- 1Ministry of Education Key Laboratory of Child Development and Disorders, Chongqing Key Laboratory of Pediatrics, National Clinical Research Center for Child Health and Disorders, China International Science and Technology Cooperation Base of Child Development and Critical Disorders, Department of Radiology, Children’s Hospital of Chongqing Medical University, Chongqing, China
- 2Department of Radiology, Children’s Hospital of Fudan University, Shanghai, China
- 3Huiying Medical Technology Co., Ltd., Beijing, China
- 4Ministry of Education Key Laboratory of Child Development and Disorders, Chongqing Key Laboratory of Pediatrics, National Clinical Research Center for Child Health and Disorders, China International Science and Technology Cooperation Base of Child Development and Critical Disorders, Department of Neurosurgery, Children’s Hospital of Chongqing Medical University, Chongqing, China
Purpose: To construct a machine learning model based on radiomics of multiparametric magnetic resonance imaging (MRI) combined with clinical parameters for predicting Sonic Hedgehog (SHH) and Group 4 (G4) molecular subtypes of pediatric medulloblastoma (MB).
Methods: The preoperative MRI images and clinical data of 95 patients with MB were retrospectively analyzed, including 47 cases of SHH subtype and 48 cases of G4 subtype. Radiomic features were extracted from T1-weighted imaging (T1), contrast-enhanced T1 weighted imaging (T1c), T2-weighted imaging (T2), T2 fluid-attenuated inversion recovery imaging (T2FLAIR), and apparent diffusion coefficient (ADC) maps, using variance thresholding, SelectKBest, and Least Absolute Shrinkage and Selection Operator (LASSO) regression algorithms. The optimal features were filtered using LASSO regression, and a logistic regression (LR) algorithm was used to build a machine learning model. The receiver operator characteristic (ROC) curve was plotted to evaluate the prediction accuracy, and verified by its calibration, decision and nomogram. The Delong test was used to compare the differences between different models.
Results: A total of 17 optimal features, with non-redundancy and high correlation, were selected from 7,045 radiomics features, and used to build an LR model. The model showed a classification accuracy with an under the curve (AUC) of 0.960 (95% CI: 0.871−1.000) in the training cohort and 0.751 (95% CI: 0.587−0.915) in the testing cohort, respectively. The location of the tumor, pathological type, and hydrocephalus status of the two subtypes of patients differed significantly (p < 0.05). When combining radiomics features and clinical parameters to construct the combined prediction model, the AUC improved to 0.965 (95% CI: 0.898−1.000) in the training cohort and 0.849 (95% CI: 0.695−1.000) in the testing cohort, respectively. There was a significant difference in the prediction accuracy, as measured by AUC, between the testing cohorts of the two prediction models, which was confirmed by Delong’s test (p = 0.0144). Decision curves and nomogram further validate that the combined model can achieve net benefits in clinical work.
Conclusion: The combined prediction model, constructed based on radiomics of multiparametric MRI and clinical parameters can potentially provide a non-invasive clinical approach to predict SHH and G4 molecular subtypes of MB preoperatively.
1. Introduction
Medulloblastoma (MB) is one of the most common malignant brain tumors, and accounts for 15−20% of central nervous system tumors in children and 40% of tumors in the posterior cranial fossa (Kumar et al., 2015; Massimino et al., 2016; Northcott et al., 2019). Prior to the emergence of molecular diagnostics, MB was classified histologically into subtypes including classic, extensive nodularity, desmoplastic or nodular, and large cell or anaplastic. However, recent studies have found that histopathological classification does not provide better prediction for the prognosis of patients and guidance of clinical treatment (Louis et al., 2016; Massimino et al., 2016).
With the development of molecular diagnostic techniques, the 2016 World Health Organization (WHO) classification of central nervous system tumors classified MB into four molecular subtypes, including wingless (WNT), sonic hedgehog (SHH, TP53 mutant, or wild type), Group 3 (G3), and Group 4 (G4) (Louis et al., 2016). Different molecular subtypes have different molecular mechanisms, clinical characteristics, and prognosis (Eid and Heabah, 2021; Fang et al., 2022). The WNT-activated type, which accounts for approximately 10% of MB, with a 1:1 male and female incidence ratio, originates in the rhombomere lip and dorsal brainstem of older children, has the best clinical outcomes, and is usually accompanied by an exon 3 activating mutation in CTNNB1 and Chromosome 6 monomers (Ramaswamy et al., 2016; Colafati et al., 2018). The SHH-activated type, which accounts for approximately 30% of MB, originating from cerebellar granule cells, has a moderate prognosis, and the common molecular variants are TP53, PTCH1, SUFU, SMO, and other genes mutations (Colafati et al., 2018; Waszak et al., 2018; Hovestadt et al., 2019). In the non-WNT/SHH-activated type (G3 and G4), which accounts for approximately 25 and 35% of MB, respectively, the main common molecular variants are frequent MYC, MYCN, and, OTX2 amplification. Patients in the G4 group with i17q or chromosome 11 deletion have a better prognosis compared to G3, but the prognosis of G4 is significantly worse compared to SHH or WNT-activated types (Zhao et al., 2016; Archer et al., 2017).
The published methods of molecular classification are invasive, relying mostly on gene expression and methylation analyses. In recent years, rapid advances in radiomics and machine learning techniques have made it possible to preoperatively predict MB molecular subtypes non-invasively. Radiomics is a quantitative analysis method of standard medical imaging that extracts a large number of quantitative features from CT, MRI, and PET images through advanced image analysis tools combined with statistical analysis and is now widely used in various clinical fields, such as increasing precision in diagnosis, predicting prognosis and therapy response (Hassani et al., 2019; Chu et al., 2021; Liang et al., 2021; Zhai et al., 2021; Guiot et al., 2022).
Recently, some studies have used a single MRI sequence or ADC values to construct prediction models for the prediction of MB molecular subtypes (Iv et al., 2019; Gonçalves et al., 2022; Saju et al., 2022). However, the results are still unstable, and there are fewer reports on constructing prediction models based on multiparametric MRI combined with clinical parameters for the prediction of MB molecular subtype. It was found that SHH and G4 were the most common molecular subtypes of MB in children, and the prognosis differed significantly between these two subtypes (Taylor et al., 2012). Total resection of the tumor in patients with G4 type has great importance in improving progression-free survival, especially in the presence of metastatic tumor spread preoperatively (Packer and Vezina, 2008; Thompson et al., 2016, 2018). Therefore, early preoperative prediction of molecular subtypes can help tailor individualized treatment and improve long-term prognosis.
In this study, we retrospectively analyzed MRI images and clinical data of 95 patients with MB to construct a machine learning model based on radiomics of multiparametric MRI combined with clinical parameters for predicting SHH and G4 molecular subtypes of pediatric MB.
2. Materials and methods
2.1. Patient characteristics
The Institutional Review Committees of our hospital approved the study. MRI imaging data and clinical data of patients with MB who were treated and followed up in the Children’s Hospital of Chongqing Medical University and the Children’s Hospital of Fudan University from October 2015 to October 2022 were collected. Inclusion criteria were: (1) availability of sufficient image quality preoperative, including axial T1, T2, T1C, T2FLAIR, and ADC maps; (2) availability of postoperative pathological and molecular subtype; (3) availability of complete clinical follow-up data. Exclusion criteria: molecular subtypes that could not be modeled due to the small number of cases were excluded. Exclusion criteria were: molecular subtypes that have fewer cases failed to build a model. 12 cases of WNT, 17 cases of G3, and 1 case of not otherwise specified (NOS) were excluded. Finally, 47 cases of SHH group and 48 cases of G4 group (90 cases from the Children’s Hospital of Chongqing Medical University and 5 cases from the Children’s Hospital of Fudan University) were enrolled.
2.2. Detection methods of molecular subtypes
The acquisition of molecular subtypes includes transcriptome-related assays and genome-related assays. Transcriptome assay use RNAseq methods to detect the expression levels of genes in the subjects, which can assist in determining the molecular subtype and prognosis of MB through the assessment of the expression levels of genes related to molecular typing. Genomic assay cover common variant types, including point mutations, insertions, deletions, amplifications, and fusions, in 687 genes related to tumors. This includes genetic variants highly related to the molecular subtype of MB molecular typing and other genetic variants related to molecular typing and drug use.
2.3. MRI acquisition
All patients underwent brain MR imaging at 1.5T or 3.0T (Signa EXCITE HD, GE Healthcare, Chicago, IL, United States; Discovery MR750, GE Healthcare, Milwaukee, WI, United States; Achieva, Philips Healthcare, Best, Netherlands), with scanning sequences encompassing axial T1, T2, T2FLAIR, T1c, and DWI (b-value taken as 1,000, with subsequent post-processing for ADC map generation). Details of the parameters for all the sequence acquisition are available in Supplementary Table 1.
2.4. Image uploading and tumor sketching
The steps of radiomics analysis are shown in Figure 1. Five sequence images of each patient were uploaded using the big data artificial intelligence research cloud platform developed by Huiying Medical Technology (Beijing) Co. A physician manually outlined regions of interest (ROI) for each sequence, layer by layer, for each case, using the platform’s built-in tools. An automatic computer-generated 3D volume of interest (VOI) of the lesion was obtained. The tumor boundary was outlined without peritumoral edema and reviewed by an experienced pediatric radiologist with 10 years of expertise in neuroimaging. If the regional variation was more than 5%, the boundary was decided by a senior physician, and neither of the two physicians knew the patient’s information during this procedure.
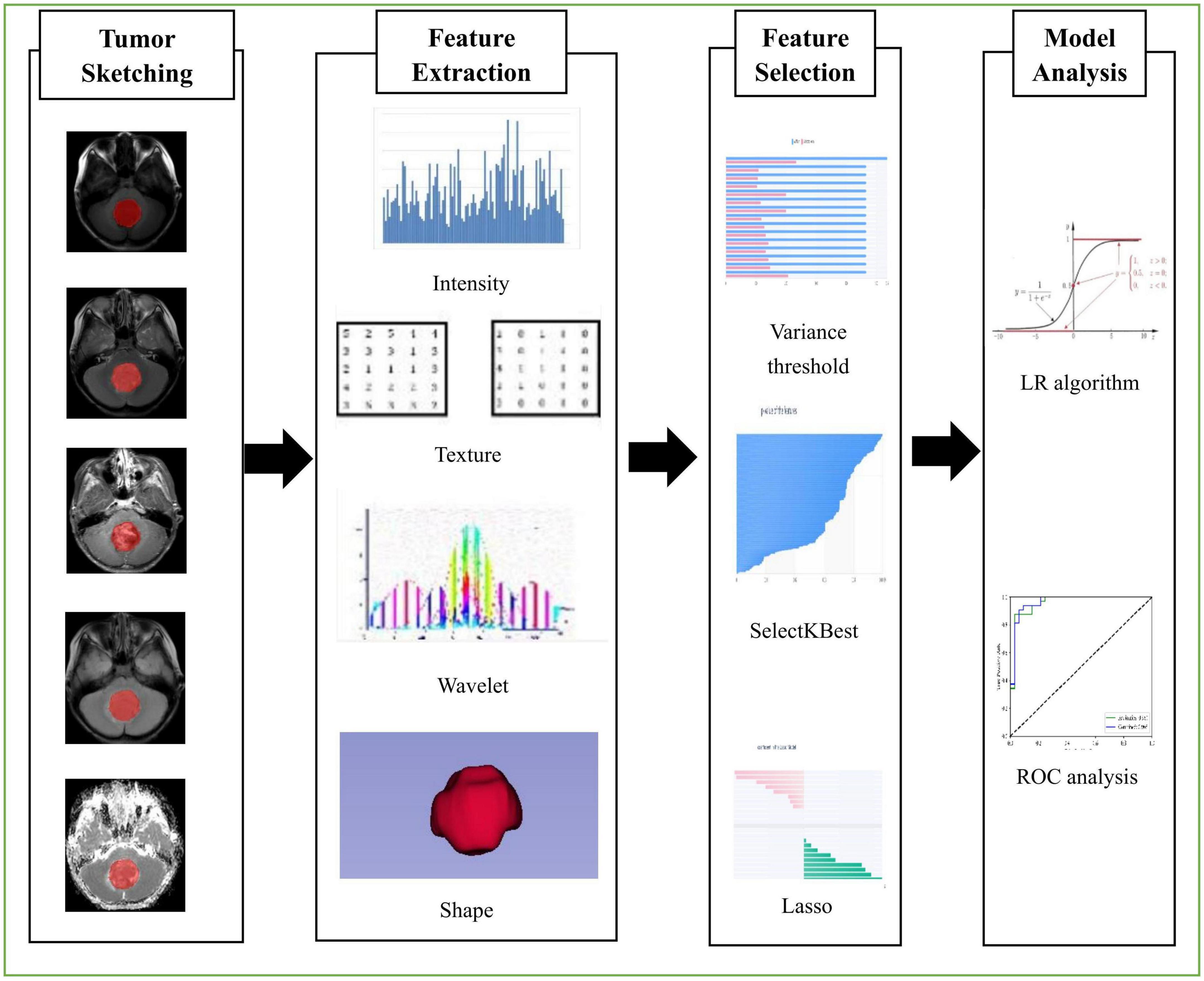
Figure 1. Flowchart shows the process of radiomics in this study, including tumor sketching, feature extraction, feature selection, and model analysis.
2.5. Radiomics feature extraction
A total of 7,045 quantitative imaging radiomics features were extracted from the outlined ROIs using the open source Python language environment toolkit Pyradiomics based on the Huiying Big Data research platform, and these features can be classified into four categories: ➀ Intensity statistics characterization: The distribution of voxel intensities within MR images is described quantitatively by commonly used and basic metrics. ➁ Shape and size features: These features reflect the shape and size of the ROI. ➂ Texture features: Based on the gray level run-length and gray level co-occurrence texture matrix calculation, we get the texture features that can quantify the difference of regional heterogeneity. ➃ High-order statistical features, intensity, and texture features of the transformed image are calculated again using various filters such as exponential, logarithmic, square, square root, and wavelet (including wavelet-LHL, wavelet-LHH, wavelet-HLL, wavelet-LLH, wavelet-HLH, wavelet-HHL, and wavelet-LLL).
2.6. Feature selection
As described above, we extracted quantitative imaging radiomics features from the five-sequence image ROIs of 95 patients. However, it is unlikely that all of these extracted features will be useful for a given task. Therefore, using feature downscaling to filter the specific features that are most relevant to this study for best performance is a necessary step. To reduce redundant features, feature selection methods include variance threshold (threshold value = 0.8), SelectKBest, and the least absolute shrinkage and selection operator (LASSO). For the variance threshold, the threshold value is 0.8, so feature values with variances less than 0.8 are removed. The SelectKBest method is a univariate feature selection method that uses p-values to analyze the relationship between features and classification results, which will allow screening all features with p-values less than 0.05. For LASSO regression, the L1 regularizer is used as the cost function, with a maximum number of iterations of 1,000. Finally, we obtain 17 optimal feature subsets.
2.7. Machine learning classification
Based on the selected feature subsets, the LR algorithm was used to construct the radiomics feature model and the combined model of radiomics features and clinical parameters, respectively, and the patients were divided into training and testing cohorts by the random grouping method in the ratio of 7:3 to obtain the classification prediction results.
2.8. Statistical analysis
Clinical data were statistically analyzed using SPSS 25.0 statistical software. Measurement data that conforms to a normal distribution were presented as x ± s, and the independent samples t-test was used to compare the differences between groups. Categorical data were tested using the χ2 test or Fisher’s exact test, and differences were considered statistically significant at P < 0.05. For the machine learning results, ROC curves were used in the training cohort and testing cohort to compare model prediction accuracy and calculate AUC, sensitivity, and specificity. The Delong test was used to compare the AUC differences between the ROC curves of the two models, and P < 0.05 was considered statistically significant.
3. Results
3.1. Clinical features
A total of 95 patients with MB were enrolled in this study, including 47 cases of SHH and 48 cases of G4. The clinical data such as gender, age, tumor location, clinical symptoms and physical signs, hydrocephalus status, metastasis or recurrence status, blood biochemical indexes, and pathological typing were collected. There were no significant differences in age, gender, renal function, liver function, intracranial hypertension, ataxia and preoperative metastases, recurrence or metastases at follow-up between these two groups (P > 0.05). Pre-dominant pathological typing was desmoplastic or nodular in SHH and classic in G4. The tumor location was mainly in the cerebellum (cerebellar hemisphere or pontine arm) in SHH and in the midline (four ventricles or cerebellar vermis) in G4. Compared with patients in SHH, those in G4 had a higher likelihood of developing hydrocephalus. These differences between groups were statistically significant (P < 0.05), as shown in Table 1.
3.2. Radiomic features
A total of 7,045 quantitative imaging features were extracted from the five sequence images of 95 patients, and 17 optimal feature sets, including 13 texture features and 4 intensity features, were obtained after dimensionality reduction using variance threshold (threshold = 0.8), SelectKBest, and the LASSO regression algorithm. The details were shown in Table 2.
3.3. Model performance
In the radiomics feature model, the AUC was 0.960 (95% CI: 0.871−1.000), and the sensitivity and specificity were 0.880 and 0.850, respectively, in the training cohort. In the testing cohort, the AUC was 0.751 (95% CI: 0.587−0.915), and the sensitivity and specificity were 0.730 and 0.730, respectively. In the radiomics features and clinical parameters combined model, the AUC was 0.965 (95% CI: 0.898−1.000), and the sensitivity and specificity were 0.910 and 0.940, respectively, in the training cohort. In the testing cohort, the AUC was 0.849 (95% CI: 0.695−1.000), and the sensitivity and specificity were 0.800 and 0.730, respectively. The ROC curves for both the training and testing cohorts are shown in Figures 2A, B and Tables 3, 4. The calibration curves show the goodness of fit between the predicted molecular subtypes and actual molecular subtypes in both the training and testing cohort for the radiomics model and the combined model (Figures 3A, B). The decision curves show that the combined model outperforms the radiomics model in terms of net benefit (Figures 4A, B). The clinical utility of both prediction models is demonstrated by the nomogram (Figure 5).
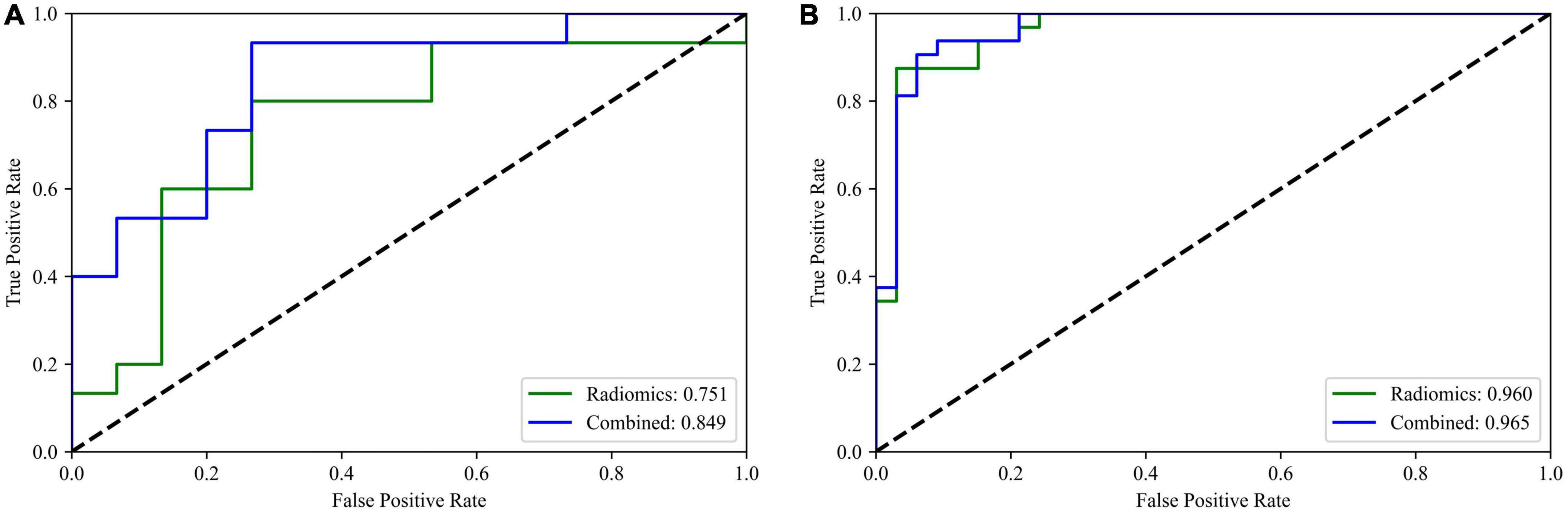
Figure 2. (A,B) ROC curves of the radiomics and combined model on the testing cohort and training cohort, respectively.
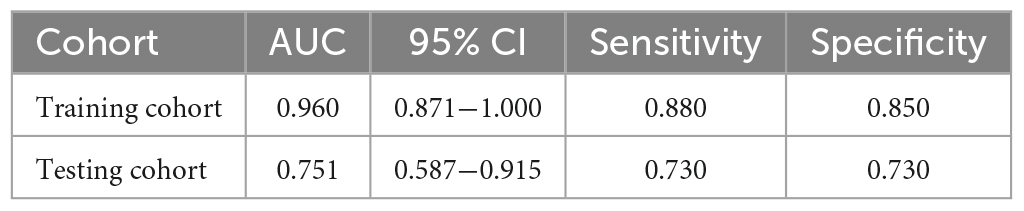
Table 3. AUC, 95% CI, sensitivity, and specificity of radiomics model in training cohort and testing cohort.
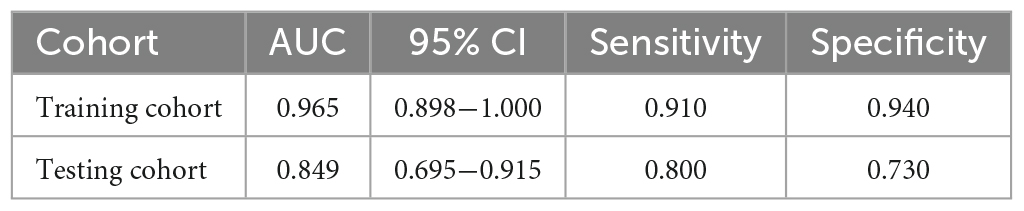
Table 4. AUC, 95% CI, sensitivity and specificity of combined model in training cohort and testing cohort.
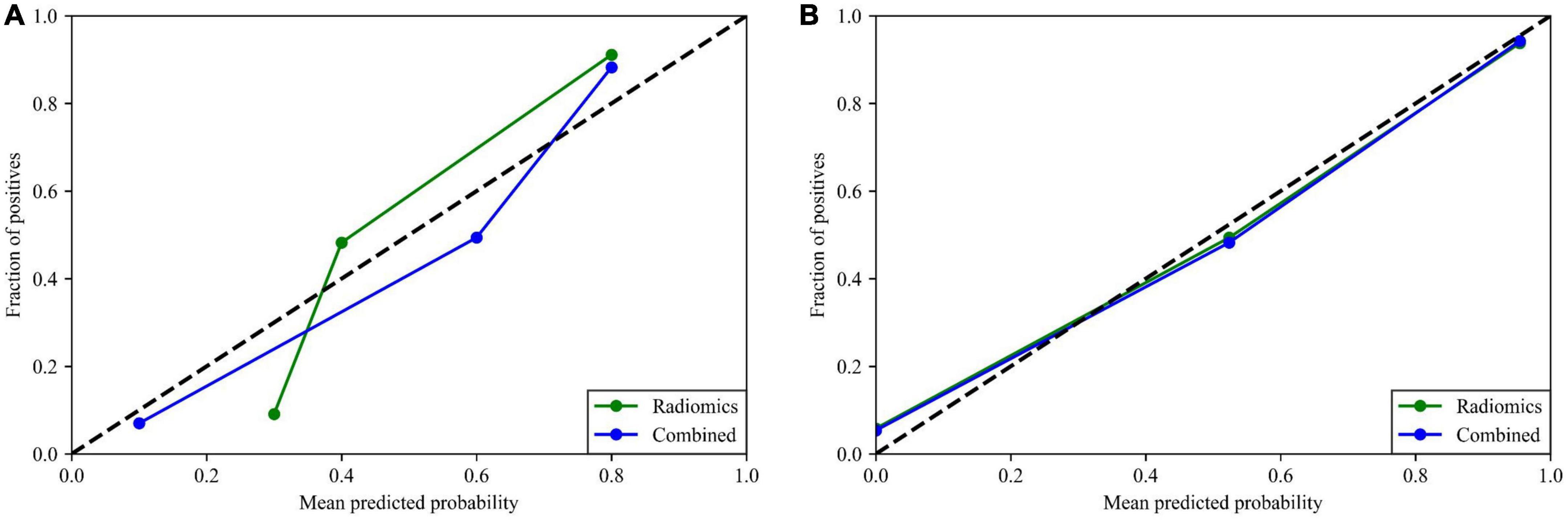
Figure 3. (A,B) Calibration curves of the radiomics and combined model on the testing cohort and training cohort, respectively.
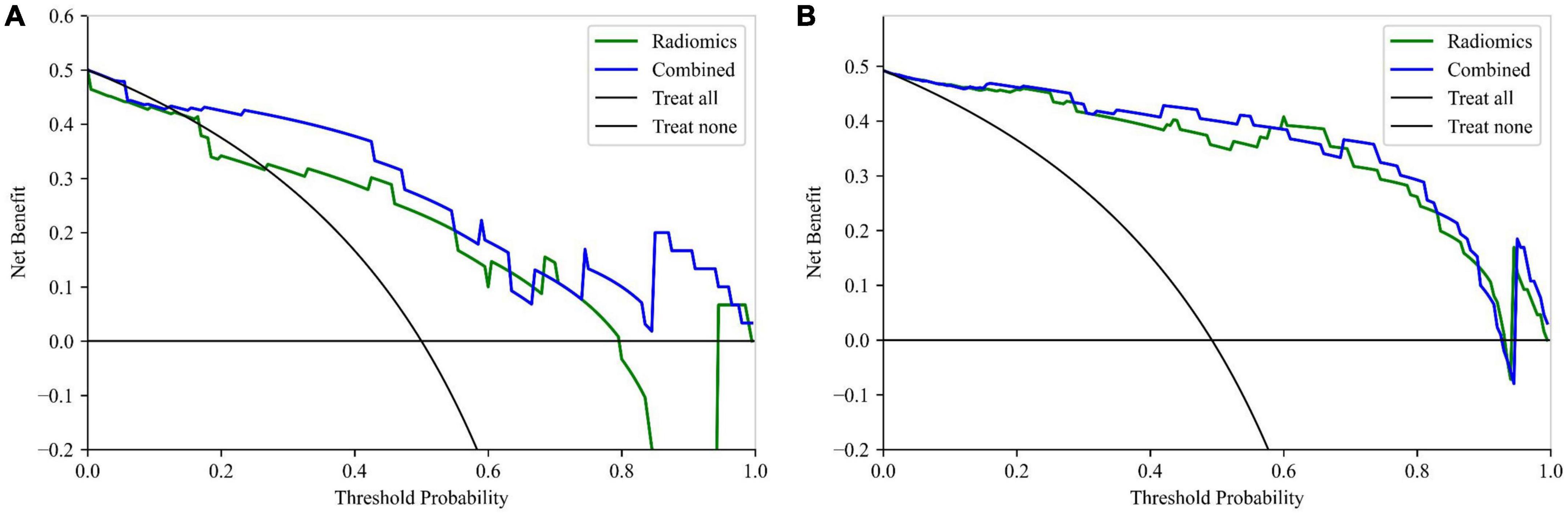
Figure 4. (A,B) Decision curves of the radiomics and combined model on the testing cohort and training cohort, respectively.
Delong test results showed the difference of prediction accuracy measured by AUC in the testing cohort of the two prediction models has statistical significance. The results were shown in Table 5.
4. Discussion
Medulloblastoma is a highly aggressive brain tumor, the therapeutic strategies and clinical prognosis vary significantly among molecular subtypes. The published methods of molecular classification are invasive, relying mostly on gene expression and methylation analyses. Due to the tumor tissue heterogeneity, the biopsy tissue specimens cannot fully capture the whole tumor tissue information. In this study, we extracted radiomics features of the entire tumor region and combined them with clinical parameters to build a prediction model for SHH and G4 molecular subtypes of pediatric MB. The results showed that the combined model had significant classification efficacy with an AUC > 0.8 in the testing cohort, potentially providing a non-invasive clinical approach to preoperatively predict SHH and G4 molecular subtypes of MB.
In this study, we used clinical characteristics, including hydrocephalus status, tumor location, and pathological type, to construct a combined model for predicting SHH and G4 molecular subtypes of MB. In our study, there were no significant differences in age and gender between the two molecular subtypes, which is not consistent with the previous studies reported by Taylor et al. (2012) and Eid and Heabah (2021). We suspect that this may be due to the small sample size of our cohort. Similar to a recent study by Yan et al. (2020) that enrolled 122 patients, our study also showed that the tumor location and hydrocephalus status differed in molecular subtypes and were combined in the model to improve predictive efficacy.
In our study, 17 optimal radiomics features were selected, containing 13 texture features and 4 intensity features. A multicenter study of 263 patients from 12 children’s hospitals reported that texture features and first-order intensity features contributed the most to improving the predictive efficacy of the model (Zhang et al., 2022). In the study by Yan et al. (2020), three texture features and eight intensity features were extracted to construct the model. Also, in the study by Chang et al. (2021), 38 children from Taipei showed significant differences in eight textural features among different molecular subtypes. In a systematic review and meta-analysis on radiomics-based machine learning for predicting molecular subtypes of MB (Karabacak et al., 2022), five articles enrolled 420 patients with MB, and the results showed that the mean AUC of prediction models for all MB molecular subtypes was >0.8, indicating a greater possibility of predicting MB molecular subtypes by radiomics studies. Compared with the above-mentioned studies, our study used comprehensive image features of five sequences including T1, T2, T1C, T2FLAIR, and ADC maps, and combined them with clinical parameters to construct a combined model with improved prediction accuracy. The AUC was 0.965 in the training cohort and 0.849 in the testing cohort, respectively, which can be used to facilitate the prediction of SHH and G4 molecular subtypes of MB preoperatively.
This study had some limitations. First, the number of cases in the SHH and G4 groups enrolled in this study was relatively small, and the WNT and G3 groups were not included in the study because the number of cases did not meet the modeling requirements. Further expansion of the sample size is needed to conduct a multicenter, prospective study. Second, the results of this study lack external validation to better assess the generalizability of the model, which still needs further investigation.
5. Conclusion
In summary, our study demonstrated that a combined prediction model based on the radiomics features of multiparametric MRI and clinical parameters can effectively predict the SHH and G4 molecular subtypes of MB prior to surgery. These findings highlight the potential of radiomics and machine learning techniques for non-invasive preoperative prediction of MB molecular subtypes.
Data availability statement
The original contributions presented in this study are included in the article/Supplementary material, further inquiries can be directed to the corresponding authors.
Ethics statement
Written informed consent was obtained from the individual(s), and minor(s)’ legal guardian/next of kin, for the publication of any potentially identifiable images or data included in this article.
Author contributions
YW wrote the manuscript and which was revised by the YZ and LL. LW, BQ, and YJ contributed to the data processing and statistical analysis. WX, XH, ZT, and SL contributed to the data collection. All authors contributed to the article and approved the submitted version.
Funding
This study was supported by the grants from Chongqing Medical Scientific Research Project (Joint Project of Chongqing Health Commission and Science and Technology Bureau) (No. 2023ZDXM023).
Conflict of interest
YJ was employed by Huiying Medical Technology Co., Ltd.
The remaining authors declare that the research was conducted in the absence of any commercial or financial relationships that could be construed as a potential conflict of interest.
Publisher’s note
All claims expressed in this article are solely those of the authors and do not necessarily represent those of their affiliated organizations, or those of the publisher, the editors and the reviewers. Any product that may be evaluated in this article, or claim that may be made by its manufacturer, is not guaranteed or endorsed by the publisher.
Supplementary material
The Supplementary Material for this article can be found online at: https://www.frontiersin.org/articles/10.3389/fnins.2023.1157858/full#supplementary-material
References
Archer, T., Mahoney, E., and Pomeroy, S. (2017). Medulloblastoma: molecular classification-based personal therapeutics. Neurotherapeutics 14, 265–273. doi: 10.1007/s13311-017-0526-y
Chang, F., Wong, T., Wu, K., Lu, C., Weng, T., Liang, M., et al. (2021). Magnetic resonance radiomics features and prognosticators in different molecular subtypes of pediatric medulloblastoma. PLoS One 16:e0255500. doi: 10.1371/journal.pone.0255500
Chu, H., Liu, Z., Liang, W., Zhou, Q., Zhang, Y., Lei, K., et al. (2021). Radiomics using CT images for preoperative prediction of futile resection in intrahepatic cholangiocarcinoma. Eur. Radiol. 31, 2368–2376. doi: 10.1007/s00330-020-07250-5
Colafati, G., Voicu, I., Carducci, C., Miele, E., Carai, A., Di Loreto, S., et al. (2018). MRI features as a helpful tool to predict the molecular subgroups of medulloblastoma: state of the art. Ther. Adv. Neurol. Disord. 11:1756286418775375. doi: 10.1177/1756286418775375
Eid, A., and Heabah, N. (2021). Medulloblastoma: clinicopathological parameters, risk stratification, and survival analysis of immunohistochemically validated molecular subgroups. J. Egypt. Natl. Canc. Inst. 33:6. doi: 10.1186/s43046-021-00060-w
Fang, F., Rosenblum, J., Ho, W., and Heiss, J. (2022). New developments in the pathogenesis, therapeutic targeting, and treatment of pediatric medulloblastoma. Cancers 14:2285. doi: 10.3390/cancers14092285
Gonçalves, F., Tierradentro-Garcia, L., Kim, J., Zandifar, A., Ghosh, A., Viaene, A., et al. (2022). The role of apparent diffusion coefficient histogram metrics for differentiating pediatric medulloblastoma histological variants and molecular groups. Pediatr. Radiol. 52, 2595–2609. doi: 10.1007/s00247-022-05411-w
Guiot, J., Vaidyanathan, A., Deprez, L., Zerka, F., Danthine, D., Frix, A., et al. (2022). A review in radiomics: making personalized medicine a reality via routine imaging. Med. Res. Rev. 42, 426–440. doi: 10.1002/med.21846
Hassani, C., Varghese, B., Nieva, J., and Duddalwar, V. (2019). Radiomics in pulmonary lesion imaging. Am. J. Roentgenol. 212, 497–504. doi: 10.2214/AJR.18.20623
Hovestadt, V., Smith, K., Bihannic, L., Filbin, M., Shaw, M., Baumgartner, A., et al. (2019). Resolving medulloblastoma cellular architecture by single-cell genomics. Nature 572, 74–79. doi: 10.1038/s41586-019-1434-6
Iv, M., Zhou, M., Shpanskaya, K., Perreault, S., Wang, Z., Tranvinh, E., et al. (2019). MR imaging-based radiomic signatures of distinct molecular subgroups of medulloblastoma. Am. J. Neuroradiol. 40, 154–161. doi: 10.3174/ajnr.A5899
Karabacak, M., Ozkara, B., Ozturk, A., Kaya, B., Cirak, Z., Orak, E., et al. (2022). Radiomics-based machine learning models for prediction of medulloblastoma subgroups: a systematic review and meta-analysis of the diagnostic test performance. Acta Radiol. [Epub ahead of print]. doi: 10.1177/02841851221143496
Kumar, L., Deepa, S., Moinca, I., Suresh, P., and Naidu, K. (2015). Medulloblastoma: a common pediatric tumor: prognostic factors and predictors of outcome. Asian J. Neurosurg. 10:50. doi: 10.4103/1793-5482.151516
Liang, H., Chen, Z., Wei, F., Yang, R., and Zhou, H. (2021). Bibliometrics research on radiomics of lung cancer. Transl. Cancer Res. 10, 3757–3771. doi: 10.21037/tcr-21-1277
Louis, D., Perry, A., Reifenberger, G., von Deimling, A., Figarella-Branger, D., Cavenee, W., et al. (2016). The 2016 World Health Organization classification of tumors of the central nervous system: a summary. Acta Neuropathol. 131, 803–820. doi: 10.1007/s00401-016-1545-1
Massimino, M., Biassoni, V., Gandola, L., Garrè, M., Gatta, G., Giangaspero, F., et al. (2016). Childhood medulloblastoma. Crit. Rev. Oncol. Hematol. 105, 35–51. doi: 10.1016/j.critrevonc.2016.05.012
Northcott, P. A., Robinson, G., Kratz, C., Mabbott, D., Pomeroy, S., Clifford, S., et al. (2019). Medulloblastoma. Nat. Rev. Dis. Primers 5:11. doi: 10.1038/s41572-019-0063-6
Packer, R., and Vezina, G. (2008). Management of and prognosis with medulloblastoma: therapy at a crossroads. Arch. Neurol. 65, 1419–1424. doi: 10.1001/archneur.65.11.1419
Ramaswamy, V., Remke, M., Bouffet, E., Bailey, S., Clifford, S., Doz, F., et al. (2016). Risk stratification of childhood medulloblastoma in the molecular era: the current consensus. Acta Neuropathol. 131, 821–831. doi: 10.1007/s00401-016-1569-6
Saju, A., Chatterjee, A., Sahu, A., Gupta, T., Krishnatry, R., Mokal, S., et al. (2022). Machine-learning approach to predict molecular subgroups of medulloblastoma using multiparametric MRI-based tumor radiomics. Br. J. Radiol. 95:20211359. doi: 10.1259/bjr.20211359
Taylor, M., Northcott, P., Korshunov, A., Remke, M., Cho, Y., Clifford, S., et al. (2012). Molecular subgroups of medulloblastoma: the current consensus. Acta Neuropathol. 123, 465–472. doi: 10.1007/s00401-011-0922-z
Thompson, E., Bramall, A., Herndon, J., Taylor, M., and Ramaswamy, V. (2018). The clinical importance of medulloblastoma extent of resection: a systematic review. J. Neurooncol. 139, 523–539. doi: 10.1007/s11060-018-2906-5
Thompson, E., Hielscher, T., Bouffet, E., Remke, M., Luu, B., Gururangan, S., et al. (2016). Prognostic value of medulloblastoma extent of resection after accounting for molecular subgroup: a retrospective integrated clinical and molecular analysis. Lancet Oncol. 17, 484–495. doi: 10.1016/S1470-2045(15)00581-1
Waszak, S., Northcott, P., Buchhalter, I., Robinson, G., Sutter, C., Groebner, S., et al. (2018). Spectrum and prevalence of genetic predisposition in medulloblastoma: a retrospective genetic study and prospective validation in a clinical trial cohort. Lancet Oncol. 19, 785–798. doi: 10.1016/S1470-2045(18)30242-0
Yan, J., Liu, L., Wang, W., Zhao, Y., Li, K., Li, K., et al. (2020). Radiomic features from multi-parameter MRI combined with clinical parameters predict molecular subgroups in patients with medulloblastoma. Front. Oncol. 10:558162. doi: 10.3389/fonc.2020.558162
Zhai, Y., Song, D., Yang, F., Wang, Y., Jia, X., Wei, S., et al. (2021). Preoperative prediction of meningioma consistency via machine learning-based radiomics. Front. Oncol. 11:657288. doi: 10.3389/fonc.2021.657288
Zhang, M., Wong, S., Wright, J., Wagner, M., Toescu, S., Han, M., et al. (2022). MRI radiogenomics of pediatric medulloblastoma: a multicenter study. Radiology 304, 406–416. doi: 10.1148/radiol.212137
Keywords: medulloblastoma, radiomics, molecular subtypes, machine learning, prediction models
Citation: Wang Y, Wang L, Qin B, Hu X, Xiao W, Tong Z, Li S, Jing Y, Li L and Zhang Y (2023) Preoperative prediction of sonic hedgehog and group 4 molecular subtypes of pediatric medulloblastoma based on radiomics of multiparametric MRI combined with clinical parameters. Front. Neurosci. 17:1157858. doi: 10.3389/fnins.2023.1157858
Received: 03 February 2023; Accepted: 27 March 2023;
Published: 11 April 2023.
Edited by:
Fahmi Khalifa, Morgan State University, United StatesReviewed by:
Angel Mota, MEDS4VETS: Medical Care for Veterans Abroad, Dominican RepublicLiwei Hu, Shanghai Children’s Medical Center, China
Copyright © 2023 Wang, Wang, Qin, Hu, Xiao, Tong, Li, Jing, Li and Zhang. This is an open-access article distributed under the terms of the Creative Commons Attribution License (CC BY). The use, distribution or reproduction in other forums is permitted, provided the original author(s) and the copyright owner(s) are credited and that the original publication in this journal is cited, in accordance with accepted academic practice. No use, distribution or reproduction is permitted which does not comply with these terms.
*Correspondence: Yuting Zhang, zhangyuting@cqmu.edu.cn; Lusheng Li, lilusheng@cqmu.edu.cn
†These authors have contributed equally to this work and share last authorship