- 1School of Electrical Engineering and Electronic Information, Xihua University, Chengdu, China
- 2School of Information and Communication Engineering, University of Electronic Science and Technology of China, Chengdu, China
Introduction: The gender disparity in autism spectrum disorder (ASD) has been one of the salient features of condition. However, its relationship between the pathogenesis and genetic transcription in patients of different genders has yet to reach a reliable conclusion.
Methods: To address this gap, this study aimed to establish a reliable potential neuro-marker in gender-specific patients, by employing multi-site functional magnetic resonance imaging (fMRI) data, and to further investigate the role of genetic transcription molecules in neurogenetic abnormalities and gender differences in autism at the neuro-transcriptional level. To this end, age was firstly used as a regression covariate, followed by the use of ComBat to remove the site effect from the fMRI data, and abnormal functional activity was subsequently identified. The resulting abnormal functional activity was then correlated by genetic transcription to explore underlying molecular functions and cellular molecular mechanisms.
Results: Abnormal brain functional activities were identified in autism patients of different genders, mainly located in the default model network (DMN) and precuneus-cingulate gyrus-frontal lobe. The correlation analysis of neuroimaging and genetic transcription further found that heterogeneous brain regions were highly correlated with genes involved in signal transmission between neurons’ plasma membranes. Additionally, we further identified different weighted gene expression patterns and specific expression tissues of risk genes in ASD of different genders.
Discussion: Thus, this work not only identified the mechanism of abnormal brain functional activities caused by gender differences in ASD, but also explored the genetic and molecular characteristics caused by these related changes. Moreover, we further analyzed the genetic basis of sex differences in ASD from a neuro-transcriptional perspective.
Introduction
The gender differences have been considered to be one of the causes of physiological heterogeneity of neurological disorders. Autism Spectrum Disorder (ASD) is a highly heritable psychiatric disorder with significant gender differences (Rossignol and Frye, 2012). Its main characteristics include a lack of social communication skills, restricted or repetitive behaviors or interests (Matta et al., 2019). Uncertainty persists regarding the connection between neurological impairment and the genetic transcription of ASD. Male have a higher susceptibility to ASD than female. To be more precise, the male-to-female ratio is close to 4:1 (Loomes et al., 2017; Maenner et al., 2020). However, this quantitative bias toward male in existing studies has results in ignoring the influence of gender bias on pathophysiology and genetic mechanisms, thereby impeding the generalizability of research findings (Werling and Geschwind, 2013).
Functional Magnetic resonance imaging (fMRI) provides an effective tool for the identification and investigation of altered structural and functional brain networks. Researchers can precisely identify heterogeneous brain areas using MRI with high pixel resolution. The failure of the default mode network (DMN) is involved in a variety of psychiatric diseases. The local functional changes of DMN and their atypical developmental trajectory are considered to be the typical neurobiological features of ASD (Padmanabhan et al., 2017). In a longitudinal study of gray matter in the brains of female with ASD, abnormal growth features were found to be more pronounced in females than in males, male patients showed increased activation in the bilateral medial frontal gyrus and female patients showed decreased activation in the midbrain and limbic regions (Schumann et al., 2010; Schneider et al., 2013). In contrast, the volume of gray matter, white matter, and hippocampus was larger in male ASD than in HC, whereas females did not experience these changes. In addition, the volume of the right putamen was smaller in females with ASD than in HC, but no such difference was found in males (Zhang et al., 2018). Although this sex difference is not unique to autism, the study of sex differences is crucial in revealing the underlying pathogenesis of ASD.
With the development of genetic transcription technology, more and more targeted researchers have found that specific gene expression patterns may play an important role in the pathogenesis of ASD (Quesnel-Vallieres et al., 2019). It suggests that transcriptomics may also influence the sex aberration in ASD. However, to date, research on sex-specific susceptibility genetic risk loci for autism is incomplete (Modabbernia et al., 2017). Studies have reported that there appears to be a “female protective pattern” in women with a higher threshold of genetic susceptibility (Robinson et al., 2013; Werling and Geschwind, 2013; Jacquemont et al., 2014). In addition, another study points out that fetal testosterone levels in amniotic fluid were positively correlated with typical behavior after maturation, while women experience chronic exposure to higher levels of androgens before birth and these high levels of androgens seem to shelter them from ASD (Auyeung et al., 2009).
In this study, we aimed to use resting-state functional magnetic resonance imaging to calculate the functional activity intensity of the Blood Oxygen Level Dependent signal (BOLD) in Amplitude of Low Frequency Fluctuation (ALFF), Degree Centrality (DC), and Regional Homogeneity (ReHo; Zang et al., 2004; Zuo et al., 2012; Yan et al., 2013; Lin et al., 2023; Wang et al., 2023) to describe the neural activity of male and female with ASD. We then use ComBat (Fortin et al., 2018; Reardon et al., 2021; Chen et al., 2022; Du et al., 2023) to remove the “scanner effect” and obtain cross-site large sample heterogeneous brain functional activity changes. Finally, we use partial least squares regression analysis (PLSr) to characterize the spatial correlation between changes in brain functional activity characteristics and genetic transcription profiles of ASD.
Materials and methods
Participant
To investigate the effects of gender differences on the brains of individuals with autism, we utilized rigorous criteria to select resting state fMRI data from autism brain imaging data exchange (ABIDE)1 of 139 male ASD, 153 male healthy control (HC), 34 female ASD, and 46 female HC, totaling 372 subjects (Di Martino et al., 2014). In order to reduce individual differences and multi-site data differences, we applied the following data screening criteria: (1) Head motion displacement was less than 2 mm or 2 degrees. (2) The average frame displacement is less than 0.5. (3) The missing volume ratio is less than 0.5. (4) The Chi-square test value of p of male and female subjects at each site was greater than 0.05.
Preprocessing of resting state fMRI data
All resting state fMRI data were preprocessed by DPARSFA in a standardized and unified channel, which included the following 9 steps: (1) The first 10 volumes were deleted. (2) The remaining images were aligned, and the data with maximum head movement exceeding 2 degrees was removed. (3) All fMRI data were matched to the standard Echo Planar Imaging (EPI) template from the Montreal Neurological Institute (MNI-152) and resampling to a mm voxel size. (4) The linear trend of fMRI data was removed. (5) The ALFF map of each subject was calculated and normalized using Z-transformation. (6) A bandpass filter was used to remove the noise signal (0.01 < f < 0.1 Hz) for the remaining BOLD time series. (7) Nuisance covariates from white matter and Cerebro-Spinal Fluid (CSF) were estimated separately and were then regressed from the resting-state fMRI data along with the motion realignment parameters, global mean signal. (8) To further reduce the effect of head movement, cubic spline interpolation was used to scrub the volume of the image with an average Frame Displacement (FD) > 0.5 mm. (9) The ReHo and DC images of each subject were normalized by z transformation. ALFF, ReHo, and DC use 8 mm Full Width at Half Maxima (FWHM) Gaussian kernel for spatial smoothing.
Measures of brain functional activity
In this study, to quantify the characteristics of brain functional activity, functional activity intensity, local and global functional connectivity were utilized to characterize the abnormal brain functional activity of autistic patients. In particular, ALFF was mainly used to reveal the intensity of the low-frequency BOLD signal in the region of spontaneous activity frequency between 0.01 and 0.1, which is generally considered to be a neural signal. The local functional connectivity model is characterized by ReHo to describe the trend of consistency between the time series of a given voxel and the time series of its 26 nearest voxels. Additionally, the global functional connectivity model was measured by DC, which describes the correlation coefficients between a given voxel time series and the whole brain voxel time series, and calculated as the mean of the correlation coefficients between all other voxels with a correlation coefficient greater than 0.25.
Intergroup analysis
To eliminate the “scanner effect” caused by the use of different MRI scanners and acquisition protocols at data acquisition sites, the variation which may affect the characterization of imaging features and lead to spurious findings need to be addressed. To prevent the interference of such unnecessary sources of variation in the experiment, we utilized ComBat to remove site effects while using age as a covariable. After controlling for age differences, the two-sample t-test was used to determine the whole-brain differences in ALFF, DC, and ReHo between ASD and HC. Statistical t-map were obtained, with a minimum cluster size >20.
Harmonization decoding of functional cognitive patterns
After identifying heterogeneous brain regions in ALFF, ReHo, and DC associated with autism, we used the Neurosynth online analysis tool2 to analyze the cognitive functions most relevant to these heterogeneous brain regions. Specifically, we divided FDR-corrected t-maps into two groups: those showing increased functional activity (positive) and those showing decreased functional activity (negative), which were input into Neurosynth. Finally, the top 10 behavioral cognition terms with the greatest correlation were selected as typical representatives of regional functions.
Prediction of clinical evaluation using brain functional activity
To evaluated whether functional activity in heterogeneous brain regions predicts clinical social performance in patients with ASD, we utilized the functional activity characteristics of these heterogeneous brain regions, as well as the data from Autism Diagnostic Interview-Revised (ADI-R) and Autism Diagnostic Observation Scale (ADOS) as input for the support vector regression model. Age is taken as the covariable of the training model, and our estimated probability is verified for accuracy by the leave-one out cross-validation method. Finally, the SVC training model’s performance was assessed using the 10-fold cross-validation approach, which employed the functional activity values of the identified heterogeneous brain areas as the feature input.
Matching of brain regions to gene expression estimates
In this study, we utilized the whole human brain gene expression dataset provided by the Allen Human Brain Atlas (AHBA),3 which collected 3,702 different parts of brain tissue (Age = 42.50 ± 13.38 years old; Male/female = 5/1) from six healthy individuals post-mortem. Abagen was used to re-annotate gene samples and match gene expression with the brain region of Brodmann Atlas.4 The following steps were taken: (1) the microarray expression matrix was re-annotated and gene probes of invalid Entrez-ID were removed; (2) the probes with expression intensity lower than the background value were discarded if they were present in less than 50% of the donor samples. After filtration, a total of 31,569 gene probes were obtained; (3) the probe whose regional expression pattern is most consistent with that of RNA-seq is selected and used in case multiple gene probes correspond to the same gene. This is determined by calculating the Spearman rank correlation between the microarray expression of each probe and the RNA-seq expression data of the corresponding gene is calculated, and selecting the probe with the highest correspondence.
Associations between functional differences and gene expression profiles
Based on the correlation analysis between the functional activities of heterogeneous brain regions and gene transcription, PLS regression5 was utilized to identify different expression patterns of weighted linear combinations of all genes. Specifically, in the experiment, gene expression transcription profiles of specific brain regions were used as predictive variables, while functional activity characteristics of specific brain regions were used as response variables. To correct for spatial autocorrelation of the functional feature data, we used permutation analysis to reorder the functional feature data (n = 10,000) to test the statistical significance of the maximum explainable variance information of the original arrangement in each PLS dimension (Li et al., 2021). Subsequently, to verify the spatial similarity between the weighted gene expression pattern obtained by PLS and brain functional characteristics, displacement analysis (n = 10,000) was performed to reorder the weighted gene expression pattern.6 Finally, Bootstrapping was used to perform random sampling on the gene weights of the significant dimensions of PLS results to estimate the gene variability, calculate the ratio of PLS gene weights to the standard error of gene variability, and then sequence the final scores to get the genes significantly related to brain functional activities.
In addition, we matched significant gene weights obtained through PLS to brain regions to obtain weighted gene expression maps.
Expression analysis of autism-related genes
To further analyze the heterogeneous expression of genes between males and females, we collected 90 identified ASD-related genes from AHBA7 and downloaded whole-brain expression maps of these genes from Neurosynth.8 The gene expression map was then divided into 82 subregions using the Brodmann template, and the average expression levels of 90 risk genes in each subregion were calculated and standardized. The co-expression patterns between genes were analyzed using Pearson correlation. To further verify the significance of co-expressed gene expression patterns, we further analyzed the spatial expression patterns of 1,028 ASD-related genes identified in SFARI.9 In addition, gene temporal-specific expression analysis was used to characterize the expression intensity locus of genes of interest collected from 50 to 30,000 days in 11 neocortical areas.10
Gene enrichment analysis
To further explore the biological function of the specifically expressed genes and their unique genetic pathways, we employed Metaspace analysis11 for gene enrichment analysis. The top 5% of the genes with the weight value after PLS sequencing were included in the gene enrichment analysis. The significance threshold of gene pathway and process enrichment analysis was 0.05, and the results were significantly corrected by the FDR-BH method. Tissue-specific expression analysis tools (TSEA)12 were used to further determine whether ASD-related genes found by PLS were specifically expressed in different human tissues. A specific expression index (PSI = 0.05, 0.01, 0.001, and 0.0001) was used to determine the significance level of tissue-specific expression of the gene.
Results
Demographic characteristics
A total of 461 subjects ranging 5 from 56 years of age were enrolled from six independent sites in this study, namely PITT, YALE, NYU I, CALTECH, SDSU, and EMC. Supplementary material details the specifics and scanning parameters of each site. Notably, there was no significant difference in age between the ASD and HC groups at each site (for details, please refer to Table 1).
Heterogeneous brain regions associated with ASD
The neuroimaging analysis of this study revealed distinct alterations in brain functional activity, similarity of local functional activity, and global functional activity among females and males with autism. The group differences are shown in Table 2; Figure 1.
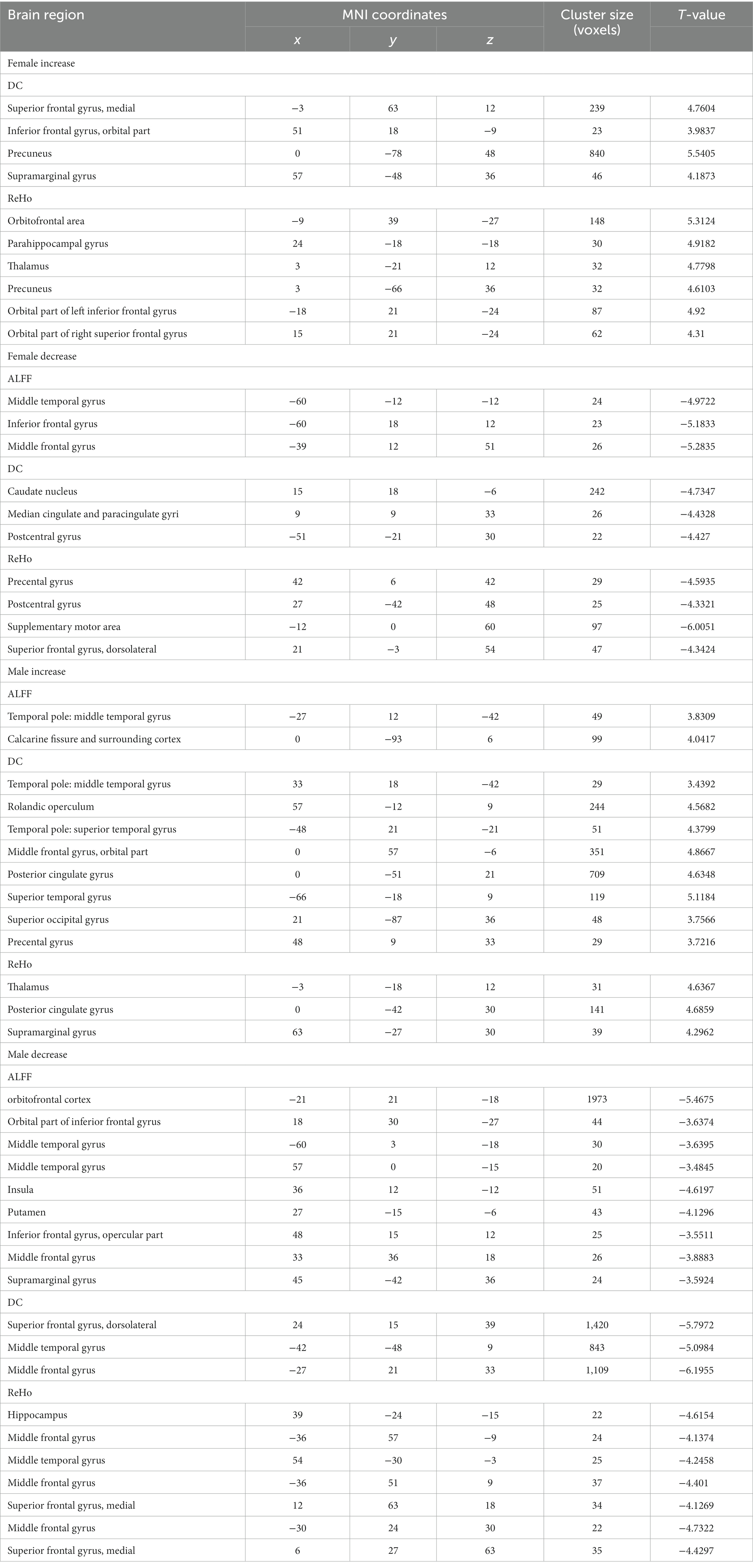
Table 2. Significant group differences in amplitude of low-frequency fluctuation (ALFF), local regional homogeneity (ReHo), and degree centrality (DC) between children with ASD and HC, and between adults with ASD and HC.
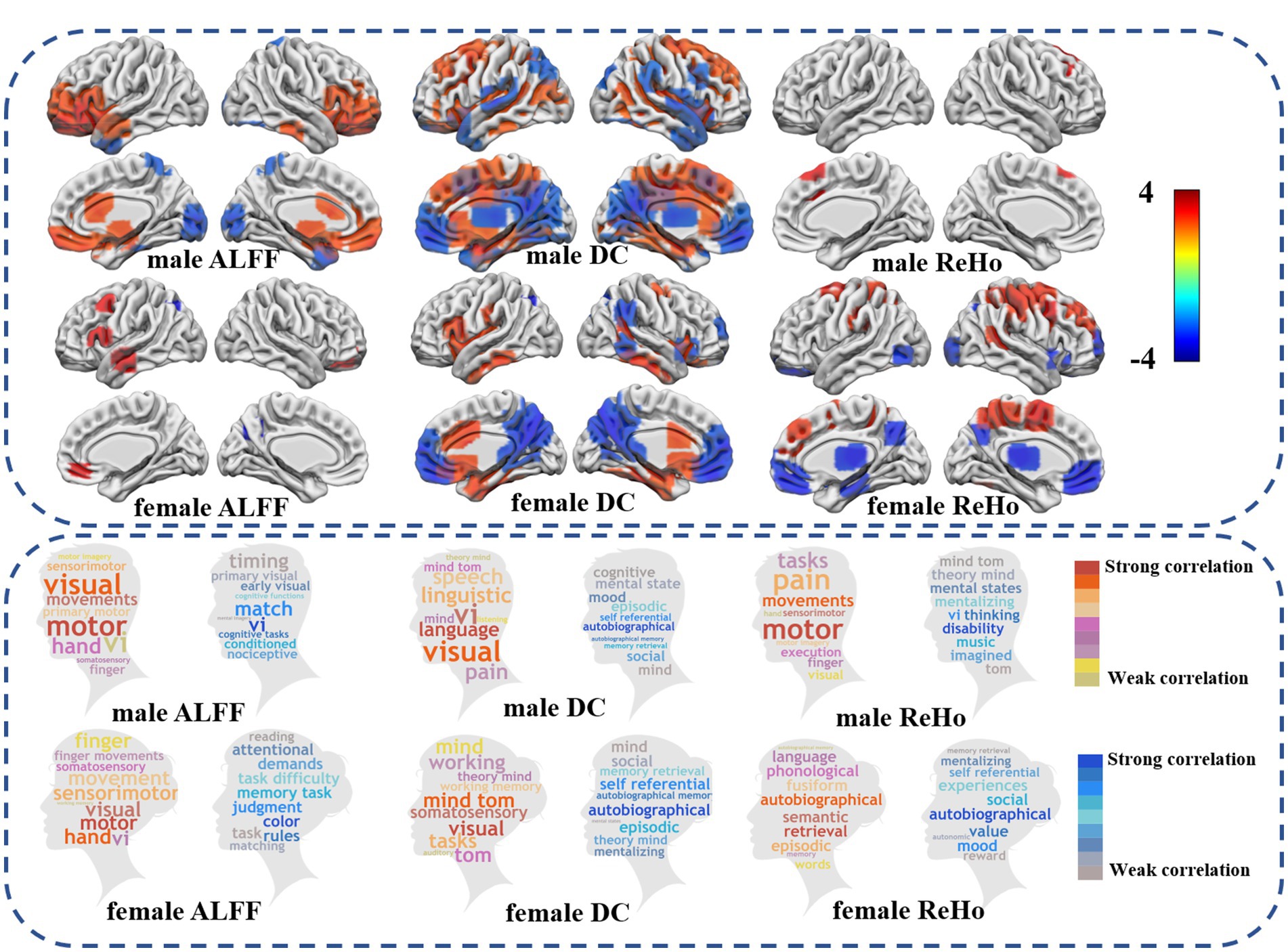
Figure 1. Differences in fMRI between autism spectrum disorders and healthy controls. The upper half showed differences between ASDs and HC in low frequency brain functional activity, global functional connectivity, and local functional connectivity similarity (FDR p < 0.05). The lower part of the word cloud map shows the difference between ASD and HC and the brain regions involved in the cognitive function of the brain. Warm colors show an increase in the functional activity of ASD compared with HC, while cool colors show a decrease in the functional activity of ASD compared with HC.
ALFF: ASD vs. HC
Specifically, compared with HC, male individuals with autism exhibited decreased amplitude of ALFF in various brain regions, including bilateral prefrontal lobes, bilateral thalamus, bilateral middle cingulate gyrus, left anterior superior temporal gyrus, right inferior temporal gyrus cortex, and increased ALFF in bilateral somatosensory association cortex, bilateral left temporal pole cortex. Conversely, in female patients with ASD, ALFF was increased in the left medial frontal gyrus, medial temporal gyrus, inferior frontal gyrus of insular cortex, right orbital medial frontal gyrus, and left suproccipital gyrus.
DC: ASD vs. HC
As compared to HC, male individuals with ASD showed decreased DC in bilateral cingulate cortex, middle occipital gyrus, and dorsolateral prefrontal cortex. On the other hand, DC was increased in bilateral medial prefrontal cortex, bilateral temporal pole, superior temporal gyrus, posterior cingulate gyrus, thalamus, left suproccipital gyrus, and right superior limbic gyrus. Conversely, female individuals with ASD displayed decreased DC in bilateral inferior temporal gyrus, anterior cingulate cortex, medial cingulate cortex, and temporal pole, while DC was increased in bilateral medial prefrontal cortex, posterior cingulate cortex, right superior limbic gyrus, inferior temporal gyrus and lateral prefrontal cortex.
ReHo: ASD vs. HC
As compared to HC, male individuals with ASD showed reduced ReHo in bilateral medial superior frontal gyrus and right medial frontal gyrus. Conversely, female individuals with ASD exhibited decreased ReHo in dorsolateral prefrontal cortex, and increased in the bilateral medial prefrontal cortex, thalamus, precuneus cortex, occipital lobe, and right inferior temporal gyrus.
Correlation between functional activity characteristics and clinical social performance
The results of SVR prediction model indicated that several the neuroimage features in both male and female individuals could serve as significant predictors of clinical performance scores. Moreover, the specific brain regions of ALFF recognition in males and females could be found to be better fit the clinical scores of ADI-R-ONSET (female ALFF: p = 8.78E-07 mean = 1.28 MSE =3.41; male ALFF: p = 4.67E-10 mean = 1.56 MSE =3.26), p is defined as the significance of the maximum explicable variance information of random permutations in 10,000 random PLS replacement tests. p-perm was defined as the significance of the correlation coefficient between the weight value of genes and the value of brain functional activity under the n dimension in 10,000 random displacement tests (please refer to Table 3 for details). It is worth mentioning that we also found that certain brain regions identified by ReHo were able to better distinguish between female individuals with ASD and HC (mean ACC = 93.9%, mean AUC = 0.95) (for details, please refer to Table 4).
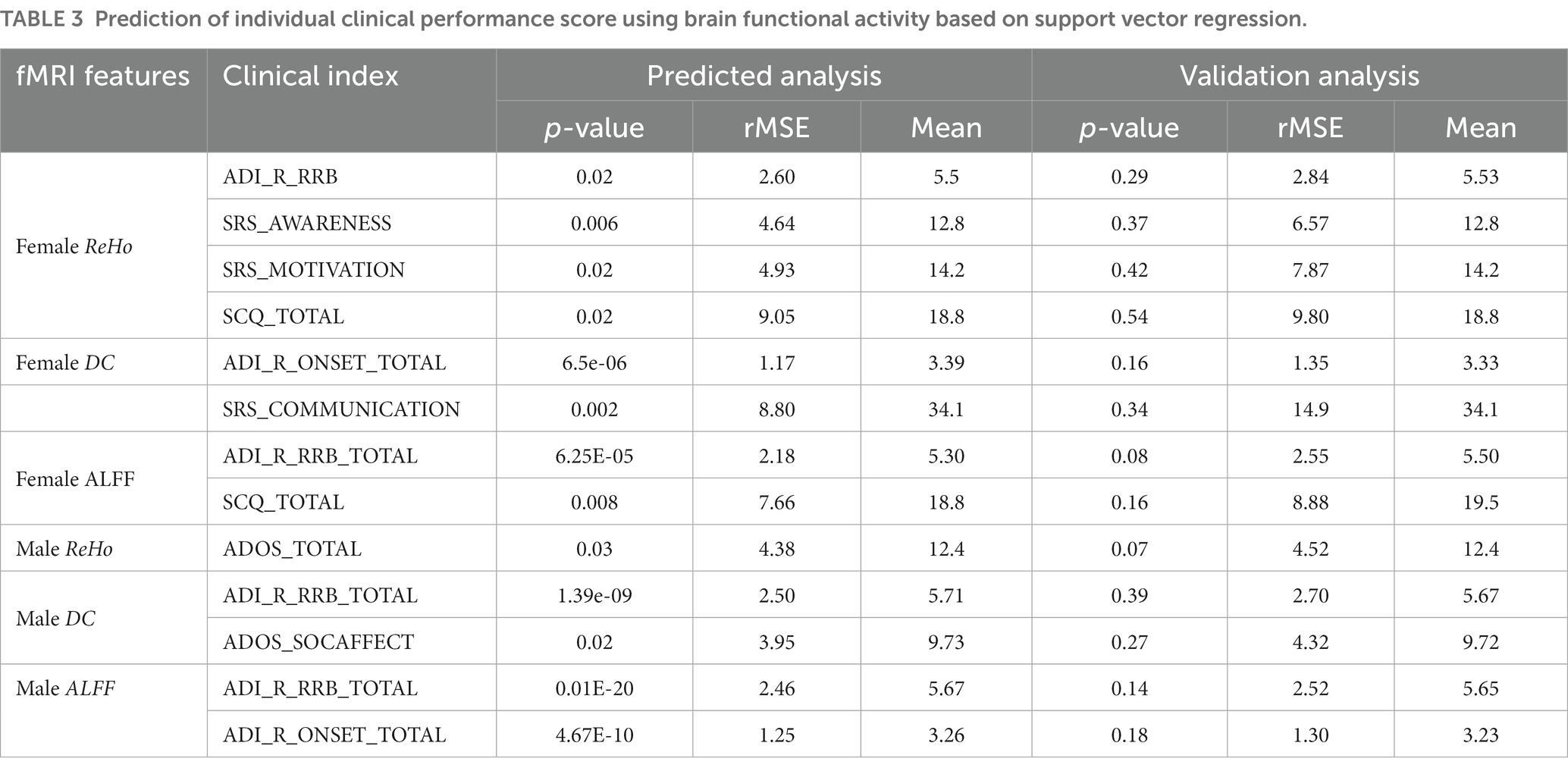
Table 3. Prediction of individual clinical performance score using brain functional activity based on support vector regression.
Correlation between abnormal brain functional activity and gene expression profile
The whole brain transcriptome data set provided by AHBA was used to construct the “bridge” between brain mapping and gene expression. Since only 2 out of 6 donors in AHBA provided samples from the right hemisphere of the brain, we restricted our analysis to the left hemisphere data only. After rematching the gene transcription profile using the Brodmann atlas, a brain region-gene transcription expression matrix was finally generated (the size was 40 region *13561 gene expression; gene samples were not collected in BA29 of the Brodmann atlas, therefore only the gene expression values of 40 brain regions in the left brain were utilized).
Next, PLS regression analysis was used to characterize the spatial similarity between ASD heterogeneous brain functional activity and gene expression profiles. The components with significant results after two spatial autocorrelation corrections were taken as functional activity features that could effectively explain the variance information of total gene expression. Specifically, the following components yielded noteworthy outcomes: (Male ReHo: PLS1 explains 43.7% variance, p = 0.003, p-perm = 0; Male ALFF: PLS2 explained 25.3% variance, p = 0.03, p-perm = 0.0007; Male DC: PLS2 explained 27.1% variance, p = 0.02, p-perm = 0.00005; Female ReHo: PLS2 explained 30.6% variance, p = 0.02, p-perm = 0.0001; Female ALFF: PLS4 interpretation 16.7% variance, p = 0.003, p-perm = 0.002; Female DC: PLS1 explained 36.8% variance, p = 0.02, p-perm = 0) (Figures 2, 3).
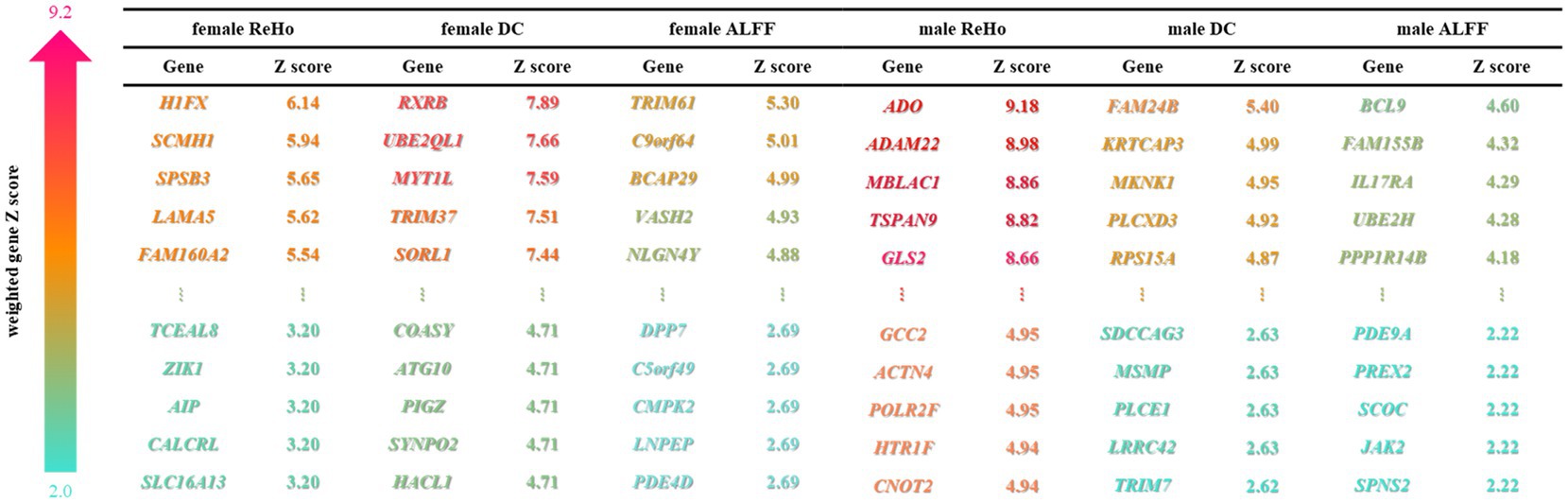
Figure 2. Weighted genes associated with autism. PLS recognizes the top 5% weighted genes of male and female brain functional characteristics, and sorts them according to gene weight Z-scores, and displays the top 5 and the bottom 5 genes of Z-scores. The depth of the color represents the Z-score.
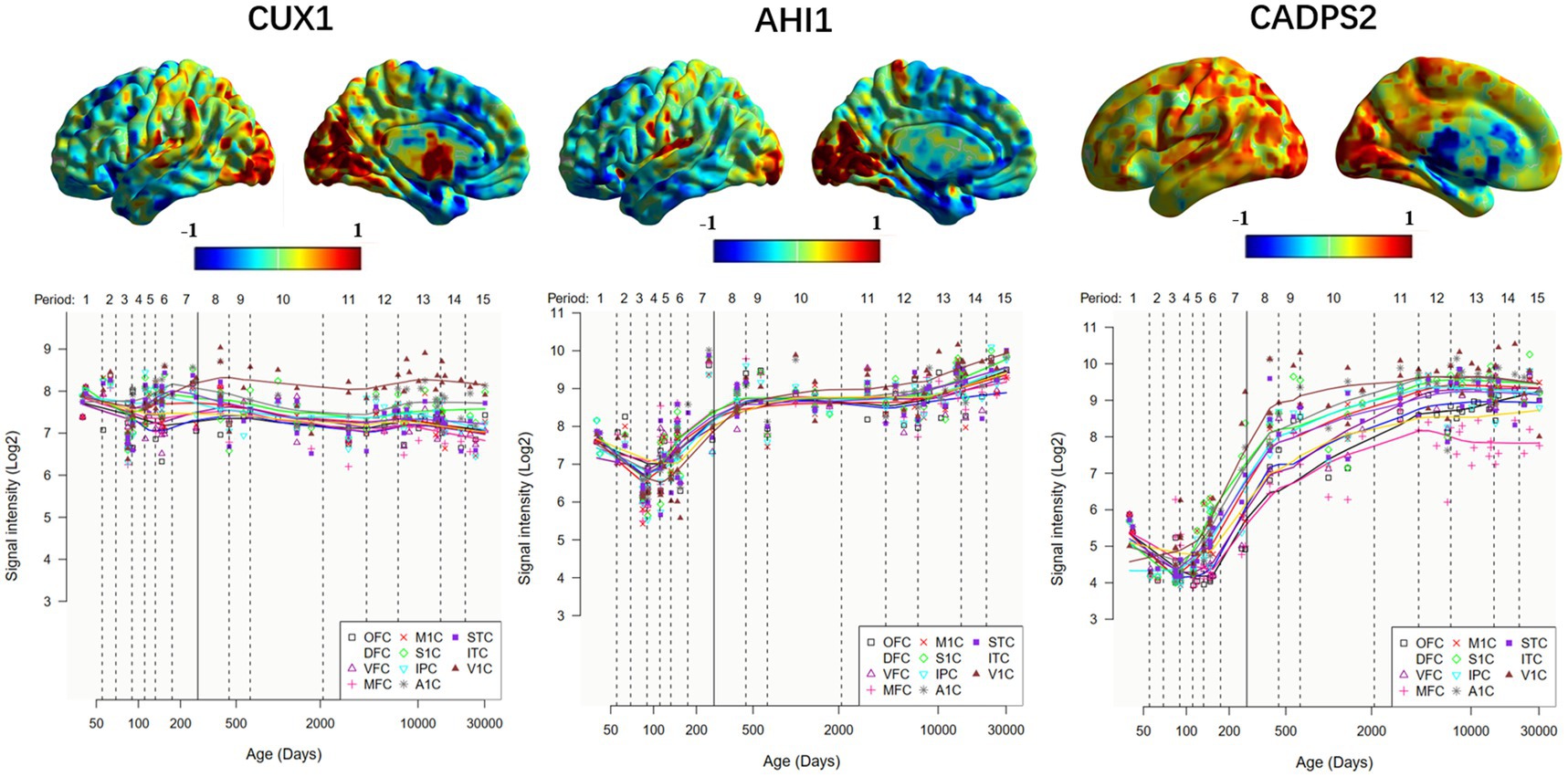
Figure 3. Expression of ASD risk genes in the left hemisphere and temporal -specific distribution in 11 cerebral cortices. The horizontal axis represents time (days); the vertical axis represents gene expression level, and different colored broken lines represent gene expression in different neocortical regions. OFC, orbital prefrontal cortex; DFC, dorsolateral prefrontal cortex; VFC, ventrolateral prefrontal cortex; MFC, medial prefrontal cortex; M1C, primary motor (M1) cortex; S1C, primary somatosensory (S1) cortex; IPC, posterior inferior parietal cortex; A1C, primary auditory (A1) cortex; STC, superior temporal cortex; ITC, inferior temporal cortex; V1C, primary visual (V1) cortex.
The similarity and personality between PLS weighted distribution of genes in male and female ASD bring another mechanism to our attention. Specifically, male individuals with ASD exhibited an evident gradient change in the whole-brain weighted gene expression map of ALFF, DC, and ReHo, whereas female individuals with ASD displayed an insignificant gradient change and even an opposite change in some regions associated with ALFF, DC, and ReHo (Figure 4).
Enrichment analysis of ASD-related genes
In order to further evaluate the biological function ontology terms of genes related to ASD brain imaging characteristics, A total of 631 genes with a gene weight among the top 5% were input into the Metaspace website. We found that the genes associated with female ALFF and DC were involved in the synaptic structure and synaptic membrane of neurons, whereas those associated with male ReHo are involved in the transmembrane transporter activity of inorganic cations and other typical important processes (Figures 5–7). In addition, tissue-specific expression analysis was performed on the identified related genes in males and females, revealing that the genes associated with ASD, identified by female DC and male ReHo, exhibited a significantly specific expression pattern in the brain (Figure 5).
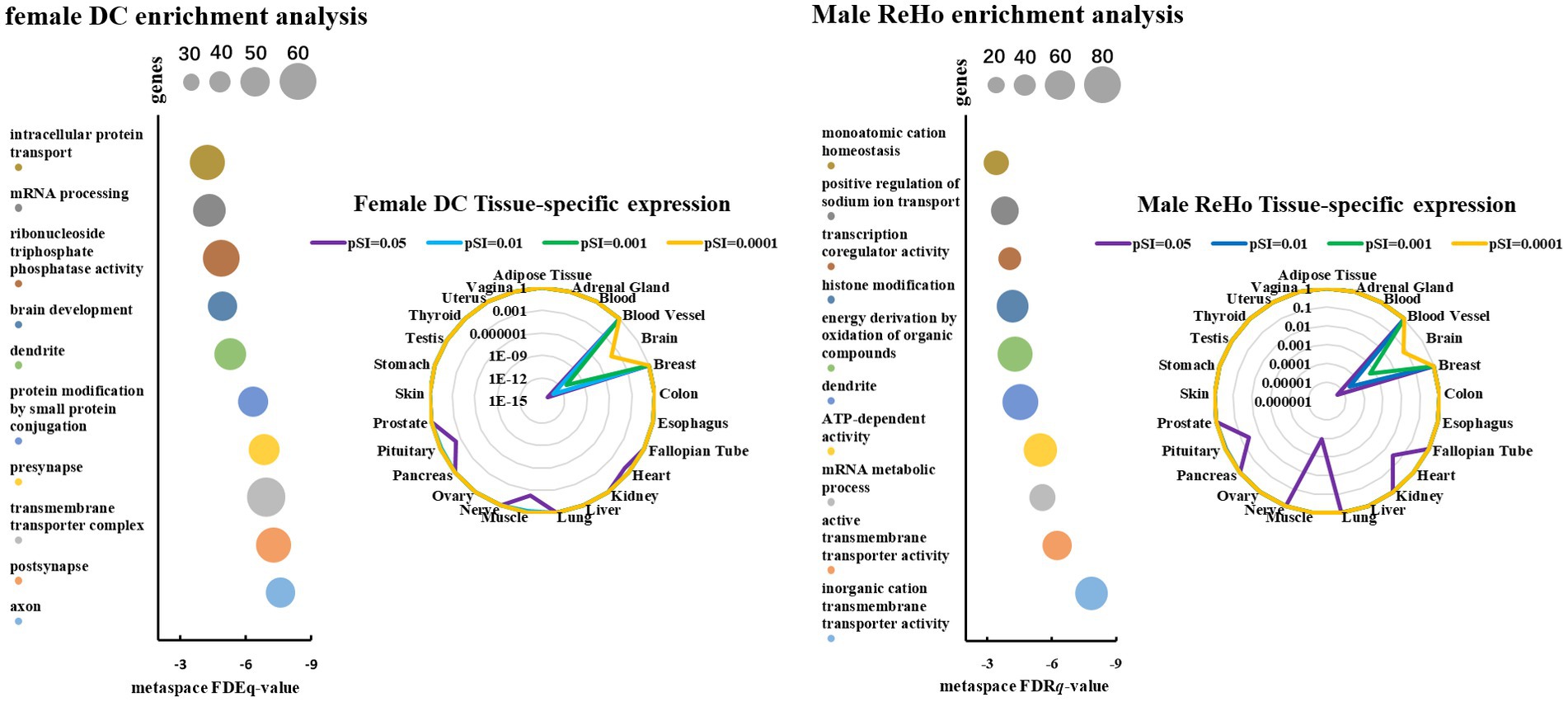
Figure 5. The scatter plot represents the genetic ontology term enrichment analysis of PLS, and the size of the circle represents the number of genes involved in the term. The radar map shows the specific expression levels of weighted genes in 26 tissues.
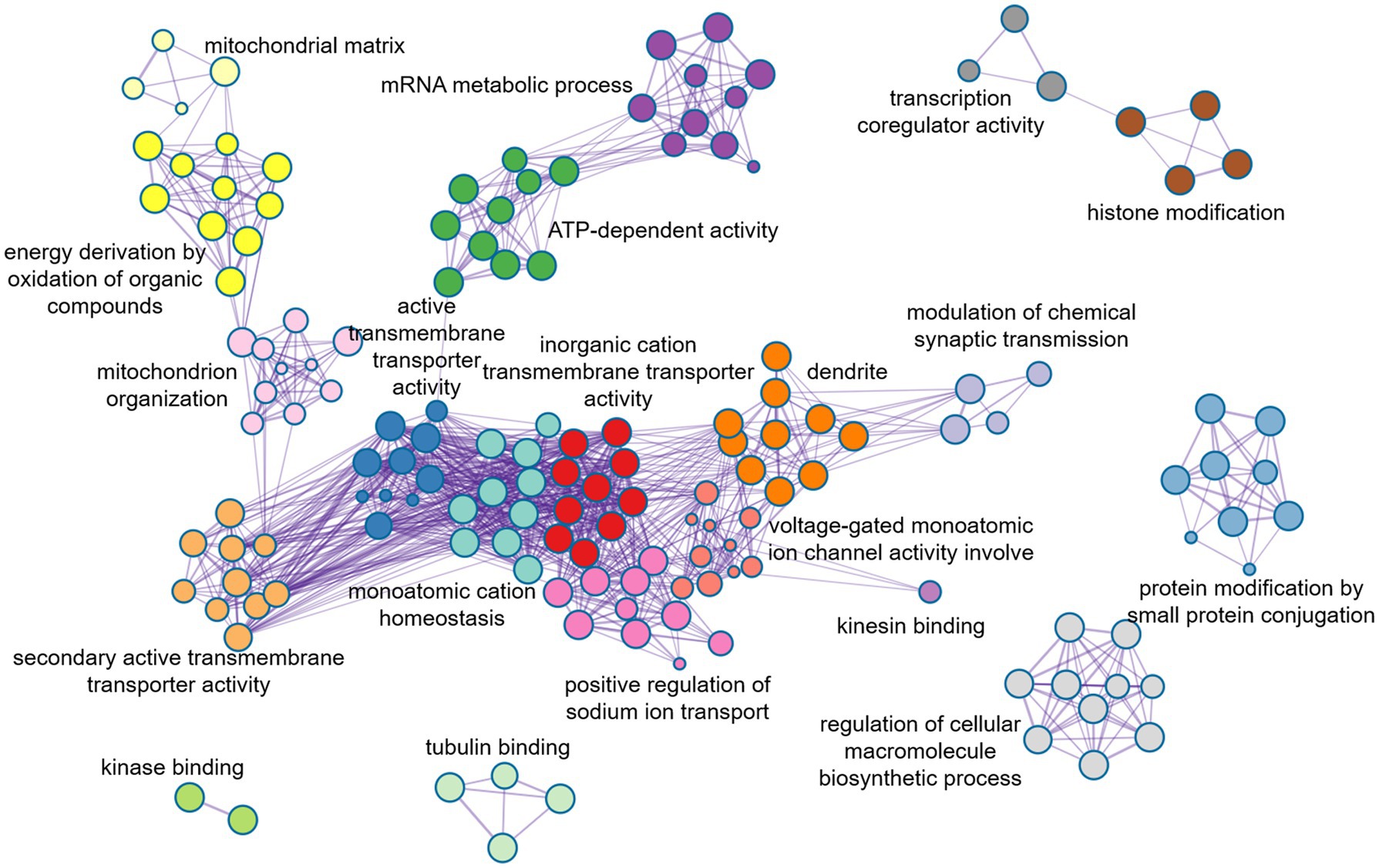
Figure 6. Metaspace was used to visualize the enrichment patterns of weighted genes, showing the link between molecular function and molecular mechanism in male ReHo.
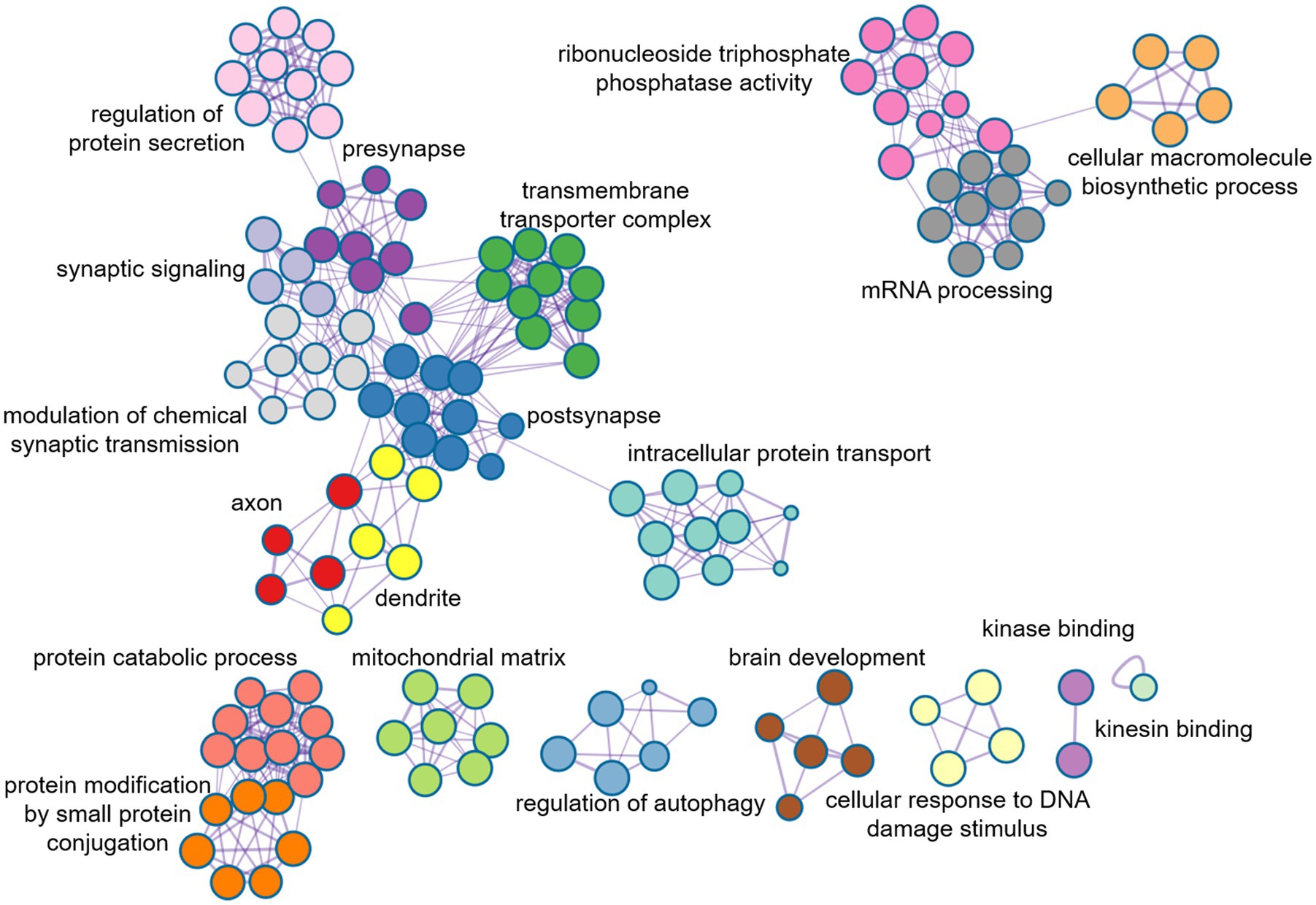
Figure 7. Metaspace was used to visualize the enrichment patterns of weighted genes, showing the link between molecular function and molecular mechanism in female DC.
Discussion
In this study, we aimed to explore alterations in neuroimaging and genetic transcription patterns in ASD patients, stratified by gender. To achieve the task, we utilized multiple-sites resting state fMRI analyses and employed ComBat to remove the “scanner effect” by a large sample data set. Through the study, we revealed multiple heterogeneous cerebral cortical regions with distinct spatial patterns of brain activity in male and female patients. Additionally, we also discovered that the functional activity of the heterogeneous brain regions identified in female patients was more effective in distinguishing ASD from HC. It is worth mentioning that the transcriptome-neuroimaging association analysis indicated that sex differences lead to both similarities and differences in gene expression patterns.
Overall, our findings suggest that atypical spontaneous brain activity in autistic patients is affected by gender, and varies in heterogeneous brain regions. Investigating the gene expression patterns in distinct brain regions may further prove to be a crucial approach in decoding the physiological origins of autism.
As is well known, brain is composed of various interconnected regions with regulatory mechanisms that form complex networks. One such network is the default mode network (DMN), which remains active even when people are not engaged in any cognitive tasks. The DMN is also involved in emotional processing, memory, emotional processing, and other cognitive tasks (Assaf et al., 2010; Lynch et al., 2013).
The rs-fMRI was used to dissect the heterogeneous changes in DMN brain regions caused by gender differences in ASD and we found that male and female patients showed heterogeneous changes in some core brain regions of the DMN. Our analysis of DMN networks in both male and female autistic patients revealed that male patients had higher low-frequency functional activity in the bilateral ventromedial prefrontal cortex than healthy controls, whereas female patients showed increased low-frequency functional activity in the right ventromedial prefrontal cortex. We observed that male and female have the similar global functional connectivity changes in the bilateral medial prefrontal cortex, the bilateral precuneus cortex, the bilateral posterior cingulate cortex, and the bilateral precuneus cortex. However, female do not show the same global functional connectivity increases in the dorsomedial prefrontal cortex as males. Based on the local similarity of functional connectivity, male and female ASD patients only exhibited similar changes in the dorsolateral prefrontal cortex, while female ASD patients have heterogeneous changes in DMN core regions, such as the bilateral ventromedial prefrontal cortex and bilateral precuneus. Overall, we found significant differences between males and females in the default mode network, particularly in the bilateral ventromedial prefrontal cortex, bilateral dorsolmedial prefrontal cortex, and bilateral precuneus. It is note that the medial ventromedial prefrontal cortex is involved in emotional regulation, social behavior, decision making, and cognitive control, and its dysfunction is considered to be a key factor leading to emotional anxiety disorder (Buhle et al., 2014; Motzkin et al., 2015). The dorsomedial prefrontal cortex shows relatively synaptic sensitivity in decision-making, reward, and pain processing in the brain (Hauser et al., 2015). The DMPFC and VMPFC are involved in different cognitive networks. The VMPFC forms a reward network with the amygdala and striatum, while the DMPFC forms a cognitive control network with the dorsal anterior cingulate cortex and posterior cingulate cortex (Cai et al., 2016; Wilson et al., 2018). Finally, the precuneus plays a crucial role in visuospatial processing, facilitate visual information processing in the brain, identifies the spatial position of objects, and is involved in the maintenance of working memory as well as the encoding and extraction of long-term memory (St Jacques et al., 2017; Schott et al., 2019).
It is worth mentioning that the functional activities of the different brain regions found in female with ASD can effectively correlate with some clinical assessment scores, such as SRS_AWARENESS, SRS_MOTIVATION, SRS_COMMUNICATION, etc. It suggests that these different brain regions may be involved in controlling behavioral cognitive functions such as consciousness, behavior, communication, etc. However, Next, the division of ASDs and HC in male using the female differences was unsatisfactory, which seems to indicate that the cerebral cortical network, consisting of the medial prefrontal cortex, middle temporal gyrus, fronto-parietal junction, thalamus, precuneus, and occipital lobe, is significantly different between male patients and female patients. Together, these regions affect the brain’s emotional regulation, memory processing, visual–spatial processing, social behavior, and cognitive control (Smith et al., 2013; Gratton et al., 2018).
Approximately one-third of the 20,000 or so genes that make up the human genome are active in the brain. They affect the development and specific functions of the brain, and ultimately our emotions, behaviors, and thoughts. The changes in brain functional activities in autism may be affected by various factors, such as external environmental changes, genetic factors (Cross-Disorder Group of the Psychiatric Genomics et al., 2013; De Rubeis et al., 2014), and molecular neuron changes. In this study, PLS method was utilized to conduct a spatial correlation analysis between gene transcriptomics and neuroimaging, and further to identify weighted genes associated with heterogeneous brain regions of ASDs. We found that the genes identified by female DC were mainly involved in the formation of neuronal synapses and the transmission of signals between synapses, while the genes identified by male ReHo were mainly involved in the controlling nerve potential signals, such as inorganic cationic transmembrane transporters, and regulating cationic transport. Additionally, the changes in neuronal potential were caused by the transmembrane transport of cations through cell membranes. Notably, the disruption of the neuronal excitation-inhibition balance mechanism is believed to be one of the important reasons for the abnormal neuronal activity in autism (Canitano and Pallagrosi, 2017; Sohal and Rubenstein, 2019). Moreover, we also found that these genes identified in male ReHo were involved in the mitochondrial matrix and influenced the secretion of adenosine triphosphate (ATP) in mitochondria. This unique mechanism may play an important role in the pathology of autism, as mitochondrial dysfunction is highly correlated with the severity of ASD symptoms. However, exactly how mitochondria are involved in autism remains unclear (Minshew et al., 1993; Rossignol and Frye, 2012).
The hierarchical structure of human cerebral cortex is closely related to its function. In this structure, different layers of neurons exhibit different patterns of gene expression, thus further demonstrating the tight link between structure and function (Maynard et al., 2021). In our study, we observed the marked difference in the whole-brain weighted genes between males and females with autism. We further noticed that there was a significant gradient change in the expression of weighted genes in the left brain of males, that is, from the frontal lobe to the occipital lobe, the weighted expression of genes shifted from low level to high level, whereas this change was not significant in females, and even reversed in parts of the cerebral cortex. It seems to prove that weighted genes are not evenly distributed in the cerebral cortex of ASD patients of different genders (Tamagawa et al., 2017).
The weighted genes we obtained were significantly associated with changes in abnormal brain regions, further identifying some ASD risk genes that are associated with changes in abnormal brain functional activities. The differences in the expression levels of different genes across different brain regions reflect the interaction or regulatory relationship between genes. Among these, CUX1 and AHI1 gene have high similarity in the whole brain gene expression level. CUX1 mutation may lead to mild intellectual disability and has familial heritability (Platzer et al., 2018). In Joubert Syndrome, the expression of AHI1 was significantly increased in the crossing axons of corticospinal tract and superior cerebellar peduncle (Ferland et al., 2004). It seems to suggest that the mutations in these two genes are common agents of certain mental illnesses. In addition, we note that CADPS2 is associated with ASD in abnormal brain function activity in both males and females, and that CADPS2 mediated neurotrophin factor release disorders contribute to increased susceptibility to autism (Sadakata et al., 2007a,b).
The expression of different genes in the development of human brain varies across different stages. Some genes are highly expressed in the critical period of the development of human organs, such as the embryonic period and the infant period, but the expression level is gradually reduced during adulthood. These difference in gene expression in different periods reflects the dynamic changes of the gene regulatory network (Franz et al., 2005; Glass et al., 2013). Since autism spectrum disorders are highly heritable and can be observed as early as childhood (Primiani et al., 2014; Christensen et al., 2016; Pierce et al., 2019), tracking the expression of specific genes at different times and studying their functions and regulation can provide better insights into the pathological mechanisms of autism spectrum disorders.
Limitations
The current study examines the gender discrepancies in autism, without categorizing subjects according to their chronological age. Due to the absence of longitudinal developmental information, it is uncertain whether the diverse neural regions identified throughout one’s lifespan persist, thus introducing the potential for age-related effects on the results.
Data availability statement
The original contributions presented in the study are included in the article/Supplementary material, further inquiries can be directed to the corresponding author.
Ethics statement
The data used in this study is obtained from the open-source dataset from ABIDE. The ethical considerations pertaining to human participants in this study were meticulously examined and approved by the Institutional Review Board. Written informed consent was obtained from all patients/participants prior to their involvement in the study. For instance, The studies involving human participants were reviewed and approved by Ethical approval was obtained from the St. James’s Hospital/AMNCH (ref: 2010/09/07) and the Linn Dara CAMHS Ethics Committees (ref: 2010/12/07). Written informed consent to participate in this study was provided by the participants’ legal guardian/next of kin.
Author contributions
YL developed the overall study objectives, designed the methodology, performed most of the experimental analysis, and wrote the manuscript. RL preprocessed experimental data and assisted in some experiments and manuscript writing. NW and JHG assisted in collating the data and interpreting the results. JJG reviewed and revised the manuscript. All authors contributed to the article and approved the submitted version.
Funding
This work was supported by the National Natural Science Foundation of China (Nos. 61701078 and 62276049), Sichuan Province Science and Technology Support Program (Nos. 2019YJ0193, 2021YFG0126, and 2022YFS0180), the Medico-Engineering Cooperation Funds from University of Electronic Science and Technology of China (No. ZYGX2021YGLH014).
Acknowledgments
We acknowledge the ABIDE for providing open access to fMRI data, and the global scientific researchers and subjects who participate in the data collection. We are also grateful to the Allen Institute for Brain Science for providing the brain gene expression dataset and the corresponding staff.
Conflict of interest
The authors declare that the research was conducted in the absence of any commercial or financial relationships that could be construed as a potential conflict of interest.
Publisher’s note
All claims expressed in this article are solely those of the authors and do not necessarily represent those of their affiliated organizations, or those of the publisher, the editors and the reviewers. Any product that may be evaluated in this article, or claim that may be made by its manufacturer, is not guaranteed or endorsed by the publisher.
Supplementary material
The Supplementary material for this article can be found online at: https://www.frontiersin.org/articles/10.3389/fnins.2023.1203690/full#supplementary-material
Footnotes
1. ^http://fcon_1000.projects.nitrc.org/indi/abide/
3. ^http://human.brain-map.org
4. ^https://abagen.readthedocs.io/en/stable/
5. ^https://github.com/helab207/Alterations-in-Connectome-Dynamics-in-ASD-main
6. ^https://github.com/frantisekvasa/rotate_parcellation/tree/master/Matlab
7. ^https://human.brain-map.org/microarray/search
8. ^https://neurosynth.org/genes/
9. ^https://gene.sfari.org/database/human-gene/
10. ^https://hbatlas.org/pages/hbtd
References
Assaf, M., Jagannathan, K., Calhoun, V. D., Miller, L., Stevens, M. C., Sahl, R., et al. (2010). Abnormal functional connectivity of default mode sub-networks in autism spectrum disorder patients. Neuroimage 53, 247–256. doi: 10.1016/j.neuroimage.2010.05.067
Auyeung, B., Baron-Cohen, S., Ashwin, E., Knickmeyer, R., Taylor, K., Hackett, G., et al. (2009). Fetal testosterone predicts sexually differentiated childhood behavior in girls and in boys. Psychol. Sci. 20, 144–148. doi: 10.1111/j.1467-9280.2009.02279.x
Buhle, J. T., Silvers, J. A., Wager, T. D., Lopez, R., Onyemekwu, C., Kober, H., et al. (2014). Cognitive reappraisal of emotion: a meta-analysis of human neuroimaging studies. Cereb. Cortex 24, 2981–2990. doi: 10.1093/cercor/bht154
Cai, W., Chen, T., Ryali, S., Kochalka, J., Li, C. S., and Menon, V. (2016). Causal interactions within a frontal-cingulate-parietal network during cognitive control: convergent evidence from a multisite-multitask investigation. Cereb. Cortex 26, 2140–2153. doi: 10.1093/cercor/bhv046
Canitano, R., and Pallagrosi, M. (2017). Autism spectrum disorders and schizophrenia spectrum disorders: excitation/inhibition imbalance and developmental trajectories. Front. Psych. 8:69. doi: 10.3389/fpsyt.2017.00069
Chen, P., Yao, H., Tijms, B. M., Wang, P., Wang, D., Song, C., et al. (2022). Four distinct subtypes of Alzheimer's disease based on resting-state connectivity biomarkers. Biol. Psychiatry 93, 759–769. doi: 10.1016/j.biopsych.2022.06.019
Christensen, D. L., Bilder, D. A., Zahorodny, W., Pettygrove, S., Durkin, M. S., Fitzgerald, R. T., et al. (2016). Prevalence and characteristics of autism spectrum disorder among 4-year-old children in the autism and developmental disabilities monitoring network. J. Dev. Behav. Pediatr. 37, 1–8. doi: 10.1097/DBP.0000000000000235
Cross-Disorder Group of the Psychiatric GenomicsLee, S. H., Ripke, S., Neale, B. M., Faraone, S. V., Purcell, S. M., et al. (2013). Genetic relationship between five psychiatric disorders estimated from genome-wide SNPs. Nat. Genet. 45, 984–994. doi: 10.1038/ng.2711
De Rubeis, S., He, X., Goldberg, A. P., Poultney, C. S., Samocha, K., Cicek, A. E., et al. (2014). Synaptic, transcriptional and chromatin genes disrupted in autism. Nature 515, 209–215. doi: 10.1038/nature13772
Di Martino, A., Yan, C.-G., Li, Q., Denio, E., Castellanos, F. X., Alaerts, K., et al. (2014). The autism brain imaging data exchange: towards a large-scale evaluation of the intrinsic brain architecture in autism. Mol. Psychiatry. 19, 659–667. doi: 10.1038/mp.2013.78
Du, X., Wei, X., Ding, H., Yu, Y., Xie, Y., Ji, Y., et al. (2023). Unraveling schizophrenia replicable functional connectivity disruption patterns across sites. Hum. Brain Mapp. 44, 156–169. doi: 10.1002/hbm.26108
Ferland, R. J., Eyaid, W., Collura, R. V., Tully, L. D., Hill, R. S., Al-Nouri, D., et al. (2004). Abnormal cerebellar development and axonal decussation due to mutations in AHI1 in Joubert syndrome. Nat. Genet. 36, 1008–1013. doi: 10.1038/ng1419
Fortin, J. P., Cullen, N., Sheline, Y. I., Taylor, W. D., Aselcioglu, I., Cook, P. A., et al. (2018). Harmonization of cortical thickness measurements across scanners and sites. Neuroimage 167, 104–120. doi: 10.1016/j.neuroimage.2017.11.024
Franz, H., Ullmann, C., Becker, A., Ryan, M., Bahn, S., Arendt, T., et al. (2005). Systematic analysis of gene expression in human brains before and after death. Genome Biol. 6:R112. doi: 10.1186/gb-2005-6-13-r112
Glass, D., Viñuela, A., Davies, M. N., Ramasamy, A., Parts, L., Knowles, D., et al. (2013). Gene expression changes with age in skin, adipose tissue, blood and brain. Genome Biol. 14:R75. doi: 10.1186/gb-2013-14-7-r75
Gratton, C., Laumann, T. O., Nielsen, A. N., Greene, D. J., Gordon, E. M., Gilmore, A. W., et al. (2018). Functional brain networks are dominated by stable group and individual factors, not cognitive or daily variation. Neuron 98, 439–452.e5. doi: 10.1016/j.neuron.2018.03.035
Hauser, T. U., Hunt, L. T., Iannaccone, R., Walitza, S., Brandeis, D., Brem, S., et al. (2015). Temporally dissociable contributions of human medial prefrontal subregions to reward-guided learning. J. Neurosci. 35, 11209–11220. doi: 10.1523/JNEUROSCI.0560-15.2015
Jacquemont, S., Coe, B. P., Hersch, M., Duyzend, M. H., Krumm, N., Bergmann, S., et al. (2014). A higher mutational burden in females supports a “female protective model” in neurodevelopmental disorders. Am. J. Hum. Genet. 94, 415–425. doi: 10.1016/j.ajhg.2014.02.001
Li, J., Seidlitz, J., Suckling, J., Fan, F., Ji, G. J., Meng, Y., et al. (2021). Cortical structural differences in major depressive disorder correlate with cell type-specific transcriptional signatures. Nat. Commun. 12:1647. doi: 10.1038/s41467-021-21943-5
Lin, J., Li, L., Pan, N., Liu, X., Zhang, X., Suo, X., et al. (2023). Neural correlates of neuroticism: a coordinate-based meta-analysis of resting-state functional brain imaging studies. Neurosci. Biobehav. Rev. 146:105055. doi: 10.1016/j.neubiorev.2023.105055
Loomes, R., Hull, L., and Mandy, W. P. L. (2017). What is the male-to-female ratio in autism spectrum disorder? A systematic review and meta-analysis. J. Am. Acad. Child Adolesc. Psychiatry 56, 466–474. doi: 10.1016/j.jaac.2017.03.013
Lynch, C. J., Uddin, L. Q., Supekar, K., Khouzam, A., Phillips, J., and Menon, V. (2013). Default mode network in childhood autism: posteromedial cortex heterogeneity and relationship with social deficits. Biol. Psychiatry 74, 212–219. doi: 10.1016/j.biopsych.2012.12.013
Maenner, M. J., Shaw, K. A., Baio, J., Washington, A., Patrick, M., DiRienzo, M., et al. (2020). Prevalence of autism spectrum disorder among children aged 8 years - autism and developmental disabilities monitoring network, 11 sites, United States, 2016. MMWR Surveill. Summ. 69, 1–12. doi: 10.15585/mmwr.ss6904a1
Matta, S. M., Hill-Yardin, E. L., and Crack, P. J. (2019). The influence of neuroinflammation in autism spectrum disorder. Brain Behav. Immun. 79, 75–90. doi: 10.1016/j.bbi.2019.04.037
Maynard, K. R., Collado-Torres, L., Weber, L. M., Uytingco, C., Barry, B. K., Williams, S. R., et al. (2021). Transcriptome-scale spatial gene expression in the human dorsolateral prefrontal cortex. Nat. Neurosci. 24, 425–436. doi: 10.1038/s41593-020-00787-0
Minshew, N. J., Goldstein, G., Dombrowski, S. M., Panchalingam, K., and Pettegrew, J. W. (1993). A preliminary 31P MRS study of autism: evidence for undersynthesis and increased degradation of brain membranes. Biol Psychiatry 33, 762–773. doi: 10.1016/0006-3223(93)90017-8
Modabbernia, A., Velthorst, E., and Reichenberg, A. (2017). Environmental risk factors for autism: an evidence-based review of systematic reviews and meta-analyses. Mol. Autism. 8:13. doi: 10.1186/s13229-017-0121-4
Motzkin, J. C., Philippi, C. L., Wolf, R. C., Baskaya, M. K., and Koenigs, M. (2015). Ventromedial prefrontal cortex is critical for the regulation of amygdala activity in humans. Biol. Psychiatry 77, 276–284. doi: 10.1016/j.biopsych.2014.02.014
Padmanabhan, A., Lynch, C. J., Schaer, M., and Menon, V. (2017). The default mode network in autism. Biol. Psychiatry Cogn. Neurosci. Neuroimaging 2, 476–486. doi: 10.1016/j.bpsc.2017.04.004
Pierce, K., Gazestani, V. H., Bacon, E., Barnes, C. C., Cha, D., Nalabolu, S., et al. (2019). Evaluation of the diagnostic stability of the early autism Spectrum disorder phenotype in the general population starting at 12 months. JAMA Pediatr. 173, 578–587. doi: 10.1001/jamapediatrics.2019.0624
Platzer, K., Cogné, B., Hague, J., Marcelis, C. L., Mitter, D., Oberndorff, K., et al. (2018). Haploinsufficiency of CUX1 causes nonsyndromic global developmental delay with possible catch-up development. Ann. Neurol. 84, 200–207. doi: 10.1002/ana.25278
Primiani, C. T., Ryan, V. H., Rao, J. S., Cam, M. C., Ahn, K., Modi, H. R., et al. (2014). Coordinated gene expression of neuroinflammatory and cell signaling markers in dorsolateral prefrontal cortex during human brain development and aging. PLoS One 9:e110972. doi: 10.1371/journal.pone.0110972
Quesnel-Vallieres, M., Weatheritt, R. J., Cordes, S. P., and Blencowe, B. J. (2019). Autism spectrum disorder: insights into convergent mechanisms from transcriptomics. Nat. Rev. Genet. 20, 51–63. doi: 10.1038/s41576-018-0066-2
Reardon, A. M., Li, K., and Hu, X. P. (2021). Improving between-group effect size for multi-site functional connectivity data via site-wise de-meaning. Front. Comput. Neurosci. 15:762781. doi: 10.3389/fncom.2021.762781
Robinson, E. B., Lichtenstein, P., Anckarsater, H., Happe, F., and Ronald, A. (2013). Examining and interpreting the female protective effect against autistic behavior. Proc. Natl. Acad. Sci. U. S. A. 110, 5258–5262. doi: 10.1073/pnas.1211070110
Rossignol, D. A., and Frye, R. E. (2012). Mitochondrial dysfunction in autism spectrum disorders: a systematic review and meta-analysis. Mol. Psychiatry 17, 290–314. doi: 10.1038/mp.2010.136
Sadakata, T., Kakegawa, W., Mizoguchi, A., Washida, M., Katoh-Semba, R., Shutoh, F., et al. (2007a). Impaired cerebellar development and function in mice lacking CAPS2, a protein involved in neurotrophin release. J. Neurosci. 27, 2472–2482. doi: 10.1523/JNEUROSCI.2279-06.2007
Sadakata, T., Washida, M., Iwayama, Y., Shoji, S., Sato, Y., Ohkura, T., et al. (2007b). Autistic-like phenotypes in Cadps2-knockout mice and aberrant CADPS2 splicing in autistic patients. J. Clin. Invest. 117, 931–943. doi: 10.1172/JCI29031
Schneider, K., Regenbogen, C., Pauly, K. D., Gossen, A., Schneider, D. A., Mevissen, L., et al. (2013). Evidence for gender-specific endophenotypes in high-functioning autism spectrum disorder during empathy. Autism Res. 6, 506–521. doi: 10.1002/aur.1310
Schott, B. H., Wustenberg, T., Lucke, E., Pohl, I. M., Richter, A., Seidenbecher, C. I., et al. (2019). Gradual acquisition of visuospatial associative memory representations via the dorsal precuneus. Hum. Brain Mapp. 40, 1554–1570. doi: 10.1002/hbm.24467
Schumann, C. M., Bloss, C. S., Barnes, C. C., Wideman, G. M., Carper, R. A., Akshoomoff, N., et al. (2010). Longitudinal magnetic resonance imaging study of cortical development through early childhood in autism. J. Neurosci. 30, 4419–4427. doi: 10.1523/JNEUROSCI.5714-09.2010
Smith, S. M., Vidaurre, D., Beckmann, C. F., Glasser, M. F., Jenkinson, M., Miller, K. L., et al. (2013). Functional connectomics from resting-state fMRI. Trends Cogn. Sci. 17, 666–682. doi: 10.1016/j.tics.2013.09.016
Sohal, V. S., and Rubenstein, J. L. R. (2019). Excitation-inhibition balance as a framework for investigating mechanisms in neuropsychiatric disorders. Mol. Psychiatry 24, 1248–1257. doi: 10.1038/s41380-019-0426-0
St Jacques, P. L., Szpunar, K. K., and Schacter, D. L. (2017). Shifting visual perspective during retrieval shapes autobiographical memories. Neuroimage 148, 103–114. doi: 10.1016/j.neuroimage.2016.12.028
Tamagawa, K., Makino, T., and Kawata, M. (2017). The effects of CpG densities around transcription start sites on sex-biased gene expression in Poecilia reticulata. Genome Biol. Evol. 9, 1204–1211. doi: 10.1093/gbe/evx083
Wang, S., Zhao, Y., and Li, J. (2023). True grit and brain: trait grit mediates the connection of DLPFC functional connectivity density to posttraumatic growth following COVID-19. J. Affect. Disord. 325, 313–320. doi: 10.1016/j.jad.2023.01.022
Werling, D. M., and Geschwind, D. H. (2013). Sex differences in autism spectrum disorders. Curr. Opin. Neurol. 26, 146–153. doi: 10.1097/WCO.0b013e32835ee548
Wilson, R. P., Colizzi, M., Bossong, M. G., Allen, P., Kempton, M., Mtac, B., et al. (2018). The neural substrate of reward anticipation in health: a meta-analysis of fMRI findings in the monetary incentive delay task. Neuropsychol. Rev. 28, 496–506. doi: 10.1007/s11065-018-9385-5
Yan, C. G., Cheung, B., Kelly, C., Colcombe, S., Craddock, R. C., Di Martino, A., et al. (2013). A comprehensive assessment of regional variation in the impact of head micromovements on functional connectomics. Neuroimage 76, 183–201. doi: 10.1016/j.neuroimage.2013.03.004
Zang, Y., Jiang, T., Lu, Y., He, Y., and Tian, L. (2004). Regional homogeneity approach to fMRI data analysis. Neuroimage 22, 394–400. doi: 10.1016/j.neuroimage.2003.12.030
Zhang, W., Groen, W., Mennes, M., Greven, C., Buitelaar, J., and Rommelse, N. (2018). Revisiting subcortical brain volume correlates of autism in the ABIDE dataset: effects of age and sex. Psychol. Med. 48, 654–668. doi: 10.1017/S003329171700201X
Keywords: autism spectrum disorder, gender effect, resting-state fMRI, neurotranscriptome, default mode network, multisite
Citation: Li Y, Li R, Wang N, Gu J and Gao J (2023) Gender effects on autism spectrum disorder: a multi-site resting-state functional magnetic resonance imaging study of transcriptome-neuroimaging. Front. Neurosci. 17:1203690. doi: 10.3389/fnins.2023.1203690
Edited by:
Yi-Fei Pu, Sichuan University, ChinaReviewed by:
Fengmei Lu, Chengdu No.4 People’s Hospital, ChinaSong Wang, Sichuan University, China
Xun Chen, University of Science and Technology of China, China
Copyright © 2023 Li, Li, Wang, Gu and Gao. This is an open-access article distributed under the terms of the Creative Commons Attribution License (CC BY). The use, distribution or reproduction in other forums is permitted, provided the original author(s) and the copyright owner(s) are credited and that the original publication in this journal is cited, in accordance with accepted academic practice. No use, distribution or reproduction is permitted which does not comply with these terms.
*Correspondence: Jingjing Gao, jingjing.gao@uestc.edu.cn