- 1Department of Anesthesiology, Perioperative Care and Pain Management, New York University Grossman School of Medicine, New York, NY, United States
- 2Department of Psychiatry, New York University Grossman School of Medicine, New York, NY, United States
- 3Department of Neuroscience & Physiology, Neuroscience Institute, New York University Grossman School of Medicine, New York, NY, United States
- 4Department of Biomedical Engineering, New York University Tandon School of Engineering, Brooklyn, NY, United States
Machine learning is becoming an increasingly common component of routine data analyses in clinical research. The past decade in pain research has witnessed great advances in human neuroimaging and machine learning. With each finding, the pain research community takes one step closer to uncovering fundamental mechanisms underlying chronic pain and at the same time proposing neurophysiological biomarkers. However, it remains challenging to fully understand chronic pain due to its multidimensional representations within the brain. By utilizing cost-effective and non-invasive imaging techniques such as electroencephalography (EEG) and analyzing the resulting data with advanced analytic methods, we have the opportunity to better understand and identify specific neural mechanisms associated with the processing and perception of chronic pain. This narrative literature review summarizes studies from the last decade describing the utility of EEG as a potential biomarker for chronic pain by synergizing clinical and computational perspectives.
1. Introduction
Pain is a complex and multi-dimensional process resulting from dynamic interactions of neural processes. It includes sensory-discriminative, affective-emotional, and cognitive-evaluative components (Rainville et al., 1997; Price, 2000; Perl, 2007; Sun et al., 2021). All pain initiates as acute pain but can become maladaptive and persist into a chronic phase (Gan et al., 2014; Chapman and Vierck, 2017; Gan, 2017). Numerous studies have demonstrated clear evidence that chronic pain continues to be a global public health issue, with an estimated prevalence of around 30% in adults (Johannes et al., 2010; Gardner and Sachdeva, 2019; Yong et al., 2022; Zimmer et al., 2022). The condition is not only known to significantly reduce quality of life (Yong et al., 2022) but is also associated with long-term disability; this typically requires multimodal treatment approaches, commonly results in reliance on opioid analgesics, and contributes to the opioid epidemic (Kehlet et al., 2006; Ataoğlu et al., 2013; Ladha et al., 2016; Gan, 2017; Schuchat et al., 2017; Hollmann et al., 2019). Hence, advances in pain research are urgently needed to address these healthcare issues.
By observing the brain activity that occurs during pain and trying to decode its underlying mechanism, it is believed that these pathways could be targeted earlier and more precisely, preventing pain from chronification, and thus reducing the consumption of addictive analgesics (Mouraux and Iannetti, 2018).
Over the last 20 years, advancing research has contributed to an increased understanding of the spinal, peripheral, and cortical mechanisms of pain (Apkarian et al., 2005; Rosa and Seymour, 2014; Tu et al., 2016; Ploner et al., 2017; Mouraux and Iannetti, 2018). In contrast to other sensory perceptions that are associated with a specific sensory cortex, a specific “pain cortex” associated with pain perception does not exist (Kucyi and Davis, 2015). Instead, it is the result of an activation of a distributed network of cortical and subcortical areas (Besson, 1999; Sawamoto et al., 2000; Mouraux and Iannetti, 2018; Liberati et al., 2020; Chen et al., 2022). Because of its complex nature, further research is required to better understand the mechanisms behind pain and to propose an adequate biomarker for chronic pain in particular (Tracey et al., 2019; Chen, 2021).
Studies using modern neuroimaging techniques such as functional magnetic resonance imaging (fMRI) and positron emission tomography have identified brain regions involved in sensory processing of acute pain. These brain regions include the primary somatosensory cortex (S1), anterior cingulate cortex (ACC), and insular cortex (Isnard et al., 2011; Duerden and Albanese, 2013; Vierck et al., 2013; Boccard et al., 2014; Mouraux and Iannetti, 2018; Van Der Miesen et al., 2019; Lamichhane et al., 2021; Sun et al., 2021). These techniques have limited temporal resolution, making it difficult to capture the dynamic nature of pain perception and experience (Wager et al., 2013). Therefore, some study groups have shifted focus to explore less invasive and more cost-effective alternatives with a higher temporal resolution, such as electroencephalography (EEG) (Pinheiro et al., 2016; Ploner et al., 2017; Levitt and Saab, 2019; Van Der Miesen et al., 2019; Xu and Huang, 2020; Millard et al., 2022; Chowdhury et al., 2023).
With an increasing number of human neuroimaging studies investigating the mechanism of pain, the field is steadily moving toward the development of a viable biomarker for pain (Furman et al., 2018; Seminowicz et al., 2018; Furman et al., 2019; Seminowicz et al., 2019; Furman et al., 2020). For instance, modern source localization techniques have substantially improved anatomical precision for EEG studies to enable circuit-level analysis, further facilitating the potential of biomarker development (Ferracuti et al., 1994; Le Pera et al., 2000; Chang et al., 2001; Seminowicz et al., 2019; Furman et al., 2020; Sun et al., 2021; Chowdhury et al., 2023). Recent reports have indicated that enhanced nociceptive response in EEG is manifested as abnormally elevated power in theta and gamma oscillations, suggesting that EEG could potentially predict the presence of pain and analgesic response (Babiloni et al., 2002; Wang et al., 2011; Schulz et al., 2012a, b; Rouleau et al., 2015; Peng and Tang, 2016; Taesler and Rose, 2016; Martel et al., 2017; Fallon et al., 2018; Tan et al., 2021). This provides further support for the feasibility of an EEG-based biomarker (Zhang et al., 2017; Dinh et al., 2019; May et al., 2019; Zis et al., 2022).
By applying machine learning (ML) to analyze functional brain imaging data such as EEG, we now have the capability to better identify response features to a given experiment—or stimulus, as well as to predict subjective perception and response to the same experiment (Hu and Iannetti, 2016; Lötsch et al., 2017; Fernandez Rojas et al., 2019). Hence, ML is a promising tool for the future development of biomarkers for chronic pain (Lamichhane et al., 2021; Harland et al., 2022). Recently, numerous studies have presented findings with considerable accuracy, working toward developing algorithms with improved generalizability and interpretability (Harland et al., 2022; Mari et al., 2022). The number of studies coupling ML algorithms with subjective reports on pain perception is increasing, and there is an impetus for data-sharing and collaboration within the pain research community to improve the sensitivity and efficacy of biomarker developmental methods (Van Der Miesen et al., 2019). While obstacles remain, it is clear that “decisions based on neural data will only be as good as the science behind them” (Hu and Iannetti, 2016). Hence, for the science to get better, it is imperative that we validate the results of past studies, identify the state-of-the-art methods, and provide updates on ongoing studies.
Today, the probability of presenting a non-composite, single biomarker capturing “pain” in its entirety is increasingly appearing unlikely (Tracey et al., 2019). It remains difficult to identify a standard way to qualify brain responses as specifically pain responses, especially without a dedicated pain cortex. However, by further exploring advanced analytical tools like neural networks, artificial intelligence, and machine-learning algorithms, it may be possible to combine multiple objective biomarkers into one composite pain biomarker (Tracey, 2021). Such approach could expedite success in understanding the mechanisms for pain as well as providing clinically relevant biomarkers (Baskin et al., 2016; Su et al., 2019; Eldabe et al., 2022).
To support the field in future research, we have conducted a narrative literature review by combining the following search terms: “electroencephalography” and “chronic pain” and “machine learning” using PubMed (including MEDLINE), Ovid (including EMBASE), Web of Science and the Cochrane Library. While several review articles have appeared in the literature (Reckziegel et al., 2019; Van Der Miesen et al., 2019; Mari et al., 2022; Zebhauser et al., 2023), our review focuses on studies published in the last decade (from 2012 to 2023), describing the practical utility of combining physiological data, EEG, and ML to study the mechanisms of chronic pain. Specifically, our review aims to appraise the role and potential utility of EEG as a biomarker for chronic pain. Hence, this review discusses a limited cohort and does not cover the entire breadth of publications in the pain research field. Nonetheless, we show how different computational methods and ML algorithms can help in the discovery of EEG biomarkers for chronic pain. We also discuss the future utility of today’s cutting-edge methods and how we can incorporate further analyses and neurophysiological data into an integrated biomarker model. Lastly, we discuss challenges in the chronic pain research field and offer insight on potential future directions.
2. The benefits of EEG for biomarker studies on chronic pain
2.1. Measurement of pain-related brain activity
Behavior arising from the experience of pain is seldom obscure, often manifested physically in the form of facial expressions, changes in body language, or changes in mood (Dansie and Turk, 2013). Thus, the presence of pain can be observed and measured – to a certain extent. Pain perception is a highly subjective experience (Kinnealey and Fuiek, 1999). Pain does not only vary between subjects but can vary in the same subject across time. For this reason, a number of pain assessments are available for the quantification of pain.
2.1.1. Bottom-up measurements based on pain-induced behavior
The current standard for self-reported pain intensity is assessed using the visual analog scale (VAS, typical range: 0–100 mm) (Boonstra et al., 2008) or the numeric rating scale (NRS, range: 0–10) (Haefeli and Elfering, 2006). Although these behavioral scales offer a degree of standardization across the population, individuals can still exhibit considerable and unpredictable variability in painful percepts in response to the same nociceptive stimulus (Quiton and Greenspan, 2008). Furthermore, self-reported pain intensity has been shown to at times correlate poorly with the stimulus intensity in experimental studies (Nickel et al., 2017). The mechanisms underlying such within-subject and between-subject variability in experimental pain remain insufficiently understood. The objective of top-down measurements of pain experience is to identify neural indicators that explain such perceptual variability in all types of pain (Hu and Iannetti, 2016).
2.1.2. Top-down measurements based on brain activity
A wide variety of neuroimaging techniques are used to record brain signals, at varying levels of invasiveness and across a range of spatial and temporal resolutions. For instance, microelectrode implants can record neural spikes from a single neuron or local field potentials from a small population of neurons, though at the cost of invasiveness and highly rapid time courses (Einevoll et al., 2013; Nurse et al., 2016; Merk et al., 2022). At the other end of the spectrum, whole brain imaging technologies such as fMRI can noninvasively image blood-oxygen dynamics as a proxy to region-specific activity, yet with relatively low temporal resolution and mid-range spatial resolution (Logothetis, 2008; Power et al., 2017; Woo et al., 2017; Siddiqi et al., 2022). In this review, we focus solely on EEG which measures extracellular current but at a larger spatial scale, reflecting the activity of hundreds of millions of neurons (Jackson and Bolger, 2014). EEG is noninvasive: the signal is recorded from an array of surface electrodes placed on a subject’s scalp, at a microvolt (μV) scale (Rosa and Seymour, 2014). Despite anatomical impedances, such as the presence of hair and variations in skull conductivity, EEG electrodes are capable of detecting the electrical activity of similarly oriented groups of cerebral cortical neurons near the scalp. The majority of the electrical activity sensed by scalp electrodes represents the summation of the inhibitory or excitatory postsynaptic potentials from thousands of pyramidal cells near each electrode (Britton et al., 2016). This trait enables researchers and clinicians a view into the cortical activity of the brain with low cost and effort.
EEG can be recorded using one of two methods: resting-state EEG (rs-EEG) or stimulus-evoked EEG. rs-EEG is recorded while a participant is awake but not engaged in any specific task. Though rs-EEG studies do not typically include stimuli, they may be used to evaluate the functional activity of the brain before and after a treatment or intervention, as well as to study chronic behavioral or pathological conditions such as chronic pain. Stimulus-evoked EEG, in contrast, is recorded in response to a specific stimulus. Typically, participants are subject to a set of repeated stimuli to study the dynamics of one or more regions involved in responding to said stimulus. Thus, stimulus-evoked EEG provides temporally specific information in a dynamic behavioral context, and it also offers insights into how an underlying disease condition such as chronic pain can alter temporal sensory processing. Both techniques are equally important, even as they enable fundamentally different approaches to studying chronic pain which are discussed in the next section.
2.2. Advantages and disadvantages of EEG for chronic pain studies
Despite the advances in pain research using other neuroimaging techniques, such as fMRI and PET, numerous studies have described the advantages of leveraging the non-invasiveness of EEG as a potential path to a biomarker for pain (Ploner et al., 2017; Liberati et al., 2018; Mouraux and Iannetti, 2018; May et al., 2019; Sun et al., 2021; Tracey, 2021; Vuckovic et al., 2022).
First, because of its high temporal resolution, it allows us to assess the oscillatory activity of neural pain processing. High temporal resolution is critical for understanding pain since it is a highly dynamic process (Schulz et al., 2012a,b). At the same time, correlating oscillatory activities across different brain areas enables us to detect specific brain areas associated with chronic pain. As such, in chronic pain studies, scalp EEG recordings present spontaneous synchronized postsynaptic neuronal activity of the brain cortex (Huber et al., 2006; Stern et al., 2006; Jobert et al., 2012; Mouraux and Iannetti, 2018).
A second advantage of EEG in the study of pain is that it is portable, easy to perform, well tolerated by patients, and more cost-effective than other neuroimaging modalities (Katsigiannis and Ramzan, 2017; Krigolson et al., 2017; Mussigmann et al., 2022). The ease of placement and mobility of EEG systems allows for continuous recordings of primary cortical activities in clinical settings, enabling the potential to develop a variety of biomarkers for chronic pain from a single modality (Byrom et al., 2018; Xu and Huang, 2020).
Meanwhile, a number of potential obstacles in using EEG to assess chronic pain should also be considered (Tracey, 2021). First, there are some limitations seen in past studies, where most have analyzed potentials from rs-EEG (as presented in Table 1). These potentials provide a basis for further analyses, allowing us to explore the dynamic of neural circuits involved in pain processing. The use of rs-EEG alone in understanding pain, however, can become problematic, since rs-EEG potentials may be confounded by other brain processes. As suggested by Hansen et al. (2017) studying evoked EEG potentials allows for a better understanding of the mechanism underlying nociception, which is especially important when studying a complex condition such as chronic pain (Cao et al., 2020). Thus, when studying chronic pain, rs-EEG can provide us with insights into baseline differences, whereas evoked EEG potentials can contain information about acute changes in neural pain processing, which can be more informative (Plaghki and Mouraux, 2005). Regardless of the types for potentials studied, uncertainties around whether recorded EEG responses are directly related to pain still exist, prompting the need for further research (Mouraux and Iannetti, 2018).
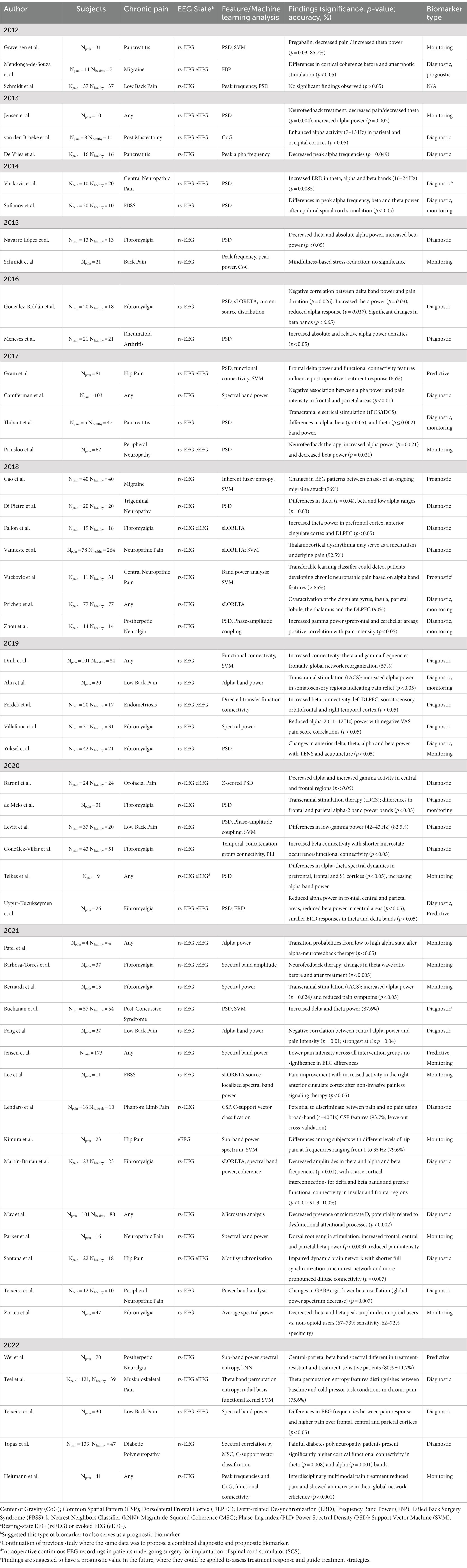
Table 1. Summary of recent studies utilizing EEG as a potential biomarker for chronic pain (review articles are excluded from this summary).
Second, since evoked potentials of EEG signals are usually brief in duration, the ability to establish generalizable features can be difficult (Iannetti et al., 2008). Instead, studies have suggested longer sensory stimuli exposure to capture the true nature of evoked pain perception, where the results have shown a positive correlation with gamma power changes in the medial prefrontal cortex (Schulz et al., 2015; Misra et al., 2017).
Third, scalp EEG electrodes only record compounded peripheral neuronal activity, meaning that the signal from deeper brain structures cannot be easily separated (Hallez et al., 2007). Further studies using depth electrodes, as in invasive intracranial EEG (iEEG) which are placed in the deep structures, such as the hippocampus, amygdala, and insula, could help us to understand better the complexities of chronic pain, and its affective-emotional and cognitive-evaluative components (Peyron et al., 2002; Mokhtari et al., 2019).
Overall, the use of EEG for chronic pain studies is appealing for its high temporal resolution, low cost, broad availability, and ease of data collection (Morton et al., 2016).
3. Extracting pain-related features from EEG data
With the advances in pain research, the field has made significant progress in improving and streamlining the analysis of EEG measurements. Following data acquisition, the first step in a neural data analysis pipeline is preprocessing. Preprocessing, including spectral filtering and artifact rejection, extracts the signals of interest while suppressing noise to maximize the signal-to-noise ratio (Hasenstab et al., 2015). Next, the preprocessed data is used to perform feature extraction, which aims to extract only the most discriminative information from a given signal (Pedroni et al., 2019).
3.1. Preprocessing: artifact removal
Human EEG recordings are highly susceptible to artifacts (e.g., head movement, eye blinks, and heartbeat). Extraction and removal of these components is typically accomplished by independent component analysis (ICA) (Urigüen and Garcia-Zapirain, 2015). Once the independent components have been identified, they can be analyzed and classified as either endogenous (e.g., muscular/ocular movement, cardiac activity) or exogenous artifacts (e.g., electronic device interference, electromagnetic radiation), and subsequently removed from the EEG data (Jas et al., 2017; Jiang et al., 2019).
3.2. Resting-state versus stimulus-evoked processing
The signal processing pipeline differs for resting-state and stimulus-evoked types of EEG data. While the activity recorded in evoked EEG can be associated with specific emotional, motor, sensory, perceptive and cognitive processes, that of rs-EEG cannot be associated with specific events; in relation, its activity is purely spontaneous. Accordingly, evoked data may consist of dozens to hundreds of repeated, seconds-long epochs (Aunon et al. 1978; Hu et al. 2019) while rs-EEG data is composed of one recording ranging from a few minutes to several hours in duration (Khanna et al., 2015; Olejarczyk et al., 2017), Therefore, evoked EEG data is accompanied by trial time-stamps, trial labels, and subject responses, while rs-EEG data may only contain sparse annotations. The differences in processing pipelines for the two data types are summarized below.
Common resting-state processing techniques:
• Omit trace segments with amplitude values above a set threshold for each electrode.
• Visually or programmatically omit trace segments containing movement artifacts.
• Set a single baseline as amplitude reference
• Cropped total duration.
Common evoked signal processing techniques:
• Programmatically drop entire epochs containing movement artifacts.
• Scale amplitude values across epochs, especially for cross-subject analyses.
• Set a single baseline as reference, or independent baseline preceding each epoch.
3.3. EEG feature extraction
The extraction of features from EEG data involves prior knowledge of the brain activity potentially related to pain processing (Hu and Zhang, 2019). Examples of such prior knowledge may include which brain regions are involved in pain processing, the timing and synchronization of activity—both within and between regions, and the degree of connectivity between those regions. Features commonly used in EEG studies of chronic pain can be represented in the spatial, temporal, or spectral domains (or a combination of the three) and computed from either sensor space or source space data. These features are typically analyzed for temporal dynamics more than rs-EEG and the different feature representations contribute to the investigation of pain processing from distinct yet meaningful perspectives.
3.3.1. Spatial features
Extracting meaningful spatial patterns in EEG data by methods such as dimensionality reduction and pattern optimization allows for the identification of specific regions involved in pain processing. For instance, common spatial patterns is a linear algebra-based technique that works by finding the most discriminative EEG components between different classes in a given dataset, such as trials during painful stimulation versus trials without (Blankertz et al., 2008; Lu et al., 2010; Wu et al., 2014).
3.3.2. Temporal and spectral features
Pain processing is associated with complex temporal-spectral patterns of brain activity. Brain oscillations are patterns of synchronized electrical activity that arise from the coordinated activity of large populations of neurons; they can vary in amplitude, timing, and frequency. Features constructed from brain oscillations may take the form of power spectral density, relative power ratio, amplitude, phase coherence, and phase synchrony (Riaz et al., 2015). Pain-evoked event-related potentials (ERPs) are associated with an increase in theta band (4–8 Hz) power, also referred to as the theta-ERS (Pinheiro et al., 2016). In evoked pain, EEG studies have shown increased activity in the high-gamma band (60–100 Hz) (Ploner et al., 2017). In chronic pain, decreases in the power of the alpha band have also been observed (see Table 1) (De Vries et al., 2013). An increase or decrease in the power of a certain frequency band is referred to as non-phase-locked event-related synchronization (ERS) or event-related desynchronization (ERD), respectively (Pfurtscheller 2001; Hadjileontiadis, 2015). Figure 1 illustrates the differences between the ERP and ERD/ERS analysis techniques.
Higher-order information can also be extracted from both temporal and spectral features. One such example based on spectral features is the center of gravity (CoG). Assuming a defined region of interest (ROI) composed of either a subset of channels or a current source density distribution, CoG is defined as the frequency at which the whole EEG power within the empirically defined window is split into two equal parts, each part possessing the same overall power (Schmidt et al., 2012). Another example of a higher-order feature is entropy, also known as complexity. Based on information theory, entropy is a method for quantifying the irregularity of the EEG signal. When applied to the EEG power spectrum, entropy can measure the “peakedness” or “flatness” of the power distribution, representing the rhythmicity of the signal based on changes in the proportions of power at each frequency (Inouye et al., 1991).
3.3.3. Source localization
Source localization in EEG is a method of estimating the location and intensity of current sources generated from cortical and even subcortical regions (Seeber et al., 2019). Minimum norm estimate, Low-resolution electromagnetic tomography (LORETA), and Beamforming are some examples of source localization algorithms (Chen et al., 2002; So, 2011; Michel and Brunet, 2019). Following EEG source localization with a subsequent functional connectivity analysis is commonly done (Schoffelen and Gross, 2009; Sohrabpour et al., 2016).
3.3.4. Connectivity patterns
Functional connectivity (FC) analysis in EEG typically involves computing the statistical dependence or relationship between different brain regions or networks (Sakkalis, 2011). Some common examples of these algorithms include coherence, correlation, partial correlation, wavelet coherence, dynamic causal modeling (DCM), and Granger causality (Guo et al., 2020). These algorithms can provide insight into the direction and strength of connectivity between brain regions, as well as the dynamic nature of these connections. For example, FC has been used to identify long-range nociceptive information flow within the brain in chronic pain conditions (Necka et al., 2019).
4. Application of machine learning to EEG studies of chronic pain
4.1. Overview of machine learning algorithms
While the preprocessing and feature selection stages extract information from EEG data, the subsequent decoding stage utilizes the extracted features to provide insights for clinical and research applications. The application of ML facilitates tasks such as classification, detection, prediction, and risk assessment to identify meaningful features from EEG data (Müller et al., 2008; Hosseini et al., 2020). Then, after meeting certain criteria such as specificity, sensitivity, and generalizability, these features can be deemed biomarkers. In the context of chronic pain, this would allow us to identify patterns in EEG that could serve as putative neural codes for diagnosis, prognosis, monitoring, or prediction of chronic pain (Brodersen et al., 2012; Wager et al., 2013; Chen, 2021; Tracey, 2021; Harland et al., 2022).
Supervised and unsupervised ML approaches remain the most common approaches used in EEG studies of chronic pain cohorts (Alloghani et al., 2020). However, semi-supervised learning involves a small portion of labeled samples and a large number of unlabeled samples from which a model must learn and make predictions on new samples (Jia et al., 2014; She et al., 2019). Currently, the majority of published studies apply supervised learning to analyze EEG findings (Hammal and Cohn, 2012; Jenssen et al., 2021; Harland et al., 2022). By identifying spatial, temporal, or spectral features from the EEG data, one can train a parametric or nonparametric classifier on the labeled data to accomplish a certain task (Matsangidou et al., 2021). Examples of such tasks are described in Figure 1.
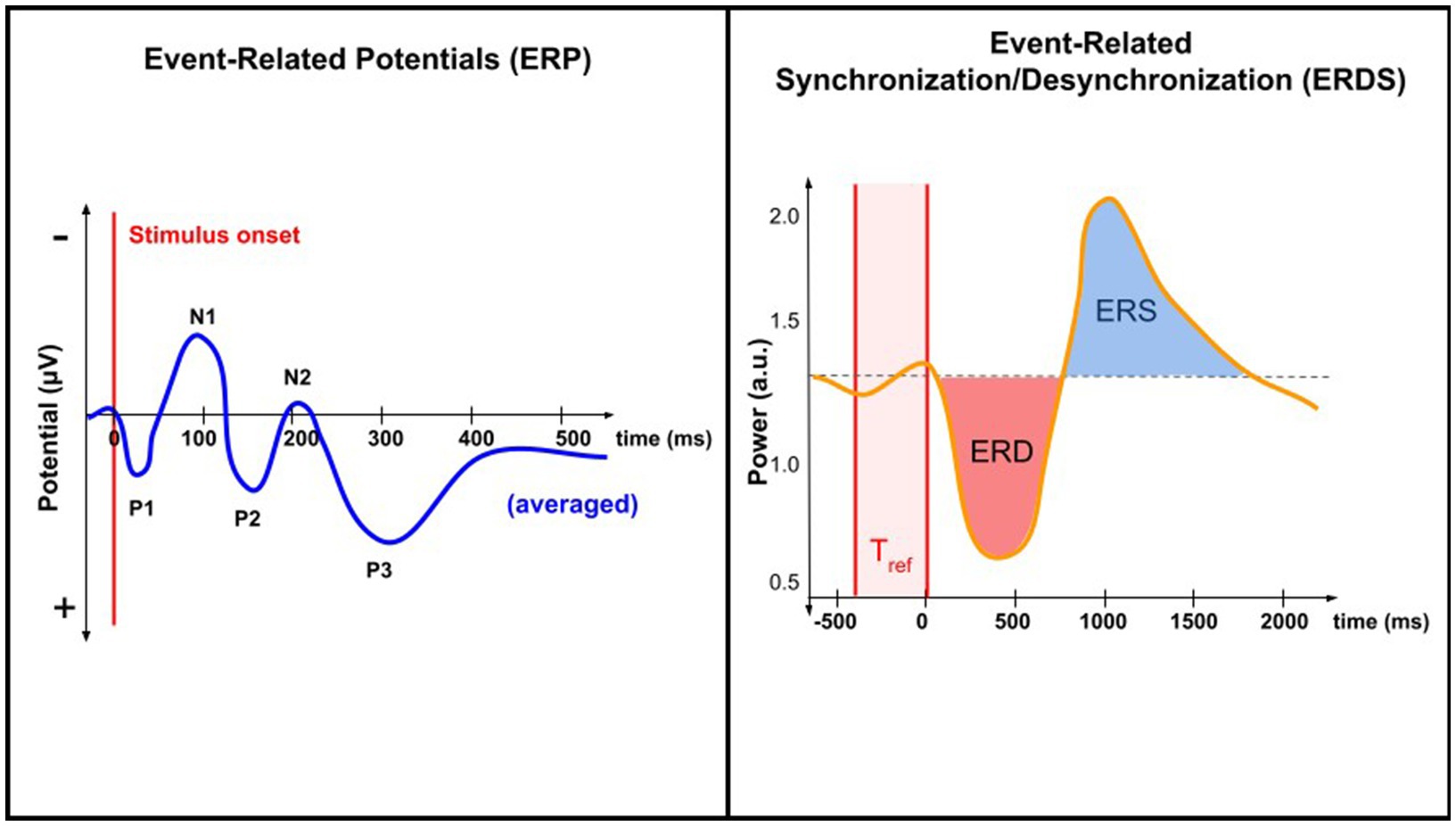
Figure 1. Comparing ERP and ERD/ERS analysis techniques. ERP analysis involves EEG data time-locked to a specific event. When averaged, ERPs reveal characteristic peaks and troughs (N1, P1, etc.). ERDS analysis involves quantifying changes in the power (rather than potential) of specific frequency bands in the EEG signal relative to a baseline period, Tref. Both techniques study changes in neural activity associated with a specific task or event, but only ERP is time-locked.
4.2. Supervised versus unsupervised learning approaches
Supervised and unsupervised learning methods differ primarily in their approaches to training, specifically in their reliance on labels (Aggarwal and Chugh, 2022). In the context of pain studies using stimulus-evoked EEG, the availability of labeled samples is dependent on many factors. For one, the collection of a sufficiently large number of trials (>100) in human subjects is time-consuming and often difficult for chronic pain patients who generally experience a heightened level of discomfort. Additionally, the selection of pain stimulus device, method, and intensity is limited and requires approval due to considerations of safety and ethics (Gatchel et al., 2016). To alleviate overfitting, both regularization and dimensionality reduction techniques are often employed. Another common concern in supervised learning is sample imbalance between classes. Under-sampling from the class with more trials is one way to alleviate the problem; though in pain studies, could lead to reducing an already low number of trials.
In a recent systematic review by Mari et al. (2022), they reviewed a total of 44 studies evaluating the effectiveness of ML algorithms on EEG data to explore the various aspects of pain. The continuous improvement of various performing models demonstrated high accuracies, ranging between 62 to 100%. These findings show that ML has the potential to predict pain outcomes, such as pain intensity, pain phenotype, and treatment response (Mari et al., 2022). The majority of the publications included were based on supervised ML methods, which are also known to present higher accuracies than their unsupervised counterparts (Hosseini et al., 2020).
Recently, Sun et al. developed an unsupervised learning method based on linear features extracted from EEG recordings to detect pain signals with a reported accuracy of 76% (Sun et al., 2021). By looking at source-localized ROIs using a state-space model, they observed that the unsupervised learning method requires fewer training trials and suggested that its performance is comparable—or perhaps better than the supervised method (Sun et al., 2021). However, this study assessed EEG signals from healthy pain-free subjects, with trials of acute evoked pain. To our knowledge, no studies describing the application of unsupervised learning methods to chronic pain data yet exist.
Unsupervised learning can alleviate the need for a large, balanced dataset of labeled samples. For example, in chronic pain research, cluster algorithms can be used when looking into pain intensities (Kragel et al., 2018). Furthermore, unsupervised learning methods have proven useful in extracting nonlinear features, making them more attractive as a decoding method in EEG pain research. However, further development is needed to demonstrate that unsupervised methods can support their generalizability with sufficiently high performance. Examples of common supervised and unsupervised classifiers employed in chronic pain research are described in Figure 2.
Semi-supervised learning approaches are worth exploring as they may offer practicality in the face of limitations: sparsely labeled data. EEG data requires administering stimuli (in the case of evoked data) or prolonged recording periods (in the case of resting-state data). For some chronic pain patients, prolonged sedentary periods can become overly uncomfortable, thereby resulting in diminishing returns with longer recordings due mostly to movement artifacts. Because semi-supervised learning requires significantly fewer labels over an entire dataset, individual recording sessions can be optimized and sped up, thereby resulting in data with higher quality and quantity. Such bottom-up approaches where the analysis step influences the data collection protocol can oftentimes lead to the best outcomes, facilitated by proper feedback.
4.3. Important considerations for ML in EEG studies of chronic pain
While choosing the right ML algorithm is indispensable, the importance of employing good ML practices cannot be understated. First-time users of ML may follow practices that lead to error-prone analyses, or to the illusion of successful results due to phenomena such as overfitting (model memorization of training data). By taking care in properly arranging input data, selecting an algorithm and its parameters deliberately, and appropriately evaluating model performance, one can be sure to maximize the potential in their dataset (Chicco, 2017).
Deep learning, a type of ML based on neural networks, can be highly effective at identifying nuanced pain-related features in EEG (Chen, 2021). However, deep learning requires a large number of labeled samples in training, restricting its use in the chronic pain cohort. While some studies have shown promising results in studying chronic pain with deep learning (Vuckovic et al., 2018), they are not as widespread because of the limited sample size.
5. Types of potential EEG biomarkers and their utility in chronic pain research
The most acknowledged definition of a biomarker is “a defined characteristic that is measured as an indicator of normal biological processes, pathogenic processes or responses to an exposure or intervention” (Group, 2016). In the context of chronic pain, a biomarker could thus serve to either confirm the presence of pain, identify the transition from one pain state to another, measure the risk of developing pain, estimate a prognosis, or predict and evaluate intervention responses (Van Der Miesen et al., 2019).
Clinical biomarkers can be further classified based on their presumed application and purpose (Group, 2016; Califf, 2018; Tracey et al., 2019; Van Der Miesen et al., 2019; Eldabe et al., 2022). The various types of biomarkers according to the most recent literature are presented in Figure 3. Until recently, the main objective of biomarker development has focused less on quantifying pain and more on delivering high-accuracy diagnoses and treatment algorithms, although based on neural mechanisms, rather than on symptoms (Dahlhamer et al., 2018; Gunn et al., 2020).
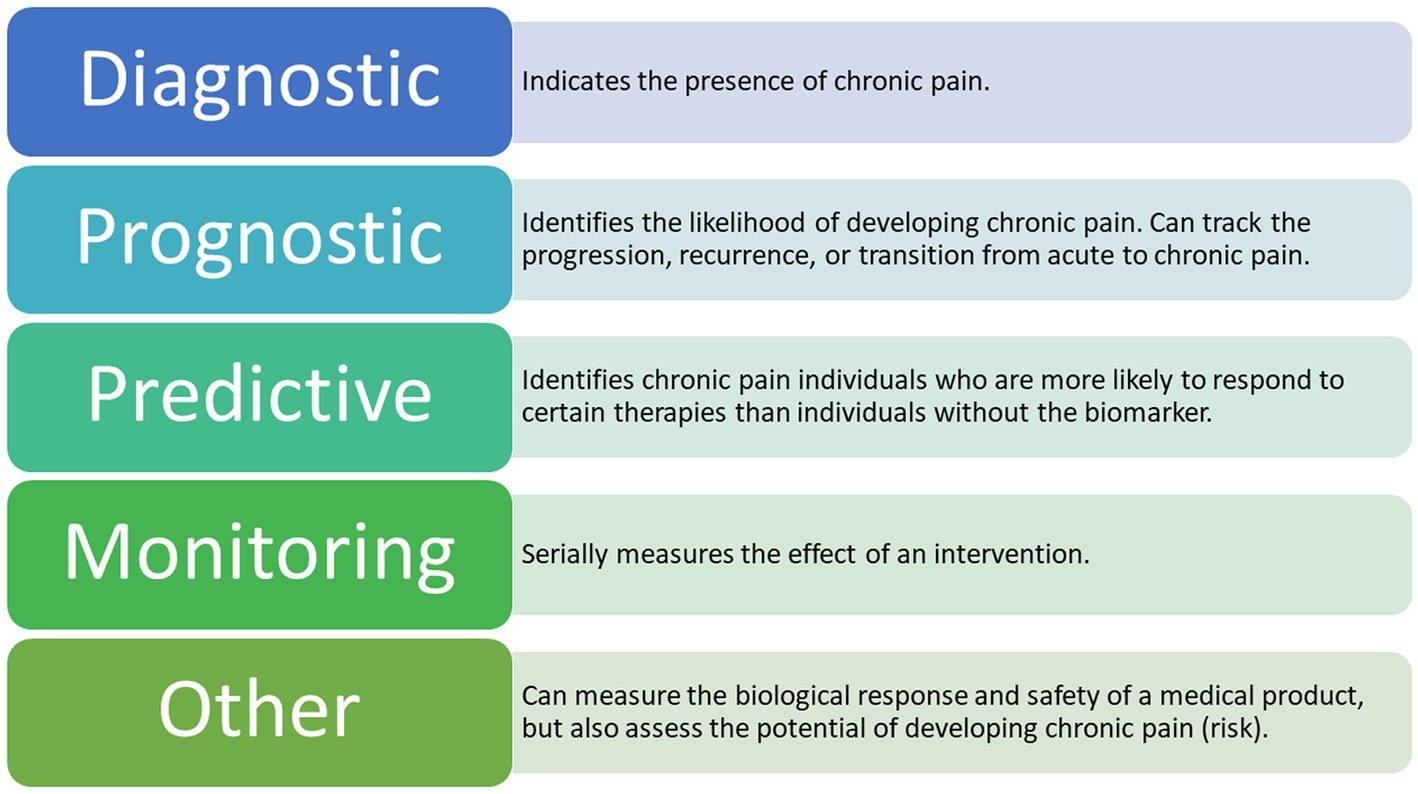
Figure 3. Types of Potential EEG Biomarkers for Chronic Pain. The following types of biomarkers have the potential to be clinically applicable in chronic pain management (Tracey et al., 2019; Van Der Miesen et al., 2019) A combination of these biomarkers is also a possible outcome for future research.
There are seven defined biomarker subtypes, each belonging to one of four categories associated with the development of biological biomarkers (Group, 2016; Tracey et al., 2019; Van Der Miesen et al., 2019). To date, the most applied biomarker subtypes in chronic pain research are diagnostic, prognostic, predictive, and monitoring (Davis et al., 2020). By identifying specific brain regions involved in the processing of chronic pain signals, we are indeed getting closer to decoding the presence of chronic pain (Graversen et al., 2012; Mendonça-de-Souza et al., 2012; Schmidt et al., 2012; De Vries et al., 2013; van den Broeke et al., 2013; Vuckovic et al., 2014; González-Roldán et al., 2016; Zebhauser et al., 2023). However, existing studies in this cohort are relatively few in number and the studies published so far have focused on the signals detected in either healthy participants exposed to acute experimental pain or in patients suffering from acute pain (Mouraux and Iannetti, 2018; Reckziegel et al., 2019). Thus, observed results mainly apply to a single type of condition at a certain point in time, which could easily be confounded with signals responsible for a long-lasting condition, such as chronic pain.
In contrast to acute pain, chronic pain involves complex peripheral and supraspinal brain mechanisms, where details on the underlying mechanisms remain incompletely known (von Hehn et al., 2012; Sun et al., 2021). Because of the multidimensional nature of chronic pain, a biomarker specific to the pathology has the potential to serve more than one purpose, thus being multifaceted and combinatorial (Ploner et al., 2017; Levitt and Saab, 2019). An overview of the studies included in this review, together with a summary of their representative features and biomarker type for each pain disease, can be seen in Tables 1–3.
5.1. Diagnostic biomarkers
A diagnostic biomarker indicates the presence of a condition or disease, like chronic pain. Most studies on chronic pain fall under this category (Van Der Miesen et al., 2019), primarily those associating chronic pain with group differences in EEG features (Reckziegel et al., 2019).
Studies assessing the potential of EEG as a diagnostic biomarker for chronic pain have emerged in the past decade and have led to the discovery of specific brain regions where relevant EEG changes associated with chronic pain are commonly observed. As presented in Table 1, the majority of the earlier studies recorded rs-EEG potentials in a few subjects. Nevertheless, they demonstrated promising findings indicating changes in specific frequency bands within targeted structures (De Vries et al., 2013; van den Broeke et al., 2013; Vuckovic et al., 2014; Navarro López et al., 2015; González-Roldán et al., 2016; Meneses et al., 2016). The described observations, mainly localized in the frontal, parietal, and occipital cortices, include both enhanced and reduced peak alpha frequency and theta responses, increased beta-band power, and increased ERD in the same bands—suggesting their potential as diagnostic biomarkers for chronic pain (De Vries et al., 2013; van den Broeke et al., 2013; Sufianov et al., 2014; Vuckovic et al., 2014; Navarro López et al., 2015; González-Roldán et al., 2016; Camfferman et al., 2017) (please see Table 2).
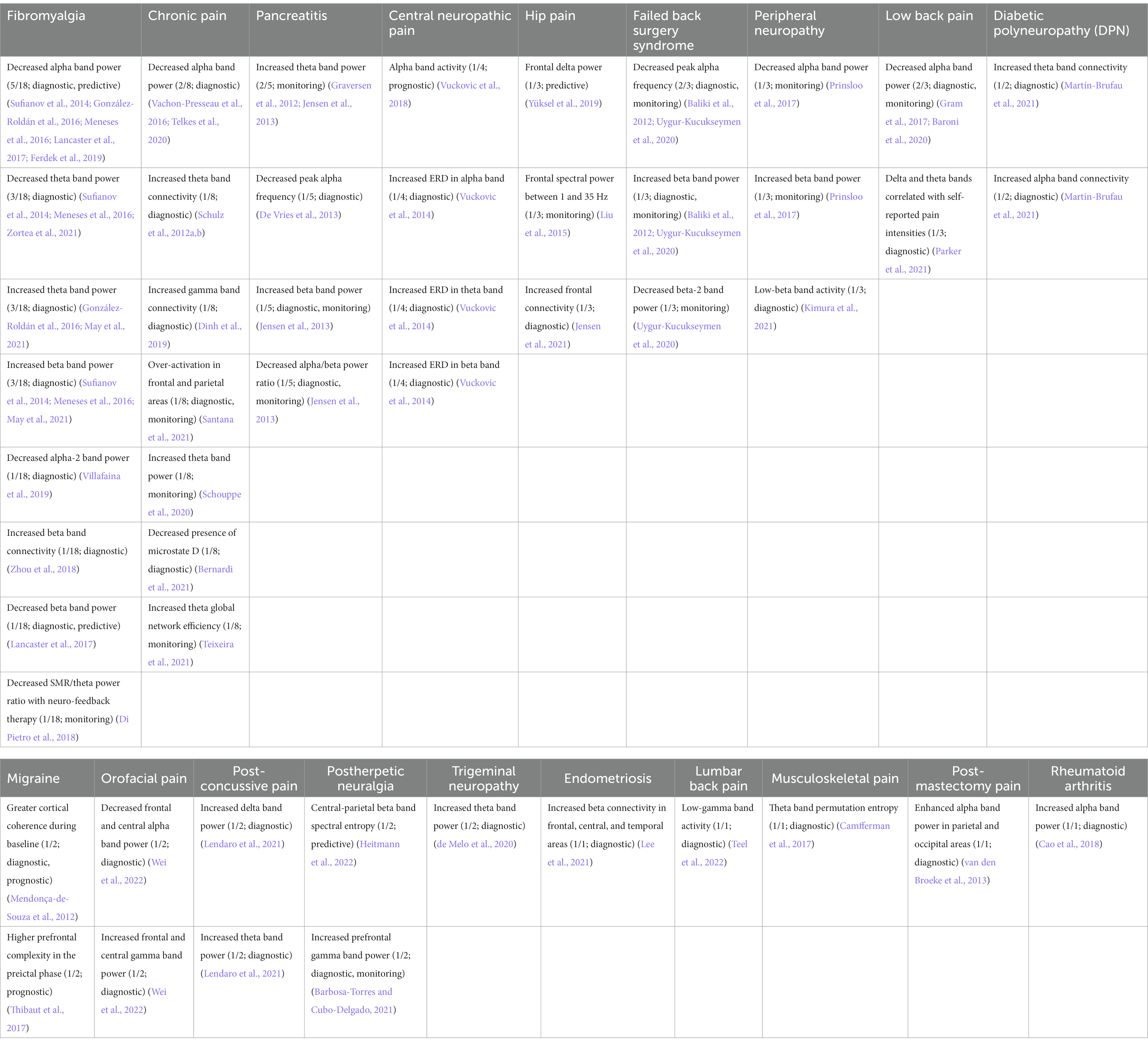
Table 2. Representative features and their biomarker types for each pain disease reviewed. Pain disease columns are sorted by descending quantity of papers reviewed; rows are sorted by descending count of observations. Format: “Potential biomarker (count of shared observations/number of papers reviewed in that pain disease; biomarker type(s)) [Reference(s)].”
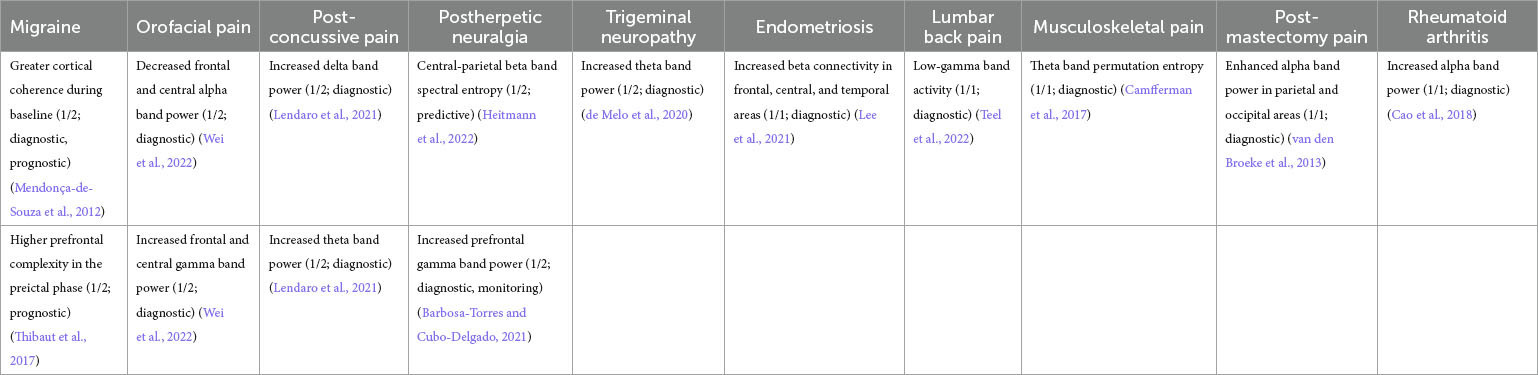
Table 3. Representative features pooled across all pain disease types. Similar features are combined within the same row in column 1.
In one of the largest studies to date, Dinh et al. used SVM to demonstrate increased connectivity at theta (4–8 Hz) and gamma (50–100 Hz) frequencies in frontal regions, as well as global network reorganization (Dinh et al., 2019). Moreover, they demonstrated a decreased global efficiency at gamma frequencies in chronic pain patients. Such patterns have previously demonstrated involvement in the pathophysiology of chronic pain and are now better investigated. However, as described in Table 2, there still seems to be a continued discrepancy in the reported power responses in the theta, alpha, and beta bands, complicating the proposal of a consistent and reliable biomarker for chronic pain (Freye and Levy, 2006; Navarro López et al., 2015; Martín-Brufau et al., 2021). Moreover, the decoder performed only at 57% accuracy—close to chance-level, leaving much room for improvement. In more recent years, researchers’ primary goal in improving decoding performance has been motivated primarily by the goal of optimizing model generalization, where the application of SVM classifiers has led to an improvement in accuracy with up to 93.7% (Misra et al., 2017; Kragel et al., 2018; Levitt et al., 2020; Buchanan et al., 2021; Lendaro et al., 2021; Zolezzi et al., 2021; Teel et al., 2022; Topaz et al., 2022).
With gradual improvements in the ML algorithms over the years, there is a trend of testing their applicability in clinical practice, especially for diagnostic, monitoring, and prognostic purposes in the context of chronic pain (Mendonça-de-Souza et al., 2012; Sufianov et al., 2014). These applications would allow us to detect individuals with an increased risk of developing a certain condition, for example, the transition from acute to chronic pain. More importantly, it allows us to track the trajectory of pain development after applying certain therapies. This stratification of patients could serve to guide and inform future treatment and adds an additional quantitative objective measure of pain (Thibaut et al., 2017; Ahn et al., 2019; Yüksel et al., 2019; Santana et al., 2021; Heitmann et al., 2022).
As an example, and as a continuation of previous studies, Vuckovic et al. (2022) developed and further trained their own classifiers to evaluate subjects with central neuropathic pain. They provided evidence for the potential of utilizing non-oscillatory, non-linear features of EEG not only as a diagnostic biomarker but also for prognostic purposes. Thus, their study suggests that ML models can be trained not only to determine the presence of pain but also to predict the delay after which patients start showing symptoms of pain-state transition. However, pain is a highly subjective experience and often presents itself heterogeneously. Studies so far have not been able to present a diagnostic biomarker with enough validation and generalizability for clinical settings.
The extraction of spatial patterns and the detection of changes in oscillations have moved the field one step closer to producing a diagnostic biomarker for the presence of chronic pain. We now know that a distributed network of cortical circuits regulates pain with knowledge of specific regions involved in pain processing—the S1, ACC, and insular cortex (Liberati et al., 2018; Van Der Miesen et al., 2019; Sun et al., 2021). Furthermore, we know that noxious stimulation can evoke neural responses from these regions, like changes in theta and high gamma power (Baliki et al., 2012; Liu et al., 2015; Ploner et al., 2017; Zhang et al., 2017; Prichep et al., 2018; Schouppe et al., 2020). Currently, these features are described primarily in studies of acute experimental pain—with relatively few on chronic pain. Hence, to propose a diagnostic biomarker of chronic pain, further studies are needed to identify and confirm discriminative features specific to chronic pain states.
5.2. Prognostic biomarkers
Prognostic biomarkers serve to identify the likelihood of developing a disease or state, to track the progression or recurrence of a disease, or to identify the transition from one disease state to another, e.g., from an acute to chronic pain state (Baliki et al., 2012; Tracey et al., 2019; Van Der Miesen et al., 2019).
In general, difficulties have been noted in the studies trying to propose the usage of EEG as a prognostic biomarker. The main concern arises from the complex, dynamic nature of pain which limits the ability to capture EEG signals of prognostic value, requiring EEG recordings over a longer period. Nonetheless, there is a possibility that features learned through ML in studies using other neuroimaging modalities, such as fMRI (Baliki et al., 2012), can be used to monitor the transition between disease states from continuous EEG signals to be used as monitoring biomarkers. Meanwhile, only a few studies have examined this possibility in a longitudinal cohort of pain patients.
In 2018, Vuckovic et al. (2022) demonstrated the potential utility of EEG as a prognostic biomarker after using previous datasets with previously recorded EEG signals in patients with painful and non-painful central neuropathic pain. By testing three classifiers (artificial neural network, SVM, and linear discriminant analysis) on EEG band power in resting state data recorded over time, they demonstrated that a transferable learning classifier learning classifier could detect patients at risk for developing painful chronic neuropathic pain with 86% accuracy. The study also suggested that it is possible to further develop and expand the purpose of a biomarker by using already existing data sets (Vuckovic et al., 2014).
To our knowledge, there are only a handful of published studies using EEG as a potential biomarker solely for prognostic purposes in chronic pain patients. Due to its complex nature and clinical importance, a prognostic biomarker for chronic pain requires rigorous model training and validation with large data sets to achieve high sensitivity and high specificity, as well as good generalizability. With an increasing number of studies on diagnostic and monitoring biomarkers, studies investigating its prognostic counterpart are likely to increase over the coming years, potentially relying on existing data from the diagnostic and monitoring arm of the field (Vachon-Presseau et al., 2016).
5.3. Predictive and monitoring biomarkers
Available knowledge of neural processes and pathways associated with the presence of pain has initiated the development of predictive biomarkers and monitoring biomarkers for chronic pain. A predictive biomarker enables the identification of individuals who are more likely to respond to certain therapies than individuals without the biomarker (Reckziegel et al., 2019; Tracey et al., 2019; Van Der Miesen et al., 2019; Eldabe et al., 2022). A monitoring biomarker , on the other hand, helps to serially measure the effect of an intervention or therapy. By combining both, we could predict a patient’s response to a certain therapy, enabling the development of a customized intervention program ahead of time.
For decades, scientists have explored the clinical implication of EEG as a monitoring biomarker for chronic pain. This has been done mainly by evaluating EEG signals before and after applying certain therapies—either individually or combined across subjects. Examples of such interventions include acupuncture, analgesics and anticonvulsants, epidural cord stimulation, neurofeedback treatment, surgical treatment, and transcranial stimulation therapies (Graversen et al., 2012; Jensen et al., 2013; Sufianov et al., 2014; Prinsloo et al., 2017; Thibaut et al., 2017; Ahn et al., 2019; Yüksel et al., 2019; de Melo et al., 2020; Barbosa-Torres and Cubo-Delgado, 2021; Heitmann et al., 2022).
As presented in Table 1, only a few studies attempting to assess EEG changes after applying targeted therapies have used healthy controls for comparison, hampering the predictive potentials of the proposed biomarker (Gram et al., 2017; Prinsloo et al., 2017; Ahn et al., 2019; de Melo et al., 2020; Lee et al., 2021; Zortea et al., 2021; Wei et al., 2022).
Notably, the majority of studies evaluating potential therapies have observed noticeable, statistically significant differences in the powers of theta, alpha, beta, and gamma activity in regions associated with chronic pain states (Zhou et al., 2018; de Melo et al., 2020; Lee et al., 2021; Patel et al., 2021; Zortea et al., 2021). These findings further strengthen the potential of EEG as a monitoring biomarker. With data over longer periods, from both healthy controls and chronic pain patients, the development of a robust composite biomarker serving diagnostic, predictive, prognostic, and monitoring purposes will be more readily achievable.
6. The future of EEG as a biomarker for chronic pain
In the last decade, the EEG pain research field has seen rapid progress. Though as we look into the future, we predict collaborative efforts will be crucial for achieving the development of any EEG biomarker for pain, especially one that is composite. Below we list some recommendations to push the EEG pain research community forward.
Data sharing and data pooling across study groups have proven to be appealing and perhaps essential methods to address the need for large data sets for ML analysis of EEG recordings (Van Der Miesen et al., 2019; Davis et al., 2020). Research groups may test various features and ML methods on the same large dataset, making for more robust comparisons between models, and facilitating faster discovery of potential features toward a composite biomarker for chronic pain. Moreover, by pooling data across various study groups, the validity of a potential biomarker would increase as the sample size gets larger, allowing for more robust cross-validation (Davis et al., 2020). In addition, the transparency of a potential EEG biomarker would be significantly improved by homogenized reporting standards. Currently, there exists a great discrepancy between reported results, and as part of an effort to improve future outcomes, new reporting guidelines have been developed and presented (Mari et al., 2022). However, large, multi-center datasets do come with a caveat: increased subject variability may hinder the clarity of cross-subject predictors.
Another benefit of data pooling is the diversity of chronic pain conditions, making a potential biomarker more generalizable. Due to slight variations in population samples, two research groups studying the same pain disease with similar experimental and analysis techniques could arrive at contrasting results. For example, a number of the studies in this review are focused on one specific pain disease cohort: fibromyalgia patients, a condition that is much more common in women than in men, creating an obstacle for a generalizable biomarker. Thus, data pooling has the potential to reduce the impact of sample population variations in small datasets by increasing the diversity in multi-center datasets.
Another innovative approach that has emerged recently is to combine rs-EEG studies with stimulus-evoked signals (Table 1). Pain is a dynamic process, and chronic pain involves both tonic and phasic components. Thus, by analyzing both resting-state and stimulus-evoked EEG potentials, we can further reduce the risk of confounders and improve sensitivity and specificity (Hansen et al., 2017).
Furthermore, Ávila et al. published an open and fully automated pipeline (DISCOVER-EEG) enabling easy, fast, and homogenous preprocessing, analysis, and visualization of rs-EEG data. This is an important step forward and should be taken as an example for future studies—where a tool like this will most likely promote open and reproducible research on brain function (Gil Avila et al., 2023). Optimally, this tool could be further developed into an unsupervised or semi-supervised ML method, allowing us to use largely unlabeled data, which would increase the generalizability of the potential biomarker itself. Moreover, standardized ML processes could contribute to the use of good ML practices, where commonly noted mistakes in current studies are results that may reflect overfitting or other anomalies in the ML implementation. Importantly, future studies also need to focus on diversity, equity, and inclusion in both training and testing datasets to further ensure good ML practice.
Advances in EEG source localization may also help improve the validity and reliability of a potential EEG biomarker for pain. Specifically, these methods could be used to inform which scalp electrodes are best positioned to record pain-associated brain activity, improving biomarker transparency and usability. As an example, the study conducted by Cao et al. demonstrated that using just a handful of leads may be enough to detect the presence of a disease, making future studies easier to conduct, but also facilitating continuous and longer EEG recording for monitoring purposes (Cao et al., 2018). This would be further facilitated by way of a portable device (Pu et al., 2021; Eldabe et al., 2022).
6.1. The potential for multimodal biomarkers
Studies on multimodal biomarkers are emerging for chronic pain patients (Prichep et al., 2018; Tracey et al., 2019; Eldabe et al., 2022). The incorporation of computational methods to conventional neurophysiological techniques such as EEG can be combined with other testing modalities, such as clinical reports, blood biomarkers, and quantitative sensory testing, to quantify pain and to predict outcomes for chronic pain patients (Califf, 2018; Mari et al., 2022).
Over recent years, a shift in paradigm for decoding chronic pain has already occurred, by incorporating objective neural signals into more subjective measurements of pain such as pain and mood questionnaires, as well as physiological data such as pulse and skin conductance measurements (Lancaster et al., 2017). With our current understanding of the EEG patterns associated with chronic pain states, and with the continuous improvement of ML algorithms, we now have the tools to propose multimodal biomarkers in the future. Another development in biomarker research is that an appropriately collected and curated database could be applied to the development of multiple biomarkers serving more than one clinical purpose (Vuckovic et al., 2014, 2018).
6.2. Limitations of this review
As a narrative review, we did not employ a systematic set of criteria for study inclusion, in part due to the relatively disparate literature in machine learning and EEG studies in pain. By presenting concepts from the perspective of clinical applicability, we aimed to facilitate an understanding of the application of EEG and machine learning in studies of chronic pain without the use of restrictive language.
6.3. Summary
In summary, impactful studies have been conducted in the past decade showing the potential for an EEG-based biomarker for chronic pain. Through the establishment of standardized practices and improved collaborations between members of the field, EEG-based techniques have the potential to become a key component of chronic pain diagnosis and treatment.
Author contributions
MR wrote the first draft of the manuscript. GK, LD, ZC, and JW wrote sections of the manuscript. All authors contributed to the article and approved the submitted version.
Acknowledgments
The authors acknowledge funding from the National Institutes of Health grants NS121776 (JW and ZC), MH118928 (ZC) and DA056394 (ZC). Any opinions, findings, and conclusions or recommendations expressed in this article are solely those of the authors and do not necessarily reflect the views of the funding agencies.
Conflict of interest
JW is a cofounder of Pallas Technologies, Inc., and ZC is a scientific advisor of Pallas Technologies, Inc. JW and ZC are inventors of a pending US patent application of pain treatment technology.
The remaining authors declare that the research was conducted in the absence of any commercial or financial relationships that could be construed as a potential conflict of interest.
Publisher’s note
All claims expressed in this article are solely those of the authors and do not necessarily represent those of their affiliated organizations, or those of the publisher, the editors and the reviewers. Any product that may be evaluated in this article, or claim that may be made by its manufacturer, is not guaranteed or endorsed by the publisher.
References
Aggarwal, S., and Chugh, N. (2022). Review of machine learning techniques for EEG based brain computer interface. Arch. Comput. Methods Eng. 29, 3001–3020. doi: 10.1007/s11831-021-09684-6
Ahn, S., Prim, J. H., Alexander, M. L., McCulloch, K. L., and Fröhlich, F. (2019). Identifying and engaging neuronal oscillations by Transcranial alternating current stimulation in patients with chronic low Back pain: a randomized, crossover, double-blind, Sham-Controlled Pilot Study. J. Pain. 20, 277.e1–277.e11. doi: 10.1016/j.jpain.2018.09.004
Alloghani, M., Al-Jumeily, D., Mustafina, J., Hussain, A., and Aljaaf, A. J. (2020). “A systematic review on supervised and unsupervised machine learning algorithms for data science” in Supervised and unsupervised learning for data science. ed. M. W. Berry (Heidelberg: Springer), 3–21.
Apkarian, A. V., Bushnell, M. C., Treede, R.-D., and Zubieta, J.-K. (2005). Human brain mechanisms of pain perception and regulation in health and disease. Eur. J. Pain 9, 463–484. doi: 10.1016/j.ejpain.2004.11.001
Ataoğlu, E., Tiftik, T., Kara, M., Tunc, H., Ersöz, M., and Akkuş, S. (2013). Effects of chronic pain on quality of life and depression in patients with spinal cord injury. Spinal Cord 51, 23–26. doi: 10.1038/sc.2012.51
Aunon, J., and Sencaj, R. (1978). Comparison of different techniques for processing evoked potentials. Med. Biol. Eng. Comput. 16, 642–650. doi: 10.1007/BF02442443
Babiloni, C., Babiloni, F., Carducci, F., Cincotti, F., Rosciarelli, F., Arendt-Nielsen, L., et al. (2002). Human brain oscillatory activity phase-locked to painful electrical stimulations: a multi-channel EEG study. Hum. Brain Mapp. 15, 112–123. doi: 10.1002/hbm.10013
Baliki, M. N., Petre, B., Torbey, S., Herrmann, K. M., Huang, L., Schnitzer, T. J., et al. (2012). Corticostriatal functional connectivity predicts transition to chronic back pain. Nature Neurosci. 15, 1117–1119. doi: 10.1038/nn.3153
Barbosa-Torres, C., and Cubo-Delgado, S. (2021). Clinical findings in SMR neurofeedback protocol training in women with fibromyalgia syndrome. Brain Sci. 11:1069. doi: 10.3390/brainsci11081069
Baroni, A., Severini, G., Straudi, S., Buja, S., Borsato, S., and Basaglia, N. (2020). Hyperalgesia and central sensitization in subjects with chronic orofacial pain: analysis of pain thresholds and EEG biomarkers. Front. Neurosci. 14:552650. doi: 10.3389/fnins.2020.552650
Baskin, I. I., Winkler, D., and Tetko, I. V. (2016). A renaissance of neural networks in drug discovery. Expert Opin. Drug Discov. 11, 785–795. doi: 10.1080/17460441.2016.1201262
Bernardi, L., Bertuccelli, M., Formaggio, E., Rubega, M., Bosco, G., Tenconi, E., et al. (2021). Beyond physiotherapy and pharmacological treatment for fibromyalgia syndrome: tailored tACS as a new therapeutic tool. Eur. Arch. Psychiatry Clin. Neurosci. 271, 199–210. doi: 10.1007/s00406-020-01214-y
Besson, J. (1999). The neurobiology of pain. Lancet 353, 1610–1615. doi: 10.1016/S0140-6736(99)01313-6
Blankertz, B., Tomioka, R., Lemm, S., Kawanabe, M., and Müller, K. (2008). Optimal spatial filters for robust EEG single-trial analysis. IEEE Signal Process. Mag. 25, 41–56. doi: 10.1109/MSP.2008.4408441
Boccard, S. G., Pereira, E. A., Moir, L., Van Hartevelt, T. J., Kringelbach, M. L., FitzGerald, J. J., et al. (2014). Deep brain stimulation of the anterior cingulate cortex: targeting the affective component of chronic pain. Neuroreport 25, 83–88. doi: 10.1097/WNR.0000000000000039
Boonstra, A. M., Preuper, H. R. S., Reneman, M. F., Posthumus, J. B., and Stewart, R. E. (2008). Reliability and validity of the visual analogue scale for disability in patients with chronic musculoskeletal pain. Int. J. Rehabil. Res. 31, 165–169. doi: 10.1097/MRR.0b013e3282fc0f93
Bressler, S. L. (2011). “Event-related potentials of the cerebral cortex. Electrophysiological recording techniques” in Electrophysiological recording techniques, Neuromethods. eds. P. Vertes and R. W. Stackman, vol. 54 (Heidelberg: Springer)
Britton, J. W., Frey, L. C., Hopp, J. L., Korb, P., Koubeissi, M. Z., Lievens, W. E., et al. (2016) in Electroencephalography (EEG): An introductory text and Atlas of Normal and abnormal findings in adults, children, and infants. eds. E. K. ST. Louis and L. C. Frey (Chicago: American Epilepsy Society)
Brodersen, K. H., Wiech, K., Lomakina, E. I., Lin, C.-S., Buhmann, J. M., Bingel, U., et al. (2012). Decoding the perception of pain from fMRI using multivariate pattern analysis. NeuroImage 63, 1162–1170. doi: 10.1016/j.neuroimage.2012.08.035
Buchanan, D. M., Ros, T., and Nahas, R. (2021). Elevated and slowed EEG oscillations in patients with post-concussive syndrome and chronic pain following a motor vehicle collision. Brain Sci. 11:537. doi: 10.3390/brainsci11050537
Byrom, B., McCarthy, M., Schueler, P., and Muehlhausen, W. (2018). Brain monitoring devices in neuroscience clinical research: the potential of remote monitoring using sensors, wearables, and mobile devices. Clin. Pharm. Therap. 104, 59–71. doi: 10.1002/cpt.1077
Califf, R. M. (2018). Biomarker definitions and their applications. Exp. Biol. Med. 243, 213–221. doi: 10.1177/1535370217750088
Camfferman, D., Moseley, G. L., Gertz, K., Pettet, M. W., and Jensen, M. P. (2017). Waking EEG cortical markers of chronic pain and sleepiness. Pain Med. 18, 1921–1931. doi: 10.1093/pm/pnw294
Cao, Z., Lai, K.-L., Lin, C.-T., Chuang, C.-H., Chou, C.-C., and Wang, S.-J. (2018). Exploring resting-state EEG complexity before migraine attacks. Cephalalgia 38, 1296–1306. doi: 10.1177/0333102417733953
Cao, T., Wang, Q., Liu, D., Sun, J., and Bai, O. (2020). Resting state EEG-based sudden pain recognition method and experimental study. Biomed. Signal Process Control 59:101925. doi: 10.1016/j.bspc.2020.101925
Chang, P., Arendt-Nielsen, L., Graven-Nielsen, T., Svensson, P., and Chen, A. C. (2001). Different EEG topographic effects of painful and non-painful intramuscular stimulation in man. Exp. Brain Res. 141, 195–203. doi: 10.1007/s002210100864
Chapman, C. R., and Vierck, C. J. (2017). The transition of acute postoperative pain to chronic pain: an integrative overview of research on mechanisms. J. Pain 18, e1–e38. doi: 10.1016/j.jpain.2016.11.004
Chen, Z. S. (2021). Decoding pain from brain activity. J. Neural Eng. 18:051002. doi: 10.1088/1741-2552/ac28d4
Chen, J. C., Yao, K., and Hudson, R. E. (2002). Source localization and beamforming. IEEE Signal Process. Mag. 19, 30–39. doi: 10.1109/79.985676
Chen, D., Zhang, H., Kavitha, P. T., Loy, F. L., Ng, S. H., Wang, C., et al. (2022). Scalp EEG-based pain detection using convolutional neural network. IEEE Trans. Neural Syst. Rehabil. Eng. 30, 274–285. doi: 10.1109/TNSRE.2022.3147673
Chicco, D. T. (2017). Quick tips for machine learning in computational biology. BioData Mining 10:35. doi: 10.1186/s13040-017-0155-3
Chowdhury, N. S., Skippen, P., Si, E., Chiang, A. K. I., Millard, S. K., Furman, A. J., et al. (2023). The reliability of two prospective cortical biomarkers for pain: EEG peak alpha frequency and TMS corticomotor excitability. J. Neurosci. Methods 385:109766. doi: 10.1016/j.jneumeth.2022.109766
Dahlhamer, J., Lucas, J., Zelaya, C., Nahin, R., Mackey, S., DeBar, L., et al. (2018). Prevalence of chronic pain and high-impact chronic pain among adults—United States, 2016. MMWR Morb. Mortal. Wkly Rep. 67:1001. doi: 10.15585/mmwr.mm6736a2
Dansie, E., and Turk, D. C. (2013). Assessment of patients with chronic pain. Br. J. Anaesth. 111, 19–25. doi: 10.1093/bja/aet124
Davis, K. D., Aghaeepour, N., Ahn, A. H., Angst, M. S., Borsook, D., Brenton, A., et al. (2020). Discovery and validation of biomarkers to aid the development of safe and effective pain therapeutics: challenges and opportunities. Nat. Rev. Neurol. 16, 381–400. doi: 10.1038/s41582-020-0362-2
de Melo, G. A., de Oliveira, E. A., dos Santos Andrade, S. M. M., Fernández-Calvo, B., and Torro, N. (2020). Comparison of two tDCS protocols on pain and EEG alpha-2 oscillations in women with fibromyalgia. Sci. Rep. 10:18955. doi: 10.1038/s41598-020-75861-5
De Vries, M., Wilder-Smith, O. H., Jongsma, M. L., van den Broeke, E. N., Arns, M., Van Goor, H., et al. (2013). Altered resting state EEG in chronic pancreatitis patients: toward a marker for chronic pain. J. Pain Res. 6:815. doi: 10.2147/JPR.S50919
Di Pietro, F., Macey, P. M., Rae, C. D., Alshelh, Z., Macefield, V. G., Vickers, E. R., et al. (2018). The relationship between thalamic GABA content and resting cortical rhythm in neuropathic pain. Hum. Brain Mapp. 39, 1945–1956. doi: 10.1002/hbm.23973
Dinh, S. T., Nickel, M. M., Tiemann, L., May, E. S., Heitmann, H., Hohn, V. D., et al. (2019). Brain dysfunction in chronic pain patients assessed by resting-state electroencephalography. Pain 160:2751. doi: 10.1097/j.pain.0000000000001666
Duerden, E. G., and Albanese, M. C. (2013). Localization of pain-related brain activation: a meta-analysis of neuroimaging data. Hum. Brain Mapp. 34, 109–149. doi: 10.1002/hbm.21416
Einevoll, G. T., Kayser, C., Logothetis, N. K., and Panzeri, S. (2013). Modelling and analysis of local field potentials for studying the function of cortical circuits. Nat. Rev. Neurosci. 14, 770–785. doi: 10.1038/nrn3599
Eldabe, S., Obara, I., Panwar, C., and Caraway, D. (2022). Biomarkers for chronic pain: significance and summary of recent advances. Pain Res. Manag. doi: 10.1155/2022/1940906
Fallon, N., Chiu, Y., Nurmikko, T., and Stancak, A. (2018). Altered theta oscillations in resting EEG of fibromyalgia syndrome patients. Eur. J. Pain 22, 49–57. doi: 10.1002/ejp.1076
Feng, L., Li, H., Cui, H., Xie, X., Xu, S., and Hu, Y. (2021). Low back pain assessment based on alpha oscillation changes in spontaneous electroencephalogram (EEG). Neural Plast. 2021. doi: 10.1155/2021/8537437
Ferdek, M. A., Oosterman, J. M., Adamczyk, A. K., van Aken, M., Woudsma, K. J., Peeters, B. W., et al. (2019). Effective connectivity of beta oscillations in endometriosis-related chronic pain during rest and pain-related mental imagery. J. Pain 20, 1446–1458. doi: 10.1016/j.jpain.2019.05.011
Fernandez Rojas, R., Huang, X., and Ou, K.-L. A. (2019). Machine learning approach for the identification of a biomarker of human pain using fNIRS. Sci. Rep. 9, 1–12. doi: 10.1038/s41598-019-42098-w
Ferracuti, S., Seri, S., Mattia, D., and Cruccu, G. (1994). Quantitative EEG modifications during the cold water pressor test: hemispheric and hand differences. Int. J. Psychophysiol. 17, 261–268. doi: 10.1016/0167-8760(94)90068-x
Freye, E., and Levy, J. (2006). The effects of tramadol on pain relief, fast EEG-power spectrum and cognitive function in elderly patients with chronic osteoarthritis (OA). Acute. Pain. 8, 55–61. doi: 10.1016/j.acpain.2006.03.001
Furman, A. J., Meeker, T. J., Rietschel, J. C., Yoo, S., Muthulingam, J., Prokhorenko, M., et al. (2018). Cerebral peak alpha frequency predicts individual differences in pain sensitivity. NeuroImage 167, 203–210. doi: 10.1016/j.neuroimage.2017.11.042
Furman, A. J., Prokhorenko, M., Keaser, M. L., Zhang, J., Chen, S., Mazaheri, A., et al. (2020). Sensorimotor peak alpha frequency is a reliable biomarker of prolonged pain sensitivity. Cereb. Cortex 30, 6069–6082. doi: 10.1093/cercor/bhaa124
Furman, A. J., Thapa, T., Summers, S. J., Cavaleri, R., Fogarty, J. S., Steiner, G. Z., et al. (2019). Cerebral peak alpha frequency reflects average pain severity in a human model of sustained, musculoskeletal pain. J. Neurophysiol. 122, 1784–1793. doi: 10.1152/jn.00279.2019
Gan, T. J. (2017). Poorly controlled postoperative pain: prevalence, consequences, and prevention. J. Pain Res. 10:2287. doi: 10.2147/JPR.S144066
Gan, T. J., Habib, A. S., Miller, T. E., White, W., and Apfelbaum, J. L. (2014). Incidence, patient satisfaction, and perceptions of post-surgical pain: results from a US national survey. Curr. Med. Res. Opin. 30, 149–160. doi: 10.1185/03007995.2013.860019
Gardner, J., and Sachdeva, H. (2019). Causes of pain worldwide. Pain, 1191–1192. doi: 10.1007/978-3-319-99124-5_253
Gatchel, R. J., Fuchs, P. N., and Allen, C. (2016). “Ethical issues in chronic pain research” in Ethical Issues in Chronic Pain Management. ed. M. E. Schatman (Boca Raton: CRC Press)
Gil Avila, C., Bott, F. S., Tiemann, L., Hohn, V. D., May, E. S., Nickel, M. M., et al. (2023). DISCOVER-EEG: an open, fully automated EEG pipeline for biomarker discovery in clinical neuroscience. bioRxiv 2023, 20:524897. doi: 10.1101/2023.01.20.524897
González-Roldán, A. M., Cifre, I., Sitges, C., and Montoya, P. (2016). Altered dynamic of EEG oscillations in fibromyalgia patients at rest. Pain Med. 17, 1058–1068. doi: 10.1093/pm/pnw023
González-Villar, A. J., Triñanes, Y., Gómez-Perretta, C., and Carrillo-de-la-Peña, M. T. (2020). Patients with fibromyalgia show increased beta connectivity across distant networks and microstates alterations in resting-state electroencephalogram. NeuroImage 223:117266. doi: 10.1016/j.neuroimage.2020.117266
Gram, M., Erlenwein, J., Petzke, F., Falla, D., Przemeck, M., Emons, M., et al. (2017). Prediction of postoperative opioid analgesia using clinical-experimental parameters and electroencephalography. Eur. J. Pain 21, 264–277. doi: 10.1002/ejp.921
Graversen, C., Olesen, S. S., Olesen, A. E., Steimle, K., Farina, D., Wilder-Smith, O. H., et al. (2012). The analgesic effect of pregabalin in patients with chronic pain is reflected by changes in pharmaco-EEG spectral indices. Br J. PClin. harmacol. 73, 363–372. doi: 10.1111/j.1365-2125.2011.04104.x
Group, F. N. B. W. (2016). “BEST (biomarkers, endpoints, and other tools) resource [internet]” in Silver spring (MD): Food and Drug Administration (US) (Bethesda (MD): National Institutes of Health (US))
Gunn, J., Hill, M. M., Cotten, B. M., and Deer, T. R. (2020). An analysis of biomarkers in patients with chronic pain. Pain Physician 23:E41. doi: 10.36076/PPJ.2020/23/E41
Guo, X., Zhang, Q., Singh, A., Wang, J., and Chen, Z. S. (2020). Granger causality analysis of rat cortical functional connectivity in pain. J. Neural Eng. 17:016050. doi: 10.1088/1741-2552/ab6cba
Hadjileontiadis, L. J. (2015). EEG-based tonic cold pain characterization using wavelet higher order spectral features. I.E.E.E. Trans. Biomed. Eng. 62, 1981–1991. doi: 10.1109/TBME.2015.2409133
Haefeli, M., and Elfering, A. (2006). Pain assessment. Eur. Spine J. 15, S17–S24. doi: 10.1007/s00586-005-1044-x
Hallez, H., Vanrumste, B., Grech, R., Muscat, J., De Clercq, W., Vergult, A., et al. (2007). Review on solving the forward problem in EEG source analysis. J. Neuroeng. Rehabil. 4, 1–29. doi: 10.1186/1743-0003-4-46
Hammal, Z., and Cohn, J. F. (2012). Automatic detection of pain intensity. Proc ACM Int. Conf. Multimodal. Interact. 2012, 47–52. doi: 10.1145/2388676.2388688
Hansen, T. M., Mark, E. B., Olesen, S. S., Gram, M., Frøkjær, J. B., and Drewes, A. M. (2017). Characterization of cortical source generators based on electroencephalography during tonic pain. J. Pain Res. 10:1401. doi: 10.2147/JPR.S132909
Harland, T., Hadanny, A., and Pilitsis, J. G. (2022). Machine learning and pain outcomes. Neurosurg. Clin. N. Am. 33, 351–358. doi: 10.1016/j.nec.2022.02.012
Hasenstab, K., Sugar, C. A., Telesca, D., McEvoy, K., Jeste, S., and Şentürk, D. (2015). Identifying longitudinal trends within EEG experiments. Biometrics 71, 1090–1100. doi: 10.1111/biom.12347
Heitmann, H., Ávila, C. G., Nickel, M. M., Dinh, S. T., May, E. S., Tiemann, L., et al. (2022). Longitudinal resting-state electroencephalography in patients with chronic pain undergoing interdisciplinary multimodal pain therapy. Pain 163, e997–e1005. doi: 10.1097/j.pain.0000000000002565
Herrmann, C. S., and Knight, R. T. (2001). Mechanisms of human attention: event-related potentials and oscillations. Neurosci. Biobehav. Rev. 25, 465–476. doi: 10.1016/s0149-7634(01)00027-6
Hollmann, M. W., Rathmell, J. P., and Lirk, P. (2019). Optimal postoperative pain management: redefining the role for opioids. Lancet 393, 1483–1485. doi: 10.1016/S0140-6736(19)30854-2
Hosseini, M.-P., Hosseini, A., and Ahi, K. (2020). A review on machine learning for EEG signal processing in bioengineering. IEEE Rev. Biomed. Eng. 14, 204–218. doi: 10.1109/RBME.2020.2969915
Hu, L., and Iannetti, G. D. (2016). Painful issues in pain prediction. Trends Neurosci. 39, 212–220. doi: 10.1016/j.tins.2016.01.004
Hu, L., and Zhang, Z., editors. EEG signal processing and feature extraction, Springer, Heidelberg (2019).
Huber, M., Bartling, J., Pachur, D. V., Woikowsky-Biedau, S., and Lautenbacher, S. (2006). EEG responses to tonic heat pain. Exp. Brain Res. 173, 14–24. doi: 10.1007/s00221-006-0366-1
Iannetti, G. D., Hughes, N. P., Lee, M. C., and Mouraux, A. (2008). Determinants of laser-evoked EEG responses: pain perception or stimulus saliency? J. Neurophysiol. 100, 815–828. doi: 10.1152/jn.00097.2008
Inouye, T., Shinosaki, K., Sakamoto, H., Toi, S., Ukai, S., Iyama, A., et al. (1991). Quantification of EEG irregularity by use of the entropy of the power spectrum. Electroencephalogr. Clin. Neurophysiol. 79, 204–210. doi: 10.1016/0013-4694(91)90138-t
Isnard, J., Magnin, M., Jung, J., Mauguière, F., and Garcia-Larrea, L. (2011). Does the insula tell our brain that we are in pain? Pain 152, 946–951. doi: 10.1016/j.pain.2010.12.025
Jackson, A. F., and Bolger, D. J. (2014). The neurophysiological bases of EEG and EEG measurement: a review for the rest of us. Psychophysiology 51, 1061–1071. doi: 10.1111/psyp.12283
Jas, M., Engemann, D. A., Bekhti, Y., Raimondo, F., and Gramfort, A. (2017). Autoreject: automated artifact rejection for MEG and EEG data. NeuroImage 159, 417–429. doi: 10.1016/j.neuroimage.2017.06.030
Jensen, M. P., Gertz, K. J., Kupper, A. E., Braden, A. L., Howe, J. D., Hakimian, S., et al. (2013). Steps toward developing an EEG biofeedback treatment for chronic pain. Appl. Psychophysiol. Biofeedback 38, 101–108. doi: 10.1007/s10484-013-9214-9
Jensen, M. P., Hakimian, S., Ehde, D. M., Day, M. A., Pettet, M. W., Yoshino, A., et al. (2021). Pain-related beliefs, cognitive processes, and electroencephalography band power as predictors and mediators of the effects of psychological chronic pain interventions. Pain 162:2036. doi: 10.1097/j.pain.0000000000002201
Jensen, M. P., Hakimian, S., Sherlin, L. H., and Fregni, F. (2008). New insights into neuromodulatory approaches for the treatment of pain. J. Pain 9, 193–199. doi: 10.1016/j.jpain.2007.11.003
Jenssen, M. D. K., Bakkevoll, P. A., Ngo, P. D., Budrionis, A., Fagerlund, A. J., Tayefi, M., et al. (2021). Machine learning in chronic pain research: a scoping review. Appl. Sci. 11:3205. doi: 10.3390/app11073205
Jia, X., Li, K., Li, X., and Zhang, A. (2014). A novel semi-supervised deep learning framework for affective state recognition on eeg signals. IEEE Int. Conf. Bioinformatics Bioeng., 30–37. doi: 10.1109/BIBE.2014.26
Jiang, X., Bian, G.-B., and Tian, Z. (2019). Removal of artifacts from EEG signals: a review. Sensors 19:987. doi: 10.3390/s19050987
Jobert, M., Wilson, F. J., Ruigt, G. S., Brunovsky, M., Prichep, L. S., Drinkenburg, W. H., et al. (2012). Guidelines for the recording and evaluation of pharmaco-EEG data in man: the international Pharmaco-EEG society (IPEG). Neuropsychobiology 66, 201–220. doi: 10.1159/000343478
Johannes, C. B., Le, T. K., Zhou, X., Johnston, J. A., and Dworkin, R. H. (2010). The prevalence of chronic pain in United States adults: results of an internet-based survey. J. Pain 11, 1230–1239. doi: 10.1016/j.jpain.2010.07.002
Katsigiannis, S., and Ramzan, N. (2017). DREAMER: a database for emotion recognition through EEG and ECG signals from wireless low-cost off-the-shelf devices. IEEE J. Biomedical. Health Inform. 22, 98–107. doi: 10.1109/JBHI.2017.2688239
Kehlet, H., Jensen, T. S., and Woolf, C. J. (2006). Persistent postsurgical pain: risk factors and prevention. Lancet 367, 1618–1625. doi: 10.1016/S0140-6736(06)68700-X
Khanna, A., Pascual-Leone, A., Michel, C. M., and Farzan, F. (2015). Microstates in resting-state EEG: current status and future directions. Neurosci. Biobehav. Rev. 49, 105–113. doi: 10.1016/j.neubiorev.2014.12.010
Kimura, A., Mitsukura, Y., Oya, A., Matsumoto, M., Nakamura, M., Kanaji, A., et al. (2021). Objective characterization of hip pain levels during walking by combining quantitative electroencephalography with machine learning. Sci. Rep. 11, 1–10. doi: 10.1038/s41598-021-82696-1
Kinnealey, M., and Fuiek, M. (1999). The relationship between sensory defensiveness, anxiety, depression and perception of pain in adults. Occup. Ther. Int. 6, 195–206. doi: 10.1002/oti.97
Kragel, P. A., Koban, L., Barrett, L. F., and Wager, T. D. (2018). Representation, pattern information, and brain signatures: from neurons to neuroimaging. Neuron 99, 257–273. doi: 10.1016/j.neuron.2018.06.009
Krigolson, O. E., Williams, C. C., Norton, A., Hassall, C. D., and Colino, F. L. (2017). Choosing MUSE: validation of a low-cost, portable EEG system for ERP research. Front. Neurosci. 11:109. doi: 10.3389/fnins.2017.00109
Kucyi, A., and Davis, K. D. (2015). The dynamic pain connectome. Trends. Neurosciences 38, 86–95. doi: 10.1016/j.tins.2014.11.006
Ladha, K. S., Patorno, E., Huybrechts, K. F., Liu, J., Rathmell, J. P., and Bateman, B. T. (2016). Variations in the use of perioperative multimodal analgesic therapy. Anesthesiology 124, 837–845. doi: 10.1097/ALN.0000000000001034
Lamichhane, B., Jayasekera, D., Jakes, R., Glasser, M. F., Zhang, J., Yang, C., et al. (2021). Multi-modal biomarkers of low back pain: a machine learning approach. Neuroimage Clin. 29:102530. doi: 10.1016/j.nicl.2020.102530
Lancaster, J., Mano, H., Callan, D., Kawato, M., and Seymour, B. (2017). Decoding acute pain with combined EEG and physiological data. IEEE Int. Conf. Neural. Eng., 521–524. doi: 10.1109/NER.2017.8008404
Le Pera, D., Svensson, P., Valeriani, M., Watanabe, I., Arendt-Nielsen, L., and Chen, A. C. (2000). Long-lasting effect evoked by tonic muscle pain on parietal EEG activity in humans. Clin. Neurophysiol. 111, 2130–2137. doi: 10.1016/s1388-2457(00)00474-0
Lee, C. H., Kim, H. S., Kim, Y.-S., Jung, S., Yoon, C. H., and Kwon, O.-Y. (2021). Cerebral current-source distribution associated with pain improvement by non-invasive painless signaling therapy in patients with failed back surgery syndrome. Korean J. Pain. 34, 437–446. doi: 10.3344/kjp.2021.34.4.437
Lendaro, E., Balouji, E., Baca, K., Muhammad, A. S., and Ortiz-Catalan, M. (2021). Common spatial pattern EEG decomposition for phantom limb pain detection. Ann. Int. Conf. IEEE Eng. Med. Biol. Soc. 2021, 726–729. doi: 10.1109/EMBC46164.2021.9630561
Levitt, J., Edhi, M. M., Thorpe, R. V., Leung, J. W., Michishita, M., Koyama, S., et al. (2020). Pain phenotypes classified by machine learning using electroencephalography features. NeuroImage 223:117256. doi: 10.1016/j.neuroimage.2020.117256
Levitt, J., and Saab, C. Y. (2019). What does a pain ‘biomarker’mean and can a machine be taught to measure pain? Neurosci. Lett. 702, 40–43. doi: 10.1016/j.neulet.2018.11.038
Liberati, G., Klöcker, A., Algoet, M., Mulders, D., Maia Safronova, M., Ferrao Santos, S., et al. (2018). Gamma-band oscillations preferential for nociception can be recorded in the human insula. Cereb. Cortex 28, 3650–3664. doi: 10.1093/cercor/bhx237
Liberati, G., Mulders, D., Algoet, M., van den Broeke, E. N., Santos, S. F., Ribeiro Vaz, J. G., et al. (2020). Insular responses to transient painful and non-painful thermal and mechanical spinothalamic stimuli recorded using intracerebral EEG. Sci. Rep. 10:22319. doi: 10.1038/s41598-020-79371-2
Liu, C., Chien, J., Chang, Y., Kim, J., Anderson, W., and Lenz, F. (2015). Functional role of induced gamma oscillatory responses in processing noxious and innocuous sensory events in humans. Neuroscience 310, 389–400. doi: 10.1016/j.neuroscience.2015.09.047
Logothetis, N. K. (2008). What we can do and what we cannot do with fMRI. Nature 453, 869–878. doi: 10.1038/nature06976
Lötsch, J., Ultsch, A., and Kalso, E. (2017). Prediction of persistent post-surgery pain by preoperative cold pain sensitivity: biomarker development with machine-learning-derived analysis. BJA. Br. J. Anaesth. 119, 821–829. doi: 10.1093/bja/aex236
Lu, H., Eng, H.-L., Guan, C., Plataniotis, K. N., and Venetsanopoulos, A. N. (2010). Regularized common spatial pattern with aggregation for EEG classification in small-sample setting. IEEE Trans. Biomed. Eng. 57, 2936–2946. doi: 10.1109/TBME.2010.2082540
Mari, T., Henderson, J., Maden, M., Nevitt, S., Duarte, R., and Fallon, N. (2022). Systematic review of the effectiveness of machine learning algorithms for classifying pain intensity, phenotype or treatment outcomes using electroencephalogram data. J. Pain. doi: 10.1016/j.jpain.2021.07.011
Martel, M., Harvey, M.-P., Houde, F., Balg, F., Goffaux, P., and Léonard, G. (2017). Unravelling the effect of experimental pain on the corticomotor system using transcranial magnetic stimulation and electroencephalography. Exp. Brain Res. 235, 1223–1231. doi: 10.1007/s00221-017-4880-0
Martín-Brufau, R., Gómez, M. N., Sanchez-Sanchez-Rojas, L., and Nombela, C. (2021). Fibromyalgia detection based on EEG connectivity patterns. J. Clin. Med. 10:3277. doi: 10.3390/jcm10153277
Matsangidou, M., Liampas, A., Pittara, M., Pattichi, C. S., and Zis, P. (2021). Machine learning in pain medicine: an up-to-date systematic review. Pain Ther., 10, 1067–1084. doi: 10.1007/s40122-021-00324-2
May, E. S., Ávila, C. G., Dinh, S. T., Heitmann, H., Hohn, V. D., Nickel, M. M., et al. (2021). Dynamics of brain function in patients with chronic pain assessed by microstate analysis of resting-state electroencephalography. Pain 162:2894. doi: 10.1097/j.pain.0000000000002281
May, E. S., Nickel, M. M., Ta Dinh, S., Tiemann, L., Heitmann, H., Voth, I., et al. (2019). Prefrontal gamma oscillations reflect ongoing pain intensity in chronic back pain patients. Hum. Brain Mapp. 40, 293–305. doi: 10.1002/hbm.24373
Mendonça-de-Souza, M., Monteiro, U. M., Bezerra, A. S., Silva-de-Oliveira, A. P., Ventura-da-Silva, B. R., Barbosa, M. S., et al. (2012). Resilience in migraine brains: decrease of coherence after photic stimulation. Front. Hum. Neurosci. 6:207. doi: 10.3389/fnhum.2012.00207
Meneses, F. M., Queirós, F. C., Montoya, P., Miranda, J. G., Dubois-Mendes, S. M., Sá, K. N., et al. (2016). Patients with rheumatoid arthritis and chronic pain display enhanced alpha power density at rest. Front. Hum. Neurosci. 10:395. doi: 10.3389/fnhum.2016.00395
Merk, T., Peterson, V., Köhler, R., Haufe, S., Richardson, R. M., and Neumann, W.-J. (2022). Machine learning based brain signal decoding for intelligent adaptive deep brain stimulation. Exp. Neurol. :113993. doi: 10.1016/j.expneurol.2022
Michel, C. M., and Brunet, D. (2019). EEG source imaging: a practical review of the analysis steps. Front. Neurol. 10:325. doi: 10.3389/fneur.2019.00325
Millard, S. K., Furman, A. J., Kerr, A., Seminowicz, D. A., Gao, F., Naidu, B. V., et al. (2022). Predicting postoperative pain in lung cancer patients using preoperative peak alpha frequency. Br. J. Anaesth. 128, e346–e348. doi: 10.1016/j.bja.2022.03.006
Misra, G., Wang, W., Archer, D. B., Roy, A., and Coombes, S. A. (2017). Automated classification of pain perception using high-density electroencephalography data. J. Neurophysiol. 117, 786–795. doi: 10.1152/jn.00650.2016
Mokhtari, T., Tu, Y., and Hu, L. (2019). Involvement of the hippocampus in chronic pain and depression. Brain Sci. Adv. 5, 288–298. doi: 10.26599/BSA.2019.90500
Morton, D. L., Sandhu, J. S., and Jones, A. K. (2016). Brain imaging of pain: state of the art. J. Pain Res. 9:613. doi: 10.2147/JPR.S60433
Mouraux, A., and Iannetti, G. D. (2018). The search for pain biomarkers in the human brain. Brain 141, 3290–3307. doi: 10.1093/brain/awy281
Müller, K.-R., Tangermann, M., Dornhege, G., Krauledat, M., Curio, G., and Blankertz, B. (2008). Machine learning for real-time single-trial EEG-analysis: from brain–computer interfacing to mental state monitoring. J. Neurosci. Methods 167, 82–90. doi: 10.1016/j.jneumeth.2007.09.022
Mussigmann, T., Bardel, B., and Lefaucheur, J.-P. (2022). Resting-state electroencephalography (EEG) biomarkers of chronic neuropathic pain. A systematic review. NeuroImage :119351. doi: 10.1016/j.neuroimage.2022.119351
Navarro López, J., Moral Bergós, R., and Marijuán, P. C. (2015). Significant new quantitative EGG patterns in fibromyalgia. Eur. J. Psychiat. 29, 277–292. doi: 10.4321/S0213-61632015000400005
Necka, E. A., Lee, I.-S., Kucyi, A., Cheng, J. C., Yu, Q., and Atlas, L. Y. (2019). Applications of dynamic functional connectivity to pain and its modulation. Pain Rep. :4:e752. doi: 10.1097/PR9.0000000000000752
Nickel, M. M., May, E. S., Tiemann, L., Postorino, M., Dinh, S. T., and Ploner, M. (2017). Autonomic responses to tonic pain are more closely related to stimulus intensity than to pain intensity. Pain 158, 2129–2136. doi: 10.1097/j.pain.0000000000001010
Nurse, E., Mashford, B. S., Yepes, A. J., Kiral-Kornek, I., Harrer, S., and Freestone, D. R. (2016). Decoding EEG and LFP signals using deep learning: heading TrueNorth. ACM Int. Conf. Comut. Front., 259–266. doi: 10.1145/2903150.2903159
Olejarczyk, E., Marzetti, L., Pizzella, V., and Zappasodi, F. (2017). Comparison of connectivity analyses for resting state EEG data. J. Neural Eng. 14:036017. doi: 10.1088/1741-2552/aa6401
Parker, T., Raghu, A., Huang, Y., Gillies, M. J., FitzGerald, J. J., Aziz, T., et al. (2021). Paired acute invasive/non-invasive stimulation (PAINS) study: a phase I/II randomized, sham-controlled crossover trial in chronic neuropathic pain. Brain Stimul. 14, 1576–1585. doi: 10.1016/j.brs.2021.10.384
Patel, K., Henshaw, J., Sutherland, H., Taylor, J. R., Casson, A. J., Lopez-Diaz, K., et al. (2021). Using EEG alpha states to understand learning during alpha neurofeedback training for chronic pain. Front. Neurosci. 14:620666. doi: 10.3389/fnins.2020.620666
Pedroni, A., Bahreini, A., and Langer, N. (2019). Automagic: standardized preprocessing of big EEG data. NeuroImage 200, 460–473. doi: 10.1016/j.neuroimage.2019.06.046
Peng, W., and Tang, D. (2016). Pain related cortical oscillations: methodological advances and potential applications. Front. Comput. Neurosci. 10:9. doi: 10.3389/fncom.2016.00009
Perl, E. R. (2007). Ideas about pain, a historical view. Nat. Rev. Neurosci. 8, 71–80. doi: 10.1038/nrn2042
Peyron, R., Frot, M., Schneider, F., Garcia-Larrea, L., Mertens, P., Barral, F.-G., et al. (2002). Role of operculoinsular cortices in human pain processing: converging evidence from PET, fMRI, dipole modeling, and intracerebral recordings of evoked potentials. NeuroImage 17, 1336–1346. doi: 10.1006/nimg.2002.1315
Pfurtscheller, G. (2001). Functional brain imaging based on ERD/ERS. Vision Res 41:1257–60. doi: 10.1016/s0042-6989(00)00235-2
Pinheiro, E. S. D. S., Queirós, F. C. D., Montoya, P., Santos, C. L., Nascimento, M. A. D., Ito, C. H., et al. (2016). Electroencephalographic patterns in chronic pain: a systematic review of the literature. PLoS One 11:e0149085. doi: 10.1371/journal.pone.0149085
Plaghki, L., and Mouraux, A. (2005). EEG and laser stimulation as tools for pain research. Curr. Opin. Investig. Drugs 6, 58–64.
Ploner, M., Sorg, C., and Gross, J. (2017). Brain rhythms of pain. Trends Cogn. Sci. 21, 100–110. doi: 10.1016/j.tics.2016.12.001
Power, J. D., Plitt, M., Laumann, T. O., and Martin, A. (2017). Sources and implications of whole-brain fMRI signals in humans. NeuroImage 146, 609–625. doi: 10.1016/j.neuroimage.2016.09.038
Price, D. D. (2000). Psychological and neural mechanisms of the affective dimension of pain. Science 288, 1769–1772. doi: 10.1126/science.288.5472.1769
Prichep, L. S., Shah, J., Merkin, H., and Hiesiger, E. M. (2018). Exploration of the pathophysiology of chronic pain using quantitative EEG source localization. Clin. EEG Neurosci. 49, 103–113. doi: 10.1177/1550059417736444
Prinsloo, S., Novy, D., Driver, L., Lyle, R., Ramondetta, L., Eng, C., et al. (2017). Randomized controlled trial of neurofeedback on chemotherapy-induced peripheral neuropathy: a pilot study. Cancer 123, 1989–1997. doi: 10.1002/cncr.30649
Pu, L., Lion, K. M., Todorovic, M., and Moyle, W. (2021). Portable EEG monitoring for older adults with dementia and chronic pain - a feasibility study. Geriatr. Nurs. 42, 124–128. doi: 10.1016/j.gerinurse.2020.12.008
Quiton, R. L., and Greenspan, J. D. (2008). Across-and within-session variability of ratings of painful contact heat stimuli. Pain 137, 245–256. doi: 10.1016/j.pain.2007.08.034
Rainville, P., Duncan, G. H., Price, D. D., Carrier, B., and Bushnell, M. C. (1997). Pain affect encoded in human anterior cingulate but not somatosensory cortex. Science 277, 968–971. doi: 10.1126/science.277.5328.968
Reckziegel, D., Vachon-Presseau, E., Petre, B., Schnitzer, T. J., Baliki, M., and Apkarian, A. V. (2019). Deconstructing biomarkers for chronic pain: context and hypothesis dependent biomarker types in relation to chronic pain. Pain 160:S37. doi: 10.1097/j.pain.0000000000001529
Riaz, F., Hassan, A., Rehman, S., Niazi, I. K., and Dremstrup, K. (2015). EMD-based temporal and spectral features for the classification of EEG signals using supervised learning. IEEE Trans. Neural Syst. Rehabil. Eng. 24, 28–35. doi: 10.1109/TNSRE.2015.2441835
Rosa, M. J., and Seymour, B. (2014). Decoding the matrix: benefits and limitations of applying machine learning algorithms to pain neuroimaging. Pain 155, 864–867. doi: 10.1016/j.pain.2014.02.013
Rouleau, R. D., Lagrandeur, L., Daigle, K., Lorrain, D., Léonard, G., Whittingstall, K., et al. (2015). Significance of non-phase locked oscillatory brain activity in response to noxious stimuli. Can. J. Neurol. Sci. 42, 436–443. doi: 10.1017/cjn.2015.294
Sakkalis, V. (2011). Review of advanced techniques for the estimation of brain connectivity measured with EEG/MEG. Comput. Biol. Med. 41, 1110–1117. doi: 10.1016/j.compbiomed.2011.06.020
Santana, J. E. R., Baptista, A. F., Lucena, R., Lopes, T. D. S., do Rosário, R. S., Xavier, M. R., et al. (2021). Altered dynamic brain connectivity in individuals with sickle cell disease and chronic pain secondary to hip osteonecrosis. Clin. EEG Neurosci. :15500594211054297. doi: 10.1177/15500594211054297
Sawamoto, N., Honda, M., Okada, T., Hanakawa, T., Kanda, M., Fukuyama, H., et al. (2000). Expectation of pain enhances responses to nonpainful somatosensory stimulation in the anterior cingulate cortex and parietal operculum/posterior insula: an event-related functional magnetic resonance imaging study. J. Neurosci. 20, 7438–7445. doi: 10.1523/JNEUROSCI.20-19-07438.2000
Schmidt, S., Gmeiner, S., Schultz, C., Löwer, M., Kuhn, K., Naranjo, J. R., et al. (2015). Mindfulness-based stress reduction (MBSR) as treatment for chronic back pain-an observational study with assessment of thalamocortical dysrhythmia. Complement. Med. Res. 22, 298–303. doi: 10.1159/000440687
Schmidt, S., Naranjo, J. R., Brenneisen, C., Gundlach, J., Schultz, C., Kaube, H., et al. (2012). Pain ratings, psychological functioning and quantitative EEG in a controlled study of chronic back pain patients. PLoS One 7:e31138. doi: 10.1371/journal.pone.0031138
Schoffelen, J. M., and Gross, J. (2009). Source connectivity analysis with MEG and EEG. Hum. Brain Mapp. 30, 1857–1865. doi: 10.1002/hbm.20745
Schouppe, S., Van Oosterwijck, S., Danneels, L., Van Damme, S., and Van Oosterwijck, J. (2020). Are functional brain alterations present in low back pain? A systematic review of EEG studies. J. Pain 21, 25–43. doi: 10.1016/j.jpain.2019.06.010
Schuchat, A., Houry, D., and Guy, G. P. (2017). New data on opioid use and prescribing in the United States. JAMA 318, 425–426. doi: 10.1001/jama.2017.8913
Schulz, E., May, E. S., Postorino, M., Tiemann, L., Nickel, M. M., Witkovsky, V., et al. (2015). Prefrontal gamma oscillations encode tonic pain in humans. Cereb. Cortex 25, 4407–4414. doi: 10.1093/cercor/bhv043
Schulz, E., Tiemann, L., Witkovsky, V., Schmidt, P., and Ploner, M. (2012a). Gamma oscillations are involved in the sensorimotor transformation of pain. J. Neurophysiol. 108, 1025–1031. doi: 10.1152/jn.00186.2012
Schulz, E., Zherdin, A., Tiemann, L., Plant, C., and Ploner, M. (2012b). Decoding an individual's sensitivity to pain from the multivariate analysis of EEG data. Cereb. Cortex 22, 1118–1123. doi: 10.1093/cercor/bhr186
Seeber, M., Cantonas, L.-M., Hoevels, M., Sesia, T., Visser-Vandewalle, V., and Michel, C. M. (2019). Subcortical electrophysiological activity is detectable with high-density EEG source imaging. Nat. Commun. 10:753. doi: 10.1038/s41467-019-08725-w
Seminowicz, D. A., Thapa, T., Furman, A. J., Summers, S. J., Cavaleri, R., Fogarty, J. S., et al. (2018). Slow peak alpha frequency and corticomotor depression linked to high pain susceptibility in transition to sustained pain. BioRxiv :278598. doi: 10.1101/278598
Seminowicz, D. A., Thapa, T., and Schabrun, S. M. (2019). Corticomotor depression is associated with higher pain severity in the transition to sustained pain: a longitudinal exploratory study of individual differences. J. Pain 20, 1498–1506. doi: 10.1016/j.jpain.2019.06.005
She, Q., Hu, B., Luo, Z., Nguyen, T., and Zhang, Y. (2019). A hierarchical semi-supervised extreme learning machine method for EEG recognition. Med. Biol. Eng. Comput. 57, 147–157. doi: 10.1007/s11517-018-1875-3
Siddiqi, S. H., Kording, K. P., Parvizi, J., and Fox, M. D. (2022). Causal mapping of human brain function. Nat. Rev. Neurosci. 23, 361–375. doi: 10.1038/s41583-022-00583-8
So, H. C. (2011). “source localization: algorithms and analysis” in Handbook of position location: Theory, practice, and advances. eds. S. A. Zekavat and M. R. Buehrer (Hoboken, Wiley), 25–66.
Sohrabpour, A., Ye, S., Worrell, G. A., Zhang, W., and He, B. (2016). Noninvasive electromagnetic source imaging and granger causality analysis: an electrophysiological connectome (eConnectome) approach. IEEE Trans. Biomed. Eng. 63, 2474–2487. doi: 10.1109/TBME.2016.2616474
Stern, J., Jeanmonod, D., and Sarnthein, J. (2006). Persistent EEG overactivation in the cortical pain matrix of neurogenic pain patients. NeuroImage 31, 721–731. doi: 10.1016/j.neuroimage.2005.12.042
Su, Q., Song, Y., Zhao, R., and Liang, M. (2019). A review on the ongoing quest for a pain signature in the human brain. Brain Sci. Adv. 5, 274–287. doi: 10.26599/BSA.2019.9050024
Sufianov, A., Shapkin, A., Sufianova, G., Elishev, V., Barashin, D., Berdichevskii, V., et al. (2014). Functional and metabolic changes in the brain in neuropathic pain syndrome against the background of chronic epidural electrostimulation of the spinal cord. Bull. Exp. Biol. Med. 157, 462–465. doi: 10.1007/s10517-014-2591-0
Sun, G., Wen, Z., Ok, D., Doan, L., Wang, J., and Chen, Z. S. (2021). Detecting acute pain signals from human EEG. J. Neurosci. Methods 347:108964. doi: 10.1016/j.jneumeth.2020.108964
Taesler, P., and Rose, M. (2016). Prestimulus theta oscillations and connectivity modulate pain perception. J. Neurosci. 36, 5026–5033. doi: 10.1523/JNEUROSCI.3325-15.2016
Tan, L. L., Oswald, M. J., and Kuner, R. (2021). Neurobiology of brain oscillations in acute and chronic pain. Trends Neurosci. 44, 629–642. doi: 10.1016/j.tins.2021.05.003
Teel, E. F., Ocay, D. D., Blain-Moraes, S., and Ferland, C. E. (2022). Accurate classification of pain experiences using wearable electroencephalography in adolescents with and without chronic musculoskeletal pain. Front. Pain. Res. 3:162. doi: 10.3389/fpain.2022.991793
Teixeira, M., Mancini, C., Wicht, C. A., Maestretti, G., Kuntzer, T., Cazzoli, D., et al. (2021). Beta electroencephalographic oscillation is a potential GABAergic biomarker of chronic peripheral neuropathic pain. Front. Neurosci. 15:594536. doi: 10.3389/fnins.2021.594536
Teixeira, P. E., Pacheco-Barrios, K., Uygur-Kucukseymen, E., Machado, R. M., Balbuena-Pareja, A., Giannoni-Luza, S., et al. (2022). Electroencephalography signatures for conditioned pain modulation and pain perception in nonspecific chronic low back pain—an exploratory study. Pain Med. 23, 558–570. doi: 10.1093/pm/pnab293
Telkes, L., Hancu, M., Paniccioli, S., Grey, R., Briotte, M., McCarthy, K., et al. (2020). Differences in EEG patterns between tonic and high frequency spinal cord stimulation in chronic pain patients. Clin. Neurophysiol. 131, 1731–1740. doi: 10.1016/j.clinph.2020.03.040
Thibaut, A., Russo, C., Hurtado-Puerto, A. M., Morales-Quezada, J. L., Deitos, A., Petrozza, J. C., et al. (2017). Effects of transcranial direct current stimulation, transcranial pulsed current stimulation, and their combination on brain oscillations in patients with chronic visceral pain: a pilot crossover randomized controlled study. Front. Neurol. 8:576. doi: 10.3389/fneur.2017.00576
Topaz, L. S., Frid, A., Granovsky, Y., Zubidat, R., Crystal, S., Buxbaum, C., et al. (2022). Electroencephalography functional connectivity—a biomarker for painful polyneuropathy. Eur. J. Neurol. doi: 10.1111/ene.15575
Tracey, I. (2021). Neuroimaging enters the pain biomarker arena. Sci. Transl. Med. 13:eabj7358. doi: 10.1126/scitranslmed.abj7358
Tracey, I., Woolf, C. J., and Andrews, N. A. (2019). Composite pain biomarker signatures for objective assessment and effective treatment. Neuron 101, 783–800. doi: 10.1016/j.neuron.2019.02.019
Tu, Y., Tan, A., Bai, Y., Hung, Y. S., and Zhang, Z. (2016). Decoding subjective intensity of nociceptive pain from pre-stimulus and post-stimulus brain activities. Front. Comput. Neurosci. 10:32. doi: 10.3389/fncom.2016.00032
Urigüen, J. A., and Garcia-Zapirain, B. (2015). EEG artifact removal—state-of-the-art and guidelines. J. Neural Eng. 12:031001. doi: 10.1088/1741-2560/12/3/031001
Uygur-Kucukseymen, E., Castelo-Branco, L., Pacheco-Barrios, K., Luna-Cuadros, M. A., Cardenas-Rojas, A., Giannoni-Luza, S., et al. (2020). Decreased neural inhibitory state in fibromyalgia pain: a cross-sectional study. Neurophysiol. Clin. 50, 279–288. doi: 10.1016/j.neucli.2020.06.002
Vachon-Presseau, E., Centeno, M., Ren, W., Berger, S., Tétreault, P., Ghantous, M., et al. (2016). The emotional brain as a predictor and amplifier of chronic pain. J. Dent. Res. 95, 605–612. doi: 10.1177/0022034516638027
van den Broeke, E. N., Wilder-Smith, O. H., van Goor, H., Vissers, K. C., and van Rijn, C. M. (2013). Patients with persistent pain after breast cancer treatment show enhanced alpha activity in spontaneous EEG. Pain Med. 14, 1893–1899. doi: 10.1111/pme.12216
Van Der Miesen, M. M., Lindquist, M. A., and Wager, T. D. (2019). Neuroimaging-based biomarkers for pain: state of the field and current directions. Pain Rep 4:e751. doi: 10.1097/PR9.0000000000000751
Vanneste, S., Song, J.-J., and De Ridder, D. (2018). Thalamocortical dysrhythmia detected by machine learning. Nat. Commun. 9, 1–13. doi: 10.1038/s41467-018-02820-0
Vierck, C. J., Whitsel, B. L., Favorov, O. V., Brown, A. W., and Tommerdahl, M. (2013). Role of primary somatosensory cortex in the coding of pain. Pain 154, 334–344. doi: 10.1016/j.pain.2012.10.021
Villafaina, S., Collado-Mateo, D., Fuentes-Garcia, J. P., Cano-Plasencia, R., and Gusi, N. (2019). Impact of fibromyalgia on alpha-2 EEG power spectrum in the resting condition: a descriptive correlational study. Biomed. Res. Int. 2019:7851047. doi: 10.1155/2019/7851047
von Hehn, C. A., Baron, R., and Woolf, C. J. (2012). Deconstructing the neuropathic pain phenotype to reveal neural mechanisms. Neuron 73, 638–652. doi: 10.1016/j.neuron.2012.02.008
Vuckovic, A., Gallardo, V. J. F., Jarjees, M., Fraser, M., and Purcell, M. (2018). Prediction of central neuropathic pain in spinal cord injury based on EEG classifier. Clin. Neurophysiol. 129, 1605–1617. doi: 10.1016/j.clinph.2018.04.750
Vuckovic, A., Hasan, M. A., Fraser, M., Conway, B. A., Nasseroleslami, B., and Allan, D. B. (2014). Dynamic oscillatory signatures of central neuropathic pain in spinal cord injury. J. Pain 15, 645–655. doi: 10.1016/j.jpain.2014.02.005
Vuckovic, A., Jarjees, M. S., Hasan, M. A., Purcell, M., and Fraser, M. (2022). “EEG biomarkers of pain and applications of machine learning” in Spinal Cord Injury Pain. eds. C. Sang and C. Hulsebosch (Amsterdam: Elsevier), 199–225.
Wager, T. D., Atlas, L. Y., Lindquist, M. A., Roy, M., Woo, C.-W., and Kross, E. (2013). An fMRI-based neurologic signature of physical pain. N. Engl. J. Med. 368, 1388–1397. doi: 10.1056/NEJMoa1204471
Wang, J., Li, D., Li, X., Liu, F.-Y., Xing, G.-G., Cai, J., et al. (2011). Phase–amplitude coupling between theta and gamma oscillations during nociception in rat electroencephalography. Neurosci. Lett. 499, 84–87. doi: 10.1016/j.neulet.2011.05.037
Wei, M., Liao, Y., Liu, J., Li, L., Huang, G., Huang, J., et al. (2022). EEG beta-band spectral entropy can predict the effect of drug treatment on pain in patients with herpes zoster. J. Clin. Neurophysiol. 39, 166–173. doi: 10.1097/WNP.0000000000000758
Woo, C.-W., Chang, L. J., Lindquist, M. A., and Wager, T. D. (2017). Building better biomarkers: brain models in translational neuroimaging. Nat. Neurosci. 20, 365–377. doi: 10.1038/nn.4478
Wu, W., Chen, Z., Gao, X., Li, Y., Brown, E. N., and Gao, S. (2014). Probabilistic common spatial patterns for multichannel EEG analysis. IEEE Trans. Pattern Anal. Mach. Intell. 37, 639–653. doi: 10.1109/TPAMI.2014.2330598
Xu, X., and Huang, Y. (2020). Objective pain assessment: a key for the management of chronic pain. F1000Res 9:F1000. doi: 10.12688/f1000research.20441.1
Yong, R. J., Mullins, P. M., and Bhattacharyya, N. (2022). Prevalence of chronic pain among adults in the United States. Pain 163, e328–e332. doi: 10.1097/j.pain.0000000000002291
Yüksel, M., Ayaş, Ş., Cabıoğlu, M. T., Yılmaz, D., and Cabıoğlu, C. (2019). Quantitative data for transcutaneous electrical nerve stimulation and acupuncture effectiveness in treatment of fibromyalgia syndrome. Evid. Based Complement. Alternat. Med. 2019:9684649. doi: 10.1155/2019/9684649
Zebhauser, P. T., Hohn, V. D., and Ploner, M. (2023). Resting-state electroencephalography and magnetoencephalography as biomarkers of chronic pain: a systematic review. Pain 164, 1200–1221. doi: 10.1097/j.pain.0000000000002825
Zhang, Q., Manders, T., Tong, A. P., Yang, R., Garg, A., Martinez, E., et al. (2017). Chronic pain induces generalized enhancement of aversion. elife 6:e25302. doi: 10.7554/eLife.25302
Zhou, R., Wang, J., Qi, W., Liu, F.-Y., Yi, M., Guo, H., et al. (2018). Elevated resting state gamma oscillatory activities in electroencephalogram of patients with post-herpetic neuralgia. Front. Neurosci. 12:750. doi: 10.3389/fnins.2018.00750
Zimmer, Z., Fraser, K., Grol-Prokopczyk, H., and Zajacova, A. (2022). A global study of pain prevalence across 52 countries: examining the role of country-level contextual factors. Pain 163, 1740–1750. doi: 10.1097/j.pain.0000000000002557
Zis, P., Liampas, A., Artemiadis, A., Tsalamandris, G., Neophytou, P., Unwin, Z., et al. (2022). EEG recordings as biomarkers of pain perception: where do we stand and where to go? Pain Ther. 11, 369–380. doi: 10.1007/s40122-022-00372-2
Zolezzi, D. M., Alonso-Valerdi, L. M., Naal-Ruiz, N. E., and Ibarra-Zarate, D. (2021). Identification of Neuropathic Pain Severity Based on Linear and Non-Linear EEG Features. Annu Int. Conf. IEEE Eng. Med. Biol. Soc. (EMBC), 169–173. doi: 10.1109/EMBC46164.2021.9630101
Keywords: chronic pain, biomarkers, neurobiomarkers, EEG, machine learning, neurophysiology, biomarker, composite biomarker
Citation: Rockholt MM, Kenefati G, Doan LV, Chen ZS and Wang J (2023) In search of a composite biomarker for chronic pain by way of EEG and machine learning: where do we currently stand? Front. Neurosci. 17:1186418. doi: 10.3389/fnins.2023.1186418
Edited by:
Amelie Haugg, Psychiatric University Hospital Zurich, SwitzerlandReviewed by:
Paul Theo Zebhauser, Technical University of Munich, GermanyLuz Maria Alonso-Valerdi, Monterrey Institute of Technology and Higher Education (ITESM), Mexico
Copyright © 2023 Rockholt, Kenefati, Doan, Chen and Wang. This is an open-access article distributed under the terms of the Creative Commons Attribution License (CC BY). The use, distribution or reproduction in other forums is permitted, provided the original author(s) and the copyright owner(s) are credited and that the original publication in this journal is cited, in accordance with accepted academic practice. No use, distribution or reproduction is permitted which does not comply with these terms.
*Correspondence: Jing Wang, SmluZy5XYW5nMkBueXVsYW5nb25lLm9yZw==