- 1Department of Neurology, The Second Hospital of Hebei Medical University, Shijiazhuang, Hebei, China
- 2The Key Laboratory of Neurology (Hebei Medical University), Ministry of Education, Shijiazhuang, Hebei, China
- 3Neurological Laboratory of Hebei Province, Shijiazhuang, Hebei, China
- 4Department of Emergency, The Second Hospital of Hebei Medical University, Shijiazhuang, Hebei, China
Introduction: Amyotrophic lateral sclerosis (ALS) is a rare, devastating neurodegenerative disease that affects upper and lower motor neurons, resulting in muscle atrophy, spasticity, hyperreflexia, and paralysis. Inflammation plays an important role in the development of ALS, and associated with rapid disease progression. Current observational studies indicate the thinning of cortical thickness in patients with ALS is associated with rapid disease progression and cognitive changes. However, the effects of inflammatory cytokines on cortical thickness in patients with ALS are unclear. Here, we investigated the relationship between inflammatory cytokines and cortical thickness in patients with ALS.
Methods: We evaluated 51 patients with ALS for inflammatory cytokines including interleukin (IL)-4, interferon (IFN)-α, IL-1β, IL-2, IL-5, IL-12, tumor necrosis factor (TNF)-α, IL-6, IL-10, IL-8, IL-17, and IFN-γ and analyzed the correlation between these indicators and the ALS functional rating scale-revised (ALSFRS-R) score or disease progression rate (ΔFS score). Twenty-six patients with ALS and 26 controls were studied using whole-cortex analysis, and post-hoc analyses were performed to examine the correlation between brain cortical thickness and ALSFRS-R or ΔFS scores.
Results: IL-4, IFN-α, IL-1β, and IL-2 levels were significantly correlated with ALSFRS-R scores, and the IL-2 level was significantly correlated with ΔFS scores. After controlling for age and sex, the ALS group had thinner cortexes in multiple clusters across the brain than the control group. Further analyses revealed that cortical thickness in the right superior temporal and lingual gyrus regions was inversely correlated with ΔFS scores. There was a significant positive correlation between the clusters in the right lingual cortex and IL-2 level.
Conclusion: These results suggest cortical thickness was reduced in patients with ALS in motor and non-motor cortical areas. Inflammatory factors (especially IL-2) were correlated with cortical thickness, and both were related to the disease progression rate, suggesting IL-2 plays an important role in ALS.
1 Introduction
Amyotrophic lateral sclerosis (ALS) is a chronic, adult-onset, progressive, and severe neurodegenerative disease that mainly affects motor neurons (MNs), resulting in muscle atrophy, spasticity, hyperreflexia, and paralysis, with a median survival of 3–5 years (Qureshi et al., 2009). Research has indicated that the pathophysiology of ALS includes oxidative stress, glutamate excitotoxicity, protein homeostasis, defects in RNA processing, impaired axonal transport, and mitochondrial dysfunction (Brown and Al-Chalabi, 2017), but the exact pathogenesis remains unknown. Although Riluzole (Bensimon et al., 1994) and Edaravone (Witzel et al., 2022) have been approved by the U.S. Food and Drug Administration, there is currently no cure or effective treatment for the disease.
Inflammation was previously thought to be the result of protein aggregation in the CNS, but there is growing evidence that immune signaling actually causes the aggregates to form in the earliest stages of the disease process (Zhang et al., 2023). So, inflammation including CNS and peripheral inflammation plays an important role in the development of ALS. Microglia and astroglia have been used as therapeutic targets for patients with ALS (Lall and Baloh, 2017; Dols-Icardo et al., 2020; Migliarini et al., 2021; Yamanaka and Komine, 2018; Izrael et al., 2020). And peripheral immune abnormalities exist in these patients with ALS (Yu et al., 2022; McCombe et al., 2020; Lyon et al., 2019; Beers and Appel, 2019; Yang et al., 2023) and modifying peripheral immune could influence ALS progression (Chiot et al., 2020; Garofalo et al., 2020). Furthermore, a number of studies (Moreau et al., 2005; Sun et al., 2021; Du et al., 2020; Ono et al., 2001) have shown that there is a correlation between serum inflammatory factors and disease severity. In addition, inflammatory cytokines from the periphery can travel to the brain via humoral, neural, and cellular pathways and can affect the function of neurons (Dantzer et al., 2000; Walker et al., 2023).
As the brain imaging technology develops, it is increasingly being used to study ALS (Dadar et al., 2020; Hsueh et al., 2023; Johannes et al., 2013; Li et al., 2023). Cerebral cortex thickness, the distance between the cortical surface and white matter, can be quantified using brain imaging and is a common measure of structural brain changes and a sensitive measure of cortical atrophy (Ambikairajah et al., 2014; Schuster et al., 2014). Several studies (Schuster et al., 2014; Tsermentseli et al., 2012) have found that the thinning of cortical thickness in patients with ALS is associated with rapid disease progression and cognitive changes, and cortical thinning correlated positively with serum neurofilament light chains (Tilsley et al., 2024). However, the association between inflammatory factors and cerebral cortex thickness in these patients is poorly understood.
We hypothesized that a reduction in cortical thickness in patients with ALS is associated with inflammatory factors. In this study, we investigated the correlation between peripheral inflammatory cytokines and cortical thickness in these patients. We also investigated the correlation between peripheral inflammatory cytokines and the rate of ALS progression. Therefore, our results not only further support the role of inflammation in ALS disease progression but also suggest that inflammation participates in the change in cortical thickness, providing new evidence for the use of anti-inflammatory therapy in ALS. Furthermore, the combination of inflammatory factors and cortical thickness may serve as an indicator of ALS disease progression and as a measure of the effectiveness of drugs.
2 Materials and methods
2.1 Subjects
All patients with ALS in this study were recruited from the Department of Neurology, Second Hospital of Hebei Medical University, between January 2021 and October 2023. Healthy controls were recruited from the Physical Examination Department during the study period. Data including sex, age, height, weight, detailed medical history, and physical, laboratory, and electrophysiological examinations were collected. All patients met the revised diagnostic criteria for ALS (Brooks et al., 2000). The ALS functional rating scale-revised (ALSFRS-R) was applied to assess the severity of the disease (Cedarbaum et al., 1999), and the rate of disease progression was evaluated using the ALSFRS-R score-to-progression rate ratio (ΔFS) according to the following formula: (48-ALSFRS-R score at the time of diagnosis)/(time from onset to diagnosis) (Labra et al., 2016; Westeneng et al., 2018). Patients diagnosed with ALS were included in this study, and the exclusion criteria were: (1) age < 45 years; (2) possible ALS; (3) familial ALS; (4) participants with a ventilator or tracheotomy or combination of these; (5) acute or chronic inflammatory diseases such as acute pneumonia or rheumatoid arthritis; (6) difficulty changing posture in bed; (7) other neurological diseases; (8) claustrophobia; and (9) patient or family members not in agreement. Healthy controls matched by sex and age were recruited, and the exclusion criteria were: (1) age < 45 years; (2) any neurological diseases; (3) refused laboratory tests; (4) any acute or chronic inflammatory diseases; and (5) claustrophobia. The study was approved by the Ethical Committee of the Second Hospital of Hebei Medical University (2023-R248). The enrolled patients underwent a detailed evaluation according to the study protocol shown in Supplementary Figure S1.
2.2 Laboratory analyses
Venous blood was drawn from the elbows of the participants in the morning after an overnight fast, and the samples were processed in the laboratory immediately after collection. The cytokine levels were measured and analyzed using a Multiplex Cytokine Assay Kit (XII) (AtomLife, Nanjing, China) according to the manufacturer's instructions. All blood indicators, including IL-5, IL-4, IL-12p70, interferon (IFN)-α, IFN-γ, TNF-α, IL-2, IL-6, IL-1β, IL-10, IL-8, and IL-17, were supplied by the laboratory division of the Second Hospital of Hebei Medical University.
2.3 Magnetic resonance imaging acquisition and analysis
All subjects underwent a T1-weighted MRI using a 3-Tesla scanner (Philips Achieva, Best, The Netherlands) with a 8-channel head coil. Three-dimensional T1-weighted images were obtained using a gradient echo sequence with the following parameters: repetition time/echo time, 10.2/4.8 ms; matrix size, 256 × 256 mm; flip angle, 8 degrees; and isotropic voxel size, 1 m3. The T1-weighted data were processed using FreeSurfer 7·2 (http://surfer.nmr.mgh.harvard.edu) with the standard recon-all pipeline. The cortex of the gray matter was parcellated and aligned into 68 distinct regions of interest (ROIs) within the Desikan–Killiany atlas (Desikan et al., 2006). The resulting scans were visually inspected for tissue misclassifications. Manual corrections were performed whenever necessary. The resulting cortical maps were smoothed with a Gaussian kernel of 10 mm full-width half-maximum.
2.4 Statistical analysis
Statistical analyses and graphing were performed using GraphPad Prism 9 (GraphPad software) and SPSS26. Continuous variables with normal distributions are presented as means ± standard deviations and with non-normal distributions are presented as medians with interquartile ranges. For normally distributed data, an unpaired t-test was used to analyze between-group differences, while Pearson's correlation coefficient was used to analyze correlations. For non-normally distributed data, the Mann–Whitney U-test was used for difference analysis, and Spearman's rank correlation coefficient was used for correlation analysis.
We used a general linear model (GLM), controlling for the effects of age and sex on cortical thickness at each vertex across the entire brain, to examine differences in cortical thickness between patients with ALS and healthy controls; for bidirectional effects, a Monte Carlo simulation corrected cluster threshold of p < 0.05 was considered to indicate significance (Greve and Fischl, 2018; Hagler et al., 2006). Following identification of statistically significant analyses, post-hoc vertex-wise analyses were performed to determine whether the region in the significant findings correlated with inflammatory cytokines. A GLM was created to examine the correlation between ALSFRS-R and ΔFS scores (cortical thickness in a hemisphere-specific vertex-wise analysis), and a Monte Carlo simulation corrected cluster threshold of p < 0.05 was considered to indicate significance for bidirectional effects. Statistical significance was set at p < 0.05.
3 Results
3.1 Demographic parameters of healthy controls and patients with ALS
We screened 119 patients with ALS and 60 healthy controls, of which 51 patients with ALS were included in the correlation analysis between inflammatory factors and the disease progression rate. The healthy control group consisted 13 females and 13 males with a mean age of 56.0 ± 10.8 and a mean education year of 9. The ALS group consisted 24 females and 27 males with a mean age of 59.1 ± 8.7 and a mean education year of 9. Finally, 26 patients with ALS and 26 healthy controls were analyzed (Figure 1). The ALS and healthy controls groups were matched by age, sex, and years of education (Table 1).
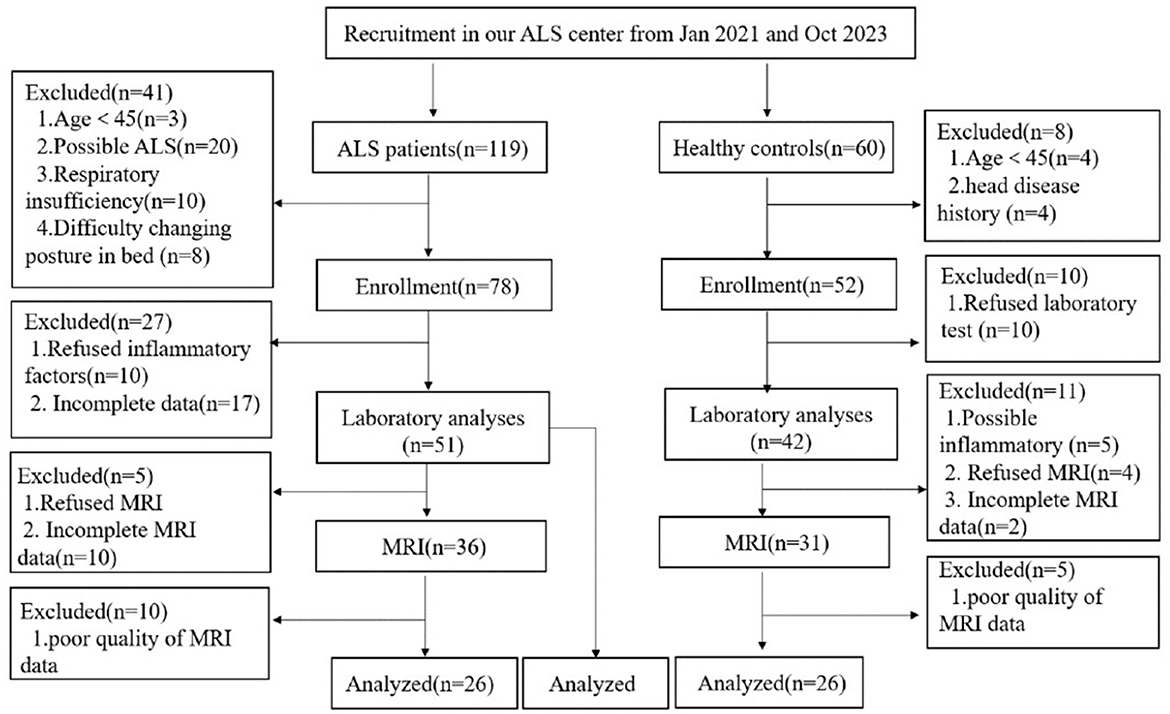
Figure 1. Study flowchart: screening protocol for healthy controls and patients with ALS. ALS, amyotrophic lateral sclerosis; MRI, magnetic resonance imaging.
3.2 Correlation between inflammatory factors and disease progression rate
Fifty-one patients with ALS were enrolled to analyze the correlation between inflammatory factors and disease progression rate. We observed that the levels of IL-4, IFN-α, IL-1β, and IL-2 were significantly positively correlated with ALSFRS-R scores (p = 0.0384, p = 0.0424, p = 0.0461, and p = 0.0469, respectively), and there was no correlation between the levels of IL-5, IL-12, TNF-α, IL-6, IL-10, IL-8, IL-17, or IFN-γ and ALSFRS-R scores (p = 0.2787, p = 0.5775, p = 0.2753, p = 0.7043, p = 0.4942, p = 0.8496, p = 0.1673, and p = 0.0788, respectively) (Figure 2A; Supplementary Figure S2A). The IL-2 level was significantly negatively related to the disease progression rate (p = 0.0411), but we observed no correlation between IL-4, IFN-α, IL-5, IL-12, TNF-α, IL-6, IL-1β, IL-10, IL-8, IL-17, or IFN-γ and the disease progression rate (p = 0.1142, p = 0.1021, p = 0.8689, p = 0.3616, p = 0.3588, p = 0.7754, p = 0.2742, p = 0.9703, p = 0.8454, p = 0.8496, and p = 0.6572, respectively) (Figure 2B; Supplementary Figure S2B).
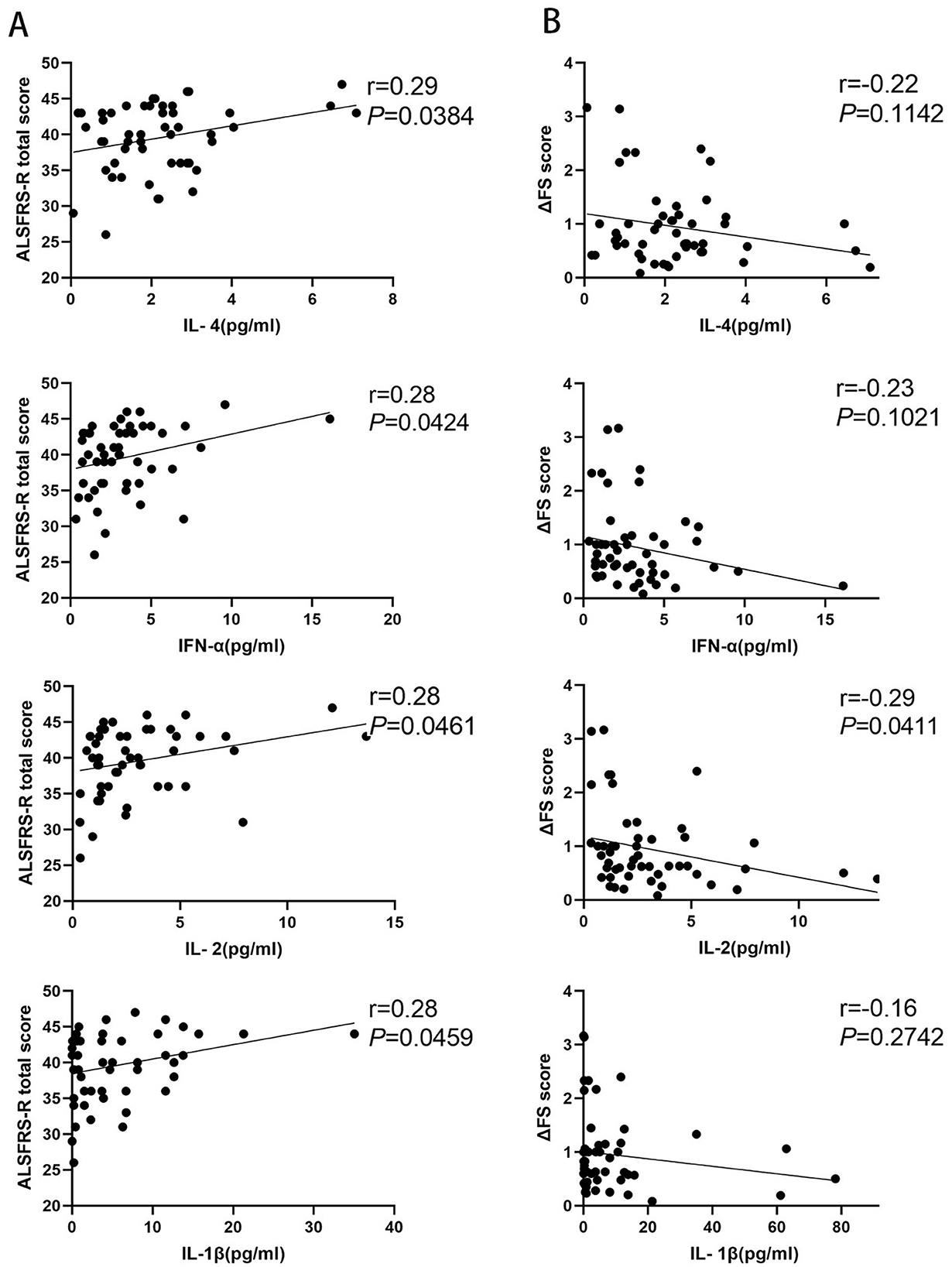
Figure 2. Correlation between inflammatory factors and disease progression rate in patients with amyotrophic lateral sclerosis (ALS). (A) The levels of IL-4, IFN-α, IL-1β, and IL-2 in patients with ALS correlated with the amyotrophic lateral sclerosis functional rating scale-revised (ALSFRS-R) scores. (B) The levels of IL-4, IFN-α, IL-1β, and IL-2 in patients with ALS correlated with the disease progression rate (ΔFS) scores.
3.3 Comparison of cortical thickness between patients with ALS and healthy controls
Twenty-six patients with ALS (55.6 ± 14.4 years of age, 14 men) and 26 matched healthy controls (56.0 ± 10.8 years of age, 13 men) were examined in this study. Compared with the healthy control group, the ALS group had thin cortexes in multiple clusters across the brain after controlling for age and sex. The results are summarized in Table 2 and Figure 3. In the left hemisphere, these clusters were in the precentral (area, 4,684.52 mm2; p = 0.01), medial orbitofrontal (area, 1,486.4 mm2; p = 0.01), cuneus (area, 1,065.32 mm2; p = 0.01), superior parietal (area, 472.37 mm2; p = 0.01), caudal middle frontal (area, 401.34 mm2; p = 0.01), inferior temporal (area 325.6 mm2; p = 0.01), interior parietal (area, 265.72 mm2; p = 0.01), and insular (area, 259.39 mm2; p = 0.01) regions. In the right hemisphere, the clusters were in the precentral (area, 6,290.67 mm2; p = 0.02), inferior parietal (area, 2,537.78 mm2; p = 0.02), lateral orbitofrontal (area, 393.17 mm2; p = 0.02), insular (area, 345.15 mm2; p = 0.02), lateral occipital (area, 308.15 mm2; p = 0.01), lingual (area, 291.93 mm2; p = 0.01), banks of the superior temporal sulcus (bankssts; area, 239.36 mm2; p = 0.04), and medial orbitofrontal (area, 231 mm2; p = 0.04) regions.
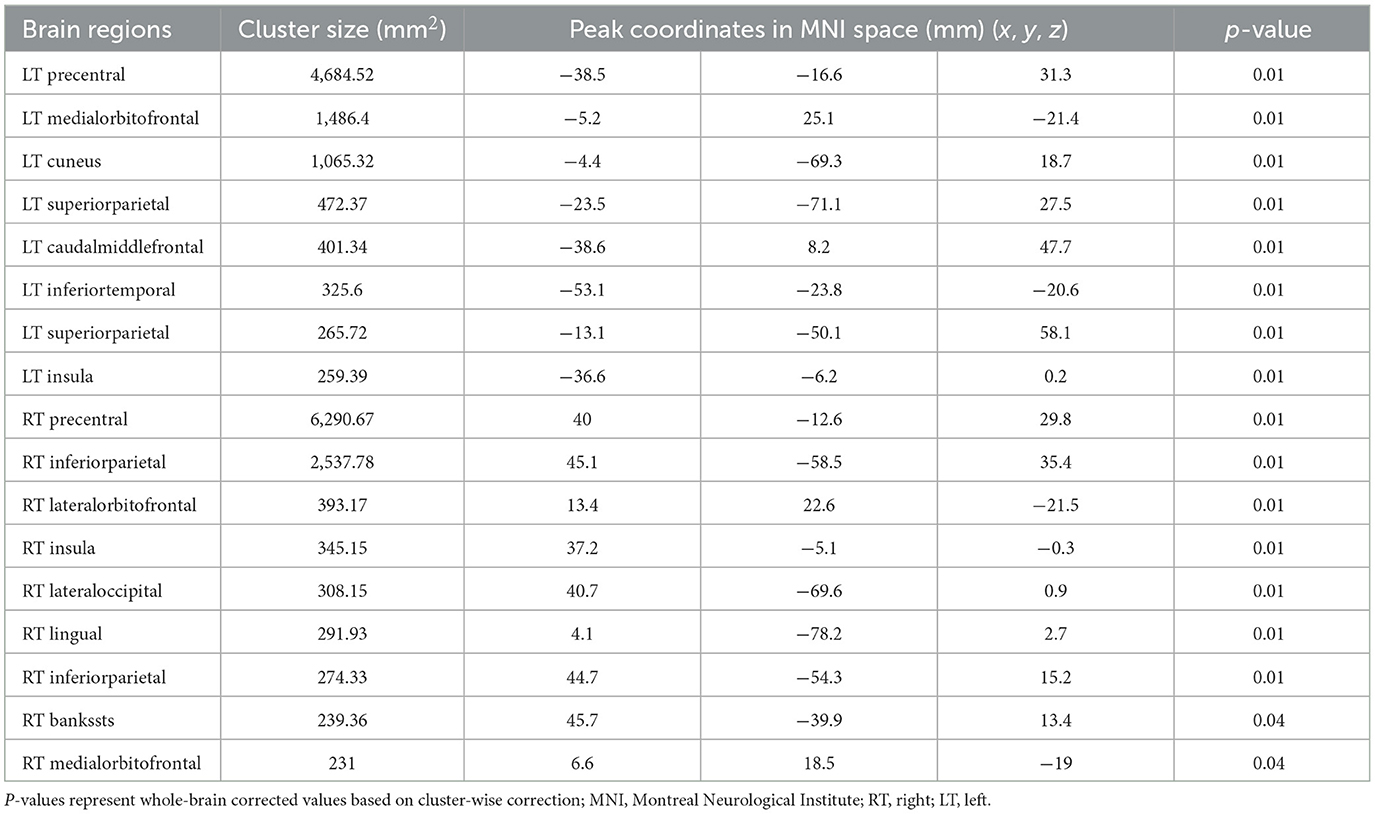
Table 2. Significant clusters exhibiting reduced cortical thickness in patients with ALS compared with healthy controls.
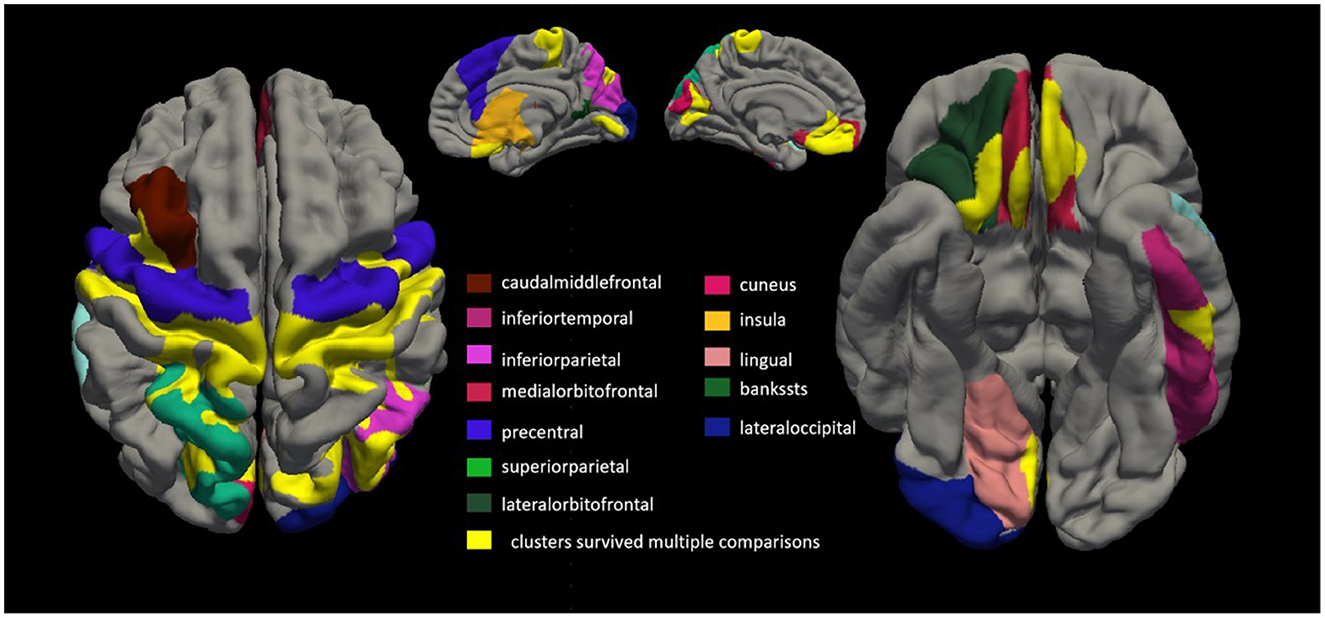
Figure 3. Comparing cortical thickness of patients with amyotrophic lateral sclerosis (ALS) with that of healthy controls. We conducted a vertex-by-vertex general linear model (GLM) analysis, controlling for age and sex. Clusters that survived multiple comparisons using the Monte Carlo simulation (10,000 permutations) are displayed on the cortical surface templates in yellow. In the left hemisphere, these clusters were in the precentral (area, 4,684.52 mm2; p = 0.01), medial orbitofrontal (area, 1,486.4 mm2; p = 0.01), cuneus (area, 1,065.32 mm2; p = 0.01), superior parietal (area 472.37 mm2; p = 0.01), caudal middle frontal (area, 401.34 mm2; p = 0.01), inferior temporal (area, 325.6 mm2; p = 0.01), superior parietal (area, 265.72 mm2; p = 0.01), and insular (area, 259.39 mm2; p = 0.01) regions. In the right hemisphere, the clusters were in the precentral (area, 6,290.67 mm2; p = 0.02), inferior parietal (area, 2,537.78 mm2; p = 0.02), lateral orbitofrontal (area, 393.17 mm2; p = 0.02), insular (area, 345.15 mm2; p = 0.02), lateral occipital (area, 308.15 mm2; p = 0.01), lingual (area, 291.93 mm2; p = 0.01), banks of the superior temporal sulcus (bankssts; area, 239.36 mm2; p = 0.04), and medial orbitofrontal (area, 231 mm2; p = 0.04) regions.
3.4 Analyses of correlation between cortical thickness and disease progression rate
We conducted correlation analyses to examine the association of cortical thickness with ΔFS. Significant clusters exhibited an inverse correlation between cortical thickness and ΔFS scores (Table 3; Figure 4). In the right hemisphere, these clusters were in the superior temporal (area, 333.61 mm2; p = 0.01) and lingual (area, 214.73 mm2; p = 0.04) regions. There were no other significant associations between ΔFS scores and cortical thickness in the left hemisphere and no significant associations between ALSFRS-R scores and cortical thickness across the whole brain.

Table 3. Significant clusters exhibiting cortical thickness correlates with ΔFS in patients with ALS.
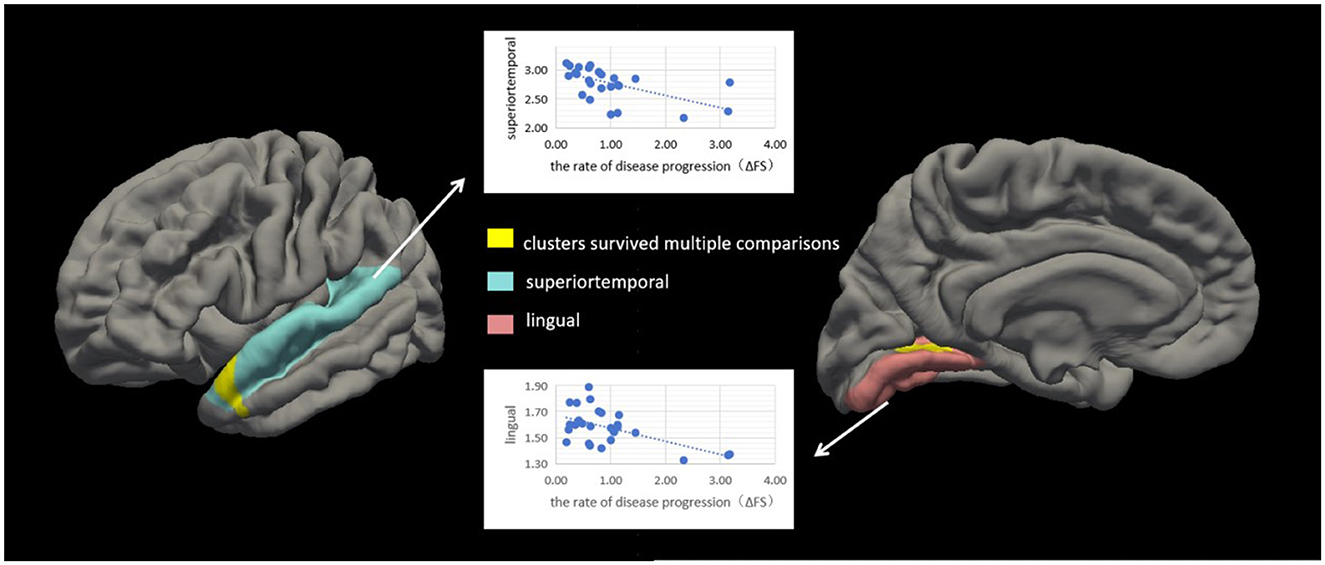
Figure 4. Correlation between cortical thickness and ΔFS scores in patients with amyotrophic lateral sclerosis (ALS). Significant clusters (yellow) exhibiting cortical thickness in the left hemisphere were negatively correlated with ΔFS scores in patients with ALS. In the left hemisphere, these clusters were in the superior temporal (area, 333.61 mm2; p = 0.01) and lingual (area, 214.73 mm2; p = 0.04) regions. There were no other significant associations between ΔFS scores and cortical thickness in the right hemisphere.
3.5 Analyses of correlation between cortical thickness and inflammatory cytokine levels
Accumulating evidence suggests that inflammation, including that in the CNS and peripheral immune system, contributes to the progression of ALS in both humans and mouse models (McCombe et al., 2020; Beers and Appel, 2019; Lu et al., 2016; Alexianu et al., 2001). This raises the question of whether inflammatory cytokine levels are related to cortical thickness.
Based on the whole-cortex analysis results, regions were selected from the Desikan–Killiany atlas to serve as ROIs for the constrained exploratory post-hoc analyses. The purpose of this exploratory post-hoc ROI analysis was to identify significant associations between the ROIs (thinner cortex in patients with ALS) and IL-2 levels to test the hypothesis that IL-2 is protective in patients with ALS. Based on the whole-cortex analysis results (Table 2), the following ROIs were selected: the left and right precentral, left and right insular, left and right medial orbitofrontal, left cuneus, left superior parietal, left caudal middle frontal, left inferior temporal, right inferior parietal, right lateral orbitofrontal, right lateral occipital, right lingual, and right banksst regions. A positive two-tailed cluster of 200.28 mm2 (p = 0.05) (Table 4; Figure 5) was found for the cortical thickness of the ROIs in the right hemisphere and IL-2 level after correction for multiple comparisons and controlling for age and sex.

Table 4. Significant clusters exhibiting cortical thickness correlates with IL-2 in patients with ALS.
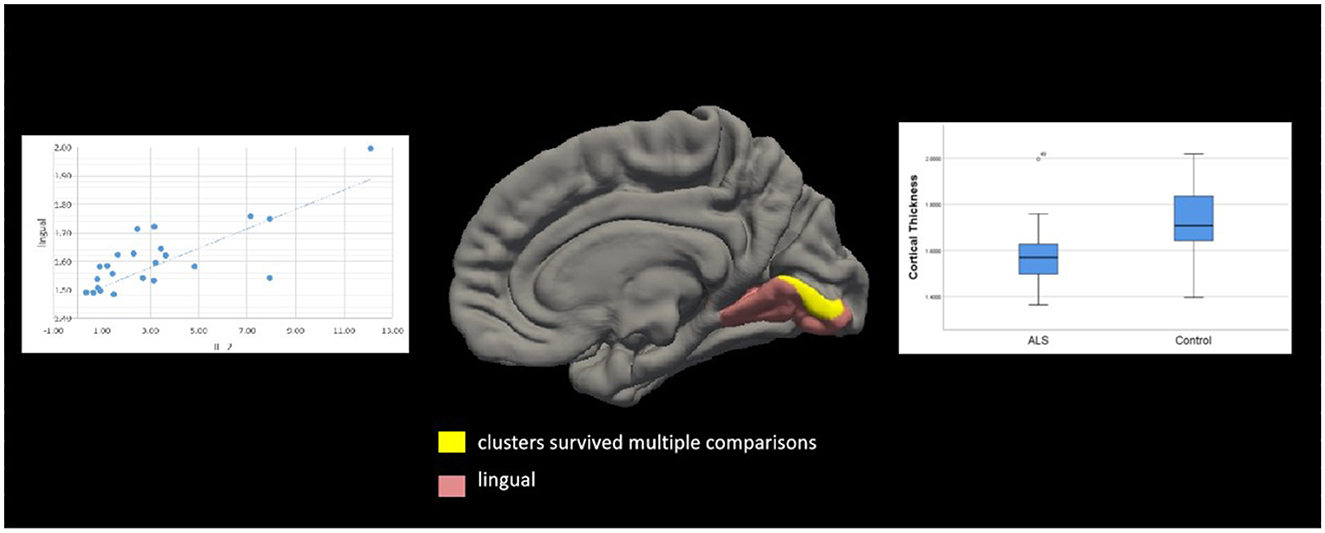
Figure 5. Correlation between cortical thickness and IL-2 level in patients with amyotrophic lateral sclerosis (ALS). Significant clusters (yellow) exhibiting cortical thickness in the right hemisphere were positively correlated with IL-2 levels in patients with ALS after controlling for age and sex. These clusters were in the left lingual region (area, 200.28 mm2; p = 0.03). Box and whisker plots show the median, quartiles, and range of the significant clusters for lingual cortical thickness in 26 healthy controls and 26 patients with ALS. There were no other significant associations between the IL-2 level and cortical thickness in the left hemisphere.
4 Discussion
Increasing evidence suggests that patients with ALS have chronic inflammation (Moreau et al., 2005; Sun et al., 2021; Pronto-Laborinho et al., 2019; Lunetta et al., 2017). It has previously been shown that these patients have elevated levels of certain cytokines, such as IL-2, IL-6, IL-10, IL-1β, IL-8, TNF-α, and IFN-γ, compared with controls (Lu et al., 2016; Tortelli et al., 2020; Hu et al., 2017). In this study, the healthy controls included were recruited from the Physical Examination Department during the study period, and in screening the healthy controls we excluded people with other neurological diseases and any acute or chronic inflammatory diseases, so we considered the cytokine levels in the peripheral blood of the controls to be very low. And we focused on the correlation between the cytokine levels and ALS disease and did not compare the cytokine levels between ALS patients and healthy controls, so we did not test the cytokine levels in the peripheral blood of healthy controls. Several studies (Moreau et al., 2005; Sun et al., 2021; Du et al., 2020; Ono et al., 2001) have also shown that elevated serum IL-6 was associated with disease severity in patients with ALS. IL-2 and IL-6 levels have also been proposed as biomarkers for ALS severity (Sun et al., 2021). In contrast to the results of previous studies, in addition to IL-2 being associated with disease severity, we found that IL-4, IFN-γ, and IL-1β were significantly correlated with disease severity in these patients. These results further support the hypothesis that patients with ALS exhibit inflammatory responses. Our analyses revealed that the IL-2 level was significantly and negatively correlated with the rate of disease progression in ALS. However, although the significant p-values in our results indicate that there is a correlation between peripheral inflammatory cytokines and the disease progression rate, the lower r indicate that the correlation between the two is weak, which may be due to the small number of samples we collected or the different detection methods. Unfortunately, we did not perform continuous monitoring of inflammatory factors in the peripheral blood of patients with ALS, so the result we obtained was only a possible correlation between inflammatory factors and disease severity or the disease progression rate. Our results provide further support for the involvement of peripheral immune cells in the pathogenesis of ALS. Previous studies have shown that Treg levels are reduced in patients with ALS and are correlated with disease severity and that decreased Treg levels may be a predictor of disease progression and poor survival (Beers et al., 2017). IL-2 is a cytokine required for the production, activation, and survival of Tregs (Pol et al., 2020), suggesting it may be a potential therapeutic drug. Previous studies have shown that low-dose IL-2 is well-tolerated and immunologically effective in patients with ALS (Camu et al., 2020), and Low-dose IL-2 promotes regulatory T-cell expansion and enhances the anti-inflammatory effects of regulatory T cells (Giovannelli et al., 2021). These findings support the possible role of inflammation in the mechanism and progression of the disease and confirm that inflammatory cytokines are elevated in patients with ALS. However, larger sample sizes and additional mechanistic studies are required to investigate the exact role of inflammation in ALS.
Consistent with the results of previous studies (Roccatagliata et al., 2009; Machts et al., 2018; Loewe et al., 2017; Kocar et al., 2021), we found that, compared with the healthy control group, the ALS group had thin cortexes in multiple clusters across the brain after controlling for age and sex; this included the precentral, medial orbitofrontal, cuneus, superior parietal, caudal middle frontal, inferior temporal, and insular regions in the left hemisphere and the precentral, inferior parietal, lateral orbitofrontal, insular, lateral occipital, lingual, bankssts, and medial orbitofrontal regions in the right hemisphere, suggesting that patients with ALS may have structural cortical changes and further substantiating extra motor involvement in ALS (Johannes et al., 2013). We found that cortical thickness in the superior temporal and lingual gyrus regions in the right hemisphere was inversely correlated with ΔFS scores in patients with ALS. However, there were no other significant associations between ΔFS scores and cortical thickness in the left hemisphere and no significant associations between ALSFRS-R scores and cortical thickness across the whole brain. It remains unclear whether changes in the cortical structure are a primary phenomenon or occur secondary to ALS. Many other studies showed that cortical thinning in the bilateral precentral gyrus, right precuneus, and right frontal and temporal lobes, and which was associated with cognitive status (Schuster et al., 2014). In this study, we not only observed the thinning of several cerebral cortical thicknesses in patients with ALS, but also explored the relationship between this altered cortical thickness and inflammatory factors.
We chose a thinner cortex for exploratory post-hoc ROI analysis and found that a positive cluster (area, 200.28 mm2; p = 0.05) in the right lingual cortex was correlated with the IL-2 level. We found that significant clusters located in the right superior temporal (area, 333.61 mm2; p = 0.01) and right lingual (area, 214.73 mm2; p = 0.04) regions were inversely correlated with ΔFS scores in patients with ALS. Atrophy in the lingual region in patients with ALS should attract our attention and our results suggest that the administration of IL-2 to patients with ALS may not only modulate Tregs but also may improve the cortical thickness. In subsequent studies, we will add scales and test indicators related to speech screening to assess patients' speech and language functions.
This study has some limitations. First, the sample size was relatively small, which may have led to the lower r. This means that our data may not be fully representative, which may have affected the generalizability of the results. Second, this study did not provide a detailed analysis of the relationship between peripheral inflammatory cytokines and cortical thickness, nor did it rule out the influence of possible confounding factors or explore the potential mechanisms. Third, there is a lack of information regarding semantic deficits in patients with ALS, resulting in the inability to analyse the lingual gyrus using MRI. Therefore, a larger sample size is required to reliably determine the correlation between peripheral inflammatory cytokine levels and cortical thickness in patients with ALS. In subsequent experiments, we will follow patients longitudinally to assess the long-term effects of inflammatory cytokines on the prognosis of these patients, as well as changes in inflammatory cytokines over time. We will also perform cellular and animal experiments to explore the possible mechanisms and identify potential targets.
Alterations in the immune system occur in ALS and may contribute to its pathological features. Our data demonstrated a relationship between peripheral inflammatory cytokines and cortical thickness in patients with ALS, further emphasizing the contribution of peripheral inflammatory cytokines to physiological processes. The IL-2 level was significantly and negatively correlated with the rate of disease progression in patients with ALS and positively correlated with cortical thickness in the right lingual region, suggesting that IL-2 is protective in these patients and that IL-2 biologics may be used in the treatment of ALS. These findings provide additional evidence for the involvement of peripheral immune cells in ALS and provide new insights for peripheral immunotherapy in ALS, which we will further verify in a follow-up study.
Data availability statement
The raw data supporting the conclusions of this article will be made available by the authors, without undue reservation.
Ethics statement
The studies involving humans were approved by the Ethical Committee of the Second Hospital of Hebei Medical University (2023-R248). The studies were conducted in accordance with the local legislation and institutional requirements. The participants provided their written informed consent to participate in this study.
Author contributions
JY: Conceptualization, Data curation, Formal analysis, Funding acquisition, Investigation, Methodology, Project administration, Writing – original draft, Resources, Software, Supervision. WL: Conceptualization, Data curation, Formal analysis, Methodology, Software, Writing – original draft. MT: Investigation, Methodology, Software, Writing – original draft. LZ: Conceptualization, Formal analysis, Investigation, Methodology, Software, Writing – original draft. FD: Data curation, Methodology, Supervision, Writing – original draft. XL: Data curation, Methodology, Software, Writing – original draft. QL: Data curation, Software, Supervision, Validation, Writing – original draft. RL: Methodology, Software, Supervision, Writing – original draft. ZL: Project administration, Resources, Software, Validation, Visualization, Writing – review & editing. HD: Methodology, Project administration, Supervision, Validation, Visualization, Writing – review & editing. YL: Conceptualization, Project administration, Resources, Supervision, Validation, Visualization, Writing – review & editing.
Funding
The author(s) declare financial support was received for the research, authorship, and/or publication of this article. This work was supported by the Health Innovation Special Project of Hebei Province 22377709D and the Health Commission of Hebei Province 20240205. The funders of the study had no role in the study design, data collection, data analysis, data interpretation, or writing of the report.
Conflict of interest
The authors declare that the research was conducted in the absence of any commercial or financial relationships that could be construed as a potential conflict of interest.
Generative AI statement
The author(s) declare that no Gen AI was used in the creation of this manuscript.
Publisher's note
All claims expressed in this article are solely those of the authors and do not necessarily represent those of their affiliated organizations, or those of the publisher, the editors and the reviewers. Any product that may be evaluated in this article, or claim that may be made by its manufacturer, is not guaranteed or endorsed by the publisher.
Supplementary material
The Supplementary Material for this article can be found online at: https://www.frontiersin.org/articles/10.3389/fnins.2024.1514554/full#supplementary-material
References
Alexianu, M. E., Kozovska, M., and Appel, S. H. (2001). Immune reactivity in a mouse model of familial ALS correlates with disease progression. Neurology 57, 1282–1289. doi: 10.1212/WNL.57.7.1282
Ambikairajah, A., Devenney, E., Flanagan, E., Yew, B., Mioshi, E., Kiernan, M. C., et al. (2014). A visual MRI atrophy rating scale for the amyotrophic lateral sclerosis-frontotemporal dementia continuum. Amyotr. Later. Scler. Frontotemp. Degener. 15, 226–234. doi: 10.3109/21678421.2014.880180
Beers, D. R., and Appel, S. H. (2019). Immune dysregulation in amyotrophic lateral sclerosis: mechanisms and emerging therapies. Lancet Neurol. 18, 211–220. doi: 10.1016/S1474-4422(18)30394-6
Beers, D. R., Zhao, W., Wang, J., Zhang, X., Wen, S., Neal, D., et al. (2017). ALS patients' regulatory T lymphocytes are dysfunctional, and correlate with disease progression rate and severity. JCI Insight 2:89530. doi: 10.1172/jci.insight.89530
Bensimon, G., Lacomblez, L., and Meininger, V. (1994). ALS/Riluzole study group. A controlled trial of riluzole in amyotrophic lateral sclerosis. N. Engl. J. Med. 330, 585–591. doi: 10.1056/NEJM199403033300901
Brooks, B. R., Miller, R. G., Swash, M., Munsat, T. L., and World Federation of Neurology Research Group on Motor Neuron Disease. (2000). El Escorial revisited: revised criteria for the diagnosis of amyotrophic lateral sclerosis. Amyotroph. Lateral Scler. Other Motor Neuron Disord. 1, 293–299. doi: 10.1080/146608200300079536
Brown, R. H., and Al-Chalabi, A. (2017). Amyotrophic lateral sclerosis. N. Engl. J. Med. 377, 162–172. doi: 10.1056/NEJMra1603471
Camu, W., Mickunas, M., Veyrune, J.-L., Payan, C., Garlanda, C., Locati, M., et al. (2020). Repeated 5-day cycles of low dose aldesleukin in amyotrophic lateral sclerosis (IMODALS): a phase 2a randomised, double-blind, placebo-controlled trial. eBioMedicine 59:102844. doi: 10.1016/j.ebiom.2020.102844
Cedarbaum, J. M., Stambler, N., Malta, E., Fuller, C., Hilt, D., Thurmond, B., et al. (1999). The ALSFRS-R: a revised ALS functional rating scale that incorporates assessments of respiratory function. BDNF ALS Study Group (Phase III). J. Neurol. Sci. 169, 13–21. doi: 10.1016/S0022-510X(99)00210-5
Chiot, A., Jolly, A., Iltis, C., Ribon, M., Berriat, F., Schiaffino, L., et al. (2020). Modifying macrophages at the periphery has the capacity to change microglial reactivity, to extend ALS survival. Nat. Neurosci. 23, 1339–1351. doi: 10.1038/s41593-020-00718-z
Dadar, M., Manera, A. L., Zinman, L., Korngut, L., Genge, A., Graham, S. J., et al. (2020). Cerebral atrophy in amyotrophic lateral sclerosis parallels the pathological distribution of TDP43. Brain Commun. 2:fcaa061. doi: 10.1093/braincomms/fcaa061
Dantzer, R., Konsman, J. P., Bluth,é, R. M., and Kelley, K. W. (2000). Neural and humoral pathways of communication from the immune system to the brain: parallel or convergent? Auton. Neurosci. 85, 60–65. doi: 10.1016/S1566-0702(00)00220-4
Desikan, R. S., Ségonne, F., Fischl, B., Quinn, B. T., Dickerson, B. C., Blacker, D., et al. (2006). An automated labeling system for subdividing the human cerebral cortex on MRI scans into gyral based regions of interest. Neuroimage 31, 968–980. doi: 10.1016/j.neuroimage.2006.01.021
Dols-Icardo, O., Montal, V., Sirisi, S., Lopez-Pernas, G., Cervera-Carles, L., Querol-Vilaseca, M., et al. (2020). Motor cortex transcriptome reveals microglial key events in amyotrophic lateral sclerosis. Neurol. Neuroimmunol. Neuroinflamm. 7:e829. doi: 10.1212/NXI.0000000000000829
Du, Y., Zhao, W., Thonhoff, J. R., Wang, J., Wen, S., Appel, S. H., et al. (2020). Increased activation ability of monocytes from ALS patients. Exp. Neurol. 328:113259. doi: 10.1016/j.expneurol.2020.113259
Garofalo, S., Cocozza, G., Porzia, A., Inghilleri, M., Raspa, M., Scavizzi, F., et al. (2020). Natural killer cells modulate motor neuron-immune cell cross talk in models of amyotrophic lateral sclerosis. Nat. Commun. 11:1773. doi: 10.1038/s41467-020-15644-8
Giovannelli, I., Bayatti, N., Brown, A., Wang, D., Mickunas, M., Camu, W., et al. (2021). Amyotrophic lateral sclerosis transcriptomics reveals immunological effects of low-dose interleukin-2. Brain Commun. 3:fcab141. doi: 10.1093/braincomms/fcab141
Greve, D. N., and Fischl, B. (2018). False positive rates in surface-based anatomical analysis. Neuroimage 171, 6–14. doi: 10.1016/j.neuroimage.2017.12.072
Hagler, D. J., Saygin, A. P., and Sereno, M. I. (2006). Smoothing and cluster thresholding for cortical surface-based group analysis of fMRI data. Neuroimage 33, 1093–1103. doi: 10.1016/j.neuroimage.2006.07.036
Hsueh, S. J., Chao, C. C., Chen, T. F., Chen, Y. F., Hsueh, H. W., Tsai, L. K., et al. (2023). Brain imaging signatures in amyotrophic lateral sclerosis: correlation with peripheral motor degeneration. Ann. Clin. Transl. Neurol. 10, 1456–1466. doi: 10.1002/acn3.51835
Hu, Y., Cao, C., Qin, X. Y., Yu, Y., Yuan, J., Zhao, Y., et al. (2017). Increased peripheral blood inflammatory cytokine levels in amyotrophic lateral sclerosis: a meta-analysis study. Sci. Rep. 7:9094. doi: 10.1038/s41598-017-09097-1
Izrael, M., Slutsky, S. G., and Revel, M. (2020). Rising stars: astrocytes as a therapeutic target for ALS disease. Front. Neurosci. 14:824. doi: 10.3389/fnins.2020.00824
Johannes, T., Jansma, H., Peschel, T., Grosskreutz, J., Mohammadi, B., Dengler, R., et al. (2013). Extent of cortical involvement in amyotrophic lateral sclerosis – an analysis based on cortical thickness. BMC Neurol. 13:148. doi: 10.1186/1471-2377-13-148
Kocar, T. D., Müller, H.-P., Ludolph, A. C., and Kassubek, J. (2021). Feature selection from magnetic resonance imaging data in ALS: a systematic review. Ther. Adv. Chronic Dis. 12:20406223211051002. doi: 10.1177/20406223211051002
Labra, J., Menon, P., Byth, K., Morrison, S., and Vucic, S. (2016). Rate of disease progression: a prognostic biomarker in ALS. J. Neurol. Neurosurg. Psychiatr. 87, 628–632. doi: 10.1136/jnnp-2015-310998
Lall, D., and Baloh, R. H. (2017). Microglia and C9orf72 in neuroinflammation and ALS and frontotemporal dementia. J. Clin. Invest. 127, 3250–3258. doi: 10.1172/JCI90607
Li, X., Liu, Q., Niu, T., Liu, T., Xin, Z., Zhou, X., et al. (2023). Sleep disorders and white matter integrity in patients with sporadic amyotrophic lateral sclerosis. Sleep Med. 109, 170–180. doi: 10.1016/j.sleep.2023.07.003
Loewe, K., Machts, J., Kaufmann, J., Petri, S., Heinze, H.-J., Borgelt, C., et al. (2017). Widespread temporo-occipital lobe dysfunction in amyotrophic lateral sclerosis. Sci. Rep. 7:srep40252. doi: 10.1038/srep40252
Lu, C. H., Allen, K., Oei, F., Leoni, E., Kuhle, J., Tree, T., et al. (2016). Systemic inflammatory response and neuromuscular involvement in amyotrophic lateral sclerosis. Neurol. Neuroimmunol. Neuroinflamm. 3:e244. doi: 10.1212/NXI.0000000000000244
Lunetta, C., Lizio, A., Maestri, E., Sansone, V. A., Mora, G., Miller, R. G., et al. (2017). Serum C-reactive protein as a prognostic biomarker in amyotrophic lateral sclerosis. JAMA Neurol. 74, 660–667. doi: 10.1001/jamaneurol.2016.6179
Lyon, M. S., Wosiski-Kuhn, M., Gillespie, R., Caress, J., and Milligan, C. (2019). Inflammation, immunity, and amyotrophic lateral sclerosis: I. Etiol. Pathol. 59, 10–22. doi: 10.1002/mus.26289
Machts, J., Cardenas-Blanco, A., Acosta-Cabronero, J., Kaufmann, J., Loewe, K., Kasper, E., et al. (2018). Prefrontal cortical thickness in motor neuron disease. NeuroImage Clin. 18, 648–655. doi: 10.1016/j.nicl.2018.03.002
McCombe, P. A., Lee, J. D., Woodruff, T. M., and Henderson, R. D. (2020). The peripheral immune system and amyotrophic lateral sclerosis. Front. Neurol. 11:279. doi: 10.3389/fneur.2020.00279
Migliarini, S., Scaricamazza, S., Valle, C., Ferri, A., Pasqualetti, M., Ferraro, E., et al. (2021). Microglia morphological changes in the motor cortex of hSOD1(G93A) transgenic ALS mice. Brain Sci. 11:807. doi: 10.3390/brainsci11060807
Moreau, C. D. D., Brunaud-Danel, V., Defebvre, L., Perez, T., Destée, A., Tonnel, A. B., et al. (2005). Elevated IL-6 and TNF-alpha levels in patients with ALS: inflammation or hypoxia? Neurology 65, 1958–1960. doi: 10.1212/01.wnl.0000188907.97339.76
Ono, S. H. J., Shimizu, N., Imai, T., and Nakagawa, H. (2001). (2001). Increased interleukin-6 of skin and serum in amyotrophic lateral sclerosis. J. Neurol. Sci. 187, 27–34. doi: 10.1016/S0022-510X(01)00514-7
Pol, J. G., Caudana, P., Paillet, J., Piaggio, E., and Kroemer, G. (2020). Effects of interleukin-2 in immunostimulation and immunosuppression. J. Exp. Med. 217:20191247. doi: 10.1084/jem.20191247
Pronto-Laborinho, A., Pinto, S., Gromicho, M., Pereira, M., Swash, M., de Carvalho, M., et al. (2019). Interleukin-6 and amyotrophic lateral sclerosis. J. Neurol. Sci. 398, 50–53. doi: 10.1016/j.jns.2019.01.026
Qureshi, M., Schoenfeld, D. A., Paliwal, Y., Shui, A., and Cudkowicz, M. E. (2009). The natural history of ALS is changing: improved survival. Amyotr. Lateral Scler. 10, 324–331. doi: 10.3109/17482960903009054
Roccatagliata, L., Bonzano, L., Mancardi, G., Canepa, C., and Caponnetto, C. (2009). Detection of motor cortex thinning and corticospinal tract involvement by quantitative MRI in amyotrophic lateral sclerosis. Amyotr. Later. Scler. 10, 47–52. doi: 10.1080/17482960802267530
Schuster, C., Kasper, E., Dyrba, M., Machts, J., Bittner, D., Kaufmann, J., et al. (2014). Cortical thinning and its relation to cognition in amyotrophic lateral sclerosis. Neurobiol. Aging 35, 240–246. doi: 10.1016/j.neurobiolaging.2013.07.020
Sun, Q., Huo, Y., Bai, J., Wang, H., Wang, H., Yang, F., et al. (2021). inflammatory cytokine levels in patients with sporadic amyotrophic lateral sclerosis. Neurodegener. Dis. 21, 87–92. doi: 10.1159/000522078
Tilsley, P., Moutiez, A., Brodovitch, A., Mendili, M. M. E., Testud, B., Zaaraoui, W., et al. (2024). Neurofilament light chain levels interact with neurodegenerative patterns and motor neuron dysfunction in amyotrophic lateral sclerosis. Am. J. Neuroradiol. 45, 494–503. doi: 10.3174/ajnr.A8154
Tortelli, R., Zecca, C., Piccininni, M., Benmahamed, S., Dell'Abate, M. T., Barulli, M. R., et al. (2020). Plasma inflammatory cytokines are elevated in ALS. Front. Neurol. 13:552295. doi: 10.3389/fneur.2020.552295
Tsermentseli, S., Leigh, P. N., and Goldstein, L. H. (2012). The anatomy of cognitive impairment in amyotrophic lateral sclerosis: more than frontal lobe dysfunction. Cortex 48, 166–182. doi: 10.1016/j.cortex.2011.02.004
Walker, K. A., Le Page, L. M., Terrando, N., Duggan, M. R., Heneka, M. T., Bettcher, B. M., et al. (2023). The role of peripheral inflammatory insults in Alzheimer's disease: a review and research roadmap. Mol. Neurodegener. 18:37. doi: 10.1186/s13024-023-00627-2
Westeneng, H.-J., Debray, T. P. A., Visser, A. E., van Eijk, R. P. A., Rooney, J. P. K., Calvo, A., et al. (2018). Prognosis for patients with amyotrophic lateral sclerosis: development and validation of a personalised prediction model. Lancet Neurol. 17, 423–433. doi: 10.1016/S1474-4422(18)30089-9
Witzel, S., Maier, A., Steinbach, R., Grosskreutz, J., Koch, J. C., Sarikidi, A., et al. (2022). Safety and effectiveness of long-term intravenous administration of edaravone for treatment of patients with amyotrophic lateral sclerosis. JAMA Neurol. 79, 121–130. doi: 10.1001/jamaneurol.2021.4893
Yamanaka, K., and Komine, O. (2018). The multi-dimensional roles of astrocytes in ALS. Neurosci. Res. 126, 31–38. doi: 10.1016/j.neures.2017.09.011
Yang, J., Liu, T., Zhang, L., Li, X., Du, F. P., Liu, Q., et al. (2023). Eosinophils at diagnosis are elevated in amyotrophic lateral sclerosis. Front. Neurol. 14:1289467. doi: 10.3389/fneur.2023.1289467
Yu, W., He, J., Cai, X., Yu, Z., Zou, Z., Fan, D., et al. (2022). Neuroimmune crosstalk between the peripheral and the central immune system in amyotrophic lateral sclerosis. Front. Aging Neurosci. 14:890958. doi: 10.3389/fnagi.2022.890958
Keywords: amyotrophic lateral sclerosis, cortical thickness, disease progression, inflammatory cytokines, MRI
Citation: Yang J, Li W, Tian M, Zhang L, Du F, Li X, Liu Q, Li R, Li Z, Dong H and Liu Y (2025) Cortical thickness correlated with peripheral inflammatory cytokines in amyotrophic lateral sclerosis. Front. Neurosci. 18:1514554. doi: 10.3389/fnins.2024.1514554
Received: 21 October 2024; Accepted: 16 December 2024;
Published: 07 January 2025.
Edited by:
Elena Niccolai, University of Florence, ItalyReviewed by:
Edith González Guevara, Neurofarmacología molecular y nanotecnología Instituto Nacional de Neurología y Neurocirugía MVS, MexicoZhangyu Zou, Fujian Medical University Union Hospital, China
Copyright © 2025 Yang, Li, Tian, Zhang, Du, Li, Liu, Li, Li, Dong and Liu. This is an open-access article distributed under the terms of the Creative Commons Attribution License (CC BY). The use, distribution or reproduction in other forums is permitted, provided the original author(s) and the copyright owner(s) are credited and that the original publication in this journal is cited, in accordance with accepted academic practice. No use, distribution or reproduction is permitted which does not comply with these terms.
*Correspondence: Yaling Liu, bGl1eWFsaW5nQGhiMmguY29t; Hui Dong, aHVpZG9uZ0BoZWJtdS5lZHUuY24=