- Department of Magnetic Resonance Imaging, The First Affiliated Hospital of Zhengzhou University, Zhengzhou, China
Introduction: Abnormal interactions among distributed brain systems are implicated in the mechanisms of nicotine addiction. However, the relationship between the structural covariance network, a measure of brain connectivity, and smoking severity remains unclear. To fill this gap, this study aimed to investigate the relationship between structural covariance network and smoking severity in smokers.
Methods: A total of 101 male smokers and 51 male non-smokers were recruited, and they underwent a T1-weighted anatomical image scan. First, an individualized structural covariance network was derived via a jackknife-bias estimation procedure for each participant. Then, a data-driven machine learning method called connectome-based predictive modeling (CPM) was conducted to infer smoking severity measured with Fagerström Test for Nicotine Dependence (FTND) scores using an individualized structural covariance network. The performance of CPM was evaluated using the leave-one-out cross-validation and a permutation testing.
Results: As a result, CPM identified the smoking severity-related structural covariance network, as indicated by a significant correlation between predicted and actual FTND scores (r = 0.23, permutation p = 0.020). Identified networks comprised of edges mainly located between the subcortical–cerebellum network and networks including the frontoparietal default model and motor and visual networks.
Discussion: These results identified smoking severity-related structural covariance networks and provided a new insight into the neural underpinnings of smoking severity.
Introduction
Nicotine addiction or smoking, characterized by compulsive tobacco seeking and smoking, is one of the leading causes of preventable disease worldwide, contributing to cancer and respiratory and cardiovascular diseases (Branstetter et al., 2016; Shen et al., 2018). Every year, more than 5 million people die of tobacco use (Wen et al., 2021). It is of great clinical significance to investigate the underlying neural substrates of nicotine addiction. However, the underlying mechanisms of smoking are still unclear.
In recent years, studies with modern neuroimaging have identified that abnormal interactions among distributed brain regions are implicated in the mechanisms of nicotine addiction (Fedota and Stein, 2015). In neuroimaging studies, the interaction between brain regions is usually measured with synchronized low-frequency fluctuations of the blood oxygen level-dependent (BOLD) signal between brain regions in the absence of explicit task, termed as resting-state functional connectivity (FC) (Biswal et al., 1995; Molecular Psychiatry). Altered FC among large-scale brain networks is well documented in smokers. For example, relative to non-smokers, chronic smokers show reduced FC within frontal–parietal executive control networks (Weiland et al., 2015), and connections within this network are predictive of smoking status (Pariyadath et al., 2014). Structural and functional deficits within frontostriatal circuits are also reported in smokers (Yuan et al., 2016), where the striatum mainly regulates the rewarding effect of smoking (Barrett et al., 2004) and motivation to smoke (Le Foll et al., 2014). In addition to functional interaction, brain regions also exhibit structural interactions, such as structural covariance. As another brain connectivity metric, structural covariance measures gray matter morphological similarity (measured with correlation) between brain regions reflecting synchronized maturation (Alexander-Bloch et al., 2013; Yun et al., 2015). Compared with FC, structural covariance represents more stable and highly heritable brain connectivity features (Lerch et al., 2006; Evans, 2013). As a complementary metric of brain connectivity, structural covariance provides a specific and distinctive measurement of network-level brain features (Subirà et al., 2016). Brain disorders are found to be accompanied by a rearranged architecture of the structural covariance network (Mitelman et al., 2005; Alexander-Bloch et al., 2013; Han et al., 2022a,b; Xue et al., 2023). However, to the best of our knowledge, there is no study investigating the association between structural covariance network and smoking severity in nicotine addiction.
Recently, a machine learning method called connectome-based predictive modeling (CPM) is proposed to predict individual behavior from whole-brain connectivity data (“connectomes”) (Finn et al., 2015; Shen et al., 2017). Unlike the traditional brain-behavior models such as correlation or regression models, CPM avoids over-fitting and increases the likelihood of generalization in novel samples. This approach is used to identify connectome fingerprints of specific behaviors, showing potential to identify novel treatment targets (Finn et al., 2015; Shen et al., 2017). To date, CPM has been used to identify connectome fingerprints of IQ (Finn et al., 2015), individual anxiety (Wang et al., 2021), and creativity anxiety (Ren et al., 2021). In addition, CPM identifies connectome fingerprints of carving intensities in Internet gaming disorder (Zhou et al., 2022), dissociable neural substrates of opioid and cocaine use (Lichenstein et al., 2021), and connectome fingerprints of cocaine abstinence (Yip et al., 2019).
In this study, we aimed to identify the structural covariance network predictive of smoking severity in smokers with CPM. A total of 101 smokers and 51 non-smokers were recruited, and they underwent T1-weighted anatomical image scans. First, we obtained an individualized structural covariance network for each participant via a jackknife-bias estimation procedure (Das et al., 2018; Han et al., 2022a,b). Then, CPM was conducted to infer smoking severity measured with Fagerström Test for Nicotine Dependence (FTND) scores (Heatherton et al., 1991) using the individualized structural covariance network. We expected that CPM could significantly predict FTND scores using the individualized structural covariance network over chance.
Methods
Samples
This study was approved by the Research Ethics Committee of the First Affiliated Hospital of Zhengzhou University. All study procedures were performed in accordance with the 1975 Declaration of Helsinki, and written informed consent was obtained from all participants before the experiment.
A total of 101 smokers and 51 non-smokers were recruited through online platforms and advertisements. All the subjects were right-handed male smokers. The smokers met the DSM-IV criteria for nicotine dependence, smoked at least once daily in the past 2 years, and had no period of smoking abstinence longer than 3 months (Shen et al., 2018). The Fagerström Test for Nicotine Dependence (FTND) was used to measure nicotine dependence (Heatherton et al., 1991). Non-smokers are those who did not currently smoke and had no history of consumption of cigarettes (or nicotine products). In addition, all the participants must meet the following inclusion criteria: (1) physical and neuropsychiatric diseases; (2) currently using or a history of psychotropic medications; (3) other current drug abuse (except nicotine); and (4) contraindications for MRI scanning.
Data acquisition
Three-dimensional high-resolution T1-weighted sagittal images were obtained with 3D magnetization prepared rapid gradient echo (3D-MPRAGE) on 3-Tsela German Siemens Prisma. The scanning parameters were as follows: repetition time = 2,000 ms, voxel size = 1 mm3, inversion time = 900 ms, echo time = 2.06 ms, flip angle = 9 degrees, FOV = 256 × 256 mm2, slices = 192, and slice thickness = 1.0 mm.
Voxel-based morphometry analysis
Gray matter volume (GMV) was measured using a voxel-based morphometry analysis (VBM) (Ashburner and Friston, 2000). This procedure was conducted following the standard pipeline of the CAT12 toolbox (http://dbm.neuro.uni-jena.de/cat12/): Structural images were first segmented into the gray matter, white matter, and cerebrospinal fluid, normalized into Montreal Neurological Institute space and resampled to 1.5 mm3. Finally, the gray matter maps were smoothed using 6 mm full width at half maximum Gaussian kernel (FWHM) (Ashburner, 2009; Han et al., 2021). The total intracranial volume (TIV) and image quality rating (IQR) were calculated (Brown et al., 2019; Han et al., 2022a,b, 2023).
Construction of individualized structural covariance network
Following previous studies (Das et al., 2018; Han et al., 2022a,b), an individualized structural covariance network was constructed using the following steps. First, a group-level N × N (N, the number of brain regions) structural covariance network was constructed (SCN) for smokers. Specifically, mean GMV values of brain regions defined in the automated anatomical labeling atlas (AAL) were extracted, and pairwise Pearson's correlations between them were calculated. Thus, a group-level 116 × 116 SCN was obtained. Second, for each subject, individualized SCN was derived via a jackknife-bias estimation procedure that determined an individual's contribution to the group-level SCN (Miller, 1974; Das et al., 2018).
Connectome-based predictive modeling
We constructed a CPM to predict FTND scores from individualized SCN in smokers. In brief, in the training dataset, structural covariance edges and FTND scores were correlated using Pearson's correlation. The whole network was divided into positive and negative predictive networks according to the sign of significant Pearson's correlation coefficients (p < 0.01). Then, the sum of significant edges in each network was obtained and entered into a linear regression model to establish the linear relationship between the edges and FTND scores. The resultant polynomial coefficients including slope and intercept were applied to the test dataset to predict FTND scores. In this study, leave-one-out cross-validation (LOOCV) was adopted. The performance of CPM was assessed by calculating Pearson's correlation between the predicted FTND scores and true ones.
To assess whether the performance of CPM (Pearson's correlation coefficients between the predicted FTND scores and true ones) was significantly higher than chance, a permutation testing was performed (5,000 times). In each run, FTND scores and connections were randomly shuffled, and CPM was rerun with the shuffled data. The significance was defined as the proportion of sampled permutations that were greater or equal to the true prediction correlation (Shen et al., 2017).
Sensitivity analysis
We also explored whether our results were biased by confounding factors including TIV or IQR. To this end, Pearson's correlation coefficients between predicted FTND scores and TIV or IQR were calculated.
Results
Demographics and clinical characteristics
Demographics and clinical characteristics are included in Table 1. There were no significant differences in terms of age, education level, and IQR between smokers and non-smokers.
Performance of CPM
Combining positive and negative structural covariance networks, CPM significantly predicted the FTND scores (r = 0.23, permutation p = 0.020, Figure 1A). We further investigated whether a negative or positive network alone could predict the FTND scores. The results showed that a negative network could significantly predict FTND scores (r = 0.19, permutation p = 0.036, Figure 1B) while a positive network could not (permutation p > 0.05).
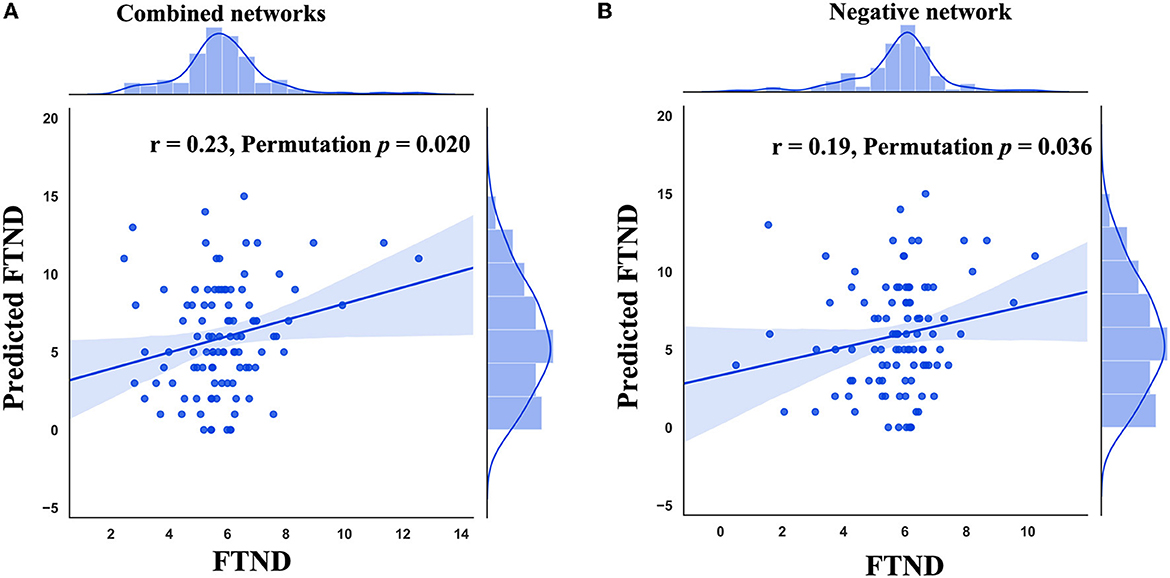
Figure 1. Performance of connectome-based predictive modeling (CPM). (A) The correlation between predicted FTND scores using combined networks and true ones. (B) The correlation between predicted FTND scores using the negative network and true ones. FTND, Fagerström Test for Nicotine Dependence.
Smoking severity-related network anatomy
The identified positive and negative networks are presented in Figure 2. The positive network included 95 edges, and the negative network included 103 edges. The node size in Figure 2 was proportional to the degree. Highest-degree nodes in the positive network were mainly located in the cerebellum, thalamus, hippocampus, and superior occipital gyrus, and those in the negative network were mainly located in the striatum, hippocampus, cingulate gyrus, and orbitofrontal cortex. The top 10 nodes with the highest degree in the positive and negative networks are listed in Table 2.
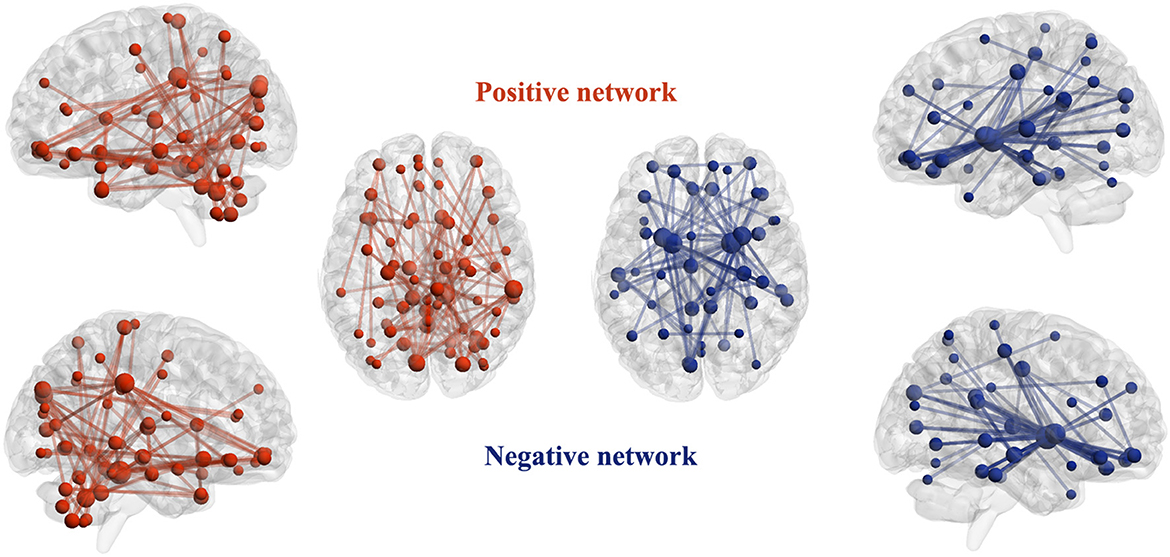
Figure 2. Identified positive and negative networks. For the positive network, increased structural covariance edges predict more smoking severity measured with Fagerström Test for Nicotine Dependence scores. For the negative network, decreased structural covariance edges predict more smoking severity measured with Fagerström Test for Nicotine Dependence scores. The size of nodes is proportional to the number of edges attached to them (degree).
Next, the edges in positive and negative networks were summarized into within and between canonical neural networks as defined in previous studies (Finn et al., 2015; Han et al., 2022a,b). The number of edges between and within the networks are presented in Figure 3. The edges in the positive network were mainly distributed within the subcortical–cerebellum network between the subcortical–cerebellum network and the visual network. The edges in the negative network were mainly located between the subcortical–cerebellum network and those including the frontoparietal network, default model network (DMN), and motor and visual network.
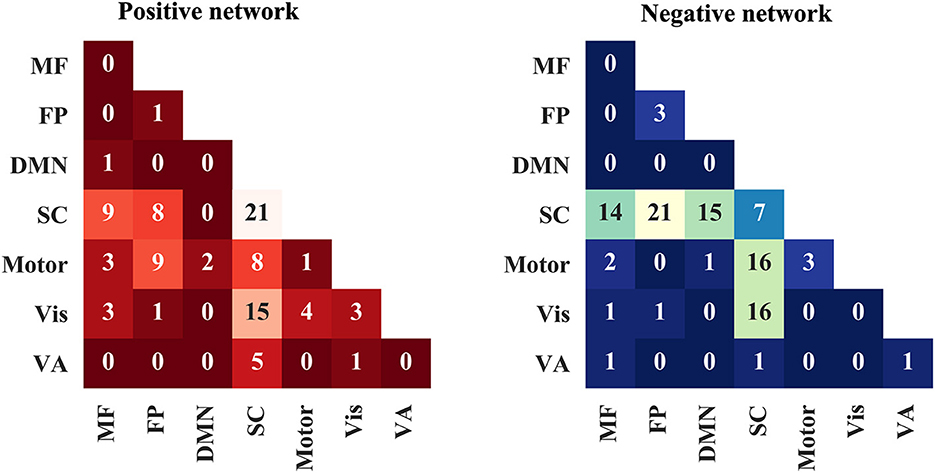
Figure 3. Positive and negative networks are summarized into within and between canonical neural networks. MF, medial frontal network; FP, frontoparietal network; DMN, default model network; SC, subcortical-cerebellum network; VA, visual association network.
Sensitivity analysis
We did not observe any significant correlation between factors including TIV and IQR and predicted FTND scores (all p-values > 0.05), excluding their effects on the performance of CPM.
Discussion
In this study, we successfully predicted smoking severity from the individualized structural covariance network using CPM and identified smoking severity-related networks. The combined and negative network could significantly predict the FTND scores, suggesting their close association with smoking severity. The identified negative network mainly comprised of structural covariance edges between the subcortical–cerebellum network and the networks including frontoparietal and motor and visual. In this network, the putamen, pallidun, hippocampus, cingulate gyrus, and orbitofrontal cortex had the highest degree reflecting their vital roles in this network. These results elucidate the neuronal substrates of smoking severity establishing the connection between structural covariance network and smoking severity for the first time.
Our results reveal that individualized structural covariance across multiple brain networks is predictive of smoking severity. With CPM, previous studies have also identified severity-related functional networks, such as nicotine addiction, cocaine, Internet gaming disorder, and opioid use disorder (Yip et al., 2019; Lichenstein et al., 2021; Lin et al., 2022; Zhou et al., 2022). As a state feature, functional connectivity may oscillate across different states and is susceptible to many confounding factors (Evans, 2013; Gorgolewski et al., 2013). In this study, we identified severity-related structural covariance networks in smokers. Compared with functional connectivity, structural covariance measures brain connectivity on a larger time scale and is hypothesized to represent trait-like connectivity features (Subirà et al., 2016). In spite of substantial concordance between functional connectivity and structural covariance, they are different and complementary metrics of brain connectivity (Subirà et al., 2016). However, to the best of our knowledge, there are only two studies investigating altered structural covariance in addiction-related disorders, namely Internet gaming disorder and alcohol dependence (Chen et al., 2021; Ottino-González and Garavan, 2022). The former elucidates the association between decreased structural covariance connections within DMN and addiction severity in Internet gaming disorder (Chen et al., 2021). The latter investigates altered graph-theoretic metrics of the structural covariance network in adults with alcohol dependence and heavy-drinking adolescents and suggests that the structural covariance network profile can be an early marker of alcohol dependence in adults (Ottino-González and Garavan, 2022). However, both of them investigate structural covariance network aberrance at the group level. With the help of a newly proposed method, for the first time, we investigated the association between structural covariance network and smoking severity at the individual level. We found that the combined and negative networks could significantly predict the FTND score while the positive network could not. These results are consistent with the study by Lin et al. (2022) investigating functional connectivity networks underpinning smoking severity. These results suggest that smoking severity is associated with impaired coordination among brain networks.
Our results revealed that decreased structural covariance connections between the subcortical–cerebellum network and other networks played vital roles in the mechanisms of nicotine addiction. The subcortical–cerebellum network defined in this study contains regions, such as the hippocampus, amygdala, striatum, thalamus, orbitofrontal gyrus, anterior insula, and cerebellum (Finn et al., 2015). Among these brain regions, the pallidum, putamen, hippocampus, and orbitofrontal gyrus had the highest degree indicating their important roles in the identified negative network. All these regions are implicated in the brain reward system that is consistently hypothesized to underlay the mechanism of addiction (Hyman et al., 2006; Haber and Knutson, 2010). For example, the putamen plays a vital role in the development of nicotine addiction due to the high concentration of nicotinic acetylcholine receptors which makes it a potential target for nicotine (Das et al., 2012). In the putamen, hyperactivity, when exposed to environmental cues triggering craving and gray matter volume abnormalities, is reported in smokers compared to non-smokers (Das et al., 2012; Pan et al., 2013; Franklin et al., 2014). In addition, gray matter volume is significantly correlated with pack years, suggesting it is a potential biomarker of the cumulative effect of smoking (Bu et al., 2016). In the hippocampus, preclinical studies consistently suggest that its function is enhanced by initial exposure to drugs, and the synaptic alterations by stimulants can facilitate the learning of drug-associated memories and eventual addiction to the drug (Avchalumov and Mandyam, 2021). Recent evidence indicates the involvement of the cerebellum in addictive behavior (Kühn et al., 2012). Tissue volume loss in the cerebellum is reported in smokers (Kühn et al., 2012), and the decreased connections between the cerebellum and DMN are also reported in smokers relative to non-smokers (Wetherill et al., 2015; Shen et al., 2018). In the cerebellum, repeated drug exposure enhances the susceptibility of its connections with the frontal gyrus that is related to impaired executive control of the prefrontal cortex on drug-seeking behavior (Miquel et al., 2009). In accordance with these findings, our results further underscore the vital role of the subcortical–cerebellum network in the smoking severity.
Other networks including frontoparietal, DMN, and motor and visual networks are also implicated in the mechanisms of addiction severity. The frontoparietal network is implicated in representing and maintaining goals during motivated behavior (Koechlin and Hyafil, 2007; Badre, 2008) and has a prominent role in inhibitory control of impulsive responses (Jentsch and Taylor, 1999). People with substance dependence and behavioral addictions show hypoactivation during motor response inhibition tasks and cognitive self-regulation (Luijten et al., 2014). In addition, its weak connections with the striatal system during a response inhibition task are associated with greater dependence severity in alcoholics (Courtney et al., 2013). The dysfunction of the frontoparietal network contributes to the transition from voluntary/goal to a more habitual drug-seeking behavior (Everitt and Robbins, 2005). The dysfunction of DMN and the abnormal interactions between DMN and other networks are hypothesized to contribute to craving and relapse in substance abuse (Zhang and Volkow, 2019). For example, as a central component of the valuation system of the brain, the medial prefrontal cortex is involved in the dysregulation of the reward and motivation circuits in addiction (Volkow et al., 2003; Bartra et al., 2013). The weaker connectivity between the putamen and Medial prefrontal cortex during an inhibition task is associated with greater alcohol dependence severity (Courtney et al., 2013). Altered connections between the midline core DMN and subcortical regions, such as the amygdala and the striatum, are responsible for the cognitive, emotional, and reward-related dysregulation in substance abuse (Koob and Volkow, 2016). In addition, connections between the subcortical–cerebellum network and the motor and visual networks are also found to be associated with smoking severity. Exposure to nicotine/cannabis can result in long-lasting dysregulation of somatosensory processing and less motor cortical plasticity (Smolka et al., 2006). In addition, previous studies have revealed that the activations of motor- and vision-related brain regions in response-related cues are correlated with smoking severity (Yalachkov et al., 2009) and are predictive of relapse (Kosten et al., 2006). In accordance with these findings, our results suggest that decreased connections between the subcortical–cerebellum network and these other networks underlay the neural underpinnings of smoking severity.
Several limitations in this study should be mentioned. First, our findings are drawn from one dataset with a moderate sample size, and whether these findings can be reproduced in other independent datasets with a larger sample size needs to be assessed. Second, only male smokers are included in this study, and future studies could investigate whether our findings hold true in female smokers. Third, we investigated the smoking severity-related structural covariance network using cross-sectional data, and whether and how the identified networks progress with increased severity need to be elucidated using longitudinal data in future.
Data availability statement
The raw data supporting the conclusions of this article will be made available by the authors, without undue reservation.
Ethics statement
The studies involving human participants were reviewed and approved by the Research Ethics Committee of the First Affiliated Hospital of the Zhengzhou University. The patients/participants provided their written informed consent to participate in this study.
Author contributions
Study concept and design: WW and YZ. Data acquisition: YK, XN, and ZZ. Data analysis and interpretation of data: WW, SL, and YK. Drafting of the initial manuscript: WW, YK, MZ, XG, JC, and YZ. All authors contributed to the article and approved the submitted version.
Funding
This study was supported by grants from the Henan Province Key Research and Development and Promotion of Special Projects (Scientific and Technological Research, 212102310699), the Joint Construction Project of Health Commission of Henan Province (LHGJ20200384), the Science and Technology Project of Henan Province (172102310391), the Key Research and Development and Promotion (Science and Technology) Support Project (212102310712), and the Scientific Research and Innovation Team of the First Affiliated Hospital of Zhengzhou University (QNCXTD2023007).
Conflict of interest
The authors declare that the research was conducted in the absence of any commercial or financial relationships that could be construed as a potential conflict of interest.
Publisher's note
All claims expressed in this article are solely those of the authors and do not necessarily represent those of their affiliated organizations, or those of the publisher, the editors and the reviewers. Any product that may be evaluated in this article, or claim that may be made by its manufacturer, is not guaranteed or endorsed by the publisher.
References
Alexander-Bloch, A., Giedd, J. N., and Bullmore, E. (2013). Imaging structural co-variance between human brain regions. Nat. Rev. Neurosci. 14, 322–336. doi: 10.1038/nrn3465
Ashburner, J. (2009). Computational anatomy with the SPM software. Magn. Reson. Imaging 27, 1163–1174. doi: 10.1016/j.mri.2009.01.006
Ashburner, J., and Friston, K. J. (2000). Voxel-based morphometry–the methods. Neuroimage 11, 805–821. doi: 10.1006/nimg.2000.0582
Avchalumov, Y., and Mandyam, C. D. (2021). Plasticity in the hippocampus, neurogenesis and drugs of abuse. Brain Sci. 11, 404. doi: 10.3390/brainsci11030404
Badre, D. (2008). Cognitive control, hierarchy, the rostro-caudal organization of the frontal lobes. Trends Cogn. Sci. 12, 193–200. doi: 10.1016/j.tics.2008.02.004
Barrett, S. P., Boileau, I., Okker, J., Pihl, R. O., and Dagher, A. (2004). The hedonic response to cigarette smoking is proportional to dopamine release in the human striatum as measured by positron emission tomography and [11C]raclopride. Synapse 54, 65–71. doi: 10.1002/syn.20066
Bartra, O., McGuire, J. T., and Kable, J. W. (2013). The valuation system: a coordinate-based meta-analysis of BOLD fMRI experiments examining neural correlates of subjective value. Neuroimage 76, 412–427. doi: 10.1016/j.neuroimage.2013.02.063
Biswal, B., Yetkin, F. Z., Haughton, V. M., and Hyde, J. S. (1995). Functional connectivity in the motor cortex of resting human brain using echo-planar MRI. Magn. Reson. Med. 34, 537–541. doi: 10.1002/mrm.1910340409
Branstetter, S. A., Horton, W. J., Mercincavage, M., and Buxton, O. M. (2016). Severity of nicotine addiction and disruptions in sleep mediated by early awakenings. Nicotine Tob. Res. 18, 2252–2259. doi: 10.1093/ntr/ntw179
Brown, J. A., Deng, J., Neuhaus, J., Sible, I. J., Sias, A. C., Lee, S. E., et al. (2019). Patient-tailored, connectivity-based forecasts of spreading brain atrophy. Neuron, 104, 856–868.e5. doi: 10.1016/j.neuron.2019.08.037
Bu, L., Yu, D., Su, S., Ma, Y., von Deneen, K. M., Luo, L., et al. (2016). Functional connectivity abnormalities of brain regions with structural deficits in young adult male smokers. Front. Hum. Neurosci. 10, 494. doi: 10.3389/fnhum.2016.00494
Chen, S., Wang, M., Dong, H., Wang, L., Jiang, Y., Hou, X., et al. (2021). Internet gaming disorder impacts gray matter structural covariance organization in the default mode network. J. Affect. Disord. 288, 23–30. doi: 10.1016/j.jad.2021.03.077
Courtney, K. E., Ghahremani, D. G., and Ray, L. A. (2013). Fronto-striatal functional connectivity during response inhibition in alcohol dependence. Addict. Biol. 18, 593–604. doi: 10.1111/adb.12013
Das, D., Cherbuin, N., Anstey, K. J., Sachdev, P. S., and Easteal, S. (2012). Lifetime cigarette smoking is associated with striatal volume measures. Addict. Biol. 17, 817–825. doi: 10.1111/j.1369-1600.2010.00301.x
Das, T., Borgwardt, S., Hauke, D. J., Harrisberger, F., Lang, U. E., Riecher-Rössler, A., et al. (2018). Disorganized gyrification network properties during the transition to psychosis. JAMA Psychiatry 75, 613–622. doi: 10.1001/jamapsychiatry.2018.0391
Evans, A. C. (2013). Networks of anatomical covariance. Neuroimage 80, 489–504. doi: 10.1016/j.neuroimage.2013.05.054
Everitt, B. J., and Robbins, T. W. (2005). Neural systems of reinforcement for drug addiction: from actions to habits to compulsion. Nat. Neurosci. 8, 1481–1489. doi: 10.1038/nn1579
Fedota, J. R., and Stein, E. A. (2015). Resting-state functional connectivity and nicotine addiction: prospects for biomarker development. Ann. N Y Acad. Sci. 1349, 64–82. doi: 10.1111/nyas.12882
Finn, E. S., Shen, X., Scheinost, D., Rosenberg, M. D., Huang, J., Chun, M. M., et al. (2015). Functional connectome fingerprinting: identifying individuals using patterns of brain connectivity. Nat. Neurosci. 18, 1664–1671. doi: 10.1038/nn.4135
Franklin, T. R., Wetherill, R. R., Jagannathan, K., Johnson, B., Mumma, J., Hager, N., et al. (2014). The effects of chronic cigarette smoking on gray matter volume: influence of sex. PLoS ONE 9, e104102. doi: 10.1371/journal.pone.0104102
Gorgolewski, K. J., Storkey, A. J., Bastin, M. E., Whittle, I., and Pernet, C. (2013). Single subject fMRI test-retest reliability metrics and confounding factors. Neuroimage 69, 231–243. doi: 10.1016/j.neuroimage.2012.10.085
Haber, S. N., and Knutson, B. (2010). The reward circuit: linking primate anatomy and human imaging. Neuropsychopharmacology 35, 4–26. doi: 10.1038/npp.2009.129
Han, S., Xu, Y., Guo, H. R., Fang, K., Wei, Y., Liu, L., et al. (2022a). Resolving heterogeneity in obsessive-compulsive disorder through individualized differential structural covariance network analysis. Cereb Cortex. 33, 1659–1668. doi: 10.1093/cercor/bhac163
Han, S., Xue, K., Chen, Y., Xu, Y., Li, S., Song, X., et al. (2023). Identification of shared and distinct patterns of brain network abnormality across mental disorders through individualized structural covariance network analysis. Psychol. Med. 6, 1–12. doi: 10.1017/S0033291723000302
Han, S., Zheng, R., Li, S., Liu, L., Wang, C., Jiang, Y., et al. (2021). Progressive brain structural abnormality in depression assessed with MR imaging by using causal network analysis. Psychol. Med. 53, 2146–2155. doi: 10.1017/S0033291721003986
Han, S., Zheng, R., Li, S., Zhou, B., Jiang, Y., Fang, K., et al. (2022b). Resolving heterogeneity in depression using individualized structural covariance network analysis. Psychol. Med. 2022, 1–10. doi: 10.1017/S0033291722002380
Heatherton, T. F., Kozlowski, L. T., Frecker, R. C., and Fagerström, K. O. (1991). The fagerström test for nicotine dependence: a revision of the fagerström tolerance questionnaire. Br. J. Addict. 86, 1119–1127. doi: 10.1111/j.1360-0443.1991.tb01879.x
Hyman, S. E., Malenka, R. C., and Nestler, E. J. (2006). Neural mechanisms of addiction: the role of reward-related learning and memory. Annu. Rev. Neurosci. 29, 565–598. doi: 10.1146/annurev.neuro.29.051605.113009
Jentsch, J. D., and Taylor, J. R. (1999). Impulsivity resulting from frontostriatal dysfunction in drug abuse: implications for the control of behavior by reward-related stimuli. Psychopharmacology (Berl) 146, 373–390. doi: 10.1007/PL00005483
Koechlin, E., and Hyafil, A. (2007). Anterior prefrontal function and the limits of human decision-making. Science 318, 594–598. doi: 10.1126/science.1142995
Koob, G. F., and Volkow, N. D. (2016). Neurobiology of addiction: a neurocircuitry analysis. Lancet Psychiat. 3, 760–773. doi: 10.1016/S2215-0366(16)00104-8
Kosten, T. R., Scanley, B. E., Tucker, K. A., Oliveto, A., Prince, C., Sinha, R., et al. (2006). Cue-induced brain activity changes and relapse in cocaine-dependent patients. Neuropsychopharmacology 31, 644–650. doi: 10.1038/sj.npp.1300851
Kühn, S., Romanowski, A., Schilling, C., Mobascher, A., Warbrick, T., Winterer, G., et al. (2012). Brain grey matter deficits in smokers: focus on the cerebellum. Brain Struct. Funct. 217, 517–522. doi: 10.1007/s00429-011-0346-5
Le Foll, B., Guranda, M., Wilson, A. A., Houle, S., Rusjan, P. M., Wing, V. C., et al. (2014). Elevation of dopamine induced by cigarette smoking: novel insights from a [11C]-+-PHNO PET study in humans. Neuropsychopharmacology 39, 415–424. doi: 10.1038/npp.2013.209
Lerch, J. P., Worsley, K., Shaw, W. P., Greenstein, D. K., Lenroot, R. K., Giedd, J., et al. (2006). Mapping anatomical correlations across cerebral cortex (MACACC) using cortical thickness from MRI. Neuroimage 31, 993–1003. doi: 10.1016/j.neuroimage.2006.01.042
Lichenstein, S. D., Scheinost, D., Potenza, M. N., Carroll, K. M., and Yip, S. W. (2021). Dissociable neural substrates of opioid and cocaine use identified via connectome-based modelling. Mol. Psychiatry 26, 4383–4393. doi: 10.1038/s41380-019-0586-y
Lin, X., Zhu, X., Zhou, W., Zhang, Z., Li, P., Dong, G., et al. (2022). Connectome-based predictive modelling of smoking severity in smokers. Addict. Biol. 27, e13242. doi: 10.1111/adb.13242
Luijten, M., Machielsen, M. W., Veltman, D. J., Hester, R., de Haan, L., Franken, I. H., et al. (2014). Systematic review of ERP and fMRI studies investigating inhibitory control and error processing in people with substance dependence and behavioural addictions. J. Psychiat. Neurosci. 39, 149–169. doi: 10.1503/jpn.130052
Miquel, M., Toledo, R., García, L. I., Coria-Avila, G. A., and Manzo, J. (2009). Why should we keep the cerebellum in mind when thinking about addiction? Curr. Drug. Abuse Rev. 2, 26–40. doi: 10.2174/1874473710902010026
Mitelman, S. A., Buchsbaum, M. S., Brickman, A. M., and Shihabuddin, L. (2005). Cortical intercorrelations of frontal area volumes in schizophrenia. Neuroimage 27, 753–770. doi: 10.1016/j.neuroimage.2005.05.024
Ottino-González, J., and Garavan, H. (2022). Brain structural covariance network differences in adults with alcohol dependence and heavy-drinking adolescents. Addiction 117, 1312–1325. doi: 10.1111/add.15772
Pan, P., Shi, H., Zhong, J., Xiao, P., Shen, Y., Wu, L., et al. (2013). Chronic smoking and brain gray matter changes: evidence from meta-analysis of voxel-based morphometry studies. Neurol. Sci. 34, 813–817. doi: 10.1007/s10072-012-1256-x
Pariyadath, V., Stein, E. A., and Ross, T. J. (2014). Machine learning classification of resting state functional connectivity predicts smoking status. Front. Hum. Neurosci. 8, 425. doi: 10.3389/fnhum.2014.00425
Ren, Z., Daker, R. J., Shi, L., Sun, J., Beaty, R. E., Wu, X., et al. (2021). Connectome-based predictive modeling of creativity anxiety. Neuroimage 225, 117469. doi: 10.1016/j.neuroimage.2020.117469
Shen, X., Finn, E. S., Scheinost, D., and Rosenberg, M. D. (2017). Using connectome-based predictive modeling to predict individual behavior from brain connectivity. Nat. Protoc. 12, 506–518. doi: 10.1038/nprot.2016.178
Shen, Z., Huang, P., Wang, C., Qian, W., Yang, Y., Zhang, M., et al. (2018). Cerebellar gray matter reductions associate with decreased functional connectivity in nicotine-dependent individuals. Nicotine Tob. Res. 20, 440–447. doi: 10.1093/ntr/ntx168
Smolka, M. N., Bühler, M., Klein, S., Zimmermann, U., Mann, K., Heinz, A., et al. (2006). Severity of nicotine dependence modulates cue-induced brain activity in regions involved in motor preparation and imagery. Psychopharmacology (Berl) 184, 577–588. doi: 10.1007/s00213-005-0080-x
Subirà, M., Cano, M., de Wit, S. J., Alonso, P., Cardoner, N., Hoexter, M. Q., et al. (2016). Structural covariance of neostriatal and limbic regions in patients with obsessive-compulsive disorder. J. Psychiat. Neurosci. 41, 115–123. doi: 10.1503/jpn.150012
Volkow, N. D., Fowler, J. S., and Wang, G. J. (2003). The addicted human brain: insights from imaging studies. J. Clin. Invest. 111, 1444–1451. doi: 10.1172/JCI18533
Wang, Z., Goerlich, K. S., Ai, H., Aleman, A., Luo, Y. J., Xu, P., et al. (2021). Connectome-based predictive modeling of individual anxiety. Cereb Cortex 31, 3006–3020. doi: 10.1093/cercor/bhaa407
Weiland, B. J., Sabbineni, A., Calhoun, V. D., Welsh, R. C., and Hutchison, K. E. (2015). Reduced executive and default network functional connectivity in cigarette smokers. Hum. Brain Mapp. 36, 872–882. doi: 10.1002/hbm.22672
Wen, M., Yang, Z., Wei, Y., Huang, H., Zheng, R., Wang, W., et al. (2021). More than just statics: Temporal dynamic changes of intrinsic brain activity in cigarette smoking. Addict. Biol. 26, e13050. doi: 10.1111/adb.13050
Wetherill, R. R., Fang, Z., Jagannathan, K., Childress, A. R., Rao, H., Franklin, T. R., et al. (2015). Cannabis, cigarettes, and their co-occurring use: Disentangling differences in default mode network functional connectivity. Drug. Alcohol. Depend 153, 116–123. doi: 10.1016/j.drugalcdep.2015.05.046
Xue, K., Guo, L., Zhu, W., Liang, S., Xu, Q., Ma, L., et al. (2023). Transcriptional signatures of the cortical morphometric similarity network gradient in first-episode, treatment-naive major depressive disorder. Neuropsychopharmacology 48, 518–528. doi: 10.1038/s41386-022-01474-3
Yalachkov, Y., Kaiser, J., and Naumer, M. J. (2009). Brain regions related to tool use and action knowledge reflect nicotine dependence. J. Neurosci. 29, 4922–4929. doi: 10.1523/JNEUROSCI.4891-08.2009
Yip, S. W., Scheinost, D., Potenza, M. N., and Carroll, K. M. (2019). Connectome-based prediction of cocaine abstinence. Am. J. Psychiatry 176, 156–164. doi: 10.1176/appi.ajp.2018.17101147
Yuan, K., Yu, D., Bi, Y., Li, Y., Guan, Y., Liu, J., et al. (2016). The implication of frontostriatal circuits in young smokers: A resting-state study. Hum. Brain Mapp. 37, 2013–2026. doi: 10.1002/hbm.23153
Yun, J. Y., Jang, J. H., Kim, S. N., Jung, W. H., and Kwon, J. S. (2015). Neural correlates of response to pharmacotherapy in obsessive-compulsive disorder: individualized cortical morphology-based structural covariance. Prog. Neuropsychopharmacol. Biol. Psychiat. 63, 126–133. doi: 10.1016/j.pnpbp.2015.06.009
Zhang, R., and Volkow, N. D. (2019). Brain default-mode network dysfunction in addiction. Neuroimage 200, 313–331. doi: 10.1016/j.neuroimage.2019.06.036
Keywords: connectome-based predictive modeling, structural covariance, nicotine addiction, smoking severity, machine learning
Citation: Wang W, Kang Y, Niu X, Zhang Z, Li S, Gao X, Zhang M, Cheng J and Zhang Y (2023) Connectome-based predictive modeling of smoking severity using individualized structural covariance network in smokers. Front. Neurosci. 17:1227422. doi: 10.3389/fnins.2023.1227422
Received: 23 May 2023; Accepted: 04 July 2023;
Published: 21 July 2023.
Edited by:
Feng Liu, Tianjin Medical University General Hospital, ChinaReviewed by:
Yang Yang, Anhui Normal University, ChinaZongya Zhao, Xinxiang Medical University, China
Copyright © 2023 Wang, Kang, Niu, Zhang, Li, Gao, Zhang, Cheng and Zhang. This is an open-access article distributed under the terms of the Creative Commons Attribution License (CC BY). The use, distribution or reproduction in other forums is permitted, provided the original author(s) and the copyright owner(s) are credited and that the original publication in this journal is cited, in accordance with accepted academic practice. No use, distribution or reproduction is permitted which does not comply with these terms.
*Correspondence: Yong Zhang, enp1emhhbmd5b25nMjAxMyYjeDAwMDQwOzE2My5jb20=