- 1National Clinical Research Center for Mental Disorders & National Center for Mental Disorders, Beijing Key Laboratory of Mental Disorders, Beijing Anding Hospital, Capital Medical University, Beijing, China
- 2Advanced Innovation Center for Human Brain Protection, Capital Medical University, Beijing, China
- 3The second Affiliated Hospital of Xinjiang Medical University, Urumqi, China
- 4Faculty of Information Technology, Beijing University of Technology, Beijing, China
- 5School of Mathematical Sciences, East China Normal University, Shanghai, China
Bipolar disorder (BD) is a severe psychiatric disease with high rates of misdiagnosis and underdiagnosis, resulting in a significant disease burden on both individuals and society. Abnormal neural oscillations have garnered significant attention as potential neurobiological markers of BD. However, untangling the mechanisms that subserve these baseline alternations requires measurement of their electrophysiological underpinnings. This systematic review investigates consistent abnormal resting-state EEG power of BD and conducted an initial exploration into how methodological approaches might impact the study outcomes. This review was conducted in Pubmed-Medline and Web-of-Science in March 2024 to summarize the oscillation changes in resting-state EEG (rsEEG) of BD. We focusing on rsEEG to report spectral power in different frequency bands. We identified 10 studies, in which neural oscillations was compared with healthy individuals (HCs). We found that BD patients had abnormal oscillations in delta, theta, beta, and gamma bands, predominantly characterized by increased power, indicating potential widespread neural dysfunction, involving multiple neural networks and cognitive processes. However, the outcomes regarding alpha oscillation in BD were more heterogeneous, which is thought to be potentially influenced by the disease severity and the diversity of samples. Furthermore, we conducted an initial exploration into how demographic and methodological elements might impact the study outcomes, underlining the importance of implementing standardized data collection methods. Key aspects we took into account included gender, age, medication usage, medical history, the method of frequency band segmentation, and situation of eye open/eye close during the recordings. Therefore, in the face of abnormal multiple oscillations in BD, we need to adopt a comprehensive research approach, consider the multidimensional attributes of the disease and the heterogeneity of samples, and pay attention to the standardized experimental design to improve the reliability and reproducibility of the research results.
1 Introduction
Bipolar disorder (BD) is a prevalent clinical mood disorder affecting approximately 1% of the global population (McIntyre et al., 2020), around 0.6% of the population in China (Huang et al., 2019). BD is characterized by early onset, frequent recurrence, high morbidity and mortality, with suicide risk being particularly prominent, posing a significant challenge to public health (Nierenberg et al., 2023; Dev et al., 2024). Due to atypical symptoms during the early stages of BD and the variability of symptoms, misdiagnosis and underdiagnosis are common, delaying effective treatment increasing the burden on patients and society (Craddock and Sklar, 2013; Bauer et al., 2018). Therefore, the quest for specific biomarkers to enhance the diagnostic accuracy of BD has become an urgent need in current research, as this will aid in early identification, timely intervention, and improved patient outcomes.
Neural oscillations have gained extensive attention as a potential biological marker of BD (Howells et al., 2018; Lu et al., 2022; Bokhan et al., 2023). Oscillations are defined as the repetitive and rhythmic electrical activity that occurs spontaneously or in response to stimuli within the central nervous system (Cole and Voytek, 2017; Han et al., 2021, 2023b; Wang et al., 2023). They play key roles in brain information processing, memory formation and emotion regulation (Fitzgerald and Watson, 2018; Cao et al., 2022; Han et al., 2022; Han et al., 2023a). Previous studies have found that bipolar disorder patients exhibit disturbances in neural oscillations, suggesting a dysfunction in brain network operations (Narayanan et al., 2015; Lu et al., 2022; Andrews et al., 2023). This dysfunction is not only manifested by altered oscillation intensity within specific frequency ranges but also by disrupted synchrony of oscillations between different brain regions. On a microscopic scale, the fine balance between excitatory and inhibitory neurons is considered one of the key pathophysiological mechanisms in BD (Schaul, 1998; Sohal and Rubenstein, 2019). When an E/I imbalance occurs, neuronal spiking becomes aberrant, leading to abnormalities in the brain’s neural oscillation patterns.
Resting-state Electroencephalography (rsEEG) as a direct, non-invasive, and relatively inexpensive assessments measured the electrical field obtained from the summations at scalp electrodes of the oscillatory component generated by postsynaptic potentials in pyramidal cortical neurons (Biasiucci et al., 2019; Figure 1). The most common way to characterize rsEEG is by breaking down oscillatory signals into the spectral power of a frequency band (Figure 2). Power changes in specific frequency bands may be an indicator for an increased firing rate within certain cell populations reflecting different stages of cognitive arousal, cognitive processing, and psychopathology (Makeig et al., 2004).
Although there has been extensive recent research on the use of frequency bands in individuals with BD to investigate and understand changes in brain activity, there is no systematic review integrated the finding of BD and rsEEG. This is probably due to the heterogeneity of demographic and methodological elements. Therefore, the main purpose of our study was to find consistent abnormal resting-state oscillation patterns of BD thus providing a reliable foundation for improving early diagnosis and treatment strategies. Furthermore, we conducted an initial exploration into how methodological approaches might impact the study outcomes, underlining the importance of implementing standardized data collection methods.
2 Materials and methods
2.1 Search strategy and information source
We conducted a comprehensive search of English-language literature until March 2024 in PubMed and Web of Science, there was no limitation on publication date in search strategy. Following the Preferred Reported Items for Systematic Reviews and Meta-Analyses (PRISMA) 2021 guidelines (Page et al., 2021). We focused on samples using terms “bipolar disorder”. In terms of techniques, our interest encompassed “resting-state EEG,” “quantitative EEG,” “brain rhythms,” and “brain oscillations.” We used the Boolean expression “AND” to join the two terminologies. Moreover, the references of the selected articles were also examined to retrieve documents missed by the literature search.
2.2 Inclusion and exclusion criteria
The objectives of this paper and the inclusion criteria were structured based on the elements of the PICOS model (Population of interest, Interventions, Comparators, Outcomes, and Study design).
An article was included if rsEEG was assessed in a group of patients with BD. Only studies that investigated control group of health controls (HCs), either exclusively or in conjunction with other disease, were included in our review. The comparison of different states of BD was excluded. Studies employing alternative techniques, such as positron emission tomography (PET), magnetic resonance imaging (MRI), or magnetoencephalography (MEG), instead of EEG were excluded. We Use rsEEG to report spectral power in different frequency bands, studies exclusively focusing on other EEG metrics (e.g., asymmetry, coherence, functional connectivity, microstates, entropy etc.) were excluded. We only included empirical studies which written in English (Table 1).
2.3 Data extraction
Information from each included article was extracted and entered into tables, the extracted data included the following information: (1) authors and year of publication, (2) demographic characteristics (sample size, sex, age, disease and medication condition), (3) recording condition (eyes closed or eyes open), (4) measures of frequency bands and range (delta- δ, theta- θ, alpha- α, beta- β, gamma- γ), (5) spectral power type utilized, (6) main findings.
The selected articles and their data have been shown in the data extraction (Tables 3, 4). whereas the study outcomes were discussed in the results section. The analysis of the results has been generally explained in the discussion part.
2.4 Risk of bias assessment
The risk of bias in the included studies was assessed with a modified Newcastle Ottawa Scale. The case–control studies subscale was used for assessing the risk of bias. NOS provides three domains: (1) selection, (2) comparability and (3) exposure. The highest score is 9. A score from 9 to 7 indicates high quality, from 6 to 4 moderate quality, and from 3 to 0 low quality.
3 Results
3.1 Literature search and assessment of risk of bias
A total of 1096 articles were initially identified from Pub-Med and Web-of-Science databases using our search terms (Figure 3). 180 reviews, systematic reviews or meta-analyses were screened by automation tools, and 302 duplicate articles were removed. After reading the titles and abstracts, 586 articles were excluded. Upon further reading the full text, 18 articles were excluded, including 8 studies that did not address the outcomes of rsEEG spectral power changes. One study included only rsEEG frequency bands power ration (delta/alpha), instead of individual band power variations. Control group of 10 studies did not included HCs. Ultimately, 10 articles were included.
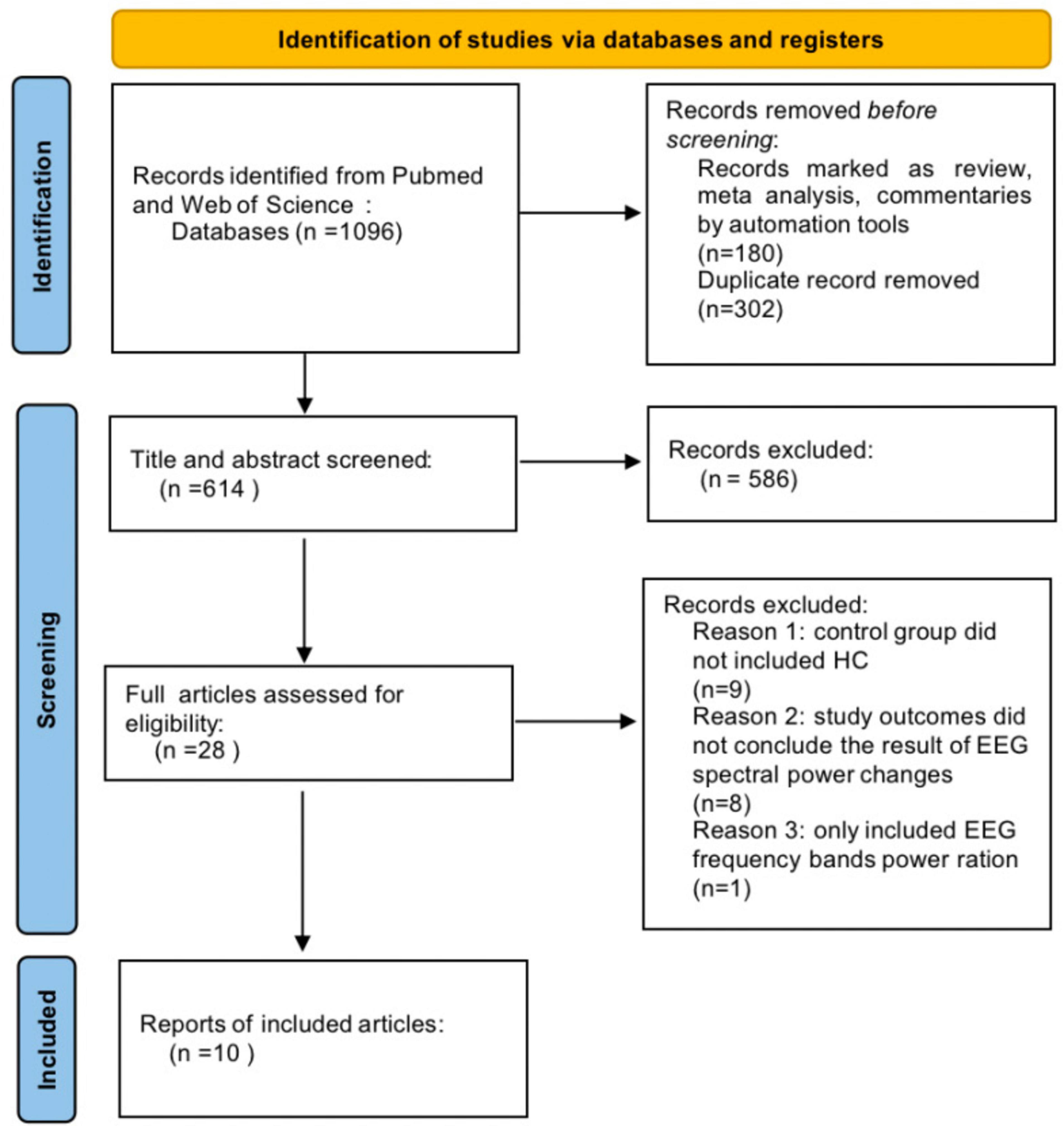
Figure 3. Preferred Reporting Items for Systematic Reviews and Meta-Analyses (PRISMA) flow diagram of study selection.
The mean score of quality assessment of the 10 studies was 5.6, indicating a moderate quality. Table 2 reports the score assigned to each article.
3.2 Character of the included studies
Table 3 show the demographic data and clinical characteristics of the 10 preferred studies. One articles only included women (Rommel et al., 2016), one article did not conclude sex information (Kano et al., 1992). Subjects of 2 studies were adolescents and young adults (El-Badri et al., 2001; Khaleghi et al., 2019). Two included articles control drug use (Başar et al., 2012; Khaleghi et al., 2019). In three studies, rsEEG was measured with eyes closed (Kano et al., 1992; Clementz et al., 1994; Arikan et al., 2019). However, three studies reported results for eyes open (Kam et al., 2013; Narayanan et al., 2015; Khaleghi et al., 2019), whereas four studies reported results for the eyes-closed and eyes-open conditions (El-Badri et al., 2001; Venables et al., 2009; Başar et al., 2012; Rommel et al., 2016).
Regarding frequency bands, of the 10 studies, 4 examined the conventional five frequency bands (delta, theta, alpha, beta, and gamma). Nevertheless, in 5 studies, the gamma band was excluded, 1 study only included alpha band (Başar et al., 2012). 4 studies split alpha or beta into sub-bands (e.g., alpha1/alpha2, beta1/beta2/beta3) (Kano et al., 1992; Clementz et al., 1994; Kam et al., 2013; Arikan et al., 2019). For each frequency band, power was mostly reported in terms of absolute spectral power, relative spectral power. For more details, see Table 4.
3.3 Reporting of frequency bands during resting state
3.3.1 Resting state delta spectral power
Table 4 shows the dominant results for frequency bands power for each study.
For Delta spectral power, 4 included studies indicated increased delta power compared to HCs (Clementz et al., 1994; El-Badri et al., 2001; Narayanan et al., 2015; Khaleghi et al., 2019). Among them, Khaleghi drew the conclusion that delta frequency band of BD depression state exhibited great power in F7, F3, Fz, T3 (Khaleghi et al., 2019), and Clementz pointed out that the promote area was in C3, C4 and Cz (Clementz et al., 1994). The other 2 studies did not indicate the specific location of elevation. Three articles did not identify any statistical differences between BD and HCs patients (Venables et al., 2009; Kam et al., 2013; Rommel et al., 2016).
3.3.2 Resting state theta spectral power
A comparison of HCs, six studies showed an increase in resting-state theta power (Clementz et al., 1994; El-Badri et al., 2001; Narayanan et al., 2015; Arikan et al., 2019; Khaleghi et al., 2019; Kim et al., 2020). In term of the elevated brain areas, Khaleghi pointed out the findings in F3 and T3 (Khaleghi et al., 2019) and Arikan indicated that the greatest power activities are in FP1, P3, P4, Pz, O1, F7, T3 and T4 electrodes (Arikan et al., 2019). In 2020, Kim concluded that the resting state theta power increased at the global level, with the majority of this increase occurring in the frontal lobe (Kim et al., 2020). Two studies did not report any changes in the groups in the theta frequency band (Venables et al., 2009; Kam et al., 2013).
3.3.3 Resting state alpha spectral power
The outcomes regarding alpha oscillation in BD were more heterogeneous, In comparison with HCs, 4 studies showed alpha band power increased (El-Badri et al., 2001; Narayanan et al., 2015; Arikan et al., 2019; Khaleghi et al., 2019), while 4 articles indicated decreased alpha activities (Kano et al., 1992; Clementz et al., 1994; Başar et al., 2012; Kam et al., 2013). In 1992 Kano firstly proposed that alpha power was decreased in F7 (Kano et al., 1992), then Clementz suggested BD C3, C4, Cz has lesser alpha activities than HCs (Clementz et al., 1994). In 2012, Basar concluded decreased alpha power in O1, Oz, O2 (Başar et al., 2012).
3.3.4 Resting state beta spectral power
In the comparison of BD to HCs, 5 studies showed an increase in beta band power (El-Badri et al., 2001; Kam et al., 2013; Narayanan et al., 2015; Arikan et al., 2019; Khaleghi et al., 2019). One study suggested that beta has greater power than HC in right temporal and left occipital particularly (El-Badri et al., 2001). Another study concluded: Beta power increased in FP1, FP2, C4, P3, O1 and F7. Beta 1 power increased in O1, and F7; Beta 2 power increased in O1 and F7; Beta 3 power increased in P3, O1 and F7 (Arikan et al., 2019).
3.3.5 Resting state gamma spectral power
In comparison between BD and HCs, 3 articles indicated increased gamma power (Kam et al., 2013; Arikan et al., 2019; Khaleghi et al., 2019). One included study indicated that gamma power increased in Fp1, F8, C3, T5, T6 and O1 (Khaleghi et al., 2019), another concluded that gamma power increased in C3, F7, with gamma 1 in C3, P3, F7, gamma 2 in Cz, P3, F7 and high gamma in C3, C4, F7 (Arikan et al., 2019).
4 Discussion
In this systematic review, our aim was to find consistent abnormal resting-state oscillation patterns of BD. Furthermore, we try to analyze the causes and mechanisms of discrepancies based on the results, while correlating them with the summarized demographic characteristics and experimental methods. We found that BD patients had abnormal oscillations in delta, theta, beta, and gamma bands, predominantly characterized by increased power, indicating potential widespread neural dysfunction, involving multiple neural networks and cognitive processes. However, the outcomes regarding alpha oscillation in BD were more heterogeneous, which is thought to be potentially influenced by the disease severity and the diversity of samples.
4.1 Delta oscillation
All these studies indicated an increase in the power of the delta frequency band in the studies included in this analysis, BD was compared with HCs. Delta oscillations may reflect homeostatic and metabolic processes. The predominance of low-frequency waves in consciousness is considered a pathological state, which is often associated with cognitive decline (Uhlhaas et al., 2008).
4.2 Theta oscillation
All of the included studies showed an increased frequency of theta waves in BD compared with HCs, and most of them indicated that they were located in the frontal region. Theta oscillations have been shown to index cognitive performance particularly in decision-making, attention focusing and learning (Klimesch, 1999; Nokia et al., 2012). Theta oscillations depend on a balance between excitatory Glx and inhibitory GABA neurons, with alternating E/I interactions being essential for the generation and maintenance of low-frequency theta rhythms (Nigbur et al., 2011). Moreover, reduced GABA levels and increased Glx levels may exist in BD patients, thereby disrupting the rhythm balance of theta oscillations but current conclusions are not yet uniform (Chase and Phillips, 2016). Theta wave oscillations play a crucial role in synaptic plasticity regulating, spatial information processing, and memory encoding within the cortical-hippocampal circuit (Buzsáki, 2002). Abnormal discharging of hippocampal cells is a common feature among patients with psychiatric disorders, providing a reliable neurophysiological model for cognitive deficits (Olypher et al., 2006). Synaptic and neural plasticity are crucial processes for brain function and development, playing an important role in mental illness (Toro and Deakin, 2007; Narayanan et al., 2015). Neurogenesis in the hippocampus is related to synaptic plasticity, memory, and learning. Abnormal hippocampal neurogenesis is closely linked to depression and other psychiatric disorders (Kempermann et al., 2008).
4.3 Alpha oscillation
The results of alpha activity are different. The power of alpha band is believed to be negatively correlated with cortical activity, concentration, and thalamus metabolism (Nusslock et al., 2012). The thalamus serves as the inlet for sensory transmission and plays a crucial role in concentration. The decrease in thalamus metabolism is correlated with an enhancement in alpha power (Lindgren et al., 1999). Therefore, it can be concluded that an increase in alpha activity is associated with attention deficits and thalamic metabolic disorder.
Moreover, increased alpha activity is associated with increased depression severity. BD patients with a high depression severity are characterized by decreased neuronal excitability. This abnormal neurophysiological pattern may affect the neurodevelopmental processes in adolescents with BD. Some researchers reported that the frequency bands of adolescents undergo changes. EEG is not as same as in adults during adolescent development (Khaleghi et al., 2019). Therefore, the different age groups of participants may be a potential reason for the controversy results. Moreover, some studies noticed that drug applications have effects on oscillation dynamics (Yener et al., 2007; Başar and Güntekin, 2008). Most of the studies we included did not control drug usage. The patient’s treatment and medication may also have impact on the results which should be taken into account.
Another potential reason for the difference in the experimental results is the inconsistency of subjects included in the experiment. One study included 11 subjects with hypomanic and 10 subjects with depression (Khaleghi et al., 2019). Another study included subjects are all in an euthymic state (El-Badri et al., 2001). Khaleghi indicated BD I have greater delta power than BD II (Khaleghi et al., 2015). BD II is distinguished from BD I primarily by the absence of full-blown manic episodes. A growing body of evidence suggests that there could be neurobiological differences between BD I and BD II patients, they presumed BD I has more deficits than BD II. These factors above may be the reason for the different results. Moreover, the EEG activity varies in different emotional states (Painold et al., 2014; Abé et al., 2016). The power of the alpha band was negatively correlated with the tension-calm (TC) score, that is, the power of the alpha exhibited higher power in the calm state than in the tension state (Wyczesany et al., 2010).
Significantly, some previous works have shown that alpha rhythms could be dissected into two components in scalp EEG, which might be related to different cognitive functions (Chiang et al., 2011; Knyazeva et al., 2018). Previous results have shown that the power in these different sub-bands (upper and lower alpha) also differed in resting state (Thorpe et al., 2016). Alpha power may be considered to inversely correlate with E/I balance, which display a negative correlation with cortical activation and metabolism (Conner et al., 2011; Podvalny et al., 2015). Thus, a signature of abnormally reduced alpha power would indicate a state of increased E/I, while the ‘high-alpha’ biotype would reflect low E/I (Bresnahan and Barry, 2002; Poil et al., 2014). In healthy subjects, alpha power increases or decreases have been found to reflect cortical inhibition or excitation, respectively (Haegens et al., 2011). This demonstrates the importance of clear band allocation.
Finally, it should be noted that during resting-state, some studies only recorded EO EEG (Kam et al., 2013; Narayanan et al., 2015; Khaleghi et al., 2019), while some studies only recorded EC EEG (Kano et al., 1992; Clementz et al., 1994; Arikan et al., 2019). According to some studies, the quantitative characteristics of the alpha rhythm can only be fully understood after one takes into account the spectral power and activation intensity (inhibition of the alpha rhythm after one opens their eyes, the Berger effect). One of the informative signs of the stability of the activation reaction is a decreased alpha power in response to the opening of the eyes (the Berger effect). This is associated with information processing (Kirschfeld, 2005).
4.4 Beta oscillation
High beta activity is associated with cortical excitability (Rangaswamy et al., 2002) and is generally considered to be facilitating (Moore et al., 2012). Some inferences can be drawn from literature reports indicating that an elevation of emotional tension is associated with an increased in beta power, particularly in the anterior region (Jacobs et al., 1996). Alpha activity is a fundamental rhythm in the brain. The increase in occipital beta power observed during manic episodes may serve as a compensatory mechanism for dysfunctional alpha responses (Wyczesany et al., 2010). Therefore, an increase in beta-band power may be accompanied by a decrease in alpha activity.
4.5 Gamma oscillation
All studies reviewed herein consistently demonstrate an increase in gamma activities in individuals with BD compared to HCs. In addition, both BD and SCZ manifest overlapping symptoms, including psychotic symptoms, disorganized thinking, and depressive symptoms (Calhoun et al., 2011; Bora and Pantelis, 2016). Researches indicate that patients diagnosed with BD and SCZ exhibit notable abnormalities in neural processes involving gamma oscillations, which are both characterized by increased gamma activities (Zhou et al., 2018; Honda et al., 2020). The occurrence of gamma oscillation is contingent upon synaptic GABA neurotransmission, which is crucial for coordinating neural network activities across different brain regions (Lu et al., 2022). Cortical gamma activity plays a crucial role in processes such as sensory perception, problem-solving, and memory. Moreover, in BD the overexpression of the neuronal calcium sensor (NCS-1) protein modulates gamma band oscillation in the pedunculopontine nucleus (PPN) in a concentration-dependent manner (Urbano et al., 2014). Consequently, it can be inferred that the increased expression of NCS-1 leads to greater gamma band activity in BD patients.
Cortical gamma oscillations are believed to be generated independently of external stimuli, facilitated by GABA interneurons engaged in mutual inhibition. These interneurons generate postsynaptic potentials that oscillate at approximately 40 Hz. Consequently, gamma oscillations are considered oscillations are considered indicative of the inhibition of cortical neurons.
5 Critical considerations in EEG band analysis for BD research
Although the articles included in this review present promising findings, it is essential to acknowledge certain limitations identified in the analysis of frequency bands carried out in each of the reviewed investigations. Addressing these limitations is crucial for the progression of EEG research and its effective application in the study of BD.
Firstly, some researchers indicated that there are significant gender differences in EEG power between genders, with females exhibiting higher power than males (Narayanan et al., 2014). Moreover, several studies indicated that blood glutamate levels of females vary across the menstrual cycle and are negatively associated with the levels of female sex hormones (Tseng et al., 2024). It is therefore possible that males and females have different states of E/I balance. The changes may have effects on band oscillation. This suggests that inconsistencies in the sex ratio of male and female in studies may have an impact on the results. While most studies showed that the results were not due to variable gender composition across groups. Although (Rommel et al., 2016; Bokhan et al., 2023) included only female, their outcomes limit the generalizability of findings in the BD patients.
Second, different experiments have defined the age range of patients differently (e.g., 12 to 18 years considered as adolescents (Khaleghi et al., 2019), and 18 to 40 years considered as young patients (El-Badri et al., 2001). A cross-sectional study on adolescents with BD indicated that the neural defects of BD may differ between adolescents and adults (Wegbreit et al., 2014). It remains unclear whether the pathology and mechanism of BD adolescents differ from those in adults. Additionally, epidemiological research suggests that there are multiple critical periods for the onset of BD, specifically in late adolescence, around the age of 20, and between 30 to 40 years (Bellivier et al., 2003). Therefore, it is imperative for further experimental designs to consider the range of age groups. 2 experiments have defined the age range of patients differently (e.g., 12 to 18 years considered as adolescents (Khaleghi et al., 2019), and 18 to 40 years considered as young patients (El-Badri et al., 2001).
Third, although many of our preferred studies suggested there is no correlation between medication and frequency bands oscillations and did not control for patients’ drug use, some scholars indicated that the EEG results can be affected by drugs in the resting-state (Spatz and Kugler, 1982; Struve, 1987; Schulz et al., 2000; de Meneses et al., 2022). For example, lithium usually increases slow wave activities (delta, theta) and decreases alpha activities. Some antidepressants (e.g., imipramine, viloxazine) may increase beta activities and simultaneously decrease alpha activities (Saletu, 1976; Bente, 1979). Amitriptyline tends to increase delta but decrease alpha activities (Peck et al., 1979). Studies on animal models have shown that mood stabilizers can exert behavioral effects by altering synaptic E/I balance (Tseng et al., 2024). Drugs used as mood stabilizers, including lithium, valproate and lamotrigine, have significant effects on the glutamatergic system (Sanacora et al., 2008).
6 Conclusion
Overall, the examined studies suggested that the electrophysiological features of BD probably could play a key role in diagnosis of this disease. In studying the characteristics of neural oscillations in BD patients, we observed abnormalities in multiple frequency bands such as delta, theta, beta, and gamma, the most notable feature of which was a general increase in oscillatory power. However, the results of studies of alpha oscillation show high heterogeneity, which may be due to the different stages and manifestations of symptoms of the disease, as well as the diversity of the samples themselves. Besides, we have preliminarily explored how the findings are influenced by demographic and methodological factors. By controlling for these variables, such as age, gender, stage of disease, comorbidities, and drugs used, researchers are better able to unravel the complexity of BD and reveal potential neurophysiological markers that inform future diagnostic and therapeutic strategies.
Data availability statement
Publicly available datasets were analyzed in this study. This data can be found here: https://pubmed.ncbi.nlm.nih.gov/?term=adult%20ADHD%20EEG&page=10.
Author contributions
XZ: Writing–original draft, Writing–review and editing, Conceptualization, Supervision, Validation, Visualization. ZS: Writing–original draft, Writing–review and editing. HZ: Writing–original draft, Writing–review and editing. YW: Writing–original draft. BC: Writing–original draft. ZZ: Writing–original draft. BW: Writing–original draft. JL: Writing–original draft. YS: Writing–original draft, Writing–review and editing.
Funding
The author(s) declare financial support was received for the research, authorship, and/or publication of the article. This work was funded by the Beijing Municipal Hospital Research and Development Project (PX2021068), the Advanced Innovation Center for Human Brain Protection Project (3500-12020137), the National Natural Science Foundation of China (82201701), and the Sci-Tech Innovation 2030–Major Project of Brain science and brain-inspired intelligence technology (2021ZD0200600).
Conflict of interest
The authors declare that the research was conducted in the absence of any commercial or financial relationships that could be construed as a potential conflict of interest.
Publisher’s note
All claims expressed in this article are solely those of the authors and do not necessarily represent those of their affiliated organizations, or those of the publisher, the editors and the reviewers. Any product that may be evaluated in this article, or claim that may be made by its manufacturer, is not guaranteed or endorsed by the publisher.
References
Abé, C., Ekman, C. J., Sellgren, C., Petrovic, P., Ingvar, M., and Landén, M. (2016). Cortical thickness, volume and surface area in patients with bipolar disorder types I and II. J. Psychiatry Neurosci. 41, 240–250. doi: 10.1503/jpn.150093
Andrews, C. M., Menkes, M. W., Suzuki, T., Lasagna, C. A., Chun, J., O’Donnell, L., et al. (2023). Reduced theta-band neural oscillatory activity during affective cognitive control in bipolar I disorder. J. Psychiatr. Res. 158, 27–35. doi: 10.1016/j.jpsychires.2022.12.012
Arikan, M. K., Metin, B., Günver, M. G., and Tarhan, N. (2019). Borderline personality and bipolar disorders cannot be differentiated electrophysiologically. Clin. EEG Neurosci. 50, 383–388. doi: 10.1177/1550059419860028
Başar, E., and Güntekin, B. (2008). A review of brain oscillations in cognitive disorders and the role of neurotransmitters. Brain Res. 1235, 172–193.
Başar, E., Güntekin, B., Atagün, I., Turp Gölbaşı, B., Tülay, E., and Ozerdem, A. (2012). Brain’s alpha activity is highly reduced in euthymic bipolar disorder patients. Cogn. Neurodyn. 6, 11–20.
Bauer, M., Andreassen, O. A., Geddes, J. R., Vedel Kessing, L., Lewitzka, U., Schulze, T. G., et al. (2018). Areas of uncertainties and unmet needs in bipolar disorders: clinical and research perspectives. Lancet Psychiatry 5, 930–939. doi: 10.1016/S2215-0366(18)30253-0
Bellivier, F., Golmard, J. L., Rietschel, M., Schulze, T. G., Malafosse, A., Preisig, M., et al. (2003). Age at onset in bipolar I affective disorder: further evidence for three subgroups. Am. J. Psychiatry 160, 999–1001.
Bente, D. (1979). Vigilance and evaluation of psychotropic drug effects on EEG. Pharmakopsychiatr. Neuropsychopharmakol. 12, 137–147.
Biasiucci, A., Franceschiello, B., and Murray, M. M. (2019). Electroencephalography. Curr. Biol. 29, R80–R85.
Bokhan, N. A., Galkin, S. A., and Vasilyeva, S. N. (2023). EEG alpha band characteristics in patients with a depressive episode within recurrent and bipolar depression. Consort Psychiatr. 4, 5–12. doi: 10.17816/CP6140
Bora, E., and Pantelis, C. (2016). Social cognition in schizophrenia in comparison to bipolar disorder: A meta-analysis. Schizophr Res. 175, 72–78.
Bresnahan, S. M., and Barry, R. J. (2002). Specificity of quantitative EEG analysis in adults with attention deficit hyperactivity disorder. Psychiatry Res. 112, 133–144.
Calhoun, V. D., Sui, J., Kiehl, K., Turner, J., Allen, E., and Pearlson, G. (2011). Exploring the psychosis functional connectome: aberrant intrinsic networks in schizophrenia and bipolar disorder. Front. Psychiatry 2, 75. doi: 10.3389/fpsyt.2011.00075
Cao, Y., Han, C., Peng, X., Su, Z., Liu, G., Xie, Y., et al. (2022). Correlation between resting theta power and cognitive performance in patients with Schizophrenia. Front. Hum. Neurosci 16, 853994. doi: 10.3389/fnhum.2022.853994
Chase, H. W., and Phillips, M. L. (2016). Elucidating neural network functional connectivity abnormalities in bipolar disorder: toward a harmonized methodological approach. Biol. Psychiatry Cogn. Neurosci. Neuroimaging 1, 288–298. doi: 10.1016/j.bpsc.2015.12.006
Chiang, A. K., Rennie, C. J., Robinson, P. A., van Albada, S. J., and Kerr, C. C. (2011). Age trends and sex differences of alpha rhythms including split alpha peaks. Clin. Neurophysiol. 122, 1505–1517. doi: 10.1016/j.clinph.2011.01.040
Clementz, B. A., Sponheim, S. R., Iacono, W. G., and Beiser, M. (1994). Resting EEG in first-episode schizophrenia-patients, bipolar psychosis patients, and their first-degree relatives. Psychophysiology 31, 486–494. doi: 10.1111/j.1469-8986.1994.tb01052.x
Cole, S. R., and Voytek, B. (2017). Brain Oscillations and the Importance of Waveform Shape. Trends Cogn. Sci. 21, 137–149.
Conner, C. R., Ellmore, T. M., Pieters, T. A., DiSano, M. A., and Tandon, N. (2011). Variability of the relationship between electrophysiology and BOLD-fMRI across cortical regions in humans. J. Neurosci. 31, 12855–12865. doi: 10.1523/JNEUROSCI.1457-11.2011
de Meneses, F. G. A., Teles, A. S., Nunes, M., da Silva Farias, D., and Teixeira, S. (2022). Neural networks to recognize patterns in topographic images of cortical electrical activity of patients with neurological diseases. Brain Topogr. 35, 464–480. doi: 10.1007/s10548-022-00901-4
Dev, D. A., Le, G. H., Kwan, A. T. H., Wong, S., Arulmozhi, A., Ceban, F., et al. (2024). Comparing suicide completion rates in bipolar I versus bipolar II disorder: A systematic review and meta-analysis. J. Affect. Disord. 361, 480–488. doi: 10.1016/j.jad.2024.06.045
El-Badri, S. M., Ashton, C. H., Moore, P. B., Marsh, V. R., and Ferrier, I. N. (2001). Electrophysiological and cognitive function in young euthymic patients with bipolar affective disorder. Bipolar Disord. 3, 79–87. doi: 10.1034/j.1399-5618.2001.030206.x
Fitzgerald, P. J., and Watson, B. O. (2018). Gamma oscillations as a biomarker for major depression: an emerging topic. Transl. Psychiatry 8, 177.
Haegens, S., Nácher, V., Luna, R., Romo, R., and Jensen, O. (2011). α-Oscillations in the monkey sensorimotor network influence discrimination performance by rhythmical inhibition of neuronal spiking. Proc. Natl. Acad. Sci. U. S. A. 108, 19377–19382. doi: 10.1073/pnas.1117190108
Han, C., Guo, M., Ke, X., Zeng, L., Li, M., Haihambo, N., et al. (2023a). Oscillatory biomarkers of autism: evidence from the innate visual fear evoking paradigm. Cogn. Neurodyn. 17, 459–466. doi: 10.1007/s11571-022-09839-6
Han, C., Zhao, X., Li, M., Haihambo, N., Teng, J., Li, S., et al. (2023b). Enhancement of the neural response during 40 Hz auditory entrainment in closed-eye state in human prefrontal region. Cogn. Neurodyn. 17, 399–410. doi: 10.1007/s11571-022-09834-x
Han, C., Wang, T., Wu, Y., Li, H., Wang, E., Zhao, X., et al. (2022). Compensatory mechanism of attention-deficit/hyperactivity disorder recovery in resting state alpha rhythms. Front. Comput. Neurosci. 16, 883065. doi: 10.3389/fncom.2022.883065
Han, C., Wang, T., Yang, Y., Wu, Y., Li, Y., Dai, W., et al. (2021). Multiple gamma rhythms carry distinct spatial frequency information in primary visual cortex. PLoS Biol. 19, e3001466. doi: 10.1371/journal.pbio.3001466
Honda, S., Matsumoto, M., Tajinda, K., and Mihara, T. (2020). Enhancing clinical trials through synergistic gamma power analysis. Front. Psychiatry 11, 537. doi: 10.3389/fpsyt.2020.00537
Howells, F. M., Temmingh, H. S., Hsieh, J. H., van Dijen, A. V., Baldwin, D. S., and Stein, D. J. (2018). Electroencephalographic delta/alpha frequency activity differentiates psychotic disorders: a study of schizophrenia, bipolar disorder and methamphetamine-induced psychotic disorder. Transl. Psychiatry 8, 75. doi: 10.1038/s41398-018-0105-y
Huang, Y., Wang, Y., Wang, H., Liu, Z., Yu, X., Yan, J., et al. (2019). Prevalence of mental disorders in China: a cross-sectional epidemiological study. Lancet Psychiatry 6, 211–224.
Jacobs, G. D., Benson, H., and Friedman, R. (1996). Topographic EEG mapping of the relaxation response. Biofeedback Self Regul. 21, 121–129.
Kam, J. W., Bolbecker, A. R., O’Donnell, B. F., Hetrick, W. P., and Brenner, C. A. (2013). Resting state EEG power and coherence abnormalities in bipolar disorder and schizophrenia. J. Psychiatr. Res. 47, 1893–1901. doi: 10.1016/j.jpsychires.2013.09.009
Kano, K., Nakamura, M., Matsuoka, T., Iida, H., and Nakajima, T. (1992). The topographical features of EEGs in patients with affective disorders. Electroencephalogr. Clin. Neurophysiol. 83, 124–129.
Kempermann, G., Krebs, J., and Fabel, K. (2008). The contribution of failing adult hippocampal neurogenesis to psychiatric disorders. Curr. Opin. Psychiatry 21, 290–295.
Khaleghi, A., Mohammadi, M. R., Moeini, M., Zarafshan, H., and Fadaei Fooladi, M. (2019). Abnormalities of Alpha Activity in Frontocentral Region of the Brain as a Biomarker to Diagnose Adolescents With Bipolar Disorder. Clin. EEG Neurosci. 50, 311–318. doi: 10.1177/1550059418824824
Khaleghi, A., Sheikhani, A., Mohammadi, M. R., and Moti Nasrabadi, A. (2015). Evaluation of cerebral cortex function in clients with bipolar mood disorder I (BMD I) compared with BMD II using QEEG analysis. Iran J. Psychiatry 10, 93–99.
Kim, S., Kim, Y. W., Shim, M., Jin, M. J., Im, C. H., and Lee, S. H. (2020). Altered cortical functional networks in patients with schizophrenia and bipolar disorder: a resting-state electroencephalographic study. Front. Psychiatry 11, 661. doi: 10.3389/fpsyt.2020.00661
Kirschfeld, K. (2005). The physical basis of alpha waves in the electroencephalogram and the origin of the “Berger effect”. Biol. Cybern. 92, 177–185. doi: 10.1007/s00422-005-0547-1
Klimesch, W. (1999). EEG alpha and theta oscillations reflect cognitive and memory performance: a review and analysis. Brain Res. Brain Res. Rev. 29, 169–195. doi: 10.1016/s0165-0173(98)00056-3
Knyazeva, M. G., Barzegaran, E., Vildavski, V. Y., and Demonet, J. F. (2018). Aging of human alpha rhythm. Neurobiol. Aging 69, 261–273.
Lindgren, K. A., Larson, C. L., Schaefer, S. M., Abercrombie, H. C., Ward, R. T., Oakes, T. R., et al. (1999). Thalamic metabolic rate predicts EEG alpha power in healthy control subjects but not in depressed patients. Biol. Psychiatry 45, 943–952. doi: 10.1016/s0006-3223(98)00350-3
Lu, Z., Wang, H., Gu, J., and Gao, F. (2022). Association between abnormal brain oscillations and cognitive performance in patients with bipolar disorder: Molecular mechanisms and clinical evidence. Synapse 76, e22247. doi: 10.1002/syn.22247
Makeig, S., Debener, S., Onton, J., and Delorme, A. (2004). Mining event-related brain dynamics. Trends Cogn. Sci 8, 204–210.
McIntyre, R. S., Berk, M., Brietzke, E., Goldstein, B. I., ópez-Jaramillo, C. L., Kessing, L. V., et al. (2020). Bipolar disorders. Lancet 396, 1841–1856.
Moore, R. A., Mills, M., Marshman, P., and Corr, P. J. (2012). Behavioural Inhibition System (BIS) sensitivity differentiates EEG theta responses during goal conflict in a continuous monitoring task. Int. J. Psychophysiol. 85, 135–144. doi: 10.1016/j.ijpsycho.2012.06.006
Narayanan, B., O’Neil, K., Berwise, C., Stevens, M. C., Calhoun, V. D., Clementz, B. A., et al. (2014). Resting state electroencephalogram oscillatory abnormalities in schizophrenia and psychotic bipolar patients and their relatives from the bipolar and schizophrenia network on intermediate phenotypes study. Biol. Psychiatry 76, 456–465. doi: 10.1016/j.biopsych.2013.12.008
Narayanan, B., Soh, P., Calhoun, V. D., Ruaño, G., Kocherla, M., Windemuth, A., et al. (2015). Multivariate genetic determinants of EEG oscillations in schizophrenia and psychotic bipolar disorder from the BSNIP study. Transl. Psychiatry 5, e588. doi: 10.1038/tp.2015.76
Nierenberg, A. A., Agustini, B., öhler-Forsberg, O. K., Cusin, C., Katz, D., Sylvia, L. G., et al. (2023). Diagnosis and treatment of bipolar disorder: a review. JAMA 330, 1370–1380.
Nigbur, R., Ivanova, G., and Stürmer, B. (2011). Theta power as a marker for cognitive interference. Clin. Neurophysiol. 122, 2185–2194. doi: 10.1016/j.clinph.2011.03.030
Nokia, M. S., Sisti, H. M., Choksi, M. R., and Shors, T. J. (2012). Learning to learn: theta oscillations predict new learning, which enhances related learning and neurogenesis. PLoS One 7, e31375. doi: 10.1371/journal.pone.0031375
Nusslock, R., Harmon-Jones, E., Alloy, L. B., Urosevic, S., Goldstein, K., and Abramson, L. Y. (2012). Elevated left mid-frontal cortical activity prospectively predicts conversion to bipolar I disorder. J. Abnorm. Psychol. 121, 592–601. doi: 10.1037/a0028973
Olypher, A. V., Klement, D., and Fenton, A. A. (2006). Cognitive disorganization in hippocampus: a physiological model of the disorganization in psychosis. J. Neurosci. 26, 158–168. doi: 10.1523/JNEUROSCI.2064-05.2006
Page, M. J., McKenzie, J. E., Bossuyt, P. M., Boutron, I., Hoffmann, T. C., Mulrow, C. D., et al. (2021). The PRISMA 2020 statement: an updated guideline for reporting systematic reviews. BMJ 372, n71.
Painold, A., Faber, P. L., Milz, P., Reininghaus, E. Z., Holl, A. K., Letmaier, M., et al. (2014). Brain electrical source imaging in manic and depressive episodes of bipolar disorder. Bipolar Disorders 16, 690–702. doi: 10.1111/bdi.12198
Peck, A. W., Bye, C. E., Clubley, M., Henson, T., and Riddington, C. (1979). A comparison of bupropion hydrochloride with dexamphetamine and amitriptyline in healthy subjects. Br. J. Clin. Pharmacol. 7, 469–478. doi: 10.1111/j.1365-2125.1979.tb00988.x
Podvalny, E., Noy, N., Harel, M., Bickel, S., Chechik, G., Schroeder, C. E., et al. (2015). A unifying principle underlying the extracellular field potential spectral responses in the human cortex. J. Neurophysiol. 114, 505–519. doi: 10.1152/jn.00943.2014
Poil, S. S., Bollmann, S., Ghisleni, C., O’Gorman, R. L., Klaver, P., Ball, J., et al. (2014). Age dependent electroencephalographic changes in attention-deficit/hyperactivity disorder (ADHD). Clin. Neurophysiol. 125, 1626–1638.
Rangaswamy, M., Porjesz, B., Chorlian, D. B., Wang, K., Jones, K. A., Bauer, L. O., et al. (2002). Beta power in the EEG of alcoholics. Biol. Psychiatry 52, 831–842.
Rommel, A. S., Kitsune, G. L., Michelini, G., Hosang, G. M., Asherson, P., McLoughlin, G., et al. (2016). Commonalities in EEG spectral power abnormalities between women with ADHD and women with bipolar disorder during rest and cognitive performance. Brain Topogr. 29, 856–866. doi: 10.1007/s10548-016-0508-0
Saletu, B. (1976). [Psychotropic drugs, brain function and sleep. Neurophysiologic aspects of psychopharmacology and pharmacopsychiatry]. Bibl. Psychiatr. 1, 245.
Sanacora, G., Zarate, C. A., Krystal, J. H., and Manji, H. K. (2008). Targeting the glutamatergic system to develop novel, improved therapeutics for mood disorders. Nat. Rev. Drug Discov. 7, 426–437.
Schaul, N. (1998). The fundamental neural mechanisms of electroencephalography. Electroencephalogr. Clin. Neurophysiol. 106, 101–107.
Schulz, C., Mavrogiorgou, P., Schröter, A., Hegerl, U., and Juckel, G. (2000). Lithium-induced EEG changes in patients with affective disorders. Neuropsychobiology 42, 33–37.
Sohal, V. S., and Rubenstein, J. L. R. (2019). Excitation-inhibition balance as a framework for investigating mechanisms in neuropsychiatric disorders. Mol. Psychiatry 24, 1248–1257. doi: 10.1038/s41380-019-0426-0
Spatz, R., and Kugler, J. (1982). Abnormal EEG activities induced by psychotropic drugs. Electroencephalogr. Clin. Neurophysiol. Suppl. 36, 549–558.
Struve, F. A. (1987). Lithium-specific pathological electroencephalographic changes: a successful replication of earlier investigative results. Clin. Electroencephalogr. 18, 46–53.
Thorpe, S. G., Cannon, E. N., and Fox, N. A. (2016). Spectral and source structural development of mu and alpha rhythms from infancy through adulthood. Clin. Neurophysiol. 127, 254–269. doi: 10.1016/j.clinph.2015.03.004
Toro, C. T., and Deakin, J. F. (2007). Adult neurogenesis and schizophrenia: a window on abnormal early brain development? Schizophr. Res. 90, 1–14.
Tseng, H. H., Wu, C. Y., Chang, H. H., Lu, T. H., Chang, W. H., Hsu, C. F., et al. (2024). Posterior cingulate and medial prefrontal excitation-inhibition balance in euthymic bipolar disorder. Psychol. Med. 1, 9. doi: 10.1017/S0033291724001326
Uhlhaas, P. J., Haenschel, C., Nikolić, D., and Singer, W. (2008). The role of oscillations and synchrony in cortical networks and their putative relevance for the pathophysiology of schizophrenia. Schizophr. Bull. 34, 927–943. doi: 10.1093/schbul/sbn062
Urbano, F. J., D’Onofrio, S. M., Luster, B. R., Beck, P. B., Hyde, J. R., Bisagno, V., et al. (2014). Pedunculopontine Nucleus Gamma Band Activity-Preconscious Awareness, Waking, and REM Sleep. Front Neurol. 5, 210. doi: 10.3389/fneur.2014.00210
Venables, N. C., Bernat, E. M., and Sponheim, S. R. (2009). Genetic and disorder-specific aspects of resting state EEG abnormalities in schizophrenia. Schizophr. Bull. 35, 826–839. doi: 10.1093/schbul/sbn021
Wang, J., Zhao, X., Bi, Y., Jiang, S., Sun, Y., Lang, J., et al. (2023). Executive function elevated by long term high-intensity physical activity and the regulation role of beta-band activity in human frontal region. Cogn. Neurodyn. 17, 1463–1472. doi: 10.1007/s11571-022-09905-z
Wegbreit, E., Cushman, G. K., Puzia, M. E., Weissman, A. B., Kim, K. L., Laird, A. R., et al. (2014). Developmental meta-analyses of the functional neural correlates of bipolar disorder. JAMA Psychiatry 71, 926–935. doi: 10.1001/jamapsychiatry.2014.660
Wyczesany, M., Kaiser, J., and Coenen, A. M. L. (2010). Associations Between Self-Report of Emotional State and the EEG Patterns in Affective Disorders Patients. J. Psychophysiol. 24, 33–40.
Yener, G. G., Güntekin, B., Oniz, A., and Başar, E. (2007). Increased frontal phase-locking of event-related theta oscillations in Alzheimer patients treated with cholinesterase inhibitors. Int. J. Psychophysiol. 64, 46–52. doi: 10.1016/j.ijpsycho.2006.07.006
Zhou, T. H., Mueller, N. E., Spencer, K. M., Mallya, S. G., Lewandowski, K. E., Norris, L. A., et al. (2018). Auditory steady state response deficits are associated with symptom severity and poor functioning in patients with psychotic disorder. Schizophr. Res. 201, 278–286. doi: 10.1016/j.schres.2018.05.027
Keywords: Bipolar disorder, biological markers, neural oscillation, rsEEG, spectral power
Citation: Su Z, Zhang H, Wang Y, Chen B, Zhang Z, Wang B, Liu J, Shi Y and Zhao X (2024) Neural oscillation in bipolar disorder: a systematic review of resting-state electroencephalography studies. Front. Neurosci. 18:1424666. doi: 10.3389/fnins.2024.1424666
Received: 28 April 2024; Accepted: 30 July 2024;
Published: 21 August 2024.
Edited by:
Yuan-Wei Yao, Free University of Berlin, GermanyReviewed by:
Mengyu Tu, Johns Hopkins University, United StatesTaicheng Huang, Shanghai Jiao Tong University, China
Copyright © 2024 Su, Zhang, Wang, Chen, Zhang, Wang, Liu, Shi and Zhao. This is an open-access article distributed under the terms of the Creative Commons Attribution License (CC BY). The use, distribution or reproduction in other forums is permitted, provided the original author(s) and the copyright owner(s) are credited and that the original publication in this journal is cited, in accordance with accepted academic practice. No use, distribution or reproduction is permitted which does not comply with these terms.
*Correspondence: Xixi Zhao, emhhb3hpeGlAY2NtdS5lZHUuY24=; Yuwei Shi, NDQzODQ2NTc5QHFxLmNvbQ==
†These authors have equally contributed to this work