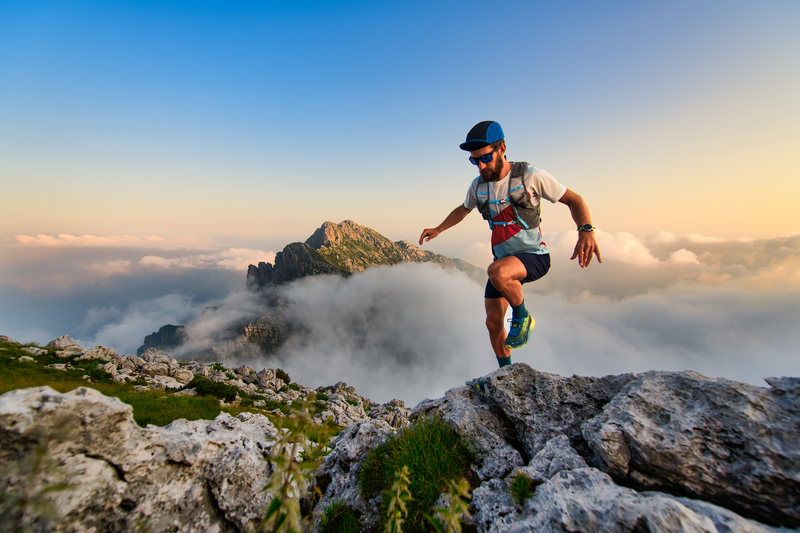
95% of researchers rate our articles as excellent or good
Learn more about the work of our research integrity team to safeguard the quality of each article we publish.
Find out more
SYSTEMATIC REVIEW article
Front. Neurosci. , 07 September 2023
Sec. Translational Neuroscience
Volume 17 - 2023 | https://doi.org/10.3389/fnins.2023.1256592
This article is part of the Research Topic Rapid and Accurate Brain Imaging and Brain Disease Diagnosis Under the Fractional Calculus System View all 7 articles
Objectives: This study aimed to assess the accuracy of artificial intelligence (AI) models in predicting the prognosis of stroke.
Methods: We searched PubMed, Embase, and Web of Science databases to identify studies using AI for acute stroke prognosis prediction from the database inception to February 2023. Selected studies were designed cohorts and had complete data. We used the Quality Assessment of Diagnostic Accuracy Studies tool to assess the qualities and bias of included studies and used a random-effects model to summarize and analyze the data. We used the area under curve (AUC) as an indicator of the predictive accuracy of AI models.
Results: We retrieved a total of 1,241 publications and finally included seven studies. There was a low risk of bias and no significant heterogeneity in the final seven studies. The total pooled AUC under the fixed-effects model was 0.872 with a 95% CI of (0.862–0.881). The DL subgroup showed its AUC of 0.888 (95%CI 0.872–0.904). The LR subgroup showed its AUC 0.852 (95%CI 0.835–0.869). The RF subgroup showed its AUC 0.863 (95%CI 0.845–0.882). The SVM subgroup showed its AUC 0.905 (95%CI 0.857–0.952). The Xgboost subgroup showed its AUC 0.905 (95%CI 0.805–1.000).
Conclusion: The accuracy of AI models in predicting the outcomes of ischemic stroke is good from our study. It could be an assisting tool for physicians in judging the outcomes of stroke patients. With the update of AI algorithms and the use of big data, further AI predictive models will perform better.
Artificial intelligence (AI) can be defined as the ability of computers or other machines to demonstrate or simulate intelligent behavior, like human beings (Krittanawong et al., 2017; Garcia-Vidal et al., 2019; Schwalbe and Wahl, 2020; Bonkhoff and Grefkes, 2022). Machine learning (ML) is one way to implement AI, which has shown the greatest potential in dealing with problems involving unstructured data, such as image recognition (Deo, 2015; Esteva et al., 2019). ML techniques utilize various methods for automated data analysis, including logistic regression (LR), random forests (RF), support vector machines (SVM), and classification trees, which allow combining features (data characteristics) with flexible decision boundaries in a non-linear manner. The advent of neural networks (NN) and deep learning (DL) techniques has changed the ML domain and achieved automatic and efficient feature recognition and processing in covert analysis networks without prior feature selection. There were some studies suggesting that ML and DL have again recently achieved substantial improvements and demonstrated comparable performance to trained physicians in the fields of other departments, like radiology and dermatology (Gulshan et al., 2016; Esteva et al., 2017; Hannun et al., 2019).
Acute stroke ranks among the leading causes of morbidity and mortality worldwide, and it can be divided into ischemic stroke and hemorrhagic stroke (Toyoda et al., 2022). In addition, predicting the outcome of a stroke often depends on the experience of the physician clinically, but it is difficult for inexperienced young physicians to judge the prognosis. In clinical, patients are most concerned about their clinical outcomes. Imagine that you are a young inexperienced physician and you are on duty in the ward, your patient asks you about the outcomes after treatment and you cannot ensure the judgment is right based on your own experience. If there is an objective tool at hand to predict the prognosis according to the patient’s condition quickly and accurately, using this tool to corroborate your judgment will make you more confident in judging the prognosis of your patient. Exactly, AI predictive models can bring objective results after learning input features and countless calculations. ML predictive models which are image-based feature recognition and segmentation and have greatly facilitated the rapid diagnosis of stroke, but stroke prognosis depends on a large number of patient-specific and clinical factors, so accurate prognostic prediction models remain challenging (Mendelson and Prabhakaran, 2021; Toyoda et al., 2022).
Although previous studies on predicting stroke prognosis also used many AI algorithms, the overall accuracy of AI models in predicting stroke prognosis is inconsistent. Tree-based algorithms own favorable interpretability and a relatively simple algorithm, and researchers that used RF algorithms performed high-accuracy prediction prognosis of acute ischemic stroke patients (AUC = 0.936 ± 0.034) and primary intracerebral hemorrhage stroke patients (AUC = 0.917) (Monteiro et al., 2018; Wang et al., 2019). However, the samples of these studies were not “big” datasets and had relatively poor representativeness. SVM is frequently used in predicting stroke outcomes relying on neuroimaging data and showing moderate to high accuracy prediction of prognosis, with an AUC ranging from 0.788 to 0.92 (Forkert et al., 2015; Giacalone et al., 2018; Lin et al., 2018; Nishi et al., 2019; Li et al., 2022; Roh et al., 2022). The present most complex algorithms, deep neural network (DNN), a model of DL, ran a bigger sample analysis and performed high accuracy prediction prognosis (AUC = 0.904) in minor stroke patients (Sung et al., 2020) and moderate accuracy prediction prognosis (AUC = 0.88 ± 0.12 and 0.888 ± 0.008) in acute ischemic stroke (Nielsen et al., 2018; Heo et al., 2019) but had poor interpretability for input variables.
This study aimed to analyze the literature to explore the accuracy of AI models in stroke outcome prediction and compare the AUC among different algorithms.
We performed this study according to the Preferred Reporting Items for Systematic Reviews and Meta-Analyses (PRISMA) guidelines (McInnes et al., 2018).
We searched “acute stroke” “artificial intelligence” “deep learning” “machine learning” “prognosis” and “outcome” in PubMed, Embase, and Web of Science databases from the inception to February 2023 and assessed eligible articles by screening titles and abstracts followed by full-text evaluation (Figure 1). In addition, we formulated our included studies as follows: (1) Population: patients diagnosed with acute ischemic or hemorrhagic stroke in retrospective and prospective cohorts and had prognosis data. The prognosis of stroke not only included the functional outcomes but also the radiological outcomes, the likelihood of morbidity, and mortality (Toyoda et al., 2022). (2) Index test: the predictive prognosis results of AI. (3) Reference standard: recognized prognosis recorded in included studies. (4) Outcomes: area under the curve (AUC) with its 95% confidence interval or standard error of receiver operator characteristic in AI models on stroke prognosis.
Two independent investigators extracted the following information from the included studies: first author, publication year, country, population data (age and sex), and outcomes. Data extraction forms included details on the included study characteristics. Two investigators assessed the quality and bias of studies independently by using The Revised Tool for the Quality Assessment of Diagnostic Accuracy Studies (QUADAS) scores. In the whole process of data extraction and quality assessment, all different opinions were solved through discussion with the third reviewer.
We pooled the outcome data using a fixed effects model. Heterogeneity among studies was evaluated using the Q statistic and I-squared test (I2). Significant heterogeneity was defined as p-value <0.05 or I2 > 50%. For sensitivity analyses, one by one elimination method was performed to investigate the robustness of the results. All statistical analyses were performed using MedCalc® statistical software version 22.009 (MedCalc Software Ltd., Ostend, Belgium1; 2023).
We retrieved a total of 1,241 publications. Finally, we included seven studies with 4,379 ischemic stroke participants. The detailed flow chart is shown in Figure 1. In the seven included studies, there were 17 predictive models and they were divided into five subgroups according to their algorithms (SVM, RF, LR, DL, and Xgboost).
The characteristics of all included studies are shown in Table 1. In our review, four articles (Monteiro et al., 2018; Heo et al., 2019; Nishi et al., 2019; Li et al., 2022) used mRS as functional outcomes, and they all thought mRS ≤ 2 was a good outcome. The other three articles (Lin et al., 2018; Nielsen et al., 2018; Grosser et al., 2020) used radiological biomarkers, follow-up lesion volume, and neurological deterioration as outcomes.
All included studies had low to moderate risks in QUADAS scores, and the risk of bias is shown in Figure 2. The heterogeneity test of studies for analyzing AUC showed I2 = 27.67%, suggesting that there was no significant heterogeneity in the study.
Forest plots (Figure 3) presented AUC and its 95% CI for the included 17 models in turn. The pooled AUC under the fixed-effects model was 0.872 with a 95% CI of (0.862–0.881) (Table 2).
Table 3 shows the subgroup results of this meta-analysis. The DL subgroup showed an AUC of 0.888 (95%CI 0.872–0.904). The LR subgroup showed an AUC of 0.852 (95%CI 0.835–0.869). The RF subgroup showed an AUC of 0.863 (95%CI 0.845–0.882). The SVM subgroup showed an AUC of 0.905 (95%CI 0.857–0.952). The Xgboost subgroup showed an AUC of 0.905 (95%CI 0.805–1.000). All results showed a good performance of AI models in predicting the outcome of stroke patients (Mandrekar, 2010).
To our knowledge, this is the first meta-analysis to study AI models’ performance in predicting stroke outcomes. In the final included studies, the participants were ischemic stroke patients. Many studies on the outcome prediction of hemorrhagic stroke did not meet our inclusion criteria. Thus, our results apply to ischemic stroke patients only. According to our results, the overall performance of the predictive model for ischemic stroke outcomes is good. In subgroup analysis, the SVM was the most accurate and the LR models were the least in terms of comparison AUC.
Previously, many studies in stroke outcomes used some variant of linear regression models, which are generally easier to interpret, but they do not automatically exploit nonlinear relationships and interactions, leading to poor prediction accuracy. The prognostic models from the Virtual International Stroke Trials Archive on functional outcome and survival are AUC 0.808 and 0.706 (Konig et al., 2008), respectively, which is a relatively accurate and simple prediction scale, but compared to AI predictive models, AI models show better accurate performance. The biomarker-based CoRisk score was AUC 0.819, of which the score components were copeptin levels, age, NIH Stroke Scale, and recanalization therapy (De Marchis et al., 2019). The prediction model score showed relatively good accuracy and interpretation, but whether it is necessary to detect the plasma copeptin levels in clinical is still to be decided. In our study, the participants that included in our analysis used rapid prediction from medical data obtained at the time of presentation at the emergency department or obtained imaging data after admission or interventional procedures. This indicates that the use of AI to build a prediction model has better clinical applicability. However, clinicians typically have a limited understanding of this methodology. Therefore, we conducted this systematic review and meta-analysis to clarify how AI models can provide stroke outcome prediction.
In addition, the points of prediction tools in clinical practice are feasibility and acceptability as overly complex scales will not be used in acute stroke. Although DL models have good predictive accuracy, the complexity and non-interpretability of models limit their clinical application. According to our results, though SVM and Xgboost models had similar AUC, SVM seemed to perform better than Xgboost in predicting stroke outcomes due to discrete linear data or proper nonlinear kernels that fit the data better by improved generalization (Noble, 2006; Roh et al., 2022). Regrettably, we did not know why researchers chose the specific algorithm for their predictive models during the literature review.
In contrast to traditional predictive scores, most AI predictive models share a common set of independent demographic variables, laboratory values, and imaging feathers. While some variables and characteristics are not well validated individually in clinical, they may add predictive value in some cases. A comparative study of ML algorithms and traditional risk models is needed. If these studies demonstrate the advantages of ML-based prediction, then optimization algorithms can be implemented through electronic health records to facilitate the application of clinical practice.
The limitations of our study are that we assessed the accuracy of the prediction only through the AUC, not exactly focusing on the sensitivity, specificity, and accuracy rate because of incomplete data for meta-analysis. We decided to use AUC to assess the accuracy of predictive models because AUC could remain stable even when the distribution of positive and negative samples in the test set changed. Therefore, despite the simplicity of our results, they were explanatory and reliable. What is more? Because of the large scope of our review, our goal was systematic rather than comprehensive. Therefore, we might miss some relevant studies, but we consider it unlikely that these studies were of higher quality than those already included. Another problem was the sample size included in the literature, none of which could be called as big data; only one study of more than 1,000 participants, and according to previous studies, there are a large number of candidate predictor variables to analyze, so future AI predictive models must be developed for a large number of patients.
AI predictive models have high accuracy in predicting the outcome of stroke, which assists physicians to judge the specific outcome of a patient and adjust the treatment plan according to the outcome of the judgment.
The original contributions presented in the study are included in the article/supplementary material, further inquiries can be directed to the corresponding authors.
YY: Conceptualization, Data curation, Formal analysis, Methodology, Software, Writing – original draft, Writing – review & editing. LT: Investigation, Methodology, Writing – review & editing. YD: Data curation, Writing – review & editing. XL: Writing – review & editing. AL: Writing – review & editing, Data curation. ZZ: Writing – review & editing. LH: review & editing. CZ: Methodology, Writing – review & editing. MZ: Funding acquisition, Methodology, Project administration, Writing – review & editing.
The author(s) declare financial support was received for the research, authorship, and/or publication of this article. The China National Key Research and Development Project, 2021YFC2500506, supported this work.
The authors declare that the research was conducted in the absence of any commercial or financial relationships that could be construed as a potential conflict of interest.
All claims expressed in this article are solely those of the authors and do not necessarily represent those of their affiliated organizations, or those of the publisher, the editors and the reviewers. Any product that may be evaluated in this article, or claim that may be made by its manufacturer, is not guaranteed or endorsed by the publisher.
AI, Artificial intelligence; ML, Machine learning; DL, Deep learning; mRS, modified Rankin Scale; AUC, area under curve; RF, random forest; SVM, support vector machine; LR, Logistic regression.
Bonkhoff, A. K., and Grefkes, C. (2022). Precision medicine in stroke: towards personalized outcome predictions using artificial intelligence. Brain 145, 457–475. doi: 10.1093/brain/awab439
De Marchis, G. M., Dankowski, T., Konig, I. R., Fladt, J., Fluri, F., Gensicke, H., et al. (2019). A novel biomarker-based prognostic score in acute ischemic stroke: the corisk score. Neurology 92, e1517–e1525. doi: 10.1212/WNL.0000000000007177
Deo, R. C. (2015). Machine learning in medicine. Circulation 132, 1920–1930. doi: 10.1161/CIRCULATIONAHA.115.001593
Esteva, A., Kuprel, B., Novoa, R. A., Ko, J., Swetter, S. M., Blau, H. M., et al. (2017). Dermatologist-level classification of skin cancer with deep neural networks. Nature 542, 115–118. doi: 10.1038/nature21056
Esteva, A., Robicquet, A., Ramsundar, B., Kuleshov, V., DePristo, M., Chou, K., et al. (2019). A guide to deep learning in healthcare. Nat. Med. 25, 24–29. doi: 10.1038/s41591-018-0316-z
Forkert, N. D., Verleger, T., Cheng, B., Thomalla, G., Hilgetag, C. C., and Fiehler, J. (2015). Multiclass support vector machine-based lesion mapping predicts functional outcome in ischemic stroke patients. PLoS One 10:e0129569. doi: 10.1371/journal.pone.0129569
Garcia-Vidal, C., Sanjuan, G., Puerta-Alcalde, P., Moreno-Garcia, E., and Soriano, A. (2019). Artificial intelligence to support clinical decision-making processes. EBioMedicine 46, 27–29. doi: 10.1016/j.ebiom.2019.07.019
Giacalone, M., Rasti, P., Debs, N., Frindel, C., Cho, T. H., Grenier, E., et al. (2018). Local spatio-temporal encoding of raw perfusion mri for the prediction of final lesion in stroke. Med. Image Anal. 50, 117–126. doi: 10.1016/j.media.2018.08.008
Grosser, M., Gellissen, S., Borchert, P., Sedlacik, J., Nawabi, J., Fiehler, J., et al. (2020). Improved multi-parametric prediction of tissue outcome in acute ischemic stroke patients using spatial features. PLoS One 15:e0228113. doi: 10.1371/journal.pone.0241917
Gulshan, V., Peng, L., Coram, M., Stumpe, M. C., Wu, D., Narayanaswamy, A., et al. (2016). Development and validation of a deep learning algorithm for detection of diabetic retinopathy in retinal fundus photographs. JAMA 316, 2402–2410. doi: 10.1001/jama.2016.17216
Hannun, A. Y., Rajpurkar, P., Haghpanahi, M., Tison, G. H., Bourn, C., Turakhia, M. P., et al. (2019). Cardiologist-level arrhythmia detection and classification in ambulatory electrocardiograms using a deep neural network. Nat. Med. 25, 65–69. doi: 10.1038/s41591-018-0268-3
Heo, J., Yoon, J. G., Park, H., Kim, Y. D., Nam, H. S., and Heo, J. H. (2019). Machine learning–based model for prediction of outcomes in acute stroke. Stroke 50, 1263–1265. doi: 10.1161/STROKEAHA.118.024293
Konig, I. R., Ziegler, A., Bluhmki, E., Hacke, W., Bath, P. M., Sacco, R. L., et al. (2008). Predicting long-term outcome after acute ischemic stroke: a simple index works in patients from controlled clinical trials. Stroke 39, 1821–1826. doi: 10.1161/STROKEAHA.107.505867
Krittanawong, C., Zhang, H., Wang, Z., Aydar, M., and Kitai, T. (2017). Artificial intelligence in precision cardiovascular medicine. J. Am. Coll. Cardiol. 69, 2657–2664. doi: 10.1016/j.jacc.2017.03.571
Li, Y., Liu, Y., Hong, Z., Wang, Y., and Lu, X. (2022). Combining machine learning with radiomics features in predicting outcomes after mechanical thrombectomy in patients with acute ischemic stroke. Comput. Methods Prog. Biomed. 225:107093. doi: 10.1016/j.cmpb.2022.107093
Lin, J., Jiang, A., Ling, M., Mo, Y., Li, M., and Zhao, J. (2018). Prediction of neurologic deterioration based on support vector machine algorithms and serum osmolarity equations. Brain Behav. 8:e01023. doi: 10.1002/brb3.1023
Mandrekar, J. N. (2010). Receiver operating characteristic curve in diagnostic test assessment. J. Thorac. Oncol. 5, 1315–1316. doi: 10.1097/JTO.0b013e3181ec173d
McInnes, M. D. F., Moher, D., Thombs, B. D., McGrath, T. A., Bossuyt, P. M., the P-DTAG, et al. (2018). Preferred reporting items for a systematic review and meta-analysis of diagnostic test accuracy studies: the prisma-dta statement. JAMA 319, 388–396. doi: 10.1001/jama.2017.19163
Mendelson, S. J., and Prabhakaran, S. (2021). Diagnosis and management of transient ischemic attack and acute ischemic stroke: a review. JAMA 325, 1088–1098. doi: 10.1001/jama.2020.26867
Monteiro, M., Fonseca, A. C., Freitas, A. T., Pinho, E. M. T., Francisco, A. P., Ferro, J. M., et al. (2018). Using machine learning to improve the prediction of functional outcome in ischemic stroke patients. IEEE/ACM Trans. Comput. Biol. Bioinform. 15, 1953–1959. doi: 10.1109/TCBB.2018.2811471
Nielsen, A., Hansen, M. B., Tietze, A., and Mouridsen, K. (2018). Prediction of tissue outcome and assessment of treatment effect in acute ischemic stroke using deep learning. Stroke 49, 1394–1401. doi: 10.1161/STROKEAHA.117.019740
Nishi, H., Oishi, N., Ishii, A., Ono, I., Ogura, T., Sunohara, T., et al. (2019). Predicting clinical outcomes of large vessel occlusion before mechanical thrombectomy using machine learning. Stroke 50, 2379–2388. doi: 10.1161/STROKEAHA.119.025411
Noble, W. S. (2006). What is a support vector machine? Nat. Biotechnol. 24, 1565–1567. doi: 10.1038/nbt1206-1565
Roh, S. B., Oh, S. K., Pedrycz, W., and Fu, Z. (2022). Dynamically generated hierarchical neural networks designed with the aid of multiple support vector regressors and pnn architecture with probabilistic selection. IEEE Trans Neural Netw Learn Syst. 33, 1385–1399. doi: 10.1109/TNNLS.2020.3041947
Schwalbe, N., and Wahl, B. (2020). Artificial intelligence and the future of global health. Lancet 395, 1579–1586. doi: 10.1016/S0140-6736(20)30226-9
Sung, S. M., Kang, Y. J., Cho, H. J., Kim, N. R., Lee, S. M., Choi, B. K., et al. (2020). Prediction of early neurological deterioration in acute minor ischemic stroke by machine learning algorithms. Clin. Neurol. Neurosurg. 195:105892. doi: 10.1016/j.clineuro.2020.105892
Toyoda, K., Yoshimura, S., Nakai, M., Koga, M., Sasahara, Y., Sonoda, K., et al. (2022). Twenty-year change in severity and outcome of ischemic and hemorrhagic strokes. JAMA Neurol. 79, 61–69. doi: 10.1001/jamaneurol.2021.4346
Keywords: artificial intelligence, machine learning, deep learning, acute stroke, outcome, prognosis, prediction
Citation: Yang Y, Tang L, Deng Y, Li X, Luo A, Zhang Z, He L, Zhu C and Zhou M (2023) The predictive performance of artificial intelligence on the outcome of stroke: a systematic review and meta-analysis. Front. Neurosci. 17:1256592. doi: 10.3389/fnins.2023.1256592
Received: 11 July 2023; Accepted: 02 August 2023;
Published: 07 September 2023.
Edited by:
Ni Zhang, Sichuan University, ChinaReviewed by:
Xia Zhang, Second Affiliated Hospital of Soochow University, ChinaCopyright © 2023 Yang, Tang, Deng, Li, Luo, Zhang, He, Zhu and Zhou. This is an open-access article distributed under the terms of the Creative Commons Attribution License (CC BY). The use, distribution or reproduction in other forums is permitted, provided the original author(s) and the copyright owner(s) are credited and that the original publication in this journal is cited, in accordance with accepted academic practice. No use, distribution or reproduction is permitted which does not comply with these terms.
*Correspondence: Cairong Zhu, Q2Fpcm9uZy56aHVAaG90bWFpbC5jb20=; Muke Zhou, bXVrZS56aG91QHNjdS5lZHUuY24=
Disclaimer: All claims expressed in this article are solely those of the authors and do not necessarily represent those of their affiliated organizations, or those of the publisher, the editors and the reviewers. Any product that may be evaluated in this article or claim that may be made by its manufacturer is not guaranteed or endorsed by the publisher.
Research integrity at Frontiers
Learn more about the work of our research integrity team to safeguard the quality of each article we publish.