale of food restriction (FR). Similarly, the expected value of a cue in a task is predicated on the richness of the task environment; for example, we would predict that greater inter-trial intervals (less overall reward in task) would decrease the across-task increase in DA but enhance the cue-specific DA response because the opportunity indicated by the discrete cue is more valuable when the task has lower overall reward availability. Conversely, increasing reward opportunities in a session would increase across-task DA while likely diminishing cue-dependent DA responses as each individual cue is proportionally less valuable in a richer task environment. In short, value computed at one timescale is always contingent upon rate of reward, or available resources, at a broader timescale.
Figure 1. Nested temporal horizons for computing and signaling average rewardand opportunity. Each temporal period is associated with availability of reward opportunity, E(V), that should presumably motivate appropriate appetitive behavior in response. However, the value of that opportunity is contingent upon the average reward in the broader, containing temporal frame, denoted by . Average reward is presented as a progressive set of nested temporally defined contexts that determines the value of opportunity and resources expended in shorter periods of time. The extent to which DA transmits opportunity, E(V) or average reward, , by “phasic” or “tonic” DA cell activity or by alterations in the DA substrate itself is tentatively indicated by vertical bars on the right.
This notion of nested timescales of value makes sense in another way. Niv et al. (2007) propose that increases in so-called tonic DA increase vigor, but this would only be useful at those moments when an opportunity to respond (e.g., press lever) is available. What function would increased DA do between response opportunities? We suggest DA signaling arising from slower timescales (e.g., task) energizes behavior without a specific target to facilitate exploration of the environment, scaled appropriately to the richness of the environment, while a surge in DA at a shorter timescale would refocus the animal on the more discrete predictive cue, reorienting behavior to the cue/task, as suggested by Nicola’s flexible approach hypothesis (Nicola, 2010). Notably, in a very rich task environment, animals may miss more opportunities for reward, which could be explained by increased DA signaling at the task timescale, promoting exploration of the environment concomitant with decrease signaling in response to discrete cues (because they are less valuable in an environment where they occur more frequently), diminishing the strength of orienting to cues within the task. While this discussion centers on timecourse(s) of DA signaling, the crucial observation for the hypothesis being developed here is that these nested timescales of average reward (broader timescale) and expected value (narrower timescale) provide a mechanism by which value computations at every temporal resolution can be computed in the context of a more general resource availability, or average rate of reward.
Implicit in the question of “what does DA encode” is the idea that DA cells integrate multiple inputs, perform some transformation (computation) and signal value to energize behavior, in which the output signal is a function of the input, i.e., DA signal = f(input1−n). This takes for granted that the cellular machinery performing this transformation/computation is constant, reliably implementing some optimizing algorithm to compute value. We know, however, that different functional components of the DA system are not constant and are subject to regulated up- and down-modulation, including expression/function of: (i) the DA transporter (DAT) regulating reuptake and the duration of signaling arising from (burst) release events (Kristensen et al., 2011); (ii) tyrosine hydroxylase, regulating the rate of synthesis of DA (Kaushik et al., 2007; Daubner et al., 2011); (iii) vmat2, regulating the packaging of DA into vesicles (Pifl et al., 2014); (iv) readily releasable pools (Turner, 2004), regulating the ability of DA terminals to sustain release in bursts; and (v) receptors (e.g., Knab and Lightfoot, 2010; Kenny et al., 2013; Petzinger et al., 2015; Friend et al., 2016), whose up- and down-regulation can alter the cellular transduction of any given DA signal. Though this regulated, functional plasticity in the DA system has been extensively studied in pathological conditions, there has been no systematic investigation of the adaptive purpose, if any, of this capacity for regulation of the DA system under non-pathological conditions. Nonetheless, if the characteristics of the substrate upon which some neuroeconomic computation is carried out are changed, it seems likely that the result of the computation would also change. That is, the function that defines the relationship between input to midbrain DA and its subsequent output and downstream effects is dependent upon the regulated properties of the various components of DA substrate mediating this function.
Our hypothesis is that the tracking of resources, both internal and external, and the subsequent incorporation of this information into neuroeconomic decision-making as affordability, is implemented at a level of adaptation that functionally alters the computational substrate itself, the DA system. When the computational substrate is altered, so is the computation, such that any abstract function that relates DA system input to output cannot be taken for granted but has to qualify the characteristics of the DA system that is performing that function: DA signal = f(K, input1−n), where K is a vector characterizing the state of different functional aspects of the DA system, such as release probability, reuptake, receptor density and so on. Simply put, we propose: (i) the DA substrate undergoes (genetic and other) regulation to adapt the computational substrate—and the computations—that determine the value of work; (ii) in accordance to the prevailing economy in which the animal finds itself, determined by the general availability of resources, both internal and external; and (iii) effectively scaling the value of work—the value of the energy expended—to match available resources, i.e., incorporating affordability into value computations (Figure 2).
Figure 2. Functional aspects of the DA system substrate available for regulation in putatively implementing affordability to adapt to economic conditions.
The idea that aspects of the DA system can change is not new, and has been examined in a variety of contexts, mostly pathological, including addiction (Lüscher and Malenka, 2011; Volkow and Morales, 2015), obesity (Baik, 2013; Kenny et al., 2013; Naef et al., 2015; Décarie-Spain et al., 2016) and Parkinson’s and Huntington’s disease (Dauer and Przedborski, 2003; Calabresi et al., 2009; Dawson et al., 2010; Chen et al., 2013; Surmeier et al., 2014; Bastide et al., 2015). In addition, it has been studied in combination with environmental factors, including diet (South and Huang, 2008; Morris et al., 2011; Speed et al., 2011; Cone et al., 2013; Adams et al., 2015; Hryhorczuk et al., 2016; Fordahl and Jones, 2017), FR (Zhen et al., 2006; Sevak et al., 2008; Carr et al., 2009; Branch et al., 2013; Stouffer et al., 2015; Fordahl and Jones, 2017), stress (Abercrombie et al., 1989; Cabib and Puglisi-Allegra, 1996, 2012; Gambarana et al., 1999; Mizoguchi et al., 2000) and exercise (Foley and Fleshner, 2008; Knab and Lightfoot, 2010; Vučković et al., 2010; Garland et al., 2011; Fisher et al., 2013; Petzinger et al., 2015). Similarly, the regulation of DA via internal signals such as insulin (Carvelli et al., 2002; Mebel et al., 2012; Labouèbe et al., 2013; Stouffer et al., 2015), leptin (Brunetti et al., 1999; Hommel et al., 2006; Perry et al., 2010; Billes et al., 2012), ghrelin (Jerlhag et al., 2007; Abizaid, 2009; van Zessen et al., 2012; Cone et al., 2014), glucocorticoids (Rougé-Pont et al., 1995, 1998; Piazza et al., 1996; Piazza and Le Moal, 1997; Van Craenenbroeck et al., 2005), GLP-1 (Dickson et al., 2012; Skibicka, 2013) and others has also been extensively studied. There is no doubt that the components of the DA system that comprise a computational substrate for economic decision-making are plastic, able to up- and down-regulate synthesis (Lindblom et al., 2006; Li et al., 2009), packaging and release (Turner, 2004; Lohr and Miller, 2014), reuptake (Jones et al., 2017) and receptor expression, trafficking and function (Tirotta et al., 2008; Knab and Lightfoot, 2010; Kenny et al., 2013; Petzinger et al., 2015; Friend et al., 2016).
However, a gap seems to exist between those that approach the question “what does DA encode”, using methods such as electrophysiology and fast-scan cyclic voltammetry (more recently photometry), often in conjunction with computational models, and those that study how the DA system itself can be altered by various environmental and organismal conditions. In particular, normative theoretical models (e.g., temporal difference learning) have not systematically or formally treated how changes in the DA substrate would alter this computational function. Conversely, those studying how a particular condition alters the DA system typically interpret findings in the context of the condition being studied—for example, how changes in DA facilitate obesity—without placing this in the larger context of the neuroeconomic role of DA in decision-making generally. This leaves a gulf between formal, normative computational accounts and physiologically oriented, systems-like engineering accounts of DA, a gap between coders and tinkerers. The result is a large lacuna: while we know the DA system can undergo adaptive changes, we study this primarily in pathological conditions and have little idea what the broader adaptive purpose of this evolved plasticity may be and how it may serve adaptive computational goals.
Approaching this lacuna requires a two-fold strategy: one, considering this substrate plasticity of DA not as arbitrary phenomena that arises under particular pathological conditions, but as an evolved mechanism serving a specific adaptive function, systematically investigating what factors, particularly environmental, determine the “set-point” of various aspects of the DA system, such as synthesis, receptor expression level and so on, and how that “set-point” alters decision-making and adaptive behavior. Two, in formal models of DA as a computational substrate, begin to ask how a change in one aspect of the DA system alters the resulting computations, matching elements of DA substrate to terms and parameters in models and adopting a normative perspective on substrate regulation of DA signaling.
In this section, we will focus on the basal ganglia, primarily the striatum, as a key substrate mediating DA-dependent neuroeconomic decision-making. Building on the distinction between value attributed to a potential reward (benefit) and the value assessed on the resources expended to obtain that reward (costs), we propose that striatal D1R signaling mediates computing the value of the reward pursued; that is, benefit or utility, while striatal D2R mediates computing the value of the resources expended. We propose that cost-benefit decision-making is modulated by the balance of between D1R and D2R signaling on the direct and indirect pathways (Figure 3), where D2R, adapting to available resources from abundance to scarcity, implements cost constraints that determine affordability.
Figure 3. Recasting the direct (GO) and indirect (NOGO) corticostriatal pathways in terms of thrift and affordability. Cortical activities associated with different behavioral options send afferents to both the direct and indirect corticostriatal pathways. D1 and D2 expressed on direct and indirect pathway MSNs, respectively, both facilitate behavioral activation by facilitating and disinhibiting the associated cortical activity. Holding D1 constant for illustration, an increase in D2 expression confers greater disinhibition in response to DA, decreasing the threshold of facilitation necessary in the direct pathway to engage a behavior. Conversely, reduction in D2 confers a smaller disinhibition in response to DA, requiring greater facilitation from the direct pathway to overcome the inhibition. In the hypothesis proposed here, we suggest that the D1-expressing, striatonigral GO pathway effectively encodes utility/benefit while the D2-expressing, striatopallidal pathway implements cost constraints by up- and down-regulating D2 expression and function in response to general economic conditions, which effectively constrains facilitation by utility/benefit, implementing affordability.
The rich DA innervation of the striatum makes it an ideal candidate substrate for regulating thrift. Though the exact function of the basal ganglia remains controversial (Redgrave et al., 1999; Nambu, 2008; Desmurget and Turner, 2010; Shiflett and Balleine, 2011), cortico-basal ganglia-cortical re-entrant loops modulate and effectively filter cortical activity (Beeler, 2011; Beeler et al., 2013). As the primary input nucleus from the cortex to the basal ganglia, the striatum is a key substrate modulating this cortical filtering (Alexander and Crutcher, 1990; Parent and Hazrati, 1995; Lovinger, 2010). The dual pathway architecture in which the direct, or GO pathway facilitates cortical activity while the indirect, NOGO pathway inhibits it, provides a functional substrate for regulating behavioral thrift by providing, in effect, an accelerator and brake, respectively (Albin et al., 1989; DeLong, 1990; Gerfen, 1992; Mink, 1996; Frank, 2005; Kravitz et al., 2010; Beeler, 2011; Durieux et al., 2012; Freeze et al., 2013). The terms “accelerator” and “brake” are synonymous with “GO” and “NOGO” and not intended to imply a novel theory. Rather, because the GO/NOGO terminology is indelibly associated with the action selection hypothesis (Redgrave et al., 1999), where the GO pathway selects one action and the NOGO suppresses others (Mink, 1996), we shift to the “accelerator/brake” metaphor to dissociate the opposing facilitatory/inhibitory actions of the dual pathways from the selection of discrete actions and to emphasize a more generalized facilitation/inhibition of cortical activity. Selective facilitation/inhibition of particular cortical activity, as connoted by the terms GO/NOGO, depends upon corticostriatal plasticity and learning, which is also DA-dependent. Activation of D1R and D2R is required for long-term potentiation and long-term depression in the direct and indirect pathways, respectively, both of which facilitate selected cortical activity in future behavior (Calabresi et al., 2007; Surmeier et al., 2009; Lovinger, 2010; Lerner and Kreitzer, 2011). Thus, DA regulates both on-going, current behavior through modulation of MSN excitability as well as future behavior by modulating corticostriatal synaptic plasticity.
Through these dual pathways, DA regulates behavioral activation by gating the inhibitory tone on cortical activity. Increased DA can result in both stereotypic and disorganized behavior (Seeman and Kapur, 2000; Lewis et al., 2007; Tanimura et al., 2009; Langen et al., 2011; van Enkhuizen et al., 2014), increasing activity and energy expenditure, consistent with a released “brake” in combination with too much activation of the “accelerator”. In contrast, low DA results in too much brake and lack of accelerator, such as described in the classic model of motor deficits in Parkinson’s (Albin et al., 1989; DeLong, 1990; Frank, 2005; Kravitz et al., 2010). While the direct and indirect pathways could operate symmetrically, such that accelerator and brake mirror each other, there is no requirement for this to be so (Park et al., 2013; Tomer et al., 2013; Cazorla et al., 2014; Oldenburg and Sabatini, 2015; Nagano-Saito et al., 2017). In fact, it has frequently been observed that one population of receptors, either D1R or D2R, is up- or down-regulated while the other is not, indicating that the balance between these two circuits can be shifted. The relative expression of these two populations of striatal DA receptors will differentially alter the sensitivity of either the brake or accelerator to DA release. For example, if D2R were maximally expressed on the indirect pathway while D1R is greatly reduced, this would “open” the indirect pathway inhibitory gate on cortical activity without providing much selective facilitation in the direct pathway, generating increased but unfocused activity. Conversely, if D1R is maximally expressed but D2R is absent, DA would not release inhibition on cortical activity exerted by the indirect pathway, allowing only the strongest inputs through the direct pathway to facilitate cortical activity, presumably resulting in a paucity of behavior, permitting only the most highly motivated and reinforced behaviors to be expressed (Figure 3).
Similarly, selective facilitation/disinhibition arising from learning depends upon complementary corticostriatal plasticity in the two pathways, such that LTP in the direct pathway selectively facilitates cortical activity while LTD in the indirect pathway selectively releases the same activity from inhibition (Lovinger, 2010; Lerner and Kreitzer, 2011). But again nothing requires these complementary processes to be symmetrical or balanced. For example, a lack of D2R activation can impair LTD in the indirect pathway, even inverting it to LTP (Calabresi et al., 1997; Picconi et al., 2003; Kreitzer, 2005; Shen et al., 2008; Thiele et al., 2014). Independent regulation of these two populations of DA receptors, then, could also affect the relative balance of selective facilitation/inhibition through synaptic plasticity and learning (Wiecki et al., 2009; Wiecki and Frank, 2010; Beeler, 2011; Beeler et al., 2012a; Zhuang et al., 2013).
As noted, though we propose that striatal D1R and D2R mediates the modulation of utility and costs, respectively, in response to DA signals, from here we will focus only on D2R and the implementation of cost control.
D2R in the striatum has been associated with the regulation of motivated, appetitive behavior, including reinforcement learning and behavioral flexibility. D2R has also been implicated in generalized behavioral activation (Tataranni et al., 2001; Kravitz et al., 2010; Klinker et al., 2013; Beeler et al., 2016; Friend et al., 2016; Lemos et al., 2016; Thanos et al., 2016). Each could potentially explain the other; that is, blocking D2R could reduce motivation, effectively reducing activity or, conversely, a generalized restriction on activity and energy expenditure could diminish apparent motivation. The two alternative interpretations can be integrated by construing D2R expression as mediating a cost threshold—encoding the value of resources expended—that gates energy expenditure, affecting both willingness to expend energy in the pursuit of specific reward as well as generalized energy expenditure.
Because of its higher affinity for DA (Rice and Cragg, 2008), D2R activation in the striatum has been proposed to be largely saturated at tonic levels of extracellular DA. In contrast, D1R is not saturated and more responsive to phasic DA (Dreyer et al., 2010), suggesting that indirect pathway D2R regulates tonic inhibitory tone on cortical activity and, in turn, basal activity and behavioral energy expenditure. This is consistent with data that suggest D2R can regulate the functional balance in basal ganglia circuitry (Cazorla et al., 2014). A transient increase in DA, then, would act primarily through D1R to facilitate cortical activity in generating a response, but D2R in the indirect pathway determines the strength of the inhibitory tone that has to be overcome by D1R facilitation. Learning (LTD) in the indirect pathway can allow release of cortical inhibition from specific afferents, exempting selected cortical activity from basal inhibition, possibly by-passing “cost control”. However, alterations in D2R also modulate synaptic plasticity at corticostriatal synapses in the indirect pathway (Calabresi et al., 2007; Shen et al., 2008; Di Filippo et al., 2009; Lovinger, 2010; Lerner and Kreitzer, 2011; Thiele et al., 2014), setting a threshold for afferent activity required to induce LTD (Kheirbek et al., 2009; Wiecki et al., 2009; Augustin et al., 2014). By regulating the stringency of requirements for learning that affects future, selective release of inhibitory tone, D2R could provide a mechanism for regulating learning rate in response to DA signals.
Data are generally consistent with this proposal. Using pharmacology and lesion methods, Salamone and colleagues, as well as others, have repeatedly demonstrated that reduced DA, including reduced D2R activation specifically, diminishes an animal’s willingness to work for reward (Koch et al., 2000; Nowend et al., 2001; Baldo et al., 2002; Woolverton and Ranaldi, 2002; Salamone et al., 2007, 2009b; Pardo et al., 2012). As this is only observed at higher costs (ratio strain, Aberman and Salamone, 1999; Salamone et al., 2001), Salamone has consistently interpreted these data as reflecting increased sensitivity to costs (implying, in converse, that D2R activation decreases sensitivity to costs, Salamone et al., 2009a). Consistent with this, Soto et al. (2011) show that D2R KO mice show greater elasticity in their demand for food, such that as costs increase, they reduce their consumption to a greater extent than wild-type controls, despite presumably being more hungry, increasing the utility of food reward, as a consequence of consuming less. Blockade of D2R also reduces general behavioral activity, such as homecage or open-field activity, demonstrated most clearly with D2R knockout/knockdown mice that show reduced activity on various measures under various conditions (Tran et al., 2002; Klinker et al., 2013; Beeler et al., 2016; Friend et al., 2016; Thanos et al., 2016), data consistent with observation of a D2R reduced function polymorphism in humans that is associated with reduced activity (Tataranni et al., 2001).
In sum, D2R in the indirect pathway regulates the extent to which inhibition of cortical activity is released by DA, effectively setting a threshold gating DA-mediated behavioral activation. D1R in the direct pathway, responsive to transient increases in DA value signals, facilitates cortical activity and behavioral activation, implementing incentive associated with expected benefit or utility. This benefit-driven facilitation, however, must overcome D2R regulated indirect pathway inhibition. We propose that D2R up- and down-regulates in accordance with resource availability, particularly energetic wealth, providing a mechanism for implementing cost control; that is, by regulating the inhibitory threshold that must be overcome by direct pathway facilitation, D2R in the indirect pathway gates the release of resources in response to expected benefit. Moreover, because of its role in regulating corticostriatal plasticity in the indirect pathway, up- and down-regulation of D2R can regulate the stringency required for selectively releasing, via learning, indirect pathway inhibition of particular cortical afferents, potentially providing a mechanism by which the modulation of learning rate could also be linked to resource availability and cost control.
The question of the relative contribution of D2R to regulating appetitive motivation vs. energy expenditure has been highlighted in recent years with the observation that D2R may be reduced in obesity (for review, Kravitz et al., 2016). Though accumulating studies have been inconsistent in this finding, the idea that D2R is reduced in obesity has fueled the notion that compulsive overeating can be construed as a food addiction, where reduced D2R signaling generates a “reward deficit” that drives behaviors, such as compulsive consumption of high energy, palatable foods (Volkow and Wise, 2005; Volkow et al., 2008; Kenny, 2011a,b; Kenny et al., 2013; Blum et al., 2014). This hypothesis centers around a role of D2R in regulating appetitive, incentive motivation, but entirely neglects any potential role of D2R in regulating energy expenditure and how that may contribute to obesity (Beeler et al., 2012c; Kravitz et al., 2016). In a recent study using D2R knockdown mice (D2KD; Beeler et al., 2016), we demonstrate that these mice show a pronounced reduction in behavioral energy expenditure, including homecage activity, open-field and wheel running, but no increase in appetitive motivation or consumption. When challenged on a high fat diet (HFD), the D2KD mice did not gain more weight than wild-type controls (slightly less, in fact) nor consume more of the palatable HFD. Provision of running wheels conferred substantial protection against dietary induced obesity in wild-type mice, but not in D2KD, who exhibited dramatically less running activity. This extended to protection against glucose dysregulation where wild-type with running wheels exhibited improved glucose clearance, while running wheels had no effect on clearance in D2KD. We further tested the mice in a concurrent choice paradigm to assess their willingness to work for preferred sucrose pellets. Again, the D2KD exhibited no evidence of increased appetitive motivation and did not work more for sucrose. Surprisingly, neither did they show decreased effort for sucrose pellets, contrary to our hypothesis. This could arise because the cost and amount consumed were low and not affected by reduced D2R signaling (i.e., low ratio strain) and/or because the reduced autoreceptor increased DA signaling that, via D1R activation in the direct pathway, effectively compensated by increasing facilitation for specific rewards. Interestingly, the D2KD mice with wheels exhibited a trend toward reduced breakpoint and greater thrift (Beeler et al., 2016, Figure 5), suggesting the possibility that the constraints on energy expenditure may be more apparent as options for behavioral activity increase. This work used a global D2R knockdown. As D2R is ubiquitously expressed, this limits the ability to attribute this regulation of energy expenditure specifically to striatal D2R on iMSNs. Friend et al. (2016) subsequently published an elegant study using selective deletion of D2R in iMSNs and obtained the same results—dramatically reduced activity but no increase in consumption, specifically confirming the importance of postsynaptic D2Rs on striatal iMSNs in regulating energy expenditure. These data suggest that while manipulations of D2R may affect appetitive behavior observed with pharmacological manipulations, this is likely to arise from a fundamental role of D2R in gating behavioral energy expenditure, which we interpret as encoding the value of resources to be expended, effectively mediating, or gating, cost considerations and implementing affordability.
One of the challenges of studying D2R is that it is ubiquitously expressed, making targeted manipulations and isolation of specific populations of D2R challenging. Even within the striatum, D2R is expressed on iMSNs, DA terminals (autoreceptors), cholinergic interneurons, afferent glutamatergic terminals and in a subset of direct pathway MSNs as D1-D2 heteromers. Though careful dissection of the function of these different populations of D2R largely remains to be tackled, the question moving forward is whether these different populations can, in a coordinated fashion, subserve different aspects of a single function—energy management, for example—and whether there is an coordination in their up- and down-regulation across different populations. For example, D2R expressed on incoming glutamatergic terminals has been proposed to act as a high pass filter, effectively filtering corticostriatal transmission selecting stronger afferent activity (Cepeda et al., 2001; Bamford et al., 2004; Centonze et al., 2004). Increases or decreases in presynaptic D2R expressed on glutamate afferents could increase or decrease the threshold determining what level of cortical activity is allowed through the high pass filter, potentially implementing a stringency for behavioral activation. A higher stringency for neurotransmission would increase the impact of prior learning in determining what cortical activity is and is not transmitted.
Regulation of energy expenditure by different striatal D2R populations might be more broadly observed in its role in regulating the DA system itself. Aside from D2R’s well-known role as autoreceptor (Ford, 2014), where it can alter DA signaling, for example enhancing sensitivity to amphetamine (Bello et al., 2011), it exerts control over DA signaling in numerous other ways. D2R is known to regulate DA reuptake and the DAT (Rougé-Pont et al., 2002; Bolan et al., 2007; Benoit-Marand et al., 2011; Owens et al., 2012) with increased D2R signaling increasing DAT function, altering the duration and summation of phasic increases in DA (Garris et al., 1994; Gonon, 1997). Chronic D2R activation can inhibit synaptogenesis in DA neurons (Fasano et al., 2008), again serving as a check on DA. In addition to D2R autoreceptors expressed on DA cells and terminals, evidence suggest that D2R expressed on MSNs may also negatively regulate DA synthesis/release and DA cell activity (Anzalone et al., 2012; Krabbe et al., 2015). D2R expressed in D1-D2 heteromers can have a tonic inhibitory effect and can enhance GABA production in the VTA (Perreault et al., 2015; Shen et al., 2015; Vekshina et al., 2017). While postsynaptic D2R on iMSNs appear to permissively gate energy expenditure, these other effects on the DA system appear, like the autoreceptor, to serve to limit dopaminergic activation. A crucial question is the relative regulation of these populations of D2R. If D2R increases on iMSNs, is this accompanied by a complementary decrease in D2R in these other, negative feedback populations, generating overall greater permissiveness, or by an similar increase, limiting the extent to which greater permissiveness in iMSNs can be leveraged, implementing a homeostatic mechanism to prevent the system from spiraling out of control.
That the DA system is modulated by circulating energy signals has been clearly demonstrated over the last two decades (Figlewicz and Sipols, 2010; Vucetic and Reyes, 2010; de Araujo et al., 2011, 2012; Figlewicz, 2015), including insulin (Liu and Borgland, 2015), leptin (Fernandes et al., 2013), ghrelin (Perello and Dickson, 2015; Wei et al., 2015) and others (for example, GLP-1, Alhadeff et al., 2012; Dickson et al., 2012; Egecioglu et al., 2013). Most of this work has been developed within the framework of DA mediating appetitive drive rather than energy expenditure, suggesting that increases in energy signals diminish DA, effectively signaling satiety and reducing DA mediated appetitive drive. However, the idea that signals such as insulin and leptin will have a simple, unidirectional effect is proving inadequate to capture the complexity of the relationship between these signals and the DA system (e.g., leptin, Leinninger et al., 2009; Opland et al., 2010; Ribeiro et al., 2011). Using insulin as an example (reviewed in Liu and Borgland, 2015), insulin can regulate DAT expression and function (Carvelli et al., 2002; Garcia et al., 2005; Speed et al., 2011; Mebel et al., 2012; Kleinridders et al., 2015), TH activity (Figlewicz et al., 1996, 1998; Li et al., 2009; Könner et al., 2011), suppress afferent input onto DA cells and facilitate synaptic LTD onto DA cells (Labouèbe et al., 2013; Liu et al., 2013) and increase basal firing rate (Könner et al., 2011). While there is evidence that insulin can reduce DA mediated appetitive drive and reward (Figlewicz et al., 2006, 2007; Mebel et al., 2012), recent work has shown that in a hungry state, insulin increases DA release via insulin receptor activation of cholinergic interneurons that, in turn, enhance release from DA terminals (Stouffer et al., 2015).
Here again, we focus on D2R and ask whether D2R interactions with circulating energy signals could comprise an assessment of available energy resources necessary to determine the value of energy expended and implement cost controls. There is considerable evidence that the DA D2R plays a critical role in energy regulation generally, including interdependent regulation with both leptin and insulin. For example, D2R can regulate and mediate/modulate leptin (Kok et al., 2006; Kim et al., 2010) and insulin signaling (Figlewicz et al., 1998; Beaulieu et al., 2007; García-Tornadú et al., 2010; DeFronzo, 2011; Heni et al., 2015). Conversely, evidence suggest that D2R function can be regulated by both leptin (Pfaffly et al., 2010) and insulin (Sevak et al., 2005; Dunn et al., 2012; Owens et al., 2012). These data suggest an intimate link between D2R and systems regulating energy homeostasis; however, these studies either do not isolate a specific D2R population or they specifically identify peripheral populations, such as D2R in pancreatic islet cells (Lopez Vicchi et al., 2016). Though this is suggestive that D2R may play a critical role in energy management (Baik et al., 1995; Rowlett et al., 1995; Kelly et al., 1998; Chausmer et al., 2002; Tanabe et al., 2004; Sevak et al., 2006; Klinker et al., 2013; Beeler et al., 2016; Friend et al., 2016), there is much less data on how striatal D2R may be regulated by circulating energy signals. One study (Pfaffly et al., 2010) has directly linked leptin to regulation of striatal D2, where leptin increases D2R binding, consistent with our proposal that increased energy availability should increase D2R expression to facilitate greater energy expenditure and utilization. Another study found a negative relationship between insulin sensitivity and D2R binding potential (Dunn et al., 2012), which suggests that increased insulin sensitivity (associated with diminished caloric intake) would decrease D2R binding, again consistent with our hypothesis where a shortage of energy would reduce energy expenditure. While these limited studies directly examine the link between circulating energy signals and D2R are consistent with our hypothesis, further, more direct investigation is needed.
Most of the relevant work relating the regulation of D2R with energy availability has been conducted in either dietary induced obesity paradigms or, the opposite, food restriction (FR). Initial evidence suggested that D2R is reduced in obesity (Volkow et al., 2001, 2008; van de Giessen et al., 2013; de Weijer et al., 2014; Kessler et al., 2014) or in response to HFD, even without obesity (van de Giessen et al., 2013), but several studies have failed to replicate this finding (Dunn et al., 2010; Caravaggio et al., 2015; Cosgrove et al., 2015; Tuominen et al., 2015) or found changes in D2R associated with obesity to vary by striatal region (Guo et al., 2014; Adams et al., 2015). Animal studies have more consistently linked reduced D2R to obesity (reviewed in Kravitz et al., 2016), but interpreting these as a response to energy availability is fraught as both DIO and HFD can induce insulin resistance, which would paradoxically signal energetic scarcity despite surplus. Notably, reduced DA function has been inconsistently observed in DIO (Liu and Borgland, 2015; Décarie-Spain et al., 2016), an effect that is likely related to the length of time the animals are on the diet (Cone et al., 2013), suggesting that long-term adaptations, such as emergent metabolic disorder and insulin resistance, may underlie these reductions in DA function. Only one study looked at the effect of HFD after a short-exposure and observed an increase in D2R (South and Huang, 2008), consistent with our hypothesis that increased energy should elevate D2R to facilitate behavioral utilization of available energy. However, Sharma and Fulton (2013) subsequently observed increased D2R following 12-weeks of HFD. Thanos et al. (2008) demonstrate that FR increases striatal D2R in obese rats, apparently in contradiction to our hypothesis; however, they also demonstrate elevated circulating insulin and leptin in the obese rats compared to food restricted, indicative of glucose dysregulation and insulin resistance (Morris et al., 2011; Speed et al., 2011; Mehran et al., 2012; Fordahl and Jones, 2017). In our view, the most reasonable interpretation of the Thanos data is that FR increased leptin and insulin sensitivity (Fordahl and Jones, 2017), normalizing those systems, allowing these to effectively signal availability of energy. In a more recent human study (Dunn et al., 2012), the authors found that BMI and leptin was positively correlated with D2R, consistent with our hypothesis. The authors speculate this increased D2R availability reflects reduced endogenous DA competing with the radioligand rather than increased D2R, though determining which interpretation is correct will require further data.
The effects of FR on the DA system has been less extensively investigated, despite the fact that the vast majority of operant behavioral studies used to characterize how the DA system modulates responding to environmental contingencies employ substantial FR, typically to 85% of baseline body weight. In an equivalent human study, this would mean reducing the weight of a 170 pound man to 145 pounds before starting the study, which we might expect to have profound effects on the subject and the behavior being studied, especially when the study centers on their response to opportunities for food. In our hypothesis here, we propose that conditions of energetic scarcity would induce an overall decrease in DA function to conserve energy. On first principles, we would predict a decrease in striatal D2R as well, to increase the stringency on the inhibitory “gate” regulating energy expenditure, particularly at basal, tonic levels of extracellular DA. Carr and colleagues have elegantly investigated the effects of FR on the DA system for many years and have found, generally, that FR sensitizes the DA system (Carr, 2002; Carr et al., 2003), enhancing the effects of drugs of abuse (Carr, 2007; Liu et al., 2011; Zheng et al., 2012). These effects, however, are complex. For example, although they observe an FR-induced up-regulation in TH mRNA, functionally this appears to occur in the context of a down-regulation of DA production (Pan et al., 2006). The net result might be characterized as a down-regulated DA system that exhibits increased sensitivity when activated (Pan et al., 2006), such as by drugs of abuse. Conceptually, this is consistent with our hypothesis: in environments of scarcity, down-regulated DA would decrease generalized energy expenditure but increase its energizing response to potential reward opportunity. However, contrary to our expectation, they have found that D2R function is increased under FR (Carr et al., 2003; Collins et al., 2008; Thanos et al., 2008; but see Sevak et al., 2008). This may reflect a compensatory up-regulation to increase sensitivity to reduced DA that results in chronically reduced receptor activation.
As with DIO, studies of FR are also confounded with potential effects on insulin signaling, as caloric restriction increases insulin sensitivity (Dostálová et al., 2007; Larson-Meyer et al., 2006; Weiss et al., 2006; Schenk et al., 2008, 2011; Mercken et al., 2012; Perez-Hedo et al., 2014; Salvador-Adriano et al., 2014), potentially enhancing its effects on DA signaling. In addition, caloric restriction also induces HPA activation and a glucocorticoid stress response (Deroche et al., 1993; Tomiyama et al., 2010; Pasiakos et al., 2011; Guarnieri et al., 2012; Grayson et al., 2014), which also affects DA system function (Abercrombie et al., 1989; Piazza and Le Moal, 1998; Yadid et al., 2001; Saal et al., 2003; Krishnan et al., 2007; Anstrom et al., 2009; Daftary et al., 2009; Rasheed et al., 2010; Cabib and Puglisi-Allegra, 2012; Tye et al., 2012; Chaudhury et al., 2013; Chang and Grace, 2014; Friedman et al., 2014; Sinclair et al., 2014; Hollon et al., 2015; Mantsch et al., 2016). In particular, chronic stress appears to induce, similar to FR characterized above, a generalized reduction in DA function with an increased sensitivity to stimuli indicative of reward (Mantsch et al., 2014; McReynolds et al., 2014; Belujon and Grace, 2015). Of course, adaptations in both insulin and stress signaling may represent primary mechanisms by which the DA system is adapted to conditions of energetic scarcity. Consistent with our hypothesis, stress has generally been shown to down-regulate D2R (Papp et al., 1994; Dziedzicka-Wasylewska et al., 1997; Gershon et al., 2007; Azzinnari et al., 2014), though this may be adaptive or maladaptive depending upon timing (Żurawek et al., 2013).
While FR certainly represents environmental scarcity, the degree to which it is a good model for environmental scarcity more broadly is questionable in that it: (a) represents an extreme, likely activating starvation responses (Duclos et al., 2013); and (b) the animal has no control over the starvation. That is, in a natural environment where an animal loses 15% of its body weight in a couple of days, this might reasonably trigger a fairly dramatic response to expend whatever energy is necessary to change the circumstances or face near-certain death; in short, this would not be the time to conserve energy. This is very different from an animal in an environment where food is available, but scarce and/or costly, requiring careful expenditure of energy in order to adapt to scarce resources.
Whether investigating dietary surplus, i.e., DIO, or FR (explicitly, or simply employed as a method to motivate behavior in tasks), studies are almost entirely conducted in standard rodent housing conditions, which is in effect an impoverished environment (Würbel, 2001). Much as FR may induce starvation-related pathophysiology, the impoverished environment of standard rodent housing may also induce pathophysiology, including effects on DA function. Bardo and colleagues have been studying the effects of enriched environment on DA (largely focusing on the PFC) and susceptibility to drugs of abuse for many years (Stairs and Bardo, 2009). In general, animals in an enriched environment are less susceptible to addictive, compulsive pursuit of drugs (e.g., cocaine) than non-enriched animals (e.g., Green et al., 2010), but as with FR and DIO, the effects on the DA system are complex. Evidence suggest that enriched environments may up-regulate DA, both decreasing DAT expression and increasing DA release (Zhu et al., 2005; Niu et al., 2007; Zakharova et al., 2009; Segovia et al., 2010); however, animals in enriched conditions also show reduced locomotor activity in the open field (Bowling et al., 1993; Bowling and Bardo, 1994; Bardo et al., 1995; Green et al., 2010). Interestingly, animals in an enriched environment show a greater response to the acute locomotor effects of psychostimulants, consistent with upregulated DAT, but do not exhibit sensitization (Bowling et al., 1993; Bowling and Bardo, 1994; Bardo et al., 1995) and are less susceptible to addiction-like behaviors (Green et al., 2010). We could find no studies that directly examined D2R expression in response to environmental enrichment.
Like obesity and FR, the concept of environmental enrichment is fraught with difficulties. Enrichment has been defined and deployed in many ways and can include factors such as amount of space allotted to animals, number of conspecifics the animal is housed with (social), the provision of novel objects (toys, tunnels, changed regularly), the provision of a running wheel, or cognitive challenge (e.g., running wheels with missing rungs). One of the enduring difficulties in enrichment studies is determining the relative contribution of different aspects of enrichment to whatever subsequent behavioral or physiological differences may be observed (Simpson and Kelly, 2011; Grégoire et al., 2014). For example, given access to running wheels, rodents will generally run almost obsessively, dramatically increasing energy expenditure with a panoply of effects, including changes in glucose regulation (Hansen et al., 1998; Borghouts and Keizer, 2000), insulin receptor sensitivity (Ropelle et al., 2006; Bradley et al., 2008; Patterson et al., 2009; Krawczewski Carhuatanta et al., 2011; van Praag et al., 2014), glucocorticoid signaling (Droste et al., 2003, 2007; Nakajima et al., 2010; Clark et al., 2015; Chen et al., 2017) and the DA system (Gilliam et al., 1984; MacRae et al., 1987; Hattori et al., 1994; Fisher et al., 2004, 2013; Petzinger et al., 2007; Vučković et al., 2010; Eddy et al., 2014; Clark et al., 2015; Dang et al., 2017). Thus, in enriched environments with running wheels, observed effects could arise from environmental enrichment or from increased voluntary exercise and energy expenditure.
When asking, as we do here, how prevailing economic conditions—abundance or scarcity of resources—may alter DA mediated decision-making, we need to distinguish between an “enriched” environment in the sense of greater environmental complexity and a “rich environment” in terms of resource abundance. The hypothesis here centers on the latter, how DA adapts behavior to an economic climate of scarcity vs. plenty. In this sense, greater environmental complexity is most interesting when that complexity is related to opportunities to obtain and utilize resources, as in foraging paradigms discussed below. While studies of environmental enrichment have provided important insights, the question is whether this speaks more to the enrichment or to correction of the impoverished conditions of standard laboratory housing; that is, is “enrichment” enriched or simply less impoverished. The crucial question may lie in how different constellations of environmental characteristics, together with an environmental economy, induce a demand for adaptive behavior and how, in response, neural mechanisms and pathways might be modified to generate environment- and economy-specific adaptation.
As data accumulate and increasingly more sophisticated methods become available, including targeted genetic tools, opto- and pharmaco- genetic methods, the need for a larger conceptual framework on which to assimilate disparate but related information grows. While the work of Carr and colleagues on FR has its own purpose (addiction), as does the work of Bardo and colleagues with enriched environment (also addiction), as well as the many investigators that study feeding and obesity, all of these can be seen as different windows onto a larger system of organismal adaptation to the environment. A larger conceptual framework allows the relationships between disparate observations to be assimilated into a richer understanding.
We are not, of course, bereft of larger conceptual frameworks. Some view behavior as arising primarily from machine-like regulatory mechanisms (physiological, homeostatic), others as learned associations that generate behavioral responses to stimuli, essentially learned reflexes, still others see behavior as a computational problem. A larger conceptual framework serves not only to guide interpretation of data, but equally the formulation of questions and the design of experiments. Here we advocate for a neuroeconomic perspective: that behavior is a series of transactions with the environment in which the organism must optimize its return on the energy and resources (time, attention, memory) it expends. This decidedly does not, in our view, mean maximizing reward, but rather balancing the expenditure of resource assets to obtain additional resources and maintain an optimal state of “wealth” given specific environmental conditions, or economic climate in which the animal finds itself.
In order to see how different interlocking pieces of the puzzle fit together, there is a need for more naturalistic, more complex behavioral paradigms that offer the animals meaningful choices in response to environmental constraints and opportunities. Building on elegant work in behavioral ethology in the 70s and 80s, there has been a growing re-emergence of interest in foraging paradigms (Pearson et al., 2014; Calhoun and Hayden, 2015; Constantino and Daw, 2015). Broadly, in the sense intended here, foraging paradigms are those behavioral tasks in which the animal’s choices substantially alter the subjective, aggregate characteristics of the environment. As a rudimentary example, in our homecage progressive ratio, the average size of meals an animal chooses to eat determines the overall, average cost of food, as illustrated by hyperdopaminergic mice in this paradigm, who work twice as much for the same amount of food. In this example, the average cost of food is not fixed in the environment but arises as a consequence of the animal’s choices; the hyperdopaminergic mice experience a more costly environment. While the more common question in such paradigms is how alterations in particular neural substrates alter behavior, an equally important but less studied question is how different environmental conditions alter the neural substrates that mediate choice, which can in turn alter the subjective experience of the environment. A review of foraging or semi-naturalistic paradigms is beyond the scope of this review (see Pearson et al., 2014; Calhoun and Hayden, 2015); however, we wish to highlight that as our knowledge accumulates on the neural substrates regulating behavior, more naturalistic paradigms that allow us to observe how atomistic components of behavior are integrated in response to complex environments will become increasingly necessary.
Richer, more complex behavioral paradigms require richer interpretive models. Theoretical, computational neuroscience is in renaissance, providing elegant models of behavior and neural function increasingly linked empirically to underlying neural substrates. Within the DA field, temporal difference learning models (Schultz et al., 1997; Sutton and Barto, 1998) have changed the theoretical landscape, providing rigorous formal theories for understanding DA function and its mediation of choice behavior. In more complex paradigms, formal modeling is often necessary to understand data that cannot be boiled down to a simple metric, such as breakpoint in progressive ratio. While such models are typically anchored in either behavioral or physiological data, they have not generally been integrated with what might be considered non-normative, non-computational physiological processes linked to more historical perspectives, such as homeostatic mechanisms or, as discussed here, changes in the computational substrate itself. That is, how up- or down-regulation of DAT, release probability, or receptors might change decisions arising from temporal difference computational algorithms has largely not been addressed, with notable exceptions. Keramati and Gutkin (2014) developed a model on how physiological “drive” mechanisms could be instantiated through TD algorithms to maintain homeostasis. Frank et al. (2009), Collins and Frank (2014) and Cox et al. (2015) are developing models in which DA value and error signals are parsed into separate channels signaling through D1R and D2R receptors, linking these with positive and negative prediction errors, respectively. Like behavior paradigms, computational models are simplifications; such simplifications often include ignoring “analog” adaptations such as up-regulating a gene, altering release probability and so on. An important task for future theoretical modeling might be to tame these messy biological adaptations by incorporating them into normative models; that is, the up-regulation of DAT changes DA signaling, but under what conditions should DAT be up- or down-regulated in order to achieve optimal decision-making and behavior? There is a broad vista open for incorporating these permutations in biological substrate as functional, parameterized components of formal models. While some changes in biological substrates may simply alter existing components of a model, such as inverse temperature (e.g., Beeler et al., 2010), learning rate (Frank et al., 2009) or delay discounting, others may be less definable within the current terms of the models, requiring elaboration and development, such as Frank et al. (2009) separating positive and negative signals based on D1R and D2R transmission. Our hypothesis, outlined here, suggest one aspect not incorporated into current models: the question of affordability—how the availability or wealth of resources necessary to pursue reward—are calculated into cost-benefit decisions.
Construing the DA system as a central regulator of resource expenditure, most fundamentally energy, provides a useful framework for integrating many observations and apparently disparate functions of the midbrain DA system. DA is often associated with compulsive behavioral disorders, such as addiction and obesity. Elsewhere, we have proposed that DA might be better construed as mediating behavioral flexibility rather than simply driving behavior toward reward (Beeler et al., 2014a,b). A critical aspect of flexible behavioral adaptation is “living within one’s means”; that is, adapting choices and expenditure of resources to resource wealth. One of the most interesting aspects of this perspective is that behavioral choices can shape individual, subjective experience of an environment as much as the actual characteristics of the environment itself (e.g., Wikenheiser et al., 2013). While psychology has long been interested in subjective experience and perceived environments (e.g., perceived stress, Gibson’s “affordances”), the notion that the computations an animal performs in assessing and interacting with its environment can substantively alter the experienced characteristics of that environment provides a window onto the idea of experienced environment that is concrete and can be formalized and studied in animal models (e.g., Wikenheiser et al., 2013). While foraging theory sought to understand how behavior is optimized (Charnov, 1976; Stephens and Krebs, 1986), and more recently the neural substrates that mediate such optimized behavior (Pearson et al., 2014; Calhoun and Hayden, 2015), an extension of this is to systematically characterize and formally describe suboptimal behavior—and its consequences—and the changes in neural substrates that produce it.
DA abnormalities have been implicated in numerous neuropsychiatric disorders, often framed in terms of reward processing. However, altered regulation of energy expenditure is a characteristic across many disorders, including depression, addiction, schizophrenia and attention-deficit hyperactivity disorder. An essential aspect of these disorders might be dysfunction in how the brain allocates energy and resources in economic decision-making. There is an increasing call to start to rethink psychiatric disorders in theoretical, computational terms, as computational systems gone awry (Sharp et al., 2012; Culbreth et al., 2016; Gillan et al., 2016; Huys et al., 2016). In doing so, we suggest that the crucial evolutionary computational problem is adapting energy expenditure to the environmental economy—to live on a budget—in order to maximize probability of survival. One approach is to link formally described suboptimal decision-making, as noted above, with observable behavioral characteristics under an umbrella of “scarcity (or surplus) phenotype”. This characterization can be further elaborated by considering that suboptimal behavior could arise from different origins: real scarcity in the environment (e.g., poverty), false scarcity arising from pathophysiology in computational substrates (e.g., insulin resistance) or induced or experienced scarcity arising from suboptimal, maladaptive choices.
In an interesting study, Mani et al. (2013) showed that cycles of economic plenty and scarcity altered cognitive function in farmers. Both human and animal studies show that animals can adapt decision-making strategies to environmental conditions (Kolling et al., 2014; Kwak et al., 2014). Intuitively, it is not difficult to imagine that our environmental conditions, above and beyond stress, could alter how our brains make computations that determine our response to the world around us—arising not just from different inputs to these computations, but from alterations in the computational substrates themselves. Such neural adaptations to environmental and economic conditions are surprisingly understudied, though presumably they underlie a great deal of behavioral variability. Given its centrality to decision-making, motivated behavior, reinforcement learning and behavioral energy expenditure—as well as well-developed formal computational models—DA is an ideal target to begin to ask and investigate the fundamental question: how does our brain adapt neural processing and decision-making to our economic environment? In our view, the evolutionarily ancient neuromodulator DA (Vidal-Gadea and Pierce-Shimomura, 2012), with its widespread modulatory effects on the mammalian brain (Decot et al., 2017), is situated to be a central substrate mediating this economic adaptation, implementing a neurobehavioral organismal resource budget and incorporating affordability into decision-making.
JAB: conceived and wrote the hypothesis and manuscript. DM: contributed to development of the hypothesis and wrote the manuscript.
The authors declare that the research was conducted in the absence of any commercial or financial relationships that could be construed as a potential conflict of interest.
This work was supported by a grant from the Whitehall Foundation (award no. 2016-12-24; JAB).
Abercrombie, E. D., Keefe, K. A., DiFrischia, D. S., and Zigmond, M. J. (1989). Differential effect of stress on in vivo dopamine release in striatum, nucleus accumbens and medial frontal cortex. J. Neurochem. 52, 1655–1658. doi: 10.1111/j.1471-4159.1989.tb09224.x
Aberman, J. E., and Salamone, J. D. (1999). Nucleus accumbens dopamine depletions make rats more sensitive to high ratio requirements but do not impair primary food reinforcement. Neuroscience 92, 545–552. doi: 10.1016/s0306-4522(99)00004-4
Abizaid, A. (2009). Ghrelin and dopamine: new insights on the peripheral regulation of appetite. J. Neuroendocrinol. 21, 787–793. doi: 10.1111/j.1365-2826.2009.01896.x
Adams, W. K., Sussman, J. L., Kaur, S., D’souza, A. M., Kieffer, T. J., and Winstanley, C. A. (2015). Long-term, calorie-restricted intake of a high-fat diet in rats reduces impulse control and ventral striatal D2 receptor signalling—two markers of addiction vulnerability. Eur. J. Neurosci. 42, 3095–3104. doi: 10.1111/ejn.13117
Albin, R. L., Young, A. B., and Penney, J. B. (1989). The functional anatomy of basal ganglia disorders. Trends Neurosci. 12, 366–375. doi: 10.1016/0166-2236(89)90074-X
Alexander, G. E., and Crutcher, M. D. (1990). Functional architecture of basal ganglia circuits: neural substrates of parallel processing. Trends Neurosci. 13, 266–271. doi: 10.1016/0166-2236(90)90107-l
Alhadeff, A. L., Rupprecht, L. E., and Hayes, M. R. (2012). GLP-1 neurons in the nucleus of the solitary tract project directly to the ventral tegmental area and nucleus accumbens to control for food intake. Endocrinology 153, 647–658. doi: 10.1210/en.2011-1443
Anstrom, K. K., Miczek, K. A., and Budygin, E. A. (2009). Increased phasic dopamine signaling in the mesolimbic pathway during social defeat in rats. Neuroscience 161, 3–12. doi: 10.1016/j.neuroscience.2009.03.023
Anzalone, A., Lizardi-Ortiz, J. E., Ramos, M., De Mei, C., Hopf, F. W., Iaccarino, C., et al. (2012). Dual control of dopamine synthesis and release by presynaptic and postsynaptic dopamine D2 receptors. J. Neurosci. 32, 9023–9034. doi: 10.1523/jneurosci.0918-12.2012
Arbuthnott, G. W., and Wickens, J. (2007). Space, time and dopamine. Trends Neurosci. 30, 62–69. doi: 10.1016/j.tins.2006.12.003
Augustin, S. M., Beeler, J. A., McGehee, D. S., and Zhuang, X. (2014). Cyclic AMP and afferent activity govern bidirectional synaptic plasticity in striatopallidal neurons. J. Neurosci. 34, 6692–6699. doi: 10.1523/jneurosci.3906-13.2014
Azzinnari, D., Sigrist, H., Staehli, S., Palme, R., Hildebrandt, T., Leparc, G., et al. (2014). Mouse social stress induces increased fear conditioning, helplessness and fatigue to physical challenge together with markers of altered immune and dopamine function. Neuropharmacology 85, 328–341. doi: 10.1016/j.neuropharm.2014.05.039
Baik, J.-H. (2013). Dopamine signaling in food addiction: role of dopamine D2 receptors. BMB Rep. 46, 519–526. doi: 10.5483/bmbrep.2013.46.11.207
Baik, J.-H., Picetti, R., Saiardi, A., Thiriet, G., Dierich, A., Depaulis, A., et al. (1995). Parkinsonian-like locomotor impairment in mice lacking dopamine D2 receptors. Nature 377, 424–428. doi: 10.1038/377424a0
Baldo, B. A., Sadeghian, K., Basso, A. M., and Kelley, A. E. (2002). Effects of selective dopamine D1 or D2 receptor blockade within nucleus accumbens subregions on ingestive behavior and associated motor activity. Behav. Brain Res. 137, 165–177. doi: 10.1016/s0166-4328(02)00293-0
Bamford, N. S., Robinson, S., Palmiter, R. D., Joyce, J. A., Moore, C., and Meshul, C. K. (2004). Dopamine modulates release from corticostriatal terminals. J. Neurosci. 24, 9541–9552. doi: 10.1523/jneurosci.2891-04.2004
Bardo, M. T., Bowling, S. L., Rowlett, J. K., Manderscheid, P., Buxton, S. T., and Dwoskin, P. (1995). Environmental enrichment attenuates locomotor sensitization, but not in vitro dopamine release, induced by amphetamine. Pharmacol. Biochem. Behav. 51, 397–405. doi: 10.1016/0091-3057(94)00413-d
Bastide, M. F., Meissner, W. G., Picconi, B., Fasano, S., Fernagut, P.-O., Feyder, M., et al. (2015). Pathophysiology of L-dopa-induced motor and non-motor complications in Parkinson’s disease. Prog. Neurobiol. 132, 96–168. doi: 10.1016/j.pneurobio.2015.07.002
Beaulieu, J.-M., Tirotta, E., Sotnikova, T. D., Masri, B., Salahpour, A., Gainetdinov, R. R., et al. (2007). Regulation of Akt signaling by D2 and D3 dopamine receptors in vivo. J. Neurosci. 27, 881–885. doi: 10.1523/jneurosci.5074-06.2007
Beeler, J. A. (2011). Preservation of function in Parkinson’s disease: what’s learning got to do with it? Brain Res. 1423, 96–113. doi: 10.1016/j.brainres.2011.09.040
Beeler, J. A. (2012). Thorndike’s law 2.0: dopamine and the regulation of thrift. Front. Neurosci. 6:116. doi: 10.3389/fnins.2012.00116
Beeler, J. A., Cools, R., Luciana, M., Ostlund, S. B., and Petzinger, G. (2014a). A kinder, gentler dopamine… highlighting dopamine’s role in behavioral flexibility. Front. Neurosci. 8:4. doi: 10.3389/fnins.2014.00004
Beeler, J. A., Cools, R., Luciana, M., Ostlund, S. B., and Petzinger, G. (Eds). (2014b). Dopamine and Behavioral Flexibility: The Problem of Modifying Established Behavior. Lausanne, Switzerland: Frontiers Media. Available online at: http://www.frontiersin.org/books/Dopamine_and_behavioral_flexibility_the_problem_of_modifying_established_behavior/273 [accessed on September 10, 2016].
Beeler, J. A., Daw, N., Frazier, C. R. M., and Zhuang, X. (2010). Tonic dopamine modulates exploitation of reward learning. Front. Behav. Neurosci. 4:170. doi: 10.3389/fnbeh.2010.00170
Beeler, J. A., Faust, R. P., Turkson, S., Ye, H., and Zhuang, X. (2016). Low dopamine D2 receptor increases vulnerability to obesity via reduced physical activity, not increased appetitive motivation. Biol. Psychiatry 79, 887–897. doi: 10.1016/j.biopsych.2015.07.009
Beeler, J. A., Frank, M. J., McDaid, J., Alexander, E., Turkson, S., Sol Bernandez, M., et al. (2012a). A role for dopamine-mediated learning in the pathophysiology and treatment of Parkinson’s disease. Cell Rep. 2, 1747–1761. doi: 10.1016/j.celrep.2012.11.014
Beeler, J. A., Frazier, C. R. M., and Zhuang, X. (2012b). Dopaminergic enhancement of local food-seeking is under global homeostatic control. Eur. J. Neurosci. 35, 146–159. doi: 10.1111/j.1460-9568.2011.07916.x
Beeler, J. A., Frazier, C. R. M., and Zhuang, X. (2012c). Putting desire on a budget: dopamine and energy expenditure, reconciling reward and resources. Front. Integr. Neurosci. 6:49. doi: 10.3389/fnint.2012.00049
Beeler, J. A., McCutcheon, J. E., Cao, Z. F. H., Murakami, M., Alexander, E., Roitman, M. F., et al. (2012d). Taste uncoupled from nutrition fails to sustain the reinforcing properties of food. Eur. J. Neurosci. 36, 2533–2546. doi: 10.1111/j.1460-9568.2012.08167.x
Beeler, J. A., Petzinger, G., and Jakowec, M. W. (2013). The enemy within: propagation of aberrant corticostriatal learning to cortical function in Parkinson’s disease. Front. Neurol. 4:134. doi: 10.3389/fneur.2013.00134
Bello, E. P., Mateo, Y., Gelman, D. M., Noain, D., Shin, J. H., Low, M. J., et al. (2011). Cocaine supersensitivity and enhanced motivation for reward in mice lacking dopamine D2 autoreceptors. Nat. Neurosci. 14, 1033–1038. doi: 10.1038/nn.2862
Belujon, P., and Grace, A. A. (2015). Regulation of dopamine system responsivity and its adaptive and pathological response to stress. Proc. Biol. Sci. 282:20142516. doi: 10.1098/rspb.2014.2516
Benoit-Marand, M., Ballion, B., Borrelli, E., Boraud, T., and Gonon, F. (2011). Inhibition of dopamine uptake by D2 antagonists: an in vivo study: D2 antagonists inhibit dopamine uptake. J. Neurochem. 116, 449–458. doi: 10.1111/j.1471-4159.2010.07125.x
Berridge, K. C. (2007). The debate over dopamine’s role in reward: the case for incentive salience. Psychopharmacology 191, 391–431. doi: 10.1007/s00213-006-0578-x
Berridge, K. C., and Robinson, T. E. (1998). What is the role of dopamine in reward: hedonic impact, reward learning, or incentive salience? Brain Res. Rev. 28, 309–369. doi: 10.1016/s0165-0173(98)00019-8
Berridge, K. C., Robinson, T. E., and Aldridge, J. W. (2009). Dissecting components of reward: ‘liking’, ‘wanting’ and learning. Curr. Opin. Pharmacol. 9, 65–73. doi: 10.1016/j.coph.2008.12.014
Billes, S. K., Simonds, S. E., and Cowley, M. A. (2012). Leptin reduces food intake via a dopamine D2 receptor-dependent mechanism. Mol. Metab. 1, 86–93. doi: 10.1016/j.molmet.2012.07.003
Blum, K., Thanos, P. K., and Gold, M. S. (2014). Dopamine and glucose, obesity and reward deficiency syndrome. Front. Psychol. 5:919. doi: 10.3389/fpsyg.2014.00919
Bolan, E. A., Kivell, B., Jaligam, V., Oz, M., Jayanthi, L. D., Han, Y., et al. (2007). D2 receptors regulate dopamine transporter function via an extracellular signal-regulated kinases 1 and 2-dependent and phosphoinositide 3 kinase-independent mechanism. Mol. Pharmacol. 71, 1222–1232. doi: 10.1124/mol.106.027763
Borghouts, L. B., and Keizer, H. A. (2000). Exercise and insulin sensitivity: a review. Int. J. Sports Med. 21, 1–12. doi: 10.1055/s-2000-8847
Bowling, S. L., and Bardo, M. T. (1994). Locomotor and rewarding effects of amphetamine in enriched, social and isolate reared rats. Pharmacol. Biochem. Behav. 48, 459–464. doi: 10.1016/0091-3057(94)90553-3
Bowling, S. L., Rowlett, J. K., and Bardo, M. T. (1993). The effect of environmental enrichment on amphetamine-stimulated locomotor activity, dopamine synthesis and dopamine release. Neuropharmacology 32, 885–893. doi: 10.1016/0028-3908(93)90144-r
Bradley, R. L., Jeon, J. Y., Liu, F.-F., and Maratos-Flier, E. (2008). Voluntary exercise improves insulin sensitivity and adipose tissue inflammation in diet-induced obese mice. Am. J. Physiol. Endocrinol. Metab. 295, E586–E594. doi: 10.1152/ajpendo.00309.2007
Branch, S. Y., Goertz, R. B., Sharpe, A. L., Pierce, J., Roy, S., Ko, D., et al. (2013). Food restriction increases glutamate receptor-mediated burst firing of dopamine neurons. J. Neurosci. 33, 13861–13872. doi: 10.1523/jneurosci.5099-12.2013
Bromberg-Martin, E. S., and Hikosaka, O. (2009). Midbrain dopamine neurons signal preference for advance information about upcoming rewards. Neuron 63, 119–126. doi: 10.1016/j.neuron.2009.06.009
Bromberg-Martin, E. S., Matsumoto, M., and Hikosaka, O. (2010). Dopamine in motivational control: rewarding, aversive, and alerting. Neuron 68, 815–834. doi: 10.1016/j.neuron.2010.11.022
Brunetti, L., Michelotto, B., Orlando, G., and Vacca, M. (1999). Leptin inhibits norepinephrine and dopamine release from rat hypothalamic neuronal endings. Eur. J. Pharmacol. 372, 237–240. doi: 10.1016/s0014-2999(99)00255-1
Cabib, S., and Puglisi-Allegra, S. (1996). Stress, depression and the mesolimbic dopamine system. Psychopharmacology 128, 331–342. doi: 10.1007/s002130050142
Cabib, S., and Puglisi-Allegra, S. (2012). The mesoaccumbens dopamine in coping with stress. Neurosci. Biobehav. Rev. 36, 79–89. doi: 10.1016/j.neubiorev.2011.04.012
Calabresi, P., Mercuri, N. B., and Di Filippo, M. (2009). Synaptic plasticity, dopamine and Parkinson’s disease: one step ahead. Brain 132, 285–287. doi: 10.1093/brain/awn340
Calabresi, P., Picconi, B., Tozzi, A., and Di Filippo, M. (2007). Dopamine-mediated regulation of corticostriatal synaptic plasticity. Trends Neurosci. 30, 211–219. doi: 10.1016/j.tins.2007.03.001
Calabresi, P., Saiardi, A., Pisani, A., Baik, J.-H., Centonze, D., Mercuri, N. B., et al. (1997). Abnormal synaptic plasticity in the striatum of mice lacking dopamine D2 receptors. J. Neurosci. 17, 4536–4544.
Calhoun, A. J., and Hayden, B. Y. (2015). The foraging brain. Curr. Opin. Behav. Sci. 5, 24–31. doi: 10.1016/j.cobeha.2015.07.003
Cameron, C. M., Wightman, R. M., and Carelli, R. M. (2014). Dynamics of rapid dopamine release in the nucleus accumbens during goal-directed behaviors for cocaine versus natural rewards. Neuropharmacology 86, 319–328. doi: 10.1016/j.neuropharm.2014.08.006
Caravaggio, F., Raitsin, S., Gerretsen, P., Nakajima, S., Wilson, A., and Graff-Guerrero, A. (2015). Ventral striatum binding of a dopamine D2/3 receptor agonist but not antagonist predicts normal body mass index. Biol. Psychiatry 77, 196–202. doi: 10.1016/j.biopsych.2013.02.017
Carelli, R. M., and Wightman, R. M. (2004). Functional microcircuitry in the accumbens underlying drug addiction: insights from real-time signaling during behavior. Curr. Opin. Neurobiol. 14, 763–768. doi: 10.1016/j.conb.2004.10.001
Carr, K. D. (2002). Augmentation of drug reward by chronic food restriction: behavioral evidence and underlying mechanisms. Physiol. Behav. 76, 353–364. doi: 10.1016/s0031-9384(02)00759-x
Carr, K. D. (2007). Chronic food restriction: enhancing effects on drug reward and striatal cell signaling. Physiol. Behav. 91, 459–472. doi: 10.1016/j.physbeh.2006.09.021
Carr, K. D., Cabeza de Vaca, S., Sun, Y., and Chau, L. S. (2009). Reward-potentiating effects of D-1 dopamine receptor agonist and AMPAR GluR1 antagonist in nucleus accumbens shell and their modulation by food restriction. Psychopharmacology 202, 731–743. doi: 10.1007/s00213-008-1355-9
Carr, K. D., Tsimberg, Y., Berman, Y., and Yamamoto, N. (2003). Evidence of increased dopamine receptor signaling in food-restricted rats. Neuroscience 119, 1157–1167. doi: 10.1016/s0306-4522(03)00227-6
Carvelli, L., Morón, J. A., Kahlig, K. M., Ferrer, J. V., Sen, N., Lechleiter, J. D., et al. (2002). PI 3-kinase regulation of dopamine uptake. J. Neurochem. 81, 859–869. doi: 10.1046/j.1471-4159.2002.00892.x
Cazorla, M., de Carvalho, F. D., Chohan, M. O., Shegda, M., Chuhma, N., Rayport, S., et al. (2014). Dopamine D2 receptors regulate the anatomical and functional balance of basal ganglia circuitry. Neuron 81, 153–164. doi: 10.1016/j.neuron.2013.10.041
Centonze, D., Usiello, A., Costa, C., Picconi, B., Erbs, E., Bernardi, G., et al. (2004). Chronic haloperidol promotes corticostriatal long-term potentiation by targeting dopamine D2L receptors. J. Neurosci. 24, 8214–8222. doi: 10.1523/JNEUROSCI.1274-04.2004
Cepeda, C., Hurst, R. S., Altemus, K. L., Flores-Hernández, J., Calvert, C. R., Jokel, E. S., et al. (2001). Facilitated glutamatergic transmission in the striatum of D2 dopamine receptor-deficient mice. J. Neurophysiol. 85, 659–670. doi: 10.1152/jn.2001.85.2.659
Chang, C., and Grace, A. A. (2014). Amygdala-ventral pallidum pathway decreases dopamine activity after chronic mild stress in rats. Biol. Psychiatry 76, 223–230. doi: 10.1016/j.biopsych.2013.09.020
Charnov, E. L. (1976). Optimal foraging, the marginal value theorem. Theor. Popul. Biol. 9, 129–136. doi: 10.1016/0040-5809(76)90040-X
Chaudhury, D., Walsh, J. J., Friedman, A. K., Juarez, B., Ku, S. M., Koo, J. W., et al. (2013). Rapid regulation of depression-related behaviours by control of midbrain dopamine neurons. Nature 493, 532–536. doi: 10.1038/nature11713
Chausmer, A. L., Elmer, G. I., Rubinstein, M., Low, M. J., Grandy, D. K., and Katz, J. L. (2002). Cocaine-induced locomotor activity and cocaine discrimination in dopamine D2 receptor mutant mice. Psychopharmacology (Berl) 163, 54–61. doi: 10.1007/s00213-002-1142-y
Cheer, J. F., Aragona, B. J., Heien, M. L. A. V., Seipel, A. T., Carelli, R. M., and Wightman, R. M. (2007). Coordinated accumbal dopamine release and neural activity drive goal-directed behavior. Neuron 54, 237–244. doi: 10.1016/j.neuron.2007.03.021
Chen, C., Nakagawa, S., An, Y., Ito, K., Kitaichi, Y., and Kusumi, I. (2017). The exercise-glucocorticoid paradox: how exercise is beneficial to cognition, mood, and the brain while increasing glucocorticoid levels. Front. Neuroendocrinol. 44, 83–102. doi: 10.1016/j.yfrne.2016.12.001
Chen, J. Y., Wang, E. A., Cepeda, C., and Levine, M. S. (2013). Dopamine imbalance in Huntington’s disease: a mechanism for the lack of behavioral flexibility. Front. Neurosci. 7:114. doi: 10.3389/fnins.2013.00114
Clark, P. J., Amat, J., McConnell, S. O., Ghasem, P. R., Greenwood, B. N., Maier, S. F., et al. (2015). Running reduces uncontrollable stress-evoked serotonin and potentiates stress-evoked dopamine concentrations in the rat dorsal striatum. PLoS One 10:e0141898. doi: 10.1371/journal.pone.0141898
Collins, G. T., Calinski, D. M., Newman, A. H., Grundt, P., and Woods, J. H. (2008). Food restriction alters N′-propyl-4,5,6,7-tetrahydrobenzothiazole-2,6-diamine dihydrochloride (pramipexole)-induced yawning, hypothermia, and locomotor activity in rats: evidence for sensitization of dopamine D2 receptor-mediated effects. J. Pharmacol. Exp. Ther. 325, 691–697. doi: 10.1124/jpet.107.133181
Collins, A. G. E., and Frank, M. J. (2014). Opponent actor learning (OpAL): modeling interactive effects of striatal dopamine on reinforcement learning and choice incentive. Psychol. Rev. 121, 337–366. doi: 10.1037/a0037015
Cone, J. J., Chartoff, E. H., Potter, D. N., Ebner, S. R., and Roitman, M. F. (2013). Prolonged high fat diet reduces dopamine reuptake without altering DAT gene expression. PLoS One 8:e58251. doi: 10.1371/journal.pone.0058251
Cone, J. J., McCutcheon, J. E., and Roitman, M. F. (2014). Ghrelin acts as an interface between physiological state and phasic dopamine signaling. J. Neurosci. 34, 4905–4913. doi: 10.1523/JNEUROSCI.4404-13.2014
Constantino, S. M., and Daw, N. D. (2015). Learning the opportunity cost of time in a patch-foraging task. Cogn. Affect. Behav. Neurosci. 15, 837–853. doi: 10.3758/s13415-015-0350-y
Cosgrove, K. P., Veldhuizen, M. G., Sandiego, C. M., Morris, E. D., and Small, D. M. (2015). Opposing relationships of BMI with BOLD and dopamine D2/3 receptor binding potential in the dorsal striatum. Synapse 69, 195–202. doi: 10.1002/syn.21809
Cox, S. M. L., Frank, M. J., Larcher, K., Fellows, L. K., Clark, C. A., Leyton, M., et al. (2015). Striatal D1 and D2 signaling differentially predict learning from positive and negative outcomes. Neuroimage 109, 95–101. doi: 10.1016/j.neuroimage.2014.12.070
Cragg, S. J., and Rice, M. E. (2004). Dancing past the DAT at a DA synapse. Trends Neurosci. 27, 270–277. doi: 10.1016/j.tins.2004.03.011
Culbreth, A. J., Westbrook, A., Daw, N. D., Botvinick, M., and Barch, D. M. (2016). Reduced model-based decision-making in schizophrenia. J. Abnorm. Psychol. 125, 777–787. doi: 10.1037/abn0000164
Daftary, S. S., Panksepp, J., Dong, Y., and Saal, D. B. (2009). Stress-induced, glucocorticoid-dependent strengthening of glutamatergic synaptic transmission in midbrain dopamine neurons. Neurosci. Lett. 452, 273–276. doi: 10.1016/j.neulet.2009.01.070
Dang, L. C., Castrellon, J. J., Perkins, S. F., Le, N. T., Cowan, R. L., Zald, D. H., et al. (2017). Reduced effects of age on dopamine D2 receptor levels in physically active adults. Neuroimage 148, 123–129. doi: 10.1016/j.neuroimage.2017.01.018
Daubner, S. C., Le, T., and Wang, S. (2011). Tyrosine hydroxylase and regulation of dopamine synthesis. Arch. Biochem. Biophys. 508, 1–12. doi: 10.1016/j.abb.2010.12.017
Dauer, W., and Przedborski, S. (2003). Parkinson’s disease: mechanisms and models. Neuron 39, 889–909. doi: 10.1016/S0896-6273(03)00568-3
Daw, N. D., O’Doherty, J. P., Dayan, P., Seymour, B., and Dolan, R. J. (2006). Cortical substrates for exploratory decisions in humans. Nature 441, 876–879. doi: 10.1038/nature04766
Dawson, T. M., Ko, H. S., and Dawson, V. L. (2010). Genetic animal models of Parkinson’s disease. Neuron 66, 646–661. doi: 10.1016/j.neuron.2010.04.034
Day, J. J., Roitman, M. F., Wightman, R. M., and Carelli, R. M. (2007). Associative learning mediates dynamic shifts in dopamine signaling in the nucleus accumbens. Nat. Neurosci. 10, 1020–1028. doi: 10.1038/nn1923
de Araujo, I. E., Ferreira, J. G., Tellez, L. A., Ren, X., and Yeckel, C. W. (2012). The gut-brain dopamine axis: a regulatory system for caloric intake. Physiol. Behav. 106, 394–399. doi: 10.1016/j.physbeh.2012.02.026
de Araujo, I. E., Ren, X., and Ferreira, J. G. (2011). “Metabolic sensing in brain dopamine systems,” in Sensory and Metabolic Control of Energy Balance, eds W. Meyerhof, U. Beisiegel and H.-G. Joost (Berlin, Heidelberg: Springer Berlin Heidelberg), 69–86.
de Weijer, B. A., van de Giessen, E., Janssen, I., Berends, F. J., van de Laar, A., Ackermans, M. T., et al. (2014). Striatal dopamine receptor binding in morbidly obese women before and after gastric bypass surgery and its relationship with insulin sensitivity. Diabetologia 57, 1078–1080. doi: 10.1007/s00125-014-3178-z
Décarie-Spain, L., Hryhorczuk, C., and Fulton, S. (2016). Dopamine signalling adaptations by prolonged high-fat feeding. Curr. Opin. Behav. Sci. 9, 136–143. doi: 10.1016/j.cobeha.2016.03.010
Decot, H. K., Namboodiri, V. M. K., Gao, W., McHenry, J. A., Jennings, J. H., Lee, S.-H., et al. (2017). Coordination of brain-wide activity dynamics by dopaminergic neurons. Neuropsychopharmacology 42, 615–627. doi: 10.1038/npp.2016.151
DeFronzo, R. A. (2011). Bromocriptine: a sympatholytic, D2-dopamine agonist for the treatment of type 2 diabetes. Diabetes Care 34, 789–794. doi: 10.2337/dc11-0064
DeLong, M. R. (1990). Primate models of movement disorders of basal ganglia origin. Trends Neurosci. 13, 281–285. doi: 10.1016/0166-2236(90)90110-v
Deroche, V., Piazza, P. V., Casolini, P., Le Moal, M., and Simon, H. (1993). Sensitization to the psychomotor effects of amphetamine and morphine induced by food restriction depends on corticosterone secretion. Brain Res. 611, 352–356. doi: 10.1016/0006-8993(93)90526-s
Desmurget, M., and Turner, R. S. (2010). Motor sequences and the basal ganglia: kinematics, not habits. J. Neurosci. 30, 7685–7690. doi: 10.1523/JNEUROSCI.0163-10.2010
Di Filippo, M., Picconi, B., Tantucci, M., Ghiglieri, V., Bagetta, V., Sgobio, C., et al. (2009). Short-term and long-term plasticity at corticostriatal synapses: implications for learning and memory. Behav. Brain Res. 199, 108–118. doi: 10.1016/j.bbr.2008.09.025
Dickson, S. L., Shirazi, R. H., Hansson, C., Bergquist, F., Nissbrandt, H., and Skibicka, K. P. (2012). The glucagon-like peptide 1 (GLP-1) analogue, exendin-4, decreases the rewarding value of food: a new role for mesolimbic GLP-1 receptors. J. Neurosci. 32, 4812–4820. doi: 10.1523/jneurosci.6326-11.2012
Dostálová, I., Smitka, K., Papežová, H., Kvasnicková, H., and Nedvídková, J. (2007). Increased insulin sensitivity in patients with anorexia nervosa: the role of adipocytokines. Physiol. Res. 56, 587–594. Available online at: http://www.biomed.cas.cz/physiolres/pdf/56/56_587.pdf
Dreyer, J. K., Herrik, K. F., Berg, R. W., and Hounsgaard, J. D. (2010). Influence of phasic and tonic dopamine release on receptor activation. J. Neurosci. 30, 14273–14283. doi: 10.1523/JNEUROSCI.1894-10.2010
Droste, S. K., Chandramohan, Y., Hill, L. E., Linthorst, A. C. E., and Reul, J. M. H. M. (2007). Voluntary exercise impacts on the rat hypothalamic-pituitary-adrenocortical axis mainly at the adrenal level. Neuroendocrinology 86, 26–37. doi: 10.1159/000104770
Droste, S. K., Gesing, A., Ulbricht, S., Müller, M. B., Linthorst, A. C. E., and Reul, J. M. H. M. (2003). Effects of long-term voluntary exercise on the mouse hypothalamic-pituitary-adrenocortical axis. Endocrinology 144, 3012–3023. doi: 10.1210/en.2003-0097
Duclos, M., Ouerdani, A., Mormède, P., and Konsman, J. P. (2013). Food restriction-induced hyperactivity: addiction or adaptation to famine? Psychoneuroendocrinology 38, 884–897. doi: 10.1016/j.psyneuen.2012.09.012
Dunn, J. P., Cowan, R. L., Volkow, N. D., Feurer, I. D., Li, R., Williams, D. B., et al. (2010). Decreased dopamine type 2 receptor availability after bariatric surgery: preliminary findings. Brain Res. 1350, 123–130. doi: 10.1016/j.brainres.2010.03.064
Dunn, J. P., Kessler, R. M., Feurer, I. D., Volkow, N. D., Patterson, B. W., Ansari, M. S., et al. (2012). Relationship of dopamine type 2 receptor binding potential with fasting neuroendocrine hormones and insulin sensitivity in human obesity. Diabetes Care 35, 1105–1111. doi: 10.2337/dc11-2250
Durieux, P. F., Schiffmann, S. N., and de Kerchove d’Exaerde, A. (2012). Differential regulation of motor control and response to dopaminergic drugs by D1R and D2R neurons in distinct dorsal striatum subregions. EMBO J. 31, 640–653. doi: 10.1038/emboj.2011.400
Dziedzicka-Wasylewska, M., Willner, P., and Papp, M. (1997). Changes in dopamine receptor mRNA expression following chronic mild stress and chronic antidepressant treatment. Behav. Pharmacol. 8, 607–618. doi: 10.1097/00008877-199711000-00017
Eddy, M. C., Stansfield, K. J., and Green, J. T. (2014). Voluntary exercise improves performance of a discrimination task through effects on the striatal dopamine system. Learn. Mem. 21, 334–337. doi: 10.1101/lm.034462.114
Egecioglu, E., Engel, J. A., and Jerlhag, E. (2013). The glucagon-like peptide 1 Analogue Exendin-4 attenuates the nicotine-induced locomotor stimulation, accumbal dopamine release, conditioned place preference as well as the expression of locomotor sensitization in mice. PLoS One 8:e77284. doi: 10.1371/journal.pone.0077284
Fasano, C., Poirier, A., DesGroseillers, L., and Trudeau, L.-E. (2008). Chronic activation of the D2 dopamine autoreceptor inhibits synaptogenesis in mesencephalic dopaminergic neurons in vitro. Eur. J. Neurosci. 28, 1480–1490. doi: 10.1111/j.1460-9568.2008.06450.x
Fernandes, M. F., Sharma, S., Hryhorczuk, C., Auguste, S., and Fulton, S. (2013). Nutritional controls of food reward. Can. J. Diabetes 37, 260–268. doi: 10.1016/j.jcjd.2013.04.004
Figlewicz, D. P. (2015). Modulation of food reward by endocrine and environmental factors: update and perspective. Psychosom. Med. 77, 664–670. doi: 10.1097/psy.0000000000000146
Figlewicz, D. P., Bennett, J. L., Naleid, A. M., Davis, C., and Grimm, J. W. (2006). Intraventricular insulin and leptin decrease sucrose self-administration in rats. Physiol. Behav. 89, 611–616. doi: 10.1016/j.physbeh.2006.07.023
Figlewicz, D. P., Brot, M. D., McCall, A. L., and Szot, P. (1996). Diabetes causes differential changes in CNS noradrenergic and dopaminergic neurons in the rat: a molecular study. Brain Res. 736, 54–60. doi: 10.1016/s0006-8993(96)00727-5
Figlewicz, D. P., Naleid, A. M., and Sipols, A. J. (2007). Modulation of food reward by adiposity signals. Physiol. Behav. 91, 473–478. doi: 10.1016/j.physbeh.2006.10.008
Figlewicz, D. P., Patterson, T. A., Johnson, L. B., Zavosh, A., Israel, P. A., and Szot, P. (1998). Dopamine transporter mRNA is increased in the CNS of Zucker fatty (fa/fa) rats. Brain Res. Bull. 46, 199–202. doi: 10.1016/s0361-9230(98)00009-4
Figlewicz, D. P., and Sipols, A. J. (2010). Energy regulatory signals and food reward. Pharmacol. Biochem. Behav. 97, 15–24. doi: 10.1016/j.pbb.2010.03.002
Fisher, B. E., Li, Q., Nacca, A., Salem, G. J., Song, J., Yip, J., et al. (2013). Treadmill exercise elevates striatal dopamine D2 receptor binding potential in patients with early Parkinson’s diseas. Neuroreport 24, 509–514. doi: 10.1097/wnr.0b013e328361dc13
Fisher, B. E., Petzinger, G. M., Nixon, K., Hogg, E., Bremmer, S., Meshul, C. K., et al. (2004). Exercise-induced behavioral recovery and neuroplasticity in the 1-methyl-4-phenyl-1,2,3,6-tetrahydropyridine-lesioned mouse basal ganglia. J. Neurosci. Res. 77, 378–390. doi: 10.1002/jnr.20162
Foley, T. E., and Fleshner, M. (2008). Neuroplasticity of dopamine circuits after exercise: implications for central fatigue. Neuromolecular Med. 10, 67–80. doi: 10.1007/s12017-008-8032-3
Ford, C. P. (2014). The role of D2-autoreceptors in regulating dopamine neuron activity and transmission. Neuroscience 282, 13–22. doi: 10.1016/j.neuroscience.2014.01.025
Fordahl, S. C., and Jones, S. R. (2017). High-fat-diet-induced deficits in dopamine terminal function are reversed by restoring insulin signaling. ACS Chem. Neurosci. 8, 290–299. doi: 10.1021/acschemneuro.6b00308
Frank, M. J. (2005). Dynamic dopamine modulation in the basal ganglia: a neurocomputational account of cognitive deficits in medicated and nonmedicated Parkinsonism. J. Cogn. Neurosci. 17, 51–72. doi: 10.1162/0898929052880093
Frank, M. J., Doll, B. B., Oas-Terpstra, J., and Moreno, F. (2009). Prefrontal and striatal dopaminergic genes predict individual differences in exploration and exploitation. Nat. Neurosci. 12, 1062–1068. doi: 10.1038/nn.2342
Freeze, B. S., Kravitz, A. V., Hammack, N., Berke, J. D., and Kreitzer, A. C. (2013). Control of basal ganglia output by direct and indirect pathway projection neurons. J. Neurosci. 33, 18531–18539. doi: 10.1523/jneurosci.1278-13.2013
Friedman, A. K., Walsh, J. J., Juarez, B., Ku, S. M., Chaudhury, D., Wang, J., et al. (2014). Enhancing depression mechanisms in midbrain dopamine neurons achieves homeostatic resilience. Science 344, 313–319. doi: 10.1126/science.1249240
Friend, D. M., Devarakonda, K., O’Neal, T. J., Skirzewski, M., Papazoglou, I., Kaplan, A. R., et al. (2016). Basal ganglia dysfunction contributes to physical inactivity in obesity. Cell Metab. 25, 312–321. doi: 10.1016/j.cmet.2016.12.001
Gambarana, C., Masi, F., Tagliamonte, A., Scheggi, S., Ghiglieri, O., and Graziella De Montis, M. (1999). A chronic stress that impairs reactivity in rats also decreases dopaminergic transmission in the nucleus accumbens: a microdialysis study. J. Neurochem. 72, 2039–2046. doi: 10.1046/j.1471-4159.1999.0722039.x
Garcia, B. G., Wei, Y., Moron, J. A., Lin, R. Z., Javitch, J. A., and Galli, A. (2005). Akt is essential for insulin modulation of amphetamine-induced human dopamine transporter cell-surface redistribution. Mol. Pharmacol. 68, 102–109. doi: 10.1124/mol.104.009092
García-Tornadú, I., Ornstein, A. M., Chamson-Reig, A., Wheeler, M. B., Hill, D. J., Arany, E., et al. (2010). Disruption of the dopamine D2 receptor impairs insulin secretion and causes glucose intolerance. Endocrinology 151, 1441–1450. doi: 10.1210/en.2009-0996
Garland, T., Schutz, H., Chappell, M. A., Keeney, B. K., Meek, T. H., Copes, L. E., et al. (2011). The biological control of voluntary exercise, spontaneous physical activity and daily energy expenditure in relation to obesity: human and rodent perspectives. J. Exp. Biol. 214, 206–229. doi: 10.1242/jeb.048397
Garris, P. A., Ciolkowski, E. L., Pastore, P., and Wightman, R. M. (1994). Efflux of dopamine from the synaptic cleft in the nucleus accumbens of the rat brain. J. Neurosci. 14, 6084–6093.
Gerfen, C. R. (1992). The neostriatal mosaic: multiple levels of compartmental organization. Trends Neurosci. 15, 133–139. doi: 10.1016/0166-2236(92)90355-c
Gershon, A. A., Vishne, T., and Grunhaus, L. (2007). Dopamine D2-like receptors and the antidepressant response. Biol. Psychiatry 61, 145–153. doi: 10.1016/j.biopsych.2006.05.031
Gillan, C. M., Kosinski, M., Whelan, R., Phelps, E. A., and Daw, N. D. (2016). Characterizing a psychiatric symptom dimension related to deficits in goal-directed control. Elife 5:e11305. doi: 10.7554/elife.11305
Gilliam, P. E., Spirduso, W. W., Martin, T. P., Walters, T. J., Wilcox, R. E., and Farrar, R. P. (1984). The effects of exercise training on [3H]-spiperone binding in rat striatum. Pharmacol. Biochem. Behav. 20, 863–867. doi: 10.1016/0091-3057(84)90008-x
Glimcher, P. (2003). Decisions, Uncertainty, and The Brain: The Science of Neuroeconomics. Cambridge, MA: MIT Press.
Glimcher, P. W., Dorris, M. C., and Bayer, H. M. (2005). Physiological utility theory and the neuroeconomics of choice. Games Econ. Behav. 52, 213–256. doi: 10.1016/j.geb.2004.06.011
Gonon, F. (1997). Prolonged and extrasynaptic excitatory action of dopamine mediated by D1 receptors in the rat striatum in vivo. J. Neurosci. 17, 5972–5978.
Grace, A. A., and Bunney, B. S. (1984a). The control of firing pattern in nigral dopamine neurons: burst firing. J. Neurosci. 4, 2877–2890.
Grace, A. A., and Bunney, B. S. (1984b). The control of firing pattern in nigral dopamine neurons: single spike firing. J. Neurosci. 4, 2866–2876.
Grayson, B. E., Hakala-Finch, A. P., Kekulawala, M., Laub, H., Egan, A. E., Ressler, I. B., et al. (2014). Weight loss by calorie restriction versus bariatric surgery differentially regulates the hypothalamo-pituitary-adrenocortical axis in male rats. Stress 17, 484–493. doi: 10.3109/10253890.2014.967677
Green, T. A., Alibhai, I. N., Roybal, C. N., Winstanley, C. A., Theobald, D. E., Birnbaum, S. G., et al. (2010). Environmental enrichment produces a behavioral phenotype mediated by low cyclic adenosine monophosphate response element binding (CREB) activity in the nucleus accumbens. Biol. Psychiatry 67, 28–35. doi: 10.1016/j.biopsych.2009.06.022
Grégoire, C.-A., Bonenfant, D., Le Nguyen, A., Aumont, A., and Fernandes, K. J. L. (2014). Untangling the influences of voluntary running, environmental complexity, social housing and stress on adult hippocampal neurogenesis. PLoS One 9:e86237. doi: 10.1371/journal.pone.0086237
Guarnieri, D. J., Brayton, C. E., Richards, S. M., Maldonado-Aviles, J., Trinko, J. R., Nelson, J., et al. (2012). Gene profiling reveals a role for stress hormones in the molecular and behavioral response to food restriction. Biol. Psychiatry 71, 358–365. doi: 10.1016/j.biopsych.2011.06.028
Guo, J., Simmons, W. K., Herscovitch, P., Martin, A., and Hall, K. D. (2014). Striatal dopamine D2-like receptor correlation patterns with human obesity and opportunistic eating behavior. Mol. Psychiatry 19, 1078–1084. doi: 10.1038/mp.2014.102
Hamid, A. A., Pettibone, J. R., Mabrouk, O. S., Hetrick, V. L., Schmidt, R., Vander Weele, C. M., et al. (2016). Mesolimbic dopamine signals the value of work. Nat. Neurosci. 19, 117–126. doi: 10.1038/nn.4173
Hansen, P. A., Nolte, L. A., Chen, M. M., and Holloszy, J. O. (1998). Increased GLUT-4 translocation mediates enhanced insulin sensitivity of muscle glucose transport after exercise. J. Appl. Physiol. 85, 1218–1222. doi: 10.1152/jappl.1998.85.4.1218
Hart, A. S., Clark, J. J., and Phillips, P. E. M. (2015). Dynamic shaping of dopamine signals during probabilistic Pavlovian conditioning. Neurobiol. Learn. Mem. 117, 84–92. doi: 10.1016/j.nlm.2014.07.010
Hart, A. S., Rutledge, R. B., Glimcher, P. W., and Phillips, P. E. M. (2014). Phasic dopamine release in the rat nucleus accumbens symmetrically encodes a reward prediction error term. J. Neurosci. 34, 698–704. doi: 10.1523/JNEUROSCI.2489-13.2014
Hattori, S., Naoi, M., and Nishino, H. (1994). Striatal dopamine turnover during treadmill running in the rat: relation to the speed of running. Brain Res. Bull. 35, 41–49. doi: 10.1016/0361-9230(94)90214-3
Heni, M., Kullmann, S., Preissl, H., Fritsche, A., and Häring, H.-U. (2015). Impaired insulin action in the human brain: causes and metabolic consequences. Nat. Rev. Endocrinol. 11, 701–711. doi: 10.1038/nrendo.2015.173
Hollon, N. G., Burgeno, L. M., and Phillips, P. E. M. (2015). Stress effects on the neural substrates of motivated behavior. Nat. Neurosci. 18, 1405–1412. doi: 10.1038/nn.4114
Hommel, J. D., Trinko, R., Sears, R. M., Georgescu, D., Liu, Z.-W., Gao, X.-B., et al. (2006). Leptin receptor signaling in midbrain dopamine neurons regulates feeding. Neuron 51, 801–810. doi: 10.1016/j.neuron.2006.08.023
Howe, M. W., Tierney, P. L., Sandberg, S. G., Phillips, P. E. M., and Graybiel, A. M. (2013). Prolonged dopamine signalling in striatum signals proximity and value of distant rewards. Nature 500, 575–579. doi: 10.1038/nature12475
Hryhorczuk, C., Florea, M., Rodaros, D., Poirier, I., Daneault, C., Des Rosiers, C., et al. (2016). Dampened mesolimbic dopamine function and signaling by saturated but not monounsaturated dietary lipids. Neuropsychopharmacology 41, 811–821. doi: 10.1038/npp.2015.207
Huys, Q. J. M., Maia, T. V., and Frank, M. J. (2016). Computational psychiatry as a bridge from neuroscience to clinical applications. Nat. Neurosci. 19, 404–413. doi: 10.1038/nn.4238
Hyland, B. I., Reynolds, J. N. J., Hay, J., Perk, C. G., and Miller, R. (2002). Firing modes of midbrain dopamine cells in the freely moving rat. Neuroscience 114, 475–492. doi: 10.1016/s0306-4522(02)00267-1
Jerlhag, E., Egecioglu, E., Dickson, S. L., Douhan, A., Svensson, L., and Engel, J. A. (2007). Ghrelin administration into tegmental areas stimulates locomotor activity and increases extracellular concentration of dopamine in the nucleus accumbens. Addict. Biol. 12, 6–16. doi: 10.1111/j.1369-1600.2006.00041.x
Jones, K. T., Woods, C., Zhen, J., Antonio, T., Carr, K. D., and Reith, M. E. A. (2017). Effects of diet and insulin on dopamine transporter activity and expression in rat caudate-putamen, nucleus accumbens and midbrain. J. Neurochem. 140, 728–740. doi: 10.1111/jnc.13930
Kable, J. W., and Glimcher, P. W. (2009). The neurobiology of decision: consensus and controversy. Neuron 63, 733–745. doi: 10.1016/j.neuron.2009.09.003
Kaushik, P., Gorin, F., and Vali, S. (2007). Dynamics of tyrosine hydroxylase mediated regulation of dopamine synthesis. J. Comput. Neurosci. 22, 147–160. doi: 10.1007/s10827-006-0004-8
Kayser, A. S., Mitchell, J. M., Weinstein, D., and Frank, M. J. (2015). Dopamine, locus of control, and the exploration-exploitation tradeoff. Neuropsychopharmacology 40, 454–462. doi: 10.1038/npp.2014.193
Kelly, M. A., Rubinstein, M., Phillips, T. J., Lessov, C. N., Burkhart-Kasch, S., Zhang, G., et al. (1998). Locomotor activity in D2 dopamine receptor-deficient mice is determined by gene dosage, genetic background and developmental adaptations. J. Neurosci. 18, 3470–3479.
Kenny, P. J. (2011a). Common cellular and molecular mechanisms in obesity and drug addiction. Nat. Rev. Neurosci. 12, 638–651. doi: 10.1038/nrn3105
Kenny, P. J. (2011b). Reward mechanisms in obesity: new insights and future directions. Neuron 69, 664–679. doi: 10.1016/j.neuron.2011.02.016
Kenny, P. J., Voren, G., and Johnson, P. M. (2013). Dopamine D2 receptors and striatopallidal transmission in addiction and obesity. Curr. Opin. Neurobiol. 23, 535–538. doi: 10.1016/j.conb.2013.04.012
Keramati, M., and Gutkin, B. (2014). Homeostatic reinforcement learning for integrating reward collection and physiological stability. Elife 3:e04811. doi: 10.7554/eLife.04811
Kessler, R. M., Zald, D. H., Ansari, M. S., Li, R., and Cowan, R. L. (2014). Changes in dopamine release and dopamine D2/3 receptor levels with the development of mild obesity: changes in dopamine release with mild obesity. Synapse 68, 317–320. doi: 10.1002/syn.21738
Kheirbek, M. A., Britt, J. P., Beeler, J. A., Ishikawa, Y., McGehee, D. S., and Zhuang, X. (2009). Adenylyl cyclase type 5 contributes to corticostriatal plasticity and striatum-dependent learning. J. Neurosci. 29, 12115–12124. doi: 10.1523/JNEUROSCI.3343-09.2009
Kim, K. S., Yoon, Y. R., Lee, H. J., Yoon, S., Kim, S. Y., Shin, S. W., et al. (2010). Enhanced hypothalamic leptin signaling in mice lacking dopamine D2 receptors. J. Biol. Chem. 285, 8905–8917. doi: 10.1074/jbc.M109.079590
Kishida, K. T., Saez, I., Lohrenz, T., Witcher, M. R., Laxton, A. W., Tatter, S. B., et al. (2016). Subsecond dopamine fluctuations in human striatum encode superposed error signals about actual and counterfactual reward. Proc. Natl. Acad. Sci. U S A 113, 200–205. doi: 10.1073/pnas.1513619112
Kleinridders, A., Cai, W., Cappellucci, L., Ghazarian, A., Collins, W. R., Vienberg, S. G., et al. (2015). Insulin resistance in brain alters dopamine turnover and causes behavioral disorders. Proc. Natl. Acad. Sci. U S A 112, 3463–3468. doi: 10.1073/pnas.1500877112
Klinker, F., Hasan, K., Paulus, W., Nitsche, M. A., and Liebetanz, D. (2013). Pharmacological blockade and genetic absence of the dopamine D2 receptor specifically modulate voluntary locomotor activity in mice. Behav. Brain Res. 242, 117–124. doi: 10.1016/j.bbr.2012.12.038
Knab, A. M., and Lightfoot, J. T. (2010). Does the difference between physically active and couch potato lie in the dopamine system? Int. J. Biol. Sci. 6, 133–150. doi: 10.7150/ijbs.6.133
Koch, M., Schmid, A., and Schnitzler, H.-U. (2000). Role of nucleus accumbens dopamine D1 and D2 receptors in instrumental and Pavlovian paradigms of conditioned reward. Psychopharmacology 152, 67–73. doi: 10.1007/s002130000505
Kok, P., Roelfsema, F., Frölich, M., van Pelt, J., Meinders, A. E., and Pijl, H. (2006). Activation of dopamine D2 receptors lowers circadian leptin concentrations in obese women. J. Clin. Endocrinol. Metab. 91, 3236–3240. doi: 10.1210/jc.2005-2529
Kolling, N., Wittmann, M., and Rushworth, M. F. S. (2014). Multiple neural mechanisms of decision making and their competition under changing risk pressure. Neuron 81, 1190–1202. doi: 10.1016/j.neuron.2014.01.033
Könner, A. C., Hess, S., Tovar, S., Mesaros, A., Sánchez-Lasheras, C., Evers, N., et al. (2011). Role for insulin signaling in catecholaminergic neurons in control of energy homeostasis. Cell Metab. 13, 720–728. doi: 10.1016/j.cmet.2011.03.021
Krabbe, S., Duda, J., Schiemann, J., Poetschke, C., Schneider, G., Kandel, E. R., et al. (2015). Increased dopamine D2 receptor activity in the striatum alters the firing pattern of dopamine neurons in the ventral tegmental area. Proc. Natl. Acad. Sci. U S A 112, E1498–E1506. doi: 10.1073/pnas.1500450112
Kravitz, A. V., Freeze, B. S., Parker, P. R. L., Kay, K., Thwin, M. T., Deisseroth, K., et al. (2010). Regulation of parkinsonian motor behaviours by optogenetic control of basal ganglia circuitry. Nature 466, 622–626. doi: 10.1038/nature09159
Kravitz, A. V., O’Neal, T. J., and Friend, D. M. (2016). Do dopaminergic impairments underlie physical inactivity in people with obesity? Front. Hum. Neurosci. 10:514. doi: 10.3389/fnhum.2016.00514
Krawczewski Carhuatanta, K. A., Demuro, G., Tschöp, M. H., Pfluger, P. T., Benoit, S. C., and Obici, S. (2011). Voluntary exercise improves high-fat diet-induced leptin resistance independent of adiposity. Endocrinology 152, 2655–2664. doi: 10.1210/en.2010-1340
Kreitzer, A. C. (2005). Dopamine modulation of state-dependent endocannabinoid release and long-term depression in the striatum. J. Neurosci. 25, 10537–10545. doi: 10.1523/JNEUROSCI.2959-05.2005
Krishnan, V., Han, M.-H., Graham, D. L., Berton, O., Renthal, W., Russo, S. J., et al. (2007). Molecular adaptations underlying susceptibility and resistance to social defeat in brain reward regions. Cell 131, 391–404. doi: 10.1016/j.cell.2007.09.018
Kristensen, A. S., Andersen, J., Jørgensen, T. N., Sørensen, L., Eriksen, J., Loland, C. J., et al. (2011). SLC6 neurotransmitter transporters: structure, function, and regulation. Pharmacol. Rev. 63, 585–640. doi: 10.1124/pr.108.000869
Kwak, S., Huh, N., Seo, J.-S., Lee, J.-E., Han, P.-L., and Jung, M. W. (2014). Role of dopamine D2 receptors in optimizing choice strategy in a dynamic and uncertain environment. Front. Behav. Neurosci. 8:3668. doi: 10.3389/fnbeh.2014.00368
Labouèbe, G., Liu, S., Dias, C., Zou, H., Wong, J. C. Y., Karunakaran, S., et al. (2013). Insulin induces long-term depression of ventral tegmental area dopamine neurons via endocannabinoids. Nat. Neurosci. 16, 300–308. doi: 10.1038/nn.3321
Langen, M., Kas, M. J. H., Staal, W. G., van Engeland, H., and Durston, S. (2011). The neurobiology of repetitive behavior: of mice…. Neurosci. Biobehav. Rev. 35, 345–355. doi: 10.1016/j.neubiorev.2010.02.004
Larson-Meyer, D. E., Heilbronn, L. K., Redman, L. M., Newcomer, B. R., Frisard, M. I., Anton, S., et al. (2006). Effect of calorie restriction with or without exercise on insulin sensitivity, β-cell function, fat cell size, and ectopic lipid in overweight subjects. Diabetes Care 29, 1337–1344. doi: 10.2337/dc05-2565
Leinninger, G. M., Jo, Y.-H., Leshan, R. L., Louis, G. W., Yang, H., Barrera, J. G., et al. (2009). Leptin acts via leptin receptor-expressing lateral hypothalamic neurons to modulate the mesolimbic dopamine system and suppress feeding. Cell Metab. 10, 89–98. doi: 10.1016/j.cmet.2009.06.011
Lemos, J. C., Friend, D. M., Kaplan, A. R., Shin, J. H., Rubinstein, M., Kravitz, A. V., et al. (2016). Enhanced GABA transmission drives bradykinesia following loss of dopamine D2 receptor signaling. Neuron 90, 824–838. doi: 10.1016/j.neuron.2016.04.040
Lerner, T. N., and Kreitzer, A. C. (2011). Neuromodulatory control of striatal plasticity and behavior. Curr. Opin. Neurobiol. 21, 322–327. doi: 10.1016/j.conb.2011.01.005
Lewis, M., Tanimura, Y., Lee, L., and Bodfish, J. (2007). Animal models of restricted repetitive behavior in autism. Behav. Brain Res. 176, 66–74. doi: 10.1016/j.bbr.2006.08.023
Li, Y., South, T., Han, M., Chen, J., Wang, R., and Huang, X.-F. (2009). High-fat diet decreases tyrosine hydroxylase mRNA expression irrespective of obesity susceptibility in mice. Brain Res. 1268, 181–189. doi: 10.1016/j.brainres.2009.02.075
Lindblom, J., Johansson, A., Holmgren, A., Grandin, E., Nedergård, C., Fredriksson, R., et al. (2006). Increased mRNA levels of tyrosine hydroxylase and dopamine transporter in the VTA of male rats after chronic food restriction. Eur. J. Neurosci. 23, 180–186. doi: 10.1111/j.1460-9568.2005.04531.x
Liu, S., and Borgland, S. L. (2015). Regulation of the mesolimbic dopamine circuit by feeding peptides. Neuroscience 289, 19–42. doi: 10.1016/j.neuroscience.2014.12.046
Liu, S., Labouèbe, G., Karunakaran, S., Clee, S. M., and Borgland, S. L. (2013). Effect of insulin on excitatory synaptic transmission onto dopamine neurons in the ventral tegmental area in a mouse model of insulinemia. Nutr. Diabetes 3:e97. doi: 10.1038/nutd.2013.38
Liu, S., Zheng, D., Peng, X.-X., Cabeza de Vaca, S., and Carr, K. D. (2011). Enhanced cocaine-conditioned place preference and associated brain regional levels of BDNF, p-ERK1/2 and p-Ser845-GluA1 in food-restricted rats. Brain Res. 1400, 31–41. doi: 10.1016/j.brainres.2011.05.028
Lohr, K. M., and Miller, G. W. (2014). VMAT2 and Parkinson’s disease: harnessing the dopamine vesicle. Expert Rev. Neurother. 14, 1115–1117. doi: 10.1586/14737175.2014.960399
Lopez Vicchi, F., Luque, G. M., Brie, B., Nogueira, J. P., Garcia Tornadu, I., and Becu-Villalobos, D. (2016). Dopaminergic drugs in type 2 diabetes and glucose homeostasis. Pharmacol. Res. 109, 74–80. doi: 10.1016/j.phrs.2015.12.029
Lovinger, D. M. (2010). Neurotransmitter roles in synaptic modulation, plasticity and learning in the dorsal striatum. Neuropharmacology 58, 951–961. doi: 10.1016/j.neuropharm.2010.01.008
Lüscher, C., and Malenka, R. C. (2011). Drug-evoked synaptic plasticity in addiction: from molecular changes to circuit remodeling. Neuron 69, 650–663. doi: 10.1016/j.neuron.2011.01.017
MacRae, P. G., Spirduso, W. W., Cartee, G. D., Farrar, R. P., and Wilcox, R. E. (1987). Endurance training effects on striatal D2 dopamine receptor binding and striatal dopamine metabolite levels. Neurosci. Lett. 79, 138–144. doi: 10.1007/bf00177922
Mani, A., Mullainathan, S., Shafir, E., and Zhao, J. (2013). Poverty impedes cognitive function. Science 341, 976–980. doi: 10.1126/science.1238041
Mantsch, J. R., Baker, D. A., Funk, D., Lê, A. D., and Shaham, Y. (2016). Stress-induced reinstatement of drug seeking: 20 years of progress. Neuropsychopharmacology 41, 335–356. doi: 10.1038/npp.2015.142
Mantsch, J. R., Vranjkovic, O., Twining, R. C., Gasser, P. J., McReynolds, J. R., and Blacktop, J. M. (2014). Neurobiological mechanisms that contribute to stress-related cocaine use. Neuropharmacology 76, 383–394. doi: 10.1016/j.neuropharm.2013.07.021
Marr, D. (1982). Vision: A Computational Investigation into The Human Representation and Processing of Visual Information. New York, NY: W.H. Freeman and Company.
McReynolds, J. R., Peña, D. F., Blacktop, J. M., and Mantsch, J. R. (2014). Neurobiological mechanisms underlying relapse to cocaine use: contributions of CRF and noradrenergic systems and regulation by glucocorticoids. Stress 17, 22–38. doi: 10.3109/10253890.2013.872617
Mebel, D. M., Wong, J. C. Y., Dong, Y. J., and Borgland, S. L. (2012). Insulin in the ventral tegmental area reduces hedonic feeding and suppresses dopamine concentration via increased reuptake: insulin attenuates somatodendritic dopamine. Eur. J. Neurosci. 36, 2336–2346. doi: 10.1111/j.1460-9568.2012.08168.x
Mehran, A. E., Templeman, N. M., Brigidi, G. S., Lim, G. E., Chu, K. Y., Hu, X., et al. (2012). Hyperinsulinemia drives diet-induced obesity independently of brain insulin production. Cell Metab. 16, 723–737. doi: 10.1016/j.cmet.2012.10.019
Mercken, E. M., Carboneau, B. A., Krzysik-Walker, S. M., and de Cabo, R. (2012). Of mice and men: the benefits of caloric restriction, exercise, and mimetics. Ageing Res. Rev. 11, 390–398. doi: 10.1016/j.arr.2011.11.005
Mink, J. W. (1996). The basal ganglia: focused selection and inhibition of competing motor programs. Prog. Neurobiol. 50, 381–425. doi: 10.1016/s0301-0082(96)00042-1
Mizoguchi, K., Yuzurihara, M., Ishige, A., Sasaki, H., Chui, D.-H., and Tabira, T. (2000). Chronic stress induces impairment of spatial working memory because of prefrontal dopaminergic dysfunction. J. Neurosci. 20, 1568–1574.
Montague, P. R., Hyman, S. E., and Cohen, J. D. (2004). Computational roles for dopamine in behavioural control. Nature 431, 760–767. doi: 10.1038/nature03015
Morris, J. K., Bomhoff, G. L., Gorres, B. K., Davis, V. A., Kim, J., Lee, P.-P., et al. (2011). Insulin resistance impairs nigrostriatal dopamine function. Exp. Neurol. 231, 171–180. doi: 10.1016/j.expneurol.2011.06.005
Naef, L., Pitman, K. A., and Borgland, S. L. (2015). Mesolimbic dopamine and its neuromodulators in obesity and binge eating. CNS Spectr. 20, 574–583. doi: 10.1017/S1092852915000693
Nagano-Saito, A., Lissemore, J. I., Gravel, P., Leyton, M., Carbonell, F., and Benkelfat, C. (2017). Posterior dopamine D2/3 receptors and brain network functional connectivity. Synapse 71:e21993. doi: 10.1002/syn.21993
Nakajima, S., Ohsawa, I., Ohta, S., Ohno, M., and Mikami, T. (2010). Regular voluntary exercise cures stress-induced impairment of cognitive function and cell proliferation accompanied by increases in cerebral IGF-1 and GST activity in mice. Behav. Brain Res. 211, 178–184. doi: 10.1016/j.bbr.2010.03.028
Nambu, A. (2008). Seven problems on the basal ganglia. Curr. Opin. Neurobiol. 18, 595–604. doi: 10.1016/j.conb.2008.11.001
Nicola, S. M. (2010). The flexible approach hypothesis: unification of effort and cue-responding hypotheses for the role of nucleus accumbens dopamine in the activation of reward-seeking behavior. J. Neurosci. 30, 16585–16600. doi: 10.1523/JNEUROSCI.3958-10.2010
Niu, X., Tahera, Y., and Canlon, B. (2007). Environmental enrichment to sound activates dopaminergic pathways in the auditory system. Physiol. Behav. 92, 34–39. doi: 10.1016/j.physbeh.2007.05.020
Niv, Y., Daw, N. D., Joel, D., and Dayan, P. (2007). Tonic dopamine: opportunity costs and the control of response vigor. Psychopharmacology 191, 507–520. doi: 10.1007/s00213-006-0502-4
Nowend, K. L., Arizzi, M., Carlson, B. B., and Salamone, J. D. (2001). D1 or D2 antagonism in nucleus accumbens core or dorsomedial shell suppresses lever pressing for food but leads to compensatory increases in chow consumption. Pharmacol. Biochem. Behav. 69, 373–382. doi: 10.1016/s0091-3057(01)00524-x
Oldenburg, I. A., and Sabatini, B. L. (2015). Antagonistic but not symmetric regulation of primary motor cortex by basal ganglia direct and indirect pathways. Neuron 86, 1174–1181. doi: 10.1016/j.neuron.2015.05.008
Opland, D. M., Leinninger, G. M., and Myers, M. G. Jr. (2010). Modulation of the mesolimbic dopamine system by leptin. Brain Res. 1350, 65–70. doi: 10.1016/j.brainres.2010.04.028
Owens, W. A., Williams, J. M., Saunders, C., Avison, M. J., Galli, A., and Daws, L. C. (2012). Rescue of dopamine transporter function in hypoinsulinemic rats by a D2 receptor-ERK-dependent mechanism. J. Neurosci. 32, 2637–2647. doi: 10.1523/JNEUROSCI.3759-11.2012
Pan, Y., Berman, Y., Haberny, S., Meller, E., and Carr, K. D. (2006). Synthesis, protein levels, activity and phosphorylation state of tyrosine hydroxylase in mesoaccumbens and nigrostriatal dopamine pathways of chronically food-restricted rats. Brain Res. 1122, 135–142. doi: 10.1016/j.brainres.2006.09.001
Papp, M., Klimek, V., and Willner, P. (1994). Parallel changes in dopamine D2 receptor binding in limbic forebrain associated with chronic mild stress-induced anhedonia and its reversal by imipramine. Psychopharmacology 115, 441–446. doi: 10.1007/bf02245566
Pardo, M., Lopez-Cruz, L., Valverde, O., Ledent, C., Baqi, Y., Müller, C. E., et al. (2012). Adenosine A2A receptor antagonism and genetic deletion attenuate the effects of dopamine D2 antagonism on effort-based decision making in mice. Neuropharmacology 62, 2068–2077. doi: 10.1016/j.neuropharm.2011.12.033
Parent, A., and Hazrati, L.-N. (1995). Functional anatomy of the basal ganglia. I. The cortico-basal ganglia-thalamo-cortical loop. Brain Res. Rev. 20, 91–127. doi: 10.1016/0165-0173(94)00007-c
Park, K., Volkow, N. D., Pan, Y., and Du, C. (2013). Chronic cocaine dampens dopamine signaling during cocaine intoxication and unbalances D1 over D2 receptor signaling. J. Neurosci. 33, 15827–15836. doi: 10.1523/JNEUROSCI.1935-13.2013
Pasiakos, S. M., Caruso, C. M., Kellogg, M. D., Kramer, F. M., and Lieberman, H. R. (2011). Appetite and endocrine regulators of energy balance after 2 days of energy restriction: insulin, leptin, ghrelin, and DHEA-S. Obesity 19, 1124–1130. doi: 10.1038/oby.2010.316
Patterson, C. M., Bouret, S. G., Dunn-Meynell, A. A., and Levin, B. E. (2009). Three weeks of postweaning exercise in DIO rats produces prolonged increases in central leptin sensitivity and signaling. AJP Regul. Integr. Comp. Physiol. 296, R537–R548. doi: 10.1152/ajpregu.90859.2008
Pearson, J. M., Watson, K. K., and Platt, M. L. (2014). Decision making: the neuroethological turn. Neuron 82, 950–965. doi: 10.1016/j.neuron.2014.04.037
Perello, M., and Dickson, S. L. (2015). Ghrelin signalling on food reward: a salient link between the gut and the mesolimbic system. J. Neuroendocrinol. 27, 424–434. doi: 10.1111/jne.12236
Perez-Hedo, M., Rivera-Perez, C., and Noriega, F. G. (2014). Starvation increases insulin sensitivity and reduces juvenile hormone synthesis in mosquitoes. PLoS One 9:e86183. doi: 10.1371/journal.pone.0086183
Perreault, M. L., Shen, M. Y. F., Fan, T., and George, S. R. (2015). Regulation of c-fos expression by the dopamine D1–D2 receptor heteromer. Neuroscience 285, 194–203. doi: 10.1016/j.neuroscience.2014.11.017
Perry, M. L., Leinninger, G. M., Chen, R., Luderman, K. D., Yang, H., Gnegy, M. E., et al. (2010). Leptin promotes dopamine transporter and tyrosine hydroxylase activity in the nucleus accumbens of Sprague-Dawley rats: leptin promotes TH and DAT activity. J. Neurochem. 114, 666–674. doi: 10.1111/j.1471-4159.2010.06757.x
Petzinger, G. M., Holschneider, D. P., Fisher, B. E., McEwen, S., Kintz, N., Halliday, M., et al. (2015). The effects of exercise on dopamine neurotransmission in Parkinson’s disease: targeting neuroplasticity to modulate basal ganglia circuitry. Brain Plast. 1, 29–39. doi: 10.3233/bpl-150021
Petzinger, G. M., Walsh, J. P., Akopian, G., Hogg, E., Abernathy, A., Arevalo, P., et al. (2007). Effects of treadmill exercise on dopaminergic transmission in the 1-methyl-4-phenyl-1,2,3,6-tetrahydropyridine-lesioned mouse model of basal ganglia injury. J. Neurosci. 27, 5291–5300. doi: 10.1523/JNEUROSCI.1069-07.2007
Pfaffly, J., Michaelides, M., Wang, G.-J., Pessin, J. E., Volkow, N. D., and Thanos, P. K. (2010). Leptin increases striatal dopamine D2 receptor binding in leptin-deficient obese (ob/ob) mice. Synapse 64, 503–510. doi: 10.1002/syn.20755
Phillips, P. E. M., Walton, M. E., and Jhou, T. C. (2007). Calculating utility: preclinical evidence for cost-benefit analysis by mesolimbic dopamine. Psychopharmacology 191, 483–495. doi: 10.1007/s00213-006-0626-6
Piazza, P. V., and Le Moal, M. (1997). Glucocorticoids as a biological substrate of reward: physiological and pathophysiological implications. Brain Res. Rev. 25, 359–372. doi: 10.1016/s0165-0173(97)00025-8
Piazza, P. V., and Le Moal, M. (1998). The role of stress in drug self-administration. Trends Pharmacol. Sci. 19, 67–74. doi: 10.1016/s0165-6147(97)01115-2
Piazza, P. V., Rougé-Pont, F., Deroche, V., Maccari, S., Simon, H., and Le Moal, M. (1996). Glucocorticoids have state-dependent stimulant effects on the mesencephalic dopaminergic transmission. Proc. Natl. Acad. Sci. U S A 93, 8716–8720. doi: 10.1073/pnas.93.16.8716
Picconi, B., Centonze, D., Håkansson, K., Bernardi, G., Greengard, P., Fisone, G., et al. (2003). Loss of bidirectional striatal synaptic plasticity in L-DOPA-induced dyskinesia. Nat. Neurosci. 6, 501–506. doi: 10.1038/nn1040
Pifl, C., Rajput, A., Reither, H., Blesa, J., Cavada, C., Obeso, J. A., et al. (2014). Is Parkinson’s disease a vesicular dopamine storage disorder? Evidence from a study in isolated synaptic vesicles of human and nonhuman primate striatum. J. Neurosci. 34, 8210–8218. doi: 10.1523/JNEUROSCI.5456-13.2014
Rasheed, N., Ahmad, A., Pandey, C. P., Chaturvedi, R. K., Lohani, M., and Palit, G. (2010). Differential response of central dopaminergic system in acute and chronic unpredictable stress models in rats. Neurochem. Res. 35, 22–32. doi: 10.1007/s11064-009-0026-5
Redgrave, P., Prescott, T. J., and Gurney, K. (1999). The basal ganglia: a vertebrate solution to the selection problem? Neuroscience 89, 1009–1023. doi: 10.1016/s0306-4522(98)00319-4
Ribeiro, A. C., Ceccarini, G., Dupré, C., Friedman, J. M., Pfaff, D. W., and Mark, A. L. (2011). Contrasting effects of leptin on food anticipatory and total locomotor activity. PLoS One 6:e23364. doi: 10.1371/journal.pone.0023364
Rice, M. E., and Cragg, S. J. (2008). Dopamine spillover after quantal release: rethinking dopamine transmission in the nigrostriatal pathway. Brain Res. Rev. 58, 303–313. doi: 10.1016/j.brainresrev.2008.02.004
Robbins, T. W., and Everitt, B. J. (1992). Functions of dopamine in the dorsal and ventral striatum. Semin. Neurosci. 4, 119–127. doi: 10.1016/1044-5765(92)90010-y
Roitman, M. F., Stuber, G. D., Phillips, P. E., Wightman, R. M., and Carelli, R. M. (2004). Dopamine operates as a subsecond modulator of food seeking. J. Neurosci. 24, 1265–1271. doi: 10.1523/JNEUROSCI.3823-03.2004
Ropelle, E., Pauli, J. R., Prada, P. O., de Souza, C. T., Picardi, P. K., Faria, M. C., et al. (2006). Reversal of diet-induced insulin resistance with a single bout of exercise in the rat: the role of PTP1B and IRS-1 serine phosphorylation. J. Physiol. 577, 997–1007. doi: 10.1113/jphysiol.2006.120006
Rougé-Pont, F., Deroche, V., Le Moal, M., and Piazza, P. V. (1998). Individual differences in stress-induced dopamine release in the nucleus accumbens are influenced by corticosterone. Eur. J. Neurosci. 10, 3903–3907. doi: 10.1046/j.1460-9568.1998.00438.x
Rougé-Pont, F., Marinelli, M., Le Moal, M., Simon, H., and Piazza, P. V. (1995). Stress-induced sensitization and glucocorticoids. II. Sensitization of the increase in extracellular dopamine induced by cocaine depends on stress-induced corticosterone secretion. J. Neurosci. 15, 7189–7195.
Rougé-Pont, F., Usiello, A., Benoit-Marand, M., Gonon, F., Piazza, P. V., and Borrelli, E. (2002). Changes in extracellular dopamine induced by morphine and cocaine: crucial control by D2 receptors. J. Neurosci. 22, 3293–3301.
Rowlett, J. K., Mattingly, B. A., and Bardo, M. T. (1995). Repeated quinpirole treatment: locomotor activity, dopamine synthesis, and effects of selective dopamine antagonists. Synapse 20, 209–216. doi: 10.1002/syn.890200304
Saal, D., Dong, Y., Bonci, A., and Malenka, R. C. (2003). Drugs of abuse and stress trigger a common synaptic adaptation in dopamine neurons. Neuron 37, 577–582. doi: 10.1016/s0896-6273(03)00021-7
Salamone, J. D., and Correa, M. (2012). The mysterious motivational functions of mesolimbic dopamine. Neuron 76, 470–485. doi: 10.1016/j.neuron.2012.10.021
Salamone, J. D., Correa, M., Farrar, A., and Mingote, S. M. (2007). Effort-related functions of nucleus accumbens dopamine and associated forebrain circuits. Psychopharmacology (Berl) 191, 461–482. doi: 10.1007/s00213-006-0668-9
Salamone, J., Correa, M., Mingote, S., and Weber, S. (2005). Beyond the reward hypothesis: alternative functions of nucleus accumbens dopamine. Curr. Opin. Pharmacol. 5, 34–41. doi: 10.1016/j.coph.2004.09.004
Salamone, J. D., Correa, M., Farrar, A. M., Nunes, E. J., and Pardo, M. (2009a). Dopamine, behavioral economics, and effort. Front. Behav. Neurosci. 3:13. doi: 10.3389/neuro.08.013.2009
Salamone, J. D., Farrar, A. M., Font, L., Patel, V., Schlar, D. E., Nunes, E. J., et al. (2009b). Differential actions of adenosine A1 and A2A antagonists on the effort-related effects of dopamine D2 antagonism. Behav. Brain Res. 201, 216–222. doi: 10.1016/j.bbr.2009.02.021
Salamone, J. D., Wisniecki, A., Carlson, B. B., and Correa, M. (2001). Nucleus accumbens dopamine depletions make animals highly sensitive to high fixed ratio requirements but do not impair primary food reinforcement. Neuroscience 105, 863–870. doi: 10.1016/s0306-4522(01)00249-4
Salvador-Adriano, A., Vargas-Chávez, S., Hernández-Vázquez, A. D. J., Ortega-Cuellar, D., Tovar, A. R., and Velázquez-Arellano, A. (2014). Insulin sensitivity is inversely related to cellular energy status, as revealed by biotin deprivation. Am. J. Physiol. Endocrinol. Metab. 306, E1442–E1448. doi: 10.1152/ajpendoajpendo.00442.2013
Schenk, S., McCurdy, C. E., Philp, A., Chen, M. Z., Holliday, M. J., Bandyopadhyay, G. K., et al. (2011). Sirt1 enhances skeletal muscle insulin sensitivity in mice during caloric restriction. J. Clin. Invest. 121, 4281–4288. doi: 10.1172/JCI58554
Schenk, S., Saberi, M., and Olefsky, J. M. (2008). Insulin sensitivity: modulation by nutrients and inflammation. J. Clin. Invest. 118, 2992–3002. doi: 10.1172/JCI34260
Schultz, W. (2007a). Behavioral dopamine signals. Trends Neurosci. 30, 203–210. doi: 10.1016/j.tins.2007.03.007
Schultz, W. (2007b). Multiple dopamine functions at different time courses. Annu. Rev. Neurosci. 30, 259–288. doi: 10.1146/annurev.neuro.28.061604.135722
Schultz, W., Apicella, P., and Ljungberg, T. (1993). Responses of monkey dopamine neurons to reward and conditioned stimuli during successive steps of learning a delayed response task. J. Neurosci. 13, 900–913.
Schultz, W., Carelli, R. M., and Wightman, R. M. (2015). Phasic dopamine signals: from subjective reward value to formal economic utility. Curr. Opin. Behav. Sci. 5, 147–154. doi: 10.1016/j.cobeha.2015.09.006
Schultz, W., Dayan, P., and Montague, P. R. (1997). A neural substrate of prediction and reward. Science 275, 1593–1599. doi: 10.1126/science.275.5306.1593
Seeman, P., and Kapur, S. (2000). Schizophrenia: more dopamine, more D2 receptors. Proc. Natl. Acad. Sci. U S A 97, 7673–7675. doi: 10.1073/pnas.97.14.7673
Segovia, G., Del Arco, A., De Blas, M., Garrido, P., and Mora, F. (2010). Environmental enrichment increases the in vivo extracellular concentration of dopamine in the nucleus accumbens: a microdialysis study. J. Neural Transm. 117, 1123–1130. doi: 10.1007/s00702-010-0447-y
Sevak, R. J., Koek, W., and France, C. P. (2005). Streptozotocin-induced diabetes differentially modifies haloperidol- and γ-hydroxybutyric acid (GHB)-induced catalepsy. Eur. J. Pharmacol. 517, 64–67. doi: 10.1016/j.ejphar.2005.05.043
Sevak, R. J., Koek, W., Owens, W. A., Galli, A., Daws, L. C., and France, C. P. (2008). Feeding conditions differentially affect the neurochemical and behavioral effects of dopaminergic drugs in male rats. Eur. J. Pharmacol. 592, 109–115. doi: 10.1016/j.ejphar.2008.07.002
Sevak, R. J., Owens, W. A., Koek, W., Galli, A., Daws, L. C., and France, C. P. (2006). Evidence for D2 receptor mediation of amphetamine-induced normalization of locomotion and dopamine transporter function in hypoinsulinemic rats: dopamine neurotransmission in diabetic rats. J. Neurochem. 101, 151–159. doi: 10.1111/j.1471-4159.2006.04358.x
Sharma, S., and Fulton, S. (2013). Diet-induced obesity promotes depressive-like behavior that is associated with neural adaptations in brain reward circuitry. Int. J. Obes. 37, 382–389. doi: 10.1038/ijo.2012.48
Sharp, C., Monterosso, J., and Montague, P. R. (2012). Neuroeconomics: a bridge for translational research. Biol. Psychiatry 72, 87–92. doi: 10.1016/j.biopsych.2012.02.029
Shen, W., Flajolet, M., Greengard, P., and Surmeier, D. J. (2008). Dichotomous dopaminergic control of striatal synaptic plasticity. Science 321, 848–851. doi: 10.1126/science.1160575
Shen, M. Y. F., Perreault, M. L., Fan, T., and George, S. R. (2015). The dopamine D1–D2 receptor heteromer exerts a tonic inhibitory effect on the expression of amphetamine-induced locomotor sensitization. Pharmacol. Biochem. Behav. 128, 33–40. doi: 10.1016/j.pbb.2014.11.011
Shiflett, M. W., and Balleine, B. W. (2011). Molecular substrates of action control in cortico-striatal circuits. Prog. Neurobiol. 95, 1–13. doi: 10.1016/j.pneurobio.2011.05.007
Simpson, J., and Kelly, J. P. (2011). The impact of environmental enrichment in laboratory rats—Behavioural and neurochemical aspects. Behav. Brain Res. 222, 246–264. doi: 10.1016/j.bbr.2011.04.002
Sinclair, D., Purves-Tyson, T. D., Allen, K. M., and Weickert, C. S. (2014). Impacts of stress and sex hormones on dopamine neurotransmission in the adolescent brain. Psychopharmacology 231, 1581–1599. doi: 10.1007/s00213-013-3415-z
Skibicka, K. P. (2013). The central GLP-1: implications for food and drug reward. Front. Neurosci. 7:181. doi: 10.3389/fnins.2013.00181
Soto, P. L., Grandy, D. K., Hursh, S. R., and Katz, J. L. (2011). Behavioral economics of food reinforcement and the effects of prefeeding, extinction and eticlopride in dopamine D2 receptor mutant mice. Psychopharmacology 215, 775–784. doi: 10.1007/s00213-011-2173-z
South, T., and Huang, X.-F. (2008). High-fat diet exposure increases dopamine D2 receptor and decreases dopamine transporter receptor binding density in the nucleus accumbens and caudate putamen of mice. Neurochem. Res. 33, 598–605. doi: 10.1007/s11064-007-9483-x
Speed, N., Saunders, C., Davis, A. R., Owens, W. A., Matthies, H. J. G., Saadat, S., et al. (2011). Impaired striatal Akt signaling disrupts dopamine homeostasis and increases feeding. PLoS One 6:e25169. doi: 10.1371/journal.pone.0025169
Stairs, D. J., and Bardo, M. T. (2009). Neurobehavioral effects of environmental enrichment and drug abuse vulnerability. Pharmacol. Biochem. Behav. 92, 377–382. doi: 10.1016/j.pbb.2009.01.016
Stephens, D. W., and Krebs, J. W. (1986). Foraging Theory. Princeton, NJ: Princeton University Press.
Stouffer, M. A., Woods, C. A., Patel, J. C., Lee, C. R., Witkovsky, P., Bao, L., et al. (2015). Insulin enhances striatal dopamine release by activating cholinergic interneurons and thereby signals reward. Nat. Commun. 6:8543. doi: 10.1038/ncomms9543
Stuber, G. D., Roitman, M. F., Phillips, P. E. M., Carelli, R. M., and Wightman, R. M. (2005). Rapid dopamine signaling in the nucleus accumbens during contingent and noncontingent cocaine administration. Neuropsychopharmacology 30, 853–863. doi: 10.1038/sj.npp.1300619
Surmeier, D. J., Graves, S. M., and Shen, W. (2014). Dopaminergic modulation of striatal networks in health and Parkinson’s disease. Curr. Opin. Neurobiol. 29, 109–117. doi: 10.1016/j.conb.2014.07.008
Surmeier, D. J., Plotkin, J., and Shen, W. (2009). Dopamine and synaptic plasticity in dorsal striatal circuits controlling action selection. Curr. Opin. Neurobiol. 19, 621–628. doi: 10.1016/j.conb.2009.10.003
Sutton, R. S., and Barto, A. G. (1998). Introduction to Reinforcement Learning. Cambridge, MA: MIT Press.
Tanabe, L. M., Suto, N., Creekmore, E., Steinmiller, C. L., and Vezina, P. (2004). Blockade of D2 dopamine receptors in the VTA induces a long-lasting enhancement of the locomotor activating effects of amphetamine. Behav. Pharmacol. 15, 387–395. doi: 10.1097/00008877-200409000-00013
Tanimura, Y., Ogoegbunam, F. C., and Lewis, M. H. (2009). Amphetamine-induced sensitization and spontaneous stereotypy in deer mice. Pharmacol. Biochem. Behav. 92, 670–675. doi: 10.1016/j.pbb.2009.03.006
Tataranni, P. A., Baier, L., Jenkinson, C., Harper, I., Del Parigi, A., and Bogardus, C. (2001). A Ser311Cys mutation in the human dopamine receptor D2 gene is associated with reduced energy expenditure. Diabetes 50, 901–904. doi: 10.2337/diabetes.50.4.901
Thanos, P. K., Hamilton, J., O’Rourke, J. R., Napoli, A., Febo, M., Volkow, N. D., et al. (2016). Dopamine D2 gene expression interacts with environmental enrichment to impact lifespan and behavior. Oncotarget 7, 19111–19123. doi: 10.18632/oncotarget.8088
Thanos, P. K., Michaelides, M., Piyis, Y. K., Wang, G.-J., and Volkow, N. D. (2008). Food restriction markedly increases dopamine D2 receptor (D2R) in a rat model of obesity as assessed with in vivo μPET imaging ([11C] raclopride) and in vitro (3H spiperone) autoradiography. Synapse 62, 50–61. doi: 10.1002/syn.20468
Thiele, S. L., Chen, B., Lo, C., Gertler, T. S., Warre, R., Surmeier, J. D., et al. (2014). Selective loss of bi-directional synaptic plasticity in the direct and indirect striatal output pathways accompanies generation of parkinsonism and l-DOPA induced dyskinesia in mouse models. Neurobiol. Dis. 71, 334–344. doi: 10.1016/j.nbd.2014.08.006
Tirotta, E., Fontaine, V., Picetti, R., Lombardi, M., Samad, T. A., Oulad-Abdelghani, M., et al. (2008). Signaling by dopamine regulates D2 receptors trafficking at the membrane. Cell Cycle 7, 2241–2248. doi: 10.4161/cc.7.14.6307
Tobler, P. N., Fiorillo, C. D., and Schultz, W. (2005). Adaptive coding of reward value by dopamine neurons. Science 307, 1642–1645. doi: 10.1126/science.1105370
Tomer, R., Slagter, H. A., Christian, B. T., Fox, A. S., King, C. R., Murali, D., et al. (2013). Love to win or hate to lose? Asymmetry of dopamine D2 receptor binding predicts sensitivity to reward versus punishment. J. Cogn. Neurosci. 26, 1039–1048. doi: 10.1162/jocn_a_00544
Tomiyama, A. J., Mann, T., Vinas, D., Hunger, J. M., DeJager, J., and Taylor, S. E. (2010). Low calorie dieting increases cortisol. Psychosom. Med. 72, 357–364. doi: 10.1097/PSY.0b013e3181d9523c
Tran, A. H., Tamura, R., Uwano, T., Kobayashi, T., Katsuki, M., Matsumoto, G., et al. (2002). Altered accumbens neural response to prediction of reward associated with place in dopamine D2 receptor knockout mice. Proc. Natl. Acad. Sci. U S A 99, 8986–8991. doi: 10.1073/pnas.132284599
Tuominen, L., Tuulari, J., Karlsson, H., Hirvonen, J., Helin, S., Salminen, P., et al. (2015). Aberrant mesolimbic dopamine-opiate interaction in obesity. Neuroimage 122, 80–86. doi: 10.1016/j.neuroimage.2015.08.001
Turner, T. J. (2004). Nicotine enhancement of dopamine release by a calcium-dependent increase in the size of the readily releasable pool of synaptic vesicles. J. Neurosci. 24, 11328–11336. doi: 10.1523/JNEUROSCI.1559-04.2004
Tye, K. M., Mirzabekov, J. J., Warden, M. R., Ferenczi, E. A., Tsai, H.-C., Finkelstein, J., et al. (2012). Dopamine neurons modulate neural encoding and expression of depression-related behaviour. Nature 493, 537–541. doi: 10.1038/nature11740
Van Craenenbroeck, K., De Bosscher, K., Vanden Berghe, W., Vanhoenacker, P., and Haegeman, G. (2005). Role of glucocorticoids in dopamine-related neuropsychiatric disorders. Mol. Cell. Endocrinol. 245, 10–22. doi: 10.1016/j.mce.2005.10.007
van de Giessen, E., la Fleur, S. E., Eggels, L., de Bruin, K., van den Brink, W., and Booij, J. (2013). High fat/carbohydrate ratio but not total energy intake induces lower striatal dopamine D2/3 receptor availability in diet-induced obesity. Int. J. Obes. 37, 754–757. doi: 10.1038/ijo.2012.128
van Enkhuizen, J., Geyer, M. A., Halberstadt, A. L., Zhuang, X., and Young, J. W. (2014). Dopamine depletion attenuates some behavioral abnormalities in a hyperdopaminergic mouse model of bipolar disorder. J. Affect. Disord. 155, 247–254. doi: 10.1016/j.jad.2013.08.041
van Praag, H., Fleshner, M., Schwartz, M. W., and Mattson, M. P. (2014). Exercise, energy intake, glucose homeostasis, and the brain. J. Neurosci. 34, 15139–15149. doi: 10.1523/JNEUROSCI.2814-14.2014
van Zessen, R., van der Plasse, G., and Adan, R. A. H. (2012). Contribution of the mesolimbic dopamine system in mediating the effects of leptin and ghrelin on feeding. Proc. Nutr. Soc. 71, 435–445. doi: 10.1017/s0029665112000614
Vekshina, N. L., Anokhin, P. K., Veretinskaya, A. G., and Shamakina, I. Y. (2017). Dopamine D1–D2 receptor heterodimers: a literature review. Biochem. Mosc. Suppl. Ser. B Biomed. Chem. 11, 111–119. doi: 10.1134/S199075081702010X
Venton, B. J., Zhang, H., Garris, P. A., Phillips, P. E. M., Sulzer, D., and Wightman, R. M. (2003). Real-time decoding of dopamine concentration changes in the caudate-putamen during tonic and phasic firing: decoding dopamine neurotransmission. J. Neurochem. 87, 1284–1295. doi: 10.1046/j.1471-4159.2003.02109.x
Vidal-Gadea, A. G., and Pierce-Shimomura, J. T. (2012). Conserved role of dopamine in the modulation of behavior. Commun. Integr. Biol. 5, 440–447. doi: 10.4161/cib.20978
Volkow, N. D., Chang, L., Wang, G. J., Fowler, J. S., Ding, Y. S., Sedler, M., et al. (2001). Low level of brain dopamine D2 receptors in methamphetamine abusers: association with metabolism in the orbitofrontal cortex. Am. J. Psychiatry 158, 2015–2021. doi: 10.1176/appi.ajp.158.12.2015
Volkow, N. D., and Morales, M. (2015). The brain on drugs: from reward to addiction. Cell 162, 712–725. doi: 10.1016/j.cell.2015.07.046
Volkow, N. D., Wang, G.-J., Telang, F., Fowler, J. S., Thanos, P. K., Logan, J., et al. (2008). Low dopamine striatal D2 receptors are associated with prefrontal metabolism in obese subjects: possible contributing factors. Neuroimage 42, 1537–1543. doi: 10.1016/j.neuroimage.2008.06.002
Volkow, N. D., and Wise, R. A. (2005). How can drug addiction help us understand obesity? Nat. Neurosci. 8, 555–560. doi: 10.1038/nn1452
Vucetic, Z., and Reyes, T. M. (2010). Central dopaminergic circuitry controlling food intake and reward: implications for the regulation of obesity. Wiley Interdiscip. Rev. Syst. Biol. Med. 2, 577–593. doi: 10.1002/wsbm.77
Vučković, M. G., Li, Q., Fisher, B., Nacca, A., Leahy, R. M., Walsh, J. P., et al. (2010). Exercise elevates dopamine D2 receptor in a mouse model of Parkinson’s disease: in vivo imaging with [18F]fallypride. Mov. Disord. 25, 2777–2784. doi: 10.1002/mds.23407
Wei, X. J., Sun, B., Chen, K., Lv, B., Luo, X., and Yan, J. Q. (2015). Ghrelin signaling in the ventral tegmental area mediates both reward-based feeding and fasting-induced hyperphagia on high-fat diet. Neuroscience 300, 53–62. doi: 10.1016/j.neuroscience.2015.05.001
Weiss, E. P., Racette, S. B., Villareal, D. T., Fontana, L., Steger-May, K., Schechtman, K. B., et al. (2006). Improvements in glucose tolerance and insulin action induced by increasing energy expenditure or decreasing energy intake: a randomized controlled trial. Am. J. Clin. Nutr. 84, 1033–1042. doi: 10.1093/ajcn/84.5.1033
Wiecki, T. V., and Frank, M. J. (2010). “Neurocomputational models of motor and cognitive deficits in Parkinson’s disease,” in Progress in Brain Research, eds A. Bjorklund and M. A. Cenci (Amsterdam: Elsevier), 275–297.
Wiecki, T. V., Riedinger, K., von Ameln-Mayerhofer, A., Schmidt, W. J., and Frank, M. J. (2009). A neurocomputational account of catalepsy sensitization induced by D2 receptor blockade in rats: context dependency, extinction, and renewal. Psychopharmacology 204, 265–277. doi: 10.1007/s00213-008-1457-4
Wikenheiser, A. M., Stephens, D. W., and Redish, A. D. (2013). Subjective costs drive overly patient foraging strategies in rats on an intertemporal foraging task. Proc. Natl. Acad. Sci. U S A 110, 8308–8313. doi: 10.1073/pnas.1220738110
Wise, R. A. (2004). Dopamine, learning and motivation. Nat. Rev. Neurosci. 5, 483–494. doi: 10.1038/nrn1406
Wise, R. A. (2006). Role of brain dopamine in food reward and reinforcement. Philos. Trans. R. Soc. B Biol. Sci. 361, 1149–1158. doi: 10.1098/rstb.2006.1854
Woolverton, W. L., and Ranaldi, R. (2002). Comparison of the reinforcing efficacy of two dopamine D2-like receptor agonists in rhesus monkeys using a progressive-ratio schedule of reinforcement. Pharmacol. Biochem. Behav. 72, 803–809. doi: 10.1016/s0091-3057(02)00749-9
Würbel, H. (2001). Ideal homes? Housing effects on rodent brain and behaviour. Trends Neurosci. 24, 207–211. doi: 10.1016/s0166-2236(00)01718-5
Yadid, G., Overstreet, D. H., and Zangen, A. (2001). Limbic dopaminergic adaptation to a stressful stimulus in a rat model of depression. Brain Res. 896, 43–47. doi: 10.1016/s0006-8993(00)03248-0
Zakharova, E., Miller, J., Unterwald, E., Wade, D., and Izenwasser, S. (2009). Social and physical environment alter cocaine conditioned place preference and dopaminergic markers in adolescent male rats. Neuroscience 163, 890–897. doi: 10.1016/j.neuroscience.2009.06.068
Zhen, J., Reith, M. E. A., and Carr, K. D. (2006). Chronic food restriction and dopamine transporter function in rat striatum. Brain Res. 1082, 98–101. doi: 10.1016/j.brainres.2006.01.094
Zheng, D., Cabeza de Vaca, S., and Carr, K. D. (2012). Food restriction increases acquisition, persistence and drug prime-induced expression of a cocaine-conditioned place preference in rats. Pharmacol. Biochem. Behav. 100, 538–544. doi: 10.1016/j.pbb.2011.10.021
Zhu, J., Apparsundaram, S., Bardo, M. T., and Dwoskin, L. P. (2005). Environmental enrichment decreases cell surface expression of the dopamine transporter in rat medial prefrontal cortex: environmental enrichment regulates dopamine transporter. J. Neurochem. 93, 1434–1443. doi: 10.1111/j.1471-4159.2005.03130.x
Zhuang, X., Mazzoni, P., and Kang, U. J. (2013). The role of neuroplasticity in dopaminergic therapy for Parkinson disease. Nat. Rev. Neurol. 9, 248–256. doi: 10.1038/nrneurol.2013.57
Keywords: neuroeconomics, energy management, dopamine, basal ganglia, affordability, dopamine D2 receptor, striatum, behavioral thrift
Citation: Beeler JA and Mourra D (2018) To Do or Not to Do: Dopamine, Affordability and the Economics of Opportunity. Front. Integr. Neurosci. 12:6. doi: 10.3389/fnint.2018.00006
Received: 08 December 2017; Accepted: 26 January 2018;
Published: 13 February 2018.
Edited by:
Elizabeth B. Torres, Rutgers University, The State University of New Jersey, United StatesReviewed by:
Alexxai V. Kravitz, National Institutes of Health (NIH), United StatesCopyright © 2018 Beeler and Mourra. This is an open-access article distributed under the terms of the Creative Commons Attribution License (CC BY). The use, distribution or reproduction in other forums is permitted, provided the original author(s) and the copyright owner are credited and that the original publication in this journal is cited, in accordance with accepted academic practice. No use, distribution or reproduction is permitted which does not comply with these terms.
*Correspondence: Jeff A. Beeler, amJlZWxlckBxYy5jdW55LmVkdQ==
Disclaimer: All claims expressed in this article are solely those of the authors and do not necessarily represent those of their affiliated organizations, or those of the publisher, the editors and the reviewers. Any product that may be evaluated in this article or claim that may be made by its manufacturer is not guaranteed or endorsed by the publisher.
Research integrity at Frontiers
Learn more about the work of our research integrity team to safeguard the quality of each article we publish.