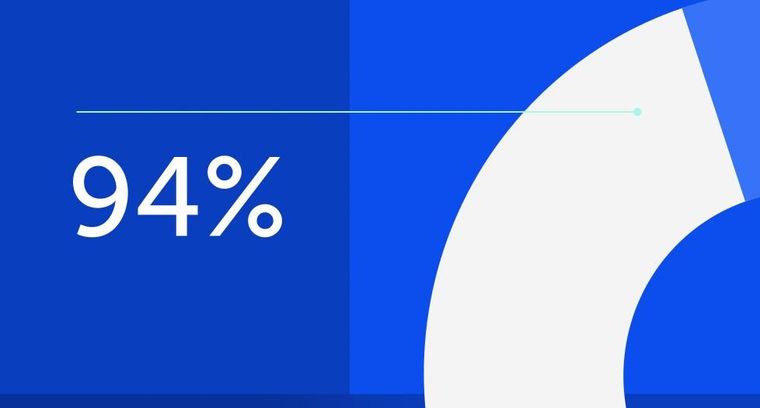
94% of researchers rate our articles as excellent or good
Learn more about the work of our research integrity team to safeguard the quality of each article we publish.
Find out more
EDITORIAL article
Front. Neurosci., 07 February 2023
Sec. Neuromorphic Engineering
Volume 17 - 2023 | https://doi.org/10.3389/fnins.2023.1144906
This article is part of the Research TopicNovel Memristor-Based Devices and Circuits for Neuromorphic and AI ApplicationsView all 6 articles
Editorial on the Research Topic
Novel memristor-based devices and circuits for neuromorphic and AI applications
This Research Topic collects different contributions that highlight various aspects related to the deployment of MR devices for neuromorphic and AI applications. These contributions provide new insights toward utilizing emerging devices and approaches to mimic biological behavior of the brain.
The first article of this Topic (Poduval et al.), proposes a brain-inspired system that displays HDC memorization ability over a graph of relationships. The authors introduce GraphHD, hyper-dimensional memorization that displays graph-based information in high-dimensional space. This approach provides superior cognitive functions over the encoded memory graph, which allows the representation of complex graph-based data structure into high-dimensional space.
The contribution proposed by Fang, Liu et al. focuses on utilizing memristor devices in the leaky integrate-and-fire (LIF) spiking model, and introduces memristive-based LIF (MLIF). The authors prove the superiority of MLIF over LIF via comparing the firing patterns of both models. It's shown that MLIF can efficiently mimic the firing behavior of biological neurons. Experimental results show that a single memristive cell can emulate the synapse and demonstrate the function of integration and filtering of both the dendrite and soma. Thus, this work could provide a basic building block for reproducing the behaviors of biological neurons and building neural networks.
As an alternative approach, a memristive-based Izhikevich (MIZH) spiking neuron model is proposed in Fang, Duan et al.. The reported approach easily mimics the spiking and bursting patterns of distinct brain neurons. The MIZH neural network demonstrates both synchronous and asynchronous collective actions. The firing patterns of excitatory, inhibitory, and other neurons are also studied in this work using the MIZH model. Due to the inherent characteristics of memristor devices, MIZH will have a great impact on the design and implementation of artificial neural network models.
The authors in Fang, Tan et al. propose replacing the resistor with a memristor in the Resistor-Capacitor (RC) neuron circuit, and name the new circuit as Memristor-Capacitor (MC) circuit. It's shown that the charging and discharging time has been reduced using the MC circuit. Thus, due to the key advantages of memristor devices through the non-linear and non-volatile switching, this work proves the potential of utilizing memristor-based circuit to reproduce the behaviors of the biological neurons.
On a different study, the work reported in Garg et al. proposed a novel approach for brain-inspired unsupervised local learning. This study focuses on voltage-dependent-synaptic plasticity (VDSP). The authors show that the new methodology helps in reducing the number of updates compared to the standard spike timing dependent plasticity (STDP). Also, results prove that VDSP can be successfully deployed in pattern recognition applications.
We hope this Research Topic will be a great asset for the reader to enrich the knowledge and understanding of utilizing the unique and promising properties of emerging memristor devices for neuromorphic and AI applications.
The author confirms being the sole contributor of this work and has approved it for publication.
The author declares that the research was conducted in the absence of any commercial or financial relationships that could be construed as a potential conflict of interest.
All claims expressed in this article are solely those of the authors and do not necessarily represent those of their affiliated organizations, or those of the publisher, the editors and the reviewers. Any product that may be evaluated in this article, or claim that may be made by its manufacturer, is not guaranteed or endorsed by the publisher.
Keywords: memristor, switching, spiking, neurons, artificial intelligence
Citation: Abunahla H (2023) Editorial: Novel memristor-based devices and circuits for neuromorphic and AI applications. Front. Neurosci. 17:1144906. doi: 10.3389/fnins.2023.1144906
Received: 15 January 2023; Accepted: 18 January 2023;
Published: 07 February 2023.
Edited and reviewed by: André van Schaik, Western Sydney University, Australia
Copyright © 2023 Abunahla. This is an open-access article distributed under the terms of the Creative Commons Attribution License (CC BY). The use, distribution or reproduction in other forums is permitted, provided the original author(s) and the copyright owner(s) are credited and that the original publication in this journal is cited, in accordance with accepted academic practice. No use, distribution or reproduction is permitted which does not comply with these terms.
*Correspondence: Heba Abunahla, aGViYS5uYWRobWlAZ21haWwuY29t
Disclaimer: All claims expressed in this article are solely those of the authors and do not necessarily represent those of their affiliated organizations, or those of the publisher, the editors and the reviewers. Any product that may be evaluated in this article or claim that may be made by its manufacturer is not guaranteed or endorsed by the publisher.
Research integrity at Frontiers
Learn more about the work of our research integrity team to safeguard the quality of each article we publish.