- 1Department of Pathology, Stanford University, Stanford, CA, United States
- 2Department of Anesthesiology, Stanford University, Stanford, CA, United States
- 3Department of Biomedical Data Science, Stanford University, Stanford, CA, United States
- 4Department of Computer Science, Stanford University, Stanford, CA, United States
- 5Department of Genome Sciences, University of Washington, Seattle, WA, United States
The cellular and molecular distinction between brain aging and neurodegenerative disease begins to blur in the oldest old. Approximately 15–25% of observations in humans do not fit predicted clinical manifestations, likely the result of suppressed damage despite usually adequate stressors and of resilience, the suppression of neurological dysfunction despite usually adequate degeneration. Factors during life may predict the clinico-pathologic state of resilience: cardiovascular health and mental health, more so than educational attainment, are predictive of a continuous measure of resilience to Alzheimer’s disease (AD) and AD-related dementias (ADRDs). In resilience to AD alone (RAD), core features include synaptic and axonal processes, especially in the hippocampus. Future focus on larger and more diverse cohorts and additional regions offer emerging opportunities to understand this counterforce to neurodegeneration. The focus of this review is the molecular basis of resilience to AD.
1 Introduction
Neurodegenerative diseases span from newborns to centenarians and from ultra-rare to highly prevalent. The more prevalent neurodegenerative diseases are an emerging pandemic as the world’s age demographic historically shifts to older age because of success in treating acute diseases, and underscores the growing need to manage chronic diseases. Although age-related neuropathologies account for a large proportion of late life cognitive decline, considerable variation remains unexplained even after considering a wide array of neuropathologies (Boyle et al., 2021). Here we will focus on the most prevalent age-related neurodegenerative disease, Alzheimer’s disease (AD), and its commonly comorbid conspirators, the so-called AD-related dementias (ADRDs).
During our lifetimes, we varyingly experience episodic social and environmental stressors, some of which appear epidemiologically to have a major impact on the risk of disease, including AD and ADRDs (Hunt et al., 2020; Livingston et al., 2020; Besser, 2021; Peterson et al., 2021; Zhao et al., 2021; Chen et al., 2022). This is the first layer of often episodic stressors that impact the brain (Figure 1, green) and include life events such as social isolation and death of a spouse (Wu-Chung et al., 2022). The molecular mechanisms by which these social and environmental stressors influence the subsequent development of neurodegeneration are not yet clear but are an active area of investigation.
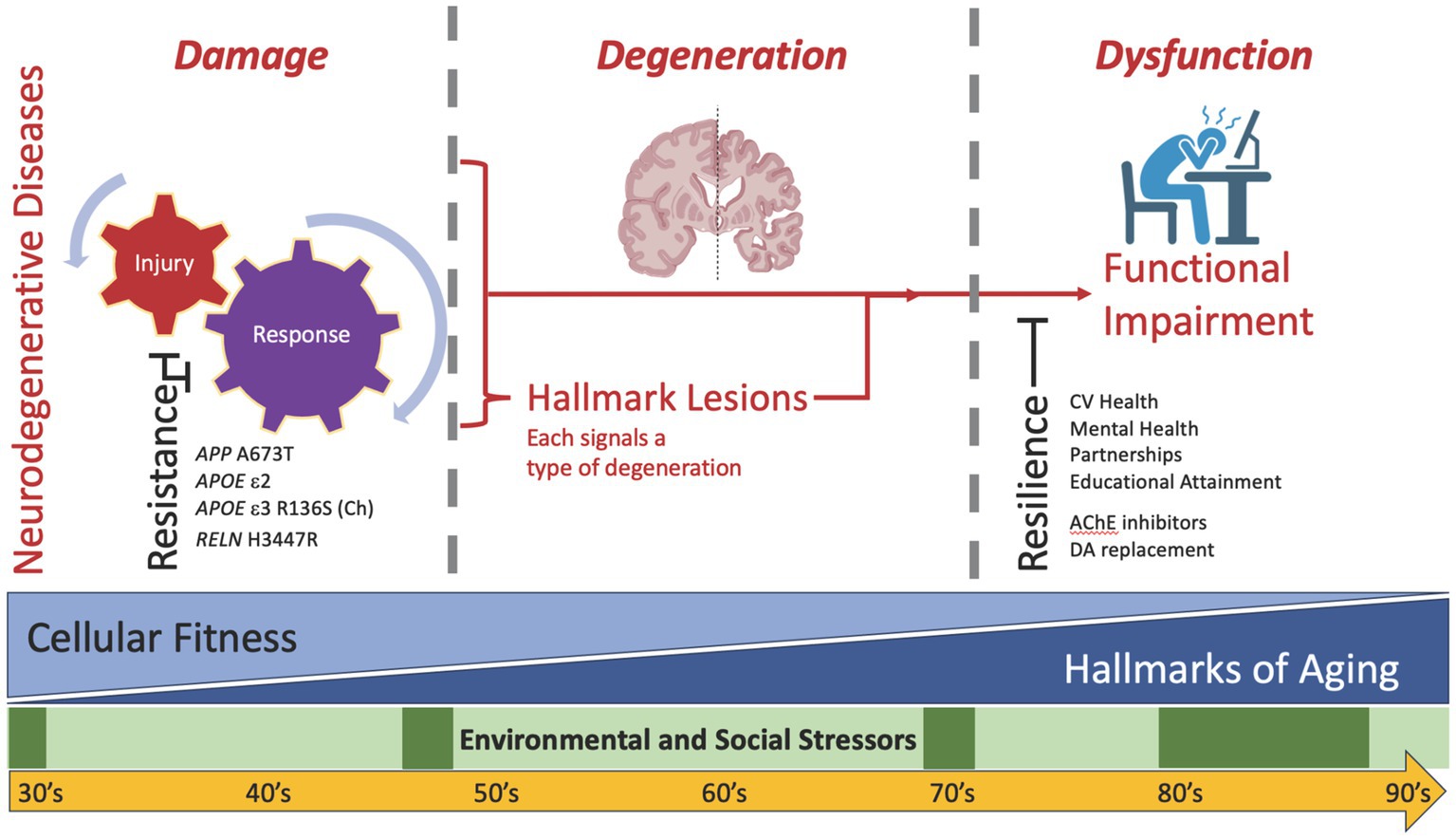
Figure 1. Diagram of the hypothetical relationships among environmental and social stressors, brain aging, and neurodegenerative disease(s). While time passes for all of us, two sources of cellular and molecular stress occur in everyone’s brain to varying extents: episodic environmental and social stressors (layer 1, green) and the biology of aging with attendant reduced fitness for all cell types in brain (layer 2, blue). Biology of aging progressively amplifies with advancing age, although at varying rates across individuals and across organs within an individual. On their own, mounting stressors from these two layers appear insufficient to cause neurodegenerative disease(s). Addition of a third source (layer 3, red)—the neurodegenerative etiologic factors underlying injury plus response to injury—initiates additional cellular and molecular damage, leading to degeneration as signaled by disease-specific formation of hallmark pathologic lesions, including amyloid beta plaques and tau tangles for AD. It is important to recognize that damage may contribute to degeneration through pathways that are dependent or independent of hallmark lesion formation. Sufficiently severe degeneration ultimately expresses as clinically detectable dysfunction. Because this direct model of damage to degeneration to dysfunction is insufficient to account for all observations in humans and experimental animals, two impedance terms are required: resistance and resilience. Resistance signifies suppressed damage despite usually adequate stressors (left), and resilience signifies suppressed functional impairment (right) despite usually adequate degeneration. Examples are provided for some resistance and resilience factors; see text for details.
An influential perspective on the cellular and molecular hallmarks of aging published a decade ago (López-Otín et al., 2013), and recently updated (López-Otín et al., 2023), highlights key features of the biology of aging. Importantly, age—the time lived by an organism—is an imperfect proxy for the underlying biology of aging. Indeed, the cellular and molecular hallmarks of aging accumulate at different rates in different species, in different individuals within a species, and in different organs within an individual. With cancer as the one exception, their net effect is to decrease cellular fitness and thereby promote age-related diseases: this is the second layer of stressors experienced by the aging brain (Figure 1, blue). Importantly, the majority of the hallmarks of aging are shared with the hallmarks of neurodegenerative diseases, including neuropathologic lesions such as amyloid beta plaques and tau tangles (Gómez-Isla and Frosch, 2022; Wilson et al., 2023). Indeed, blinded research neuropathologists would not be expected to distinguish who had dementia from who was cognitively unimpaired purely from histologic analysis of the brain, qualitatively blurring the cellular and molecular features of aging versus neurodegenerative diseases, especially in the oldest old (Montine et al., 2022). Also an active area of investigation, Mathys et al. recently generated a single-cell transcriptomic atlas to assess varying degrees of AD pathology and cognitive impairment and found neuronal (DNA damage) and microglial (genetic variation) differences between aging and neurodegeneration (Mathys et al., 2023).
Because these usual social and environmental influencers and inevitably diminished cellular fitness from aging appears to be insufficient to cause neurodegenerative disease, a third layer of stressors, etiologic determinants of neurodegenerative disease(s) (Wilson et al., 2023), appears to be required before clinical dysfunction becomes apparent (Figure 1, red). In this “direct model,” these three layers of stressors are required to drive enough damage to lead to sufficient degeneration to result in observable dysfunction. To model the real-world situation, a very common experimental paradigm is to create genetically engineered mice that drive a particular type of injury (layer 3) and then age these animals (layer 2) to introduce diminished cellular fitness that together promote degeneration that leads to dysfunction, all while maintaining tight control of the environment (layer 1). Interestingly, many groups have shown the profound impact of environment, reporting that alterations in genetic background or strain of mouse, or even changing diet in the same strain of mouse, can markedly suppress degeneration and its downstream behavioral consequences in these types of experiments despite the continued strong expression of the genetic alteration (Karsten and Geschwind, 2005; Mattson et al., 2017).
Such data from mice challenge the sufficiency of the direct model where damage to degeneration results in dysfunction and require introducing an impedance term for damage, which we refer to as resistance (Figure 1, left), meaning resistance to damage and its downstream consequences of hallmark lesions of degeneration, despite continued application of stressor(s) (Latimer et al., 2017; Montine et al., 2019; Biswas et al., 2023). In mice, one of the best- known examples of resistance is removal of apoE in a mouse model genetically engineered to overexpress human amyloid beta; this was shown 25 years ago to suppress dramatically the accumulation of human amyloid beta plaques (Bales et al., 1997). Resistance factors, although relatively uncommon, have been identified in humans. Continuing with examples from AD, the APP A673T variant suppresses amyloid beta production (Di Fede et al., 2009; Jonsson et al., 2012), while APOE R136S (Christchurch) and RELN H3447R resist downstream pathologic tau accumulation (Arboleda-Velasquez et al., 2019; Lopera et al., 2023).
Similar to resistance, a second impedance term—resilience—is commonly used to describe a mismatch between degeneration resulting from damage and predicted dysfunction (Figure 1, right). Initially observed in community-based cohorts, but now reported in virtually every research cohort that includes older people systematically assessed, somewhere between 10 to 30% of individuals (estimates vary by average age and the definitions used) harbor a high burden of degeneration sufficient to diagnose dementia in others, yet remain cognitively normal or even high cognitive performers (reviewed in Gómez-Isla and Frosch, 2022). These observations reveal another insufficiency in the direct model, at least in humans, requiring the introduction resilience, to describe the gap between neurodegeneration and expected clinical expression of cognitive impairment (Hohman et al., 2016; Montine et al., 2019; Melikyan et al., 2022). The idea of resilience is familiar to the field neurodegenerative disease, with drugs for both Parkinson’s disease (dopamine replacement therapy) and AD (acetylcholinesterase inhibitors) that promote resilience. AD resilience is estimated to be up to 10-times more common than resistance, and resilience to each of the ADRDs also has been reported (Montine et al., 2022; Walker and Richardson, 2023; Willroth et al., 2023). Here we review work our group has done to predict resilience and identify its molecular features.
2 Predicting resilience during life
Recently we defined cognitive resilience (CR) as a continuum instead of the traditional binary (present or absent) categorization, allowing a more comprehensive assessment of resilience and its relationship to other cognitive variables, and overcoming the drawbacks of binary categorization (Phongpreecha et al., 2023b). Indeed, binary categorization of CR captures only the most extreme examples and neglects its likely variable expression by different people and across different cognitive domains. To operationalize CR, we used our previously described (Phongpreecha et al., 2023a) neuropathologic damage estimate derived using a machine learning (ML) approach that combined 17 lesions (including plaques, tangles, LBs, hippocampal sclerosis, and TDP-43) into a single index, along with assessments of cognitive function as determined by neuropsychological tests or clinical assessment. Our model simplifies the relationship into linear equations:
Where cognitive measure is a result of one’s cognitive resilience (CR) minus the damage incurred from diseases such as AD and ADRDs. Thus, CR Score can be solved using quantifiable cognitive measures and damage estimates, as our ML model converts the Damage Estimate into the same unit as cognitive measures, allowing the calculation of CR Scores for different cognitive tests (Phongpreecha et al., 2023b). We conceptualized CR to derive from two components (Eq. 2):
Where reserve represents capacity built up over the lifetime prior to the onset of disease (premorbid) and compensation represents adaptation of existing capacity in response to disease (morbid), although we cannot as yet measure their relative contributions, which occur at different times in life. Reserve accumulates prior to disease onset, such as maximizing bone calcium content in youth, while compensation occurs in response to disease and so follows neuropathologic damage, such as recruitment of additional regions of the brain to subserve memory function. Increased reserve may underlie previous observations that more extensive neuropathology is found in younger AD cases (Morgan, 1992), consistent with protection from clinical manifestation until their greater reserve capacity was depleted.
Although simplistic, these equations provide a framework for the impact of varying resilience on related terms. Visualizing the interactions among these terms as one ages shows different cognitive trajectories in different situations. In Figure 2A, reserve is largely unused up to middle age (Stern, 2002; Stern, 2012). Later, if an individual suffers progressively more damage to the brain from AD and ADRDs, cognitive function will decrease as will CR, a balance between drawing down reserves and, following a short delay, launching compensatory processes (Montine et al., 2019). Figure 2B shows that individuals with higher reserve have a greater premorbid capacity to offset damage and are more likely to preserve cognitive function into older ages, an outcome supported by clinical studies showing higher baseline cognitive function is associated with reserve (Hayden et al., 2019; Beker et al., 2020; Pettigrew et al., 2023). In this scenario, resistance represents a special case where there is none-to-minimal damage (Latimer et al., 2017; Montine et al., 2022) and hence no need to draw on reserve or compensation, (Figure 2A right).
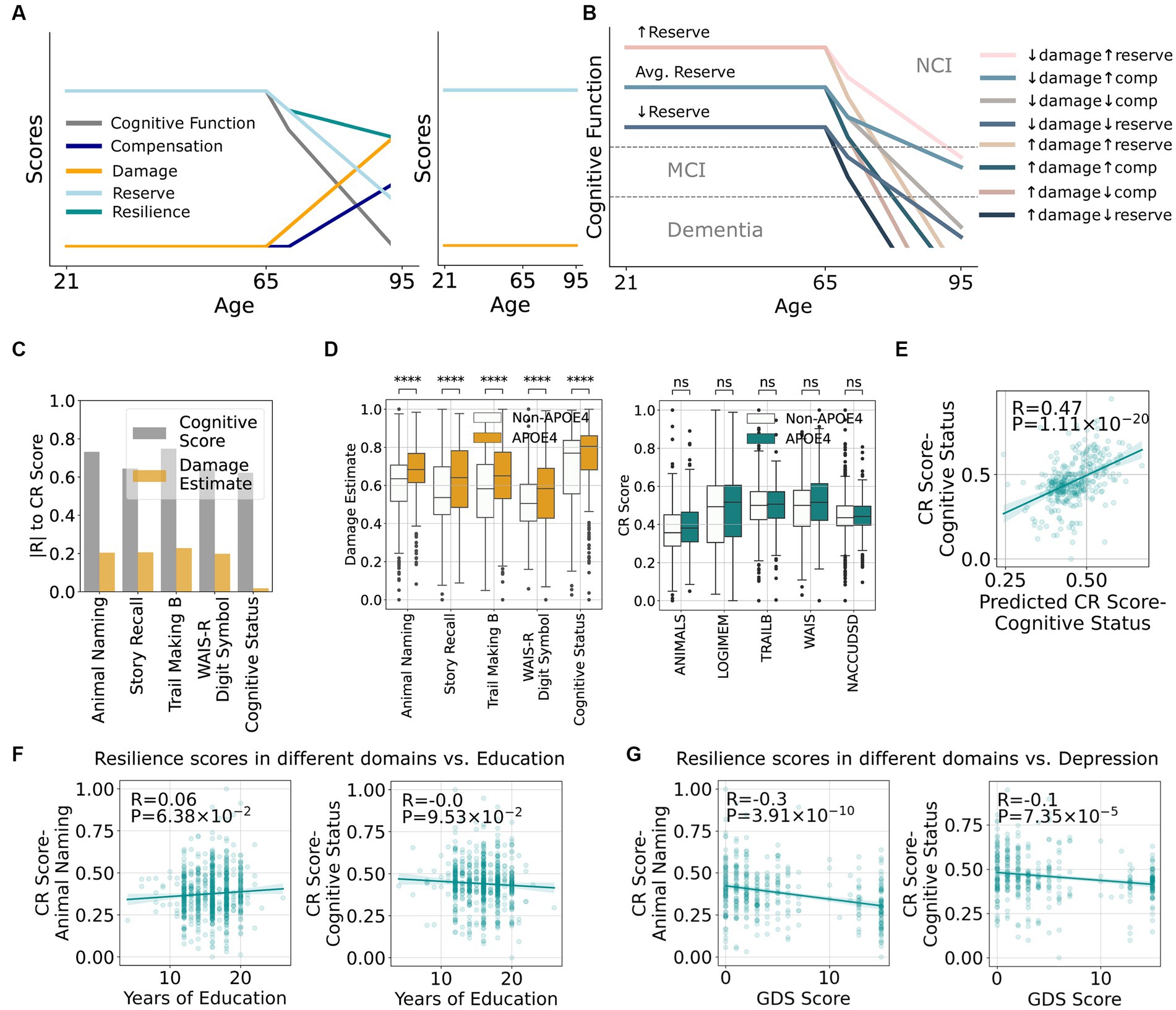
Figure 2. A framework that quantitatively defines resilience and its relationship to other cognitive terms suggest minimal overlap between mechanisms underlying damage and resilience. (A) Hypothetical relationships between increasing damage as an individual ages and how it impacts existing cognitive reserve, resilience, compensation, and cognitive function (left graph). The right graph shows an example of resistance with minimal damage across the lifespan and therefore no reduction in cognitive function. (B) Potential scenarios of cognitive impairment depending on the different quantities of damage, compensation, and reserve. (C) Correlations between estimated damage and cognitive resilience (CR score) from Eq. (1) stratified by cognitive tests. (D) Correlations between presence of APOE ε4 and damage or CR score stratified by cognitive tests. (E) Actual CR scores vs. CR scores predicted by clinical features. (F) Correlation between CR scores from number of story recall or cognitive status with educational attainment. (G) Correlation between CR scores from number of story recall or cognitive status with geriatric depression score (GDS).
While CR Scores show moderate correlations to multiple cognitive measures, including cognitive diagnosis, this is not the case for Damage Estimate (Figure 2C). This implies that the mechanisms underlying damage and resilience may be largely independent, i.e., being resilient does not meaningfully impact the amount of damage. In addition, APOE ε4 is a well-established risk factor for AD and some ADRDs, and Figure 2D confirms that the presence of APOE ε4 leads to a larger Damage Estimate in all cognitive measures. Surprisingly, APOE ε4 does not lead to lower CR Scores as described by previous studies using binary resilience concepts (Legdeur et al., 2018; Snitz et al., 2020; Wang et al., 2022) again suggesting potentially different pathways underlying damage and resilience. These results highlight the value of defining resilience quantitatively and decoupling its effects from damage as assessed by neuropathologic lesions.
With CR defined by clinico-pathological comparison, we next tested the hypothesis that CR Score might be predicted by features during life without using post-mortem data. For example, Figure 2E shows a moderate prediction performance of CR Score using a random forest model based on intra vitam features alone. Educational attainment has been so widely associated with resilience that it is sometimes used as a synonymous measure (Boots et al., 2015; Dekhtyar et al., 2019; Vonk et al., 2019; Fratiglioni et al., 2020; Ossenkoppele et al., 2020). While our study validated that educational attainment is significantly associated with CR Score as measured by some cognitive assessments (Figure 2F), the effect size was small. Other features that were used in the model, such as cardiovascular health and depression scores (Figure 2G), are more strongly correlated with CR Scores. This prediction opens venues for future research to determine genetic, medical, social, and environmental determinants of resilience and develop interventions to enhance resilience.
3 Molecular features of resilience to AD
We have also begun to define the molecular features of categorically-defined examples of resilience to high level AD. Here, we used a relatively small set of 43 highly selected brain donations from sex- and age-matched individuals who met stringent clinico-pathologic criteria for three groups: healthy control (HC), AD dementia (ADD), and resilience to AD (RAD), where all groups were free of common co-morbid pathologic changes. We then applied both unbiased data-independent peptide-based proteomics and bulk ATAQ-seq followed by cell-type deconvolution using a novel application called Cellformer to four regions of brain: superior and middle temporal gyri, inferior parietal lobule, hippocampus, and caudate nucleus.
Our approach to the proteomic work is summarized in Figure 3A and Huang et al. (2023a). The study identified 33 differentially expressed proteins across four brain regions that are associated with RAD (Figure 3B). One of the key findings of this study was the identification of lower levels of soluble amyloid beta (Aβ) in isocortical and hippocampal regions in individuals exhibiting RAD compared to ADD. This suggests that reducing soluble Aβ concentration in tissue, despite high level neuropathologic change, could play a pivotal role in mitigating cognitive impairment.
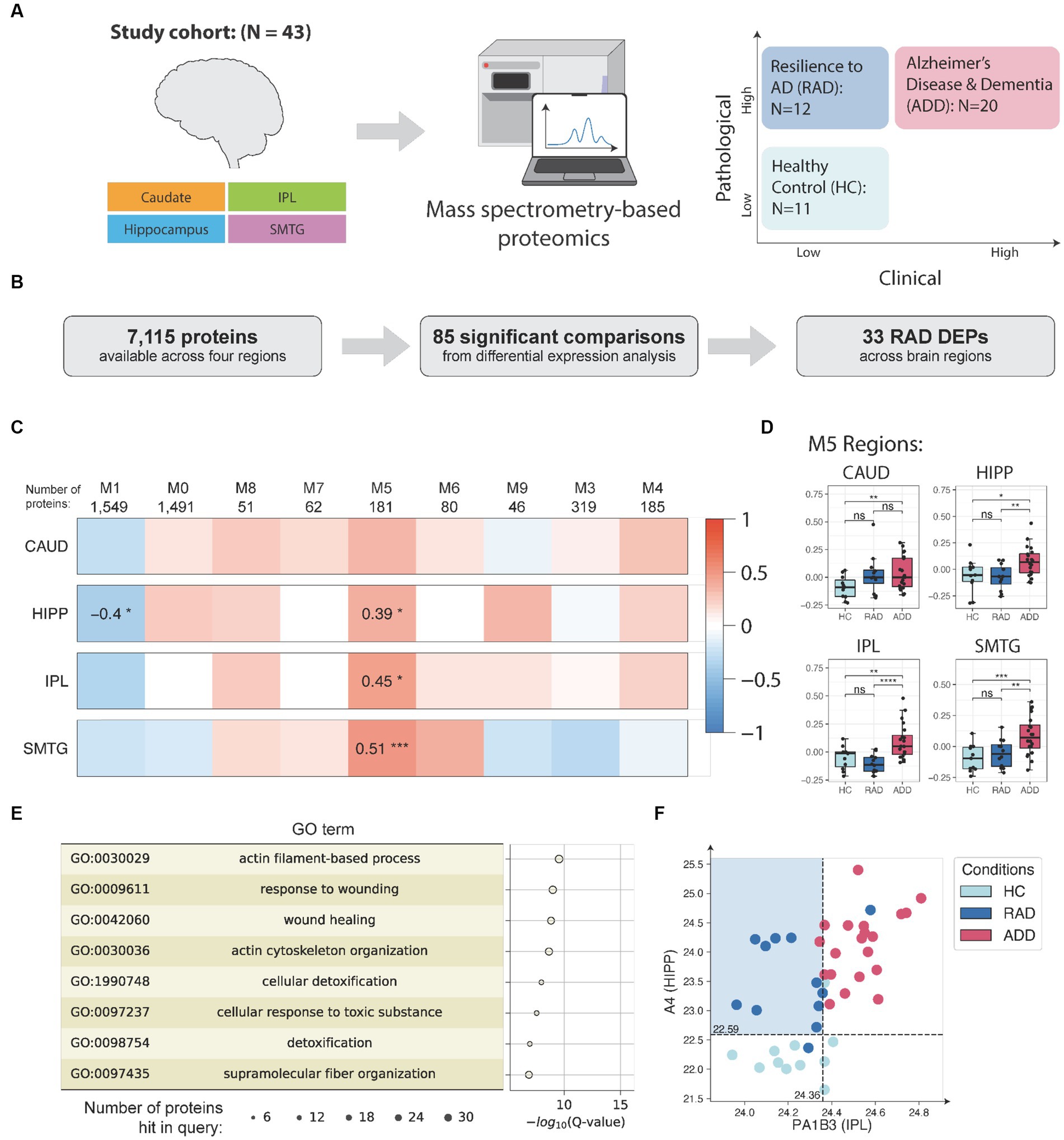
Figure 3. (A) Samples (N = 155) from up to four matched brain regions were donated by 43 research participants who were assigned to three clinico-pathologic groups: healthy control (HC), cognitive resilience to AD (RAD), or AD dementia (ADD). Samples were quantified by data independent tandem mass spectrometry and data analyzed by differential expression and co-expression network analyses. (B) Illustration of differential expression analysis and summary of the final number of RAD-associated differentially expressed proteins (RAD DEPs). (C) Consensus protein co-expression analysis identified 9 modules across four brain regions. Pearson correlation with two-sided p-values was used to evaluate the relationships between clinico-pathologic groups and eigenprotein expression. (D) Module 5 (M5) eigenprotein expressions in HC, RAD, and ADD for the study set. For the boxplots, the interior horizontal line represents the median value, the upper and lower box edges represent the 75th and 25th percentile, and the upper and lower bars represent the 90th and 10th percentiles, respectively. (E) Top enriched GO biological process terms in M5 and their enrichment analysis and the corresponding Q-values (with FDR B&H method) and number of proteins hit in query. (F) Using Aβ abundance in HIPP and PA1B3 concentration in IPL to distinguish RAD from other groups. The number of samples in the study cohort: HC = 11, RES = 12, ADD = 20. CAUD, caudate; HIPP, hippocampus; IPL, inferior parietal lobule; SMTG, superior and middle temporal gyrus; HC, healthy control; RAD, resilience to AD; ADD, AD and dementia; DLPFC, dorsolateral prefrontal cortex; PC, precuneus; GO, gene ontology. *p < 0.05; **p < 0.01; ***p < 0.001; ****p < 0.0001; ns, not significant.
Further analysis with protein co-expression analysis revealed module #5 of 181 densely interacting proteins significantly associated with RAD (Figure 3C). Module #5 contains 14 out of the 33 differentially expressed proteins, including C04A, C04B, CAPG, HSPB1, K2C7, K2C8, CLUS, GFAP, FAAA, PRDX1, PA1B3, CMBL, ICAM1, and IRGQ. Moreover, the module #5 eigenprotein is able to distinguish RAD from ADD across HIPP, IPL, and SMTG regions (Figure 3D). Further gene ontology (GO) analysis suggests that these proteins are enriched for processes related to actin filament-based processes, cellular detoxification, and wound healing (Figure 3E). In addition, a decision tree classifier (Huang et al., 2023b) allows us to identify the top two protein features, Aβ in HIPP and PA1B3 in IPL, that were best at distinguishing the three clinico-pathological groups (Figure 3F). These proteomic findings were validated using data from four independent external cohorts, comprising a total of 689 human isocortical samples. This comprehensive study provides insights into the protein features of RAD.
Leveraging 191 well-curated tissue samples from the same clinico-pathologic groups—Healthy Control (HC, n = 5), RAD (n = 12), and ADD (n = 19) from the same cohort (Berson et al., 2023)—we investigated epigenetic features underpinning RAD in three brain regions SMTG, hippocampus, and caudate (Figure 4A). We used bulk ATAC-seq data coupled with Cellformer, a deep learning algorithm that deconvolutes cell type–specific open chromatin region (OCR) accessibility from bulk data, to provide a comprehensive understanding of heterogeneity in chromatin accessibility across cell populations and cell-specific OCR variation in different brain regions of people with categorically defined RAD.
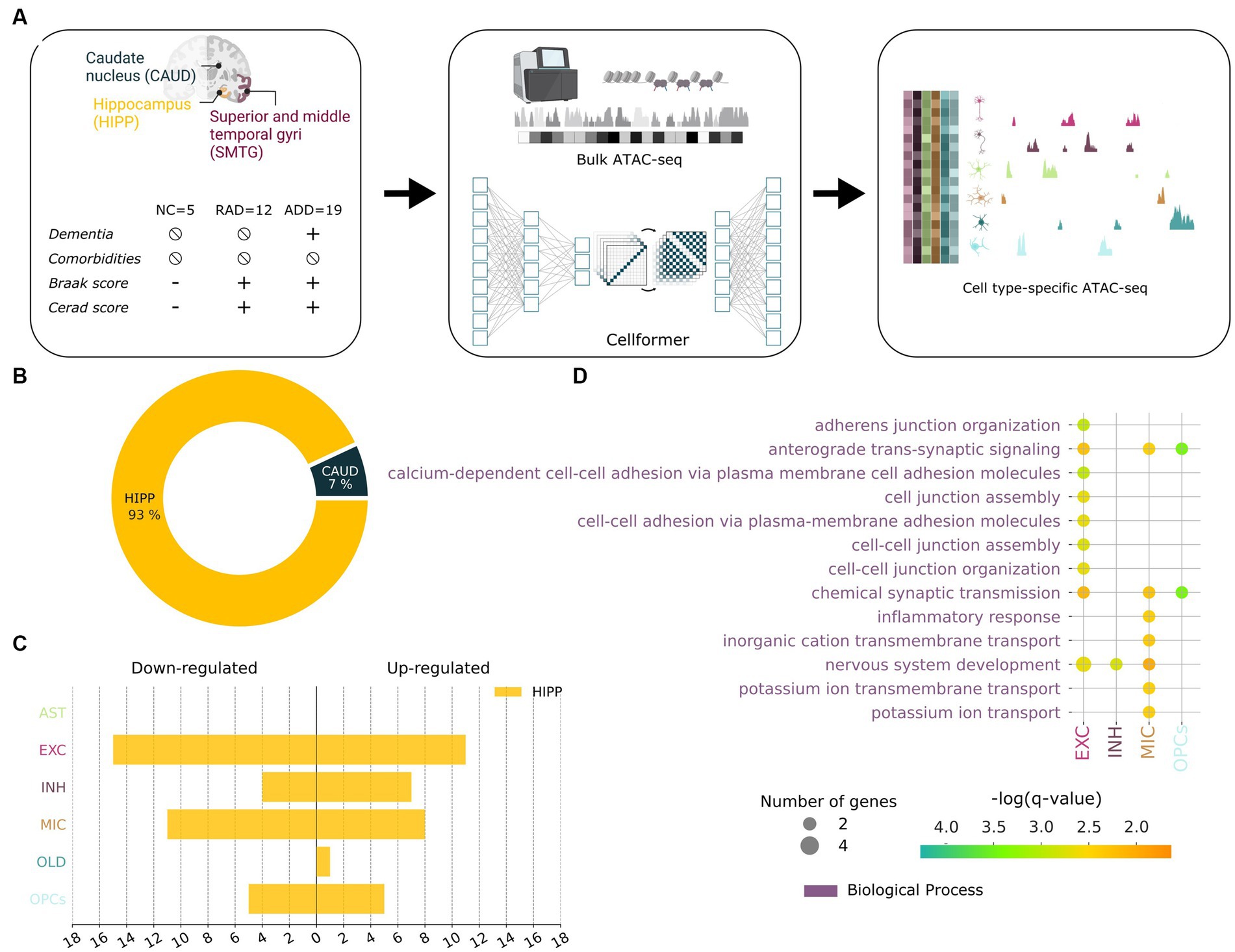
Figure 4. Cellformer deconvolutes epigenetic bulk expression into cell type–specific expression enabling an unprecedented open chromatin profiling of RAD. (A) Cellformer was fed data from comorbidity-free bulk samples from individuals with clinico-pathologic characterization as normal control (NC), Resilient to AD (RAD), and AD dementia (ADD). Three brain regions were used per individual to gain insight into the regional and cellular epigenetic profile of RAD. Cellformer generated cell type–specific expression for 6 main cell types across the whole genome, leading to an unprecedented chromatin profiling of RAD. (B) Cell type–specific open chromatin region (OCR) between RAD and ADD/NC were mainly found in HIPP (93%) and distributed between microglia (28%), and neuron cells (55%) (adjusted p < 0.05, logFC >0.5). (C) Number of OCRs (x-axis) differentially upregulated and downregulated in RAD compared to ADD/NC across cell types (y-axis). (D) GO enrichment applied to RAD-specific OCR (FDR 5%).
Cellformer predicted that OCR differences between RAD and the other two groups that are on the AD continuum are very strongly localized to HIPP, which subserves declarative memory formation and is the primary target of AD (Figure 4B). At the cellular level, most predicted RAD-specific OCR were characterized by changes in both inhibitory and excitatory neurons followed by microglia (Duggan and Parikh, 2021; Figure 4C). From the perspective of cellular processes, GO analysis of RAD-specific OCR highlighted neuronal development, inflammatory response, and synaptic transmission processes (Figure 4D). These pathways were highlighted in previous studies using proteomics and mouse models of AD (Kaczorowski et al., 2011; Arnold et al., 2013; Seto et al., 2021; Neuner et al., 2022). Overall these highly plausible predictions suggest that individuals with RAD are distinguished from the AD continuum by epigenetic upregulation in support of hippocampal neuronal processes and synapses. This difference in the regulome might confer RAD the ability to preserve the number of neuronal projections and synapses that has been observed through histopathological studies (Perez-Nievas et al., 2013).
4 Discussion
Episodic social and environmental stressors combine with the progressively amplifying biology of aging to reduce cellular fitness in brain as we age. Although on their own, these two stressors of the aging brain are insufficient to cause neurodegenerative disease, the cellular and molecular distinction between brain aging and neurodegenerative disease begins to blur in the oldest old. Usually, a third stressor, the etiologic factors that drive damage from neurodegenerative disease(s), conspires with social/environmental influences and processes of aging to yield cellular and molecular damage that leads to degeneration with hallmark pathologic lesions that lead to neurological dysfunction. Approximately 15 to 25% of observations in humans do not fit this hypothetical direct model of damage to degeneration to dysfunction, requiring the introduction of two impedance terms: resistance, which suppress damage despite usually adequate stressors, and the more common resilience, which suppress neurological dysfunction despite usually adequate degeneration. Here, we reviewed recent work to determine factors during life that predict the clinico-pathologic state of resilience and showed that cardiovascular health and mental health, more so than educational attainment, are predictive of a continuous measure of resilience to AD and ADRDs. We next focused on molecular features of RAD—resilience to AD alone—and observed that synaptic and axonal processes, especially in the hippocampus, are core features of RAD. Future work may focus on larger and more diverse cohorts that include ADRDs and explore additional regions of brain for their contribution to this counterforce to neurodegeneration.
Author contributions
KM: Project administration, Writing – original draft, Writing – review & editing. EB: Visualization, Writing – original draft, Writing – review & editing. TP: Visualization, Writing – original draft, Writing – review & editing. ZH: Visualization, Writing – original draft, Writing – review & editing. NA: Funding acquisition, Resources, Supervision, Writing – review & editing. JZ: Funding acquisition, Resources, Supervision, Writing – review & editing. MM: Funding acquisition, Resources, Writing – review & editing. TM: Conceptualization, Funding acquisition, Supervision, Writing – original draft, Writing – review & editing.
Funding
The author(s) declare financial support was received for the research, authorship, and/or publication of this article. This work was supported by NIH grants AG053959 (MM and TM), AG065156 (MM and TM), AG072573 (TM), AG077443 (NA and TM), and GM138353 (NA).
Conflict of interest
The authors declare that the research was conducted in the absence of any commercial or financial relationships that could be construed as a potential conflict of interest.
Publisher’s note
All claims expressed in this article are solely those of the authors and do not necessarily represent those of their affiliated organizations, or those of the publisher, the editors and the reviewers. Any product that may be evaluated in this article, or claim that may be made by its manufacturer, is not guaranteed or endorsed by the publisher.
References
Arboleda-Velasquez, J. F., Lopera, F., O’Hare, M., Delgado-Tirado, S., Marino, C., Chmielewska, N., et al. (2019). Resistance to autosomal dominant Alzheimer’s disease in an APOE3 Christchurch homozygote: a case report. Nat. Med. 25, 1680–1683. doi: 10.1038/s41591-019-0611-3
Arnold, S. E., Louneva, N., Cao, K., Wang, L. S., Han, L. Y., Wolk, D. A., et al. (2013). Cellular, synaptic, and biochemical features of resilient cognition in Alzheimer’s disease. Neurobiol. Aging 34, 157–168. doi: 10.1016/j.neurobiolaging.2012.03.004
Bales, K. R., Verina, T., Dodel, R. C., Du, Y., Altstiel, L., Bender, M., et al. (1997). Lack of apolipoprotein E dramatically reduces amyloid beta-peptide deposition. Nat. Genet. 17, 263–264. doi: 10.1038/ng1197-263
Beker, N., Sikkes, S. A. M., Hulsman, M., Tesi, N., van der Lee, S. J., Scheltens, P., et al. (2020). Longitudinal maintenance of cognitive health in centenarians in the 100-plus study. JAMA Netw. Open 3:e200094. doi: 10.1001/jamanetworkopen.2020.0094
Berson, E., Sreenivas, A., Phongpreecha, T., Perna, A., Grandi, F. C., Xue, L., et al. (2023). Whole genome deconvolution unveils Alzheimer’s resilient epigenetic signature. Nat. Commun. 14:4947. doi: 10.1038/s41467-023-40611-4
Besser, L. (2021). Outdoor green space exposure and brain health measures related to Alzheimer’s disease: a rapid review. BMJ Open 11:e043456. doi: 10.1136/bmjopen-2020-043456
Biswas, R., Kawas, C., Montine, T. J., Bukhari, S. A., Jiang, L., and Corrada, M. M. (2023). Superior global cognition in oldest-old is associated with resistance to neurodegenerative pathologies: results from the 90+ study. J. Alzheimers Dis. 93, 561–575. doi: 10.3233/jad-221062
Boots, E. A., Schultz, S. A., Almeida, R. P., Oh, J. M., Koscik, R. L., Dowling, M. N., et al. (2015). Occupational complexity and cognitive reserve in a middle-aged cohort at risk for Alzheimer’s disease. Arch. Clin. Neuropsychol. 30, 634–642. doi: 10.1093/arclin/acv041
Boyle, P. A., Wang, T., Yu, L., Wilson, R. S., Dawe, R., Arfanakis, K., et al. (2021). To what degree is late life cognitive decline driven by age-related neuropathologies? Brain 144, 2166–2175. doi: 10.1093/brain/awab092
Chen, X., Lee, C., and Huang, H. (2022). Neighborhood built environment associated with cognition and dementia risk among older adults: a systematic literature review. Soc. Sci. Med. 292:114560. doi: 10.1016/j.socscimed.2021.114560
Dekhtyar, S., Marseglia, A., Xu, W., Darin-Mattsson, A., Wang, H. X., and Fratiglioni, L. (2019). Genetic risk of dementia mitigated by cognitive reserve: a cohort study. Ann. Neurol. 86, 68–78. doi: 10.1002/ana.25501
Di Fede, G., Catania, M., Morbin, M., Rossi, G., Suardi, S., Mazzoleni, G., et al. (2009). A recessive mutation in the APP gene with dominant-negative effect on amyloidogenesis. Science 323, 1473–1477. doi: 10.1126/science.1168979
Duggan, M. R., and Parikh, V. (2021). Microglia and modifiable life factors: potential contributions to cognitive resilience in aging. Behav. Brain Res. 405:113207. doi: 10.1016/j.bbr.2021.113207
Fratiglioni, L., Marseglia, A., and Dekhtyar, S. (2020). Ageing without dementia: can stimulating psychosocial and lifestyle experiences make a difference? Lancet Neurol. 19, 533–543. doi: 10.1016/s1474-4422(20)30039-9
Gómez-Isla, T., and Frosch, M. P. (2022). Lesions without symptoms: understanding resilience to Alzheimer disease neuropathological changes. Nat. Rev. Neurol. 18, 323–332. doi: 10.1038/s41582-022-00642-9
Hayden, K. M., Gaussoin, S. A., Hunter, J. C., Manson, J. E., Sachs, B. C., Shadyab, A. H., et al. (2019). Cognitive resilience among APOE ε4 carriers in the oldest old. Int. J. Geriatr. Psychiatry 34, 1833–1844. doi: 10.1002/gps.5199
Hohman, T. J., McLaren, D. G., Mormino, E. C., Gifford, K. A., Libon, D. J., and Jefferson, A. L. (2016). Asymptomatic Alzheimer disease: defining resilience. Neurology 87, 2443–2450. doi: 10.1212/wnl.0000000000003397
Huang, Z., Merrihew, G. E., Larson, E. B., Park, J., Plubell, D., Fox, E. J., et al. (2023b). Unveiling resilience to Alzheimer’s disease: insights from brain regional proteomic markers. Neuroscience Insights 18:26331055231201600. doi: 10.1177/26331055231201600
Huang, Z., Merrihew, G. E., Larson, E. B., Park, J., Plubell, D., Fox, E. J., et al. (2023a). Brain proteomic analysis implicates actin filament processes and injury response in resilience to Alzheimer’s disease. Nat. Commun. 14:2747. doi: 10.1038/s41467-023-38376-x
Hunt, J. F. V., Buckingham, W., Kim, A. J., Oh, J., Vogt, N. M., Jonaitis, E. M., et al. (2020). Association of neighborhood-level disadvantage with cerebral and hippocampal volume. JAMA Neurol. 77, 451–460. doi: 10.1001/jamaneurol.2019.4501
Jonsson, T., Atwal, J. K., Steinberg, S., Snaedal, J., Jonsson, P. V., Bjornsson, S., et al. (2012). A mutation in APP protects against Alzheimer’s disease and age-related cognitive decline. Nature 488, 96–99. doi: 10.1038/nature11283
Kaczorowski, C. C., Sametsky, E., Shah, S., Vassar, R., and Disterhoft, J. F. (2011). Mechanisms underlying basal and learning-related intrinsic excitability in a mouse model of Alzheimer’s disease. Neurobiol. Aging 32, 1452–1465. doi: 10.1016/j.neurobiolaging.2009.09.003
Karsten, S. L., and Geschwind, D. H. (2005). Exercise your amyloid. Cells 120, 572–574. doi: 10.1016/j.cell.2005.02.027
Latimer, C. S., Keene, C. D., Flanagan, M. E., Hemmy, L. S., Lim, K. O., White, L. R., et al. (2017). Resistance to Alzheimer disease neuropathologic changes and apparent cognitive resilience in the Nun and Honolulu-Asia aging studies. J. Neuropathol. Exp. Neurol. 76, 458–466. doi: 10.1093/jnen/nlx030
Legdeur, N., Badissi, M., Carter, S. F., de Crom, S., van de Kreeke, A., Vreeswijk, R., et al. (2018). Resilience to cognitive impairment in the oldest-old: design of the EMIF-AD 90+ study. BMC Geriatr. 18:289. doi: 10.1186/s12877-018-0984-z
Livingston, G., Huntley, J., Sommerlad, A., Ames, D., Ballard, C., Banerjee, S., et al. (2020). Dementia prevention, intervention, and care: 2020 report of the lancet commission. Lancet 396, 413–446. doi: 10.1016/s0140-6736(20)30367-6
Lopera, F., Marino, C., Chandrahas, A. S., O’Hare, M., Villalba-Moreno, N. D., Aguillon, D., et al. (2023). Resilience to autosomal dominant Alzheimer’s disease in a Reelin-COLBOS heterozygous man. Nat. Med. 29, 1243–1252. doi: 10.1038/s41591-023-02318-3
López-Otín, C., Blasco, M. A., Partridge, L., Serrano, M., and Kroemer, G. (2013). The hallmarks of aging. Cells 153, 1194–1217. doi: 10.1016/j.cell.2013.05.039
López-Otín, C., Blasco, M. A., Partridge, L., Serrano, M., and Kroemer, G. (2023). Hallmarks of aging: An expanding universe. Cells 186, 243–278. doi: 10.1016/j.cell.2022.11.001
Mathys, H., Peng, Z., Boix, C. A., Victor, M. B., Leary, N., Babu, S., et al. (2023). Single-cell atlas reveals correlates of high 529 cognitive function, dementia, and resilience to Alzheimer’s disease pathology. Cells 186, 4365–4385.e27. doi: 10.1016/j.cell.2023.08.039
Mattson, M. P., Longo, V. D., and Harvie, M. (2017). Impact of intermittent fasting on health and disease processes. Ageing Res. Rev. 39, 46–58. doi: 10.1016/j.arr.2016.10.005
Melikyan, Z. A., Corrada, M. M., Leiby, A. M., Sajjadi, S. A., Bukhari, S., Montine, T. J., et al. (2022). Cognitive resilience to three dementia-related neuropathologies in an oldest-old man: a case report from the 90+ study. Neurobiol. Aging 116, 12–15. doi: 10.1016/j.neurobiolaging.2022.03.009
Montine, T. J., Cholerton, B. A., Corrada, M. M., Edland, S. D., Flanagan, M. E., Hemmy, L. S., et al. (2019). Concepts for brain aging: resistance, resilience, reserve, and compensation. Alzheimers Res. Ther. 11:22. doi: 10.1186/s13195-019-0479-y
Montine, T. J., Corrada, M. M., Kawas, C., Bukhari, S., White, L., Tian, L., et al. (2022). Association of cognition and dementia with neuropathologic changes of Alzheimer disease and other conditions in the oldest-old. Neurology 99, e1067–e1078. doi: 10.1212/wnl.0000000000200832
Morgan, D. G. (1992). “Neurochemical changes with aging: predisposition toward age-related mental disorders” in Handbook of Mental Health and Aging. eds. J. E. Birren, R. B. Sloane, and G. D. Cohen. 2nd ed cambridge, ma (Academic Press), 175–199.
Neuner, S. M., Telpoukhovskaia, M., Menon, V., O’Connell, K. M. S., Hohman, T. J., and Kaczorowski, C. C. (2022). Translational approaches to understanding resilience to Alzheimer’s disease. Trends Neurosci. 45, 369–383. doi: 10.1016/j.tins.2022.02.005
Ossenkoppele, R., Lyoo, C. H., Jester-Broms, J., Sudre, C. H., Cho, H., Ryu, Y. H., et al. (2020). Assessment of demographic, genetic, and imaging variables associated with brain resilience and cognitive resilience to pathological tau in patients with Alzheimer disease. JAMA Neurol. 77, 632–642. doi: 10.1001/jamaneurol.2019.5154
Perez-Nievas, B. G., Stein, T. D., Tai, H. C., Dols-Icardo, O., Scotton, T. C., Barroeta-Espar, I., et al. (2013). Dissecting phenotypic traits linked to human resilience to Alzheimer’s pathology. Brain 136, 2510–2526. doi: 10.1093/brain/awt171
Peterson, R. L., George, K. M., Tran, D., Malladi, P., Gilsanz, P., Kind, A. J. H., et al. (2021). Operationalizing social environments in cognitive aging and dementia research: a scoping review. Int. J. Environ. Res. Public Health 18:7166. doi: 10.3390/ijerph18137166
Pettigrew, C., Nazarovs, J., Soldan, A., Singh, V., Wang, J., Hohman, T., et al. (2023). Alzheimer’s disease genetic risk and cognitive reserve in relationship to long-term cognitive trajectories among cognitively normal individuals. Alzheimers Res. Ther. 15:66. doi: 10.1186/s13195-023-01206-9
Phongpreecha, T., Cholerton, B., Bukhari, S., Chang, A. L., De Francesco, D., Thuraiappah, M., et al. (2023a). Prediction of neuropathologic lesions from clinical data. Alzheimers Dement. 19, 3005–3018. doi: 10.1002/alz.12921
Phongpreecha, T., Godrich, D., Berson, E., Espinosa, C., Kim, Y., Cholerton, B., et al. (2023b). Quantitative estimate of cognitive resilience and its medical and genetic associations. Alzheimers Res. Ther. 15:192. doi: 10.1186/s13195-023-01329-z
Seto, M., Weiner, R. L., Dumitrescu, L., and Hohman, T. J. (2021). Protective genes and pathways in Alzheimer’s disease: moving towards precision interventions. Mol. Neurodegener. 16:29. doi: 10.1186/s13024-021-00452-5
Snitz, B. E., Chang, Y., Tudorascu, D. L., Lopez, O. L., Lopresti, B. J., DeKosky, S. T., et al. (2020). Predicting resistance to amyloid-beta deposition and cognitive resilience in the oldest-old. Neurology 95, e984–e994. doi: 10.1212/wnl.0000000000010239
Stern, Y. (2002). What is cognitive reserve? Theory and research application of the reserve concept. J. Int. Neuropsychol. Soc. 8, 448–460. doi: 10.1017/S1355617702813248
Stern, Y. (2012). Cognitive reserve in ageing and Alzheimer’s disease. Lancet Neurol. 11, 1006–1012. doi: 10.1016/s1474-4422(12)70191-6
Vonk, J. M. J., Arce Rentería, M., Medina, V. M., Pericak-Vance, M. A., Byrd, G. S., Haines, J., et al. (2019). Education moderates the relation between APOE ɛ4 and memory in nondemented non-Hispanic black older adults. J. Alzheimers Dis. 72, 495–506. doi: 10.3233/jad-190415
Walker, J. M., and Richardson, T. E. (2023). Cognitive resistance to and resilience against multiple comorbid neurodegenerative pathologies and the impact of APOE status. J. Neuropathol. Exp. Neurol. 82, 110–119. doi: 10.1093/jnen/nlac115
Wang, T., Huynh, K., Giles, C., Mellett, N. A., Duong, T., Nguyen, A., et al. (2022). APOE ε2 resilience for Alzheimer’s disease is mediated by plasma lipid species: analysis of three independent cohort studies. Alzheimers Dement. 18, 2151–2166. doi: 10.1002/alz.12538
Willroth, E. C., James, B. D., Graham, E. K., Kapasi, A., Bennett, D. A., and Mroczek, D. K. (2023). Well-being and cognitive resilience to dementia-related neuropathology. Psychol. Sci. 34, 283–297. doi: 10.1177/09567976221119828
Wilson, D. M. 3rd, Cookson, M. R., Van Den Bosch, L., Zetterberg, H., Holtzman, D. M., and Dewachter, I. (2023). Hallmarks of neurodegenerative diseases. Cells 186, 693–714. doi: 10.1016/j.cell.2022.12.032
Wu-Chung, E. L., Leal, S. L., Denny, B. T., Cheng, S. L., and Fagundes, C. P. (2022). Spousal caregiving, widowhood, and cognition: a systematic review and a biopsychosocial framework for understanding the relationship between interpersonal losses and dementia risk in older adulthood. Neurosci. Biobehav. Rev. 134:104487. doi: 10.1016/j.neubiorev.2021.12.010
Keywords: aging, cognition, computational models, dementia, proteomic analysis, neuropathologic lesion, machine learning
Citation: Montine KS, Berson E, Phongpreecha T, Huang Z, Aghaeepour N, Zou JY, MacCoss MJ and Montine TJ (2023) Understanding the molecular basis of resilience to Alzheimer’s disease. Front. Neurosci. 17:1311157. doi: 10.3389/fnins.2023.1311157
Edited by:
David Cook, University of Washington, United StatesReviewed by:
Marina Avila Villanueva, Procesos Cognitivos y Logopedia UCM, SpainDavid Morgan, Michigan State University, United States
Copyright © 2023 Montine, Berson, Phongpreecha, Huang, Aghaeepour, Zou, MacCoss and Montine. This is an open-access article distributed under the terms of the Creative Commons Attribution License (CC BY). The use, distribution or reproduction in other forums is permitted, provided the original author(s) and the copyright owner(s) are credited and that the original publication in this journal is cited, in accordance with accepted academic practice. No use, distribution or reproduction is permitted which does not comply with these terms.
*Correspondence: Thomas J. Montine, dG1vbnRpbmVAc3RhbmZvcmQuZWR1