- 1SciLore LLC, Kingsbury, TX, United States
- 2Instiute of Biology, College of Science, University of the Philippines Diliman, Quezon City, Philippines
- 3Center for Research and Innovation, Ateneo de Manila University School of Medicine and Public Health, Pasig City, Philippines
- 4Cancer Immunology Program, Peter MacCallum Cancer Centre, Melbourne, VIC, Australia
- 5Sir Peter MacCallum Department of Oncology, The University of Melbourne, Melbourne, VIC, Australia
- 6National Institute of Molecular Biology and Biotechnology, College of Science, University of the Philippines Diliman, Quezon City, Philippines
- 7Institute for Dementia Care Asia, Quezon City, Philippines
- 8Institute of Molecular Biology and Biotechnology, National Institutes of Health, University of the Philippines Manila, Manila, Philippines
- 9Mental Health Research Unit, National Center for Mental Health, Mandaluyong City, Philippines
Alzheimer’s disease (AD) is the leading cause of dementia globally, having a pathophysiology that is complex and multifactorial. Recent findings highlight the significant role of non-coding RNAs (ncRNAs), specifically microRNAs (miRNAs), long non-coding RNAs (lncRNAs), circular RNAs (circRNAs), and piwi-interacting RNAs (piRNAs) in the molecular mechanisms underlying AD. These ncRNAs are involved in critical biological processes such as cell proliferation, apoptosis, oxidative stress, amyloid-beta aggregation, tau phosphorylation, neuroinflammation, and autophagy, which are pivotal in AD development and progression. This systematic review aims to consolidate current scientific knowledge on the role of ncRNAs in AD, making it the first to encompass the four types of ncRNAs associated with the disease. Our comprehensive search and analysis reveal that ncRNAs not only play crucial roles in the pathogenesis of AD but also hold potential as biomarkers for its early detection and as novel therapeutic targets. Specifically, the findings underscore the significance of miRNAs in regulating genes involved in key AD pathways such as activin receptor signaling pathway, actomyosin contractile ring organization, and advanced glycation endproducts-receptor advanced glycation endproducts (AGE-RAGE) signaling pathway. This review also highlights the potential of ncRNAs in unveiling novel diagnostic and therapeutic strategies, emphasizing the need for further research to validate their clinical utility. Our systematic exploration provides a foundation for future bioinformatic analyses and the development of ncRNA-based precision medicine approaches for AD, offering new insights into the disease’s molecular pathology and paving the way for innovative treatment strategies.
Systematic review registration: PROSPERO, https://www.crd.york.ac.uk/prospero/, CRD42022355307.
Introduction
Alzheimer’s disease (AD) is the most prevalent form of dementia, accounting for 60%–80% of cases worldwide (Scheltens et al., 2016; Alzheimer’s Association, 2023). In 2019, the global cost of dementia reached $1.3 trillion, with approximately 50% attributed to informal care (Wimo et al., 2023). According to the estimate of World Health Organization (2023), over 55 million people are living with dementia, with an additional 10 million new cases added each year. Notably, more than half of these cases (60%) originate from low- and middle-income countries, highlighting the substantial economic burden that dementia imposes. From 1990 to 2019, the incidence of disability adjusted life-years (DALYs) due to AD and other forms of dementia increased from 9.7 to 25.3 million worldwide (Li X. et al., 2022).
Dementia is a general term that refers to the decline in cognitive function severe enough to interfere with daily life and activities (National Institute on Aging, 2022). This decline predominantly affects thinking, memory, and reasoning functions—leading to a wide array of symptoms with varying degrees of severity. These symptoms include memory loss, difficulty in communication or finding words, challenges with visual and spatial abilities, trouble solving problems or reasoning, inability to handle complex tasks, difficulties in planning and organizing, confusion, disorientation, and changes in personality leading to agitation or withdrawal (National Institute on Aging, 2022; Alzheimer’s Disease and Dementia, 2023). While AD accounts for most cases of dementia, other types also exist including frontotemporal dementia, Lewy body dementia, vascular dementia, and mixed dementia, which can be a combination of two or more types.
Previously, definitive diagnosis for AD was only through the postmortem detection of amyloid-beta plaques and neurofibrillary tangles in autopsied brain tissues (McKhann et al., 1984; Scheltens et al., 2016). However, the research framework provided in 2018 by the National Institute on Aging and the Alzheimer’s Association (NIA-AA Research Framework) broadened the definition and diagnostic criteria of AD by introducing the use of in vivo biomarkers (Jack et al., 2018). These in vivo biomarkers can be imaging and biofluids that measure amyloid-beta deposition, pathologic tau, and neurodegeneration (ATN framework). Indeed, this biological definition of AD has allowed the definitive diagnosis in living patients, enabling the development of scientific research geared toward a comprehensive understanding of the disease.
Based on the age of onset, AD can be categorized as early-onset (EOAD) if the disease develops before the age of 65, or as late-onset (LOAD) if it manifests at 65 years old or later. Among AD cases, EOAD accounts for only 5%–6% while the rest are LOAD (Mendez, 2019). In terms of clinical characteristics, EOAD and LOAD are similar except in baseline cognition, rate of cognitive decline, and survival duration (Seath et al., 2023). In terms of genetic etiology, EOAD is clearly defined while LOAD is less definitive (Long and Holtzman, 2019; Uddin et al., 2021; Andrade-Guerrero et al., 2023). EOAD is linked to the autosomal dominant mutations in amyloid-beta precursor protein (APP), presenilin-1 (PSEN1), and presenilin-2 (PSEN2). In contrast, while many genes are associated with the different aspects of LOAD, they do not provide a comprehensive explanation for its etiology. As such, there is still this huge need to fully elucidate the origins of LOAD. Having a deeper comprehension of its etiology might pave the way for its early detection, novel therapeutic interventions, and better disease management.
There are a few systematic reviews and meta-analyses that have evaluated the association of different lifestyle factors with AD pathophysiology. These factors include physical activity or the lack thereof (Zhu et al., 2015; Stephen et al., 2017; Demurtas et al., 2020; Andrade et al., 2022; López-Ortiz et al., 2023), the use of non-steroidal drugs (Etminan et al., 2003), and diet (Muñoz Fernández et al., 2017; Yusufov et al., 2017; Xu Lou et al., 2023). Other systematic reviews involve the plasma nutrient status of patients with AD (Lopes da Silva et al., 2014), the prevalence of the disease and neuropsychiatric symptoms (Zhao Q. F. et al., 2016; Niu et al., 2017), neuroimaging markers (Bloudek et al., 2011; Ferreira et al., 2011; Liu et al., 2015), potential pharmacological treatment of the disease (Kaduszkiewicz et al., 2005; Hyde et al., 2013; Lu et al., 2020; David et al., 2022), and infection (Ou et al., 2020).
The limited understanding of the etiology of the most prevalent type of AD might be attributed to the focus on protein-coding genes in previous disease studies. However, over the past decade, the vital role of noncoding RNAs (ncRNAs) in AD’s pathophysiology has started to emerge (Maoz et al., 2017; Jain et al., 2019; Shobeiri et al., 2023). ncRNA are functional RNA molecules that are not translated into proteins. They are broadly categorized into housekeeping ncRNAs and regulatory ncRNAs. Housekeeping ncRNAs are essential for basic cellular functions, while regulatory ncRNAs are crucial in gene expression which affects various cellular activities. Major classes of ncRNA include, microRNAs (miRNAs), long noncoding RNAs (lncRNAs), circular RNAs (circRNAs), and piwi-interacting RNAs (piRNAs) (Liu et al., 2023). These ncRNAs were found to play key roles in development, immunity, cell proliferation, apoptosis, oxidative stress, amyloid-beta aggregation, tau phosphorylation, neuroinflammation, and autophagy, which all contribute to AD development (Chen et al., 2019; Zhang et al., 2021). They constitute a class of RNA molecules that, despite not encoding proteins, interact with DNA, RNA, proteins, and even other ncRNAs to modulate a wide range of biological processes, such as gene transcription, RNA turnover, mRNA translation, and protein assembly.
Specifically, miRNAs, lncRNAs, circRNAs, and piRNAs have attracted significant attention for their interaction with diverse molecular entities and for their pivotal roles in the regulation of gene expression (Nikolac Perkovic et al., 2021). In particular, miRNAs were found to influence the expression of genes involved in amyloid-beta deposition, tau phosphorylation, and neuroinflammation (Lei et al., 2015; Zhong et al., 2018). miRNAs are formed when miRNA genes are transcribed by RNA polymerase II to form long primary miRNA (pri-miRNA) transcripts. These pri-miRNAs undergo processing through DROSHA and DICER to form a miRNA duplex, which is loaded into Argonaute proteins that forms mature miRNAs (Komatsu et al., 2023). Likewise, lncRNAs are also transcribed by RNA polymerase II from intergenic, exonic, or distal protein-coding regions of the genome. These transcripts are capped with a 5′ methyl-cytosine cap and a 3′ poly (A) tail to form lncRNAs (Dhanoa et al., 2018; Loganathan and Doss, 2023). LncRNAs were observed to regulate amyloid-beta production, tau phosphorylation, and synaptic function in AD (Zhang et al., 2021). Interestingly, they were also found to bind to miRNAs or proteins and can therefore modulate their activity. In the same sense, circRNAs were observed to function as miRNA sponges that affect AD onset and progression (Zhao Y. et al., 2016; Kristensen et al., 2019). They are formed through the backsplicing of canonical splice sites. Specifically, exons are spliced in the opposite direction and combines the upstream and downstream 3′ and 5′ splice sites, which forms circRNA (Nisar et al., 2021; Loganathan and Doss, 2023). Numerous piRNAs have also been found to be differentially expressed in AD brains. Most of these were linked with genome-wide significant AD risk single nucleotide polymorphisms (SNPs) in the apolipoprotein E (APOE) cluster. piRNAs were also linked with the dysregulation of different AD-associated pathways, such as pituitary adenylate cyclase activating polypeptide (PACAP) signaling in neurons, axonal transport, and α-amino-3-hydroxy-5-methyl-4-isoxazolepropionate (AMPA) receptor trafficking (Sato et al., 2023). RNA precursors transcribed in the nucleus are exported into the cytoplasm to form piRNA precursors which undergo primary and secondary amplification cycles to form mature piRNAs that form complexes with PIWI proteins (Loganathan and Doss, 2023).
In this paper, our overall objective is to systematically review all scientific studies that evaluate the role of ncRNAs in the brain and circulating tissues of individuals with confirmed AD. To the best of our knowledge, this is the first systematic review which consolidates the four types of ncRNAs (miRNAs, lncRNAs, circRNAs, and piRNAs) that are associated with AD. This systematic review further looks at some of the top significant miRNAs associated with AD, among the associated ncRNAs, and to discover how it contributes to AD pathology. In addition, the consolidated lists of lncRNAs, circRNAs, and piRNAs hold potential for future bioinformatic analyses to further elucidate the role of ncRNAs as biomarkers and therapeutic targets of AD. Understanding the roles of ncRNA in AD may be pivotal in developing treatment strategies that prevent the progression of the disease through precision medicine.
Materials and methods
This systematic review implemented a workflow (Figure 1) following the Preferred Reporting Items for Systematic Reviews and Meta-Analyses (PRISMA) (Page et al., 2021). The protocol was registered to PROSPERO with registration number: CRD42022355307.
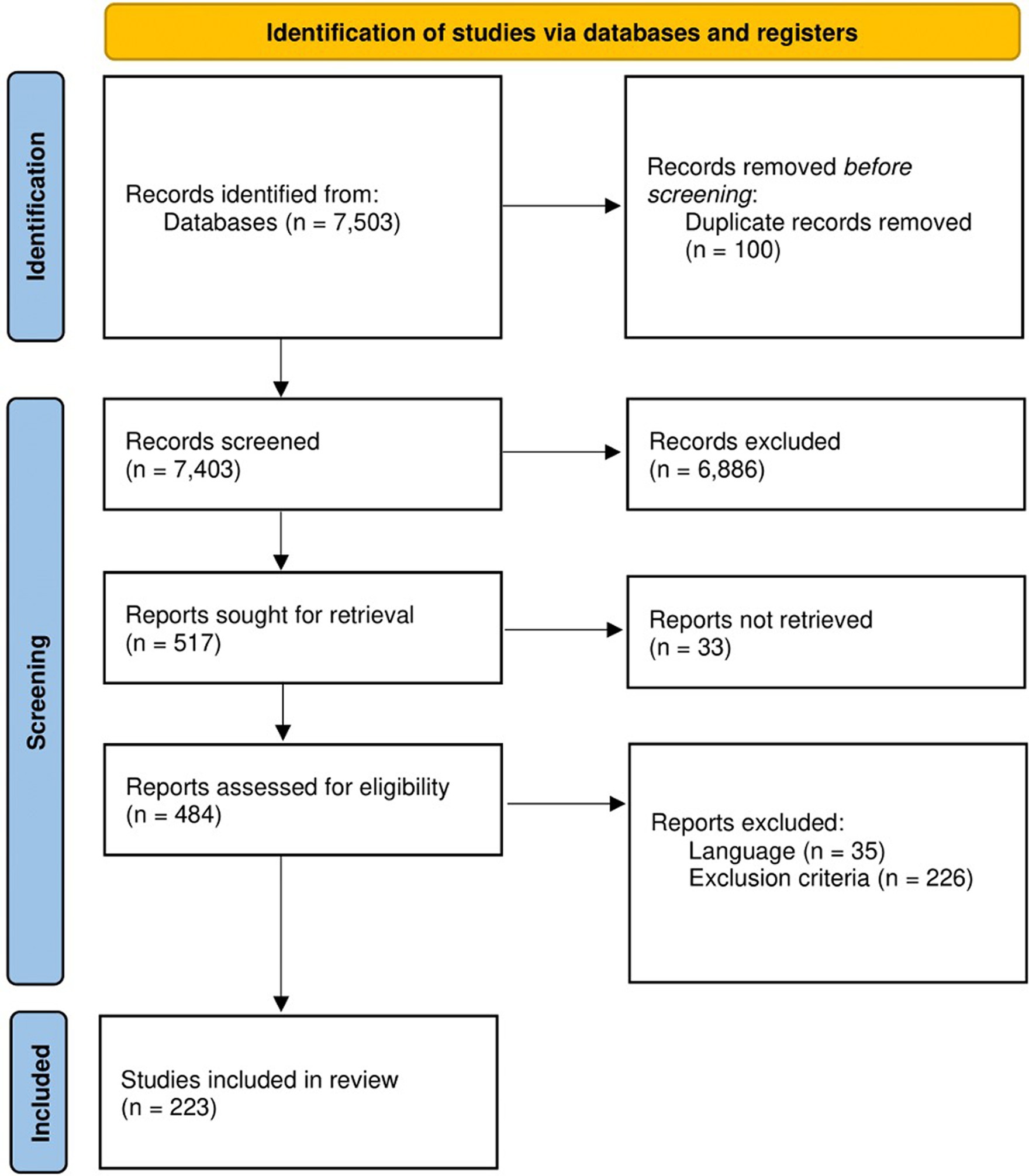
Figure 1. Workflow utilized in this systematic review as adopted from PRISMA. At the start, 7,503 studies were retrieved from PubMed, Google Scholar, and Scopus. After the title and abstract screenings, the retrieved studies were reduced to 484 and were subjected further for full-text screening following set inclusion and exclusion criteria. Finally, a total of 223 studies were included in this systematic review for downstream analyses.
Search strategy
Database searches were conducted on PubMed, Google Scholar, and Scopus, which are commonly accessed databases that contain peer-reviewed biomedical literature, in 21–23 July 2023 by three independent reviewers. Different keywords were used to search each database which are provided in Supplementary Table 1. All results of each database search, regardless of publication year and study design, were exported and compiled by a fourth independent reviewer. Duplicates of studies were removed before proceeding to abstract screening.
Abstract screening
The abstracts of each article from the compiled list were used as basis for further inclusion or exclusion from the study. Specifically, the studies included for further screening were those that were published in English and were independent original research that evaluated the role of ncRNAs on the development of AD. The studies that were excluded were reviews, case reports, abstracts, letters, commentaries, methodological studies, conference poster presentation, or any article that was not peer-reviewed. Those that did not involve human research subjects or were not accessible were also excluded. The liberal acceleration policy was used, such that excluded studies were screened by a second independent reviewer. Any disagreement was settled by a third independent reviewer.
Full text screening and data extraction
The included studies were further assessed, and the data for each study was extracted by an independent reviewer. Apart from the criteria presented in the abstract screening, studies that did not specify the type of dementia in their study population were excluded. Studies were also excluded if the type of ncRNA was not specified, and if there were no control group present in the study.
Google Forms was used to record the following information: basic study information (authors, title, year of publication, DOI, and journal published), participant demographics (total number of cases and controls, mean age, number of males and females, race), tissue samples, and methodology/technology used. Data related to ncRNA expression profiles was also extracted, including ncRNA gene expression fold change, p-values, and the functions of the ncRNAs, when available. The liberal acceleration policy was also implemented for full-text screening. Any discrepancies were adjudicated by a third independent reviewer.
Quality appraisal
Each study after data extraction was appraised of its quality by two independent researchers using the Quality of Genetic Association Studies Tool (Q-Genie) tool (Sohani et al., 2015). This tool was specifically developed to identify high quality genetic studies, and it has been shown to exhibit good inter-rater reliability, internal consistency, and overall reliability. It consists of 11 questions which are answered using a Likert scale ranging from 1 (poor) to 7 (excellent). The questions were classified into nine categories: rationale for the study, sample selection, exposure classification, outcome classification, identification of sources of bias, presentation of the statistical plan, quality of statistical methods, testing of assumptions in genetic studies, and result interpretation. The studies were scored and categorized as low, moderate, and high-quality studies based on their average total scores. Those studies with scores ≤ 35 were considered low-quality studies, >35 to ≤45 were of moderate quality, and > 45 were high quality studies. The low-quality studies were then subsequently excluded from this systematic review. All included studies (223) were compiled in Supplementary Table 2.
miRNA gene-target prediction and pathway enrichment analysis
Potential gene targets of the miRNAs obtained from the literature review were identified using the multiMiR package (version 3.18) (Ru et al., 2014). The multiMiR package facilitates the identification of gene targets of miRNAs from 14 validated and predicted miRNA-target interaction databases. As required by the package, only miRNAs with precursor-derived arm designation information (i.e., “-3p” or “-5p” suffix) were included in the analysis. miRNA-gene target pairs with an “all.sum score” of at least 5 (indicating presence in at least 5 of the databases queried), were considered significant.
Of the significant miRNAs, only the top 3 with the highest number of gene targets were subjected to exploratory functional analysis via the STRING 12.0 database (Search Tool for the Retrieval of Interacting Genes/Proteins; https://string-db.org) (Szklarczyk et al., 2023). Specifically, the target genes of the top 3 miRNAs were subjected to pathway enrichment analysis to elucidate the potential molecular pathways associated with these miRNA gene targets. Only the enriched pathways from Gene Ontology (Biological Processes), KEGG and Reactome classification systems were recorded. Protein–protein interaction network analysis was also performed to visualize and appraise the biological connectivity of the gene targets.
Results
This systematic review shows that the research on ncRNAs in the context of AD has grown significantly over the years (Supplementary Figure 1). All studies included in this systematic review involved the detection or validation of ncRNAs in individuals with AD, compared with neurotypical controls. The sample sizes of these included studies ranged from one to 1,021 cases and from one to 1,385 control participants and their ages ranged from 58 to 93 years (Supplementary Figure 2).
The race of participants across these studies were varied, although dominated by East Asians, Europeans, and Americans (Figure 2A). Among the included studies, 19 categorically classified their participants or samples as EOAD while 186 studies classified theirs as LOAD (Figure 2B). At least two AD diagnosis criteria were used by most of the studies (Figure 2C). Popular among AD diagnosis criteria utilized were the National Institute of Neurological and Communicative Disorders and Stroke and the Alzheimer’s Disease and Related Disorders Association (NINCDS-ADRDA) Alzheimer’s Criteria, the Mini Mental State Exam (MMSE), and the NIA-AA Diagnostic Criteria for Alzheimer’s.
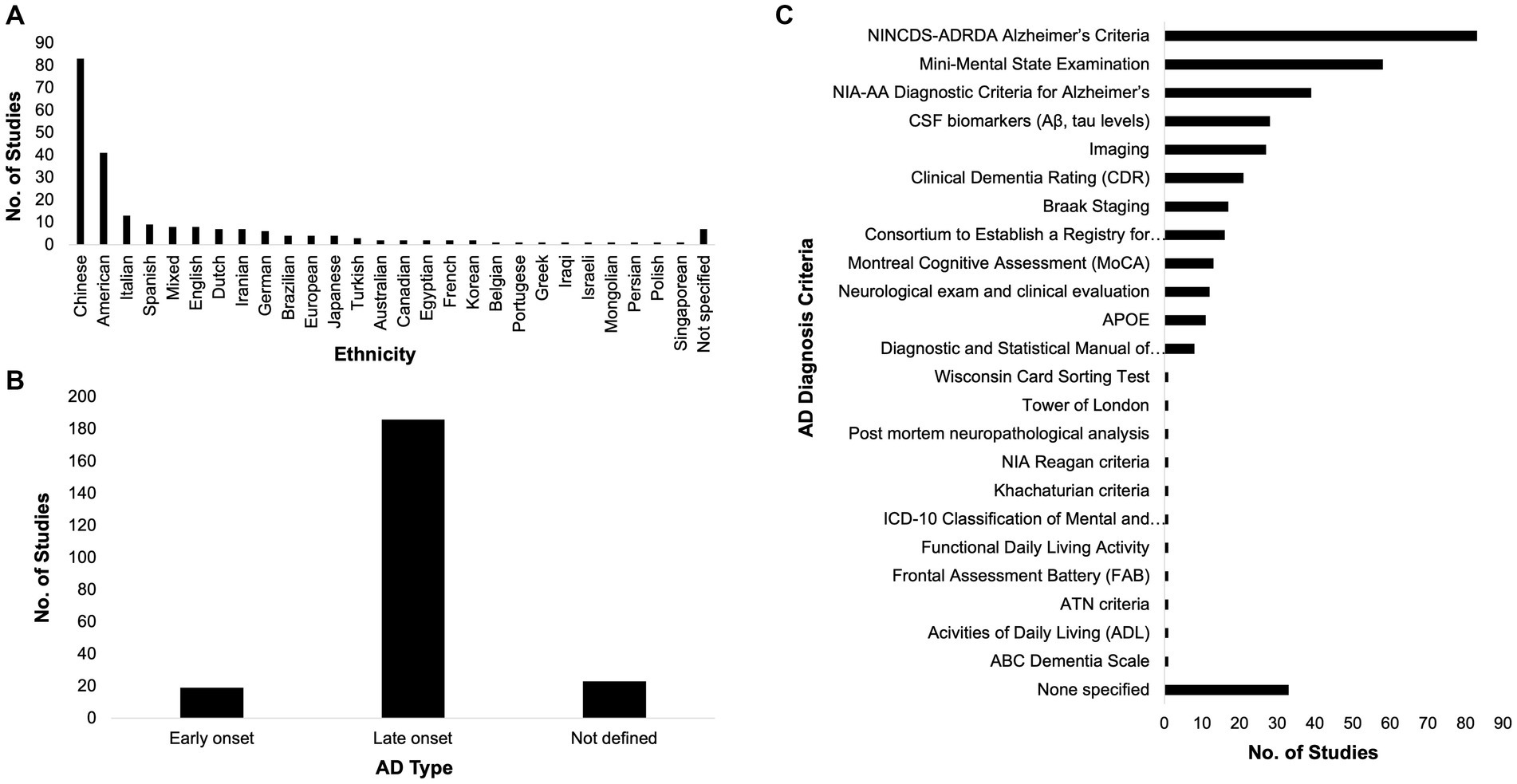
Figure 2. Race, Alzheimer’s disease (AD) type, and diagnosis criteria. The participants across the included studies in this systematic review span different ethnicities (A). Most of the included studies dealt with the late-onset type of AD (B) and most of them utilized at least two AD diagnosis criteria (C).
To investigate the ncRNAs with respect to AD, brain and circulating tissue samples (Figures 3A,B) were collected by the included studies, where most of them used the qPCR technology (Figure 3C) to investigate the role of ncRNAs in AD pathology. From these, unique miRNAs, lncRNAs, circRNAs, and piRNAs were compiled (Figure 3D; Table 1; Supplementary Table 3). It is interesting to note that some of these studies did not just investigate only one ncRNA type. There was one study that analyzed lncRNAs and circRNAs, three studies that analyzed miRNAs and circRNAs, three studies that studied miRNAs and lncRNA, and one study that studied miRNAs and piRNAs together. These studies have also suggested that the each ncRNA type affects AD pathophysiology through different mechanisms, such as amyloid-beta accumulation, tau phosphorylation, cell apoptosis, and neurotoxicity (Table 1).
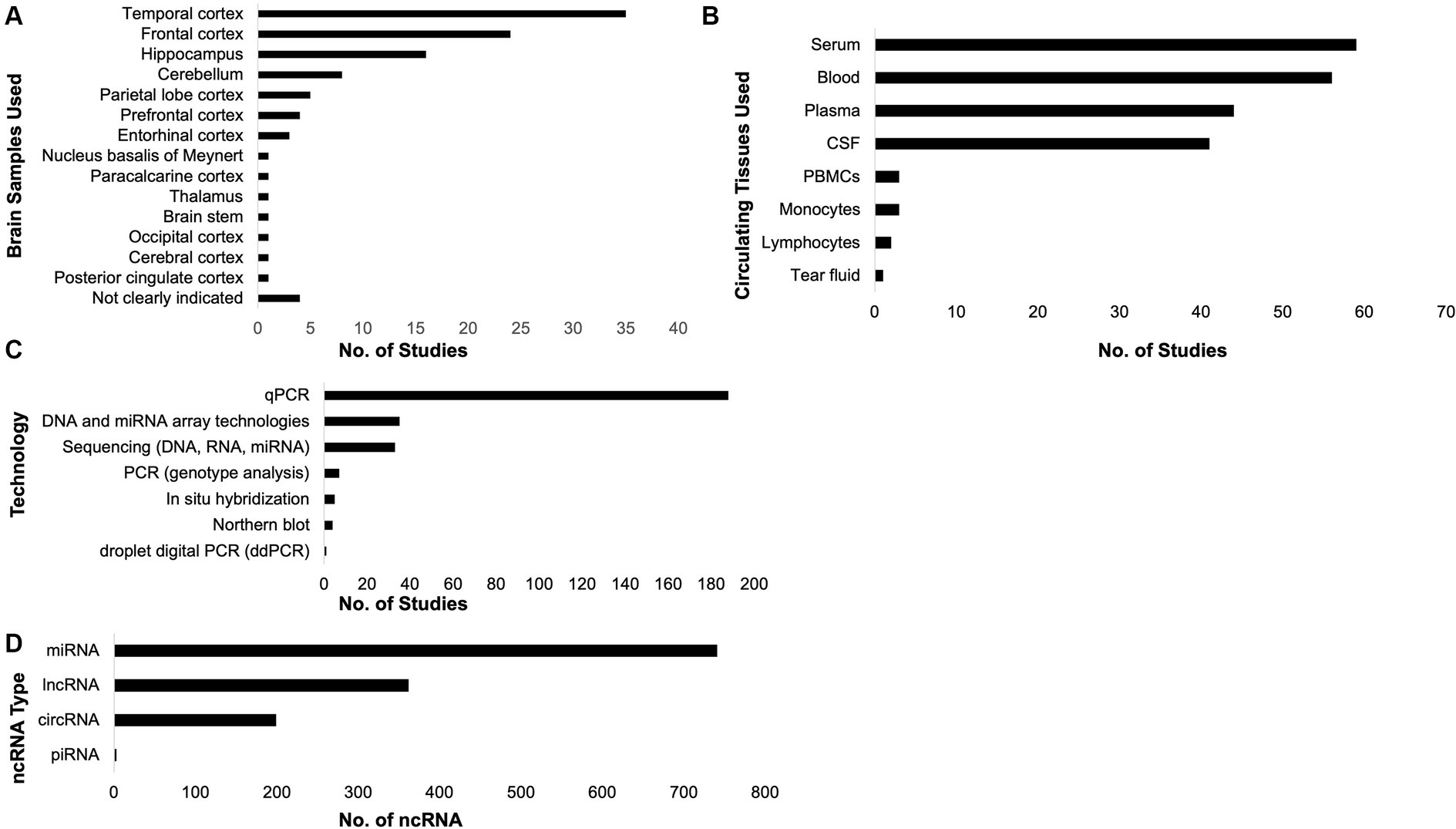
Figure 3. Samples collected, technologies utilized, and non-coding RNAs (ncRNAs) compiled. The included studies in this systematic review collected brain (A) and circulating (B) tissues. Most of the studies utilized qPCR to investigate the role of ncRNAs in Alzheimer’s disease (C). From the included studies, the unique ncRNAs associated with AD pathology were compiled (D).
To illustrate the potential of our compiled ncRNA list, we focused on the 150 miRNAs that were found to be highly significant (p < 0.001) by their respective studies. We performed gene target prediction analysis on all the miRNAs and identified the top 3 miRNAs (let-7b-5p, let-7 g-5p, and mir-15b-5p) with the most gene targets. Pathway enrichment analysis (Supplementary Table 4) of the gene targets of the top miRNAs uncovered pathways that can be related to AD pathology such as the Activin Receptor Signaling Pathway concerning the protection and survival of neurons, the Actomyosin Contractile Ring Organization which can be linked to neurons re-entering the cell cycle that leads to apoptosis, and the advanced glycation end products-receptor advanced glycation endproducts (AGE-RAGE) Signaling Pathway.
Discussion
The increasing number of published studies over the years have shown the great potential of ncRNAs as biomarkers and therapeutic targets of AD. Literature reviews that highlight the role of ncRNAs in AD have also been published in recent years (Lukiw, 2007; Zhang et al., 2021; Yoon et al., 2022; Olufunmilayo and Holsinger, 2023; Pierouli et al., 2023). These studies hypothesize the role of ncRNAs in disease pathogenesis by examining the involvement of ncRNAs in amyloid-beta peptide and tau accumulation, neuroinflammation, synaptic dysfunction, neuronal apoptosis, and neuronal loss (Liu Y. et al., 2022; Li L. et al., 2023; Olufunmilayo and Holsinger, 2023). Other literature reviews also examined the potential of ncRNAs as biomarkers for diagnosis (Swarbrick et al., 2019; Noor Eddin et al., 2023; Shobeiri et al., 2023).
miRNAs
Majority (57%) of the compiled ncRNAs in this systematic review are miRNAs, some of them have been implicated in the amyloid-beta pathway. Specifically, miRNAs affect APP and the enzymes that produce amyloid-beta. MiR-16, miR-29a/b-1 and c, miR-186, and miR-195 have been shown to be downregulated in AD patients and AD mice models (Hébert et al., 2009; Zhu et al., 2012; Lei et al., 2015; Kim et al., 2016; Zhong et al., 2018). MiR-16 affects the expression of APP and beta-secratase 1 (BACE1), which are enzymes involved in the production of amyloid-beta, and it has also been shown to decrease tau phosphorylation in neuronal cell lines (Parsi et al., 2015). MiR-29a and 29b-1 were decreased in AD subjects with increased BACE1 (Hébert et al., 2009), and miR-195 was also decreased in AD patients who were carriers of the epsilon 4 allele of apolipoprotein E (ApoE ε4; Cao et al., 2021). The ApoE ε4 affects the phospholipid composition and neuronal homeostasis of the brain. It plays a role in amyloid-beta clearance, neurofibrillary tangle accumulation, and synaptic plasticity. miRNAs also play a role in neuroinflammation. MiR-132 and miR-212 were upregulated in AD patients and led to the decrease in silent information regulator 1 (SIRT1) expression, which has anti-inflammatory roles in AD (Hadar et al., 2018).
As it is a main hallmark of AD, miRNAs have been investigated as novel biomarkers due to their diverse functions. The downregulation of miRNAs results in the upregulation of AD-related genes, such as APP, PSEN1, and PSEN2. Expression changes for miRNAs were found in AD patients’ brains and cerebrospinal fluid (CSF). Peripheral blood miRNAs were also being explored as biomarkers, but there is a need to further validate these studies. According to Wu et al. (2015), 20 distinct miRNAs were upregulated and 32 miRNAs were significantly downregulated in AD patients, compared to controls. The miR-29b, miR-181c, miR-15b, miR-146a, and miR-107 were consistently found to be downregulated in at least two independent studies (Wu et al., 2015). MiR-26b-5p, miR-615-3p, miR-4722-5p, and miR23a-3p were also selected as miRNAs with the highest accuracy for the diagnosis of the AD group, compared to those with Parkinson’s disease (Zotarelli-Filho et al., 2023). In a meta-analysis involving 10 studies, the diagnostic accuracy of miRNAs yielded a combined area under the curve (AUC) of 0.88, with a pooled sensitivity of 0.80, and the specificity of discriminating cases from controls was 0.83 (Zhang et al., 2019). Similar results were obtained in a separate study by Hu et al. (2016) which included 7 seven studies; a pooled sensitivity of 0.86, pooled specificity of 0.87 and AUC of 0.87 was reported. These findings suggest that miRNAs are excellent biomarkers for the diagnosis of AD (Hu et al., 2016; Zhang et al., 2019).
lncRNAs
The second most studied ncRNAs are lncRNAs. LncRNAs can impact AD pathogenesis through chromatin modulation, post-transcriptional and post-translational regulation, and protein complex organization (Luo and Chen, 2016; Zhang, 2016). As such, it is being explored as a biomarker for AD. Similar to miRNAs, lncRNA also affect the expression of BACE1. The antisense transcript of BACE1 (BACE1-AS) is a lncRNA that upregulates BACE1 and is highly expressed in patients (Modarresi et al., 2011; Zhang et al., 2018). Specifically, BACE1-AS affects BACE1 stability through the formation of an RNA duplex that results in the upregulation of BACE1 (Zeng et al., 2019). The cortex of patients with AD showed increased levels of APP, BACE1, BACE1-AS, and amyloid-beta (Kang et al., 2014). Due to its effect, BACE1-AS has also been explored in mice models. Knockdown models of BACE1-AS by siRNA resulted in reduced BACE1, APP production, and tau phosphorylation in the hippocampus in SAMP8 mice, a neurodegeneration model used to study AD (Zhang et al., 2018). Overall, this led to enhanced memory and improved learning behavior. The lncRNA X inactive specific transcript (XIST) has also been found to regulate the expression of BACE1, and was found to be increased in AD mice and cell models, compared to controls (Yue et al., 2020; Yan et al., 2022). XIST knockdowns in mice hippocampal neurons showed reduced amyloid-beta-induced neurotoxicity, neuroinflammation, apoptosis, and oxidative stress. lncRNAs in plasma are also being explored as biomarkers. Differential expression of AL133415.1, AC020916.1, and ASMTL-AS were significantly lower in AD patients compared to controls in the Chinese population (Cheng et al., 2023). LncRNAs have also been explored as therapeutic targets for AD. The nuclear enriched abundant transcript 1 (NEAT1) is a lncRNA that plays a role in neuroplasticity and long-term memory formation (Asadi et al., 2021). In APP/PS1 transgenic mice, there has been an increased level of NEAT1, which caused amyloid-beta accumulation and impaired cognitive skills (Huang et al., 2020). It served as a sponge for miR-107, causing a lower expression of miR-107 which leads to tau phosphorylation in vitro (Ke et al., 2019). The knockdown of NEAT1 mitigated the effects of the miRNA by upregulating miR-107. It was also found to target miR-193a, which reduced apoptosis, inflammatory cytokine levels, and oxidative stress in mice models (Li Y. et al., 2023). This suggests that NEAT1 may serve as a suitable target for the treatment of AD.
circRNAs
circRNAs serve as sponges of miRNAs and influence AD onset and progression. The circular RNA ciRS-7 is one of the most characterized circRNA which contains binding sites for miR-7 (Zhao Y. et al., 2016; Kristensen et al., 2019). A decrease in ciRS-7 has been detected in AD brains which lead to a decrease in the sponging activity of miR-7. Overall, this results in an increase in BACE1 and APP production and decrease in ubiquitin protein ligase A (UBE2A), a protein that hinders amyloid-beta production. A separate study has also identified circAXL, circGPHN, and circPCCA and their predictive value for AD through a receiver operating characteristic (ROC) curve and AUC analyses (Li et al., 2020). These circRNA were correlated with MMSE scores, amyloid-beta-42 levels, and t-tau levels. Their mechanism of action is hypothesized to affect the transcription of their parental genes, which are critical in neuroinflammation. They are hypothesized to regulate neuroinflammation and neuronal cell death by competitively binding to miRNAs that also increase AD risk. CircKCCN2, circHOMER2, circFMN1, circDOCK1, circMAP7, circRTN4, and circPICALM were also correlated with Braak scores (Zhang and Bian, 2021). Overall this suggests that circRNA may serve as potential novel biomarkers and therapeutic targets for AD.
piRNAs
piRNAs silence transposable elements in genes, and regulate gene expression by interacting with PIWI-clade Argonaute proteins (Olufunmilayo and Holsinger, 2023). In AD, more extensive research on the roles of piRNAs are necessary. However, there are a few studies which have identified the role of piRNA in AD pathophysiology. piRNAs promote oxidative stress and amyloid-beta and tau protein accumulation, which contribute to the onset and progression of AD. Specifically, piR-34393 and piR-38240 were found to decrease cytochrome C somatic (CYCS) and karyopherin subunit alpha 6 (KPNA6) expression, which have both been correlated to AD (Roy et al., 2017). A total of 103 differentially expressed piRNAs have also been identified that were associated with SNPs in AD cases, compared to controls (Qiu et al., 2017). Owing to their profound influence on the regulatory processes that control disease progression, piRNAs are increasingly recognized as promising biomarker candidates (Zhang et al., 2021).
Activin receptor signaling pathway
The activin receptor complex plays a role in decreasing neuroinflammation and stimulating oligodendrocyte progenitor differentiation (De Berdt et al., 2018). Specifically, activin A is a member of the transforming growth factor β superfamily, and it is crucial in cell proliferation, migration, differentiation, and immune regulation (Link et al., 2016; Li Y. et al., 2022). It is also synthesized in neurons, thus playing a role in neuroprotection. Activin A binds to type II receptors, which enables type I receptors to phosphorylate Smad family member 2 (SMAD2) and Smad family member 3 (SMAD3), which are intracellular signaling proteins. These signaling proteins form the SMAD (abbreviated from the Caenorhabditis elegans Sma genes and the Drosophila Mad, mothers against decapetaplegic genes) complex by assembling with Smad family member 4 (SMAD4), and this complex regulates the expression of specific genes in the brain (Zheng et al., 2017).
In AD models, activin was found to induce neuronal development and neurite outgrowth in transgenic mice overexpressing familial AD mutations (Park et al., 2016). AD mice exhibited decreased neuronal development, but was recovered through the addition of activin A. In vitro models have also shown that activin A produced from nerve fibroblasts facilitate the migration and proliferation of Schwann cells, implicating its role in peripheral nerve repair (Li Y. et al., 2022). Hsa-let-7 g-5p is a circulating miRNA that has been found to be downregulated in AD (Kumar and Reddy, 2016; Kafshdooz et al., 2023). Neuronal loss in the brain has also been found in the downregulation of let-7 g (Derkow et al., 2018). However, it’s active role in the pathophysiology of AD is yet to be described.
Actomyosin contractile ring organization
Neurons rely on the cytoskeletal networks for its morphology due to its highly polarized nature (Kang and Woo, 2019). The microtubule system and actin microfilaments in the cytoskeleton largely affect synapse morphology, which impacts synapse function (Torres Cleuren and Boonstra, 2012). Actin dynamics, including polymerization, depolymerization, severing, and bundling, modulate synaptic plasticity (Kang and Woo, 2019).
Tau proteins, which accumulate to form neurofibrillary tangles, accumulate in the axons in brain tissue by binding to microtubules. In AD patients, there is a lower affinity for microtubules and higher tau polymerization. In addition, the actin-depolymerizing factors (ADF)/cofilin family play a large role in regulating the actin dynamics in the brain (Torres Cleuren and Boonstra, 2012; Kang and Woo, 2019; Rahman et al., 2020; Bamburg et al., 2021). Cofilin functions to enhance actin polymerization, enhancing nucleation and filament formation.
In AD patients, ADF/cofilin-actin rod aggregates have been detected in amyloid plaques. These aggregates were determined to aid in tau recruitment, resulting in defects in axonal and vesicular transport (Torres Cleuren and Boonstra, 2012; Bamburg et al., 2021). MiR-15b-5p targets mRNA involved in cell cycle regulation and apoptotic pathways. It is downregulated in AD patients, compared to controls (Wu et al., 2020). In line with this, in vitro models showed that miR-15b inhibits the expression of BACE1 and APP (Li and Wang, 2018; Liu et al., 2019).
AGE-RAGE signaling pathway
AGEs are proteins or lipids that are glycated with sugar molecules. They have been linked with AD, such that patients exhibit increased levels of AGEs compared to healthy controls (Cai et al., 2016). AGEs interact with their multi-ligand cell receptors RAGE, which leads to the formation of reactive oxygen species (ROS). Due to the role of AGE-RAGE signaling in the formation of ROS, a link between AD and diabetes has been hypothesized. This association may be due to various factors, including insulin resistance, chronic inflammation, impaired glucose metabolism, and oxidative stress. The blood brain barrier is disrupted by the formation of ROS, which results in dysfunction and stimulates neuroinflammation that may progress to AD (Dhapola et al., 2024).
The glycation of proteins and sugars contribute to the development of age-related AD by triggering the AGE-RAGE axis, promoting synaptic dysfunction, and triggering oxidative stress response. An accumulation of AGEs, due to the imbalance in its generation and clearance, has been found to affect the permeability of the mitochondrial membrane, effectively resulting in oxidative stress (Dhapola et al., 2024).
An increased level of AGEs were also correlated with decreased cognitive and functional performance (Cai et al., 2016; Prasad, 2019). Imaging studies have found that AGEs localize in the senile plaques and extracellular spaces in AD patients (Khoury and Luster, 2008). The let-7b-5p miRNA mediates apoptosis caused by hypoxic spinal cord injury (Piscopo et al., 2018). It has been found to be downregulated in a study involving blood biomarkers in AD patients (Leidinger et al., 2013).
Conclusion
This systematic review emphasizes the pivotal role of ncRNAs in the molecular pathology of AD, underscoring their potential as biomarkers and therapeutic targets. As the field of ncRNA research continues to develop, it holds the promise of unveiling novel strategies for the diagnosis, treatment, and management of AD, contributing to better outcomes for individuals affected by this devastating neurodegenerative disorder. The findings from this systematic review suggest that ncRNAs hold promise not only as biomarkers for early detection and diagnosis of AD but also as novel therapeutic targets. Although, our study is limited by several factors. The AD diagnosis criteria, AD type, and technology used varied across the studies included in the review. The ethnicity of the participants also varied, potentially affecting the generalizability of the results. Additionally, there was a noticeable difference in the number of studies involving miRNAs compared to lncRNAs, circRNAs, and piRNAs. As such, future studies can highlight the association of lncRNAs, circRNAs, and piRNAs with the risk of AD. More detailed studies on how ncRNAs are involved in AD are needed to identify the role of these potential biomarkers as predictive and therapeutic targets. Identifying the mechanism of action of ncRNAs is also crucial to identify how they can serve as treatment targets in the long run. As seen in this review, the identification of specific ncRNAs associated with AD pathogenesis, such as those involved in the Activin Receptor Signaling Pathway, Actomyosin Contractile Ring Organization, and AGE-RAGE Signaling Pathway, provides valuable insights into the molecular mechanisms driving the disease. These insights pave the way for the development of targeted interventions that could potentially modulate the activity of these ncRNAs, thereby offering new avenues for the treatment and management of AD. Furthermore, the systematic review highlights the importance of advancing our understanding of ncRNAs in AD through further research. It is imperative to validate the roles of these ncRNAs in larger and more diverse populations to strengthen their utility as clinical biomarkers and therapeutic agents. Additionally, exploring the intricate network of interactions among different types of ncRNAs and their combined impact on AD pathology could unveil new dimensions of the disease mechanism.
Data availability statement
The original contributions presented in the study are included in the article/Supplementary material, further inquiries can be directed to the corresponding author.
Author contributions
RC: Conceptualization, Data curation, Formal analysis, Funding acquisition, Investigation, Methodology, Project administration, Resources, Supervision, Validation, Writing – original draft, Writing – review & editing. JSy: Conceptualization, Data curation, Formal analysis, Investigation, Methodology, Project administration, Supervision, Validation, Writing – original draft, Writing – review & editing. CD: Methodology, Writing – review & editing. CC: Methodology, Writing – review & editing. LD: Methodology, Writing – review & editing. BP: Methodology, Writing – review & editing. WP: Methodology, Writing – review & editing. JY: Conceptualization, Methodology, Writing – review & editing. JV: Conceptualization, Methodology, Writing – review & editing. JSe: Conceptualization, Methodology, Writing – review & editing. AG: Funding acquisition, Writing – review & editing.
Funding
The author(s) declare that financial support was received for the research, authorship, and/or publication of this article. This systematic review received support from the research funds of SciLore, LLC. The funder was not involved in the study design, collection, analysis, interpretation of data, the writing of this article, or the decision to submit it for publication.
Acknowledgments
The authors are grateful for the technical support of Lystra Dayapera for her help in collating and summarizing the data.
Conflict of interest
RC, JSy, and AG were employed by SciLore LLC.
The remaining authors declare that the research was conducted in the absence of any commercial or financial relationships that could be construed as a potential conflict of interest.
Publisher’s note
All claims expressed in this article are solely those of the authors and do not necessarily represent those of their affiliated organizations, or those of the publisher, the editors and the reviewers. Any product that may be evaluated in this article, or claim that may be made by its manufacturer, is not guaranteed or endorsed by the publisher.
Supplementary material
The Supplementary material for this article can be found online at: https://www.frontiersin.org/articles/10.3389/fnins.2024.1421675/full#supplementary-material
SUPPLEMENTARY FIGURE 1 Number of independent original published studies on non-coding RNAs (ncRNAs) with respect to Alzheimer’s disease (AD) from 2007 to 2023. From only two studies in 2007, the number of studies has increased to more than 25 from 2020 onwards, highlighting the increasing awareness and potential of ncRNAs as possible biomarkers or therapeutic targets for AD.
SUPPLEMENTARY FIGURE 2 Total number of cases and control participants of the included studies according to age. Whether case or control, most of the participants in the study population of the included studies in this systematic review were more than 65 years old.
SUPPLEMENTARY TABLE 1 Keywords and search items used for each database.
SUPPLEMENTARY TABLE 2 Compiled studies included in this systematic review.
SUPPLEMENTARY TABLE 3 Compiled unique ncRNAs that are associated with Alzheimer’s disease.
SUPPLEMENTARY TABLE 4 Pathway enrichment analysis of gene targets of the top miRNAs.
References
Alzheimer’s Association (2023). 2023 Alzheimer’s disease facts and figures. Alzheimers Dement. 19, 1598–1695. doi: 10.1002/alz.13016
Alzheimer’s Disease and Dementia. (2023). What Is Dementia? Available at: https://alz.org/alzheimers-dementia/what-is-dementia
Andrade, A., Siqueira, T. C., D’Oliveira, A., and Dominski, F. H. (2022). Effects of exercise in the treatment of Alzheimer’s disease: an umbrella review of systematic reviews and Meta-analyses. J. Aging Phys. Act. 30, 535–551. doi: 10.1123/japa.2021-0033
Andrade-Guerrero, J., Santiago-Balmaseda, A., Jeronimo-Aguilar, P., Vargas-Rodríguez, I., Cadena-Suárez, A. R., Sánchez-Garibay, C., et al. (2023). Alzheimer’s disease: an updated overview of its genetics. Int. J. Mol. Sci. 24:3754. doi: 10.3390/ijms24043754
Asadi, M. R., Hassani, M., Kiani, S., Sabaie, H., Moslehian, M. S., Kazemi, M., et al. (2021). The perspective of dysregulated LncRNAs in Alzheimer’s disease: a systematic scoping review. Front. Aging Neurosci. 13:568. doi: 10.3389/fnagi.2021.709568
Bamburg, J. R., Minamide, L. S., Wiggan, O., Tahtamouni, L. H., and Kuhn, T. B. (2021). Cofilin and actin dynamics: multiple modes of regulation and their impacts in neuronal development and degeneration. Cells 10:2726. doi: 10.3390/cells10102726
Bloudek, L. M., Spackman, D. E., Blankenburg, M., and Sullivan, S. D. (2011). Review and Meta-analysis of biomarkers and diagnostic imaging in Alzheimer’s disease. J. Alzheimers Dis. 26, 627–645. doi: 10.3233/JAD-2011-110458
Cai, Z., Liu, N., Wang, C., Qin, B., Zhou, Y., Xiao, M., et al. (2016). Role of RAGE in Alzheimer’s disease. Cell. Mol. Neurobiol. 36, 483–495. doi: 10.1007/s10571-015-0233-3
Cao, J., Huang, M., Guo, L., Zhu, L., Hou, J., Zhang, L., et al. (2021). MicroRNA-195 rescues ApoE4-induced cognitive deficits and lysosomal defects in Alzheimer’s disease pathogenesis. Mol. Psychiatry 26, 4687–4701. doi: 10.1038/s41380-020-0824-3
Chen, C., and Fang, F. (2023). hsa_circ_0006916 exerts effect on amyloid Beta-induced neuron injury by targeting miR-217/HOMER1. Ann. Clin. Lab. Sci. 53, 181–191.
Chen, L., Guo, X., Li, Z., and He, Y. (2019). Relationship between long non-coding RNAs and Alzheimer’s disease: a systematic review. Pathol. Res. Pract. 215, 12–20. doi: 10.1016/j.prp.2018.11.012
Cheng, Y., Zhou, X., Zou, T., Zhang, L., Li, L., Yang, C., et al. (2023). Plasma long non-coding RNAs ASMTL-AS1, AP001363.1, AC005730.3 and AL133415.1 as a potential biomarker for Alzheimer’s disease. Neurol. Res. 45, 804–817. doi: 10.1080/01616412.2023.2203616
Dakterzada, F., Targa, A., Benítez, I. D., Romero-ElKhayat, L., de Gonzalo-Calvo, D., Torres, G., et al. (2020). Identification and validation of endogenous control miRNAs in plasma samples for normalization of qPCR data for Alzheimer’s disease. Alzheimers Res Ther 12:163. doi: 10.1186/s13195-020-00735-x
David, M. C. B., Giovane, M. D., Liu, K. Y., Gostick, B., Rowe, J. B., Oboh, I., et al. (2022). Cognitive and neuropsychiatric effects of noradrenergic treatment in Alzheimer’s disease: systematic review and meta-analysis. J. Neurol. Neurosurg. Psychiatry 93, 1080–1090. doi: 10.1136/jnnp-2022-329136
De Berdt, P., Bottemanne, P., Bianco, J., Alhouayek, M., Diogenes, A., Llyod, A., et al. (2018). Stem cells from human apical papilla decrease neuro-inflammation and stimulate oligodendrocyte progenitor differentiation via activin-a secretion. Cell. Mol. Life Sci. 75, 2843–2856. doi: 10.1007/s00018-018-2764-5
Demurtas, J., Schoene, D., Torbahn, G., Marengoni, A., Grande, G., Zou, L., et al. (2020). Physical activity and exercise in mild cognitive impairment and dementia: an umbrella review of intervention and observational studies. J. Am. Med. Dir. Assoc. 21, 1415–1422.e6. doi: 10.1016/j.jamda.2020.08.031
Derkow, K., Rössling, R., Schipke, C., Krüger, C., Bauer, J., Fähling, M., et al. (2018). Distinct expression of the neurotoxic microRNA family let-7 in the cerebrospinal fluid of patients with Alzheimer’s disease. PLoS One 13:e0200602. doi: 10.1371/journal.pone.0200602
Dhanoa, J. K., Sethi, R. S., Verma, R., Arora, J. S., and Mukhopadhyay, C. S. (2018). Long non-coding RNA: its evolutionary relics and biological implications in mammals: a review. J Anim Sci Technol. 60:25. doi: 10.1186/s40781-018-0183-7
Dhapola, R., Beura, S. K., Sharma, P., Singh, S. K., and HariKrishnaReddy, D. (2024). Oxidative stress in Alzheimer’s disease: current knowledge of signaling pathways and therapeutics. Mol. Biol. Rep. 51:48. doi: 10.1007/s11033-023-09021-z
Ding, Y., Luan, W., Shen, X., Wang, Z., and Cao, Y. (2022). LncRNA BDNF-AS AS ceRNA regulates the miR-9-5p/BACE1 pathway affecting neurotoxicity in Alzheimer’s disease. Arch. Gerontol. Geriatr. 99:104614. doi: 10.1016/j.archger.2021.104614
Etminan, M., Gill, S., and Samii, A. (2003). Effect of non-steroidal anti-inflammatory drugs on risk of Alzheimer’s disease: systematic review and meta-analysis of observational studies. BMJ 327, 128–120. doi: 10.1136/bmj.327.7407.128
Feng, L., Liao, Y. T., He, J. C., Xie, C. L., Chen, S. Y., Fan, H. H., et al. (2018). Plasma long non-coding RNA BACE1 as a novel biomarker for diagnosis of Alzheimer disease. BMC Neurol. 18:4. doi: 10.1186/s12883-017-1008-x
Ferreira, L. K., Diniz, B. S., Forlenza, O. V., Busatto, G. F., and Zanetti, M. V. (2011). Neurostructural predictors of Alzheimer’s disease: a meta-analysis of VBM studies. Neurobiol. Aging 32, 1733–1741. doi: 10.1016/j.neurobiolaging.2009.11.008
Greco, S., Piella, S. N., Made', A., Tascini, A. S., Garcia-Manteiga, J. M., Castelvecchio, S., et al. (2022). LncRNA BACE1-AS: a link between heart failure and Alzheimer’s disease. Eur. Heart J. 43:2945. doi: 10.1093/eurheartj/ehac544.2945
Guan, F., Gao, Q., Dai, X., Li, L., Bao, R., and Gu, J. (2022). LncRNA RP11-59J16.2 aggravates apoptosis and increases tau phosphorylation by targeting MCM2 in AD. Front. Genet. 13:824495. doi: 10.3389/fgene.2022.824495
Guévremont, D., Tsui, H., Knight, R., Fowler, C. J., Masters, C. L., Martins, R. N., et al. (2022). Plasma microRNA vary in association with the progression of Alzheimer’s disease. Alzheimers Dement. 14:e12251. doi: 10.1002/dad2.12251
Hadar, A., Milanesi, E., Walczak, M., Puzianowska-Kuźnicka, M., Kuźnicki, J., Squassina, A., et al. (2018). SIRT1, miR-132 and miR-212 link human longevity to Alzheimer’s disease. Sci. Rep. 8:8465. doi: 10.1038/s41598-018-26547-6
Hébert, S. S., Horré, K., Nicolaï, L., Bergmans, B., Papadopoulou, A. S., Delacourte, A., et al. (2009). MicroRNA regulation of Alzheimer’s amyloid precursor protein expression. Neurobiol. Dis. 33, 422–428. doi: 10.1016/j.nbd.2008.11.009
Hu, Y. B., Li, C. B., Song, N., Zou, Y., Chen, S. D., Ren, R. J., et al. (2016). Diagnostic value of microRNA for Alzheimer’s disease: a systematic review and Meta-analysis. Front. Aging Neurosci. 8:13. doi: 10.3389/fnagi.2016.00013
Huang, Z., Zhao, J., Wang, W., Zhou, J., and Zhang, J. (2020). Depletion of LncRNA NEAT1 rescues mitochondrial dysfunction through NEDD4L-dependent PINK1 degradation in animal models of Alzheimer’s disease. Front. Cell. Neurosci. 14:28. doi: 10.3389/fncel.2020.00028
Hyde, C., Peters, J., Bond, M., Rogers, G., Hoyle, M., Anderson, R., et al. (2013). Evolution of the evidence on the effectiveness and cost-effectiveness of acetylcholinesterase inhibitors and memantine for Alzheimer’s disease: systematic review and economic model†. Age Ageing 42, 14–20. doi: 10.1093/ageing/afs165
Idda, M. L., Munk, R., Abdelmohsen, K., and Gorospe, M. (2018). Noncoding RNAs in Alzheimer’s disease. WIREs RNA 9:1463. doi: 10.1002/wrna.1463
Jack, C. R., Bennett, D. A., Blennow, K., Carrillo, M. C., Dunn, B., Haeberlein, S. B., et al. (2018). NIA-AA research framework: toward a biological definition of Alzheimer’s disease. Alzheimers Dement. 14, 535–562. doi: 10.1016/j.jalz.2018.02.018
Jain, G., Stuendl, A., Rao, P., Berulava, T., Pena Centeno, T., Kaurani, L., et al. (2019). A combined miRNA–piRNA signature to detect Alzheimer’s disease. Transl. Psychiatry 9, 1–12. doi: 10.1038/s41398-019-0579-2
Kaduszkiewicz, H., Zimmermann, T., Beck-Bornholdt, H. P., and van den Bussche, H. (2005). Cholinesterase inhibitors for patients with Alzheimer’s disease: systematic review of randomised clinical trials. BMJ 331, 321–327. doi: 10.1136/bmj.331.7512.321
Kafshdooz, T., Farajnia, S., Sharifi, R., and Najmi, S. (2023). Hsa-let-7g-5p, a circulating microRNA, as a biomarker for Alzheimer’s disease. Informat Med Unlock. 38:101203. doi: 10.1016/j.imu.2023.101203
Kang, M. J., Abdelmohsen, K., Hutchison, E. R., Mitchell, S. J., Grammatikakis, I., Guo, R., et al. (2014). HuD regulates coding and noncoding RNA to induce APP→Aβ processing. Cell Rep. 7, 1401–1409. doi: 10.1016/j.celrep.2014.04.050
Kang, D. E., and Woo, J. A. (2019). Cofilin, a master node regulating cytoskeletal pathogenesis in Alzheimer’s disease. J. Alzheimers Dis. 72, S131–S144. doi: 10.3233/JAD-190585
Ke, S., Yang, Z., Yang, F., Wang, X., Tan, J., and Liao, B. (2019). Long noncoding RNA NEAT1 aggravates Aβ-induced neuronal damage by targeting miR-107 in Alzheimer’s disease. Yonsei Med. J. 60, 640–650. doi: 10.3349/ymj.2019.60.7.640
Khoury, J. E., and Luster, A. D. (2008). Mechanisms of microglia accumulation in Alzheimer’s disease: therapeutic implications. Trends Pharmacol. Sci. 29, 626–632. doi: 10.1016/j.tips.2008.08.004
Kim, J., Yoon, H., Chung, D., Brown, J. L., Belmonte, K. C., and Kim, J. (2016). miR-186 is decreased in aged brain and suppresses BACE1 expression. J. Neurochem. 137, 436–445. doi: 10.1111/jnc.13507
Komatsu, S., Kitai, H., and Suzuki, H. I. (2023). Network regulation of microRNA biogenesis and target interaction. Cells 12:306. doi: 10.3390/cells12020306
Kristensen, L. S., Andersen, M. S., Stagsted, L. V. W., Ebbesen, K. K., Hansen, T. B., and Kjems, J. (2019). The biogenesis, biology and characterization of circular RNAs. Nat. Rev. Genet. 20, 675–691. doi: 10.1038/s41576-019-0158-7
Kumar, S., and Reddy, P. H. (2016). Are circulating microRNAs peripheral biomarkers for Alzheimer's disease? Biochim. Biophys. Acta Mol. Basis Dis. 1862, 1617–1627. doi: 10.1016/j.bbadis.2016.06.001
Kumar, S., and Reddy, P. H. (2018). MicroRNA-455-3p as a potential biomarker for Alzheimer’s disease: an update. Front. Aging Neurosci. 10:41. doi: 10.3389/fnagi.2018.00041
Lei, X., Lei, L., Zhang, Z., Zhang, Z., and Cheng, Y. (2015). Downregulated miR-29c correlates with increased BACE1 expression in sporadic Alzheimer’s disease. Int. J. Clin. Exp. Pathol. 8, 1565–1574.
Leidinger, P., Backes, C., Deutscher, S., Schmitt, K., Mueller, S. C., Frese, K., et al. (2013). A blood based 12-miRNA signature of Alzheimer disease patients. Genome Biol. 14:R78. doi: 10.1186/gb-2013-14-7-r78
Li, Y., Cheng, Z., Yu, F., Zhang, Q., Yu, S., Ding, F., et al. (2022). Activin a secreted from peripheral nerve fibroblasts promotes proliferation and migration of Schwann cells. Front Mol Neurosci 15:349. doi: 10.3389/fnmol.2022.859349
Li, Y., Fan, H., Ni, M., Zhang, W., Fang, F., Sun, J., et al. (2023). Targeting lncRNA NEAT1 hampers Alzheimer’s disease progression. Neuroscience 529, 88–98. doi: 10.1016/j.neuroscience.2023.02.016
Li, Y., Fan, H., Sun, J., Ni, M., Zhang, L., Chen, C., et al. (2020). Circular RNA expression profile of Alzheimer’s disease and its clinical significance as biomarkers for the disease risk and progression. Int. J. Biochem. Cell Biol. 123:105747. doi: 10.1016/j.biocel.2020.105747
Li, X., Feng, X., Sun, X., Hou, N., Han, F., and Liu, Y. (2022). Global, regional, and national burden of Alzheimer’s disease and other dementias, 1990–2019. Front. Aging Neurosci. 14:937486. doi: 10.3389/fnagi.2022.937486
Li, L., Jin, M., Tan, J., and Xiao, B. (2023). NcRNAs: A synergistically antiapoptosis therapeutic tool in Alzheimer’s disease. CNS Neurosci. Ther. 30:e14476. doi: 10.1111/cns.14476
Li, J., and Wang, H. (2018). miR-15b reduces amyloid-β accumulation in SH-SY5Y cell line through targetting NF-κB signaling and BACE1. Biosci. Rep. 38:51. doi: 10.1042/BSR20180051
Link, A. S., Zheng, F., and Alzheimer, C. (2016). Activin signaling in the pathogenesis and therapy of neuropsychiatric diseases. Front Mol Neurosci 9:32. doi: 10.3389/fnmol.2016.00032
Liu, Y., Cheng, X., Li, H., Hui, S., Zhang, Z., Xiao, Y., et al. (2022). Non-coding RNAs as novel regulators of Neuroinflammation in Alzheimer’s disease. Front. Immunol. 13:8076. doi: 10.3389/fimmu.2022.908076
Liu, Y., Ding, W., Wang, J., Ao, X., and Xue, J. (2023). Non-coding RNAs in lung cancer: molecular mechanisms and clinical applications. Front. Oncol. 13:1256537. doi: 10.3389/fonc.2023.1256537
Liu, S., Fan, M., Zheng, Q., Hao, S., Yang, L., Xia, Q., et al. (2022). MicroRNAs in Alzheimer’s disease: potential diagnostic markers and therapeutic targets. Biomed. Pharmacother. 148:112681. doi: 10.1016/j.biopha.2022.112681
Liu, H. Y., Fu, X., Li, Y. F., Li, X. L., Ma, Z. Y., Zhang, Y., et al. (2019). miR-15b-5p targeting amyloid precursor protein is involved in the anti-amyloid eflect of curcumin in swAPP695-HEK293 cells. Neural Regen. Res. 14, 1603–1609. doi: 10.4103/1673-5374.255979
Liu, Y., Yu, J. T., Wang, H. F., Han, P. R., Tan, C. C., Wang, C., et al. (2015). APOE genotype and neuroimaging markers of Alzheimer’s disease: systematic review and meta-analysis. J. Neurol. Neurosurg. Psychiatry 86, 127–134. doi: 10.1136/jnnp-2014-307719
Liu, D. Y., and Zhang, L. (2019). MicroRNA-132 promotes neurons cell apoptosis and activates tau phosphorylation by targeting GTDC-1 in Alzheimer’s disease. Eur. Rev. Med. Pharmacol. Sci. 23, 8523–8532. doi: 10.26355/eurrev_201910_19166
Loganathan, T., and Doss, C. G. P. (2023). Non-coding RNAs in human health and disease: potential function as biomarkers and therapeutic targets. Funct. Integr. Genomics 23:33. doi: 10.1007/s10142-022-00947-4
Long, J. M., and Holtzman, D. M. (2019). Alzheimer disease: an update on pathobiology and treatment strategies. Cell 179, 312–339. doi: 10.1016/j.cell.2019.09.001
Lopes da Silva, S., Vellas, B., Elemans, S., Luchsinger, J., Kamphuis, P., Yaffe, K., et al. (2014). Plasma nutrient status of patients with Alzheimer’s disease: systematic review and meta-analysis. Alzheimers Dement. 10, 485–502. doi: 10.1016/j.jalz.2013.05.1771
López-Ortiz, S., Lista, S., Valenzuela, P. L., Pinto-Fraga, J., Carmona, R., Caraci, F., et al. (2023). Effects of physical activity and exercise interventions on Alzheimer’s disease: an umbrella review of existing meta-analyses. J. Neurol. 270, 711–725. doi: 10.1007/s00415-022-11454-8
Lu, L., Dai, W. Z., Zhu, X. C., and Ma, T. (2021). Analysis of serum miRNAs in Alzheimer’s disease. Am. J. Alzheimers Dis. Other Demen. 36:153331752110217. doi: 10.1177/15333175211021712
Lu, L., Zheng, X., Wang, S., Tang, C., Zhang, Y., Yao, G., et al. (2020). Anti-Aβ agents for mild to moderate Alzheimer’s disease: systematic review and meta-analysis. J. Neurol. Neurosurg. Psychiatry 91, 1316–1324. doi: 10.1136/jnnp-2020-323497
Lukiw, W. J. (2007). Micro-RNA speciation in fetal, adult and Alzheimer’s disease hippocampus. Neuroreport 18, 297–300. doi: 10.1097/WNR.0b013e3280148e8b
Luo, Q., and Chen, Y. (2016). Long noncoding RNAs and Alzheimer’s disease. Clin. Interv. Aging 11, 867–872. doi: 10.2147/CIA.S107037
Mao, Q., Fan, L., Wang, X., Lin, X., Cao, Y., Zheng, C., et al. (2019). Transcriptome-wide piRNA profiling in human brains for aging genetic factors. Jacobs J Genet. 4:014.
Maoz, R., Garfinkel, B. P., and Soreq, H. (2017). “Alzheimer’s disease and ncRNAs” in Neuroepigenomics in aging and disease [internet]. ed. R. Delgado-Morales (Cham: Springer International Publishing), 337–361.
McKhann, G., Drachman, D., Folstein, M., Katzman, R., Price, D., and Stadlan, E. M. (1984). Clinical diagnosis of Alzheimer’s disease: report of the NINCDS-ADRDA work group* under the auspices of Department of Health and Human Services Task Force on Alzheimer’s disease. Neurology 34:939. doi: 10.1212/WNL.34.7.939
Mendez, M. F. (2019). Early-onset Alzheimer disease and its variants. Continuum 25, 34–51. doi: 10.1212/CON.0000000000000687
Modarresi, F., Faghihi, M. A., Patel, N. S., Sahagan, B. G., Wahlestedt, C., and Lopez-Toledano, M. A. (2011). Knockdown of BACE1-AS nonprotein-coding transcript modulates Beta-amyloid-related hippocampal neurogenesis. Int. J. Alzheimers Dis. 2011:e929042, 1–11. doi: 10.4061/2011/929042
Muñoz Fernández, S. S., Ivanauskas, T., and Lima Ribeiro, S. M. (2017). Nutritional strategies in the Management of Alzheimer Disease: systematic review with network Meta-analysis. J. Am. Med. Dir. Assoc. 18, 897.e13–897.e30. doi: 10.1016/j.jamda.2017.06.015
National Institute on Aging. (2022). What Is Dementia? Symptoms, Types, and Diagnosis. Available at: https://www.nia.nih.gov/health/what-is-dementia
Nikolac Perkovic, M., Videtic Paska, A., Konjevod, M., Kouter, K., Svob Strac, D., Nedic Erjavec, G., et al. (2021). Epigenetics of Alzheimer’s disease. Biomol. Ther. 11:195. doi: 10.3390/biom11020195
Nisar, S., Bhat, A. A., Singh, M., Karedath, T., Rizwan, A., Hashem, S., et al. (2021). Insights into the role of CircRNAs: biogenesis, characterization, functional, and clinical impact in human malignancies. Front. Cell Dev. Biol. 9:7281. doi: 10.3389/fcell.2021.617281
Niu, H., Álvarez-Álvarez, I., Guillén-Grima, F., and Aguinaga-Ontoso, I. (2017). Prevalence and incidence of Alzheimer’s disease in Europe: a meta-analysis. Neurologia 32, 523–532. doi: 10.1016/j.nrleng.2016.02.009
Noor Eddin, A., Hamsho, K., Adi, G., Al-Rimawi, M., Alfuwais, M., Abdul Rab, S., et al. (2023). Cerebrospinal fluid microRNAs as potential biomarkers in Alzheimer’s disease. Front. Aging Neurosci. 15:1210191. doi: 10.3389/fnagi.2023.1210191
Olufunmilayo, E. O., and Holsinger, R. M. D. (2023). Roles of non-coding RNA in Alzheimer’s disease pathophysiology. Int. J. Mol. Sci. 24:12498. doi: 10.3390/ijms241512498
Ou, Y. N., Zhu, J. X., Hou, X. H., Shen, X. N., Xu, W., Dong, Q., et al. (2020). Associations of infectious agents with Alzheimer’s disease: a systematic review and Meta-analysis. J. Alzheimers Dis. 75, 299–309. doi: 10.3233/JAD-191337
Page, M. J., McKenzie, J. E., Bossuyt, P. M., Boutron, I., Hoffmann, T. C., Mulrow, C. D., et al. (2021). The PRISMA 2020 statement: an updated guideline for reporting systematic reviews. Int. J. Surg. 88:105906. doi: 10.1016/j.ijsu.2021.105906
Pan, W., Hu, Y., Wang, L., and Li, J. (2022). Circ_0003611 acts as a miR-885-5p sponge to aggravate the amyloid-β-induced neuronal injury in Alzheimer’s disease. Metab. Brain Dis. 37, 961–971. doi: 10.1007/s11011-022-00912-x
Park, S. E., Lee, J., Chang, E. H., Kim, J. H., Sung, J. H., Na, D. L., et al. (2016). Activin a secreted by human mesenchymal stem cells induces neuronal development and neurite outgrowth in an in vitro model of Alzheimer’s disease: neurogenesis induced by MSCs via activin a. Arch. Pharm. Res. 39, 1171–1179. doi: 10.1007/s12272-016-0799-4
Parsi, S., Smith, P. Y., Goupil, C., Dorval, V., and Hébert, S. S. (2015). Preclinical evaluation of miR-15/107 family members as multifactorial drug targets for Alzheimer’s disease. Nucleic acids 4:e256. doi: 10.1038/mtna.2015.33
Pierouli, K., Papakonstantinou, E., Papageorgiou, L., Diakou, I., Mitsis, T., Dragoumani, K., et al. (2023). Role of non-coding RNAs as biomarkers and the application of omics technologies in Alzheimer’s disease (review). Int. J. Mol. Med. 51, 1–11. doi: 10.3892/ijmm.2022.5208
Piscopo, P., Grasso, M., Puopolo, M., D’Acunto, E., Talarico, G., Crestini, A., et al. (2018). Circulating miR-127-3p as a potential biomarker for differential diagnosis in frontotemporal dementia. J. Alzheimers Dis. 65, 455–464. doi: 10.3233/JAD-180364
Prasad, K. (2019). AGE–RAGE stress: a changing landscape in pathology and treatment of Alzheimer’s disease. Mol. Cell. Biochem. 459, 95–112. doi: 10.1007/s11010-019-03553-4
Qiu, W., Guo, X., Lin, X., Yang, Q., Zhang, W., Zhang, Y., et al. (2017). Transcriptome-wide piRNA profiling in human brains of Alzheimer’s disease. Neurobiol. Aging 57, 170–177. doi: 10.1016/j.neurobiolaging.2017.05.020
Rahman, M. R., Islam, T., Zaman, T., Shahjaman, M., Karim, M. R., Huq, F., et al. (2020). Identification of molecular signatures and pathways to identify novel therapeutic targets in Alzheimer’s disease: insights from a systems biomedicine perspective. Genomics 112, 1290–1299. doi: 10.1016/j.ygeno.2019.07.018
Roy, J., Sarkar, A., Parida, S., Ghosh, Z., and Mallick, B. (2017). Small RNA sequencing revealed dysregulated piRNAs in Alzheimer’s disease and their probable role in pathogenesis. Mol. Biosyst. 13, 565–576. doi: 10.1039/C6MB00699J
Ru, Y., Kechris, K. J., Tabakoff, B., Hoffman, P., Radcliffe, R. A., Bowler, R., et al. (2014). The multiMiR R package and database: integration of microRNA–target interactions along with their disease and drug associations. Nucleic Acids Res. 42:e133. doi: 10.1093/nar/gku631
Sato, K., Takayama, K., and Inoue, S. (2023). Role of piRNA biogenesis and its neuronal function in the development of neurodegenerative diseases. Front. Aging Neurosci. 15:1157818. doi: 10.3389/fnagi.2023.1157818
Scheltens, P., Blennow, K., Breteler, M. M. B., de Strooper, B., Frisoni, G. B., Salloway, S., et al. (2016). Alzheimer’s disease. Lancet 388, 505–517. doi: 10.1016/S0140-6736(15)01124-1
Seath, P., Macedo-Orrego, L. E., and Velayudhan, L. (2023). Clinical characteristics of early-onset versus late-onset Alzheimer’s disease: a systematic review and meta-analysis. Int. Psychogeriatr. 11, 1–17. doi: 10.1017/S1041610223000509
Shobeiri, P., Alilou, S., Jaberinezhad, M., Zare, F., Karimi, N., Maleki, S., et al. (2023). Circulating long non-coding RNAs as novel diagnostic biomarkers for Alzheimer’s disease (AD): a systematic review and meta-analysis. PLoS One 18:e0281784. doi: 10.1371/journal.pone.0281784
Sohani, Z. N., Meyre, D., de Souza, R. J., Joseph, P. G., Gandhi, M., Dennis, B. B., et al. (2015). Assessing the quality of published genetic association studies in meta-analyses: the quality of genetic studies (Q-genie) tool. BMC Genet. 16:50. doi: 10.1186/s12863-015-0211-2
Song, C., Zhang, Y., Huang, W., Shi, J., Huang, Q., Jiang, M., et al. (2022). Circular RNA Cwc27 contributes to Alzheimer’s disease pathogenesis by repressing Pur-α activity. Cell Death Differ. 29, 393–406. doi: 10.1038/s41418-021-00865-1
Stephen, R., Hongisto, K., Solomon, A., and Lönnroos, E. (2017). Physical activity and Alzheimer’s disease: a systematic review. J Gerontol Series A. 72, glw251–glw259. doi: 10.1093/gerona/glw251
Swarbrick, S., Wragg, N., Ghosh, S., and Stolzing, A. (2019). Systematic review of miRNA as biomarkers in Alzheimer’s disease. Mol. Neurobiol. 56, 6156–6167. doi: 10.1007/s12035-019-1500-y
Szklarczyk, D., Kirsch, R., Koutrouli, M., Nastou, K., Mehryary, F., Hachilif, R., et al. (2023). The STRING database in 2023: protein–protein association networks and functional enrichment analyses for any sequenced genome of interest. Nucleic Acids Res. 51, D638–D646. doi: 10.1093/nar/gkac1000
Torres Cleuren, Y., and Boonstra, J. (2012). Actin: Structure, function and disease. Netherlands: Nova Science Publishers, Inc, 61–96.
Uddin, M. S., Hasana, S., Hossain, M. F., Islam, M. S., Behl, T., Perveen, A., et al. (2021). Molecular genetics of early- and late-onset Alzheimer’s disease. Curr. Gene Ther. 21, 43–52. doi: 10.2174/1566523220666201123112822
Wimo, A., Seeher, K., Cataldi, R., Cyhlarova, E., Dielemann, J. L., Frisell, O., et al. (2023). The worldwide costs of dementia in 2019. Alzheimers Dement. 19, 2865–2873. doi: 10.1002/alz.12901
World Health Organization. (2023). Dementia. Available at: https://www.who.int/news-room/fact-sheets/detail/dementia
Wu, L., Du, Q., and Wu, C. (2021). CircLPAR1/miR-212-3p/ZNF217 feedback loop promotes amyloid β-induced neuronal injury in Alzheimer’s disease. Brain Res. 1770:147622. doi: 10.1016/j.brainres.2021.147622
Wu, H. Z. Y., Ong, K. L., Seeher, K., Armstrong, N. J., Thalamuthu, A., Brodaty, H., et al. (2015). Circulating microRNAs as biomarkers of Alzheimer’s disease: a systematic review. J. Alzheimers Dis. 49, 755–766. doi: 10.3233/JAD-150619
Wu, H. Z. Y., Thalamuthu, A., Cheng, L., Fowler, C., Masters, C. L., Sachdev, P., et al. (2020). Differential blood miRNA expression in brain amyloid imaging-defined Alzheimer’s disease and controls. Alzheimers Res. Ther. 12:59. doi: 10.1186/s13195-020-00627-0
Xu Lou, I., Ali, K., and Chen, Q. (2023). Effect of nutrition in Alzheimer’s disease: a systematic review. Front. Neurosci. 17:1147177. doi: 10.3389/fnins.2023.1147177
Yan, X. W., Liu, H. J., Hong, Y. X., Meng, T., Du, J., and Chang, C. (2022). lncRNA XIST induces Aβ accumulation and neuroinflammation by the epigenetic repression of NEP in Alzheimer’s disease. J. Neurogenet. 36, 11–20. doi: 10.1080/01677063.2022.2028784
Yoon, S., Kim, S. E., Ko, Y., Jeong, G. H., Lee, K. H., Lee, J., et al. (2022). Differential expression of MicroRNAs in Alzheimer’s disease: a systematic review and meta-analysis. Mol. Psychiatry 27, 2405–2413. doi: 10.1038/s41380-022-01476-z
Yue, D., Guanqun, G., Jingxin, L., Sen, S., Shuang, L., Yan, S., et al. (2020). Silencing of long noncoding RNA XIST attenuated Alzheimer’s disease-related BACE1 alteration through miR-124. Cell Biol. Int. 44, 630–636. doi: 10.1002/cbin.11263
Yusufov, M., Weyandt, L. L., and Piryatinsky, I. (2017). Alzheimer’s disease and diet: a systematic review. Int. J. Neurosci. 127, 161–175. doi: 10.3109/00207454.2016.1155572
Zeng, T., Ni, H., Yu, Y., Zhang, M., Wu, M., Wang, Q., et al. (2019). BACE1-AS prevents BACE1 mRNA degradation through the sequestration of BACE1-targeting miRNAs. J. Chem. Neuroanat. 98, 87–96. doi: 10.1016/j.jchemneu.2019.04.001
Zhang, Z. (2016). Long non-coding RNAs in Alzheimer’s disease. Curr. Top. Med. Chem. 16, 511–519. doi: 10.2174/1568026615666150813142956
Zhang, Y. H., Bai, S. F., and Yan, J. Q. (2019). Blood circulating miRNAs as biomarkers of Alzheimer’s disease: a systematic review and meta-analysis. Biomark. Med. 13, 1045–1054. doi: 10.2217/bmm-2018-0341
Zhang, M., and Bian, Z. (2021). The emerging role of circular RNAs in Alzheimer’s disease and Parkinson’s disease. Front. Aging Neurosci. 13:512. doi: 10.3389/fnagi.2021.691512
Zhang, Y., Zhao, Y., Ao, X., Yu, W., Zhang, L., Wang, Y., et al. (2021). The role of non-coding RNAs in Alzheimer’s disease: from regulated mechanism to therapeutic targets and diagnostic biomarkers. Front. Aging Neurosci. 13:978. doi: 10.3389/fnagi.2021.654978
Zhang, W., Zhao, H., Wu, Q., Xu, W., and Xia, M. (2018). Knockdown of BACE1-AS by siRNA improves memory and learning behaviors in Alzheimer’s disease animal model. Exp. Ther. Med. 16, 2080–2086. doi: 10.3892/etm.2018.6359
Zhao, Y., Alexandrov, P. N., Jaber, V., and Lukiw, W. J. (2016). Deficiency in the ubiquitin conjugating enzyme UBE2A in Alzheimer’s disease (AD) is linked to deficits in a natural circular miRNA-7 sponge (circRNA; ciRS-7). Genes. 7:116. doi: 10.3390/genes7120116
Zhao, Q. F., Tan, L., Wang, H. F., Jiang, T., Tan, M. S., Tan, L., et al. (2016). The prevalence of neuropsychiatric symptoms in Alzheimer’s disease: systematic review and meta-analysis. J. Affect. Disord. 190, 264–271. doi: 10.1016/j.jad.2015.09.069
Zheng, F., Link, A., and Alzheimer, C. (2017). Role of activin in cognitive functions, affective behavior and neuronal survival. e-Neuroforum 23, 85–92. doi: 10.1515/nf-2016-A058
Zhong, Z., Yuan, K., Tong, X., Hu, J., Song, Z., Zhang, G., et al. (2018). MiR-16 attenuates β-amyloid-induced neurotoxicity through targeting β-site amyloid precursor protein-cleaving enzyme 1 in an Alzheimer’s disease cell model. Neuroreport 29, 1365–1372. doi: 10.1097/WNR.0000000000001118
Zhu, H. C., Wang, L. M., Wang, M., Song, B., Tan, S., Teng, J. F., et al. (2012). MicroRNA-195 downregulates Alzheimer’s disease amyloid-β production by targeting BACE1. Brain Res. Bull. 88, 596–601. doi: 10.1016/j.brainresbull.2012.05.018
Zhu, X. C., Yu, Y., Wang, H. F., Jiang, T., Cao, L., Wang, C., et al. (2015). Physiotherapy intervention in Alzheimer’s disease: systematic review and Meta-analysis. J. Alzheimers Dis. 44, 163–174. doi: 10.3233/JAD-141377
Zotarelli-Filho, I. J., Mogharbel, B. F., Irioda, A. C., Stricker, P. E. F., de Oliveira, N. B., Saçaki, C. S., et al. (2023). State of the art of microRNAs signatures as biomarkers and therapeutic targets in Parkinson’s and Alzheimer’s diseases: a systematic review and Meta-analysis. Biomedicines. 11:1113. doi: 10.3390/biomedicines11041113
Keywords: ncRNA, miRNA, lncRNA, circRNA, piRNA, Alzheimer’s disease, molecular pathology, biomarkers
Citation: Canoy RJ, Sy JC, Deguit CD, Castro CB, Dimaapi LJ, Panlaqui BG, Perian W, Yu J, Velasco JM, Sevilleja JE and Gibson A (2024) Non-coding RNAs involved in the molecular pathology of Alzheimer’s disease: a systematic review. Front. Neurosci. 18:1421675. doi: 10.3389/fnins.2024.1421675
Edited by:
Lucia Carboni, University of Bologna, ItalyCopyright © 2024 Canoy, Sy, Deguit, Castro, Dimaapi, Panlaqui, Perian, Yu, Velasco, Sevilleja and Gibson. This is an open-access article distributed under the terms of the Creative Commons Attribution License (CC BY). The use, distribution or reproduction in other forums is permitted, provided the original author(s) and the copyright owner(s) are credited and that the original publication in this journal is cited, in accordance with accepted academic practice. No use, distribution or reproduction is permitted which does not comply with these terms.
*Correspondence: Reynand Jay Canoy, cmNjYW5veUB1cC5lZHUucGg=
†These authors have contributed equally to this work and share first authorship