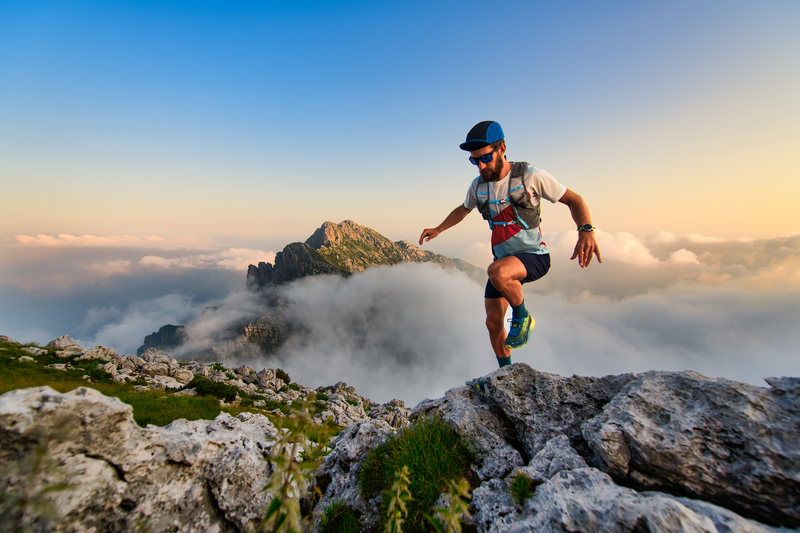
94% of researchers rate our articles as excellent or good
Learn more about the work of our research integrity team to safeguard the quality of each article we publish.
Find out more
PERSPECTIVE article
Front. Neurosci. , 14 July 2023
Sec. Brain Imaging Methods
Volume 17 - 2023 | https://doi.org/10.3389/fnins.2023.934138
This article is part of the Research Topic Insights in Brain Imaging Methods: 2021 View all 9 articles
Functional magnetic resonance imaging (fMRI) is an indirect measure of neural activity with the hemodynamic response function (HRF) coupling it with unmeasured neural activity. The HRF, modulated by several non-neural factors, is variable across brain regions, individuals and populations. Yet, a majority of human resting-state fMRI connectivity studies continue to assume a non-variable HRF. In this article, with supportive prior evidence, we argue that HRF variability cannot be ignored as it substantially confounds within-subject connectivity estimates and between-subjects connectivity group differences. We also discuss its clinical relevance with connectivity impairments confounded by HRF aberrations in several disorders. We present limited data on HRF differences between women and men, which resulted in a 15.4% median error in functional connectivity estimates in a group-level comparison. We also discuss the implications of HRF variability for fMRI studies in the spinal cord. There is a need for more dialogue within the community on the HRF confound, and we hope that our article is a catalyst in the process.
Functional magnetic resonance imaging (fMRI) has contributed significantly to the advancement of neuroscience, psychiatry, and neurology over the past three decades (Power et al., 2011; Rosazza and Minati, 2011; Nathan et al., 2014). While neural activity can be directly measured in vivo through invasive procedures, blood oxygenation level-dependent (BOLD) fMRI is a complex, indirect measure of neural activity (Logothetis et al., 2001; Figure 1A), measuring local blood oxygenation variations in response to active neurons. Dilation and constriction of blood vessels modulates this process, which, in turn, is modulated through numerous non-neural and neural factors that are difficult to delineate (Biessmann et al., 2012). The combination of factors that lie between neural activity and BOLD is the hemodynamic response function (HRF; Duarte et al., 2015; Kim and Ress, 2016). HRF shape is characterized by its amplitude (response height, RH), latency (time-to-peak, TTP) and width (full-width at half max, FWHM; Figure 1B). Representing neurovascular coupling in the BOLD signal, the HRF is modulated by several non-neural factors (Biessmann et al., 2012; Yang et al., 2019) such as hematocrit, variable size/density of vasculature, global magnetic susceptibilities, alcohol/caffeine/lipid ingestion, pulse/respiration differences, and partial volume imaging of veins (Aguirre et al., 1998; Levin et al., 1998; Noseworthy et al., 2003; Handwerker et al., 2004; Buxton, 2010; Tong and Frederick, 2014; Bernier et al., 2018; Yang et al., 2019). The HRF shape varies across the brain and individuals (Aguirre et al., 1998; Handwerker et al., 2004; Lewis et al., 2018).
Figure 1. (A) FMRI is an indirect measure of neural activity. What stands between them is the hemodynamic response function (HRF). (B) The HRF is the BOLD response to a neural impulse. It has a peak (response height) occurring sometime after the neural impulse (time-to-peak) (HRF width = FWHM). The HRF always has this shape (biological), but these parameters are variable.
The current ‘perspective’ article focuses on this HRF variability (HRFv) and its impact on fMRI data processing and subsequent outcome measures within the scope of human fMRI research. This topic is important because thousands of human fMRI studies are published each year, but a significant portion of those do not account for HRFv. Resting-state fMRI (rs-fMRI) and connectivity studies dominate that list. Thus, there is a need for more dialogue within the community on HRFv. We argue that HRFv causes a measurable impact on the BOLD time series, which, if ignored, will confound fMRI outcomes such as connectivity. We substantiate our argument with prior human HRFv research, including those focusing on rs-fMRI connectivity. We also present limited new data (to substantiate our ‘perspective’) on HRFv across two important demographic variables: age and sex. Lastly, to cover structures of the central nervous system beyond the brain, we also discuss the implications of HRFv for spinal cord fMRI.
HRFv was first demonstrated in 1998 (Aguirre et al., 1998) and further examined in later years (Miezin et al., 2000; Handwerker et al., 2004; de Zwart et al., 2005; Badillo et al., 2013). The HRF of a given brain region was identified to be different across individuals, and within a given individual, it was different across brain regions. Non-neural factors affecting the HRF is not merely the concern because a variable known HRF could be accounted for. Instead, the concern is that we do not understand these variable factors (and thus the HRF). The issue is alleviated in task fMRI studies by often modeling the HRF confound as time/dispersion derivatives of the canonical HRF in a general linear model (GLM) framework, which works well since BOLD is time-locked to an external stimulus. However, the outlook is different in rs-fMRI studies, which mostly ignore HRFv. The notion of HRF not being variable enough to be a serious confound was challenging to test, if not impossible, a few years ago because of the inability to estimate the HRF from rs-fMRI data. Necessary technical advancements [e.g., point-process theory (Tagliazucchi et al., 2012; Power et al., 2015)] have now resulted in rs-fMRI deconvolution techniques that are capable of HRF estimation from resting-state fMRI data (Havlicek et al., 2011; Karahanoğlu et al., 2013; Wu et al., 2013; Havlicek et al., 2015). We take a closer look at these techniques next.
HRF estimation can be straightforward with two known quantities (fMRI and neural activity) and one unknown (HRF). This is possible with simultaneous fMRI and invasive recordings [(e.g., David et al., 2008; Wang et al., 2017b)], which is hardly feasible in humans. Obtaining simultaneous rs-EEG/fMRI data and considering EEG as the neural input to deconvolve fMRI [using AFNI’s 3dDeconvolve (Feige et al., 2017)] is problematic because generative fMRI models do not consider scalp EEG as properly representing BOLD-inducing neural activity (Logothetis et al., 2001). A viable alternative is estimating HRF latency from a hypercapnic challenge because breath-hold causes vasodilation and modulates cerebral blood flow (CBF) (Thomason et al., 2007; Hall et al., 2016; McDonough et al., 2019) independent of neural activity, allowing us to measure vascular latency. Chang et al. (Chang et al., 2008) utilized this to correct for vascular latency prior to connectivity analysis. The disadvantage is that an additional breath-hold scan is not always feasible or available. Breath-hold is prone to subjective performance and can be challenging in those with some neurological diseases (Spano et al., 2013; Urback et al., 2017). Moreover, it only measures one aspect of HRF shape (TTP), while the entire HRF impacts BOLD.
To circumvent these concerns, an alternative is to perform blind deconvolution; that is, solve the mathematically ill-posed inverse problem of having two unknowns (HRF and neural activity) and one known (fMRI). This is feasible because the natural limits and biophysics of HRF and fMRI are well understood. HRF estimation then becomes a constrained optimization exercise. Such deconvolution techniques primarily focusing on task fMRI data (Gitelman et al., 2003; Gaudes et al., 2011; Hernandez-Garcia and Ulfarsson, 2011; Khalidov et al., 2011; Lopes et al., 2012; Bush and Cisler, 2013; Caballero Gaudes et al., 2013; Bush et al., 2015) are generally robust to HRF misspecification within a narrow physiological range, but are not viable for estimating voxel-specific HRFs in the entire brain, especially in rs-fMRI data. Whole-brain HRF estimation is preferable with rs-fMRI data because, with task fMRI, a given task does not activate the entire brain uniformly (Taylor et al., 2018), and even among activated voxels, the BOLD response is mostly non-uniform (Gonzalez-Castillo et al., 2012), sometimes leading to biologically implausible HRF estimates (Taylor et al., 2018). Some techniques that might, in principle, be viable for rs-fMRI have never been tested using rs-fMRI data (Sreenivasan et al., 2015; Cherkaoui et al., 2019).
This leaves us with four rs-fMRI deconvolution techniques that have been more widely adopted: (i) Wu et al.’s (2013) data-driven rsHRF method (Wu et al., 2021), (ii) parametric generative state-space models proposed within the stochastic dynamic causal modeling (DCM) framework (Havlicek et al., 2011; Friston et al., 2013, 2014), (iii) physiologically informed DCM (Havlicek et al., 2015; developed for task fMRI but can be extended to rs-fMRI), and (iv) Total Activation (Karahanoğlu et al., 2013; estimates an “activity-inducing signal” from BOLD, from which it is possible in principle to estimate the HRF by Wiener deconvolution), which has been applied in the brain (Karahanoğlu and Van De Ville, 2015; Zöller et al., 2021) as well as the spinal cord (Kinany et al., 2020). Notably, a substantial number of studies examining HRFv (described later) utilized Wu et al.’s technique (Wu et al., 2013), hence we describe it briefly here. The Wu et al. technique models rs-fMRI data as event-related time series, with events modeled as point-processes (Saad et al., 2012). Then it estimates the best-fit HRF in a least-squares sense, using the BOLD time series at identified events. Finally, the latent neural time series is estimated using Wiener deconvolution from the measured BOLD and the estimated HRF (Glover, 1999). This technique has been validated using simulations, non-invasive and invasive data (Tagliazucchi et al., 2012; Wu et al., 2013; Rangaprakash et al., 2018b; Wu et al., 2021), and has been applied in many recent papers (Amico et al., 2014; Lamichhane et al., 2014; Boly et al., 2015; Rangaprakash et al., 2017a,b,c, 2018a,b). HRFs separated by 4 weeks demonstrated moderate test–retest reliability (ICC = 0.51; Rangaprakash et al., 2020), which is impressive by current neuroimaging standards (Noble et al., 2019). A vast majority of human rs-fMRI studies do not perform additional breath-hold scans or do not have invasive recordings. This article focuses on the HRF confound in such studies, although some of the material is applicable generally to all fMRI studies. We next present the impact of HRFv on rs-fMRI data.
We focus here on functional connectivity (FC; Power et al., 2011) because most rs-fMRI studies investigate FC, or metrics derived from FC such as dynamic connectivity and graph measures. It is, however, notable that the HRF confound has also been investigated for effective connectivity models such as DCM (Friston et al., 2013), Granger causality (Deshpande et al., 2010; Lacey et al., 2014; Feng et al., 2015; Sreenivasan et al., 2015; Hampstead et al., 2016), and multivariate dynamical system models (Ryali et al., 2011, 2016a,b). Effective connectivity estimated from fMRI data is viable (Deshpande et al., 2010; Handwerker et al., 2012) as well as accurate (David et al., 2008; Havlicek et al., 2010, 2011; Wang et al., 2017a) only after deconvolution.
FC studies have, however, largely ignored HRFv either with the assumption that the HRF is similar enough among brain regions and individuals or due to the unavailability of HRF estimation methods. Recent evidence suggests that these assumptions must be re-evaluated. Although researchers have been aware, since the early days of fMRI, that BOLD measures blood oxygenation and not neural activity, the magnitude of HRF confound on FC is being investigated only recently with the availability of deconvolution techniques. We take a closer look at these. It has been demonstrated that the HRF is variable (Aguirre et al., 1998; Handwerker et al., 2004; Tak et al., 2015; Rangaprakash et al., 2018b; Bright et al., 2020) and recent reports suggest that ignoring it can introduce confounds in FC estimates (Rangaprakash et al., 2017b,c, 2018b; Yan et al., 2017, 2018). In fact, our study reported significant HRFv in the brain’s default mode network (DMN) that confounded FC by about 14.7% (Rangaprakash et al., 2018b). This error in FC estimates due to HRFv (FC-error) was smaller for within-lobe FC (12.6%) vs. between-lobes (15.6%), perhaps due to more variable vasculature in the latter case.
The HRF is not only different across individuals, but also different across clinical populations due to impairments in various factors contributing to HRFv. Our prior work has highlighted this in autism (Yan et al., 2018), post-traumatic stress disorder (PTSD) (Rangaprakash et al., 2017b), obsessive–compulsive disorder (OCD) (Rangaprakash et al., 2020), bipolar disorder and schizophrenia (Yan et al., 2022). They also demonstrated that HRF impairments are significant enough to confound FC group differences; and sometimes the confound was of a similar order of magnitude as FC impairments in these diseases. Other labs have made similar observations. For example, another study found that HRF alterations confound FC (Archila-Meléndez et al., 2020). HRFv also confounded rat FC (Peng et al., 2019). Using variable HRFs that are person-specific (vs. fixed HRF) improved connectivity estimates (Duffy et al., 2021). Taken together, emerging evidence indicates that HRFv is concerning for rs-fMRI connectivity and clinical applications.
Non-invasive measurements are not as “clean” as invasive ones. There is always an effort to make fMRI data as “clean” as possible by maximizing relative variance from neural sources. Examples of such efforts include minimizing physiological/thermal noise through ultra-high-field imaging (7T or greater field strength; Barry et al., 2018), improved acquisition sequences (Barth et al., 2016; Islam et al., 2019), and better denoising (Glover et al., 2000; Power et al., 2015). Today we are in a reproducibility crisis in functional imaging (Gorgolewski and Poldrack, 2016; Poldrack et al., 2017; Osmanlıoğlu et al., 2020), indicating that further advancements are needed to make this technology clinically more useful. We still do not understand the substantial intra- and inter-subject variability of fMRI outcomes. Further characterization of this variability is timely to maximize the percentage variance in BOLD explained by the underlying neural activity.
Effective modeling of HRFv could potentially contribute to this effort. Like head motion or physiological noise, HRFv is an undesirable confound reducing fMRI data fidelity. We predict that minimizing HRFv in fMRI data will improve data quality and enhance clinical discovery. Concerns about the HRF confound also exists among the broader neuroscience community. Examples include the viewpoint of cellular neuroscientists (Hall et al., 2016), special issue articles on HRFv (Ekstrom, 2021), studies on non-neural factors and BOLD (Das et al., 2021), and investigating the link between fMRI and neural activity (Mishra et al., 2021).
Evidence suggests that HRFv is relevant for clinical and geriatric research. HRFs in older adults are different from their younger counterparts. Older adults have longer TTP and shorter RH, largely due to vascular factors (West et al., 2019). Such change is associated with Alzheimer’s disease as well (Shan et al., 2016). Longer TTP is also linked to reduced intelligence (Anderson et al., 2020). Aberrant HRFs have been observed in stress (Elbau et al., 2018), mild traumatic brain injury (Mayer et al., 2014), aging (West et al., 2019; Yabluchanskiy et al., 2020; Tsvetanov et al., 2021), isolated cervical dystonia (Berman et al., 2020), and levels of consciousness (Gemma et al., 2009; Wu et al., 2019). Such HRF changes are concerning because they are at least partly driven by non-neural factors and can confound FC group differences. Although studying non-neural factors in brain disorders is a valid enterprise, attributing FC group differences entirely to neural activity is problematic. HRFv in neurological disorders is yet to be investigated; however, our prior work in psychiatric conditions [autism (Yan et al., 2018), PTSD (Rangaprakash et al., 2017b), schizophrenia and bipolar disorder (Yan et al., 2022)] demonstrated that HRF impairments in these conditions invariably confounds FC group differences, and the confound can sometimes be of the same order of magnitude as group differences. Taken together, HRFv has implications across a spectrum of cognitive, psychiatric and geriatric domains, and perhaps also in other cases in which HRFv has yet to be investigated.
Non-neural factors that affect the HRF also differ across two highly relevant variables – age and sex. Aging causes vascular degradation; blood vessels of older adults are stiffer and less pulsatile, typically resulting in weaker BOLD responses to the same magnitude of neural activity (i.e., shorter RH) as well as longer time for peak BOLD activity (longer TTP; West et al., 2019; Yabluchanskiy et al., 2020; Tsvetanov et al., 2021). There is evidence for altered HRF in older adults (West et al., 2019; Tsvetanov et al., 2021), although the confound of HRFv on young vs. old FC group differences has not yet been studied. There is motivation to hypothesize that accounting for HRFv is essential for minimizing these vascular and other non-neural age-related confounds in rs-fMRI data. In fact, a recent report (Tsvetanov et al., 2021) noted that “vascular confounds in fMRI studies are common. Despite over 10,000 BOLD-fMRI papers on aging, fewer than 20 have applied techniques to correct for vascular effects.”
Factors affecting the HRF also differ between women and men. Men have lower CBF independent of neural activity (Ibaraki et al., 2010; Aanerud et al., 2017), which affects the HRF (Golestani et al., 2016; Kim and Ress, 2016). Other factors that exhibit sex differences include vascular physiology (Boese et al., 2017), capillary microcirculation (Huxley and Kemp, 2018), and overall cerebral hemodynamics (Barnes, 2017). Ignoring these factors by assuming a fixed canonical HRF is a potential confound in rs-fMRI studies that report data from both sexes, and/or perform women vs. men group comparisons. Despite this, HRF sex differences have never been directly studied. Hence, we next present limited data in this context.
We utilized 7 T rs-fMRI data from our earlier HRF study [N = 47, 22F/25M, healthy adults; Rangaprakash et al., 2018b; data made public (Rangaprakash et al., 2018c)] (please refer to these publications for data details). Kindly note that since this is a perspective article, we have not presented comprehensive results, but we hope that the results herein will encourage extensive follow-up studies. Briefly, upon standard pre-processing, we extracted mean region-of-interest (ROI) time series from DMN regions defined by the Power-Petersen atlas (Power et al., 2011) and estimated FC between all DMN ROI pairs using Pearson’s correlation (Mejia et al., 2018; Noble et al., 2019). This procedure was repeated for two separate pipelines: data with deconvolution (DC) and no deconvolution (NDC), which differed only in HRFv. We also obtained HRF parameters for each ROI during deconvolution.
We compared ROI-level HRF parameters between men and women (p < 0.05, FDR corrected). T-test was used for RH, and Wilcoxon rank-sum test was used for discrete variables (TTP, FWHM). There were no RH differences, but men had significantly longer TTP and/or FWHM in four regions (Figure 2A). Longer TTP/FWHM in men could be due to CBF and vascular differences between sexes (Ibaraki et al., 2010; Golestani et al., 2016; Aanerud et al., 2017; Barnes, 2017; Boese et al., 2017; Huxley and Kemp, 2018). The mean values averaged across all ROIs and subjects were as follows: RH (women = 5.01, men = 4.77), TTP (women = 5.61 s, men = 5.73 s), FWHM (women = 5.62 s, men = 5.79 s).
Figure 2. HRF results for women vs. men. (A) Comparing HRF parameters between men and women, showing the four regions that exhibited significant HRF time-to-peak (TTP) and/or full-width-at-half-max (FWHM) differences. TTP and/or FWHM were significantly higher in men. The 5th and 95th percentile values were used for range. (B) A flowchart illustrating our methodology to compute errors in FC estimates arising from HRF variability, and the results presented below that. The histogram shows the distribution of % FC error arising from men vs. women HRF differences. The median FC error was 15.4%, with a 95% confidence interval of [1.5–131.6%].
Next, we computed the error in FC group difference T-stats resulting from HRF differences. For this, women vs. men FC stats were performed separately for DC and NDC pipelines, and the percentage difference in resulting statistical maps was computed (Figure 2B). HRF differences between sexes resulted in a 15.4% median FC error. After thresholding (p < 0.05, FDR corrected), all three identified FCs were false positives (FC of temporal_inf_L/frontal_sup_L/fusiform_L with temporal_mid_L). Although only four regions and three connections exhibited significant HRF and FC differences, respectively, it must not be inferred that the rest of the regions or connections were unaffected by HRFv. These HRF/FC differences were large enough to be detected for our sample size and data quality, but that does not mean that the difference can be ignored in the other connections. The testament to this fact is that HRF differences between sexes resulted in 15.4% median FC error with contribution even from a vast number of regions/FCs outside of the significant ones (Figure 2). Our prior work has demonstrated the same (Rangaprakash et al., 2017b, 2018b; Yan et al., 2018, 2022). Taken together, a measurable portion of sex differences in DMN were attributable to HRFv.
As a secondary result not related to sex differences, we also report whole-brain-level average metrics of variability in HRF parameters within and between healthy young adult subjects, because these were not reported in our earlier publication (Rangaprakash et al., 2018b), but they support our conclusions and encourage future work. Of course, for the nature of this article, these results could be more complex and extensive. Using the same FC data, we (i) computed within-subjects variability of each HRF parameter as the mean percentage difference between all ROI pairs; and (ii) computed between-subjects variability of each HRF parameter as the mean percentage difference between all pairs of subjects. We used a t-test for RH and a rank-sum test for TTP/FWHM (p < 0.05, FDR corrected). Within-subjects, we found on average 14.1% RH variability, 13.5% TTP and 13.4% FWHM variability. L/R orbitofrontal had the largest HRF difference with other regions (86%/65% RH, 43%/24% TTP, 42%/24% FWHM variability), perhaps due to susceptibility (Buxton, 2010) and/or vascular (Tsvetanov et al., 2021) differences. Temporal lobe had larger variability than other lobes in RH (p = 0.034), TTP (p = 0.039) and FWHM (p = 0.037). Between-lobe variability was higher than within-lobe in RH (p = 0.039), TTP (p = 0.028) and FWHM (p = 0.041) (possibly due to vastly different vasculature across lobes). Between-subjects HRFv (RH: 29.8%, TTP: 28.5%, FWHM: 28.1%) was larger than within-subjects. The occipital lobe varied significantly less across subjects than other lobes in RH (p = 0.048) and TTP (p = 0.045).
The central nervous system (CNS), which includes the brain and the spinal cord, is a single continuous entity. But prior HRF literature is exclusively focused on the brain. Spinal cord rs-fMRI studies have so far not accounted for HRFv, except for a recent study (Kinany et al., 2020). If systematic brain HRF changes in pathological conditions translate to the cord, it is concerning because the cord is clinically relevant for several neurological diseases [e.g., multiple sclerosis (Conrad et al., 2018), chronic pain (Reckziegel et al., 2019), amyotrophic lateral sclerosis (de Albuquerque et al., 2017), transverse myelitis (Cacciaguerra et al., 2019), ataxia (Faber et al., 2021), and spinal cord injury (Freund et al., 2019)]. Cord impairments are being discovered in other pathologies [Alzheimer’s disease (Lorenzi et al., 2020) and cerebral palsy (Trevarrow et al., 2021)], suggesting that more disorders could involve the cord than we currently understand. HRFv could confound cord FC impairments in these diseases as well. Hence, characterizing HRFv in the spinal cord is clinically relevant and novel.
Herein we described prior evidence for HRFv and its confound on rs-fMRI FC and elaborated on this research’s importance and clinical relevance. Unexplained variability in BOLD is a more significant concern today than before because connectivity is used in sophisticated contexts such as dynamics (Preti et al., 2016), single-subject-level prediction (Jollans et al., 2019), laminar fMRI (Finn et al., 2020), and precision medicine (Finn et al., 2015). HRFv matters to a larger extent for all the desired ‘precision’ and fidelity expected of rs-fMRI today. With fast fMRI acquisition becoming prevalent (Preibisch et al., 2015; Barth et al., 2016), accounting for HRFv is even more critical (Lewis et al., 2018) to determine the neural/vascular origin of fMRI timing differences. Thus far, FC error arising only from spatial HRFv has been quantified, and only in parts of the brain and in small samples (Rangaprakash et al., 2018b). We provided limited data for FC error between sexes. Further research is required to quantify HRFv and FC error across various within- and between-subject comparison scenarios and demographic variables.
Taken together, measurements of FC often involve unexplained variance between 40% (ML prediction) and 70% (behavioral association; Finn et al., 2015; Jollans et al., 2019). While the underlying prediction/association models may be statistically significant and perform above chance, there is still a sizeable unexplained variance. We, therefore, argue that the HRF confound, typically in the range of 10–30%, may explain a part of this variance. We thus conclude that HRFv cannot be ignored in rs-fMRI studies, and it should be commonly accounted for during rs-fMRI data pre-processing.
The datasets presented in this study can be found in online repositories. The names of the repository/repositories and accession number(s) can be found at: doi.org/10.1016/j.dib.2018.01.003.
The studies involving human participants were reviewed and approved by Auburn University Institutional Review Board. The patients/participants provided their written informed consent to participate in this study.
DR: conceptualization, methodology, software, data analysis, investigation, visualization, writing–original draft, and reviewing and editing. RB: investigation, writing–reviewing and editing, and supervision. GD: conceptualization, methodology, data acquisition, investigation, writing–reviewing and editing, and supervision. All authors contributed to the article and approved the submitted version.
This work was supported by National Institutes of Health (NIH) through grants R01EB027779 and R21EB031211 (RB) and R01EY025978 (GD). Support was also received through the Athinoula A. Martinos Center for Biomedical Imaging (RB) and the Auburn University MRI Research Center (GD). The content is solely the responsibility of the authors and does not necessarily represent the official views of the NIH.
The authors declare that the research was conducted in the absence of any commercial or financial relationships that could be construed as a potential conflict of interest.
All claims expressed in this article are solely those of the authors and do not necessarily represent those of their affiliated organizations, or those of the publisher, the editors and the reviewers. Any product that may be evaluated in this article, or claim that may be made by its manufacturer, is not guaranteed or endorsed by the publisher.
Aanerud, J., Borghammer, P., Rodell, A., Jónsdottir, K. Y., and Gjedde, A. (2017). Sex differences of human cortical blood flow and energy metabolism. J. Cereb. Blood Flow Metab. 37, 2433–2440. doi: 10.1177/0271678X16668536
Aguirre, G. K., Zarahn, E., and D'esposito, M. (1998). The variability of human, BOLD hemodynamic responses. NeuroImage 8, 360–369. doi: 10.1006/nimg.1998.0369
Amico, E., Gomez, F., Di Perri, C., Vanhaudenhuyse, A., Lesenfants, D., Boveroux, P., et al. (2014). Posterior cingulate cortex-related co-activation patterns: a resting state FMRI study in propofol-induced loss of consciousness. PLoS One 9:e100012. doi: 10.1371/journal.pone.0100012
Anderson, A. E., Diaz-Santos, M., Frei, S., Dang, B. H., Kaur, P., Lyden, P., et al. (2020). Hemodynamic latency is associated with reduced intelligence across the lifespan: an fMRI DCM study of aging, cerebrovascular integrity, and cognitive ability. Brain Struct. Funct. 225, 1705–1717. doi: 10.1007/s00429-020-02083-w
Archila-Meléndez, M. E., Sorg, C., and Preibisch, C. (2020). Modeling the impact of neurovascular coupling impairments on BOLD-based functional connectivity at rest. NeuroImage 218:116871. doi: 10.1016/j.neuroimage.2020.116871
Badillo, S., Vincent, T., and Ciuciu, P. (2013). Group-level impacts of within- and between-subject hemodynamic variability in fMRI. NeuroImage 82, 433–448. doi: 10.1016/j.neuroimage.2013.05.100
Barnes, J. N. (2017). Sex-specific factors regulating pressure and flow. Exp. Physiol. 102, 1385–1392. doi: 10.1113/EP086531
Barry, R. L., Vannesjo, S. J., By, S., Gore, J. C., and Smith, S. A. (2018). Spinal cord MRI at 7T. NeuroImage 168, 437–451. doi: 10.1016/j.neuroimage.2017.07.003
Barth, M., Breuer, F., Koopmans, P., Norris, D., and Poser, B. (2016). Simultaneous multislice (SMS) imaging techniques. Magn. Reson. Med. 75, 63–81. doi: 10.1002/mrm.25897
Berman, B. D., Groth, C. L. S. E., Sillau, S. H., Sutton, B., Legget, K. T., and Tregellas, J. R. (2020). Hemodynamic responses are abnormal in isolated cervical dystonia. J. Neurosci. Res. 98, 692–703. doi: 10.1002/jnr.24547
Bernier, M., Cunnane, S. C., and Whittingstall, K. (2018). The morphology of the human cerebrovascular system. Hum. Brain Mapp. 39, 4962–4975. doi: 10.1002/hbm.24337
Biessmann, F., Murayama, Y., Logothetis, N., Müller, K., and Meinecke, F. (2012). Improved decoding of neural activity from fMRI signals using non-separable spatiotemporal deconvolutions. NeuroImage 61, 1031–1042. doi: 10.1016/j.neuroimage.2012.04.015
Boese, A. C., Kim, S. C., Yin, K. J., Lee, J. P., and Hamblin, M. H. (2017). Sex differences in vascular physiology and pathophysiology: estrogen and androgen signaling in health and disease. Am. J. Physiol. Heart Circ. Physiol. 313, H524–H545. doi: 10.1152/ajpheart.00217.2016
Boly, M., Sasai, S., Gosseries, O., Oizumi, M., Casali, A., Massimini, M., et al. (2015). Stimulus set meaningfulness and neurophysiological differentiation: a functional magnetic resonance imaging study. PLoS One 10:e0125337. doi: 10.1371/journal.pone.0125337
Bright, M. G., Whittaker, J. R., Driver, I. D., and Murphy, K. (2020). Vascular physiology drives functional brain networks. NeuroImage 217:116907. doi: 10.1016/j.neuroimage.2020.116907
Bush, K., and Cisler, J. (2013). Decoding neural events from fMRI BOLD signal: a comparison of existing approaches and development of a new algorithm. Magn. Reson. Imaging 31, 976–989. doi: 10.1016/j.mri.2013.03.015
Bush, K., Cisler, J., Bian, J., Hazaroglu, G., Hazaroglu, O., and Kilts, C. (2015). Improving the precision of fMRI BOLD signal deconvolution with implications for connectivity analysis. Magn. Reson. Imaging 33, 1314–1323. doi: 10.1016/j.mri.2015.07.007
Buxton, R. B. (2010). Introduction to functional magnetic resonance imaging: principles and techniques. 2nd Edn. Cambridge: Cambridge University Press.
Caballero Gaudes, C., Petridou, N., Francis, S. T., Dryden, I. L., and Gowland, P. A. (2013). Paradigm free mapping with sparse regression automatically detects single-trial functional magnetic resonance imaging blood oxygenation level dependent responses. Hum. Brain Mapp. 34, 501–518. doi: 10.1002/hbm.21452
Cacciaguerra, L., Meani, A., Mesaros, S., Radaelli, M., Palace, J., Dujmovic-Basuroski, I., et al. (2019). Brain and cord imaging features in neuromyelitis optica spectrum disorders. Ann. Neurol. 85, 371–384. doi: 10.1002/ana.25411
Chang, C., Thomason, M. E., and Glover, G. H. (2008). Mapping and correction of vascular hemodynamic latency in the BOLD signal. NeuroImage 43, 90–102. doi: 10.1016/j.neuroimage.2008.06.030
Cherkaoui, H., Moreau, T., Halimi, A., and Ciuciu, P., (2019). “Sparsity-based blind Deconvolution of neural activation signal in FMRI,” in IEEE International Conference on Acoustics, Speech and Signal Processing (ICASSP), Brighton, UK.
Conrad, B. N., Barry, R. L., Rogers, B. P., Maki, S., Mishra, A., Thukral, S., et al. (2018). Multiple sclerosis lesions affect intrinsic functional connectivity of the spinal cord. Brain 141, 1650–1664. doi: 10.1093/brain/awy083
Das, A., Murphy, K., and Drew, P. J. (2021). Rude mechanicals in brain haemodynamics: non-neural actors that influence blood flow. Philos. Trans. R. Soc. Lond. Ser. B Biol. Sci. 376:20190635. doi: 10.1098/rstb.2019.0635
David, O., Guillemain, I., Saillet, S., Reyt, S., Deransart, S., Segebarth, C., et al. (2008). Identifying neural drivers with functional MRI: an electrophysiological validation. PLoS Biol. 6, 2683–2697. doi: 10.1371/journal.pbio.0060315
de Albuquerque, M., Branco, L. M., Rezende, T. J., de Andrade, H. M., Nucci, A., and França, M. C. (2017). Longitudinal evaluation of cerebral and spinal cord damage in amyotrophic lateral sclerosis. Neuroimage: Clinical 14, 269–276. doi: 10.1016/j.nicl.2017.01.024
de Zwart, J. A., Silva, A. C., van Gelderen, P., Kellman, P., Fukunaga, M., Chu, R., et al. (2005). Temporal dynamics of the BOLD fMRI impulse response. NeuroImage 24, 667–677. doi: 10.1016/j.neuroimage.2004.09.013
Deshpande, G., Sathian, K., and Hu, X. (2010). Effect of hemodynamic variability on granger causality analysis of fMRI. NeuroImage 52, 884–896. doi: 10.1016/j.neuroimage.2009.11.060
Duarte, J. V., Pereira, J. M., Quendera, B., Raimundo, M., Moreno, C., Gomes, L., et al. (2015). Early disrupted neurovascular coupling and changed event level hemodynamic response function in type 2 diabetes: an fMRI study. J. Cereb. Blood Flow Metab. 35, 1671–1680. doi: 10.1038/jcbfm.2015.106
Duffy, K. A., Fisher, Z. F., Arizmendi, C. A. M. P., Hopfinger, J., Cohen, J. R., Beltz, A. M., et al. (2021). Detecting task-dependent functional connectivity in group iterative multiple model estimation with person-specific hemodynamic response functions. Brain Connect. 11, 418–429. doi: 10.1089/brain.2020.0864
Ekstrom, A. D. (2021). Regional variation in neurovascular coupling and why we still lack a Rosetta stone. Philos. Trans. R. Soc. Lond. Ser. B Biol. Sci. 376:20190634. doi: 10.1098/rstb.2019.0634
Elbau, I. G., Brücklmeier, B., Uhr, M., Arloth, J., Czamara, D., Spoormaker, V. I., et al. (2018). The brain's hemodynamic response function rapidly changes under acute psychosocial stress in association with genetic and endocrine stress response markers. Proc. Natl. Acad. Sci. U. S. A. 115, E10206–E10215. doi: 10.1073/pnas.1804340115
Faber, J., Schaprian, T., Berkan, K., Reetz, K., França, M. C., De Rezende, T., et al. (2021). Regional brain and spinal cord volume loss in Spinocerebellar Ataxia type 3. Mov. Disord. 36, 2273–2281. doi: 10.1002/mds.28610
Feige, B., Spiegelhalder, K., Kiemen, A., Bosch, O. G., Tebartz van Elst, L., Hennig, J., et al. (2017). Distinctive time-lagged resting-state networks revealed by simultaneous EEG-fMRI. NeuroImage 145, 1–10. doi: 10.1016/j.neuroimage.2016.09.027
Feng, C., Deshpande, G., Liu, C., Gu, R., Luo, Y.-J., and Krueger, F. (2015). Diffusion of responsibility attenuates altruistic punishment: a functional magnetic resonance imaging effective connectivity study. Hum. Brain Mapp. 37, 663–677. doi: 10.1002/hbm.23057
Finn, E. S., Huber, L., and Bandettini, P. A. (2020). Higher and deeper: bringing layer fMRI to association cortex. Prog. Neurobiol. 207:101930. doi: 10.1016/j.pneurobio.2020.101930
Finn, E. S., Shen, X., Scheinost, D., Rosenberg, M. D., Huang, J., Chun, M. M., et al. (2015). Functional connectome fingerprinting: identifying individuals using patterns of brain connectivity. Nat. Neurosci. 18, 1664–1671. doi: 10.1038/nn.4135
Freund, P., Seif, M., Weiskopf, N., Friston, K., Fehlings, M. G., Thompson, A. J., et al. (2019). MRI in traumatic spinal cord injury: from clinical assessment to neuroimaging biomarkers. Lancet Neurol. 18, 1123–1135. doi: 10.1016/S1474-4422(19)30138-3
Friston, K. J., Harrison, L., and Penny, W. (2013). Dynamic causal modelling. NeuroImage 19, 1273–1302. doi: 10.1016/S1053-8119(03)00202-7
Friston, K. J., Kahan, J., Biswal, B., and Razi, A. (2014). A DCM for resting state fMRI. NeuroImage 94, 396–407. doi: 10.1016/j.neuroimage.2013.12.009
Gaudes, C. C., Petridou, N., Dryden, I. L., Bai, L., Francis, S. T., and Gowland, P. A. (2011). Detection and characterization of single-trial fMRI bold responses: paradigm free mapping. Hum. Brain Mapp. 32, 1400–1418. doi: 10.1002/hbm.21116
Gemma, M., de Vitis, A., Baldoli, C., Calvi, M. R., Blasi, V., Scola, E., et al. (2009). Functional magnetic resonance imaging (fMRI) in children sedated with propofol or midazolam. J. Neurosurg. Anesthesiol. 21, 253–258. doi: 10.1097/ANA.0b013e3181a7181d
Gitelman, D. R., Penny, W. D., Ashburner, J., and Friston, K. J. (2003). Modeling regional and psychophysiologic interactions in fMRI: the importance of hemodynamic deconvolution. NeuroImage 19, 200–207. doi: 10.1016/S1053-8119(03)00058-2
Glover, G. (1999). Deconvolution of impulse response in event-related BOLD fMRI. NeuroImage 9, 416–429. doi: 10.1006/nimg.1998.0419
Glover, G. H., Li, T. Q., and Ress, D. (2000). Image-based method for retrospective correction of physiological motion effects in fMRI: RETROICOR. Magn. Reson. Med. 44, 162–167. doi: 10.1002/1522-2594(200007)44:1<162::AID-MRM23>3.0.CO;2-E
Golestani, A. M., Wei, L. L., and Chen, J. J. (2016). Quantitative mapping of cerebrovascular reactivity using resting-state BOLD fMRI: validation in healthy adults. NeuroImage 138, 147–163. doi: 10.1016/j.neuroimage.2016.05.025
Gonzalez-Castillo, J., Saad, Z., Handwerker, D., Inati, S., Brenowitz, N., and Bandettini, P. (2012). Whole-brain, time-locked activation with simple tasks revealed using massive averaging and model-free analysis. Proc. Natl. Acad. Sci. U. S. A. 109, 5487–5492. doi: 10.1073/pnas.1121049109
Gorgolewski, K. J., and Poldrack, R. A. (2016). A practical guide for improving transparency and reproducibility in neuroimaging research. PLoS Biol. 14:e1002506. doi: 10.1371/journal.pbio.1002506
Hall, C. N., Howarth, C., Kurth-Nelson, Z., and Mishra, A. (2016). Interpreting BOLD: towards a dialogue between cognitive and cellular neuroscience. Philos. Trans. R. Soc. Lond. B 371:20150348. doi: 10.1098/rstb.2015.0348
Hampstead, B. M., Khoshnoodi, M., Yan, W., Deshpande, G., and Sathian, K. (2016). Patterns of effective connectivity between memory encoding and retrieval differ between patients with mild cognitive impairment and healthy older adults. NeuroImage 124, 997–1008. doi: 10.1016/j.neuroimage.2015.10.002
Handwerker, D. A., Gonzalez-Castillo, J., D'Esposito, M., and Bandettini, P. A. (2012). The continuing challenge of understanding and modeling hemodynamic variation in fMRI. NeuroImage 62, 1017–1023. doi: 10.1016/j.neuroimage.2012.02.015
Handwerker, D. A., Ollinger, J. M., and D'Esposito, M. (2004). Variation of BOLD hemodynamic responses across subjects and brain regions and their effects on statistical analyses. NeuroImage 21, 1639–1651. doi: 10.1016/j.neuroimage.2003.11.029
Havlicek, M., Friston, K. J., Jan, J., Brazdil, M., and Calhoun, V. D. (2011). Dynamic modeling of neuronal responses in fMRI using cubature Kalman filtering. NeuroImage 56, 2109–2128. doi: 10.1016/j.neuroimage.2011.03.005
Havlicek, M., Jan, J., Brazdil, M., and Calhoun, V. (2010). Dynamic granger causality based on Kalman filter for evaluation of functional network connectivity in fMRI data. NeuroImage 53, 65–77. doi: 10.1016/j.neuroimage.2010.05.063
Havlicek, M., Roebroeck, A., Friston, K., Gardumi, A., Ivanov, D., and Uludag, K. (2015). Physiologically informed dynamic causal modeling of fMRI data. NeuroImage 122, 355–372. doi: 10.1016/j.neuroimage.2015.07.078
Hernandez-Garcia, L., and Ulfarsson, M. O. (2011). Neuronal event detection in fMRI time series using iterative deconvolution techniques. Magn. Reson. Imaging 29, 353–364. doi: 10.1016/j.mri.2010.10.012
Huxley, V. H., and Kemp, S. S. (2018). Sex-specific characteristics of the microcirculation. Adv. Exp. Med. Biol. 1065, 307–328. doi: 10.1007/978-3-319-77932-4_20
Ibaraki, M., Shinohara, Y., Nakamura, K., Miura, S., Kinoshita, F., and Kinoshita, T. (2010). Interindividual variations of cerebral blood flow, oxygen delivery, and metabolism in relation to hemoglobin concentration measured by positron emission tomography in humans. J. Cereb. Blood Flow Metab. 30, 1296–1305. doi: 10.1038/jcbfm.2010.13
Islam, H., Law, C., Weber, K. A., Mackey, S. C., and Glover, G. H. (2019). Dynamic per slice shimming for simultaneous brain and spinal cord fMRI. Magn. Reson. Med. 81, 825–838. doi: 10.1002/mrm.27388
Jollans, L., Boyle, R., Artiges, E., Banaschewski, T., Desrivières, S., Grigis, A., et al. (2019). Quantifying performance of machine learning methods for neuroimaging data. NeuroImage 199, 351–365. doi: 10.1016/j.neuroimage.2019.05.082
Karahanoğlu, F. I., Caballero-Gaudes, C., Lazeyras, F., and Van de Ville, D. (2013). Total activation: fMRI deconvolution through spatio-temporal regularization. NeuroImage 73, 121–134. doi: 10.1016/j.neuroimage.2013.01.067
Karahanoğlu, F. I., and Van De Ville, D. (2015). Transient brain activity disentangles fMRI resting-state dynamics in terms of spatially and temporally overlapping networks. Nat. Commun. 6:7751. doi: 10.1038/ncomms8751
Khalidov, I., Fadili, J., Lazeyras, F., Van De Ville, D., and Unser, M. (2011). Activelets: wavelets for sparse representation of hemodynamic responses. Signal Process. 91, 2810–2821. doi: 10.1016/j.sigpro.2011.03.008
Kim, J. H., and Ress, D. (2016). Arterial impulse model for the BOLD response to brief neural activation. NeuroImage 124, 394–408. doi: 10.1016/j.neuroimage.2015.08.068
Kinany, N., Pirondini, E., Micera, S., and Van De Ville, D. (2020). Dynamic functional connectivity of resting-state spinal cord fMRI reveals fine-grained intrinsic architecture. Neuron 108, 424–435.e4. doi: 10.1016/j.neuron.2020.07.024
Lacey, S., Stilla, R., Sreenivasan, K., Deshpande, G., and Sathian, K. (2014). Spatial imagery in haptic shape perception. Neuropsychologia 60, 144–158. doi: 10.1016/j.neuropsychologia.2014.05.008
Lamichhane, B., Adhikari, B. M., Brosnan, S. F., and Dhamala, M. (2014). The neural basis of perceived unfairness in economic exchanges. Brain Connect. 4, 619–630. doi: 10.1089/brain.2014.0243
Levin, J., Ross, M., Mendelson, J., Kaufman, M., Lange, N., Maas, L., et al. (1998). Reduction in BOLD fMRI response to primary visual stimulation following alcohol ingestion. Psychiatry Res. 82, 135–146. doi: 10.1016/S0925-4927(98)00022-5
Lewis, L. D., Setsompop, K., Rosen, B. R., and Polimeni, J. R. (2018). Stimulus-dependent hemodynamic response timing across the human subcortical-cortical visual pathway identified through high spatiotemporal resolution 7T fMRI. NeuroImage 181, 279–291. doi: 10.1016/j.neuroimage.2018.06.056
Logothetis, N. K., Pauls, J., Augath, M., Trinath, T., and Oeltermann, A. (2001). Neurophysiological investigation of the basis of the fMRI signal. Nature 412, 150–157. doi: 10.1038/35084005
Lopes, R., Lina, J. M., Fahoum, F., and Gotman, J. (2012). Detection of epileptic activity in fMRI without recording the EEG. NeuroImage 60, 1867–1879. doi: 10.1016/j.neuroimage.2011.12.083
Lorenzi, R. M., Palesi, F., Castellazzi, G., Vitali, P., Anzalone, N., Bernini, S., et al. (2020). Unsuspected involvement of spinal cord in Alzheimer disease. Front. Cell. Neurosci. 14:6. doi: 10.3389/fncel.2020.00006
Mayer, A. R., Toulouse, T., Klimaj, S., Ling, J. M., Pena, A., and Bellgowan, P. S. (2014). Investigating the properties of the hemodynamic response function after mild traumatic brain injury. J. Neurotrauma 31, 189–197. doi: 10.1089/neu.2013.3069
McDonough, I. M., Bender, A., Patihis, L., Stinson, E. A., Letang, S. K., and Miller, W. S. (2019). The trouble interpreting fMRI studies in populations with cerebrovascular risk: the use of a subject-specific hemodynamic response function in a study of age, vascular risk, and memory. bioRxiv [Preprint]. doi: 10.1101/512343
Mejia, A. F., Nebel, M. B., Barber, A. D., Choe, A. S., Pekar, J. J., Caffo, B. S., et al. (2018). Improved estimation of subject-level functional connectivity using full and partial correlation with empirical Bayes shrinkage. NeuroImage 172, 478–491. doi: 10.1016/j.neuroimage.2018.01.029
Miezin, F. M., Maccotta, L., Ollinger, J. M., Petersen, S. E., and Buckner, R. L. (2000). Characterizing the hemodynamic response: effects of presentation rate, sampling procedure, and the possibility of ordering brain activity based on relative timing. NeuroImage 11, 735–759. doi: 10.1006/nimg.2000.0568
Mishra, A., Hall, C. N., Howarth, C., and Freeman, R. D. (2021). Key relationships between non-invasive functional neuroimaging and the underlying neuronal activity. Philos. Trans. R. Soc. Lond. Ser. B Biol. Sci. 376:20190622. doi: 10.1098/rstb.2019.0622
Nathan, P. J., Phan, K. L., Harmer, C. J., Mehta, M. A., and Bullmore, E. T. (2014). Increasing pharmacological knowledge about human neurological and psychiatric disorders through functional neuroimaging and its application in drug discovery. Curr. Opin. Pharmacol. 14, 54–61. doi: 10.1016/j.coph.2013.11.009
Noble, S., Scheinost, D., and Constable, R. (2019). A decade of test-retest reliability of functional connectivity: a systematic review and meta-analysis. NeuroImage 203:116157. doi: 10.1016/j.neuroimage.2019.116157
Noseworthy, M. D., Alfonsi, J., and Bells, S. (2003). Attenuation of brain BOLD response following lipid ingestion. Hum. Brain Mapp. 20, 116–121. doi: 10.1002/hbm.10131
Osmanlıoğlu, Y., Alappatt, J. A., Parker, D., and Verma, R., (2020). “Analysis of consistency in structural and functional connectivity of human brain,” in Proceedings. IEEE International Symposium on Biomedical Imaging.
Peng, S. L., Chen, C. M., Huang, C. Y., Shih, C. T., Huang, C. W., Chiu, S. C., et al. (2019). Effects of hemodynamic response function selection on rat fMRI statistical analyses. Front. Neurosci. 13:400. doi: 10.3389/fnins.2019.00400
Poldrack, R. A., Baker, C. I., Durnez, J., Gorgolewski, K. J., Matthews, P. M., Munafò, M. R., et al. (2017). Scanning the horizon: towards transparent and reproducible neuroimaging research. Nat. Rev. Neurosci. 18, 115–126. doi: 10.1038/nrn.2016.167
Power, J. D., Cohen, A. L., Nelson, S. M., Wig, G. S., Barnes, K. A., Church, J. A., et al. (2011). Functional network organization of the human brain. Neuron 72, 665–678. doi: 10.1016/j.neuron.2011.09.006
Power, J. D., Schlaggar, B. L., and Petersen, S. E. (2015). Recent progress and outstanding issues in motion correction in resting state fMRI. NeuroImage 105, 536–551. doi: 10.1016/j.neuroimage.2014.10.044
Preibisch, C., Castrillón, J., Bührer, M., and Riedl, V. (2015). Evaluation of multiband EPI acquisitions for resting state fMRI. PLoS One 10:e0136961. doi: 10.1371/journal.pone.0136961
Preti, M., Bolton, T., and Van De Ville, D. (2016). The dynamic functional connectome: state-of-the-art and perspectives. NeuroImage 160, 41–54. doi: 10.1016/j.neuroimage.2016.12.061
Rangaprakash, D., Deshpande, G., Daniel, T., Goodman, A., Robinson, J., Salibi, N., et al. (2017a). Compromised Hippocampus-striatum pathway as a potential imaging biomarker of mild traumatic brain injury and posttraumatic stress disorder. Hum. Brain Mapp. 38, 2843–2864. doi: 10.1002/hbm.23551
Rangaprakash, D., Dretsch, M. N., Venkatraman, A., Katz, J. S., Denney, T. S., and Deshpande, G. (2018a). Identifying disease foci from static and dynamic effective connectivity networks: illustration in soldiers with trauma. Hum. Brain Mapp. 39, 264–287. doi: 10.1002/hbm.23841
Rangaprakash, D., Dretsch, M. N., Yan, W., Katz, J. S., Denney, T. S., and Deshpande, G. (2017b). Hemodynamic variability in soldiers with trauma: implications for functional MRI connectivity studies. NeuroImage: Clinical 16, 409–417. doi: 10.1016/j.nicl.2017.07.016
Rangaprakash, D., Dretsch, M. N., Yan, W., Katz, J. S., Denney, T. S., and Deshpande, G. (2017c). Hemodynamic response function parameters obtained from resting-state functional MRI data in soldiers with trauma. Data Brief 14, 558–562. doi: 10.1016/j.dib.2017.07.072
Rangaprakash, D., Tadayonnejad, R., Deshpande, G., O'Neill, J., and Feusner, J. (2020). FMRI hemodynamic response function (HRF) as a novel marker of brain function: applications for understanding obsessive-compulsive disorder pathology and treatment response. Brain Imaging Behav. 15, 1622–1640. doi: 10.1007/s11682-020-00358-8
Rangaprakash, D., Wu, G.-R., Marinazzo, D., Hu, X., and Deshpande, G. (2018b). Hemodynamic response function (HRF) variability confounds resting-state fMRI functional connectivity. Magn. Reson. Med. 80, 1697–1713. doi: 10.1002/mrm.27146
Rangaprakash, D., Wu, G.-R., Marinazzo, D., Hu, X., and Deshpande, G. (2018c). Parameterized hemodynamic response function data of healthy individuals obtained from resting-state functional MRI in a 7T MRI scanner. Data Brief 17, 1175–1179. doi: 10.1016/j.dib.2018.01.003
Reckziegel, D., Vachon-Presseau, E., Petre, B., Schnitzer, T., Baliki, M., and Apkarian, A. (2019). Deconstructing biomarkers for chronic pain: context- and hypothesis-dependent biomarker types in relation to chronic pain. Pain 160, S37–S48. doi: 10.1097/j.pain.0000000000001529
Rosazza, C., and Minati, L. (2011). Resting-state brain networks: literature review and clinical applications. Neurol. Sci. 32, 773–785. doi: 10.1007/s10072-011-0636-y
Ryali, S., Chen, T., Supekar, K., Tu, T., Kochalka, J., Cai, W., et al. (2016a). Multivariate dynamical systems-based estimation of causal brain interactions in fMRI: group-level validation using benchmark data, neurophysiological models and human connectome project data. J. Neurosci. Methods 268, 142–153. doi: 10.1016/j.jneumeth.2016.03.010
Ryali, S., Shih, Y. Y., Chen, T., Kochalka, J., Albaugh, D., Fang, Z., et al. (2016b). Combining optogenetic stimulation and fMRI to validate a multivariate dynamical systems model for estimating causal brain interactions. NeuroImage 132, 398–405. doi: 10.1016/j.neuroimage.2016.02.067
Ryali, S., Supekar, K., Chen, T., and Menon, V. (2011). Multivariate dynamical systems models for estimating causal interactions in fMRI. NeuroImage 54, 807–823. doi: 10.1016/j.neuroimage.2010.09.052
Saad, Z. S., Gotts, S. J., Murphy, K., Chen, G., Jo, H. J., Martin, A., et al. (2012). Trouble at rest: how correlation patterns and group differences become distorted after global signal regression. Brain Connect. 2, 25–32. doi: 10.1089/brain.2012.0080
Shan, Z. Y., Vinkhuyzen, A., Thompson, P. M., McMahon, K. L., Blokland, G., de Zubicaray, G. I., et al. (2016). Genes influence the amplitude and timing of brain hemodynamic responses. NeuroImage 124, 663–671. doi: 10.1016/j.neuroimage.2015.09.016
Spano, V. R., Mandell, D. M., Poublanc, J., Sam, K., Battisti-Charbonney, A., Pucci, O., et al. (2013). CO2 blood oxygen level-dependent MR mapping of cerebrovascular reserve in a clinical population: safety, tolerability, and technical feasibility. Radiology 266, 592–598. doi: 10.1148/radiol.12112795
Sreenivasan, K. R., Havlicek, M., and Deshpande, G. (2015). Non-parametric hemodynamic deconvolution of fMRI using homomorphic filtering. IEEE Trans. Med. Imaging 34, 1155–1163. doi: 10.1109/TMI.2014.2379914
Tagliazucchi, E., Balenzuela, P., Fraiman, D., and Chialvo, D. R. (2012). Criticality in large-scale brain fMRI dynamics unveiled by a novel point process analysis. Front. Physiol. 3:15. doi: 10.3389/fphys.2012.00015
Tak, S., Polimeni, J. R., Wang, D. J., Yan, L., and Chen, J. J. (2015). Associations of resting-state fMRI functional connectivity with flow-BOLD coupling and regional vasculature. Brain Connect. 5, 137–146. doi: 10.1089/brain.2014.0299
Taylor, A. J., Kim, J. H., and Ress, D. (2018). Characterization of the hemodynamic response function across the majority of human cerebral cortex. NeuroImage 173, 322–331. doi: 10.1016/j.neuroimage.2018.02.061
Thomason, M. E., Foland, L. C., and Glover, G. H. (2007). Calibration of BOLD fMRI using breath holding reduces group variance during a cognitive task. Hum. Brain Mapp. 28, 59–68. doi: 10.1002/hbm.20241
Tong, Y., and Frederick, B. D. (2014). Tracking cerebral blood flow in BOLD fMRI using recursively generated regressors. Hum. Brain Mapp. 35, 5471–5485. doi: 10.1002/hbm.22564
Trevarrow, M. P., Baker, S. E., Wilson, T. W., and Kurz, M. J. (2021). Microstructural changes in the spinal cord of adults with cerebral palsy. Dev. Med. Child Neurol. 63, 998–1003. doi: 10.1111/dmcn.14860
Tsvetanov, K. A., Henson, R., and Rowe, J. B. (2021). Separating vascular and neuronal effects of age on fMRI BOLD signals. Philos. Trans. R. Soc. Lond. Ser. B Biol. Sci. 376:20190631. doi: 10.1098/rstb.2019.0631
Urback, A. L., MacIntosh, B. J., and Goldstein, B. I. (2017). Cerebrovascular reactivity measured by functional magnetic resonance imaging during breath-hold challenge: a systematic review. Neurosci. Biobehav. Rev. 79, 27–47. doi: 10.1016/j.neubiorev.2017.05.003
Wang, Y., David, O., Hu, X., and Deshpande, G. (2017a). Can Patel's τ accurately estimate directionality of connections in brain networks from fMRI? Magn. Reson. Med. 78, 2003–2010. doi: 10.1002/mrm.26583
Wang, Y., Katwal, S., Rogers, B., Gore, J., and Deshpande, G. (2017b). Experimental validation of dynamic granger causality for inferring stimulus-evoked sub-100ms timing differences from fMRI. IEEE Trans. Neural Syst. Rehabil. Eng. 25, 539–546. doi: 10.1109/TNSRE.2016.2593655
West, K. L., Zuppichini, M. D., Turner, M. P., Sivakolundu, D. K., Zhao, Y., Abdelkarim, D., et al. (2019). BOLD hemodynamic response function changes significantly with healthy aging. NeuroImage 188, 198–207. doi: 10.1016/j.neuroimage.2018.12.012
Wu, G., Colenbier, N., Van Den Bossche, S., Clauw, K., Johri, A., Tandon, M., et al. (2021). rsHRF: a toolbox for resting-state HRF estimation and deconvolution. NeuroImage 244:118591. doi: 10.1016/j.neuroimage.2021.118591
Wu, G. R., Di Perri, C., Charland-Verville, V., Martial, C., Carrière, M., Vanhaudenhuyse, A., et al. (2019). Modulation of the spontaneous hemodynamic response function across levels of consciousness. NeuroImage 200, 450–459. doi: 10.1016/j.neuroimage.2019.07.011
Wu, G. R., Liao, W., Stramaglia, S., Ding, J. R., Chen, H., and Marinazzo, D. (2013). A blind deconvolution approach to recover effective connectivity brain networks from resting state fMRI data. Med. Image Anal. 17, 365–374. doi: 10.1016/j.media.2013.01.003
Yabluchanskiy, A., Nyul-Toth, A., Csiszar, A., Gulej, R., Saunders, D., Towner, R., et al. (2020). Age-related alterations in the cerebrovasculature affect neurovascular coupling and BOLD fMRI responses: insights from animal models of aging. Psychophysiology 58:e13718. doi: 10.1111/psyp.13718
Yan, W., Palaniyappan, L., Liddle, P. F., Rangaprakash, D., Wei, W., and Deshpande, G. (2022). Characterization of hemodynamic alterations in schizophrenia and bipolar disorder and their effect on resting-state fMRI functional connectivity. Schizophr. Bull. 48, 695–711. doi: 10.1093/schbul/sbab140
Yan, W., Rangaprakash, D., and Deshpande, G. (2017). Hemodynamic response function parameters obtained from resting state BOLD fMRI data in subjects with autism Spectrum disorder and matched healthy controls. Data Brief 14, 558–562. doi: 10.1016/j.dib.2017.07.072
Yan, W., Rangaprakash, D., and Deshpande, G. (2018). Aberrant hemodynamic responses in autism: implications for resting state fMRI functional connectivity studies. NeuroImage: Clinical 19, 320–330. doi: 10.1016/j.nicl.2018.04.013
Yang, H. S., Liang, Z., Yao, J. F., Shen, X., Frederick, B. D., and Tong, Y. (2019). Vascular effects of caffeine found in BOLD fMRI. J. Neurosci. Res. 97, 456–466. doi: 10.1002/jnr.24360
Keywords: BOLD fMRI, HRF, resting state connectivity (rsfMRI), aging, sex differences, confound
Citation: Rangaprakash D, Barry RL and Deshpande G (2023) The confound of hemodynamic response function variability in human resting-state functional MRI studies. Front. Neurosci. 17:934138. doi: 10.3389/fnins.2023.934138
Received: 02 May 2022; Accepted: 07 April 2023;
Published: 14 July 2023.
Edited by:
Xi-Nian Zuo, Beijing Normal University, ChinaReviewed by:
Fuqiang Zhao, Takeda, United StatesCopyright © 2023 Rangaprakash, Barry and Deshpande. This is an open-access article distributed under the terms of the Creative Commons Attribution License (CC BY). The use, distribution or reproduction in other forums is permitted, provided the original author(s) and the copyright owner(s) are credited and that the original publication in this journal is cited, in accordance with accepted academic practice. No use, distribution or reproduction is permitted which does not comply with these terms.
*Correspondence: D. Rangaprakash, UkRlc2hwYW5kZTNAbWdoLmhhcnZhcmQuZWR1
Disclaimer: All claims expressed in this article are solely those of the authors and do not necessarily represent those of their affiliated organizations, or those of the publisher, the editors and the reviewers. Any product that may be evaluated in this article or claim that may be made by its manufacturer is not guaranteed or endorsed by the publisher.
Research integrity at Frontiers
Learn more about the work of our research integrity team to safeguard the quality of each article we publish.