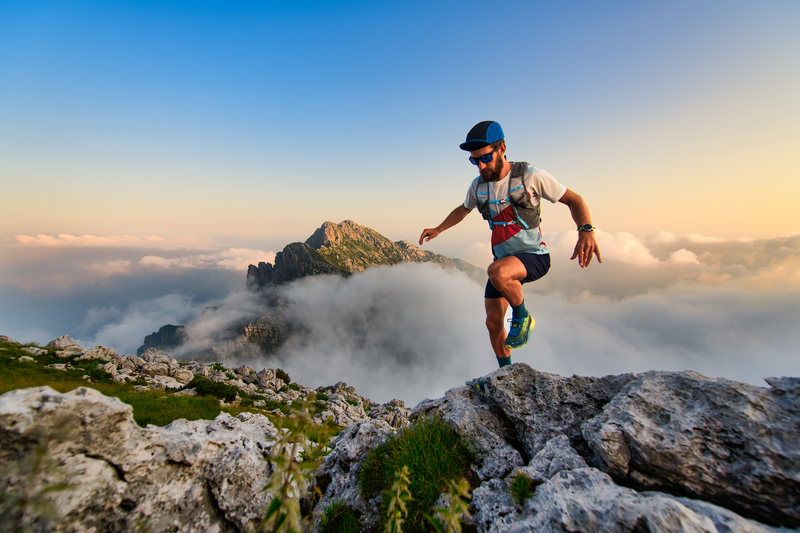
94% of researchers rate our articles as excellent or good
Learn more about the work of our research integrity team to safeguard the quality of each article we publish.
Find out more
BRIEF RESEARCH REPORT article
Front. Neurosci. , 09 August 2024
Sec. Decision Neuroscience
Volume 18 - 2024 | https://doi.org/10.3389/fnins.2024.1408526
This article is part of the Research Topic How Do Affective Stimuli Impact Actions? Unveiling the Relationship Between Emotional Stimuli and Motor Behavior View all 9 articles
This study investigated the impact of the emotional valence of external situations (EVES) on cognitive performance and electrophysiological (EEG) responses during decision-making. 26 healthy adults underwent a modified version of the Trier social stress test, performing five interview-style discourses. Each discourse entailed preparing a speech under increasingly stressful conditions. Participants were also exposed to gradually increasing EVES (i.e., an examining committee displaying progressively more negative-connoted emotional facial expressions). In addition, after each speech, participants completed an arithmetic task to test how emotional manipulation affected cognitive performance. Behavioral data (preparation times) and EEG data (frequency bands) were collected to assess stress regulation, stress resilience, and cognitive performance. The results indicate that EVES significantly influenced stress regulation and resilience, as reflected in the behavioral data. Neurophysiological findings showed increased parietal lobe activity (P4) in the theta and delta bands with rising emotional valence, plateauing from the preparation of the second discourse onward. This suggests enhanced emotional processing and attentional demands. However, gamma band activity decreased in P4 during the preparations for the two discourses following the first, indicating a shift of cognitive resources from higher cognitive functions to emotional processing. This highlights the cognitive cost of maintaining performance and stress regulation under emotionally charged conditions. Such findings suggest that emotional valence modulates cognitive performance and that specific neural mechanisms are involved in managing stress responses. The findings underscore the complex relationship between emotion, cognition, and neural mechanisms, offering valuable insights for stress regulation and resilience, and enhancing performance under pressure.
In everyday life, individuals are constantly exposed to several external stimuli and impactful situations, some of which are of particular significance due to their emotional valence. Emotional valence is a critical factor when examining cognitive functioning (Ferri et al., 2010; Kauschke et al., 2019). Recent research has revealed how exposure to negative audiovisual stimuli impacts information processing times and performance, compared to neutral stimuli (Bowling, 2015; Román et al., 2015). Researchers argue that this effect is driven by the allocation of attentional resources demanded by these stimuli (Plancher et al., 2019), in accordance with the selective visual attention research model proposed by Treisman and Gelade (1980). Aligning with prior research and theories on emotional biases (Pool et al., 2016), the tendency of people to respond more positively than negatively to mild emotional stimuli (i.e., positivity offset) and the tendency to respond more strongly to very negative stimuli than to matched positive stimuli (i.e., negativity bias) can be attributed to emotional and attentional biases (Vanutelli et al., 2017; Balconi and Pozzoli, 2005; Yuan et al., 2019). These biases can significantly influence our perception, attention, memory (Norris et al., 2011), our emotional regulation, cognitive control load, our actions and executive functions (Goldstein and Brockmole, 2002). In particular, the relationship between emotions and executive functions has been extensively studied, considering the common neural circuits involved in both processes, including the ventromedial prefrontal cortex and the amygdala (Tranel, 2002; Manuel et al., 2020).
However, there has been little research focused on understanding how the EVES impact decision making and cognitive performance. Additionally, to the best of our knowledge, no study has investigated individuals’ capacity to plan a performance when confronted with progressively intensifying EVES.
To examine these aspects, we used a modified version of the Trier social stress test (TSST; Allen et al., 2017), named social stress test (SST).
The SST used in this research required participants to engage in the preparation and delivery of five different discourses (D1–5). For each discourse, participants were asked to read a request, specific to that discourse (see Table 1), and then prepare a speech that aligned with the request. This phase (Preparation phase, Pp) was chosen as the most critical part of the task, as participants were informed beforehand that achieving a higher score required them to prepare their speech as quickly as possible.
Instead, to examine how healthy adults respond to different EVES, participants were asked to watch a video depicting an evaluation committee immediately after each preparation. Unlike the TSST, which exclusively features negative emotional valence, in this modified version, the intensity and the valence of the EVES was gradually manipulated through the examining committee’s facial expressions across five distinct phases (see Supplementary File 1).
Subsequently, participants were asked to orally present their speech.
The individuals’ capacity to plan a performance with progressively intensifying EVES, was assessed by recording and examining the length of the Pp, i.e., the Preparation time (PT). This was defined as the interval between the key press that signals the start of the Pp and the subsequent key press that marks the end of this phase. The PT had a maximum duration of 120 s.
We computed two indexes: the stress regulation index (RegStress)—defined as the ability to effectively manage physiological and psychological stress reactions, playing a central role in modulating neurocognitive efficiency (Williams et al., 2009; Crum et al., 2020)—and the stress resilience index (ResStress), defined as the ability to respond promptly and appropriately to more acute and intense stress conditions (Fleshner et al., 2011; Khayrutdinov et al., 2020).
Furthermore, we also examined the impact of the increasing EVES measuring the outcome of the participants’ cognitive performance. Following each speech, they were asked to complete an arithmetic task (aT), and performance accuracy was recorded.
Additionally, to gain a better understanding of the impact of valence on neurocognitive performance, we recorded brain activity via electroencephalography (EEG frequency band) (Balconi et al., 2015b). Different frequency bands have significant functional roles in modulating attention, cognitive workload, emotional engagement, and higher-order cognitive functions. Thus, by exploring changes of bands we aim to get insights into the neural mechanisms handle the EVES. For instance, gamma oscillations are essential for integrating sensory information and emotional processing, while alpha and beta bands are involved in attention modulation and cognitive workload, respectively (Vernet et al., 2012; Tu et al., 2015). The EEG analyses aimed to detect differences in frequency bands across task phases according to different emotional and cognitive contexts.
According to the above-cited literature, we hypothesize that exposure to different experimental contexts would induce an attentional bias toward heightened emotional valence (i.e., negativity bias); (Kauschke et al., 2019) leading to decreased PT. The reduction in PT may be attributed to negative EVES, which enhance cognitive processes such as attention and perception, thereby eliciting faster responses. We also hypothesized that performance in the aT would improve following a stressful situation, due to the higher allocation of attentional resources that foster regulation and resilience.
To determine the minimum required sample size, a priori power analysis for repeated measures ANOVA was conducted, revealing that a total sample size of 21 (alpha error probability = 0.05; power = 0.80; number of groups = 1; Effect size f = 0.25), was necessary to detect a significant within-effect or interaction between factors (G*Power 3.1; Faul et al., 2007). Thus, a total of 26 healthy adults were selected as participants for this study (Mage = 23.038, SDage = 1.455, age range: 22–28, Nfemale = 16). All participants were Caucasian residents of Lombardy, Italy, with a mean educational attainment of 16.38 years (SD = 1.04). They were naïve to the purposes of the experiment, right handed, and had a normal or corrected visual and auditory acuity. Furthermore, they have no significant distress or burnout, no history of neurological or psychiatric illnesses, no current psychoactive substance therapy, and no major stressful life events in the past six months.
All participants provided written informed consent without compensation. The study was approved by the Ethics Committee of the Department of Psychology (approval code: 2021 PhDTD), Catholic University of The Sacred Heart, Milan, Italy, and was conducted in adherence to the guidelines outlined in the Declaration of Helsinki (2013) and according to the General Data Protection Regulation–Reg. UE 2016/679 and its ethical guidelines.
The experiments took place in a moderately lit room. Participants were seated facing a workstation equipped with a computer and a mouse, positioned 100 cm away. They were informed they would participate in a digital job interview to assess their ability to respond to occupational positions in the future, competing with other candidates. They were also informed that their performance would be evaluated by a virtual committee, i.e., participants were aware that these was a fictitious interview. After these instructions, they were asked to sign the informed consent.
Before starting the experiment, the EEG baseline activity was recorded with eyes closed and open. Autonomic activity was also monitored during the experiment. Additional information is reported in the Supplementary File 1.
A web-based experiment management platform (Qualtrics XM platform; Qualtrics LLC, Provo, UT, USA) was employed for administration. For each of the five discourses, participants were asked to: (i) read the requests (REQ1–5, Table 1); (ii) prepare the discourses (Pp1–5; maximum time: 120 s); (iii) watch the evaluation committee video (see Supplementary File 1); and (iv) deliver the speech (maximum time: 60 s). Finally, after each speech, participants engaged in the aT (aT1–5) of similar difficulty to evaluate the effect of emotional manipulation on cognitive performance. These tasks required participants to verbally subtract numbers in a series, aiming to generate as many sequences as possible within a 30-s timeframe (e.g., aT1: “Begin with the number 200 and subtract the number 6 consecutively”).
The experimental procedure lasted approximately 50 min (Figure 1, see also Supplementary File 1).
Figure 1. Sequence of the discourses (D1–5) and arithmetic Task (aT1–5) within the experimental procedure. For every discourse, the participants were required: (i) to read the requests (REQ1–5); (ii) to prepare the discourses (Pp1–5; max-time: 120 s); (iii) to watch the evaluation committee videos; (iv) to present the discourses (max-time: 60 s). EEG and autonomic activity were monitored from the baseline throughout the SST together with behavioral data recording.
Three experts in the psychology of emotions and stress who did not take part in the experiment assessed the emotional situations of the study to ensure their consistency and reliability in eliciting the intended experimental outcomes.
To assess the influence of EVES on neurocognitive response two sets of behavioral data were collected and analyzed.
First, PT of each discourse were computed. These data were then processed offline to derive RegStress and ResStress scores. We used objective and qualitative criteria to evaluate the relevance, coherence of content, and appropriateness of each discourse. Discourses failing to meet productivity criteria—characterized by lack of substance, inclusion of off-task or inconsistent topics—were excluded from analysis and deleted from the database (0.77% data exclusion rate, see Supplementary File 1). This procedure ensured content equivalence across the five analyzed discourses, enabling an objectively comparable assessment of performance originating from the Pp.
To compute RegStress and ResStress scores, we followed three steps. First, each raw score (see Supplementary Table 1a: PTraw1; PTraw2; PTraw3; PTraw4; PTraw5) was assigned a score on a scale from 1 (low performance) to 5 (high performance): PT1, PT2, PT3, PT4, PT5. The scoring was determined through an analysis of the normal distribution of results from a preliminary test (N = 131), conducted before the current research.
Subsequently, data were converted into two distinct scores:
(a) a score representing stress regulation, calculated as follows:
(b) a score representing stress resilience, calculated as follow:
Finally, to further enhance the compatibility and comparability of our findings with future studies, we converted the RegStress and ResStress scores into deciles. This approach allows for a more standardized and reliable measure, facilitating the interpretation and comparison of results across studies (see also Supplementary File 1).
Secondly, for each aT, an accuracy index was computed by comparing the number of correct sequences with the total number of sequences performed by each participant in the series.
Before starting the experiment, we performed a resting-state Recording the EEG with the eyes open and closed. Each condition lasted 120 s (Christie et al., 2017; Ceh et al., 2020; Angioletti and Balconi, 2022).
EEG data for baseline and for the Pp1–5 of the SST was collected using an 18-channel DC amplifier (SYNAMPS) and NEUROSCAN 4.2 software. ElectroCap with Ag/AgCl electrodes was used, placed on 18 scalp sites following the 10/20 system, referencing the earlobes (Jasper, 1958). Furthermore, two electrooculographic electrodes were placed above and below the left eye of each participant, avoiding visual interference. Data were sampled at 1,000 Hz and filtered with a 50 Hz notch input filter. Electrode impedance was checked before data collection, ensuring it remained below 5 kΩ. Following, data from eyes-open and eyes-closed resting states and related to the Pp1–5 were processed offline (IIR bandpass filter 0.1–50 Hz, 48 dB/octave) using Vision Analyzer2 software (Brain Products GmbH, Gilching, Germany), and were corrected with an ICA-based algorithm (Jung et al., 2000). Following EOG correction and meticulous visual inspection, only segments devoid of muscle or eye artifacts and other disturbances were considered (rejected epochs, 3%; average number of epochs selected for Pp: Pp1—20.8; Pp2—13.7; Pp3—15.6; Pp4—19.1; Pp5—13.1). All the electrodes were used for the successive statistical analysis (AFz, Fp1, F7, F3, Fz, F4, F8, Fp2, T7, C3, Cz, C4, T8, P3, Pz, P4, O1, O2). Successively, the data were epoched with a 2,000 ms window to maintain EEG data integrity. Artifact-free data were then used to calculate condition-specific Power Spectral Density (PSD) using fast Fourier transform (Hamming window, resolution: 0.5 Hz). Lastly, average PSD for the main EEG frequency bands [delta (0.5–3.5 Hz); theta (4–7.5 Hz); alpha (8–12.5 Hz); beta (13–30 Hz); and gamma (30.5–50 Hz)] was extracted for each phase considered. Additionally, all task-related data were normalized to the eyes-open baseline of each participant.
Repeated measures ANOVAs (analysis of variance) were applied to behavioral and EEG data.
For behavioral data, a repeated measures ANOVA with Pp (5 levels) as the within-independent subject factor was applied to the PT scores, RegStress and ResStress, as dependent variables. For the aT, a repeated measures ANOVA with Task (5 levels) as the within-subject factor was applied to the accuracy index. Finally, correlation analyses (Pearson correlation coefficients) between RegStress and ResStress, respectively, and the accuracy index of the five aT were computed.
For EEG data, five repeated measures ANOVAs considering Electrode (18) and Pp (5) as within-subject independent variables were carried out for the five different frequency bands.
Degrees of freedom in all ANOVA tests were adjusted using the Greenhouse–Geisser epsilon when appropriate. Significant interactions were explored using pairwise comparisons with Bonferroni correction. Partial eta squared values were computed to estimate the effect sizes. IBM SPSS 29 (IBM Corp., Chicago, IL, USA) was used for the statistical analysis.
The normal distribution of the data was assessed by using skewness and kurtosis test. Furthermore, as preliminary analysis showed no statistically significant main effects or interactions concerning gender; this variable was excluded from subsequent analyses.
All the descriptive statistics are reported in the Supplementary Table 1.
The analysis on the behavioral data showed significant main effect of PT [F(2.2, 55.5) = 5.455, p = 0.005, ηp2 = 0.179]. Post hoc tests showed that PT1 was longer than PT2 (p = 0.035; 95% CI [0.94, 40.88]), and PT5 (p = 0.018; 95% CI [3.07, 47.77]) (Figure 2A).
Figure 2. Behavioral Preparation time (PT) scores results and correlations between the accuracy index of the arithmetic Task (aT) and RegStress and RegStress indexes. (A) The bar graph shows the PT scores for each preparation phase (Pp). Bars represent ± 1 Standard Error and stars (*) mark statistically significant comparisons. (B) The scatter plots at the left display the correlation between the accuracy index and the RegStress score in the aT4. The scatter plots at the right display the correlation between accuracy index and ResStress score in the aT3. The different colors of the dots correspond to each Pp.
No significant differences were found for the ANOVAs performed on the accuracy index of the aT.
The Pearson correlation analyses revealed a negative correlation between the RegStress and accuracy index in aT4 (r = −0.465, p = 0.017) and a negative correlation between the ResStress and accuracy index in aT3 (r = −0.453, p = 0.020) (Figure 2B).
The repeated measures ANOVAs performed on each of the five different frequency bands (delta, theta, alpha, beta, and gamma) are reported.
For delta band, a significant interaction effect was revealed for Electrode × Preparation [F(68, 1292) = 2.158, p ≤ 0.001, ηp2 = 0.102] (Figure 3A). Significant pairwise comparisons revealed higher mean values in P4 for the Pp2 (p = 0.001; 95% CI [−0.93, −0.21]), Pp3 (p = 0.012; 95% CI [−0.78, −0.06]), Pp4 (p = 0.003; 95% CI [−1.27, −0.20]), Pp5 (p = 0.006; 95% CI [−1.30, −0.16]) compared to the Pp1.
Figure 3. EEG results. The bar graph shows the significant differences for the delta, theta, and gamma bands in Electrode × Preparation. For all graph, bars represent ± 1 Standard Error and stars (*) mark statistically significant comparisons. For topographic EEG maps, red represented an increase in power for the considered frequency band. (A) The bar graph displays the significantly higher mean values in P4 for the Pp2, Pp3, Pp4, and Pp5, compared to the Pp1 for the delta band. The topographic EEG maps of spectral power density for the delta band are reported (Software: Vision Analyzer2, Brain Products GmbH, Gilching, Germany). (B) The bar graph displays the significantly higher mean values in P4 for the Pp2, Pp3, and Pp4, compared to the Pp1 for theta band. The topographic EEG maps of spectral power density for the theta band are reported. (C) The bar graph displays the significantly higher mean values in P4 for the Pp1 compared to the Pp2 and the Pp3 for the gamma band. The topographic EEG maps of spectral power density for the gamma band are reported.
In the same way, for theta band, a significant interaction effect was revealed for Electrode × Preparation [F(68, 1292) = 1.940, p ≤ 0.001, ηp2 = 0.093] (Figure 3B). Significant pairwise comparisons revealed higher mean values in P4 for the Pp2 (p = 0.003; 95% CI [−0.90, −0.15]), Pp3 (p = 0.017; 95% CI [−0.77, −0.05]), Pp4 (p = 0.001; 95% CI [−0.94, −0.20]), compared to the Pp1.
Lastly, for gamma band, a significant interaction effect was revealed for Electrode × Preparation [F(68, 1292) = 1.508, p = 0.006, ηp2 = 0.074] (Figure 3C). Significant pairwise comparisons revealed higher mean values in P4 for Pp1 compared to the Pp2 (p = 0.025; 95% CI [0.35, 7.91]), and Pp3 (p = 0.031; 95% CI [0.27, 7.80]).
No other significant differences were found for the ANOVAs performed on the EEG Pp data, as well as on the alpha and beta bands.
All the results for P4 are reported in the Supplementary Table 2. Plot of PSD across frequency at P4 for each of the five discourses are reported in the Supplementary Figure 2, in the Supplementary File 1.
This pilot study explored the relationship between the progressively increasing negativity valence during the presentation of different ecologically EVES and the corresponding behavioral and neurophysiological responses.
Behavioral findings revealed a decrease in the PT, according to heightened negativity. This is more evident in Pp2, when the emotional valence starts to become more negative and in Pp5, when the negative valence is maximal. These findings can be explained by an increase of attentional and emotional biases in participants when the context becomes more and more negative. Such interpretation aligns with previous research indicating that low-negative valence situations tend to elicit a positive response, whereas high-negative emotional valence situations often result in a negativity bias. This is primarily due to the heightened attention and reactivity typically evoked by negative situations, leading to shorter PT (Vanutelli et al., 2017; Balconi and Pozzoli, 2005; Yuan et al., 2019).
The behavioral PT data show a plateau effect starting from the Pp2. This means there was no significant variation in performance after the first discourse, although the negative intensity progressively increased. This phenomenon might be related to the “negativity saturation threshold.” Although the degree of negativity increases, participants’ subjective perception does not seem to increase further. This suggests that there is a limit beyond which negative emotional situations stabilize. This is supported by the fact that significant differences were only observed between Pp1 and Pp2, and between Pp1 and Pp5, but not between the preparations after the first (for example, no difference was found between Pp5 and Pp4, or between Pp4 and Pp3). These findings imply that, after reaching a certain point, additional negative stimuli do not elicit a proportionate increase in the participants’ subjective negative experience, thereby supporting the concept of a negativity saturation threshold.
Instead, the RegStress and ResStress indexes did not show significant differences. Considering the functional significance attributed to such indexes, this suggests that people can respond similarly despite the emotional situations turn to be more negative. However, this stability comes at a cost. Some researchers suggest that the increasing negativity of emotional situations could potentially reduce people’s ability to effectively process information, lowering performance (Li et al., 2022). In fact, considering cognitive performance, we found a negative correlation between the RegStress index and accuracy in the fourth arithmetic task, as well as between the ResStress index and accuracy in the third arithmetic task. This result could be due to the fact that people, to maintain consistent levels of stress regulation and resilience, allocate a significant amount of cognitive and emotional resources in this process, subtracting cognitive resources reducing the ability to effectively perform complex cognitive tasks.
In terms of neurophysiological findings, an augmentation in parietal lobe activity (P4), has been noted in the theta and delta bands as the negative valence of situations increases. This trend seems to plateau from Pp2 onward, paralleling the pattern observed in the PT data. It has been shown that an increase in parietal lobe activity in theta band and delta band indicates emotional central processing, attentional demands, as well as stimulus evaluation (Balconi and Pozzoli, 2009; Balconi et al., 2015b; Balconi and Vanutelli, 2017; Balconi and Angioletti, 2021). Additionally, the parietal lobe is involved in top-down attentional processes guided by episodic retrieval goals, while ventral parts of the parietal lobe support bottom-up attentional processes captured by retrieval output (Dobbins et al., 2012). Instead, the inferior parietal lobe is involved in matching perceptual information about observed actions with motoric representations, enabling the understanding of actions and intentions (Thakkar et al., 2014). The heightened activity observed in this region indicate a greater level of engagement in the assessment and interpretation of EVES (Viviani, 2013). In fact, the processing of EVES involves heightened theta and delta activity, which aids in reinterpreting the stimulus and regulating emotional responses over time (Lapomarda et al., 2022). This regulation is primarily supported by visuospatial networks located in the right parieto-occipital lobe (Wang et al., 2010; Maksimenko et al., 2018). This explains the involvement of electrode P4, representing a region of the parietal lobe known to be involved in complex cognitive processes, including those related to the integration of information (Gonzalez and Flindall, 2015; García-Martínez et al., 2019; Steber et al., 2020).
Conversely, a decrease in gamma-band activity associated with higher cognitive demand, and metacognition (Herrmann et al., 2010), was observed in P4 in Pp2 and Pp3 compared to Pp1. The reduction in gamma activity during the central phases of the SST may reflect a redistribution of cognitive resources toward emotional processing at the expense of higher cognitive functions, as also supported by the negative correlations between accuracy in aT in central tasks and the two stress response indexes. This can represent a “cost to pay” for maintaining an adequate performance and stress regulation to the increasing EVES when the interaction becomes more and more engaging because of a greater negative charge.
These findings should be taken with caution, as they do not unequivocally establish a direct correlation between parietal lobe activity and PT or accuracy. In this context, it is conceivable that the progressive intensification of negatively EVES not only augments readiness for processing subsequent discourses (i.e., increased PT), but may also foster a different mode of processing.
Concluding, this study suggests that individuals need to engage their cognitive resources to maintain stability and resist stress when faced with EVES. The impact of the EVES can vary greatly depending on the person’s evaluation of a presented stimulus according to the context in which he/she operates (Balconi et al., 2015a,b; Calbi et al., 2022; Mirabella et al., 2023; Montalti and Mirabella, 2023), aligning with the appraisal theories of emotions (Moors and Fischer, 2019; Scherer and Moors, 2019).
However, these results warrant further examination. Future research with larger samples and counterbalancing the sequence of events are necessary to fully explore participants’ reactions. Contrary to our expectations, the accuracy of the aT did not change with increasing negative EVES (Supplementary Figure 1), indicating that the cognitive performance was not affected by the emotional valence of the discourses. Thus, all interpretations tying emotional valence to cognitive performance were based on the correlations of the aT performance with RegStress and ResStress. This might indicate that the task was not sufficiently challenging to reveal the impact of emotional valence on cognitive performance or that participants progressively familiarized with the experimental set up. Furthermore, it’s unclear if EEG and behavioral differences during the Pp1–5 are due to initial speech requests or progressive intensification of negative valence. However, it appears plausible that the observed differences in emotional response are primarily due to the stress condition (EVES) rather than task difficulty changes from REQs, as the EVES’s negative valence intensification was specifically designed to increment perceived stress levels.
Notwithstanding these limitations, this approach can be used to measure the impact of EVES in diverse contexts and with varying demands, including occupational settings, political sector, clinical environments, marketing, and advertising.
The raw data supporting the conclusions of this article will be made available by the authors, without undue reservation.
The studies involving humans were approved by the Ethics Committee of the Department of Psychology, Catholic University of the Sacred Heart, Milan, Italy. The studies were conducted in accordance with the local legislation and institutional requirements. The participants provided their written informed consent to participate in this study. Written informed consent was obtained from the individual(s) for the publication of any potentially identifiable images or data included in this article.
MB: Conceptualization, Methodology, Project administration, Resources, Supervision, Validation, Writing – review & editing. KR: Conceptualization, Data curation, Formal analysis, Investigation, Methodology, Software, Visualization, Writing – original draft.
The authors declare that no financial support was received for the research, authorship, and/or publication of this article.
The authors declare that the research was conducted in the absence of any commercial or financial relationships that could be construed as a potential conflict of interest.
All claims expressed in this article are solely those of the authors and do not necessarily represent those of their affiliated organizations, or those of the publisher, the editors and the reviewers. Any product that may be evaluated in this article, or claim that may be made by its manufacturer, is not guaranteed or endorsed by the publisher.
The Supplementary Material for this article can be found online at: https://www.frontiersin.org/articles/10.3389/fnins.2024.1408526/full#supplementary-material
Allen, A. P., Kennedy, P. J., Dockray, S., Cryan, J. F., Dinan, T. G., and Clarke, G. (2017). The trier social stress test: Principles and practice. Neurobiol. Stress 6:113. doi: 10.1016/J.YNSTR.2016.11.001
Angioletti, L., and Balconi, M. (2022). EEG brain oscillations are modulated by interoception in response to a synchronized motor vs. cognitive task. Front. Neuroanat. 16:991522. doi: 10.3389/FNANA.2022.991522
Balconi, M., and Angioletti, L. (2021). One’s interoception affects the representation of seeing others’ pain: A randomized controlled qEEG study. Pain Res. Manag. 2021:5585060.
Balconi, M., and Pozzoli, U. (2009). Arousal effect on emotional face comprehension: Frequency band changes in different time intervals. Physiol. Behav. 97:455. doi: 10.1016/J.PHYSBEH.2009.03.023
Balconi, M., and Vanutelli, M. E. (2017). Cooperation and competition with hyperscanning methods: Review and future application to emotion domain. Front. Comput. Neurosci. 11:86. doi: 10.3389/fncom.2017.00086
Balconi, M., and Pozzoli, U. (2005). Morphed facial expressions elicited a N400 ERP effect: A domain-specific semantic module? Scand. J. Psychol. 46, 467–474. doi: 10.1111/j.1467-9450.2005.00478.x
Balconi, M., Grippa, E., and Vanutelli, M. E. (2015a). Resting lateralized activity predicts the cortical response and appraisal of emotions: An fNIRS study. Soc. Cogn. Affect. Neurosci. 10, 1607–1614.
Balconi, M., Grippa, E., and Vanutelli, M. E. (2015b). What hemodynamic (fNIRS), electrophysiological (EEG) and autonomic integrated measures can tell us about emotional processing. Brain Cogn. 95, 67–76.
Bowling, A. J. (2015). Emotional interference: The impact of task-relevant emotional stimuli on cognitive performance.
Calbi, M., Montalti, M., Pederzani, C., Arcuri, E., Umiltà, M. A., Gallese, V., et al. (2022). Emotional body postures affect inhibitory control only when task-relevant. Front. Psychol. 13:1035328. doi: 10.3389/fpsyg.2022.1035328
Ceh, S. M., Annerer-Walcher, S., Körner, C., Rominger, C., Kober, S. E., Fink, A., et al. (2020). Neurophysiological indicators of internal attention: An electroencephalography–eye-tracking coregistration study. Brain Behav. 10:e01790. doi: 10.1002/BRB3.1790
Christie, S., di Fronso, S., Bertollo, M., and Werthner, P. (2017). Individual alpha peak frequency in ice hockey shooting performance. Front. Psychol. 8:260760. doi: 10.3389/FPSYG.2017.00762/BIBTEX
Crum, A. J., Jamieson, J. P., and Akinola, M. (2020). Optimizing stress: An integrated intervention for regulating stress responses. Emotion 20:120. doi: 10.1037/EMO0000670
Dobbins, I. G., Jaeger, A., Studer, B., and Simons, J. S. (2012). Use of explicit memory cues following parietal lobe lesions. Neuropsychologia 50, 2992–3003. doi: 10.1016/J.NEUROPSYCHOLOGIA.2012.07.037
Faul, F., Erdfelder, E., Lang, A. G., and Buchner, A. (2007). G*Power 3: A flexible statistical power analysis program for the social, behavioral, and biomedical sciences. Behav. Res. Methods 39, 175–191. doi: 10.3758/BF03193146/METRICS
Ferri, F., Stoianov, I. P., Gianelli, C., D’Amico, L., Borghi, A. M., and Gallese, V. (2010). When action meets emotions: How facial displays of emotion influence goal-related behavior. PLoS One 5:e13126. doi: 10.1371/JOURNAL.PONE.0013126
Fleshner, M., Maier, S. F., Lyons, D. M., and Raskind, M. A. (2011). The neurobiology of the stress-resistant brain. Stress 14, 498–502. doi: 10.3109/10253890.2011.596865
García-Martínez, B., Martinez-Rodrigo, A., Alcaraz, R., and Fernández-Caballero, A. (2019). A review on nonlinear methods using electroencephalographic recordings for emotion recognition. IEEE Trans. Affect. Comput. 12, 801–820.
Goldstein, E. B., and Brockmole, J. R. (2002). Sensation and perception. Pacific Grove, CA: Wadsworth-Thomson Learning.
Gonzalez, C. L. R., and Flindall, J. W. (2015). Parietal lobe. Int. Encyclop. Soc. Behav. Sci. Second Edn. 28, 506–510. doi: 10.1016/B978-0-08-097086-8.55043-0
Herrmann, C. S., Fründ, I., and Lenz, D. (2010). Human gamma-band activity: A review on cognitive and behavioral correlates and network models. Neurosci. Biobehav. Rev. 34, 981–992. doi: 10.1016/J.NEUBIOREV.2009.09.001
Jasper, H. H. (1958). The ten-twenty electrode system of international federation EEG. Electroencephalogr. Clin. Neurophysiol. 10, 371–375.
Jung, T.-P., Makeig, S., Humphries, C., Lee, T.-W., McKeown, M. J., Iragui, V., et al. (2000). Removing electroencephalographic artifacts by blind source separation. Psychophysiology 37, 163–178. doi: 10.1111/1469-8986.3720163
Kauschke, C., Bahn, D., Vesker, M., and Schwarzer, G. (2019). The role of emotional valence for the processing of facial and verbal stimuli–positivity or negativity bias? Front.Psychol. 10:1654. doi: 10.3389/fpsyg.2019.01654
Khayrutdinov, R. R., Mukhametzyanova, F. G., Bashkireva, T. V., Bashkireva, A. V., and Morozov, A. V. (2020). Stress resistance of personality in the conditions of development of professional activity. Int. J. High. Educ. 9, 100–104. doi: 10.5430/ijhe.v9n8p100
Lapomarda, G., Valer, S., Job, R., and Grecucci, A. (2022). Built to last: Theta and delta changes in resting-state EEG activity after regulating emotions. Brain Behav. 12:2597. doi: 10.1002/BRB3.2597
Li, L., Li, R., Shen, F., Wang, X., Zou, T., Deng, C., et al. (2022). Negative bias effects during audiovisual emotional processing in major depression disorder. Hum. Brain Mapp. 43, 1449. doi: 10.1002/HBM.25735
Maksimenko, V. A., Runnova, A. E., Frolov, N. S., Makarov, V. V., Nedaivozov, V., Koronovskii, A. A., et al. (2018). Multiscale neural connectivity during human sensory processing in the brain. Phys. Rev. 97:052405. doi: 10.1103/PhysRevE.97.052405
Manuel, A. L., Roquet, D., Landin-Romero, R., Kumfor, F., Ahmed, R. M., Hodges, J. R., et al. (2020). Interactions between decision-making and emotion in behavioral-variant frontotemporal dementia and Alzheimer’s disease. Soc. Cogn. Affect. Neurosci. 15:681. doi: 10.1093/SCAN/NSAA085
Mirabella, G., Grassi, M., Mezzarobba, S., and Bernardis, P. (2023). Angry and happy expressions affect forward gait initiation only when task relevant. Emotion 23, 387–399. doi: 10.1037/EMO0001112
Montalti, M., and Mirabella, G. (2023). Unveiling the influence of task-relevance of emotional faces on behavioral reactions in a multi-face context using a novel Flanker-Go/No-go task. Sci. Rep. 1, 1–12. doi: 10.1038/s41598-023-47385-1
Moors, A., and Fischer, M. (2019). Demystifying the role of emotion in behaviour: Toward a goal-directed account. Cogn. Emot. 33, 94–100. doi: 10.1080/02699931.2018.1510381
Norris, C. J., Larsen, J. T., Crawford, L. E., and Cacioppo, J. T. (2011). Better (or worse) for some than others: Individual differences in the positivity offset and negativity bias. J. Res. Pers. 45, 100–111. doi: 10.1016/j.jrp.2010.12.001
Plancher, G., Massol, S., Dorel, T., and Chainay, H. (2019). Effect of negative emotional content on attentional maintenance in working memory. Cogn. Emot. 33, 1489–1496. doi: 10.1080/02699931.2018.1561420
Pool, E., Brosch, T., Delplanque, S., and Sander, D. (2016). Attentional bias for positive emotional stimuli: A meta-analytic investigation. Psychol. Bull. 142:79.
Román, F. J., García-Rubio, M. J., Privado, J., Kessel, D., López-Martín, S., Martínez, K., et al. (2015). Adaptive working memory training reveals a negligible effect of emotional stimuli over cognitive processing. Pers. Individ. Differ. 74, 165–170.
Scherer, K. R., and Moors, A. (2019). The emotion process: Event appraisal and component differentiation. Annu. Rev. Psychol. 70, 719–745. doi: 10.1146/ANNUREV-PSYCH-122216-011854/CITE/REFWORKS
Steber, S., König, N., Stephan, F., and Rossi, S. (2020). Uncovering electrophysiological and vascular signatures of implicit emotional prosody. Sci. Rep. 1, 1–14. doi: 10.1038/s41598-020-62761-x
Thakkar, K. N., Peterman, J. S., and Park, S. (2014). Altered brain activation during action imitation and observation in schizophrenia: A translational approach to investigating social dysfunction in schizophrenia. Am. J. Psychiatry 171, 539–548. doi: 10.1176/APPI.AJP.2013.13040498/ASSET/IMAGES/LARGE/539F4.JPEG
Tranel, D. (2002). Emotion, decision making, and the ventromedial prefrontal cortex, eds D. T. Stuss and R. T. Knight (Oxford: Oxford University Press), doi: 10.1093/ACPROF:OSO/9780195134971.003.0022
Treisman, A. M., and Gelade, G. (1980). A feature-integration theory of attention. Cogn. Psychol. 12, 97–136. doi: 10.1016/0010-0285(80)90005-5
Tu, Y., Zhang, Z., Tan, A., Peng, W., Hung, Y. S., Moayedi, M., et al. (2015). Alpha and gamma oscillation amplitudes synergistically predict the perception of forthcoming nociceptive stimuli. Hum. Brain Mapp. 37, 501–514. doi: 10.1002/hbm.23048
Vanutelli, M. E., Venturella, I., Angioletti, L., and Balconi, M. (2017). Mirroring the emotions of others by autonomic system: Intra-species effect in children. Neuropsychol. Trends 22, 109–119.
Vernet, M., Bashir, S., Yoo, W., Perez, J., Najib, U., and Pascual-Leone, Á (2012). Insights on the Neural Basis of Motor Plasticity Induced by Theta Burst Stimulation From TMS-EEG. Eur. J. Neurosci. 37, 598–606. doi: 10.1111/ejn.12069
Viviani, R. (2013). Emotion regulation, attention to emotion, and the ventral attentional network. Front. Hum. Neurosci. 7:746. doi: 10.3389/FNHUM.2013.00746
Wang, J., Conder, J. A., Blitzer, D. N., and Shinkareva, S. V. (2010). Neural representation of abstract and concrete concepts: A meta-analysis of neuroimaging studies. Hum. Brain Mapp. 31, 1459–1468. doi: 10.1002/HBM.20950
Williams, P. G., Suchy, Y., and Rau, H. K. (2009). Individual differences in executive functioning: Implications for stress regulation. Ann. Behav. Med. 37, 126–140. doi: 10.1007/S12160-009-9100-0
Keywords: emotional valence, decision making, EEG, social stress test, cognitive performance
Citation: Balconi M and Rovelli K (2024) Does emotional valence affect cognitive performance and neurophysiological response during decision making? A preliminary study. Front. Neurosci. 18:1408526. doi: 10.3389/fnins.2024.1408526
Received: 28 March 2024; Accepted: 26 July 2024;
Published: 09 August 2024.
Edited by:
Giovanni Mirabella, University of Brescia, ItalyReviewed by:
Dinavahi V. P. S. Murty, University of Maryland, College Park, United StatesCopyright © 2024 Balconi and Rovelli. This is an open-access article distributed under the terms of the Creative Commons Attribution License (CC BY). The use, distribution or reproduction in other forums is permitted, provided the original author(s) and the copyright owner(s) are credited and that the original publication in this journal is cited, in accordance with accepted academic practice. No use, distribution or reproduction is permitted which does not comply with these terms.
*Correspondence: Katia Rovelli, a2F0aWEucm92ZWxsaUB1bmljYXR0Lml0
Disclaimer: All claims expressed in this article are solely those of the authors and do not necessarily represent those of their affiliated organizations, or those of the publisher, the editors and the reviewers. Any product that may be evaluated in this article or claim that may be made by its manufacturer is not guaranteed or endorsed by the publisher.
Research integrity at Frontiers
Learn more about the work of our research integrity team to safeguard the quality of each article we publish.