- 1School of Psychology, Zhejiang Normal University, Jinhua, China
- 2Key Laboratory of Intelligent Education Technology and Application of Zhejiang Province, Zhejiang Normal University, Jinhua, China
- 3Department of Radiology, Changshu No.2 People’s Hospital, The Affiliated Changshu Hospital of Xuzhou Medical University, Changshu, Jiangsu, China
- 4School of Western Languages, Heilongjiang University, Harbin, China
Migraine—a disabling neurological disorder, imposes a tremendous burden on societies. To reduce the economic and health toll of the disease, insight into its pathophysiological mechanism is key to improving treatment and prevention. Resting-state functional magnetic resonance imaging (rs-fMRI) studies suggest abnormal functional connectivity (FC) within the default mode network (DMN) in migraine patients. This implies that DMN connectivity change may represent a biomarker for migraine. However, the FC abnormalities appear inconsistent which hinders our understanding of the potential neuropathology. Therefore, we performed a meta-analysis of the FC within the DMN in migraine patients in the resting state to identify the common FC abnormalities. With efficient search and selection strategies, nine studies (published before July, 2022) were retrieved, containing 204 migraine patients and 199 healthy subjects. We meta-analyzed the data using the Anisotropic Effect Size version of Signed Differential Mapping (AES-SDM) method. Compared with healthy subjects, migraine patients showed increased connectivity in the right calcarine gyrus, left inferior occipital gyrus, left postcentral gyrus, right cerebellum, right parahippocampal gyrus, and right posterior cingulate gyrus, while decreased connectivity in the right postcentral gyrus, left superior frontal gyrus, right superior occipital gyrus, right orbital inferior frontal gyrus, left middle occipital gyrus, left middle frontal gyrus and left inferior frontal gyrus. These results provide a new perspective for the study of the pathophysiology of migraine and facilitate a more targeted treatment of migraine in the future.
1. Introduction
Migraine is the commonest primary headache in clinical practice. This disabling neurological disorder is characterized by recurrent unilateral or bilateral pulsating headaches (Robbins, 2021). Epidemiological surveys showed that 1.1 billion people suffer from migraine worldwide which constitutes the second leading cause of disability globally (Ashina et al., 2021b; Safiri et al., 2022). Unfortunately, the surveys also illustrated an upward trend in the incidence of migraine attacks (Global Burden of Disease Study 2013 Collaborators, 2015; Burch et al., 2019). Given that the disabling effect of the disease foists colossal socio-economic and health costs on its sufferers (Vo et al., 2018), scholars found the lack of progress in diagnosis and treatment over the years lamentable (Katsarava and Steiner, 2012; Katsarava et al., 2018; Ashina et al., 2021a). We contend that the stagnation in scientific breakthroughs in this area is mainly due to the lack of insight into the disease’s pathophysiology.
Resting-state functional magnetic resonance imaging (rs-fMRI)—a non-invasive method, has been widely used in brain functional research (Yu et al., 2020; Bi et al., 2021). This method has contributed to the understanding of the underlying physiological mechanism of migraine greatly (Colombo et al., 2015; Russo et al., 2017; Ellingson et al., 2019; Kim et al., 2021). Previous rs-fMRI studies demonstrated the close relationship between both the default mode network (DMN) and its associated brain regions and migraine (Jia and Yu, 2017; Chong et al., 2019; Argaman et al., 2020). The DMN is a highly active brain network when the brain is at rest and is associated with an individual’s stressful experiences and his/her coping strategies that promote adaptation to the (stressful) environment (McEwen and Gianaros, 2011; Soares et al., 2013).
A growing body of evidences have revealed the role of non-adaptive responses in migraine mechanisms (Borsook et al., 2012; Maleki et al., 2012). These suggested that recurrent migraine attacks alter the functional connectivity (FC) of the DMN and that these changes may disrupt individual stress response mechanisms (Ellerbrock et al., 2013). Extant studies showed that abnormal FC of DMN has been found in patients with different pain disorders, suggesting that pain has a broad impact on the DMN (Baliki et al., 2008; Napadow et al., 2010). In addition, DMN was involved in the pain inhibition process and affected the efficiency of pain processing (Baliki et al., 2008; Nahman-Averbuch et al., 2014; Youssef et al., 2016). The above studies indicate the critical role DMN plays in the neuropathological mechanisms of migraine. So, a fine understanding of DMN may facilitate workable treatment options.
The identification of spatial patterns of DMN in migraine patients can be based on FC. That is because the FC is capable of exploring the connectivity between brain regions and depicting the complex functional coupling patterns between various brain regions (Biswal et al., 1995), and has been applied in exploring FC abnormalities in patients (Bi et al., 2018). Currently, many scholars have used seed-based FC analysis (Androulakis et al., 2017; Cao et al., 2022) and independent component analysis (Giugni et al., 2010; Xue et al., 2012; Colon et al., 2019) to explore FC abnormalities within the DMN of migraine patients. However, the inconsistent results of the resting-state FC (rs-FC) within the DMN in migraine patients must not be ignored. Some studies identified decreased rs-FC of the DMN in migraine patients compared to healthy subjects. Decreased functional connectivity was found in the right cerebellum and left frontal lobe in Amin et al. (2016) study, Tessitore et al. (2013) found decreased connectivity in the prefrontal and temporal regions of the DMN, Zou et al. (2021) found reduced connectivity in the left precuneus of the DMN while others detected increased rs-FC in the DMN, Chou et al. (2021) observed increased DMN FC in the left precentral gyrus, left postcentral gyrus and right cerebellum, Zhang et al. (2016) only found increased FC in the left precuneus and posterior cingulate cortex within the DMN. Those discrepant findings may be due to small sample size, patients’ clinical heterogeneity, and the different sample selections (Skorobogatykh et al., 2019). Fortunately, meta-analysis can synthesize neuroimaging findings to reduce the discrepant results (Kaiser et al., 2015). Specifically, the method can aggregate and integrate a large amount of data from studies and distinguish positive results in the process of replication (Müller et al., 2018). With the increasing number of published studies about FC in the DMN during the resting state of migraine patients, conducting a meta-analysis to identify common functional abnormalities in the brain is urgently needed to further deepen our understanding of migraine’s pathophysiological mechanisms.
Accordingly, we performed a meta-analysis to assess common alterations in the connectivity of DMN in migraine patients by using the Anisotropic Effect Size version of Signed Differential Mapping (AES-SDM (Radua et al., 2014). Our findings could help harmonize the contradictory results found in the literature and advance the understanding of migraine.
2. Methods
2.1. Search strategy and literature screening
Methods and analyses of the current study were pre-registered on the PROSPERO (ID: CRD42022348891).1 This study was conducted under the Preferred Reporting Items for Rigorous Systematic Reviews and Meta-Analyses (PRISMA) guidelines (Liberati et al., 2009). We searched comprehensively in PubMed, Web of Science, and Embase databases for literature up to July 2022, using the combined keywords: (“migraine”) AND (“resting state” OR “rest”) AND (“DMN” OR “default mode network”). Further, we searched the reference list of the qualified literature compiled by our manual search from related reviews and references. For studies that did not provide detailed information in the paper (e.g., peak effect coordinates), we contacted the authors by email to obtain relevant information.
For literature selection, we used the following inclusion criteria: (i) original rs-fMRI study written in English; (Giugni et al., 2010) patients included in the study meet the diagnostic criteria for migraine; (iii) used seed-based or ICA methods to conduct rs-fMRI data analysis; (iv) direct comparison of rs-FC between migraine patients and healthy subjects; (v) peak coordinates of between-group effects were reported in a standard space, such as Talairach or Montreal Neurological Institute (MNI). Studies were excluded if (i) it is a book chapter or review article; (Giugni et al., 2010) the coordinates of significant statistical differences in rs-FC between groups could not be retrieved even by contacting the author; (iii) the sample in the study overlapped with another published study; and (iv) the seeds were not selected in DMN. According to the above inclusion and exclusion criteria, eligible articles were screened and included in this meta-analysis. The literature search and screening were conducted by two investigators independently (S.H and JJ.W) and the relevant studies were double-checked by the two investigators (S.H and JJ.W). Inconsistent studies were discussed and resolved via consensus.
2.2. Quality assessment and data extraction
To ensure high data quality, we assessed each included study using a 20-point checklist (Iwabuchi et al., 2015; Pan et al., 2017a), which was adapted from previous rs-fMRI meta-analyses. The checklist mainly contains an assessment of the quality of subjects (e.g., demographic and clinical characteristics) and the study methodology (e.g., image procedure, method description), the details of the checklist are presented in Supplementary Table S1. After quality assessment, important information from each included study was extracted and collated, mainly including the first author, migraine type, sample size, sex ratio, age distribution, disease duration, disease frequency analytical method, seed region, scanner type, and statistical threshold. Furthermore, the current meta-analysis was based on peak coordinates showing the regions of significant differences in rs-FC between-group, therefore, the peak coordinates and their effect values (e.g., T-values or Z-scores) of each research were also obtained and sorted into a corresponding text file.
2.3. Main meta-analysis
An anisotropic effect-size version of seed-based d mapping (AES-SDM) software package version 5.15 for Windows2 was used in the current meta-analysis. SDM is a powerful statistical technique that uses peak coordinates to perform meta-analyses to assess differences in brain activity and has been widely used in recent neuroimaging meta-analyses (Radua et al., 2012; Lim et al., 2020; Yao et al., 2021). Following the guidance of the AES-SDM tutorial, this meta-analysis was completed in three parts. In the first step, we retrieved the peak coordinates and their corresponding effect size from the results reported by each study. It is worth noting that all Z-scores were transformed into T-value using the SDM online converter. If the effect size was not specified in the study, we will use “p” for hyper-connectivity and “n” for hypo-connectivity to represent the effect size of these peak coordinates. The peak coordinates and effect values were then saved into a text file. After the data preparation, we preprocessed the datasets and reconstructed a mean effect-size map of differences between groups for each study using an anisotropic unnormalized Gaussian kernel (Radua et al., 2014). In the last step, individual maps from each study were combined using meta-analytic calculations, which were weighted by intra-study variance and inter-study heterogeneity. We then calculated the mean of the study maps with a random-effects model (Radua and Mataix-Cols, 2012; Pan et al., 2017a). Here we adopted the recommended SDM parameters in the main meta-analysis, setting full-width at half-maximum (FWHM) to 20 mm, uncorrected voxel p = 0.005, anisotropy = 1, peak height SDM-Z > 1, and cluster extent ≥10 voxels (Radua et al., 2012; Pan et al., 2017c).
2.4. Subgroup analysis
In the current study, four types of subgroup analyses were performed. (1) subgroup analyses of migraineurs (MIG) and migraineurs without aura (MowA) to investigate the effect of disease type on FC abnormalities; (2) The five ICA studies were selected as a subgroup and the four studies based on seed-based FC as an additional subgroup to explore the alteration of FC in both methods. (3) To explore the effect of age, a subgroup analysis was performed on adults (eight studies). (4) To control for medication status, we conducted subgroup analyses of whether medication was taken.
2.5. Analyses of sensitivity, heterogeneity, and publication bias
A systematic Jackknife sensitivity analysis was conducted to test the robustness and reliability of the main results. In short, Jackknife sensitivity analysis consists of repeated analyses, discarding one study at a time, for assessing the reproduction of the results (Radua et al., 2012). In this analysis, we used the “leave-one-out cross-validation” (LOOCV) method to verify the repeatability of the results (Radua and Mataix-Cols, 2009). To be specific, the main analysis was repeated n times (n = the number of total studies), while discarding a different study each time. If the significant brain areas obtained after each LOOCV analysis were present in all or most of the previous significant brain regions, this result is then considered stable and reproducible (Duko et al., 2020). Similarly, we applied the default SDM kernel size and thresholds to obtain the heterogeneous brain regions (Radua et al., 2014), identifying brain areas that were not explained by differences between studies by using random effects models and Q test, H test, I-square statistics (Iwabuchi et al., 2015; Ueno et al., 2016). Heterogeneity in the present study was evaluated by converting Q-statistics to Z-scores. Clusters showing significant heterogeneity and overlapped with the primary outcome were considered to be between-study heterogeneity (Viechtbauer, 2005; Albajes-Eizagirre and Radua, 2018). The heterogeneous brain regions obtained cannot be interpreted simply from the cause of the disease; it may result from clinical differences or demographic information of the patients, so the interpretation of the heterogeneous brain regions needs to be done with caution. Specifically, the results were thresholded using FWHM = 20 mm, uncorrected p = 0.005, peak height Z > 1, and cluster extent ≥10 voxels (Pan et al., 2017c; Zhen et al., 2018). In addition, funnel plots and Egger’s test in SDM were conducted to calculate the probability of publication bias. Publication bias is the tendency for statistically significant findings (positive results) to be reported and published more frequently than non-significant findings (negative results) or invalid findings (Egger et al., 1997). And the Egger’s test was conducted by extracting values from the peak coordinates of abnormal brain regions in the main meta-analysis results (Zhen et al., 2018). Specifically, we first created a mask that using the peak coordinate from the main meta-analysis results, and then extracted value within mask. A result of p < 0.05 in Egger’s test was considered to reflect significant publication bias (Pan et al., 2017b; Zhen et al., 2018).
2.6. Meta-regression analysis
The meta-regression analysis was conducted to examine the potential effects of the relevant variables on the FC alteration, in which the main variables involved were mainly age, disease duration, and frequency of attack. For the reduction of spurious relations, the significance level was set at p < 0.0005 as well as an extent threshold of 100 voxels (Radua and Mataix-Cols, 2009).
3. Results
3.1. Included studies and sample characteristics
Following strict inclusion and exclusion criteria, we found nine studies that met our requirements and consisted of 204 migraine patients and 199 healthy individuals. The flow chart for the search and selection strategies are shown in Figure 1. One of the studies didn’t report the sex ratio and another did not report age distribution. In the nine included studies, patients in three studies with medication status. In Hubbard et al. (2014) study, the majority of migraine took prophylactic medication, whereas over a third of migraine took abortive medications in Lo Buono et al. (2017) study, patients took simple analgesics, simple triptans, and combination analgesics during a migraine attack; in Messina et al. (2020) study, patients were taking flunarizine and ginkgolide for migraine prevention at the time of MRI. All the studies were acquired using a 3.0 T MRI scanner. There are five studies for ICA analysis and four studies for seed-based rs-FC analysis. The detailed sample characteristics and methodological details of included studies are summarized in Table 1.
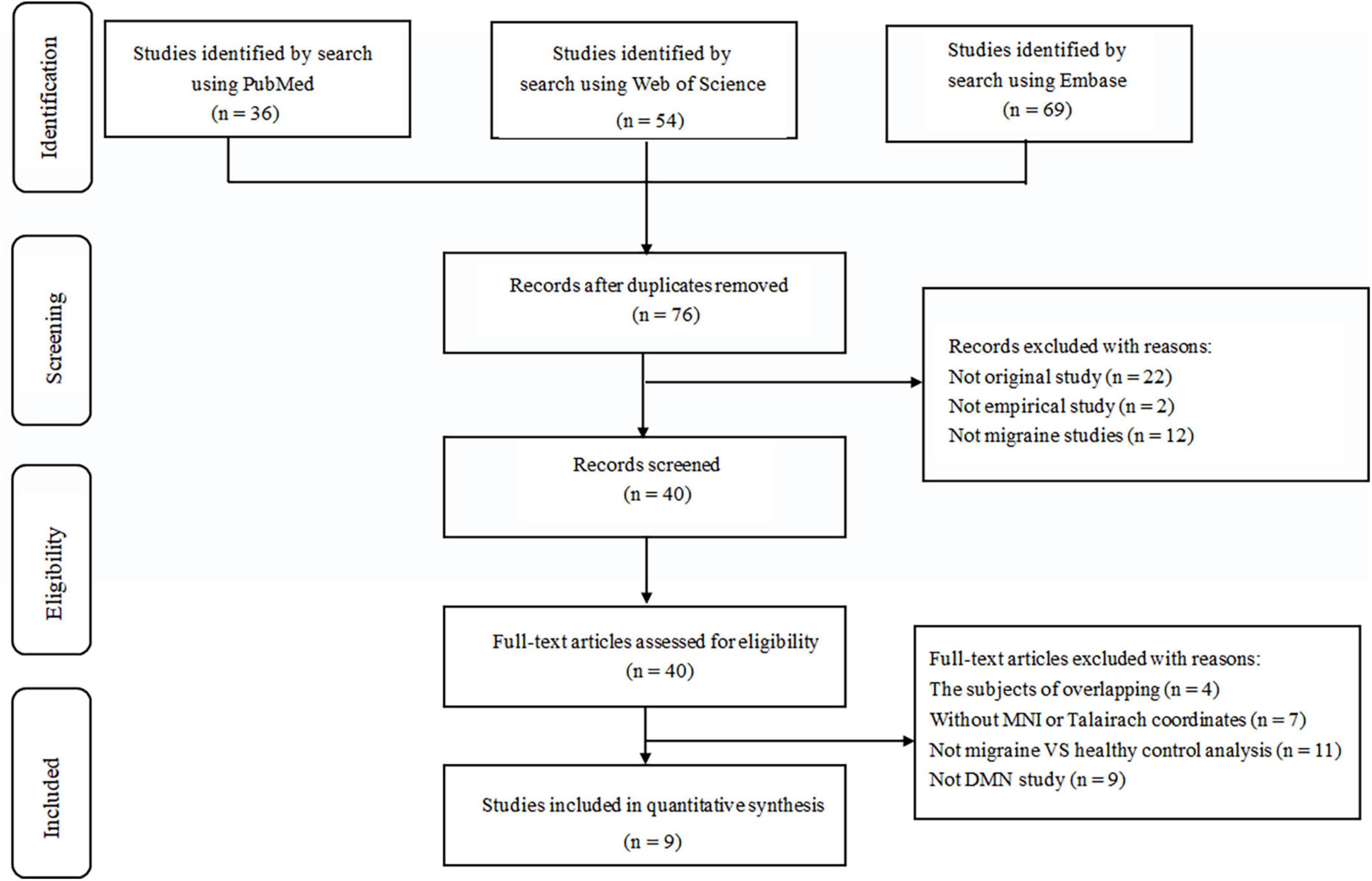
Figure 1. Flow diagram for the identification and exclusion of studies. MNI, Montreal Neurological Institute; DMN, default mode network.
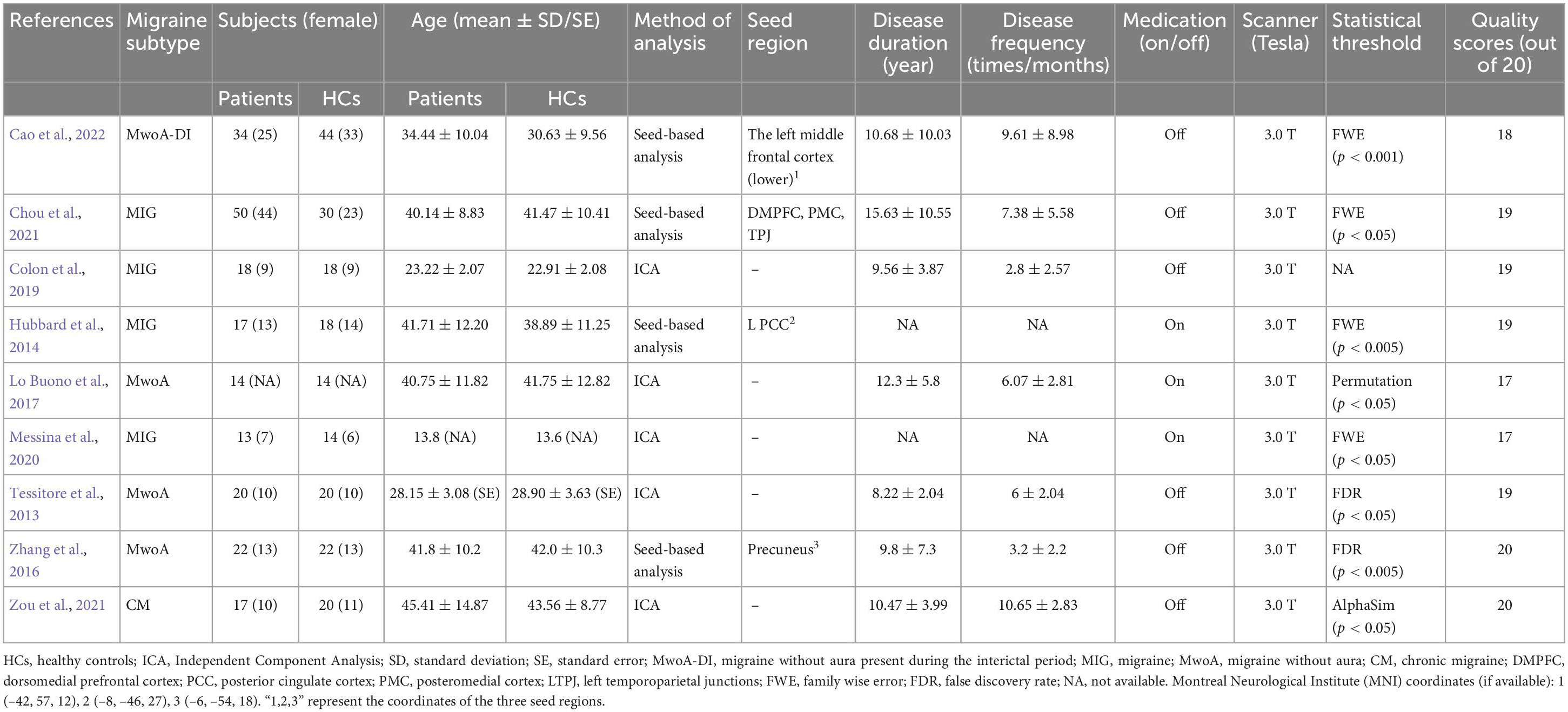
Table 1. Characteristics of resting-state functional magnetic resonance imaging (rs-fMRI) studies included in the meta-analysis.
3.2. Main meta-analysis of rs-FC between migraine and HCs
Compared with the HCs, we found migraine patients to show significantly increased rs-FC, including right calcarine gyrus (Calcarine_R), left inferior occipital gyrus (Occipital_Inf_L), left postcentral gyrus (Postcentral_L), right cerebellum (lobule IV/V, Cerebelum_4_5_R), right parahippocampal gyrus (ParaHippocampal_R), and right posterior cingulate gyrus (Cingulum_Post_R). Meanwhile, decreased rs-FC were discovered in the right postcentral gyrus (Postcentral_R), left superior frontal gyrus (Frontal_Sup_L), right superior occipital gyrus (Occipital_Sup_R), right orbital inferior frontal gyrus (Frontal_Inf_Orb_R), left middle occipital gyrus (Occipital_Mid_L), left middle frontal gyrus (Frontal_Mid_L), and left inferior frontal gyrus triangular part (Frontal_Inf_Tri_L). The detailed results from the meta-analysis are summarized in Figure 2, Table 2.
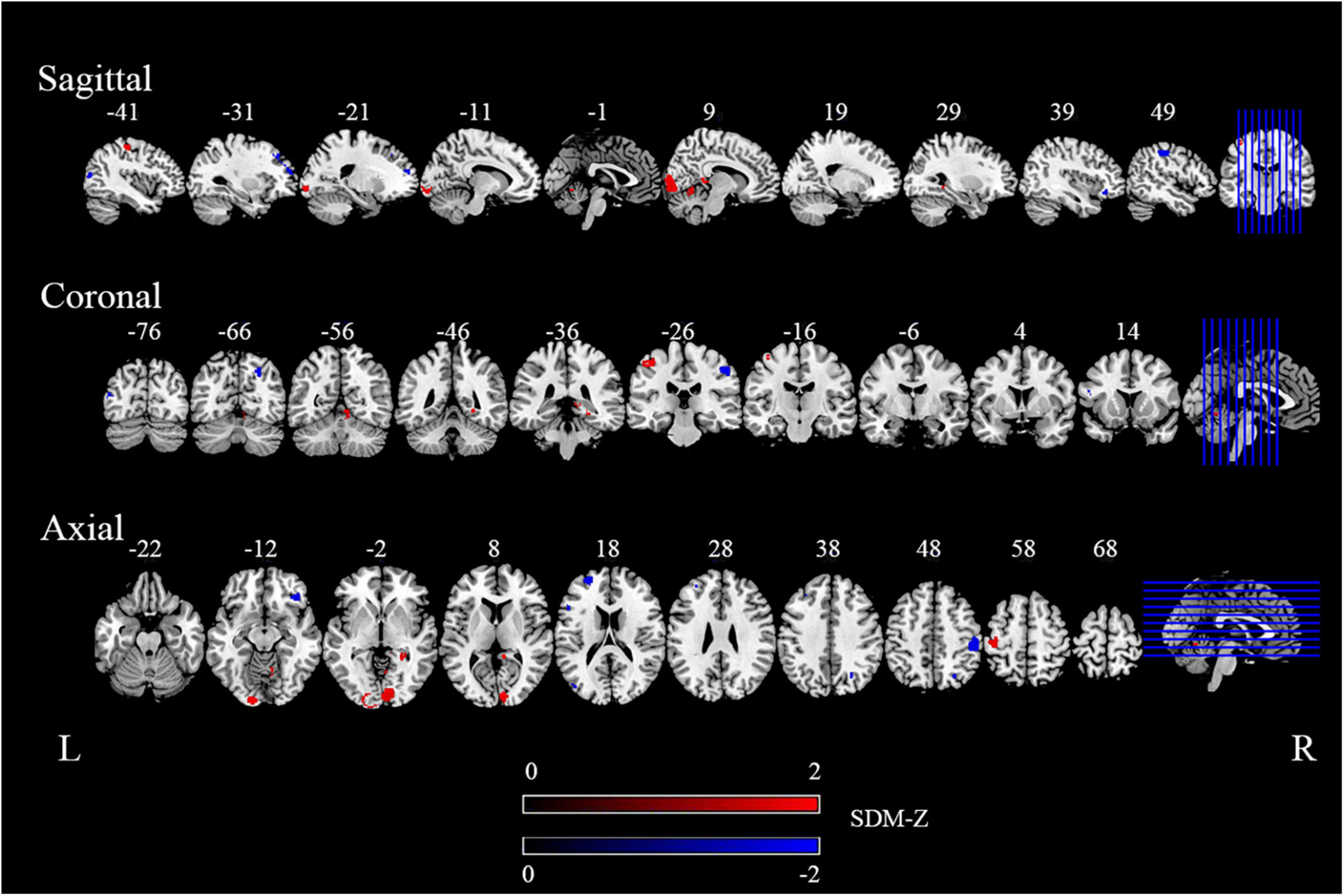
Figure 2. The areas of increased (red) and decreased (blue) functional connectivity (FC) in the main meta-analysis. “R” and “L” denote the right and left sides of the brain, respectively. The color bar indicates the maximum and minimum seed-based d mapping (SDM)-Z value.
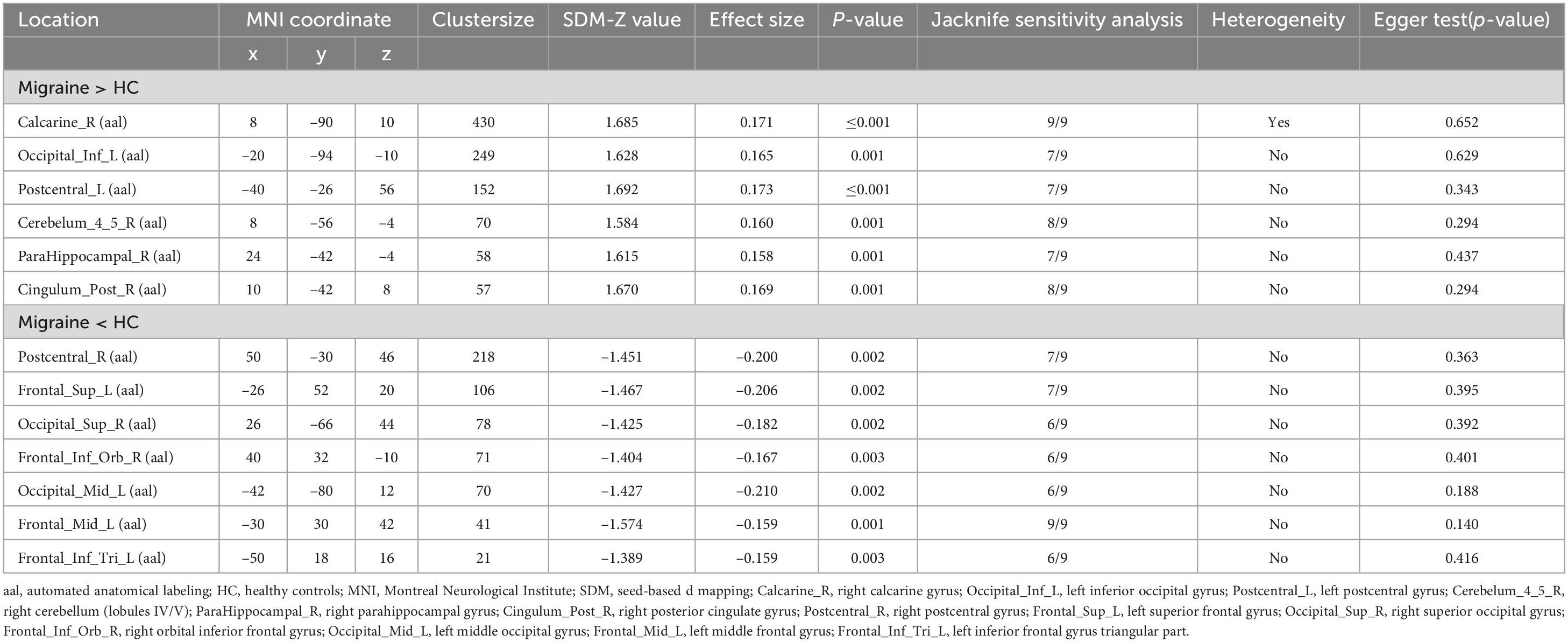
Table 2. Clusters showing resting-state functional connectivity (rs-FC) differences in migraine patients compared with healthy control (HC).
3.3. Subgroup meta-analysis
Subgroup analyses of ICA studies suggested that decreased FC was still found in the Occipital_Mid_L, Frontal_Sup_L and we also found decreased FC in the Calcarine_R in patients compared with healthy subjects. While the increased FC showed some subtle difference. Increased FC was found in the right cuneus gyrus (Cuneus_R) and the left temporal pole of superior temporal gyrus (Temporal_Pole_Sup_L) (Supplementary Table S2 and Supplementary Figure S1). Subgroup analysis of seed-based FC revealed that decreased FC was still found in the Occipital_Sup_R, the Frontal_Mid_L, and the Frontal_Inf_Orb_L. At the same time, we also found increased FC in cerebellum, such as right Crus I and II of cerebellar hemisphere gyrus. Besides, we found decreased FC in the right supramarginal gyrus (SupraMarginal_R), the inferior frontal gyrus of orbital part (Frontal_Inf_Orb) while increased FC was found in the right gyrus rectus (Rectus_R), right lingual gyrus (Lingual_R) and lobule of vermis (Supplementary Table S3 and Supplementary Figure S2). For the migraine without aura (MwoA), increased FC was revealed in left lingual gyrus (Lingual_L), the left superior temporal gyrus (Temporal_Pole_Sup_L), right calcarine gyrus (Calcarine_R), and left superior frontal gyrus (Frontal_Sup_L), while decreased FC was found right median cingulate and paracingulate gyri (Cingulum_Mid_R), Frontal_Mid_L, and right precuneus (Precuneus_R) (Supplementary Table S2 and Supplementary Figure S1); About migraine (MIG) group, we also found increased FC in the Postcentral_L, decreased FC in Frontal_Inf_Tri_L and Frontal_Sup_L, which is consistent with the main results. Besides, we found some different brain region in MIG group, the left temporal pole of superior temporal gyrus (Temporal_Pole_Sup_L), the left precentral gyrus (Precentral_L), the left superior parietal gyrus (Parietal_Sup_L), and the left precuneus gyrus (Precuneus_L) was increase in FC compared with healthy subjects, while the left inferior parietal (Parietal_Inf_L) and the left precuneus was decrease in FC relative to healthy subjects (Supplementary Table S5 and Supplementary Figure S4). For the adult subgroup meta-analysis, the great majority of the results were consistent with the main meta-analysis results. However, the increased FC was also found in the Precuneus_R and Lingual_R, the decreased FC was also showed in the right supramarginal gyrus (SurpraMarginal_R) and the left inferior frontal gyrus of opercular part (Frontal_Inf_Oper_L) in adult group (Supplementary Table S6 and Supplementary Figure S5). For the no-medication subgroup analysis, decreased FC in the Frontal_Mid_L and Frontal_Sup_L was also found, while increased FC was showed in the precentral gyrus (Precentral_L) and left middle temporal gyrus (Temporal_Mid_L) in migraine relative to healthy subjects. About subgroup analyses of medication status, Clusters still showing increase FC in the calcarine gyrus, decreased in Postcentral_R, Occipital_Sup_R, Frontal_Inf_Orb_R in migraine patients compared with healthy subjects (Supplementary Tables S7, S8 and Supplementary Figures S6, S7).
3.4. Sensitivity test for the main findings
Jackknife sensitivity analysis revealed that rs-FC results remained largely consistent. After nine repeated meta-analyses using the “leave-one-out cross-validation” (LOOCV), as shown in Table 2, the Calcarine_R and Frontal_Mid_L were replicated in all nine meta-analyses. The right cerebellum and Cingulum_Post_R were stably preserved in 8/9 of the datasets. The results in the Occipital_Inf_L, Postcentral_L, ParaHippocampal_R, Postcentral_R, and Frontal_Sup_L remained significant in seven meta-analyses. Other brain regions remained significant in six meta-analyses. The heterogeneity analysis revealed significant between-study variability of FC changes in the right calcarine gyrus; this may have arisen due to the clinical differences between studies (Sterne et al., 2011). In addition, none of the brain regions showed significant publication bias based on Egger’s test (p > 0.05). And we provided funnel plots of the meta-analysis to visualize the possibility of publication bias in Supplementary Figures S8–S11.
3.5. Meta-regression analysis
Age, the duration of the disease and the frequency of attacks of patients in included studies were collated by us in current study. In the meta-regression analysis, no significant correlation was found between the mean age of the patients (available in all studies), the duration of the disease [available in all studies but two (Hubbard et al., 2014; Messina et al., 2020)] or attack frequency [available in all studies but two (Hubbard et al., 2014; Messina et al., 2020)] and the abnormality of FC.
4. Discussion
This meta-analysis was the first study to systematically examine the rs-FC within DMN in migraine patients. The current study comprehensively reviewed nine studies, using a quantitative SDM meta-analysis to reveal abnormalities associated with DMN in migraine. We found that compared with healthy subjects, migraine patients showed abnormal connectivity of rs-FC in key nodes of DMN, such as ParaHippocampal_R, Frontal_Mid_L, Cingulum_Post_R. In addition to this, there were abnormalities of rs-FC presented in other cortical areas, like the occipital lobe, cerebellum, and some region of the injury perception pathway. The present study highlighted the crucial role of the DMN in migraine pathology, and provides a reliable reference for further understanding of its pathogenesis.
In specific terms, we found reduced FC in the Frontal_Mid_L, Frontal_Sup_L, and Frontal_Inf_Tri_L, Frontal_Mid_L as part of the prefrontal cortex, was engaged in the cognitive assessment and regulation of pain (Katja et al., 2008). In a prior functional brain pathway study, FC of the middle frontal gyrus and the dorsal anterior cingulate cortex were reduced in migraine patients without aura compared to healthy subjects (Russo et al., 2012). This reduced connectivity of the middle frontal gyrus was negatively correlated with the pain intensity of migraine attack (Russo et al., 2012). Brain structure in migraine patients revealed that the gray matter volume and the folding coefficient of the middle frontal gyrus were reduced in migraine patients without aura (Jia and Yu, 2017). The superior frontal gyrus was involved in emotional responses and the participative feelings of pain (Bluhm et al., 2007). The left inferior frontal gyrus played a role in cognitive modulation of pain as well as memory retrieval and emotional pain regulation (Ochsner et al., 2012). Several studies supported linking migraine physiopathology with the left inferior frontal gyrus (Arkink et al., 2012; Zhang et al., 2020). For example, aberrant cerebral perfusion changes in the left inferior frontal gyrus in migraineurs have been found (Arkink et al., 2012). In our subgroup analysis, we also found abnormalities of FC in other brain regions (inferior frontal gyrus of orbital part and opercular part) of the frontal lobe. These brain regions of rs-FC abnormalities were associated with migraine and other pain disorders in the past studies (Xu et al., 2022; Yang et al., 2022), indirectly suggesting that the frontal cortex plays an important role in pain perception and regulation, and to a certain extent, it is possibly involved in the development of migraine.
In the current study, the right calcarine gyrus (Calcarine_R), superior occipital gyrus (Occipital_Sup_R), left middle occipital gyrus (Occipital_Mid_L), and inferior occipital gyrus (Occipital_Inf_L), which are part of the occipital lobe, showed abnormal FC in migraine patients. Abnormalities in the occipital lobe were found to closely relate to migraine (Schoenen et al., 2003; Vincent and Hadjikhani, 2007a). For example, the theory of cortical spreading of depression is an important hypothesis of migraine and is widely considered to be the pathological mechanism of migraine with aura, and emphasizes the importance of abnormalities in the occipital lobe in migraine (Hadjikhani et al., 2001; Tfelt-Hansen, 2010). Besides, the occipital lobe is now generally considered to be hyperexcitable in migraine (Welch, 2005). Such excitability leads to a lower threshold for migraine attacks, and that cortical diffusion inhibition arises when certain stimuli are present, leading to migraine attacks. In other words, when patients suffer migraine attacks, the body’s self-protective mechanisms may reduce the frequency and the degree of pain of migraine attacks by decreasing the excitability of the occipital cortex. Consistent with these studies, we also found abnormal FC in brain regions associated with the occipital cortex. This reflected the idea that the occipital lobe related to visual function may be an important pathogenesis of migraine. Besides, we also found abnormal FC in the cuneus and lingual gyrus in some subgroup analyses, these regions are the major regions of the visual network in previous studies (Boulloche et al., 2010; Lee et al., 2019). Established studies illustrated the hypermetabolism of the lingual gyrus and the cuneus, which is involved in the perceptual abnormalities associated with visual, such as photophobia (Denuelle et al., 2011; Schankin et al., 2014). It is necessary to note that Calcarine_R is heterogeneous in the results, which may be due to clinical variations in patients between studies (Sterne et al., 2011). Hence this abnormality in FC of this brain region needs to be treated with caution and suggests that we can pay further attention to abnormal variations in Calcarine_R in migraine patients in the future.
Our meta-analysis also revealed enhanced rs-FC in the right posterior cingulate gyrus (Cingulum_Post_R) and the right parahippocampal gyrus (ParaHippocampal_R) in migraine patients. Migraine patients often suffer from pain. While the pain processing is complex and it may be regulated by brain regions of DMN, such as the cingulate gyrus and ParaHippocampal_R (Tomasi and Volkow, 2011). For example, the ParaHippocampal_R participates in the abnormal processing of allodynia (Iadarola et al., 1998). Metabolic abnormalities in the posterior cingulate gyrus have been suggested in previous studies in migraine patients without aura (Kim et al., 2010), and a significant negative correlation between the gray matter density of the posterior cingulate gyrus bilaterally and pain sensitivity was also noted (Emerson et al., 2014). The present results imply that these regions may be involved in pain modulation for migraine patients.
Migraine patients also showed FC abnormalities on the postcentral gyrus in the main results and abnormalities on the precentral gyrus in the subgroup analyses compared to healthy subjects. The postcentral gyrus precentral gyrus is not only a key brain region in the central brain network of pain modulation responsible for information processing of painful stimuli (Apkarian et al., 2005; Schweizer et al., 2008; Tracey, 2008; Torta et al., 2017), it has also been suggested to be involved in the trigeminal-thalamo-cortical injury perception pathway, which is related to the pathophysiology of migraine (Goadsby et al., 2017). For example, migraine patients demonstrated significantly decreased FC between the right postcentral gyrus and right substantia nigra, and the altered FC was negatively associated with migraine duration (Huang et al., 2019). Meanwhile, the reduced low-frequency amplitude of the postcentral gyrus in patients was captured in the neural activity during the migraine interval (Wang et al., 2016) and cortical thinning within the postcentral gyrus in migraine patients (Mehnert et al., 2017). Consistent with the results of these studies, the present study also found a decrease in FC of postcentral gyrus in migraine patients. Additionally, MRI studies showed that the postcentral gyrus is activated by painful stimulation (Carlsson et al., 2006; Wager et al., 2013). Increase in FC also has been found in the left postcentral gyrus in this study, this may be due to subtle differences in brain function between the left and right hemispheres. Therefore, it is necessary to explore the abnormalities of the left and right postcentral gyrus in migraine patients in the future. Abnormalities of the postcentral gyrus in patients may be associated with disruptions in networks related to pain modulation. This further suggests that the postcentral gyrus in the “central brain network of pain modulation” may be characteristically altered in migraine patients.
Enhanced FC was found in the right cerebellum (lobule IV/V) in this study, which is consistent with the results of previous studies. The cerebellum is primarily responsible for nociceptive avoidance behavior. For instance, when there is an increased spontaneous cerebellar activity, the cerebellum has stronger resistance to injury (Pereira et al., 2017). Some studies have found that the cerebellum plays a regulatory role in pain processing and pain perception (Ruscheweyh et al., 2014; Mehnert et al., 2017). Established studies provided increasing evidence that the cerebellum is associated with migraine. For example, studies have found abnormal cerebellar function in migraine patients, mainly in terms of cerebellar hyperactivation and abnormal spontaneous cerebellar activity when stimulated by negative emotional images (Wang et al., 2016, 2017). In terms of structure, it has also been found that the gray matter volume of cerebellar regions is smaller in patients with chronic migraine compared to healthy subjects (Bilgiç et al., 2016; Lai et al., 2016). The present results further support the potential association of the cerebellum with the pathogenesis of migraine (Vincent and Hadjikhani, 2007b).
In additional, influenced by different methods, disease subtypes and medication use, we also found abnormalities of FC in other brain regions. The temporal lobe (temporal pole of superior temporal gyrus, middle temporal gyrus and inferior temporal gyrus) is significantly affected by migraine in our analyses, which is consistent with the results of previous studies (Afridi et al., 2005). And the temporal lobe is an associative multisensory area that also processes visual and auditory information have been confirmed (Moulton et al., 2011). The precuneus is a core region of the DMN involved in migraine in our subgroup analyses. Abnormalities in the precuneus have been found to potentially affect information transmission, multimodal integration, and pain sensitivity and processing in patients with MwoA (Zhang et al., 2016; Li et al., 2022). Abnormalities in FC of the supramarginal gyrus have also been reported frequently in subgroup analyses. The supramarginal gyrus is particularly engaged in the cognitive assessment of pain (Lamm et al., 2011; Moulton et al., 2012), and reduced pain-related activity in the suprachiasmatic gyrus has been reported in patients with overmedicated headaches (Ferraro et al., 2012). The results of these subgroup analyses suggested that FC abnormalities between patients with different clinical information could be studied in detail to more precisely understand the pathogenesis of migraine.
Several limitations need to be considered in this study. Firstly, the nine studies included in our meta-analysis differ in age and gender of the subjects, and migraine patients of different genders and age stages may exhibit different DMN abnormalities. We recommend that future studies systematically analyze data from the same age level or the same gender population. Secondly, the AES-SDM approach used in the present study is based on the reported coordinates of previous studies rather than the raw imaging data, future studies could conduct meta-analyses based on the brain maps to add more detailed information. Finally, despite our efforts to obtain information, the number of included studies was relatively small (i.e., nine studies), further research in this field is necessary to confirm and extend these findings.
5. Conclusion
In the current study, we included all available publications using an rs-FC analysis within DMN and performed a meta-analysis to determine the consistency and robustness of altered FC within the DMN in migraine patients. Migraine patients exhibited abnormal FC within DMN as well as some other regions associated with the central brain network of pain modulation. The present study can be considered an exploratory study that provides the primary evidence for the study of functional abnormalities in the DMN in migraine patients. The findings of this study improved our understanding of the pathophysiological mechanisms of migraine from a systemic perspective.
Author contributions
SH, XJ, and JR contributed to the conception of the study. ML, MZ, JW, YG, and QW performed the experiment and analysis preparation. SH and ZH wrote the first manuscript. HX and CA helped coordinate the study and reviewed the manuscript. All authors read and approved the final manuscript.
Funding
This project was supported by the Open Research Fund of College of Teacher Education, Zhejiang Normal University (No. jykf22011), and National Natural Science Foundation of China (No. 82001898).
Acknowledgments
We are grateful to all authors in the research involved in the meta-analysis for using their data and coordinates.
Conflict of interest
The authors declare that the research was conducted in the absence of any commercial or financial relationships that could be construed as a potential conflict of interest.
Publisher’s note
All claims expressed in this article are solely those of the authors and do not necessarily represent those of their affiliated organizations, or those of the publisher, the editors and the reviewers. Any product that may be evaluated in this article, or claim that may be made by its manufacturer, is not guaranteed or endorsed by the publisher.
Supplementary material
The Supplementary Material for this article can be found online at: https://www.frontiersin.org/articles/10.3389/fnins.2023.1136790/full#supplementary-material
Footnotes
References
Afridi, S. K., Giffin, N. J., Kaube, H., Friston, K. J., Ward, N. S., Frackowiak, R. S., et al. (2005). A positron emission tomographic study in spontaneous migraine. Arch. Neurol. 62, 1270–1275. doi: 10.1001/archneur.62.8.1270
Albajes-Eizagirre, A., and Radua, J. (2018). What do results from coordinate-based meta-analyses tell us? Neuroimage 176, 550–553. doi: 10.1016/j.neuroimage.2018.04.065
Amin, F. M., Hougaard, A., Magon, S., Asghar, M. S., Ahmad, N. N., Rostrup, E., et al. (2016). Change in brain network connectivity during PACAP38-induced migraine attacks: a resting-state functional MRI study. Neurology 86, 180–187. doi: 10.1212/WNL.0000000000002261
Androulakis, X. M., Krebs, K., Peterlin, B. L., Zhang, T., Maleki, N., Sen, S., et al. (2017). Modulation of intrinsic resting-state fMRI networks in women with chronic migraine. Neurology 89, 163–169. doi: 10.1212/WNL.0000000000004089
Apkarian, A. V., Bushnell, M. C., Treede, R. D., and Zubieta, J. K. (2005). Human brain mechanisms of pain perception and regulation in health and disease. Eur. J. Pain 9, 463–484. doi: 10.1016/j.ejpain.2004.11.001
Argaman, Y., Kisler, L. B., Granovsky, Y., Coghill, R. C., Sprecher, E., Manor, D., et al. (2020). The endogenous analgesia signature in the resting brain of healthy adults and migraineurs. J. Pain 21, 905–918. doi: 10.1016/j.jpain.2019.12.006
Arkink, E. B., Bleeker, E. J., Schmitz, N., Schoonman, G. G., Wu, O., Ferrari, M. D., et al. (2012). Cerebral perfusion changes in migraineurs: a voxelwise comparison of interictal dynamic susceptibility contrast MRI measurements. Cephalalgia 32, 279–288. doi: 10.1177/0333102411435985
Ashina, M., Buse, D. C., Ashina, H., Pozo-Rosich, P., Peres, M. F. P., Lee, M. J., et al. (2021a). Migraine: integrated approaches to clinical management and emerging treatments. Lancet 397, 1505–1518. doi: 10.1016/S0140-6736(20)32342-4
Ashina, M., Katsarava, Z., Do, T. P., Buse, D. C., Pozo-Rosich, P., Özge, A., et al. (2021b). Migraine: epidemiology and systems of care. Lancet 397, 1485–1495. doi: 10.1016/S0140-6736(20)32160-7
Baliki, M. N., Geha, P. Y., Apkarian, A. V., and Chialvo, D. R. (2008). Beyond feeling: chronic pain hurts the brain, disrupting the default-mode network dynamics. J. Neurosci. 28, 1398–1403. doi: 10.1523/JNEUROSCI.4123-07.2008
Bi, X. A., Hu, X., Xie, Y., and Wu, H. (2021). A novel CERNNE approach for predicting Parkinson’s disease-associated genes and brain regions based on multimodal imaging genetics data. Med. Image Anal. 67:101830. doi: 10.1016/j.media.2020.101830
Bi, X. A., Zhao, J., Xu, Q., Sun, Q., and Wang, Z. (2018). Abnormal functional connectivity of resting state network detection based on linear ICA analysis in autism spectrum disorder. Front. Physiol. 9:475. doi: 10.3389/fphys.2018.00475
Bilgiç, B., Kocaman, G., Arslan, A. B., Noyan, H., Sherifov, R., Alkan, A., et al. (2016). Volumetric differences suggest involvement of cerebellum and brainstem in chronic migraine. Cephalalgia 36, 301–308. doi: 10.1177/0333102415588328
Biswal, B., Yetkin, F. Z., Haughton, V. M., and Hyde, J. S. (1995). Functional connectivity in the motor cortex of resting human brain using echo-planar MRI. Magn. Reson. Med. 34, 537–541. doi: 10.1002/mrm.1910340409
Bluhm, R. L., Miller, J., Lanius, R. A., Osuch, E. A., Boksman, K., Neufeld, R. W., et al. (2007). Spontaneous low-frequency fluctuations in the BOLD signal in schizophrenic patients: anomalies in the default network. Schizophr. Bull. 33, 1004–1012. doi: 10.1093/schbul/sbm052
Borsook, D., Maleki, N., Becerra, L., and McEwen, B. (2012). Understanding migraine through the lens of maladaptive stress responses: a model disease of allostatic load. Neuron 73, 219–234. doi: 10.1016/j.neuron.2012.01.001
Boulloche, N., Denuelle, M., Payoux, P., Fabre, N., Trotter, Y., and Géraud, G. (2010). Photophobia in migraine: an interictal PET study of cortical hyperexcitability and its modulation by pain. J. Neurol. Neurosurg. Psychiatry 81, 978–984. doi: 10.1136/jnnp.2009.190223
Burch, R. C., Buse, D. C., and Lipton, R. B. (2019). Migraine: epidemiology. burden, and comorbidity. Neurol. Clin. 37, 631–649. doi: 10.1016/j.ncl.2019.06.001
Cao, Z., Yu, W., Zhang, Z., Xu, M., Lin, J., Zhang, L., et al. (2022). Decreased gray matter volume in the frontal cortex of migraine patients with associated functional connectivity alterations: a VBM and rs-FC study. Pain Res. Manag. 2022:2115956. doi: 10.1155/2022/2115956
Carlsson, K., Andersson, J., Petrovic, P., Petersson, K. M., Ohman, A., and Ingvar, M. (2006). Predictability modulates the affective and sensory-discriminative neural processing of pain. Neuroimage 32, 1804–1814. doi: 10.1016/j.neuroimage.2006.05.027
Chong, C. D., Schwedt, T. J., and Hougaard, A. (2019). Brain functional connectivity in headache disorders: a narrative review of MRI investigations. J. Cereb. Blood Flow Metab. 39, 650–669. doi: 10.1177/0271678X17740794
Chou, K. H., Kuo, C. Y., Liang, C. S., Lee, P. L., Tsai, C. K., Tsai, C. L., et al. (2021). Shared patterns of brain functional connectivity for the comorbidity between migraine and insomnia. Biomedicines 9:1420. doi: 10.3390/biomedicines9101420
Colombo, B., Rocca, M. A., Messina, R., Guerrieri, S., and Filippi, M. (2015). Resting-state fMRI functional connectivity: a new perspective to evaluate pain modulation in migraine? Neurol. Sci. 36(Suppl. 1), 41–45. doi: 10.1007/s10072-015-2145-x
Colon, E., Ludwick, A., Wilcox, S. L., Youssef, A. M., Danehy, A., Fair, D. A., et al. (2019). Migraine in the young brain: adolescents vs. young adults. Front. Hum. Neurosci. 13:87. doi: 10.3389/fnhum.2019.00087
Denuelle, M., Boulloche, N., Payoux, P., Fabre, N., Trotter, Y., and Géraud, G. (2011). A PET study of photophobia during spontaneous migraine attacks. Neurology 76, 213–218. doi: 10.1212/WNL.0b013e3182074a57
Duko, B., Ayano, G., Pereira, G., Betts, K., and Alati, R. (2020). Prenatal tobacco use and the risk of mood disorders in offspring: a systematic review and meta-analysis. Soc. Psychiatry Psychiatr. Epidemiol. 55, 1549–1562. doi: 10.1007/s00127-020-01949-y
Egger, M., Davey Smith, G., Schneider, M., and Minder, C. (1997). Bias in meta-analysis detected by a simple, graphical test. BMJ 315, 629–634. doi: 10.1136/bmj.315.7109.629
Ellerbrock, I., Engel, A. K., and May, A. (2013). Microstructural and network abnormalities in headache. Curr. Opin. Neurol. 26, 353–359. doi: 10.1097/WCO.0b013e3283633714
Ellingson, B. M., Hesterman, C., Johnston, M., Dudeck, N. R., Charles, A. C., and Villablanca, J. P. (2019). Advanced Imaging in the evaluation of migraine headaches. Neuroimaging Clin. N. A. 29, 301–324. doi: 10.1016/j.nic.2019.01.009
Emerson, N. M., Zeidan, F., Lobanov, O. V., Hadsel, M. S., Martucci, K. T., Quevedo, A. S. D., et al. (2014). Pain sensitivity is inversely related to regional grey matter density in the brain. Pain 155, 566–573. doi: 10.1016/j.pain.2013.12.004
Ferraro, S., Grazzi, L., Mandelli, M. L., Aquino, D., Di Fiore, D., Usai, S., et al. (2012). Pain processing in medication overuse headache: a functional magnetic resonance imaging (fMRI) study. Pain Med. 13, 255–262. doi: 10.1111/j.1526-4637.2011.01183.x
Giugni, E., Vadalà, R., De Vincentiis, C., Colica, C., and Bastianello, S. (2010). The brain’s default mode network: a mind “sentinel” role? Funct. Neurol. 25, 189–190.
Global Burden of Disease Study 2013 Collaborators (2015). Global, regional, and national incidence, prevalence, and years lived with disability for 301 acute and chronic diseases and injuries in 188 countries, 1990-2013: a systematic analysis for the Global Burden of Disease Study 2013. Lancet 386, 743–800.
Goadsby, P. J., Holland, P. R., Martins-Oliveira, M., Hoffmann, J., Schankin, C., and Akerman, S. (2017). Pathophysiology of migraine: a disorder of sensory processing. Physiol. Rev. 97, 553–622. doi: 10.1152/physrev.00034.2015
Hadjikhani, N., Sanchez Del Rio, M., Wu, O., Schwartz, D., Bakker, D., et al. (2001). Mechanisms of migraine aura revealed by functional MRI in human visual cortex. Proc. Natl. Acad. Sci. U S A. 98, 4687–4692. doi: 10.1073/pnas.071582498
Huang, X., Zhang, D., Chen, Y., Wang, P., Mao, C., Miao, Z., et al. (2019). Altered functional connectivity of the red nucleus and substantia nigra in migraine without aura. J. Headache Pain 20, 104. doi: 10.1186/s10194-019-1058-0
Hubbard, C. S., Khan, S. A., Keaser, M. L., Mathur, V. A., Goyal, M., and Seminowicz, D. A. (2014). Altered brain structure and function correlate with disease severity and pain catastrophizing in migraine patients. eNeuro 1:e20.14. doi: 10.1523/ENEURO.0006-14.2014
Iadarola, M. J., Berman, K. F., Zeffiro, T. A., Byas-Smith, M. G., Gracely, R. H., Max, M. B., et al. (1998). Neural activation during acute capsaicin-evoked pain and allodynia assessed with PET. Brain 121(Pt 5), 931–947. doi: 10.1093/brain/121.5.931
Iwabuchi, S. J., Krishnadas, R., Li, C., Auer, D. P., Radua, J., and Palaniyappan, L. (2015). Localized connectivity in depression: a meta-analysis of resting state functional imaging studies. Neurosci. Biobehav. Rev. 51, 77–86. doi: 10.1016/j.neubiorev.2015.01.006
Jia, Z., and Yu, S. (2017). Grey matter alterations in migraine: a systematic review and meta-analysis. Neuroimage Clin. 14, 130–140. doi: 10.1016/j.nicl.2017.01.019
Kaiser, R. H., Andrews-Hanna, J. R., Wager, T. D., and Pizzagalli, D. A. (2015). Large-scale network dysfunction in major depressive disorder: a meta-analysis of resting-state functional connectivity. JAMA Psychiatry 72, 603–611. doi: 10.1001/jamapsychiatry.2015.0071
Katja, W., Markus, P., and Irene, T. (2008). Neurocognitive aspects of pain perception. Trends Cong. Sci. 12, 306–313. doi: 10.1016/j.tics.2008.05.005
Katsarava, Z., Mania, M., Lampl, C., Herberhold, J., and Steiner, T. J. (2018). Poor medical care for people with migraine in Europe - evidence from the Eurolight study. J. Headache Pain 19:10. doi: 10.1186/s10194-018-0839-1
Katsarava, Z., and Steiner, T. J. (2012). Neglected headache: ignorance, arrogance or insouciance? Cephalalgia 32, 1019–1020. doi: 10.1177/0333102412459478
Kim, J. H., Kim, S., Suh, S. I., Koh, S. B., Park, K. W., and Oh, K. (2010). Interictal metabolic changes in episodic migraine: a voxel-based FDG-PET study. Cephalalgia 30, 53–61. doi: 10.1111/j.1468-2982.2009.01890.x
Kim, Y. E., Kim, M. K., Suh, S. I., and Kim, J. H. (2021). Altered trigeminothalamic spontaneous low-frequency oscillations in migraine without aura: a resting-state fMRI study. BMC Neurol. 21:342. doi: 10.1186/s12883-021-02374-7
Lai, T. H., Chou, K. H., Fuh, J. L., Lee, P. L., Kung, Y. C., Lin, C. P., et al. (2016). Gray matter changes related to medication overuse in patients with chronic migraine. Cephalalgia 36, 1324–1333. doi: 10.1177/0333102416630593
Lamm, C., Decety, J., and Singer, T. (2011). Meta-analytic evidence for common and distinct neural networks associated with directly experienced pain and empathy for pain. Neuroimage 54, 2492–2502. doi: 10.1016/j.neuroimage.2010.10.014
Lee, M. J., Park, B. Y., Cho, S., Kim, S. T., Park, H., and Chung, C. S. (2019). Increased connectivity of pain matrix in chronic migraine: a resting-state functional MRI study. J. Headache Pain 20:29. doi: 10.1186/s10194-019-0986-z
Li, M., Li, X., Zhu, W., Zhu, J., Wang, H., Gao, Z., et al. (2022). The contribution of the left precuneus to emotion memory in migraine without aura patients. Front. Neurosci. 16:905942. doi: 10.3389/fnins.2022.905942
Liberati, A., Altman, D. G., Tetzlaff, J., Mulrow, C., Gøtzsche, P. C., Ioannidis, J. P., et al. (2009). The PRISMA statement for reporting systematic reviews and meta-analyses of studies that evaluate healthcare interventions: explanation and elaboration. BMJ 339:b2700. doi: 10.1136/bmj.b2700
Lim, L., Howells, H., Radua, J., and Rubia, K. (2020). Aberrant structural connectivity in childhood maltreatment: a meta-analysis. Neurosci. Biobehav. Rev. 116, 406–414. doi: 10.1016/j.neubiorev.2020.07.004
Lo Buono, V., Bonanno, L., Corallo, F., Pisani, L. R., Lo Presti, R., Grugno, R., et al. (2017). Functional connectivity and cognitive impairment in migraine with and without aura. J. Headache Pain 18:72. doi: 10.1186/s10194-017-0782-6
Maleki, N., Becerra, L., and Borsook, D. (2012). Migraine: maladaptive brain responses to stress. Headache 52(Suppl. 2), 102–106. doi: 10.1111/j.1526-4610.2012.02241.x
McEwen, B. S., and Gianaros, P. J. (2011). Stress- and allostasis-induced brain plasticity. Annu. Rev. Med. 62, 431–445. doi: 10.1146/annurev-med-052209-100430
Mehnert, J., Schulte, L., Timmann, D., and May, A. (2017). Activity and connectivity of the cerebellum in trigeminal nociception. Neuroimage 150, 112–118. doi: 10.1016/j.neuroimage.2017.02.023
Messina, R., Rocca, M. A., Colombo, B., Valsasina, P., Meani, A., Falini, A., et al. (2020). Dysregulation of multisensory processing stands out from an early stage of migraine: a study in pediatric patients. J. Neurol. 267, 760–769. doi: 10.1007/s00415-019-09639-9
Moulton, E. A., Becerra, L., Maleki, N., Pendse, G., Tully, S., Hargreaves, R., et al. (2011). Painful heat reveals hyperexcitability of the temporal pole in interictal and ictal migraine states. Cereb. Cortex 21, 435–448. doi: 10.1093/cercor/bhq109
Moulton, E. A., Pendse, G., Becerra, L. R., and Borsook, D. (2012). BOLD responses in somatosensory cortices better reflect heat sensation than pain. J. Neurosci. 32, 6024–6031. doi: 10.1523/JNEUROSCI.0006-12.2012
Müller, V. I., Cieslik, E. C., Laird, A. R., Fox, P. T., Radua, J., Mataix-Cols, D., et al. (2018). Ten simple rules for neuroimaging meta-analysis. Neurosci. Biobehav. Rev. 84, 151–161. doi: 10.1016/j.neubiorev.2017.11.012
Nahman-Averbuch, H., Martucci, K. T., Granovsky, Y., Weissman-Fogel, I., Yarnitsky, D., and Coghill, R. C. (2014). Distinct brain mechanisms support spatial vs temporal filtering of nociceptive information. Pain 155, 2491–2501. doi: 10.1016/j.pain.2014.07.008
Napadow, V., LaCount, L., Park, K., As-Sanie, S., Clauw, D. J., and Harris, R. E. (2010). Intrinsic brain connectivity in fibromyalgia is associated with chronic pain intensity. Arthritis Rheum. 62, 2545–2555. doi: 10.1002/art.27497
Ochsner, K. N., Silvers, J. A., and Buhle, J. T. (2012). Functional imaging studies of emotion regulation: a synthetic review and evolving model of the cognitive control of emotion. Ann. N. Y. Acad. Sci. 1251, E1–E24. doi: 10.1111/j.1749-6632.2012.06751.x
Pan, P., Zhan, H., Xia, M., Zhang, Y., Guan, D., and Xu, Y. (2017a). Aberrant regional homogeneity in Parkinson’s disease: a voxel-wise meta-analysis of resting-state functional magnetic resonance imaging studies. Neurosci. Biobehav. Rev. 72, 223–231. doi: 10.1016/j.neubiorev.2016.11.018
Pan, P., Zhang, Y., Liu, Y., Zhang, H., Guan, D., and Xu, Y. (2017b). Abnormalities of regional brain function in Parkinson’s disease: a meta-analysis of resting state functional magnetic resonance imaging studies. Sci. Rep. 7:40469. doi: 10.1038/srep40469
Pan, P., Zhu, L., Yu, T., Shi, H., Zhang, B., Qin, R., et al. (2017c). Aberrant spontaneous low-frequency brain activity in amnestic mild cognitive impairment: a meta-analysis of resting-state fMRI studies. Ageing Res. Rev. 35, 12–21. doi: 10.1016/j.arr.2016.12.001
Pereira, M., Rafiq, B., Chowdhury, E., Babayev, J., Boo, H., Metwaly, R., et al. (2017). Anodal cerebellar tDCS modulates lower extremity pain perception. NeuroRehabilitation 40, 195–200. doi: 10.3233/NRE-161404
Radua, J., and Mataix-Cols, D. (2009). Voxel-wise meta-analysis of grey matter changes in obsessive-compulsive disorder. Br. J. Psychiatry 195, 393–402. doi: 10.1192/bjp.bp.108.055046
Radua, J., and Mataix-Cols, D. (2012). Meta-analytic methods for neuroimaging data explained. Biol. Mood Anxiety Disord. 2:6. doi: 10.1186/2045-5380-2-6
Radua, J., Mataix-Cols, D., Phillips, M. L., El-Hage, W., Kronhaus, D. M., Cardoner, N., et al. (2012). A new meta-analytic method for neuroimaging studies that combines reported peak coordinates and statistical parametric maps. Eur. Psychiatry 27, 605–611. doi: 10.1016/j.eurpsy.2011.04.001
Radua, J., Rubia, K., Canales-Rodríguez, E. J., Pomarol-Clotet, E., Fusar-Poli, P., and Mataix-Cols, D. (2014). Anisotropic kernels for coordinate-based meta-analyses of neuroimaging studies. Front. Psychiatry 5:13. doi: 10.3389/fpsyt.2014.00013
Robbins, M. S. (2021). Diagnosis and management of headache: a review. JAMA 325, 1874–1885. doi: 10.1001/jama.2021.1640
Ruscheweyh, R., Kühnel, M., Filippopulos, F., Blum, B., Eggert, T., and Straube, A. (2014). Altered experimental pain perception after cerebellar infarction. Pain 155, 1303–1312. doi: 10.1016/j.pain.2014.04.006
Russo, A., Silvestro, M., Tedeschi, G., and Tessitore, A. (2017). Physiopathology of migraine: what have we learned from functional imaging? Curr. Neurol. Neurosci. Rep. 17:95. doi: 10.1007/s11910-017-0803-5
Russo, A., Tessitore, A., Giordano, A., Corbo, D., Marcuccio, L., De Stefano, M., et al. (2012). Executive resting-state network connectivity in migraine without aura. Cephalalgia 32, 1041–1048. doi: 10.1177/0333102412457089
Safiri, S., Pourfathi, H., Eagan, A., Mansournia, M. A., Khodayari, M. T., Sullman, M. J. M., et al. (2022). Global, regional, and national burden of migraine in 204 countries and territories, 1990 to 2019. Pain 163, e293–e309. doi: 10.1097/j.pain.0000000000002275
Schankin, C. J., Maniyar, F. H., Sprenger, T., Chou, D. E., Eller, M., and Goadsby, P. J. (2014). The relation between migraine, typical migraine aura and “visual snow”. Headache 54, 957–966. doi: 10.1111/head.12378
Schoenen, J., Ambrosini, A., Sándor, P. S., and Maertens de Noordhout, A. (2003). Evoked potentials and transcranial magnetic stimulation in migraine: published data and viewpoint on their pathophysiologic significance. Clin. Neurophysiol. 114, 955–972. doi: 10.1016/S1388-2457(03)00024-5
Schweizer, R., Voit, D., and Frahm, J. (2008). Finger representations in human primary somatosensory cortex as revealed by high-resolution functional MRI of tactile stimulation. Neuroimage 42, 28–35. doi: 10.1016/j.neuroimage.2008.04.184
Skorobogatykh, K., van Hoogstraten, W. S., Degan, D., Prischepa, A., Savitskaya, A., Ileen, B. M., et al. (2019). Functional connectivity studies in migraine: what have we learned? J. Headache Pain 20, 108. doi: 10.1186/s10194-019-1047-3
Soares, J. M., Sampaio, A., Ferreira, L. M., Santos, N. C., Marques, P., Marques, F., et al. (2013). Stress impact on resting state brain networks. PLoS One 8:e66500. doi: 10.1371/journal.pone.0066500
Sterne, J. A., Sutton, A. J., Ioannidis, J. P., Terrin, N., Jones, D. R., Lau, J., et al. (2011). Recommendations for examining and interpreting funnel plot asymmetry in meta-analyses of randomised controlled trials. BMJ 343:d4002. doi: 10.1136/bmj.d4002
Tessitore, A., Russo, A., Giordano, A., Conte, F., Corbo, D., De Stefano, M., et al. (2013). Disrupted default mode network connectivity in migraine without aura. J. Headache Pain 14:89. doi: 10.1186/1129-2377-14-89
Tfelt-Hansen, P. C. (2010). History of migraine with aura and cortical spreading depression from 1941 and onwards. Cephalalgia 30, 780–792. doi: 10.1111/j.1468-2982.2009.02015.x
Tomasi, D., and Volkow, N. D. (2011). Association between functional connectivity hubs and brain networks. Cereb. Cortex 21, 2003–2013. doi: 10.1093/cercor/bhq268
Torta, D. M., Legrain, V., Mouraux, A., and Valentini, E. (2017). Attention to pain! A neurocognitive perspective on attentional modulation of pain in neuroimaging studies. Cortex 89, 120–134. doi: 10.1016/j.cortex.2017.01.010
Ueno, T., Fastrich, G. M., and Murayama, K. (2016). Meta-analysis to integrate effect sizes within an article: possible misuse and type I error inflation. J. Exp. Psychol. Gen. 145, 643–654. doi: 10.1037/xge0000159
Viechtbauer, W. (2005). Bias and efficiency of meta-analytic variance estimators in the random-effects model. J. Educ. Behav. Stat. 30, 261–293. doi: 10.3102/10769986030003261
Vincent, M. B., and Hadjikhani, N. (2007a). Migraine aura and related phenomena: beyond scotomata and scintillations. Cephalalgia 27, 1368–1377. doi: 10.1111/j.1468-2982.2007.01388.x
Vincent, M., and Hadjikhani, N. (2007b). The cerebellum and migraine. Headache 47, 820–833. doi: 10.1111/j.1526-4610.2006.00715.x
Vo, P., Fang, J., Bilitou, A., Laflamme, A. K., and Gupta, S. (2018). Patients’ perspective on the burden of migraine in Europe: a cross-sectional analysis of survey data in France, Germany, Italy, Spain, and the United Kingdom. J. Headache Pain 19:82. doi: 10.1186/s10194-018-0907-6
Wager, T. D., Atlas, L. Y., Lindquist, M. A., Roy, M., Woo, C. W., and Kross, E. (2013). An fMRI-based neurologic signature of physical pain. N. Engl. J. Med. 368, 1388–1397. doi: 10.1056/NEJMoa1204471
Wang, J. J., Chen, X., Sah, S. K., Zeng, C., Li, Y. M., Li, N., et al. (2016). Amplitude of low-frequency fluctuation (ALFF) and fractional ALFF in migraine patients: a resting-state functional MRI study. Clin. Radiol. 71, 558–564. doi: 10.1016/j.crad.2016.03.004
Wang, M., Su, J., Zhang, J., Zhao, Y., Yao, Q., Zhang, Q., et al. (2017). Visual cortex and cerebellum hyperactivation during negative emotion picture stimuli in migraine patients. Sci. Rep. 7:41919. doi: 10.1038/srep41919
Welch, K. M. (2005). Brain hyperexcitability: the basis for antiepileptic drugs in migraine prevention. Headache 45(Suppl. 1), S25–S32. doi: 10.1111/j.1526-4610.2005.4501008.x
Xu, H., Chen, Y., Tao, Y., Zhang, Y., Zhao, T., Wang, M., et al. (2022). Modulation effect of acupuncture treatment on chronic neck and shoulder pain in female patients: evidence from periaqueductal gray-based functional connectivity. CNS Neurosci. Ther. 28, 714–723. doi: 10.1111/cns.13803
Xue, T., Yuan, K., Zhao, L., Yu, D., Zhao, L., Dong, T., et al. (2012). Intrinsic brain network abnormalities in migraines without aura revealed in resting-state fMRI. PLoS One 7:e52927. doi: 10.1371/journal.pone.0052927
Yang, H., Li, X., Guo, X. L., Zhou, J., Shen, Z. F., Liu, L. Y., et al. (2022). Moxibustion for primary dysmenorrhea: a resting-state functional magnetic resonance imaging study exploring the alteration of functional connectivity strength and functional connectivity. Front. Neurosci. 16:969064. doi: 10.3389/fnins.2022.969064
Yao, L., Yang, C., Zhang, W., Li, S., Li, Q., Chen, L., et al. (2021). A multimodal meta-analysis of regional structural and functional brain alterations in type 2 diabetes. Front. Neuroendocrinol. 62:100915. doi: 10.1016/j.yfrne.2021.100915
Youssef, A. M., Macefield, V. G., and Henderson, L. A. (2016). Cortical influences on brainstem circuitry responsible for conditioned pain modulation in humans. Hum. Brain Mapp. 37, 2630–2644. doi: 10.1002/hbm.23199
Yu, H., Li, M. L., Li, Y. F., Li, X. J., Meng, Y., Liang, S., et al. (2020). Anterior cingulate cortex, insula and amygdala seed-based whole brain resting-state functional connectivity differentiates bipolar from unipolar depression. J. Affect. Disord. 274, 38–47. doi: 10.1016/j.jad.2020.05.005
Zhang, D., Huang, X., Su, W., Chen, Y., Wang, P., Mao, C., et al. (2020). Altered lateral geniculate nucleus functional connectivity in migraine without aura: a resting-state functional MRI study. J. Headache Pain 21:17. doi: 10.1186/s10194-020-01086-6
Zhang, J., Su, J., Wang, M., Zhao, Y., Yao, Q., Zhang, Q., et al. (2016). Increased default mode network connectivity and increased regional homogeneity in migraineurs without aura. J. Headache Pain 17:98. doi: 10.1186/s10194-016-0692-z
Zhen, D., Xia, W., Yi, Z. Q., Zhao, P. W., Zhong, J. G., Shi, H. C., et al. (2018). Alterations of brain local functional connectivity in amnestic mild cognitive impairment. Transl. Neurodegener. 7:26. doi: 10.1186/s40035-018-0134-8
Keywords: migraine, meta-analysis, resting-state functional magnetic resonance imaging, default mode network, functional connectivity
Citation: Hu S, Hao Z, Li M, Zhao M, Wen J, Gao Y, Wang Q, Xi H, Antwi CO, Jia X and Ren J (2023) Resting-state abnormalities in functional connectivity of the default mode network in migraine: A meta-analysis. Front. Neurosci. 17:1136790. doi: 10.3389/fnins.2023.1136790
Received: 03 January 2023; Accepted: 15 February 2023;
Published: 01 March 2023.
Edited by:
Mingrui Xia, Beijing Normal University, ChinaReviewed by:
Bo Hu, Fourth Military Medical University, ChinaHuaibin Liang, Shanghai Jiao Tong University, China
Lin-Feng Yan, Air Force Medical University, China
Copyright © 2023 Hu, Hao, Li, Zhao, Wen, Gao, Wang, Xi, Antwi, Jia and Ren. This is an open-access article distributed under the terms of the Creative Commons Attribution License (CC BY). The use, distribution or reproduction in other forums is permitted, provided the original author(s) and the copyright owner(s) are credited and that the original publication in this journal is cited, in accordance with accepted academic practice. No use, distribution or reproduction is permitted which does not comply with these terms.
*Correspondence: Jun Ren, cmVuakB6am51LmNu
†These authors have contributed equally to this work and share first authorship