Gradient-free training of recurrent neural networks using random perturbations
- Department of Machine Learning and Neural Computing, Donders Institute for Brain, Cognition and Behaviour, Radboud University, Nijmegen, Netherlands
A Corrigendum on
Gradient-free training of recurrent neural networks using random perturbations
by Fernández, J. G., Keemink, S., and van Gerven, M. (2024). Front. Neurosci. 18:1439155. doi: 10.3389/fnins.2024.1439155
In the published article, there was an error in Figures 5B, C as published.
The training and test sequences for this task were identical due to an issue with seed initialization in the dataset generation function. This occurred due to a mistake merging old and new code, which caused an unintentionally re-initialized seed every time a sequence was generated. Thankfully, the impact of this mistake is very small. The updated results show almost no change in overall performance. In fact, the slightly different results for this task now better align with those obtained in the other tasks (the Mackey-Glass time series and the real-world weather prediction task), further strengthening our claims about the robustness and generalization of our proposed approach (ANP).
The corrected Figures 5B, C and its caption appear below:
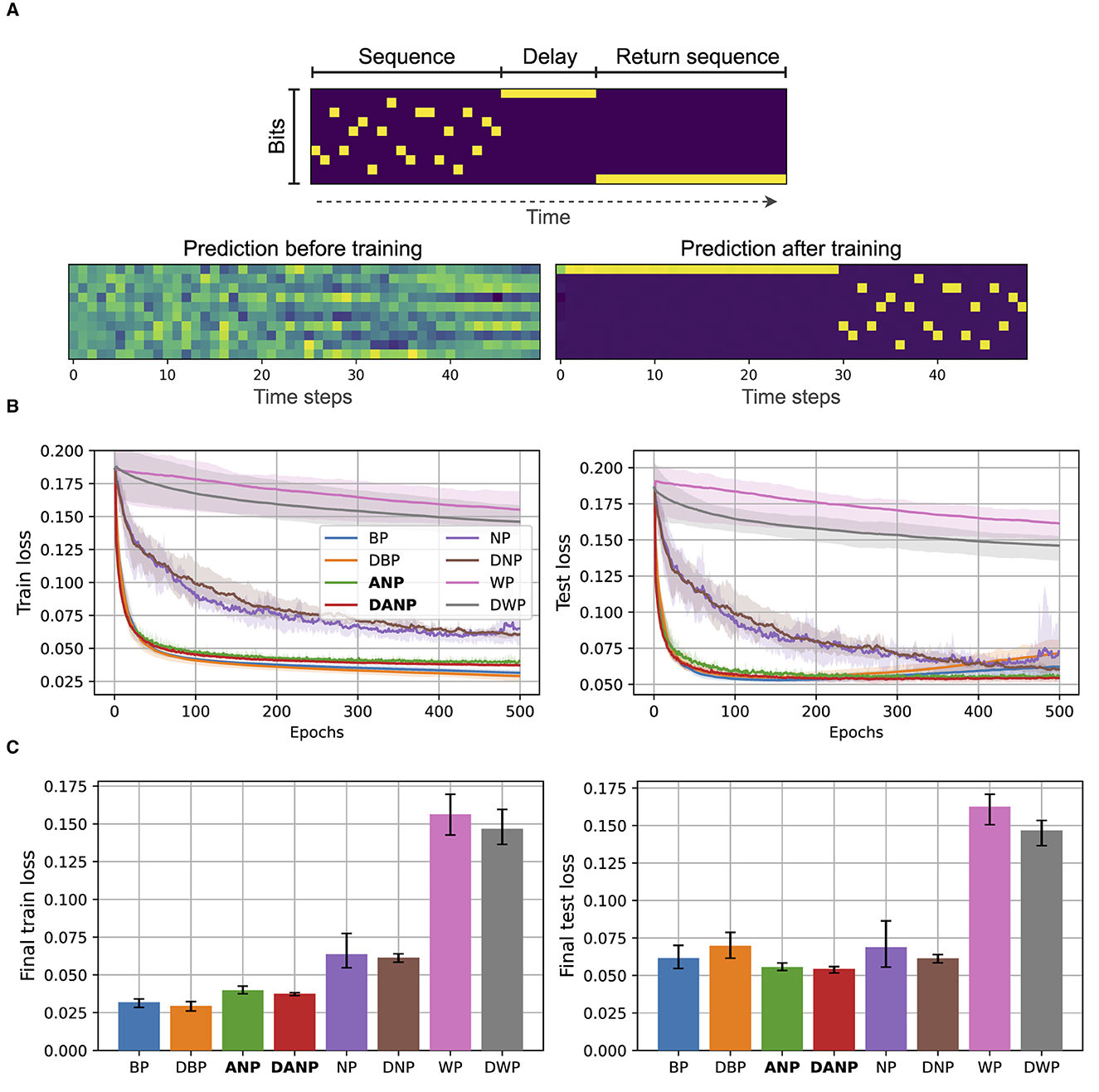
Figure 5. Copying memory data and results. (A) At the top, we depict an example of an input with annotations. The sequence length is 20 and the delay period is 10. At the bottom, we show the predictions of a BP-trained model before and after training. (B) Performance during training over the train and test set for the different methods. (C) Final performance for the different methods, computed as the mean performance over the last 50 epochs.
The authors apologize for this error and state that this does not change the scientific conclusions of the article in any way. The original article has been updated.
Publisher's note
All claims expressed in this article are solely those of the authors and do not necessarily represent those of their affiliated organizations, or those of the publisher, the editors and the reviewers. Any product that may be evaluated in this article, or claim that may be made by its manufacturer, is not guaranteed or endorsed by the publisher.
Keywords: recurrent neural network, artificial neural network, gradient approximation, BPTT, node perturbation learning
Citation: Fernández JG, Keemink S and van Gerven M (2024) Corrigendum: Gradient-free training of recurrent neural networks using random perturbations. Front. Neurosci. 18:1511916. doi: 10.3389/fnins.2024.1511916
Received: 15 October 2024; Accepted: 25 October 2024;
Published: 05 November 2024.
Edited and reviewed by: Amirreza Yousefzadeh, University of Twente, Netherlands
Copyright © 2024 Fernández, Keemink and van Gerven. This is an open-access article distributed under the terms of the Creative Commons Attribution License (CC BY). The use, distribution or reproduction in other forums is permitted, provided the original author(s) and the copyright owner(s) are credited and that the original publication in this journal is cited, in accordance with accepted academic practice. No use, distribution or reproduction is permitted which does not comply with these terms.
*Correspondence: Jesús García Fernández, amVzdXMuZ2FyY2lhZmVybmFuZGV6QGRvbmRlcnMucnUubmw=