- 1College of Medical Technology and Engineering, Henan University of Science and Technology, Luoyang, China
- 2Business School, The University of Sydney, Sydney, NSW, Australia
- 3College of Agricultural Equipment Engineering, Henan University of Science and Technology, Luoyang, China
- 4School of Information Engineering, Henan University of Science and Technology, Luoyang, China
White matter disconnection is the primary cause of cognition and affection abnormality in mild cognitive impairment (MCI). Adequate understanding of behavioral disturbances, such as cognition and affection abnormality in MCI, can help to intervene and slow down the progression of Alzheimer’s disease (AD) promptly. Diffusion MRI is a non-invasive and effective technique for studying white matter microstructure. This review searched the relevant papers published from 2010 to 2022. Sixty-nine studies using diffusion MRI for white matter disconnections associated with behavioral disturbances in MCI were screened. Fibers connected to the hippocampus and temporal lobe were associated with cognition decline in MCI. Fibers connected to the thalamus were associated with both cognition and affection abnormality. This review summarized the correspondence between white matter disconnections and behavioral disturbances such as cognition and affection, which provides a theoretical basis for the future diagnosis and treatment of AD.
Introduction
The significance of research for behavioral disturbances in MCI
Mild cognitive impairment (MCI) is the prodromal stage of Alzheimer’s disease (AD) (Petersen et al., 1999; Gauthier et al., 2006). As the progression of AD showed in Figure 1, the neuronal destruction in the AD stage is so extensive for the whole brain that it is difficult to reverse. Many treatments are only effective for MCI with subtle changes in neural structure.
Moving the development window for biomarkers forward to the MCI stage can halt or slow AD progression (Wang et al., 2013). Therefore, the MCI stage is the best opportunity for intervention in AD timely.
Mild cognitive impairment often presents with behavioral disturbances, mainly memory loss, reduced attention and executive functions, disorientation, and impaired language skills, collectively referring to cognition decline (Catani et al., 2013; Arvanitakis et al., 2019). In addition, MCI often have other affective symptoms, including depression, anxiety, and apathy (Catani et al., 2012). These affective symptoms may exacerbate the transition from MCI to AD. Therefore, fully understanding the behavioral disturbances in MCI can help promptly intervene and delay AD development (Pantel et al., 2016).
White matter disconnections caused behavioral disturbances in MCI
The behavioral disturbances in MCI are mainly caused by the disconnection of neuronal pathways in the brain due to white matter degeneration (Alves et al., 2017; Yu et al., 2021). During the development of AD, the lesioned areas propagate from the lower to the higher cortex according to specific white matter pathways (Gainotti et al., 2014; Miller et al., 2016). During the MCI stage, selective degeneration in fibers is mainly in the limbic system (Mito et al., 2018). In the late AD stage, fibers gradually spread from the limbic system to the higher cortices, such as frontal, temporal and parietal, for extensive degeneration throughout the brain (Pini et al., 2016; Zimmermann et al., 2018).
White matter connections between the hippocampus, precuneus and posterior cingulate cortex form the memory network. The Papez circuit formed between the hippocampus and thalamus has also been shown to be related to working memory (Li K. et al., 2020). The arcuate fasciculus, which connects the frontal Broca’s area to the temporal Veronica area, is associated with language ability (Friederici and Gierhan, 2013). The inferior longitudinal fasciculus and the inferior frontal-occipital fasciculus, which runs through the temporal lobe and reaches the occipital lobe, are associated with visuospatial ability (Urbanski et al., 2008). Damage to the white matter of these fibers in MCI leads to cognitive impairment.
Besides memory deficits, MCI has executive function deficits such as attention and information processing speed (Saunders and Summers, 2011). As a relay station for transmitting information from subordinate neurons to the cerebral cortex, the thalamus has extensive white matter fiber connections from the subcortical nuclei to the cerebral cortex (Abivardi and Bach, 2017; Zheng et al., 2019). In addition to the Papez circuit with the hippocampus and other subcortical nuclei in the limbic systems, the thalamus is responsible for memory processing (Bubb et al., 2017). The thalamus is also connected to the frontal and parietal cortex via projection fibers (Gerstenecker et al., 2017), which regulate cognition and affection (Gu and Zhang, 2019).
In addition, there are overlaps and interactions on some neural pathways between brain networks related to cognition and affection in MCI (Tan et al., 2019), which may be essential in converting MCI to AD (Barca et al., 2017; Sui et al., 2020). However, current research has focused on the relationship between white matter damage and cognition decline in MCI. But it is unclear which fibers are associated with affection symptoms in MCI. The comorbid pathways of brain networks related to cognition and affection in MCI are indistinct. Therefore, it is necessary for relevant studies to organize and summarize the relationship between white matter disconnections and behavioral disturbances in MCI.
Parameters of diffusion MRI could explain white matter disconnections effetely
Due to the sensitivity of the diffusion MRI signals for the moving of water molecules, it can effectively probe tissue microstructures. The water molecules’ movement is restricted and obstructed by the fiber structure of neuronal axons. So the motion trail of the water molecules can be used to infer intra-voxel fiber orientation and outline the path of white matter by using appropriate fiber tracking algorithms. Diffusion tensor imaging (DTI) focuses on obtaining reliable indicators of key microstructural parameters. The fractional anisotropy (FA) is quantitatively described using the proportion of diffusion anisotropy included in the diffusion tensor, reflecting the integrity of the protein fibers’ myelin sheath and density (Richards et al., 1992). The axial diffusivity (DA) represents the diffusion rate of water molecules along the central axis and is usually used to reflect the degeneration of axons (Alexander et al., 2007). Radial diffusivity (DR) could reflect the permeability of water molecules along the radial direction (Song et al., 2002). Mean diffusivity (MD) indicates the average diffusivity of water molecules in brain tissue (Le et al., 2001). Based on DTI, diffusion kurtosis imaging (DKI), diffusion spectrum imaging (DSI) and neurite orientation dispersion and density imaging (NODDI) have been developed (Pasternak et al., 2018). The advanced diffusion MRI techniques were showed in Figure 2. The parameters of diffusion imaging can be used to quantitatively characterize the degeneration of white matter and further analyze the relationship between the white matter disconnection and behavioral disturbances such as cognitive and emotional disorders in MCI.
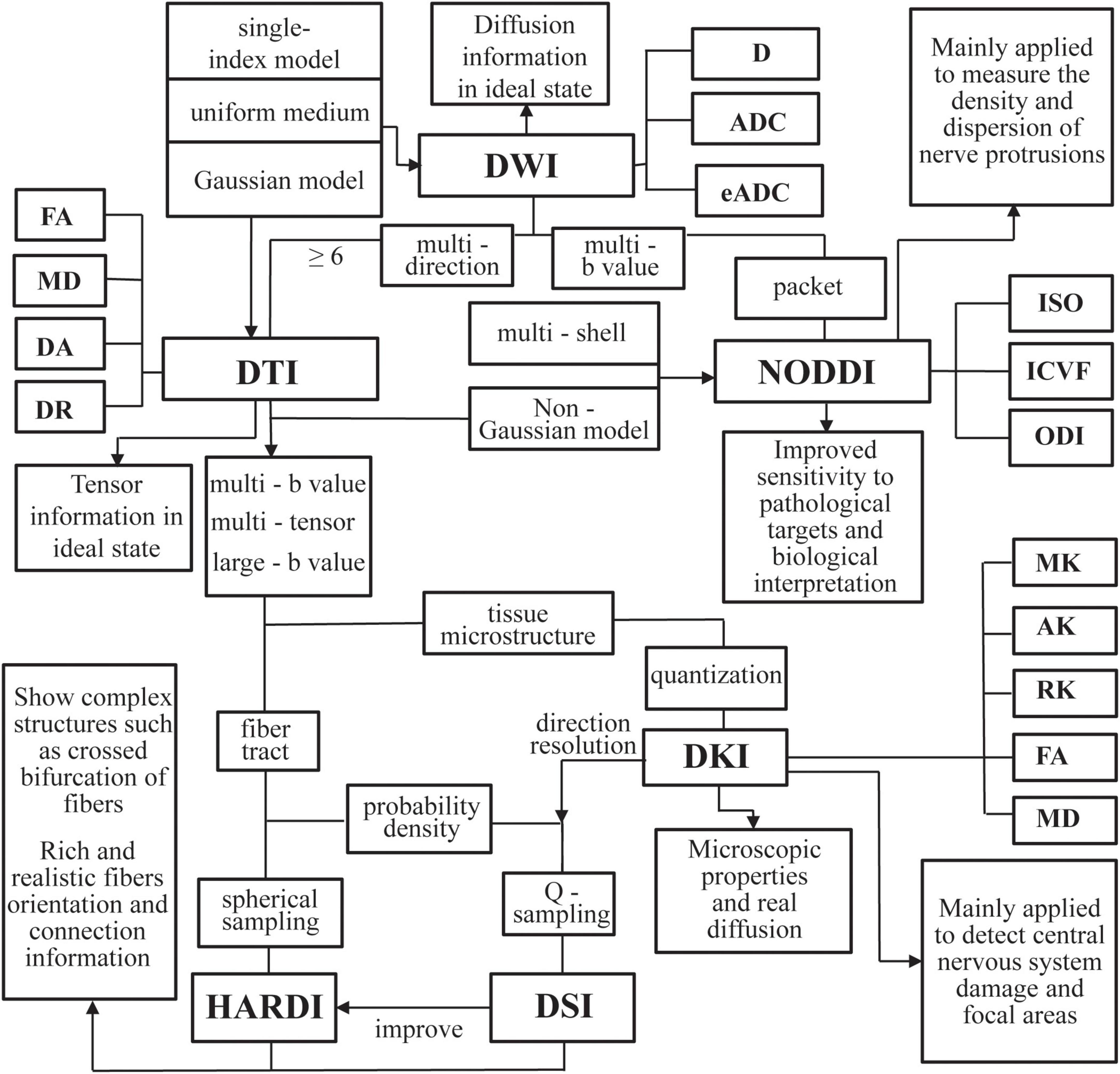
Figure 2. An overview of the advanced diffusion magnetic resonance imaging (MRI) techniques examined in this article. DWI, diffusion weighted imaging; D, diffusion; ADC, apparent diffusion coefficient; eADC, exponential ADC; DTI, diffusion tensor imaging; FA, fractional anisotropy; DA, axial diffusivity; DR, radial diffusivity; MD, mean diffusivity; DSI, diffusion spectrum imaging; HARDI, high angular diffusion magnetic imaging; DKI, diffusion kurtosis imaging; MK, mean kurtosis; AK, axial kurtosis; RK, radial kurtosis; NODDI, neurite orientation dispersion and density imaging; ODI, orientation dispersion index; ICVF, intracellular volume fraction; ISO, isotropic volume fraction.
Contribution of this article
Despite the outstanding achievements of neuroimaging methods for white matter, it remains unclear which fibers’ degeneration causes cognition and affection abnormalities, and whether these white matter disconnections are associated with different patterns and severity of behavioral disturbances. For this purpose, our review combined with evidence of white matter disconnections and behavioral disturbances in MCI, provided more insight by integrating and analyzing all studies of white matter from diffusion MRI methods.
The current work aims to review diffusion MRI findings of behavioral disturbances in MCI, focusing on the relationship between diffusion parameters of white matter and behavioral scores. We acknowledge that the number of studies conducted to date is not significant. For the second purpose, our review critically discussed the comorbid pathways related to cognition and affection according to the neurobiological mechanisms in MCI.
In summary, this review provided new development in how diffusion imaging methods have been used for cognition and affection symptoms in MCI. The potential role of neuroimaging evidence was highlighted for the early diagnosis of AD. The basis could be provided for the targeted treatment of specific fiber tracts.
Methods
The present research review followed the Problem Intervention Comparison Outcomes (PICO) search strategy based on the brain mechanisms of white matter disconnections related to behavioral disturbances in MCI. Diffusion imaging characteristics of white matter in MCI were summarized and compared with healthy controls. The relationship between different brain regions’ white matter disconnections and behavioral disturbances was discussed.
In this review, PubMed and Web of Science databases were systematically searched for relevant literature from 2010 to 2022. Three sets of keywords were used for the literature search: (i) mild cognitive impairment; (ii) white matter; (iii) behavior. Keywords included in the title or abstract of the paper are also included in this review. In addition to the systematic electronic database search, a targeted search of the bibliographies of relevant articles was conducted to identify any additional papers to be included.
Only original articles published in English between January 2010 and December 2022 were considered. All articles investigated the relationship between white matter disconnections and behavioral disturbances in MCI through diffusion MRI methods. Articles were excluded if they: (i) did not use diffusion MRI to investigate the white matter; (ii) studied other disorders such as cerebrovascular disease, sclerosis, hypertension, cerebral infarction, stroke, Parkinson’s, Lewy body dementia, Down syndrome, and schizophrenia; (iii) were review articles.
Results
Search results
Using the search method mentioned above, a total of 479 articles were retrieved from the Web of Science database, 179 from the PubMed database, and five from other databases. After the initial screening, duplicate and irrelevant papers were removed. 330 articles were excluded according to the exclusion criteria. 91 articles investigating the white matter without diffusion MRI were excluded based on case (i); 223 articles (55 in cerebrovascular disease, three in sclerosis, three in hypertension, nine in cerebral infarction, 69 in stroke, 70 in Parkinson’s, 10 in Lewy body dementia, three in Down syndrome, one in schizophrenia) were excluded based on case (ii), and 16 review articles were excluded based on case (iii). Finally, 69 articles were selected for this review. The preferred reporting items for systematic reviews and meta-analyses (PRISMA) diagram in Figure 3 illustrates the screening and inclusion process.
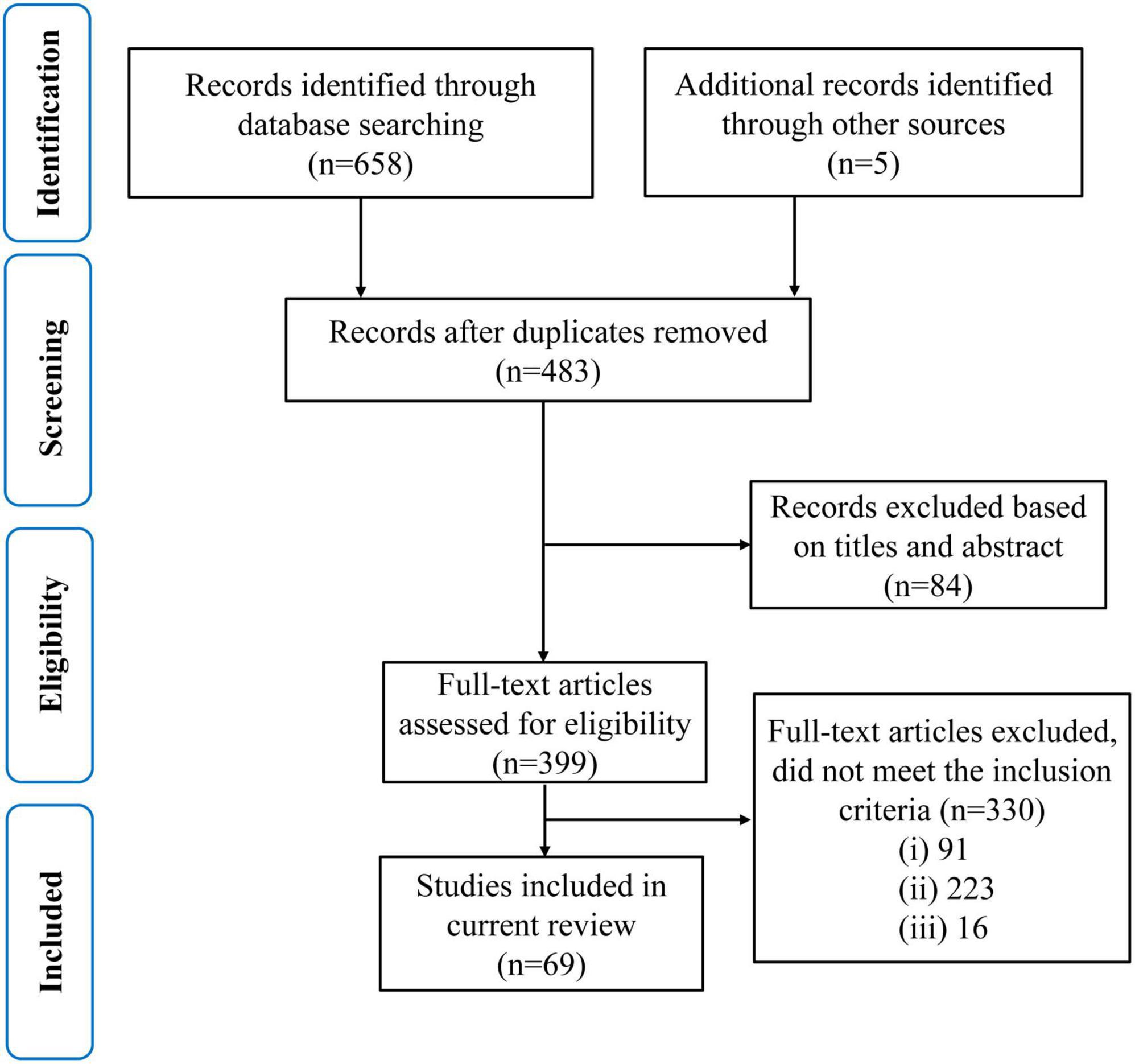
Figure 3. A modified preferred reporting items for systematic reviews and meta-analyses (PRISMA) flow diagram through the selection study.
Behavior testing scales
The different behaviors of the subjects were divided into cognition testing scales as well as affection testing scales. The scales used to test various cognition for MCI are summarized in Table 1, including memory, language, visual space, attention and execution. Table 2 summarizes the scales used to test different affections in MCI, including depression, anxiety, and apathy.
Assessment of white matter disconnection and behavioral disturbances
The fibers were divided into four groups according to their location and function. The four groups were limbic, projection, association, and commissural fibers (Table 3). Research on the correlation between cognition testing scale scores and diffusion parameters of limbic, projection, association, and commissural fibers in MCI were summarized in Tables 4–7, respectively. Research on the correlation between affection testing scale scores and diffusion parameters of limbic and projection fibers were summarized in Tables 8, 9. The following patterns can be found in the tables. Firstly, the limbic, association and commissural fibers were mainly related to cognition. Secondly, the limbic and projection fibers were primarily related to affection. Finally, the limbic fibers were associated with both cognition and affection.
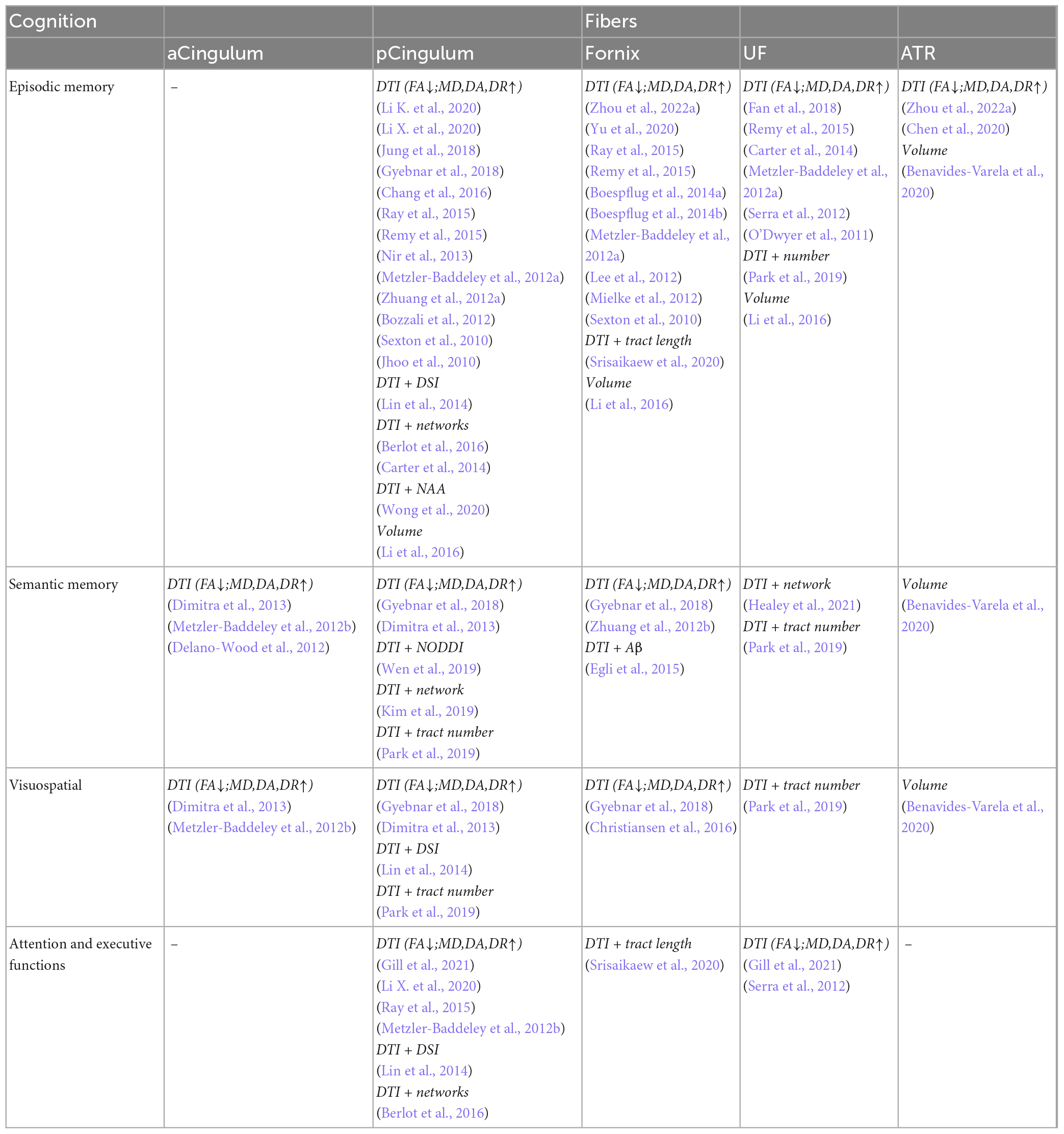
Table 4. Correlation analysis between white matter parameters and cognition scales in limbic fibers.

Table 5. Correlation analysis between white matter parameters and cognition scales in projection fibers.
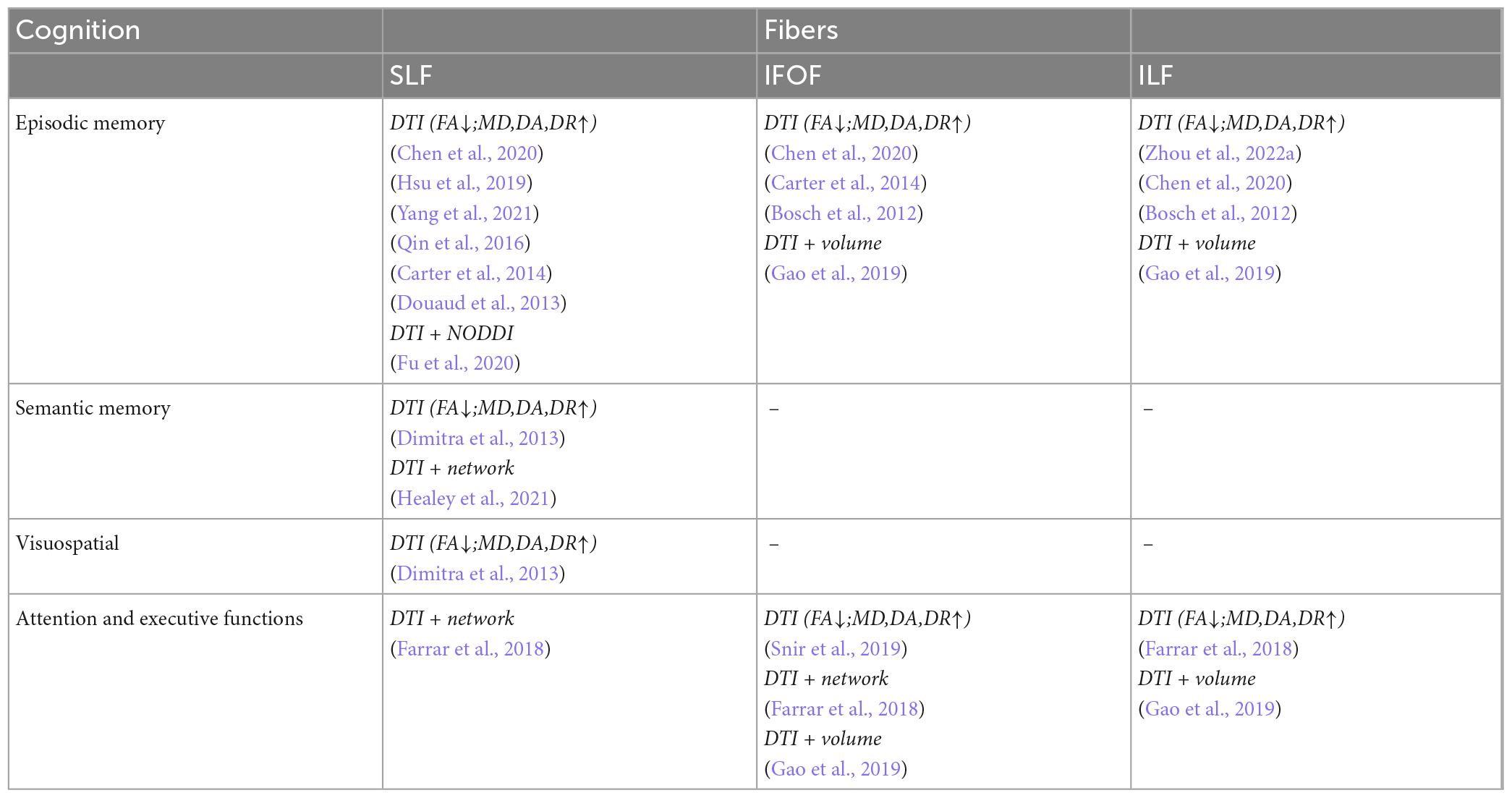
Table 6. Correlation analysis between white matter parameters and cognition scales in association fibers.
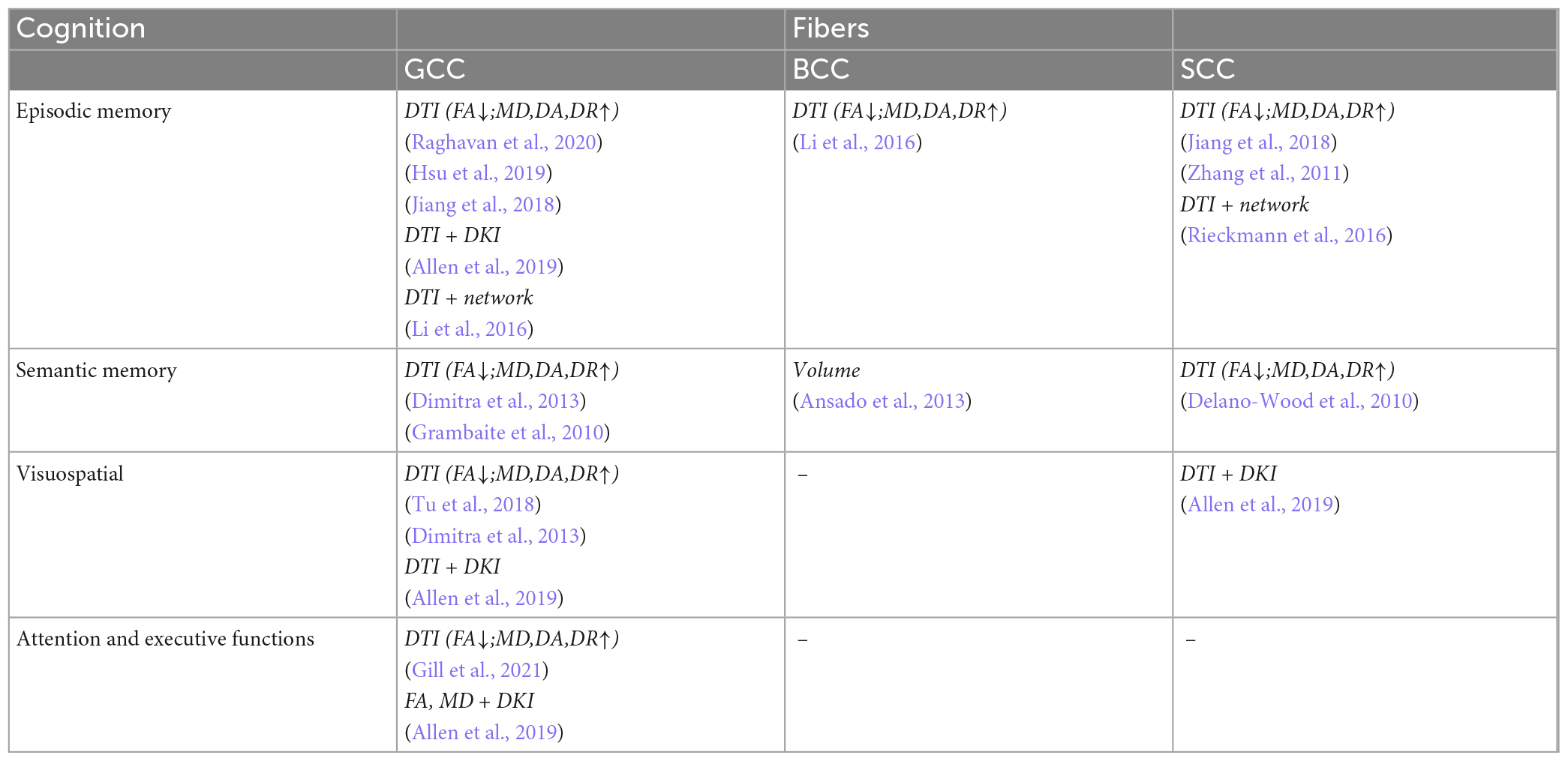
Table 7. Correlation analysis between white matter parameters and cognition scales in commissural fibers.
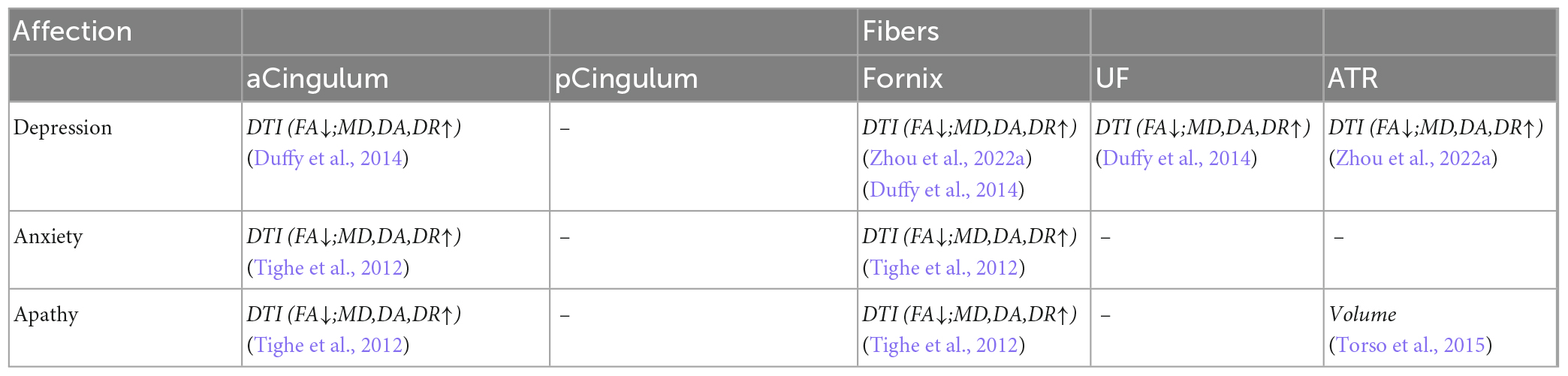
Table 8. Correlation analysis between white matter parameters and affection scales in limbic fibers.
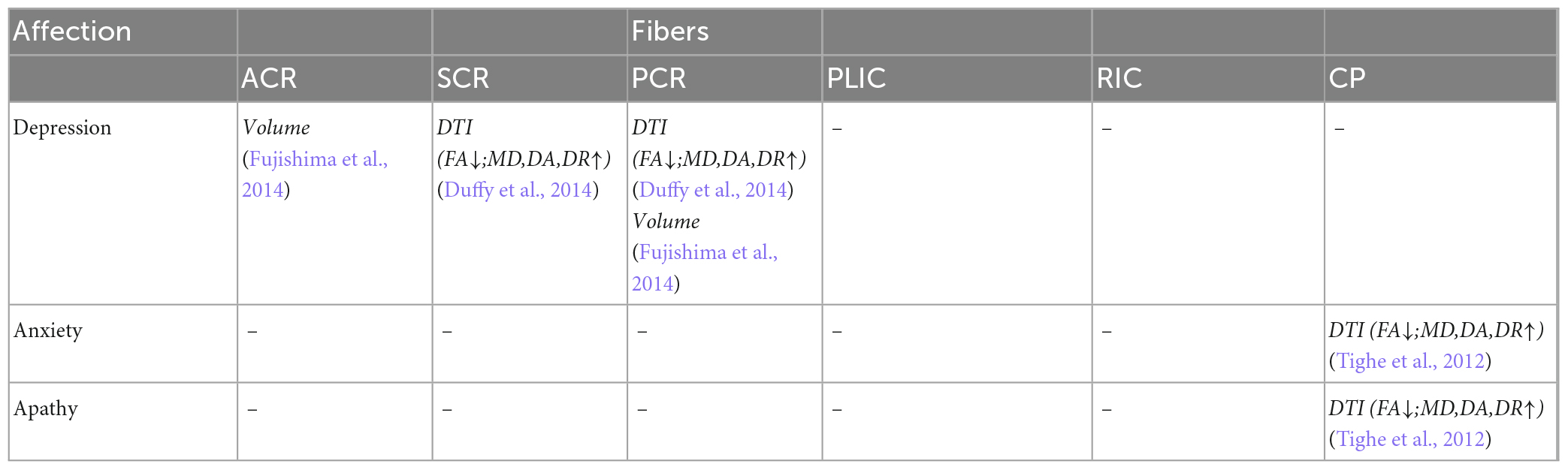
Table 9. Correlation analysis between white matter parameters and affection scales in projection fibers.
Discussion
This review evaluated the relationship between white matter disconnections and behavioral disturbances in MCI. The white matter connections were classified into limbic, projection, association, and commissural fibers according to their connecting brain regions. The correlation studies on diffusion parameters of white matter and the behavior test scales were performed. Cognitions such as episodic memory, semantic memory, visuospatial, attention and executive functions were mainly related to the limbic, association, and commissural fibers. Affections such as depression, anxiety, and apathy are primarily associated with white matter disconnections in the limbic and projection fibers.
Hippocampus and temporal lobe related fibers associated with cognition
Memory loss is the principal clinical manifestation of MCI (Gainotti et al., 2014). The hippocampus is responsible for memory as a critical limbic system component (Bender et al., 2020). Studies on molecular biomarkers, gray matter structure, and functional networks suggest that white matter fibers connected to the hippocampus and temporal lobe appear to be the earliest degeneration in MCI.
Molecular deposition evidence
Molecular biomarkers studies show that the medial temporal lobe and hippocampus are vital sites for amyloid β (Aβ) and hyperphosphorylated tau (pTau) deposition during MCI. Aβ and Tau are detected near the hippocampus before the MCI phase (Rieckmann et al., 2016; Rabin et al., 2019) and deposited in the temporal lobe region near the hippocampus during the MCI (Blamire, 2018). From MCI to AD, the deposition of Aβ and Tau spreads from the medial temporal lobe to the precuneus in the parietal lobe (Pegueroles et al., 2017; Rabin et al., 2019). Aβ and Tau deposition has been found to lead to the demyelination of white matter fibers (Jagust, 2018). Aβ and Tau are deposited first in the hippocampus and temporal lobe during MCI, damaging the white matter structures connected to the hippocampus and temporal lobe.
Gray matter atrophy evidence
Gray matter structure studies have revealed that structures in the temporal lobe, especially the hippocampus, are the critical area of gray matter atrophy during MCI (Jack et al., 2012; Brueggen et al., 2019). In MCI, gray matter atrophy begins with the hippocampus and gradually spreads to the entorhinal cortex, amygdala and other parahippocampal tissues in the temporal lobe (Lee et al., 2014; Lombardi et al., 2020). The hippocampus in the temporal lobe is connected to the parahippocampal tissues by white matter fibers (Zhuo et al., 2016). It was found that the damage to the white matter is an essential cause of the gray matter atrophy (Agosta et al., 2011). The white matter fibers connecting the hippocampus to the temporal lobe first degenerate during MCI and cause gray matter atrophy in the hippocampus and temporal lobe.
Functional connectivity declined evidence
Functional network studies have shown that the functional connectivity between the hippocampus and temporal lobe is significantly reduced in MCI (Lee et al., 2014). In the medial temporal lobe, the amygdala and parahippocampal gyrus have decreased functional connectivity with the hippocampus in MCI (Cai et al., 2017). The medial temporal lobe is an important component of the DMN network, closely related to memory (Li X. et al., 2020). In addition, the hippocampus has decreased functional connectivity with the superior and middle temporal gyrus in the temporal lobe (Liu et al., 2021). The medial and superior temporal gyrus are involved in cognitions such as language comprehension. It has been found that the degeneration of white matter fibers causes the weakening of functional connectivity (Vazquez-Rodriguez et al., 2019). The weakened functional connectivity between the hippocampus and temporal lobe in MCI suggests the white matter disconnections between the hippocampus and temporal lobe.
Therefore, white matter abnormalities related to the hippocampus and temporal lobe are associated with cognition decline in MCI.
Thalamus related fibers–common pathways for cognition and affection
The main cause of cognition decline and affection abnormalities is the white matter disconnection of the neuronal pathways (Jiang and Lou, 2023). The thalamus serves as a relay station for transmitting information from subordinate neurons to the cerebral cortex. The thalamus has extensive white matter connections to the subcortical nuclei and the cerebral cortex (Abivardi and Bach, 2017). The studies on gray matter structure and functional network suggest abnormalities in the thalamus related fibers in MCI.
Gray matter atrophy evidence
Studies on gray matter have revealed that the ventral medial thalamic area undergoes atrophy during the MCI (Nie et al., 2017). Furthermore, gray matter atrophy progressed to the frontal and parietal lobes during AD (Gong et al., 2017). It has been confirmed that white matter degeneration precedes the atrophy of gray matter (Zhuang et al., 2012a; Jack and Holtzman, 2013). Additionally, the projection fibers connect the dorsolateral thalamic area with the parietal and frontal lobes, indicating a potential degeneration of the projection fibers that connect the subcortical nuclei to the cerebral cortex.
Functional connectivity declined evidence
Functional network studies have shown that functional connectivity between the thalamus and the medial temporal, prefrontal and precuneus brain regions in the default network is reduced in MCI (Cai et al., 2015). Reduced functional connectivity between the thalamus and the medial temporal lobe affects the memory capacity of MCI (Min et al., 2019). Reduced functional connectivity between the thalamus and the prefrontal and precuneus affects executive and emotion in MCI (Fjell et al., 2017; Scott et al., 2017). In AD, the functional connectivity between the thalamus and the frontal and parietal lobes is further reduced, leading to aphasia, dysfunction, and dyscognition (Raj et al., 2015). It has been shown that white matter degeneration causes functional connectivity to weaken (Vazquez-Rodriguez et al., 2019). The reduced functional connectivity suggests projection fibers connecting the thalamus to the frontal and parietal lobes may abnormal.
Comorbidity fiber pathways
Besides cognition decline, MCI often suffer from depression, anxiety, apathy and other affection symptoms (Velayudhan, 2023). The thalamus is responsible for memory processing in the limbic system together with the hippocampus. Meanwhile, the thalamus is connected to the amygdala, insula, anterior cingulate gyrus, and parts of the frontal lobe through the projection fibers, which are responsible for affection regulation. Damage to the white matter pathway of the projection fibers between the thalamus and the medial frontal lobe leads to a disruption of information transmission between the cortex and subcortical nuclei, altering the response to external stimuli and increasing the likelihood of cognition and affection abnormalities (Korgaonkar et al., 2014, Yatawara et al., 2019).
Therefore, the white matter degenerations of the thalamus related fibers are associated with cognition decline as well as affection abnormalities in MCI.
Prediction for cognition and affection is crucial for AD early diagnosis
Although clinicians can currently screen MCI with behavior scales, relying on behavior scales alone to confirm MCI is too subjective. It is insensitive to detecting early symptoms of AD influenced by individual differences. Therefore, a more objective, accurate and reliable method is needed to identify and diagnose MCI in the early stage.
Artificial intelligence applied in MCI prediction
The ultimate goal of neuroimaging is to provide physicians with an objective diagnostic basis for screening, diagnosis, and prediction. Data-driven approaches have emerged as a new way of early diagnosis of MCI (Mechelli and Vieira, 2019). Research on individualized prediction based on neuroimaging is increasing yearly, with the prediction of cognition and affection accounting for the current research hotspot (Sui et al., 2020). For cognition and affection in MCI, artificial intelligence (AI) algorithms can perform in-depth analysis based on patients’ multidimensional data such as biomarkers, neuroimaging, and behavioral measures (Dwyer et al., 2018). In addition, AI algorithms can reduce the interference of subjective factors, optimize the model and improve the precision of prediction (Auffermann et al., 2019).
Feature extraction
The selection of the appropriate modality in the acquired imaging data and the accurate feature extraction method is usually more important than the underlying algorithm (Zhang et al., 2021; Zhou et al., 2022b). The methods for extracting white matter information in MCI brain images include reduced density map feature-based methods, predefined region-based methods, discriminative voxel selection-based methods, and connectivity network measure-based methods (Rathore et al., 2017). In addition, multimodal data provide a wider variety of features for MCI prediction. Previous studies have combined structural, functional, and diffusion MRI brain imaging features. Capturing disease information from different modalities and complementary features from multiple perspectives, thus enhancing model performance (Venugopalan et al., 2021).
Algorithms for prediction
Kernel functions and partial least squares correlation analysis capturing the relationship between white matter features and behavior is an effective measure to predict cognition and affection in MCI (Rashid and Calhoun, 2020). Kernel function-based methods often use a local linear weighted regression model that assigns weights to data points using a Gaussian kernel near each prediction point (Mihalik et al., 2020). Partial least squares methods often use regularization to reduce model overfitting by introducing penalty factors to constrain regression coefficients (Koutsouleris et al., 2018). In addition, using multimodal data to establish multivariate maps of different characteristics and behaviors can effectively improve the fit of regression models (Sui and Qi, 2018).
Generalization
The generalization of a model indicates the degree to which a statistical model generated in a set of data performs accurately in a new group or individual. The current scheme to support generalization is nested cross-validation (CV), where a training set is used internally to loop CV with the validation set to select the optimal parameters of the model. A test set is used externally to loop CV to obtain the model performance at the average level (Zhao et al., 2020). The CV has a generalization hierarchy with single-site CV, pooled multisite CV, leave-site-out CV, external validation and prospective validation in descending order of test stringency, with the most stringent being validation of unknown individuals (Dwyer et al., 2018). The training of generalized models relies on a multisite database of multiple samples. The Alzheimer’s disease neuroimaging initiative (ADNI) has a large publicly available dataset with brain imaging data from diagnosed AD, MCI, and healthy controls (Weber et al., 2021). Using a multicenter, extensive sample database with nested CV as a technical tool can fully ensure the model’s generalization (Dou et al., 2020).
Therefore, AI technology has a broad application prospect in the early diagnosis and treatment of MCI, which deserves further exploration and research.
Limitations and perspectives
Exploring the relationship between white matter disconnections and behavioral disturbances such as cognition and affection in MCI is a hot topic of current research. However, there are currently the following problems:
Firstly, studies of specific fibers have focused only on changes in white matter parameters on single nerve tracts in MCI and lack comprehensiveness of the global degenerative mechanisms of MCI. Secondly, it leads to difficulties in feature extraction due to the lack of quantitative indicators for the global white matter network composed of specific fibers. Previous studies have focused on brain regions and network nodes. Quantitative descriptions of neuronal pathway disconnections were laked to analyze the intrinsic relationships between nodes and edges in the network.
Furthermore, most of the studies used small sample sizes for the datasets. There was a significant negative correlation between model prediction accuracy and sample size. Better predictions manifest likely on small samples, which indicate overfitting in the construction of the model. Finally, predicting MCI cognition and affection requires regression models between features and behavior. However, multimodal data with high-dimensional data have interdependent complex multivariate relationships. The optimization method to select relevant variables by constraints is computationally intensive, and the correlation between features is weak. There is a lack of a multivariate regression model based on a comorbid pathway of cognition and affection in MCI to establish the mapping relationship between features and behaviors effectively.
In the future, the sample set should be expanded to analyze changes in specific fibers using multimodal data. White matter networks should combine with graph theory analysis. The edge-centered network clustering approach should be used to extract the combined features of multiple fibers to predict behavioral disturbances such as cognition and affection in MCI.
Conclusion
This article reviews the recent 12 years of studies using diffusion MRI techniques on white matter disconnections associated with behavioral impairment in MCI. The studies showed that degenerated fibers related to the hippocampus and temporal lobe were associated with cognition decline in MCI. Degenerated fibers related to the thalamus were associated with both cognition decline and affection abnormalities in MCI. The sensitivity of diffusion MRI to fiber microstructures can provide a reliable indicator of white matter disconnections in MCI, which can be further quantified in combination with behavioral scales of the patients. This review integrated the correspondence between specific fibers in MCI and behavioral disturbances, which provides a theoretical basis for the subsequent early diagnosis and targeted treatment of AD.
Author contributions
YZ designed and conceptualized the research. YZ, LW, and SG acquisition, analysis, and interpretation of literature. YZ wrote the manuscript. JW and ZH supervision. All authors contributed to the article and approved the submitted version.
Funding
This work was supported by the Key Specialized Research and Development Breakthrough of Henan Province (Grant Nos. 232102210030 to YZ and 222102110067 to SG).
Acknowledgments
The authors thank all the subjects.
Conflict of interest
The authors declare that the research was conducted in the absence of any commercial or financial relationships that could be construed as a potential conflict of interest.
Publisher’s note
All claims expressed in this article are solely those of the authors and do not necessarily represent those of their affiliated organizations, or those of the publisher, the editors and the reviewers. Any product that may be evaluated in this article, or claim that may be made by its manufacturer, is not guaranteed or endorsed by the publisher.
References
Abivardi, A., and Bach, D. R. (2017). Deconstructing white matter connectivity of human amygdala nuclei with thalamus and cortex subdivisions in vivo. Hum. Brain Mapp. 38, 3927–3940. doi: 10.1002/hbm.23639
Agosta, F., Pievani, M., Sala, S., Geroldi, C., Galluzzi, S., Frisoni, G. B., et al. (2011). White matter damage in Alzheimer disease and its relationship to gray matter atrophy. Radiology 258, 853–863.
Ahmed, S., Arnold, R., Thompson, S. A., Graham, K. S., and Hodges, J. R. (2008). Naming of objects, faces and buildings in mild cognitive impairment. Cortex 44, 746–752. doi: 10.1016/j.cortex.2007.02.002
Alexander, A. L., Lee, J. E., Lazar, M., and Field, A. S. (2007). Diffusion tensor imaging of the brain. Neurotherapeutics 4, 316–329.
Allen, J. W., Yazdani, M., Kang, J., Magnussen, M. J., Qiu, D., and Hu, W. (2019). Patients with mild cognitive impairment may be stratified by advanced diffusion metrics and neurocognitive testing. J. Neuroimaging 29, 79–84. doi: 10.1111/jon.12588
Alves, G. S., Carvalho, A. F., de Carvalho, L. D., Sudo, F. K., Siqueira-Neto, J. I., Oertel-Knochel, V., et al. (2017). Neuroimaging findings related to behavioral disturbances in alzheimer’s disease: A systematic review. Curr. Alzheimer Res. 14, 61–75. doi: 10.2174/1567205013666160603010203
Ansado, J., Collins, L., Joubert, S., Fonov, V., Monchi, O., Brambati, S. M., et al. (2013). Interhemispheric coupling improves the brain’s ability to perform low cognitive demand tasks in Alzheimer’s disease and high cognitive demand tasks in normal aging. Neuropsychology 27, 464–480. doi: 10.1037/a0032854
Arvanitakis, Z., Shah, R. C., and Bennett, D. A. (2019). Diagnosis and management of dementia: Review. JAMA 322, 1589–1599.
Ashendorf, L., Jefferson, A. L., O’Connor, M. K., Chaisson, C., Green, R. C., and Stern, R. A. (2008). Trail Making Test errors in normal aging, mild cognitive impairment, and dementia. Arch. Clin. Neuropsychol. 23, 129–137.
Auffermann, W. F., Gozansky, E. K., and Tridandapani, S. (2019). Artificial intelligence in cardiothoracic radiology. Am. J. Roentgenol. 212, 1–5.
Barca, M. L., Persson, K., Eldholm, R., Benth, J. S., Kersten, H., Knapskog, A. B., et al. (2017). Trajectories of depressive symptoms and their relationship to the progression of dementia. J. Affect. Disord. 222, 146–152. doi: 10.1016/j.jad.2017.07.008
Bayard, S., Erkes, J., and Moroni, C. (2011). Victoria stroop test: Normative data in a sample group of older people and the study of their clinical applications in the assessment of inhibition in Alzheimer’s disease. Arch. Clin. Neuropsychol. 26, 653–661. doi: 10.1093/arclin/acr053
Benavides-Varela, S., Burgio, F., Weis, L., Mitolo, M., Palmer, K., Toffano, R., et al. (2020). The role of limbic structures in financial abilities of mild cognitive impairment patients. Neuroimage Clin. 26:102222.
Bender, A. R., Brandmaier, A. M., Duzel, S., Keresztes, A., Pasternak, O., Lindenberger, U., et al. (2020). Hippocampal subfields and limbic white matter jointly predict learning rate in older adults. Cereb. Cortex 30, 2465–2477.
Berlot, R., Metzler-Baddeley, C., Ikram, M. A., Jones, D. K., and O’Sullivan, M. J. (2016). Global efficiency of structural networks mediates cognitive control in mild cognitive impairment. Front. Aging Neurosci. 8:292. doi: 10.3389/fnagi.2016.00292
Blamire, A. M. (2018). MR approaches in neurodegenerative disorders. Prog. Nucl. Magn. Reson. Spectrosc. 108, 1–16.
Boada, M., Cejudo, J. C., Tarraga, L., Lopez, O. L., and Kaufer, D. (2002). Neuropsychiatric Inventory Questionnaire (NPI-Q): Spanish validation of a brief clinical form of the Neuropsychiatric inventory (NPI). Neurologia 17, 317–323.
Boespflug, E. L., Eliassen, J., Welge, J., and Krikorian, R. (2014a). Associative learning and regional white matter deficits in mild cognitive impairment. J. Alzheimers Dis. 41, 421–430.
Boespflug, E. L., Storrs, J., Sadat-Hossieny, S., Eliassen, J., Shidler, M., Norris, M., et al. (2014b). Full diffusion characterization implicates regionally disparate neuropathology in mild cognitive impairment. Brain Struct. Funct. 219, 367–379. doi: 10.1007/s00429-013-0506-x
Bosch, B., Arenaza-Urquijo, E. M., Rami, L., Sala-Llonch, R., Junque, C., Sole-Padulles, C., et al. (2012). Multiple DTI index analysis in normal aging, amnestic MCI and AD. Relationship with neuropsychological performance. Neurobiol. Aging 33, 61–74. doi: 10.1016/j.neurobiolaging.2010.02.004
Bozzali, M., Giulietti, G., Basile, B., Serra, L., Spano, B., Perri, R., et al. (2012). Damage to the cingulum contributes to alzheimer’s disease pathophysiology by deafferentation mechanism. Hum. Brain Mapp. 33, 1295–1308. doi: 10.1002/hbm.21287
Brueggen, K., Dyrba, M., Cardenas-Blanco, A., Schneider, A., Fliessbach, K., Buerger, K., et al. (2019). Structural integrity in subjective cognitive decline, mild cognitive impairment and Alzheimer’s disease based on multicenter diffusion tensor imaging. J. Neurol. 266, 2465–2474. doi: 10.1007/s00415-019-09429-3
Bubb, E. J., Kinnavane, L., and Aggleton, J. P. (2017). Hippocampal - diencephalic - cingulate networks for memory and emotion: An anatomical guide. Brain Neurosci. Adv. 1:2398212817723443. doi: 10.1177/2398212817723443
Burke, W. J., Roccaforte, W. H., and Wengel, S. P. (1991). The short form of the geriatric depression scale: A comparison with the 30-item form. Top. Geriat. 4, 173–178. doi: 10.1177/089198879100400310
Cai, S., Chong, T., Peng, Y., Shen, W., Li, J., von Deneen, K., et al. (2017). Altered functional brain networks in amnestic mild cognitive impairment: A resting-state fMRI study. Brain Imaging Behav. 11, 619–631.
Cai, S., Huang, L., Zou, J., Jing, L., Zhai, B., Ji, G., et al. (2015). Changes in thalamic connectivity in the early and late stages of amnestic mild cognitive impairment: A resting-state functional magnetic resonance study from ADNI. PLoS One 10:e0115573. doi: 10.1371/journal.pone.0115573
Carter, S. F., Embleton, K. V., Anton-Rodriguez, J. M., Burns, A., Ralph, M. A. L., and Herholz, K. (2014). Regional neuronal network failure and cognition in late-onset sporadic Alzheimer disease. Am. J. Neuroradiol. 35, S18–S30. doi: 10.3174/ajnr.A3895
Catani, M., Dell’acqua, F., and Thiebaut de Schotten, M. (2013). A revised limbic system model for memory, emotion and behaviour. Neurosci. Biobehav. Rev. 37, 1724–1737.
Catani, M., Dell’acqua, F., Bizzi, A., Forkel, S. J., Williams, S. C., Simmons, A., et al. (2012). Beyond cortical localization in clinico-anatomical correlation. Cortex 48, 1262–1287. doi: 10.1016/j.cortex.2012.07.001
Chang, Y. L., Yen, Y. S., Chen, T. F., Yan, S. H., and Tseng, W. Y. I. (2016). Clinical dementia rating scale detects white matter changes in older adults at risk for Alzheimer’s disease. J. Alzheimers Dis. 50, 411–423.
Chen, H. F., Huang, L. L., Li, H. Y., Qian, Y., Yang, D., Qing, Z., et al. (2020). Microstructural disruption of the right inferior fronto-occipital and inferior longitudinal fasciculus contributes to WMH-related cognitive impairment. CNS Neurosci. Therapeut. 26, 576–588. doi: 10.1111/cns.13283
Christiansen, K., Aggleton, J. P., Parker, G. D., O’Sullivan, M. J., Vann, S. D., and Metzler-Baddeley, C. (2016). The status of the precommissural and postcommissural fornix in normal ageing and mild cognitive impairment: An MRI tractography study. Neuroimage 130, 35–47. doi: 10.1016/j.neuroimage.2015.12.055
Delano-Wood, L., Bondi, M. W., Jak, A. J., Horne, N. R., Schweinsburg, B. C., Frank, L. R., et al. (2010). Stroke risk modifies regional white matter differences in mild cognitive impairment. Neurobiol. Aging 31, 1721–1731.
Delano-Wood, L., Stricker, N. H., Sorg, S. F., Nation, D. A., Jak, A. J., Woods, S. P., et al. (2012). Posterior cingulum white matter disruption and its associations with verbal memory and stroke risk in mild cognitive impairment. J. Alzheimers Dis. 29, 589–603. doi: 10.3233/JAD-2012-102103
Dimitra, S., Verganelakis, D. A., Gotsis, E., Toulas, P., Papatriantafillou, J., Karageorgiou, C., et al. (2013). Diffusion tensor imaging (DTI) in the detection of white matter lesions in patients with mild cognitive impairment (MCI). Acta Neurol. Belgica 113, 441–451.
Dou, X., Yao, H., Feng, F., Wang, P., Zhou, B., Jin, D., et al. (2020). Characterizing white matter connectivity in Alzheimer’s disease and mild cognitive impairment: An automated fiber quantification analysis with two independent datasets. Cortex 129, 390–405. doi: 10.1016/j.cortex.2020.03.032
Douaud, G., Menke, R. A. L., Gass, A., Monsch, A. U., Rao, A., Whitcher, B., et al. (2013). Brain microstructure reveals early abnormalities more than two years prior to clinical progression from mild cognitive impairment to Alzheimer’s disease. J. Neurosci. 33, 2147–2155. doi: 10.1523/JNEUROSCI.4437-12.2013
Duffy, S. L., Paradise, M., Hickie, B., Lewis, S. J. G., Naismith, S. L., and Lagopoulos, J. (2014). Cognitive impairment with and without depression history: An analysis of white matter microstructure. J. Psychiatry Neurosci. 39, 135–143.
Dwyer, D. B., Falkai, P., and Koutsouleris, N. (2018). Machine learning approaches for clinical psychology and psychiatry. Annu. Rev. Clin. Psychol. 14, 91–118.
Egli, S. C., Hirni, D. I., Taylor, K. I., Berres, M., Regeniter, A., Gass, A., et al. (2015). Varying strength of cognitive markers and biomarkers to predict conversion and cognitive decline in an early-stage-enriched mild cognitive impairment sample. J. Alzheimers Dis. 44, 625–633. doi: 10.3233/JAD-141716
Fan, L. Y., Lai, Y. M., Chen, T. F., Hsu, Y. C., Chen, P. Y., Huang, K. Z., et al. (2018). Diminution of context association memory structure in subjects with subjective cognitive decline. Hum. Brain Mapp. 39, 2549–2562. doi: 10.1002/hbm.24022
Farrar, D. C., Mian, A. Z., Budson, A. E., Moss, M. B., Koo, B. B., Killiany, R. J., et al. (2018). Retained executive abilities in mild cognitive impairment are associated with increased white matter network connectivity. Eur. Radiol. 28, 340–347. doi: 10.1007/s00330-017-4951-4
Fjell, A. M., Sneve, M. H., Grydeland, H., Storsve, A. B., and Walhovd, K. B. (2017). The disconnected brain and executive function decline in aging. Cereb. Cortex 27, 2303–2317.
Friederici, A. D., and Gierhan, S. M. E. (2013). The language network. Curr. Opin. Neurobiol. 23, 250–254.
Fu, X., Wu, Q., and Luo, Y. (2020). A study on the white matter microstructure in amnestic mild cognitive impairment based on neurite orientation dispersion and density imaging. J. Clin. Radiol. 39, 461–465.
Fujishima, M., Maikusa, N., Nakamura, K., Nakatsuka, M., Matsuda, H., and Meguro, K. (2014). Mild cognitive impairment, poor episodic memory, and late-life depression are associated with cerebral cortical thinning and increased white matter hyperintensities. Front. Aging Neurosci. 6:306. doi: 10.3389/fnagi.2014.00306
Gainotti, G., Marra, C., Villa, G., Parlato, V., and Chiaretti, F. (1998). Sensitivity and specificity of some neuropsychological markers of Alzheimer dementia. Alzheimer Dis. Assoc. Disord. 12, 152–162.
Gainotti, G., Quaranta, D., Vita, M. G., and Marra, C. (2014). Neuropsychological predictors of conversion from mild cognitive impairment to Alzheimer’s disease. J. Alzheimers Dis. 38, 481–495.
Gao, S., Chen, Y., Sang, F., Yang, Y., Xia, J., Li, X., et al. (2019). White matter microstructural change contributes to worse cognitive function in patients with type 2 diabetes. Diabetes 68, 2085–2094. doi: 10.2337/db19-0233
Gauthier, S., Reisberg, B., Zaudig, M., Petersen, R. C., Ritchie, K., Broich, K., et al. (2006). Mild cognitive impairment. Lancet 367, 1262–1270.
Gerstenecker, A., Hoagey, D. A., Marson, D. C., and Kennedy, K. M. (2017). White matter degradation is associated with reduced financial capacity in mild cognitive impairment and Alzheimer’s disease. J. Alzheimers Dis. 60, 537–547. doi: 10.3233/JAD-170341
Gill, S., Wang, M., Mouches, P., Rajashekar, D., Sajobi, T., MacMaster, F. P., et al. (2021). Neural correlates of the impulse dyscontrol domain of mild behavioral impairment. Int. J. Geriat. Psychiatry 36, 1398–1406. doi: 10.1002/gps.5540
Gong, N. J., Chan, C. C., Leung, L. M., Wong, C. S., Dibb, R., and Liu, C. (2017). Differential microstructural and morphological abnormalities in mild cognitive impairment and Alzheimer’s disease: Evidence from cortical and deep gray matter. Hum. Brain Mapp. 38, 2495–2508. doi: 10.1002/hbm.23535
Grambaite, R., Stenset, V., Reinvang, I., Walhovd, K. B., Fjell, A. M., and Fladby, T. (2010). White matter diffusivity predicts memory in patients with subjective and mild cognitive impairment and normal CSF total tau levels. J. Int. Neuropsychol. Soc. 16, 58–69.
Gu, L., and Zhang, Z. (2019). Exploring structural and functional brain changes in mild cognitive impairment: A whole brain ALE meta-analysis for multimodal MRI. ACS Chem. Neurosci. 10, 2823–2829. doi: 10.1021/acschemneuro.9b00045
Gyebnar, G., Szabo, A., Siraly, E., Fodor, Z., Sakovics, A., Salacz, P., et al. (2018). What can DTI tell about early cognitive impairment? - Differentiation between MCI subtypes and healthy controls by diffusion tensor imaging. Psychiatry Res. Neuroimaging 272, 46–57. doi: 10.1016/j.pscychresns.2017.10.007
Healey, M., Howard, E., Ungrady, M., Olm, C. A., Nevler, N., Irwin, D. J., et al. (2021). More than words: Extra-sylvian neuroanatomic networks support indirect speech act comprehension and discourse in behavioral variant frontotemporal dementia. Front. Hum. Neurosci. 14:598131. doi: 10.3389/fnhum.2020.598131
Hsu, Y. H., Huang, C. F., Huang, W. H., Deng, J. F., and Tu, M. C. (2019). Microstructural correlates and laterality effect of prospective memory in non-demented adults with memory complaints. Dement. Geriatr. Cogn. Disord. 47, 375–384. doi: 10.1159/000501366
Jack, C. R. Jr., and Holtzman, D. M. (2013). Biomarker modeling of Alzheimer’s disease. Neuron 80, 1347–1358.
Jack, C. R. Jr., Knopman, D. S., Weigand, S. D., Wiste, H. J., Vemuri, P., Lowe, V., et al. (2012). An operational approach to National Institute on Aging-Alzheimer’s Association criteria for preclinical Alzheimer disease. Ann. Neurol. 71, 765–775. doi: 10.1002/ana.22628
Jagust, W. (2018). Imaging the evolution and pathophysiology of Alzheimer disease. Nat. Rev. Neurosci. 19, 687–700.
Jhoo, J. H., Lee, D. Y., Choo, H., Seo, E. H., Oh, J. S., Lee, J. S., et al. (2010). Discrimination of normal aging, MCI and AD with multimodal imaging measures on the medial temporal lobe. Psychiatry Res. Neuroimaging 183, 237–243. doi: 10.1016/j.pscychresns.2010.03.006
Jiang, N., and Lou, V. W. Q. (2023). Caregivers’ depressive symptom trajectories and risk of cognitive impairment among older adults with functional limitations: A prospective cohort study. Int. J. Geriatr. Psychiatry 38:e5850. doi: 10.1002/gps.5850
Jiang, Z., Yang, H., and Tang, X. (2018). Deformation-based statistical shape analysis of the corpus callosum in mild cognitive impairment and Alzheimer’s disease. Curr. Alzheimer Res. 15, 1151–1160.
Jung, W. S., Um, Y. H., Kang, D. W., Lee, C. U., Woo, Y. S., Bahk, W. M., et al. (2018). Diagnostic validity of an automated probabilistic tractography in amnestic mild cognitive impairment. Clin. Psychopharmacol. Neurosci. 16, 144–152. doi: 10.9758/cpn.2018.16.2.144
Kim, D., Lee, S., Choi, M., Youn, H., Suh, S., Jeong, H. G., et al. (2019). Diffusion tensor imaging reveals abnormal brain networks in elderly subjects with subjective cognitive deficits. Neurol. Sci. 40, 2333–2342. doi: 10.1007/s10072-019-03981-6
Kohnert, K. J., Hernandez, A. E., and Bates, E. (1998). Bilingual performance on the boston naming test: Preliminary norms in spanish and english. Brain Lang. 65, 422–440.
Korgaonkar, M. S., Williams, L. M., Song, Y. J., Usherwood, T., and Grieve, S. M. (2014). Diffusion tensor imaging predictors of treatment outcomes in major depressive disorder. Br. J. Psychiatry 205, 321–328.
Koutsouleris, N., Kambeitz-Ilankovic, L., Ruhrmann, S., Rosen, M., Ruef, A., Dwyer, D. B., et al. (2018). Prediction models of functional outcomes for individuals in the clinical high-risk state for psychosis or with recent-onset depression: A multimodal, multisite machine learning analysis. JAMA Psychiatry 75, 1156–1172.
Le, B. D., Mangin, J., Poupon, C., Clark, C., Pappata, S., Molko, N., et al. (2001). Diffusion tensor imaging: Concepts and applications. J. Magnet. Reson. Imaging 13, 534–546. doi: 10.1002/jmri.1076
Lee, D. Y., Fletcher, E., Carmichael, O. T., Singh, B., Mungas, D., Reed, B., et al. (2012). Sub-regional hippocampal injury is associated with fornix degeneration in Alzheimer’s disease. Front. Aging Neurosci. 4:1. doi: 10.3389/fnagi.2012.00001
Lee, H., Swanwick, G. R., Coen, R. F., and Lawlor, B. A. (1996). Use of the clock drawing task in the diagnosis of mild and very mild Alzheimer’s disease. Int. Psychogeriatr. 8, 469–476.
Lee, S., Seo, J., Lee, J., Park, H., and Adni. (2014). Differences in early and late mild cognitive impairment tractography using a diffusion tensor MRI. Neuroreport 25, 1393–1398. doi: 10.1097/WNR.0000000000000279
Li, K., Wang, S. Y., Luo, X., Zeng, Q. Z., Jiaerken, Y., Xu, X. P., et al. (2020). Progressive memory circuit impairments along with alzheimer’s disease neuropathology spread: Evidence from in vivo neuroimaging. Cereb. Cortex 30, 5863–5873. doi: 10.1093/cercor/bhaa162
Li, X., Wang, H., Tian, Y., Zhou, S., Li, X., Wang, K., et al. (2016). Impaired white matter connections of the limbic system networks associated with impaired emotional memory in Alzheimer’s disease. Front. Aging Neurosci. 8:250. doi: 10.3389/fnagi.2016.00250
Li, X., Xia, J., Ma, C., Chen, K., Xu, K., Zhang, J., et al. (2020). Accelerating structural degeneration in temporal regions and their effects on cognition in aging of MCI patients. Cereb. Cortex 30, 326–338. doi: 10.1093/cercor/bhz090
Lin, Y. C., Shih, Y. C., Tseng, W. Y. I., Chu, Y. H., Wu, M. T., Chen, T. F., et al. (2014). Cingulum correlates of cognitive functions in patients with mild cognitive impairment and early Alzheimer’s disease: A diffusion spectrum imaging study. Brain Topogr. 27, 393–402. doi: 10.1007/s10548-013-0346-2
Liu, L., Jiang, H., Wang, D., and Zhao, X. F. (2021). A study of regional homogeneity of resting-state functional magnetic resonance imaging in mild cognitive impairment. Behav. Brain Res. 402:113103.
Lombardi, G., Crescioli, G., Cavedo, E., Lucenteforte, E., Casazza, G., Bellatorre, A. G., et al. (2020). Structural magnetic resonance imaging for the early diagnosis of dementia due to Alzheimer’s disease in people with mild cognitive impairment. Cochrane Database Syst. Rev. 3:CD009628.
Mascalchi, M., Salvadori, E., Toschi, N., Giannelli, M., Orsolini, S., Ciulli, S., et al. (2019). DTI-derived indexes of brain WM correlate with cognitive performance in vascular MCI and small-vessel disease. A TBSS study. Brain Imaging Behav. 13, 594–602. doi: 10.1007/s11682-018-9873-5
Mechelli, A., and Vieira, S. (2019). Machine learning: Methods and applications to brain disorders. Amsterdam: Elsevier.
Metzler-Baddeley, C., Hunt, S., Jones, D. K., Leemans, A., Aggleton, J. P., and O’Sullivan, M. J. (2012a). Temporal association tracts and the breakdown of episodic memory in mild cognitive impairment. Neurology 79, 2233–2240.
Metzler-Baddeley, C., Jones, D. K., Steventon, J., Westacott, L., Aggleton, J. P., and O’Sullivan, M. J. (2012b). Cingulum microstructure predicts cognitive control in older age and mild cognitive impairment. J. Neurosci. 32, 17612–17619.
Mielke, M. M., Okonkwo, O. C., Oishi, K., Mori, S., Tighe, S., Miller, M. I., et al. (2012). Fornix integrity and hippocampal volume predict memory decline and progression to Alzheimer’s disease. Alzheimers Dement. 8, 105–113.
Mihalik, A., Ferreira, F. S., Moutoussis, M., Ziegler, G., Adams, R. A., Rosa, M. J., et al. (2020). Multiple holdouts with stability: Improving the generalizability of machine learning analyses of brain-behavior relationships. Biol. Psychiatry 87, 368–376. doi: 10.1016/j.biopsych.2019.12.001
Miller, K. L., Alfaro-Almagro, F., Bangerter, N. K., Thomas, D. L., Yacoub, E., Xu, J. Q., et al. (2016). Multimodal population brain imaging in the UK Biobank prospective epidemiological study. Nat. Neurosci. 19, 1523–1536.
Min, J., Zhou, X. X., Zhou, F., Tan, Y., and Wang, W. D. (2019). A study on changes of the resting-state brain function network in patients with amnestic mild cognitive impairment. Braz. J. Med. Biol. Res. 52:e8244. doi: 10.1590/1414-431X20198244
Mito, R., Raffelt, D., Dhollander, T., Vaughan, D. N., Tournier, J. D., Salvado, O., et al. (2018). Fibre-specific white matter reductions in Alzheimer’s disease and mild cognitive impairment. Brain 141, 888–902.
Nie, X., Sun, Y., Wan, S., Zhao, H., Liu, R., Li, X., et al. (2017). Subregional structural alterations in hippocampus and nucleus accumbens correlate with the clinical impairment in patients with alzheimer’s disease clinical spectrum: Parallel combining volume and vertex-based approach. Front. Neurol. 8:399. doi: 10.3389/fneur.2017.00399
Nir, T. M., Jahanshad, N., Villalon-Reina, J. E., Toga, A. W., Jack, C. R., Weiner, M. W., et al. (2013). Effectiveness of regional DTI measures in distinguishing Alzheimer’s disease, MCI, and normal aging. Neuroimage Clin. 3, 180–195. doi: 10.1016/j.nicl.2013.07.006
O’Dwyer, L., Lamberton, F., Bokde, A. L. W., Ewers, M., Faluyi, Y. O., Tanner, C., et al. (2011). Multiple indices of diffusion identifies white matter damage in mild cognitive impairment and Alzheimer’s disease. PLoS One 6:e21745. doi: 10.1371/journal.pone.0021745
Pantel, J., Laks, J., Boecker, H., Knochel, C., Jurcoane, A., Oertel-Knochel, V., et al. (2016). Neuroimaging findings related to behavioral disturbances in Alzheimer’s disease: A systematic review. Curr. Alzheimer Res. 14, 61–75. doi: 10.2174/1567205013666160603010203
Paolo, A. M., Axelrod, B. N., Troster, A. I., Blackwell, K. T., and Koller, W. C. (1996). Utility of a wisconsin card sorting test short form in persons with Alzheimer’s and Parkinson’s disease. J. Clin. Exp. Neuropsychol. 18, 892–897. doi: 10.1080/01688639608408310
Park, C. H., Kim, S. H., and Jung, H. Y. (2019). Characteristics of the uncinate fasciculus and cingulum in patients with mild cognitive impairment: Diffusion tensor tractography study. Brain Sci. 9:377. doi: 10.3390/brainsci9120377
Pasternak, O., Kelly, S., Sydnor, V. J., and Shenton, M. E. (2018). Advances in microstructural diffusion neuroimaging for psychiatric disorders. Neuroimage 182, 259–282.
Pegueroles, J., Vilaplana, E., Montal, V., Sampedro, F., Alcolea, D., Carmona-Iragui, M., et al. (2017). Longitudinal brain structural changes in preclinical Alzheimer’s disease. Alzheimers Dement. 13, 499–509.
Petersen, R. C., Smith, G. E., Waring, S. C., Ivnik, R. J., Tangalos, E. G., and Kokmen, E. (1999). Mild cognitive impairment - Clinical characterization and outcome. Arch. Neurol. 56, 303–308.
Pini, L., Pievani, M., Bocchetta, M., Altomare, D., Bosco, P., Cavedo, E., et al. (2016). Brain atrophy in Alzheimer’s Disease and aging. Ageing Res. Rev. 30, 25–48.
Qin, Y., Zhang, S., Guo, L., Zhang, M., and Zhu, W. (2016). Atlas-based deep gray matter and white matter analysis in Alzheimer’s disease: Diffusion abnormality and correlation with cognitive function. Chin. J. Radiol. 50, 348–352.
Rabin, J. S., Perea, R. D., Buckley, R. F., Neal, T. E., Buckner, R. L., Johnson, K. A., et al. (2019). Global white matter diffusion characteristics predict longitudinal cognitive change independently of amyloid status in clinically normal older adults. Cereb. Cortex 29, 1251–1262. doi: 10.1093/cercor/bhy031
Rabin, L. A., Pare, N., Saykin, A. J., Brown, M. J., Wishart, H. A., Flashman, L. A., et al. (2009). Differential memory test sensitivity for diagnosing amnestic mild cognitive impairment and predicting conversion to Alzheimer’s disease. Aging Neuropsychol. Cogn. 16, 357–376.
Radakovic, R., and Abrahams, S. (2014). Developing a new apathy measurement scale: Dimensional apathy scale. Psychiatry Res. 219, 658–663.
Raghavan, S., Przybelski, S. A., Reid, R. I., Graff-Radford, J., Lesnick, T. G., Zuk, S. M., et al. (2020). Reduced fractional anisotropy of the genu of the corpus callosum as a cerebrovascular disease marker and predictor of longitudinal cognition in MCI. Neurobiol. Aging 96, 176–183. doi: 10.1016/j.neurobiolaging.2020.09.005
Raj, A., LoCastro, E., Kuceyeski, A., Tosun, D., Relkin, N., Weiner, M., et al. (2015). Network diffusion model of progression predicts longitudinal patterns of atrophy and metabolism in Alzheimer’s disease. Cell Rep. 10, 359–369. doi: 10.1016/j.celrep.2014.12.034
Rashid, B., and Calhoun, V. (2020). Towards a brain-based predictome of mental illness. Hum. Brain Mapp. 41, 3468–3535. doi: 10.1002/hbm.25013
Rathore, S., Habes, M., Iftikhar, M. A., Shacklett, A., and Davatzikos, C. (2017). A review on neuroimaging-based classification studies and associated feature extraction methods for Alzheimer’s disease and its prodromal stages. Neuroimage 155:530. doi: 10.1016/j.neuroimage.2017.03.057
Ray, N. J., Metzler-Baddeley, C., Khondoker, M. R., Grothe, M. J., Teipel, S., Wright, P., et al. (2015). Cholinergic basal forebrain structure influences the reconfiguration of white matter connections to support residual memory in mild cognitive impairment. J. Neurosci. 35, 739–747. doi: 10.1523/JNEUROSCI.3617-14.2015
Reedy, S. D., Boone, K. B., Cottingham, M. E., Glaser, D. F., Lu, P. H., Victor, T. L., et al. (2013). Cross validation of the lu and colleagues (2003) Rey-Osterrieth Complex Figure Test effort equation in a large known-group sample. Arch. Clin. Neuropsychol. 28, 30–37. doi: 10.1093/arclin/acs106
Remy, F., Vayssiere, N., Saint-Aubert, L., Barbeau, E., and Pariente, J. (2015). White matter disruption at the prodromal stage of Alzheimer’s disease: Relationships with hippocampal atrophy and episodic memory performance. Neuroimage Clin. 7, 482–492. doi: 10.1016/j.nicl.2015.01.014
Richards, T., Heide, A., Tsuruda, J., and Alvord, E. (1992). Vector analysis of diffusion images in experimental allergic encephalomyelitis. Soc. Magn. Resonan. Med. 11:412. doi: 10.1038/jcbfm.2013.12
Rieckmann, A., Van Dijk, K. R., Sperling, R. A., Johnson, K. A., Buckner, R. L., and Hedden, T. (2016). Accelerated decline in white matter integrity in clinically normal individuals at risk for Alzheimer’s disease. Neurobiol. Aging 42, 177–188.
Sarazin, M. (2008). Amnestic syndrome of the medial temporal type identifies prodromal AD: A longitudinal study. Neurology 70, 2016–2016. doi: 10.1212/01.wnl.0000279336.36610.f7
Saunders, N. L. J., and Summers, M. J. (2011). Longitudinal deficits to attention, executive, and working memory in subtypes of mild cognitive impairment. Neuropsychology 25, 237–248.
Scott, J. A., Tosun, D., Braskie, M. N., Maillard, P., Thompson, P. M., Weiner, M., et al. (2017). Independent value added by diffusion MRI for prediction of cognitive function in older adults. Neuroimage Clin. 14, 166–173. doi: 10.1016/j.nicl.2017.01.026
Serra, L., Cercignani, M., Basile, B., Spano, B., Perri, R., Fadda, L., et al. (2012). White matter damage along the uncinate fasciculus contributes to cognitive decline in AD and DLB. Curr. Alzheimer Res. 9, 326–333. doi: 10.2174/156720512800107555
Serrano, C., Allegri, R. F., Drake, M., Butman, J., Harris, P., Nagle, C., et al. (2001). A shortened form of the Spanish Boston naming test: A useful tool for the diagnosis of Alzheimer’s disease. Rev. Neurol. 33, 624–627.
Sexton, C. E., Mackay, C. E., Lonie, J. A., Bastin, M. E., Terriere, E., O’Carroll, R. E., et al. (2010). MRI correlates of episodic memory in Alzheimer’s disease, mild cognitive impairment, and healthy aging. Psychiatry Res. Neuroimaging 184, 57–62.
Shi, J., Tian, J., Wei, M., Miao, Y., and Wang, Y. (2012). The utility of the Hopkins Verbal Learning Test (Chinese version) for screening dementia and mild cognitive impairment in a Chinese population. BMC Neurol. 12:136. doi: 10.1186/1471-2377-12-136
Shi, J., Wei, M., Tian, J., Snowden, J., Zhang, X., Ni, J., et al. (2014). The Chinese version of story recall: A useful screening tool for mild cognitive impairment and Alzheimer’s disease in the elderly. BMC Psychiatry 14:71. doi: 10.1186/1471-244X-14-71
Shim, G., Choi, K. Y., Kim, D., Suh, S.-I., Lee, S., Jeong, H.-G., et al. (2017). Predicting neurocognitive function with hippocampal volumes and DTI metrics in patients with Alzheimer’s dementia and mild cognitive impairment. Brain Behav. 7:e00766. doi: 10.1002/brb3.766
Snir, J. A., Bartha, R., and Montero-Odasso, M. (2019). White matter integrity is associated with gait impairment and falls in mild cognitive impairment. Results from the gait and brain study. Neuroimage Clin. 24:101975.
Song, S.-K., Sun, S.-W., Ramsbottom, M. J., Chang, C., Russell, J., and Cross, A. H. (2002). Dysmyelination revealed through MRI as increased radial (but unchanged axial) diffusion of water. Neuroimage 17, 1429–1436. doi: 10.1006/nimg.2002.1267
Srisaikaew, P., Wongpakaran, N., Anderson, N. D., Chen, J. J., Kothan, S., Varnado, P., et al. (2020). Fornix Integrity Is Differently Associated With Cognition in Healthy Aging and Non-amnestic Mild Cognitive Impairment: A Pilot Diffusion Tensor Imaging Study in Thai Older Adults. Front. Aging Neurosci. 12:594002. doi: 10.3389/fnagi.2020.594002
Sui, J., and Qi, S. (2018). Multimodal neuromarkers in schizophrenia via cognition-guided MRI fusion. Nat. Commun. 9:3028. doi: 10.1038/s41467-018-05432-w
Sui, J., Jiang, R., Bustillo, J., and Calhoun, V. (2020). Neuroimaging-based Individualized Prediction of Cognition and Behavior for Mental Disorders and Health: Methods and Promises. Biol. Psychiatry 88, 818–828. doi: 10.1016/j.biopsych.2020.02.016
Tan, E. Y. L., Kohler, S., Hamel, R. E. G., Munoz-Sanchez, J. L., Verhey, F. R. J., and Ramakers, I. H. G. B. (2019). Depressive Symptoms in Mild Cognitive Impairment and the Risk of Dementia: A Systematic Review and Comparative Meta-Analysis of Clinical and Community-Based Studies. J. Alzheimers Dis. 67, 1319–1329. doi: 10.3233/JAD-180513
Tighe, S. K., Oishi, K., Mori, S., Smith, G. S., Albert, M., Lyketsos, C. G., et al. (2012). Diffusion Tensor Imaging of Neuropsychiatric Symptoms in Mild Cognitive Impairment and Alzheimer’s Dementia. J. Neuropsychiatry Clin. Neurosci. 24, 484–488.
Torralva, T., Laffaye, T., Baez, S., Gleichgerrcht, E., Bruno, D., Chade, A., et al. (2015). Verbal Fluency as a Rapid Screening Test for Cognitive Impairment in Early Parkinson’s Disease. J. Neuropsychiatry Clin. Neurosci. 27, 244–247.
Torso, M., Serra, L., Giulietti, G., Spano, B., Tuzzi, E., Koch, G., et al. (2015). Strategic lesions in the anterior thalamic radiation and apathy in early Alzheimer’s disease. PLoS One 10:e0124998. doi: 10.1371/journal.pone.0124998
Tu, M. C., Lo, C. P., Huang, C. F., Huang, W. H., Deng, J. F., and Hsu, Y. H. (2018). Visual attention performances and related cerebral microstructural integrity among subjects with subjective cognitive decline and mild cognitive impairment. Front. Aging Neurosci. 10:268. doi: 10.3389/fnagi.2018.00268
Urbanski, M., Thiebaut de Schotten, M., Rodrigo, S., Catani, M., Oppenheim, C., Touze, E., et al. (2008). Brain networks of spatial awareness: Evidence from diffusion tensor imaging tractography. J. Neurol. Neurosurg. Psychiatry 79, 598–601.
Vazquez-Rodriguez, B., Suarez, L. E., Markello, R. D., Shafiei, G., Paquola, C., Hagmann, P., et al. (2019). Gradients of structure-function tethering across neocortex. Proc. Natl. Acad. Sci. U. S. A. 116, 21219–21227. doi: 10.1073/pnas.1903403116
Velayudhan, L. (2023). Apathy and depression as risk factors for dementia conversion in mild cognitive impairment. Int. Psychogeriatr. 1–7. [Epub ahead of print]. doi: 10.1017/S1041610223000042
Venugopalan, J., Tong, L., Hassanzadeh, H. R., and Wang, M. D. (2021). Multimodal deep learning models for early detection of Alzheimer’s disease stage. Sci. Rep. 11:3254.
Vogel, A., Mortensen, E. L., Gade, A., and Waldemar, G. (2007). The Category Cued Recall test in very mild Alzheimer’s disease: Discriminative validity and correlation with semantic memory functions. Eur. J. Neurol. 14, 102–108. doi: 10.1111/j.1468-1331.2006.01568.x
Wang, J., Zuo, X., Dai, Z., Xia, M., Zhao, Z., Zhao, X., et al. (2013). Disrupted functional brain connectome in individuals at risk for Alzheimer’s disease. Biol. Psychiatry 73, 472–481.
Weber, C. J., Carrillo, M. C., Jagust, W., Jack, C. R., Shaw, L. M., Trojanowski, J. Q., et al. (2021). The Worldwide Alzheimer’s Disease Neuroimaging Initiative: ADNI-3 updates and global perspectives. Alzheimers Dement. 7:e12226. doi: 10.1002/trc2.12226
Wen, Q., Mustafi, S. M., Li, J., Risacher, S. L., Tallman, E., Brown, S. A., et al. (2019). White matter alterations in early-stage Alzheimer’s disease: A tract-specific study. Alzheimers Dement. 11, 576–587. doi: 10.1016/j.dadm.2019.06.003
Wong, D., Atiya, S., Fogarty, J., Montero-Odasso, M., Pasternak, S. H., Brymer, C., et al. (2020). Reduced hippocampal glutamate and posterior cingulate n-acetyl aspartate in mild cognitive impairment and alzheimer’s disease is associated with episodic memory performance and white matter integrity in the cingulum: A pilot study. J. Alzheimers Dis. 73, 1385–1405. doi: 10.3233/JAD-190773
Yang, F. P. G., Bal, S. S., Lee, J. F., and Chen, C. C. (2021). White matter differences in networks in elders with mild cognitive impairment and Alzheimer’s disease. Brain Connect. 11, 180–188.
Yatawara, C., Lee, D., Ng, K. P., Chander, R., Ng, D., Ji, F., et al. (2019). Mechanisms linking white matter lesions, tract integrity, and depression in Alzheimer disease. Am. J. Geriatr. Psychiatry 27, 948–959.
Yu, J., Lee, T. M. C., and Alzheimers Disease Neuroimaging. (2020). Verbal memory and hippocampal volume predict subsequent fornix microstructure in those at risk for Alzheimer’s disease. Brain Imaging Behav. 14, 2311–2322. doi: 10.1007/s11682-019-00183-8
Yu, M., Sporns, O., and Saykin, A. J. (2021). The human connectome in Alzheimer disease - relationship to biomarkers and genetics. Nat. Rev. Neurol. 17, 545–563.
Zhang, L., Zheng, L., Zhang, G., Wang, X., Hu, Y., and Ling, R. (2011). MR diffusion tensor imaging of white matter abnormality: Correlation with cognitive function in elders. Chin. Comput. Med. Imaging 17, 12–17.
Zhang, Y. P., Wang, S. H., Xia, K. J., Jiang, Y. Z., Qian, P. J., and Initia, A. D. N. (2021). Alzheimer’s disease multiclass diagnosis via multimodal neuroimaging embedding feature selection and fusion. Inform. Fusion 66, 170–183.
Zhao, K., Ding, Y., Han, Y., Fan, Y., Alexander-Bloch, A., Han, T., et al. (2020). Independent and reproducible hippocampal radiomic biomarkers for multisite Alzheimer?s disease: Diagnosis, longitudinal progress and biological basis. Sci. Bull. 65, 1103–1113. doi: 10.1016/j.scib.2020.04.003
Zheng, F., Liu, Y., Yuan, Z., Gao, X., He, Y., Liu, X., et al. (2019). Age-related changes in cortical and subcortical structures of healthy adult brains: A surface-based morphometry study. J. Magn. Reson. Imaging 49, 152–163. doi: 10.1002/jmri.26037
Zhou, Y., Si, X. P., Chao, Y. P., Chen, Y. Y., Lin, C. P., Li, S. C., et al. (2022b). Automated classification of mild cognitive impairment by machine learning with hippocampus-related white matter network. Front. Aging Neurosci. 14:866230. doi: 10.3389/fnagi.2022.866230
Zhou, Y., Si, X. P., Chen, Y. Y., Chao, Y. P., Lin, C. P., Li, S. C., et al. (2022a). Hippocampus- and thalamus-related fiber-specific white matter reductions in mild cognitive impairment. Cereb. Cortex 32, 3159–3174. doi: 10.1093/cercor/bhab407
Zhuang, L., Sachdev, P. S., Trollor, J. N., Kochan, N. A., Reppermund, S., Brodaty, H., et al. (2012a). Microstructural white matter changes in cognitively normal individuals at risk of amnestic MCI. Neurology 79, 748–754.
Zhuang, L., Wen, W., Trollor, J. N., Kochan, N. A., Reppermund, S., Brodaty, H., et al. (2012b). Abnormalities of the fornix in mild cognitive impairment are related to episodic memory loss. J. Alzheimers Dis. 29, 629–639.
Zhuo, J. J., Fan, L. Z., Liu, Y., Zhang, Y. C., Yu, C. S., and Jiang, T. Z. (2016). Connectivity profiles reveal a transition subarea in the parahippocampal region that integrates the anterior temporal-posterior medial systems. J. Neurosci. 36, 2782–2795. doi: 10.1523/JNEUROSCI.1975-15.2016
Zimmermann, J., Perry, A., Breakspear, M., Schirner, M., Sachdev, P., Wen, W., et al. (2018). Differentiation of Alzheimer’s disease based on local and global parameters in personalized Virtual Brain models. Neuroimage Clin. 19, 240–251. doi: 10.1016/j.nicl.2018.04.017
Zimny, A., Szewczyk, P., Bladowska, J., Trypka, E., Wojtynska, R., Leszek, J., et al. (2012). Quantitative evaluation of changes in the selected white matter tracts using diffusion tensor imaging in patients with Alzheimer’s disease and mild cognitive impairment. Neuroradiol. J. 25, 300–310. doi: 10.1177/197140091202500304
Keywords: mild cognitive impairment, diffusion MRI, white matter disconnection, cognition, affection
Citation: Zhou Y, Wei L, Gao S, Wang J and Hu Z (2023) Characterization of diffusion magnetic resonance imaging revealing relationships between white matter disconnection and behavioral disturbances in mild cognitive impairment: a systematic review. Front. Neurosci. 17:1209378. doi: 10.3389/fnins.2023.1209378
Received: 20 April 2023; Accepted: 23 May 2023;
Published: 08 June 2023.
Edited by:
Yanyan Jiang, Shandong University, ChinaReviewed by:
Chih-Hong Chou, Shanghai Jiao Tong University, ChinaShinichi Yamada, Wakayama Medical University, Japan
Chi-Heng Lu, Linkou Chang Gung Memorial Hospital, Taiwan
Copyright © 2023 Zhou, Wei, Gao, Wang and Hu. This is an open-access article distributed under the terms of the Creative Commons Attribution License (CC BY). The use, distribution or reproduction in other forums is permitted, provided the original author(s) and the copyright owner(s) are credited and that the original publication in this journal is cited, in accordance with accepted academic practice. No use, distribution or reproduction is permitted which does not comply with these terms.
*Correspondence: Jun Wang, d2FuZ2p1bl9oYXVzdEAxNjMuY29t; Zhigang Hu, aHUucm9iZXJ0QDE2My5jb20=