- 1Department of Neuronal Surgery, The Second Affiliated Hospital of Fujian Medical University, Quanzhou, Fujian, China
- 2Department of Anesthesiology, The Second Affiliated Hospital of Fujian Medical University, Quanzhou, China
- 3Department of Neurosurgery, Fuzong Clinical Medical College, Fuzhou, Fujian, China
- 4Centre of Neurological and Metabolic Research, The Second Affiliated Hospital of Fujian Medical University, Quanzhou, Fujian, China
- 5Department of Endocrinology, The Second Affiliated Hospital of Fujian Medical University, Quanzhou, Fujian, China
Background and objective: Predicting mortality from traumatic brain injury facilitates early data-driven treatment decisions. Machine learning has predicted mortality from traumatic brain injury in a growing number of studies, and the aim of this study was to conduct a meta-analysis of machine learning models in predicting mortality from traumatic brain injury.
Methods: This systematic review and meta-analysis included searches of PubMed, Web of Science and Embase from inception to June 2023, supplemented by manual searches of study references and review articles. Data were analyzed using Stata 16.0 software. This study is registered with PROSPERO (CRD2023440875).
Results: A total of 14 studies were included. The studies showed significant differences in the overall sample, model type and model validation. Predictive models performed well with a pooled AUC of 0.90 (95% CI: 0.87 to 0.92).
Conclusion: Overall, this study highlights the excellent predictive capabilities of machine learning models in determining mortality following traumatic brain injury. However, it is important to note that the optimal machine learning modeling approach has not yet been identified.
Systematic review registration: https://www.crd.york.ac.uk/PROSPERO/display_record.php?RecordID=440875, identifier CRD2023440875.
1 Introduction
Traumatic brain injury (TBI) has a high rate of disability and mortality and is one of the leading causes of death worldwide (Capizzi et al., 2020). Predicting mortality from TBI is essential for making informed clinical decisions and providing guidance to patients’ families. Traditional statistical methods have been commonly used for this purpose. However, in recent years, there has been a surge in research using machine learning (ML) to predict mortality from TBI.
ML algorithms can autonomously learn from data, generate patterns, and use these patterns to predict unknown outcomes. As a result, various ML-based models for predicting mortality in TBI have emerged (Moyer et al., 2022; Bischof and Cross, 2023; Wu et al., 2023). However, the predictive performance of these models varies across multiple studies due to factors such as the inclusion of different sample data and the use of different types of ML models. In this context, we conducted a meta-analysis to evaluate the effectiveness of ML in predicting TBI mortality and better characterize the overall performance of these models.
2 Methods
Our study was registered with PROSPERO (CRD2023440875) and was conducted in accordance with the guidelines provided by the Preferred Reporting Items for Systematic Reviews and Meta-Analyses (PRISMA) and PRISMA-2020 (Page et al., 2021). The review was based on a systematic search and predefined inclusion and exclusion criteria. Meta-analyses were carried out according to a predetermined analysis plan.
2.1 Search strategy
Systematic literature searches using PubMed, Web of Science, and Embase followed PRISMA guidelines (from inception to May 2023). Our search strategy uses medical topic headlines and natural language text terms, and search formulas are provided in the Supplementary Table.
2.2 Selection process
This meta-analysis excluded non-English studies and non-original studies. Studies involving pediatric populations, animals, all enrolled patients who received a specific treatment, or all enrolled patients who developed a specific TBI complication were also excluded. Additionally, studies that did not use machine learning for prediction were excluded; these studies focused primarily on assessing risk factors rather than predicting prognosis and lacked sufficient data to infer the performance of the machine learning models. In terms of outcomes, studies that predicted mortality for more than 6 months were excluded. Two authors (WZ and LJQ) independently screened each search record and removed duplicate studies using Endnote X9. Full-text assessment was performed if it was challenging to determine eligibility based on title and abstract alone.
2.3 Data extraction
Evaluation of the model’s performance focused on its ability to accurately discriminate between in-hospital mortality or mortality within 6 months of TBI. Two authors (WZ and LJQ) independently extracted data using the Checklist for critical Appraisal and data extraction for systematic Reviews of prediction Modeling Studies (CHARMS) checklist. In cases of disagreement, a third party assisted in the adjudication or facilitated the process of reaching consensus.
2.4 Risk of bias assessment
The quality and applicability of the included studies were assessed using the Predictive Model Risk of Bias Assessment Tool (PROBAST) (Wolff et al., 2019). Two review authors (WZ and LJQ) independently evaluated the studies based on four domains: participants, predictors, outcomes, and analysis.
2.5 Statistical analysis
Data were synthesized and analyzed using Stata 14.0 (Stata Corporation, College Station, TX, USA) software. Sensitivity and specificity were measured using the corresponding 95% confidence intervals (CIs). Additionally, a summary ROC (sROC) curve with a 95% CI was generated using a hierarchical summary receiver operating characteristic (HSROC) model to assess the collective discriminatory performance of published post-TBI mortality prediction models (Reitsma et al., 2005). A p-value of < 0.05 was considered statistically significant. To quantify statistical heterogeneity between studies, I2 and Cochran Q statistics were utilized. Furthermore, meta-regression and subgroup analyses were carried out to explore potential sources of heterogeneity among studies (Ioannidis, 2008).
3 Results
The search strategy yielded a total of 618 articles from three databases: PubMed, Embase, and Web of Science (Figure 1). Initially, 196 duplicate articles were removed. Based on the evaluation of titles and abstracts, 293 irrelevant studies were excluded. Subsequently, 63 conference articles and articles lacking full text were excluded. Finally, 52 studies were excluded following a full-text assessment. Ultimately, 14 studies met the eligibility criteria and were included in this review.
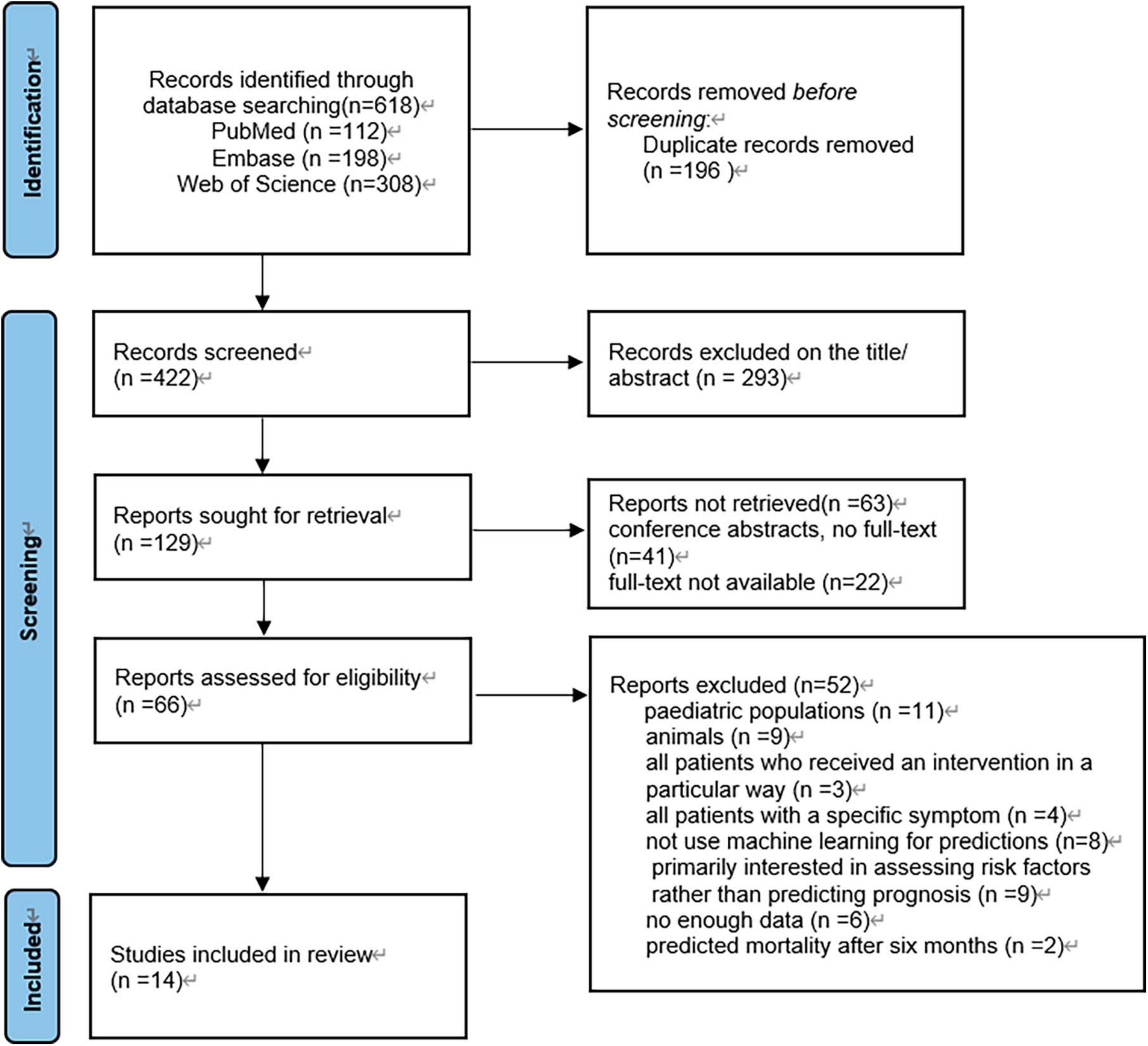
Figure 1. Article selection flow diagram. PRISMA (preferred reporting items for systematic reviews and meta-analyses) flow diagram for study selection.
3.1 Description of included studies
3.1.1 Study characteristics
The earliest included studies were published in 1997, while the majority of studies were published between 2020 and 2023. These studies were conducted in different countries on different continents, with nine studies from Asia (Rau et al., 2018; Abujaber et al., 2020; Matsuo et al., 2020, 2023; Lee et al., 2022; Tu et al., 2022; Wang et al., 2022; Song et al., 2023; Wu et al., 2023), four from North America (Lang et al., 1997; Pease et al., 2022; Satyadev et al., 2022; Warman et al., 2022), two from Europe (Güiza et al., 2013; Wu et al., 2023), and one from Africa (Warman et al., 2022). Of the included studies, eight were retrospective (Güiza et al., 2013; Abujaber et al., 2020; Matsuo et al., 2020, 2023; Lee et al., 2022; Pease et al., 2022; Satyadev et al., 2022; Song et al., 2023), three were prospective (Lang et al., 1997; Warman et al., 2022; Wu et al., 2023), and three did not provide a clear description (Rau et al., 2018; Satyadev et al., 2022; Wang et al., 2022). Six studies did not specify criteria for inclusion or exclusion of patient data (Güiza et al., 2013; Rau et al., 2018; Satyadev et al., 2022; Warman et al., 2022; Wu et al., 2023), all of which included more than 200 cases. Eight of these studies exceeded 1,000 cases (Lang et al., 1997; Rau et al., 2018; Abujaber et al., 2020; Satyadev et al., 2022; Tu et al., 2022; Warman et al., 2022; Matsuo et al., 2023; Song et al., 2023; Wu et al., 2023), nine focused on in-hospital mortality outcomes (Rau et al., 2018; Abujaber et al., 2020; Matsuo et al., 2020, 2023; Tu et al., 2022; Wang et al., 2022; Warman et al., 2022; Song et al., 2023; Wu et al., 2023), four on 6-month mortality outcomes (Lang et al., 1997; Güiza et al., 2013; Pease et al., 2022; Satyadev et al., 2022), and one on 14-day mortality outcomes (Supplementary Table 1; Lee et al., 2022).
3.1.2 Types of machine learning
In the included studies, except for Wu et al. (2023), the authors used two or more different machine learning methods to construct multiple predictive models within the same study. These models were then compared to determine the best performing machine learning algorithm. Figure 2 provides an overview of the machine learning algorithms used, with a total of 18 algorithms from 14 studies included studies.
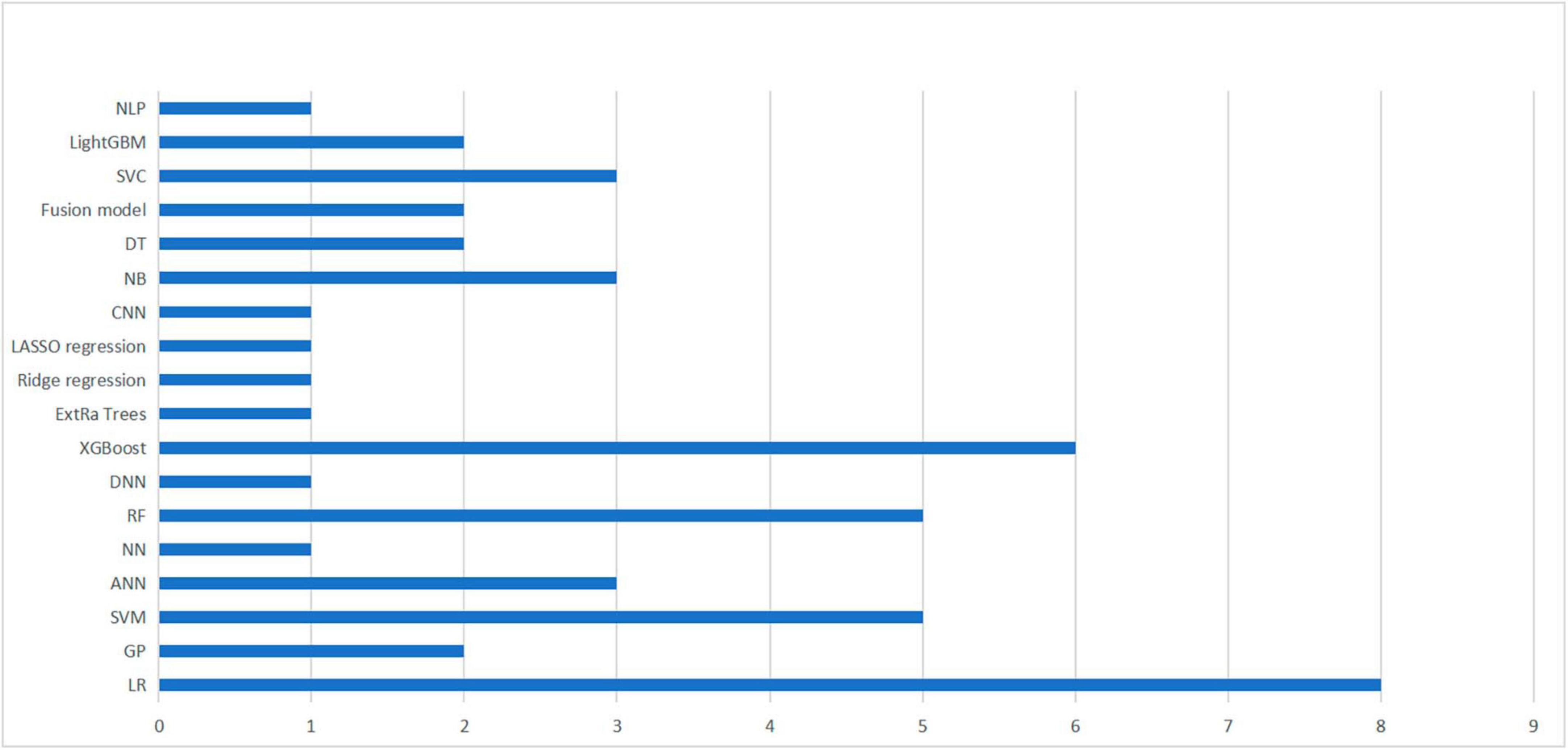
Figure 2. Summary of machine learning methods used in 14 studies. A summary of machine learning methods used to build TBI mortality prediction models.
Nonetheless, logistic regression remained the most commonly utilized method, performing best in two studies (Lang et al., 1997; Tu et al., 2022). Additionally, four studies identified XGBoost as the optimal algorithm for constructing prediction models (Wang et al., 2022; Warman et al., 2022; Matsuo et al., 2023; Wu et al., 2023), followed by SVM (Abujaber et al., 2020; Lee et al., 2022), and RF (Matsuo et al., 2020; Satyadev et al., 2022), respectively, which were considered to be the best performing models in both studies. It is worth noting that the selection of an appropriate machine learning algorithm does not completely determine the performance of the model, as it may also be influenced by the included predictors, the choice of hyperparameters and various other factors (Greener et al., 2022).
3.1.3 Model performance and validation
Performance metrics, including accuracy, sensitivity, specificity, AUC, and F1 score, were used to assess and characterize the performance of the model. Supplementary Table 2 provides detailed information about the AUC values, ranging from 0.72 to 0.96, indicating good performance in most studies. Out of the total of 14 studies, 5 did not conduct any validation (Lang et al., 1997; Rau et al., 2018; Abujaber et al., 2020; Lee et al., 2022; Wang et al., 2022), 7 studies solely conducted only internal validation (Güiza et al., 2013; Matsuo et al., 2020, 2023; Pease et al., 2022; Satyadev et al., 2022; Warman et al., 2022; Song et al., 2023), while 1 study exclusively performed external validation (Tu et al., 2022). Only 1 study conducted both internal and external validation (Supplementary Table 1; Wu et al., 2023). Of the studies that performed internal validation, five used cross-validation methods (Matsuo et al., 2020; Satyadev et al., 2022; Warman et al., 2022; Song et al., 2023; Wu et al., 2023), one used bootstrap validation (Güiza et al., 2013), and the remaining two did not explicitly describe their internal validation methods (Lang et al., 1997; Wang et al., 2022). For the studies that performed external validation, one study validated the model by recruiting an additional 200 patients with similar characteristics and outcomes, while the other validated using clinical data from other centers.
3.2 Meta-analysis
We summarized the results of 15 studies (one of which constructed two different machine learning models using two different datasets). Based on these studies, the AUC of merging was calculated as 0.90 (95% CI: 0.87 to 0.92), as shown in Figure 3. In addition, the sensitivity of merging was found to be 0.74 (95% CI: 0.69 to 0.78; I2 = 87.19%, p = 0.00), while the specificity of merging was determined to be 0.92 (95% CI: 0.89 to 0.94; I2 = 99.08%, p = 0.00), as shown in Figure 4. This data demonstrates that machine learning techniques exhibit good predictive performance for mortality in TBI patients.
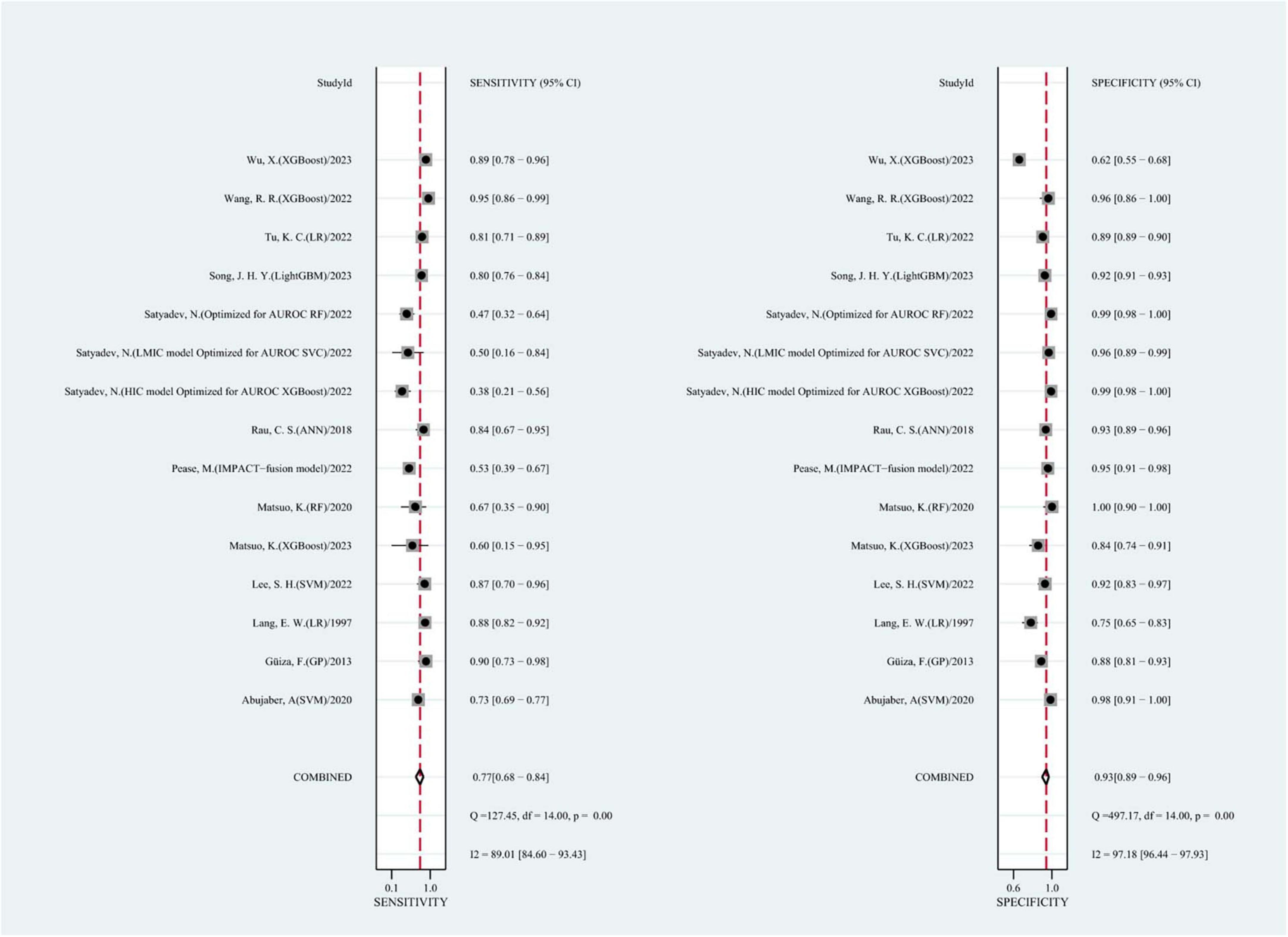
Figure 4. The overall pooled sensitivity and specificity of machine learning models for predicting mortality after TBI. The first author of each study was listed along the y-axis.
Meta-regression and subgroup analyses were performed because of the substantial heterogeneity observed in the study (Figure 5). It was speculated that this heterogeneity could be due to several factors, including whether the model was log-regressed, whether there were reports of missing data processing, whether the model was validated, and whether the outcomes were identical (such as 6-month mortality or in-hospital mortality). the same (e.g., 6-month mortality or in-hospital mortality). The results indicate that the heterogeneity in sensitivity may be attributable to the reporting of missing data handling and model validation.
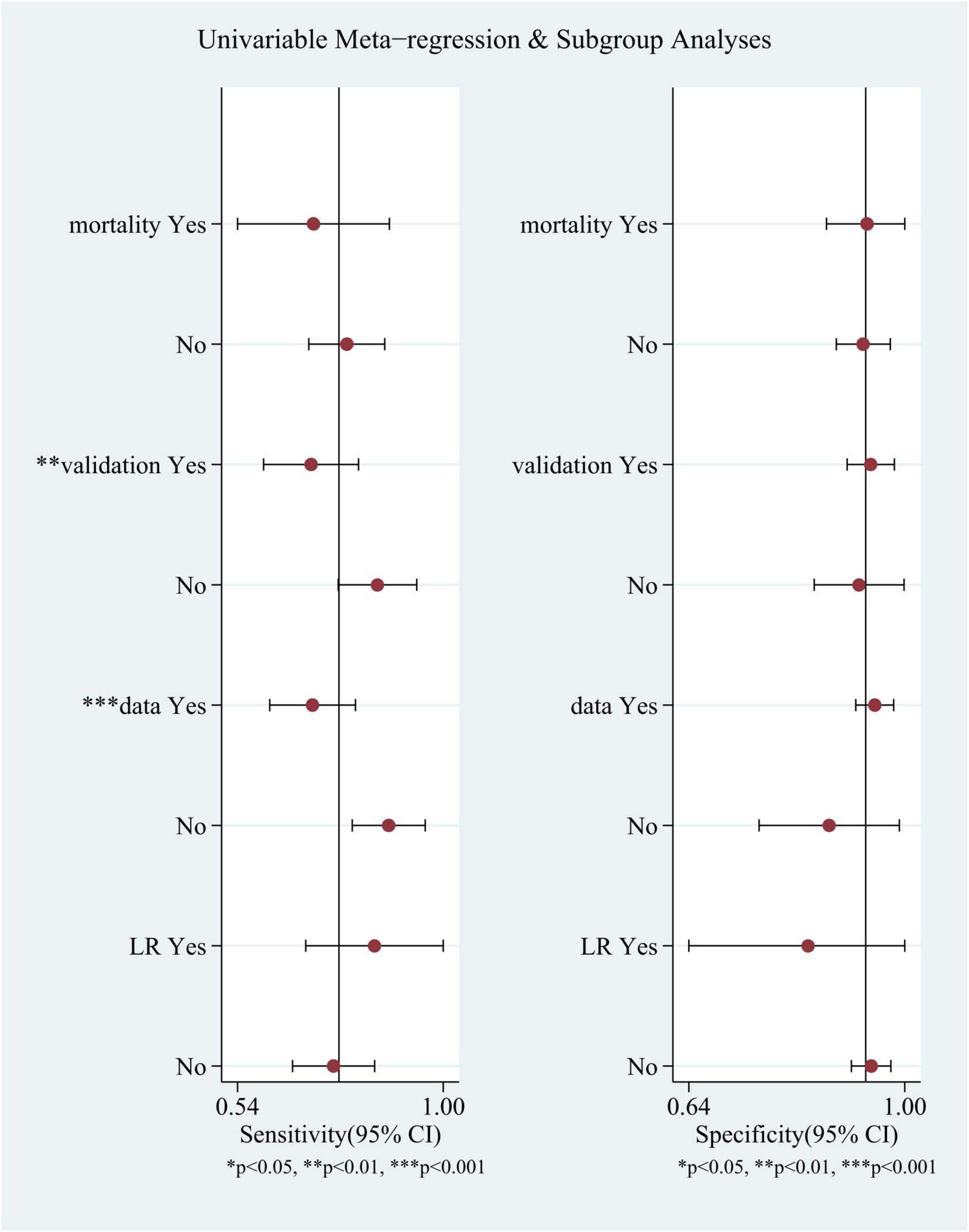
Figure 5. Univariable meta-regression and subgroup analyses. Comparison of sensitivity and specificity of different subgroups in TBI mortality prediction by machine learning models.
3.3 Critical appraisal
The 14 studies included in our study were assessed using PROBAST (Figures 6, 7), all considered to be at high risk of bias, with the analysis process the highest. The PROBAST tool recommends the Events Per Variable criterion (EPV) to assess overfitting. The EPV of most included studies is <10, indicating a risk of overfitting (Austin and Steyerberg, 2017). Additionally, only a few studies reported whether they considered and interpreted the complexity of the data, which is a potential reason for bias.
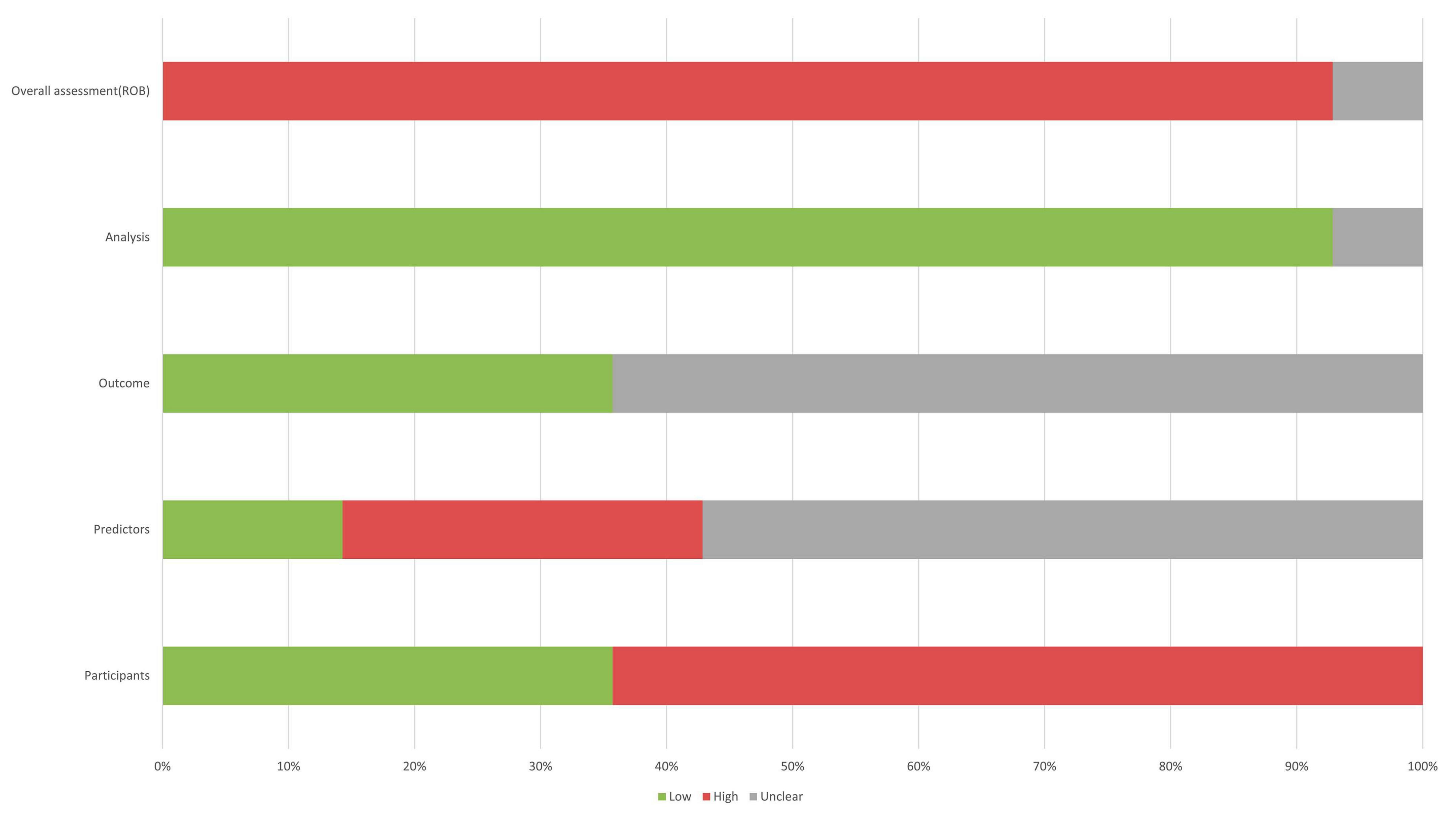
Figure 6. Risk of bias assessment for the predictive model studies. Study compliance with the predictive model risk of bias assessment tool (PROBAST).
4 Discussion
Prognostic prediction of TBI has always been a critical clinical issue, especially due to the high mortality rate and potential long-term vegetative state faced by patients with moderate to severe TBI (Figure 8; Stocchetti and Zanier, 2016). Therefore, early mortality prediction plays a crucial role in helping healthcare professionals and families make informed decisions. The International Mission for Prognosis and Analysis of Clinical Trials in TBI (IMPACT) and the Corticosteroid Randomization After Significant Head Injury (CRASH) are two previously developed models that aimed to predict the prognosis of TBI patients (Bracken, 2005; MRC CRASH Trial Collaborators, Perel et al., 2008). These models utilized a sizable sample obtained from many countries and were internally and externally validated during the initial development process, demonstrating favorable performance. With the continuous advancement of machine learning technology, various machine learning algorithms have been used to build prognostic models of TBI patients, with different types of data, including data obtained from head CT scans and blood biomarkers. Comprehensive analysis of these data can effectively predict the mortality of TBI patients. However, the overall performance of these predictive models remains unclear. Therefore, this systematic review and meta-analysis aimed to assess the effectiveness of machine learning-based models in predicting mortality after TBI.
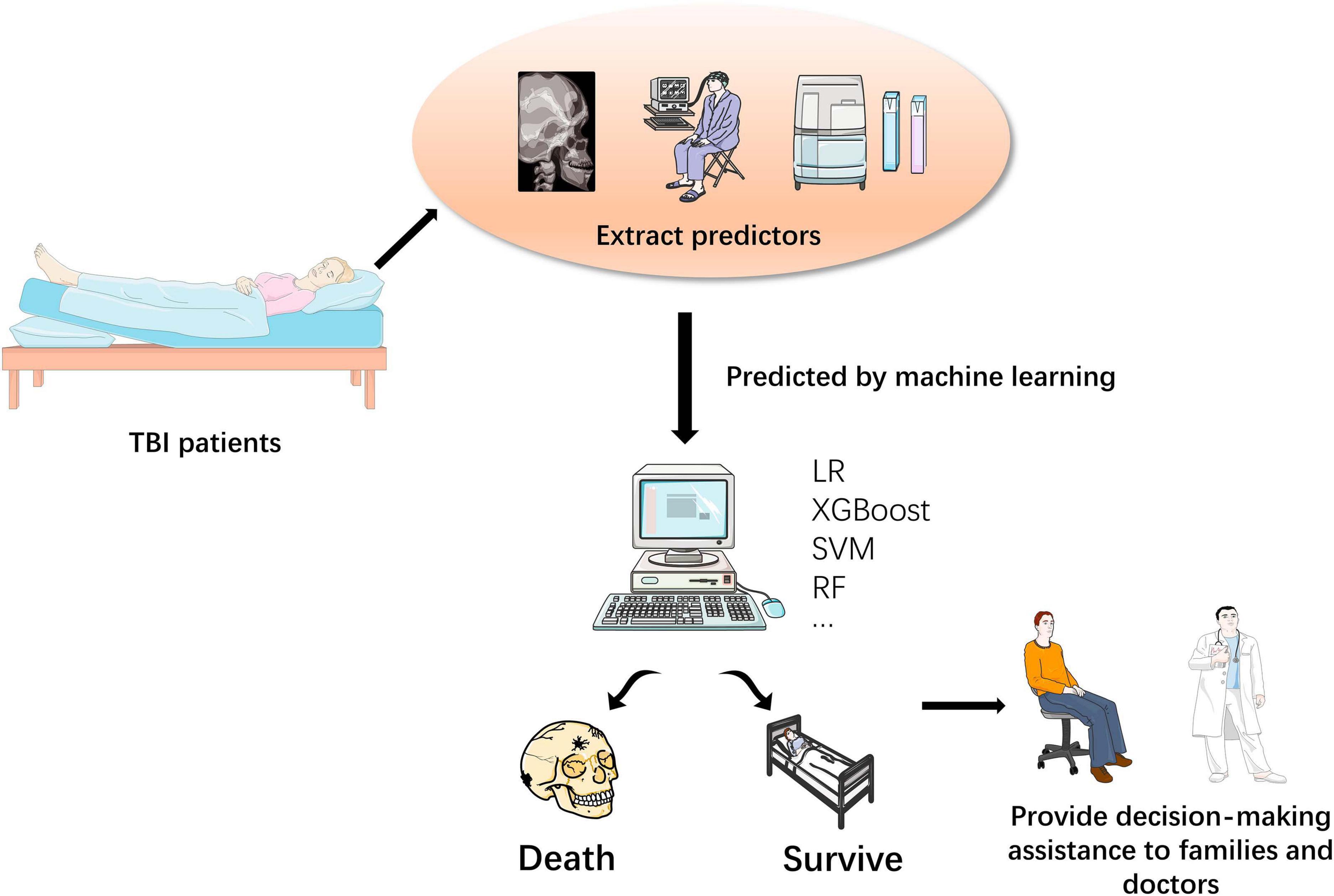
Figure 8. Process for predicting mortality in TBI patients using machine learning. The TBI mortality prediction model built by machine learning helps doctors and patients’ families make decisions.
In this study, we included 15 machine learning-based predictive models from 14 studies with a total AUC = 0.90, outperforming IMPACT and CRASH in an external validation of a large dataset (Roozenbeek et al., 2012). However, the PROBAST assessment showed that these 14 studies showed a high risk of bias, which makes it challenging to accurately assess the overall performance of these predictive models. While most of the included studies were validated internally using the cross-validation methods accepted by the PROBAST tool, only one study conducted both internal and external validation, so more follow-up studies are needed to further validate the performance of the proposed model to ensure the reliability of the predictive model in clinical applications.
From data sources, all studies included case data from more than 200 people, of which eight studies involved more than 1,000 cases. However, due to the relatively limited number of events (number of deaths) in these patients, the vast majority of predictive models EPV < 10 (van Smeden et al., 2019). In addition, the quality of data for patients in retrospective studies was lower than in prospective studies, whereas most of the studies we included were retrospective. Therefore, it is better if the EPV of the included data sample is as high as possible to 20 in future studies, as recommended by the PROBAST tool, and to try to select more data from prospective studies to ensure further reliable model performance. Although the performance and reliability of the predictive models in the current research do not mean that all models using machine learning perform better and more reliably than traditional models, with the development of machine learning technology, this may indicate that the TBI predictive model based on machine learning has broader prospects in future clinical applications.
Whilst this study comprehensively explores the field of machine-learning based prediction of mortality in patients with TBI, it is important to recognize that rapid advances in machine-learning technology may lead to a significant amount of research in related areas in a short period of time. Therefore, this is one of the limitations of the current study. Furthermore, it is worth noting that this study assessed the overall performance of the included machine learning models without identifying the best performing algorithm. Consequently, further research is needed to determine the most effective algorithm. Finally, because the cohort of patients included in this study were from different countries and exhibited different medical conditions, these factors may potentially affect the predictive performance of the models.
Data availability statement
The original contributions presented in the study are included in the article/Supplementary material, further inquiries can be directed to the corresponding authors.
Author contributions
ZW: Writing – original draft. JL: Writing – original draft. QH: Writing – original draft. LL: Writing – review and editing. SL: Supervision, Writing – review and editing, Validation. XC: Supervision, Writing – review and editing. YH: Writing-original draft, Supervision, Writing – review and editing.
Funding
The author(s) declare financial support was received for the research, authorship, and/or publication of this article. This work was supported in part by grants from National Natural Science Foundation of China (Grant Nos. 82200871 and 82371390), the Major Scientific Research Program for Young and Middle-aged Health Professionals of Fujian Province, China (Grant No. 2022ZQNZD007), and Fujian Province Scientific Foundation (Grant No. 2023J01725).
Conflict of interest
The authors declare that the research was conducted in the absence of any commercial or financial relationships that could be construed as a potential conflict of interest.
Publisher’s note
All claims expressed in this article are solely those of the authors and do not necessarily represent those of their affiliated organizations, or those of the publisher, the editors and the reviewers. Any product that may be evaluated in this article, or claim that may be made by its manufacturer, is not guaranteed or endorsed by the publisher.
Supplementary material
The Supplementary Material for this article can be found online at: https://www.frontiersin.org/articles/10.3389/fnins.2023.1285904/full#supplementary-material
Abbreviations
CIs, confidence intervals; PRISMA, preferred reporting items for systematic reviews and meta-analyses; sROC, summary ROC; HSROC, hierarchical summary receiver operating characteristic; CHARMS, checklist for critical appraisal and data extraction for systematic reviews of prediction modeling studies; PROBAST, predictive model risk of bias assessment tool; LR, logistic regression; GP, Gaussian process; SVM, support vector machines; ANN, artificial neural network; NN, neural network; NB, naive Bayes; RF, random forest; DNN, deep neural networks; XGBoost, Xtreme gradient boosting; Extra trees, extremely randomized trees; CNN, convolutional neural networks; DT, decision tree; NLP, natural language processing; MLP, multi-layer perceptron; IMPACT, international mission for prognosis and analysis of clinical trials in TBI; CRASH, corticosteroid randomization after significant head injury; HIC, high-income country; LMIC, low- and middle-income country
References
Abujaber, A., Fadlalla, A., Gammoh, D., Abdelrahman, H., Mollazehi, M., and El-Menyar, A. (2020). Prediction of in-hospital mortality in patients on mechanical ventilation post traumatic brain injury: Machine learning approach. BMC Med. Inform. Decis. Mak. 20:336. doi: 10.1186/s12911-020-01363-z
Austin, P., and Steyerberg, E. (2017). Events per variable (EPV) and the relative performance of different strategies for estimating the out-of-sample validity of logistic regression models. Stat. Methods Med. Res. 26, 796–808. doi: 10.1177/0962280214558972
Bischof, G., and Cross, D. (2023). Brain trauma imaging. J. Nucl. Med. 64, 20–29. doi: 10.2967/jnumed.121.263293
Bracken, M. (2005). CRASH (Corticosteroid Randomization after Significant Head Injury Trial): Landmark and storm warning. Neurosurgery 57, 1300-2; discussion 1300-2. doi: 10.1227/01.neu.0000187320.71967.59
Capizzi, A., Woo, J., and Verduzco-Gutierrez, M. (2020). Traumatic brain injury: An overview of epidemiology, pathophysiology, and medical management. Med. Clin. North Am. 104, 213–238. doi: 10.1016/j.mcna.2019.11.001
Greener, J., Kandathil, S., Moffat, L., and Jones, D. T. (2022). A guide to machine learning for biologists. Nat. Rev. Mol. Cell Biol. 23, 40–55. doi: 10.1038/s41580-021-00407-0
Güiza, F., Depreitere, B., Piper, I., Van den Berghe, G., and Meyfroidt, G. (2013). Novel methods to predict increased intracranial pressure during intensive care and long-term neurologic outcome after traumatic brain injury: Development and validation in a multicenter dataset. Crit. Care Med. 41, 554–564. doi: 10.1097/CCM.0b013e3182742d0a
Ioannidis, J. (2008). Interpretation of tests of heterogeneity and bias in meta-analysis. J. Eval. Clin. Pract. 14, 951–957. doi: 10.1111/j.1365-2753.2008.00986.x
Lang, E., Pitts, L., Damron, S., and Rutledge, R. (1997). Outcome after severe head injury: An analysis of prediction based upon comparison of neural network versus logistic regression analysis. Neurol. Res. 19, 274–280. doi: 10.1080/01616412.1997.11740813
Lee, S., Lee, C., Hwang, S., and Kang, D. H. A. (2022). Machine learning-based prognostic model for the prediction of early death after traumatic brain injury: Comparison with the corticosteroid randomization after significant head injury (CRASH) Model. World Neurosurg. 166, e125–e134. doi: 10.1016/j.wneu.2022.06.130
Matsuo, K., Aihara, H., Hara, Y., Morishita, A., Sakagami, Y., Miyake, S., et al. (2023). Machine learning to predict three types of outcomes after traumatic brain injury using data at admission: A multi-center study for development and validation. J. Neurotrauma. 40, 1694–1706. doi: 10.1089/neu.2022.0515
Matsuo, K., Aihara, H., Nakai, T., Morishita, A., Tohma, Y., and Kohmura, E. (2020). Machine learning to predict in-hospital morbidity and mortality after traumatic brain injury. J. Neurotrauma. 37, 202–210. doi: 10.1089/neu.2018.6276
Moyer, J., Lee, P., Bernard, C., Henry, L., Lang, E., Cook, F., et al. (2022). Machine learning-based prediction of emergency neurosurgery within 24 h after moderate to severe traumatic brain injury. World J. Emerg. Surg. 17:42. doi: 10.1186/s13017-022-00449-5
MRC CRASH Trial Collaborators, Perel, P., Arango, M., Clayton, T., Edwards, P., Komolafe, E., et al. (2008). Predicting outcome after traumatic brain injury: Practical prognostic models based on large cohort of international patients. BMJ 336, 425–429. doi: 10.1136/bmj.39461.643438.25
Page, M., McKenzie, J., Bossuyt, P., Boutron, I., Hoffmann, T., Mulrow, C., et al. (2021). The PRISMA 2020 statement: An updated guideline for reporting systematic reviews. BMJ 372:n71. doi: 10.1136/bmj.n71
Pease, M., Arefan, D., Barber, J., Yuh, E., Puccio, A., Hochberger, K., et al. (2022). Outcome prediction in patients with severe traumatic brain injury using deep learning from head CT scans. Radiology 304, 385–394. doi: 10.1148/radiol.212181
Rau, C., Kuo, P., Chien, P., Huang, C., Hsieh, H., and Hsieh, C. (2018). Mortality prediction in patients with isolated moderate and severe traumatic brain injury using machine learning models. PLoS One 13:e0207192. doi: 10.1371/journal.pone.0207192
Reitsma, J., Glas, A., Rutjes, A., Scholten, R., Bossuyt, P., and Zwinderman, A. (2005). Bivariate analysis of sensitivity and specificity produces informative summary measures in diagnostic reviews. J. Clin. Epidemiol. 58, 982–990. doi: 10.1016/j.jclinepi.2005.02.022
Roozenbeek, B., Lingsma, H., Lecky, F., Lu, J., Weir, J., Butcher, I., et al. (2012). Prediction of outcome after moderate and severe traumatic brain injury: External validation of the International Mission on Prognosis and Analysis of Clinical Trials (IMPACT) and Corticoid Randomisation After Significant Head injury (CRASH) prognostic models. Crit. Care Med. 40, 1609–1617. doi: 10.1097/CCM.0b013e31824519ce
Satyadev, N., Warman, P., Seas, A., Kolls, B., Haglund, M., Fuller, A., et al. (2022). Machine learning for predicting discharge disposition after traumatic brain injury. Neurosurgery 90, 768–774. doi: 10.1227/neu.0000000000001911
Song, J., Shin, S., Jamaluddin, S., Chiang, W., Tanaka, H., Song, K., et al. (2023). Prediction of mortality among patients with isolated traumatic brain injury using machine learning models in Asian countries: An international multi-center cohort study. J. Neurotrauma. 40, 1376–1387. doi: 10.1089/neu.2022.0280
Stocchetti, N., and Zanier, E. (2016). Chronic impact of traumatic brain injury on outcome and quality of life: A narrative review. Crit. Care 20:148. doi: 10.1186/s13054-016-1318-1
Tu, K. C., Eric Nyam, T., Wang, C., Chen, N., Chen, K., Chen, C., et al. (2022). A computer-assisted system for early mortality risk prediction in patients with traumatic brain injury using artificial intelligence algorithms in emergency room triage. Brain Sci. 12:612.
van Smeden, M., Moons, K., de Groot, J., Collins, G., Altman, D., Eijkemans, M., et al. (2019). Sample size for binary logistic prediction models: Beyond events per variable criteria. Stat. Methods Med. Res. 28, 2455–2474. doi: 10.1177/0962280218784726
Wang, R., Wang, L., Zhang, J., He, M., and Xu, J. (2022). XGBoost machine learning algorism performed better than regression models in predicting mortality of moderate-to-severe traumatic brain injury. World Neurosurg. 163, e617–e622. doi: 10.1016/j.wneu.2022.04.044
Warman, P., Seas, A., Satyadev, N., Adil, S., Kolls, B., Haglund, M., et al. (2022). Machine learning for predicting in-hospital mortality after traumatic brain injury in both high-income and low- and middle-income countries. Neurosurgery 90, 605–612. doi: 10.1227/neu.0000000000001898
Wolff, R. F., Moons, K., Riley, R., Whiting, P., Westwood, M., Collins, G., et al. (2019). PROBAST: A tool to assess the risk of bias and applicability of prediction model studies. Ann. Internal Med. 170, 51–58.
Keywords: traumatic brain injury, machine learning, mortality predictor, meta-analysis, inpatient mortality
Citation: Wu Z, Lai J, Huang Q, Lin L, Lin S, Chen X and Huang Y (2023) Machine learning-based model for predicting inpatient mortality in adults with traumatic brain injury: a systematic review and meta-analysis. Front. Neurosci. 17:1285904. doi: 10.3389/fnins.2023.1285904
Received: 30 August 2023; Accepted: 30 October 2023;
Published: 14 December 2023.
Edited by:
Min Tang-Schomer, UConn Health, United StatesReviewed by:
Luis Rafael Moscote-Salazar, Colombian Clinical Research Group in Neurocritical Care, ColombiaStefano Maria Priola, Health Sciences North, Canada
Copyright © 2023 Wu, Lai, Huang, Lin, Lin, Chen and Huang. This is an open-access article distributed under the terms of the Creative Commons Attribution License (CC BY). The use, distribution or reproduction in other forums is permitted, provided the original author(s) and the copyright owner(s) are credited and that the original publication in this journal is cited, in accordance with accepted academic practice. No use, distribution or reproduction is permitted which does not comply with these terms.
*Correspondence: Yinqiong Huang, eWlucWlvbmdoQGZqbXUuZWR1LmNu; orcid.org/0000-0001-9186-3896; Xiangrong Chen, eGlhbmdyb25nX2NoZW4yODFAMTI2LmNvbQ==; Shu Lin, c2h1bGluMTk1NkAxMjYuY29t; orcid.org/0000-0002-4239-2028