- 1Department of Mechanical Engineering, The University of Alabama, Tuscaloosa, AL, United States
- 2Department of Computer Science, The University of Alabama, Tuscaloosa, AL, United States
Introduction: Human locomotion is affected by several factors, such as growth and aging, health conditions, and physical activity levels for maintaining overall health and well-being. Notably, impaired locomotion is a prevalent cause of disability, significantly impacting the quality of life of individuals. The uniqueness and high prevalence of human locomotion have led to a surge of research to develop experimental protocols for studying the brain substrates, muscle responses, and motion signatures associated with locomotion. However, from a technical perspective, reproducing locomotion experiments has been challenging due to the lack of standardized protocols and benchmarking tools, which impairs the evaluation of research quality and the validation of previous findings.
Methods: This paper addresses the challenges by conducting a systematic review of existing neuroimaging studies on human locomotion, focusing on the settings of experimental protocols, such as locomotion intensity, duration, distance, adopted brain imaging technologies, and corresponding brain activation patterns. Also, this study provides practical recommendations for future experiment protocols.
Results: The findings indicate that EEG is the preferred neuroimaging sensor for detecting brain activity patterns, compared to fMRI, fNIRS, and PET. Walking is the most studied human locomotion task, likely due to its fundamental nature and status as a reference task. In contrast, running has received little attention in research. Additionally, cycling on an ergometer at a speed of 60 rpm using fNIRS has provided some research basis. Dual-task walking tasks are typically used to observe changes in cognitive function. Moreover, research on locomotion has primarily focused on healthy individuals, as this is the scenario most closely resembling free-living activity in real-world environments.
Discussion: Finally, the paper outlines the standards and recommendations for setting up future experiment protocols based on the review findings. It discusses the impact of neurological and musculoskeletal factors, as well as the cognitive and locomotive demands, on the experiment design. It also considers the limitations imposed by the sensing techniques used, including the acceptable level of motion artifacts in brain-body imaging experiments and the effects of spatial and temporal resolutions on brain sensor performance. Additionally, various experiment protocol constraints that need to be addressed and analyzed are explained.
1. Introduction
About 28% of American adults older than 50 in the general community presented with impaired locomotion and its prevalence increased with age (p < 0.001) (Mahlknecht et al., 2013). The causes of impaired locomotion are divided into neurological (e.g., Parkinson's disease, stroke, Multiple Sclerosis, and dementia) (Allali et al., 2018; Buckley et al., 2019) and/or musculoskeletal drivers, such as arthritis and cardiovascular conditions (Blyth et al., 2019; Andonian and Huffman, 2020; Minetto et al., 2020). Among these neurological and musculoskeletal impacts, previous research has identified different patterns of gait disorders, such as parkinsonian (De Bartolo et al., 2020; Guayacán and Mart́ınez, 2021), frontal (Hülser et al., 2022), or spastic gait (Muñoz-Lasa et al., 2019; Norbye et al., 2020). Furthermore, researchers have argued that locomotion should be defined as a syndrome for pre-clinical outcomes, such as motoric cognitive risk syndrome for pre-dementia (Xiang et al., 2021; Li et al., 2022a). Because of the neurological and musculoskeletal correlates of locomotion, rehabilitation treatments have been explored to utilize locomotion training, such as treadmill gait exercise, to improve patients' musculoskeletal ability and further induce neurological benefits (Smania et al., 2011; Hornby et al., 2016; Bassiri et al., 2022). Therefore, each human locomotion contains unique features of neural and musculoskeletal drivers, clinical conditions, the complexities of human development and aging, and signifiers of physical activity for health and wellness (Runge and Hunter, 2006; Pons et al., 2013; Kerkman et al., 2018).
Understanding the neurological and musculoskeletal correlates of locomotion is a pivotal need to pave the way for successful rehabilitation, improve performing locomotion tasks, especially in older adults, or create a digital twin of humans while doing a locomotion task (Dai et al., 2022). Various techniques, such as electroencephalography (EEG), functional near-infrared spectroscopy (fNIRS), and magnetic resonance imaging (MRI), have been developed and deployed to study age-related changes and specific diseases in the neurological and musculoskeletal correlates (Lewis et al., 2019). These various techniques enable us access to the movement phenotypes, such as brain structures (Chenausky and Tager-Flusberg, 2022), the functional substrates (Magrinelli et al., 2021), and motion signatures (Klibaite et al., 2022), in locomotion control during different experimental settings. However, these neuroimaging techniques present advantages and limitations, such as sensitivity to motion artifacts (Abtahi et al., 2020; Bonnal et al., 2022), portability (Sejdić et al., 2019), and spatial and temporal resolutions (Martini et al., 2020; Kumar and Michmizos, 2022). These limitations set up constraints on the design of the experimental protocols. For instance, MRI studies mainly investigated imagined locomotion rather than real-world locomotion (Stolbkov et al., 2019; Skinner et al., 2022). Although the sensitivity of fNIRS and EEG to artifacts has been improved, still most existing studies focus on low-intensity movement (e.g., walking) rather than high intensity, such as running.
To overcome these challenges and limitations of traditional brain imaging techniques, most recent research argued that brain-body imaging techniques that enable simultaneous measurements of dynamics of brain activities and body movements could be a promising method to reveal the profound relationship between brain, body, and behavior (Makeig et al., 2009; Gramann et al., 2010; Gwin et al., 2010; Wagner et al., 2012). The brain/body imaging techniques integrated portable devices, reliable sensing methods against motion artifacts, and capable of monitoring brain activities and locomotion with appropriate temporal and spatial resolutions, and sophisticated data analysis approaches for multi-modal data preprocessing and curation. Thus far, some specialized centers and clinics such as GE HealthCare (HealthCare, 2023), SCL Health (Group, 2023), Stratus (Stratus, 2023), Zeto (Zeto, 2023), CMS (CMS, 2023), CNS (Calyx, 2023), NordicNeuroLab (NordicNeuroLab, 2023), and NIRx (NIRx, 2023) have adopted these brain/body imaging tools. With multiple-channel EEG (more than 64) capturing brain activities, these tools track spatial information of brain correlates of human motion and provide high-precision data at a sampling rate of 200–5,00 Hz (Cortney Bradford et al., 2019). Simultaneously, they utilized standard locomotion tools, such as optical motion capture systems (Divya and Peter, 2022), inertial measurement units (Khaksar et al., 2021), and EMG sensors (Hallett et al., 2021) to capture the locomotion data at the same sampling rate as brain activity data. As a result, sophisticated data analysis approaches have been developed to study the relationship between brain, body, and behavior, such as cognitive-motor interference and coherence (Zhu et al., 2022).
However, before these brain-body imaging tools can truly be adopted for clinical use, their effectiveness must be carefully assessed. From a technical point of view, reproducing human locomotion experiments has been problematic due to the lack of protocols, standardization, and benchmarking tools, which ultimately impairs the evaluation of previous research quality and validation of prior understandings (Parmentier et al., 2020; Kameli et al., 2021). Notably, only a few studies focused on developing standard protocols and related design methodology as an open research issue. Therefore, this paper focuses on reviewing the settings of existing experiment protocols, such as locomotion intensity, duration, distance, brain sensor technologies, and corresponding brain activation expressions. First, we develop a conceptual framework to identify the design methodology of experiment protocols. Limitations of each brain-body imaging technique and the corresponding constraints on protocol design are then characterized and mapped into the scope of the systematic review approach. Next, we review existing studies that implement various types of experiment protocols, demonstrating the current gaps in design methodology. Finally, metrics for evaluating the research quality and implications for reproducing prior knowledge are proposed.
Overall, we aim to provide a roadmap for the future development of locomotion analysis methods based on brain-body imaging techniques, including highlighting current progress, identifying various constraints, and suggesting potential research directions. The main contributions of this review paper are to:
• Establish intrinsic links between neurological and musculoskeletal correlates of locomotion characteristics and quantifiable measures that brain-body imaging tools can capture;
• Review existing experimental protocols for studying neurological and musculoskeletal correlates of locomotion;
• Examine the feasibility of replicating the experiments in the laboratory systems, and finally,
• Identify gaps and lay out a roadmap for the design methodology of experiment protocols.
2. Methodology
The design methodology of experimental protocols for studies on neurological and musculoskeletal correlates of human locomotion is based on careful considerations of cognitive and locomotive demands, observation constrained by the sensing technology, the pathology derived from the research interests, and energetic costs invested by the human subjects. Ideally, the cognitive and locomotive demands are designed in specific thresholds to stimulate certain intertwined relationships between neurological and/or musculoskeletal activities. However, the sensing technology's thresholds of these demands are constrained, including sensitivity to motion artifacts, portability in different environments, and spatial and temporal resolutions for capturing dynamics. For instance, current sensing techniques, such as EEG and fNIRS, claim they have lowered their sensitivity to motion artifacts. Still, most researchers design low-intensity locomotion (e.g., slow walking) to avoid difficulty in denoising efforts. Sometimes, in high-intensity locomotion experiments (e.g., running), the data become useless due to a high amount of movement artifacts (Gwin et al., 2011). Figure 1 illustrates the conceptual framework of the design methodology of experimental protocols.
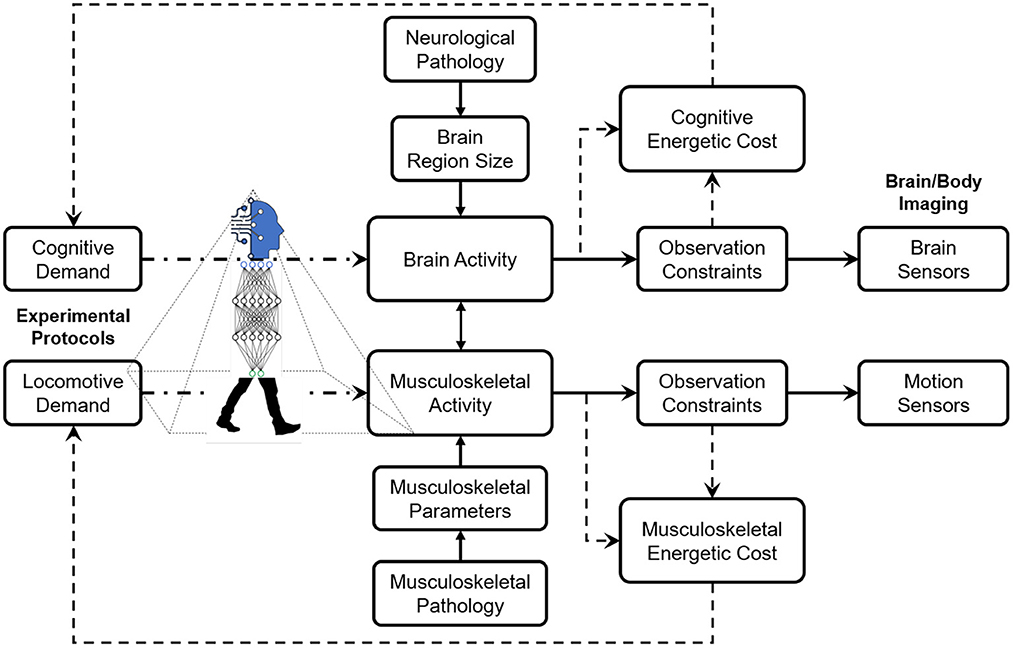
Figure 1. The design methodology of experimental protocols is based on comprehensive considerations of cognitive and locomotive demands, observation constraints by the sensing technology, and energetic costs determined by the human subjects.
We developed a systematic search of existing studies based on the conceptual framework. To find the papers, these keywords were searched for in Google Scholar: human brain locomotion/locomotion, OR EEG, OR nirs, OR MRI/ Brain-Body Imaging. Then, all the papers were examined, and the related papers were added to the tables of this paper. The criteria for a paper to be reviewed are: 1) it must examine the brain's signals while the participants perform a locomotion task. 2) The experiment participants should be healthy; the results of this systematic search are shown in Figure 2. Although exploring walking disorders is highly valuable, there are thorough reviews for each locomotion disease. Then, the critical information on protocol design has been extracted and inserted into the tables. In this respect, these parameters in each protocol have been extracted: the type of the surface or the device used for experimenting (e.g., overground and treadmill), the speed of performing the task, the distance that participants have moved, the duration of the task, the type of the sensors used in the experiment (it is more focused on the sensors to read the brain's data), number and age of the participants, special conditions of each research, and the contribution of the research.
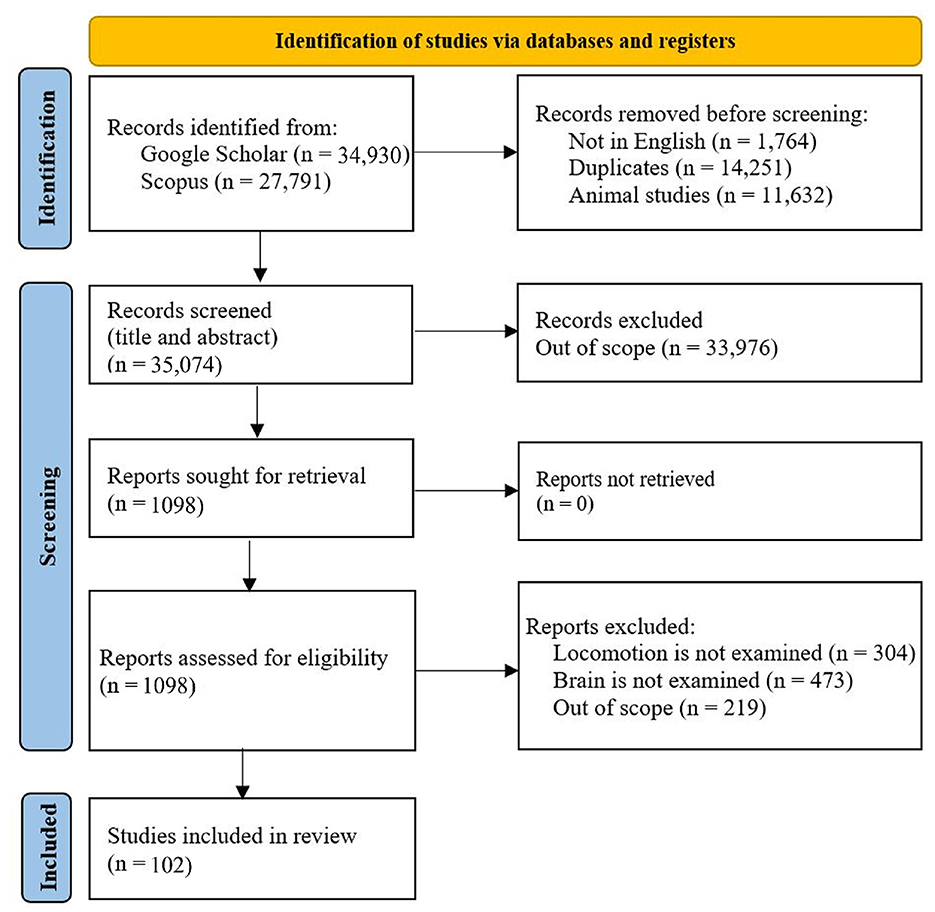
Figure 2. Mobile body imaging study selection: Preferred items for systematic reviews and meta-analyzes (PRISMA) flow diagram.
All the results were converted to the same units to make the protocols comparable. Accordingly, the distance is stated in meters (m), the speed is in kilometers per hour (km/h), and the duration of the task is in minutes (min); otherwise, the unit is stated.
3. Locomotive and cognitive demands
To examine neural activities during human locomotion, single tasks without cognitive demands (e.g., walking) and dual tasks with cognitive demands (e.g., talking while walking) are examined. In this paper, the tasks are divided into walking, running, cycling, and Dual-Task-Walking (DTW). The abbreviations used in this paper are explained in Table 1.
• In the participant section, M shows the number of male participants and the rest are Females.
• a ± b is used to describe the participants' age statistics in which a is mean and b is standard deviation.
• If participants are older than 60, they are considered as OA.
3.1. Type 1: Walking
Walking has been examined in different forms: actual walking, imaginary walking, and simulated walking. Nonetheless, the common point between these studies is the activation of the brain and involvement of higher cognition control areas (Al-Yahya et al., 2011). The studies that have investigated the walking as the human locomotion are shown in Table 2.
3.2. Type 2: Running
Only one paper was found that merely focused on examining running as a locomotion task and studying brain activities. This paper's information is described in Table 3.
3.3. Type 3: Cycling
Cycling is another locomotive task to study the brain correlates of locomotion. The locomotive demands span from low to high intensity, with various cycling speeds, while few studies gave self-paced instructions to participants. Most studies expected to utilize the locomotive demands to stimulate the dynamics of brain activities for identifying neurological and musculoskeletal benefits. The research studies related to the cycling are demonstrated in Table 4.
3.4. Type 1-2: Walking-running
In this section, three research were found that have investigated both walking and running their studies, which meet the defined criteria for research selection in this review. These studies are investigated in Table 5.
3.5. Type 1–2: Walking-cycling
Only one paper shown in Table 6 was found that has examined both walking and cycling.
3.6. Type 4: Dual walking task-spontaneous locomotive and cognitive demands
By switching from single tasks to dual tasks, the age-related gait changes are more distinguished (Beurskens and Bock, 2012) the reason roots in the fact that the cognitive resources should compensate for the motor impairments (Mirelman et al., 2017). Because of this reason, in this type of locomotion task, usually both young adults (YA) and old adults (OA) are examined. The Dual task walking studies are presented in Table 7.
4. Results
4.1. Task analysis
The filtered papers based on the mentioned criteria are 102 papers. Most of the papers, 65, conducted research on low-intensity locomotion, walking, only 4 papers examined running, 17 papers examined cycling, and 18 papers conducted the experiment on dual task, which considers the locomotive and cognitive demands simultaneously. Figure 3 shows the visualization of the tasks, the surface that the experiment has been conducted on, and the brain sensor that has been employed for the experiment. In this regard, the most outer layer of the circle shows the investigated locomotion tasks (walking, running, cycling, and dual-task walking). The middle circle demonstrates the type of the surface (Tr: treadmill, IM: imaginary, OG: overground, Erg: Ergometer bicycle, B: on a board). The type of brain sensor used in the research are shown in the most inner circle (EEG, NIRS, MRI, and PET).
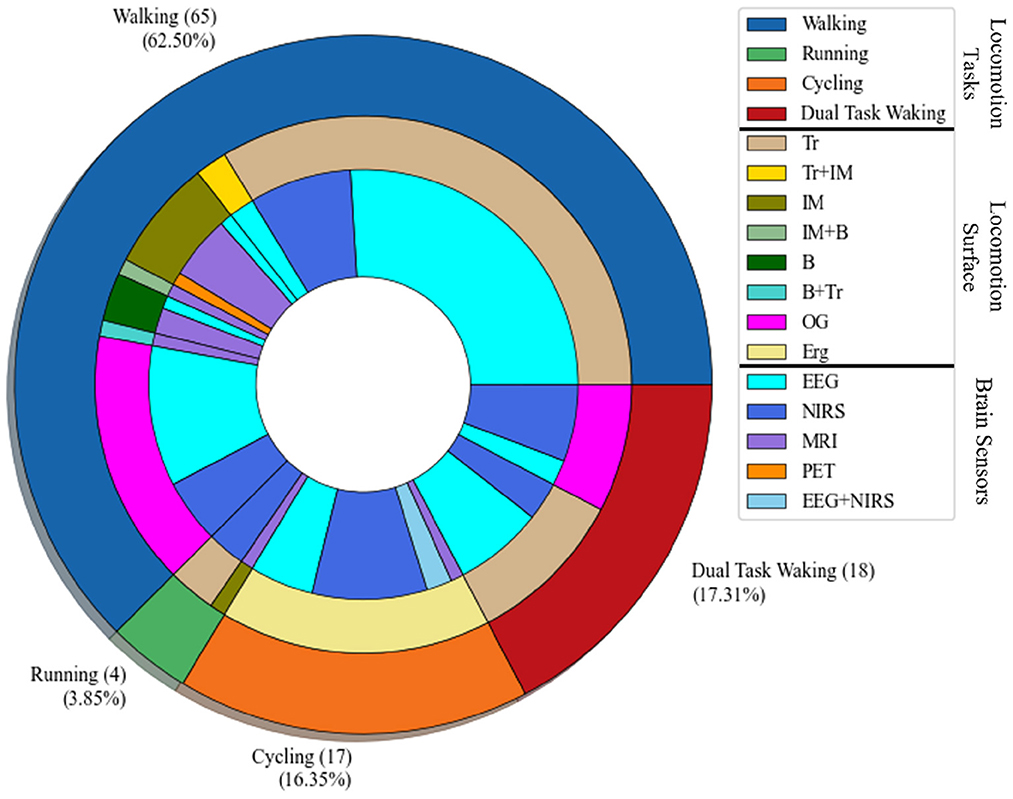
Figure 3. Task-Surface-Brain sensor visualization: the most outer layer shows the type of the locomotion task; walking, running, cycling, and dual-task walking. The middle layer shows the type of the surface used for the body-brain imaging experiment, which are Tr: treadmill, IM: imaginary task, B: Board with pedals, OG: overground, Erg: Ergometer cycling. The most inner layer shows the type of the brain sensor used in the brain-body imaging.
4.2. Analysis of locomotive intensity
Among the researches that have declared the intensity (e.g., speed) that locomotion has been performed, the papers that have stated their speed (or an average of speed) have been compared with each other. However, the researches that the speed was not defined quantitatively and constant (e.g., the speed was dependent on the length of the participants' feet length Wagner et al., 2012, 2014; Seeber et al., 2014) were excluded from the visualization. Accordingly, the rotational velocities when the brain-body imaging experiment is using an ergometer or a pedaling board are visualized in Figure 4. The experiment using 60 rpm in cycling has been considered a reference speed for most researchers. Besides the rotational velocities of Figure 4, the linear speed for SW and DTW on different surfaces (i.e., Tr and OG) for YA and OA are shown in Figure 5. The bigger the bulb, the more repetition on reported speed. For example, walking at the speed of 2 Km/h is the main standard for researchers on the treadmill when the participants are YA. In addition, in each experiment surface, a trend of using a higher speed for YA compared to the speed used for OA can also be observed in the reviewed papers in this figure. For instance, in DTW OG, the maximum speed reported for YA is 4.6 Km/h, although the maximum speed reported for OA in DTW OG is about 3.8 Km/h.
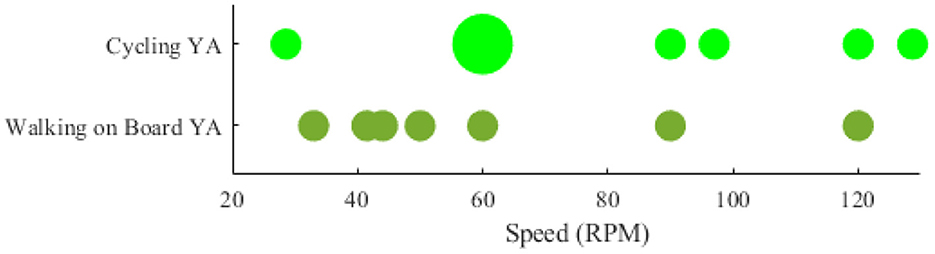
Figure 4. Comparing locomotive intensity in two types of brain-body imaging tasks performed with different rotational speeds: 1) cycling 2) walking on a board with pedals. Only younger adults have been employed to perform these tasks.
4.3. Analysis of participants' age
To analyze the age of the participants, when the age of the participant is unknown, the research is excluded in the visualization of Figure 6. In this regard, there is a significant gap in the ages between 40 and 60 years old as only studies had participants in this range of age.
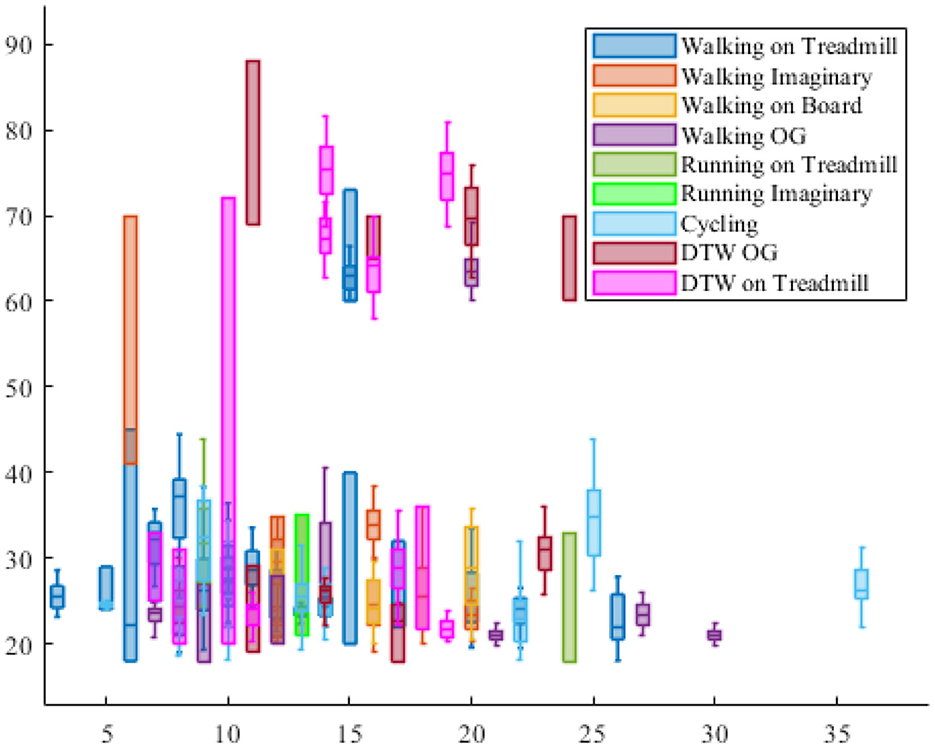
Figure 6. The participants' age distribution in each locomotion experiment. The vertical axis shows the age of the participants and the horizontal axis shows the number of participant in the reported experiment protocol.
4.4. Analysis of locomotion duration
The duration of locomotion task varies from seconds to hours (Figure 7). In this regard, when it comes to single walking, the experiment on the treadmill with a duration of 12 min is the primary source for experiments. To make the results of studies with different duration comparable, the effect of fatigue on the muscles needs to be studied, and one solution could be recording the data after the locomotion task for a specific time.
4.5. Analysis of locomotion distance
Besides the duration, another parameter for assessing the locomotion demands is the distance when the participants were asked to walk overground. The walk distance falls in a range of 1 to 150 meters. For better demonstration, only the distances up to 30 meters are shown in Figure 8.
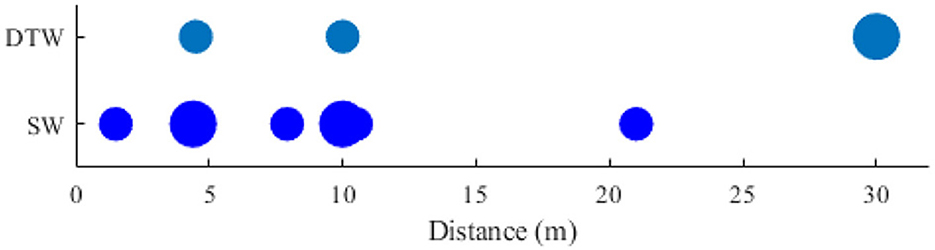
Figure 8. Comparing distance the walk distance in two types of Brain-body imaging experiments: 1) DTW and 2) SW.
4.6. Brain activation in brain-body imaging experiments
In this section, the corresponding brain activation to the locomotion is presented. Comparison of the brain activation areas for the locomotion tasks are shown in Figure 9. In this figure, we have only shown the results of the reviewed papers that have specifically mentioned the brain regions' activation sources and their effects. However, if a study generally describes the cortex area is excluded from this visualization. In this figure, ∝ is the proportional symbol, ↑ shows increasing, and ↓ shows reduction of an item. For instance, when the OA's walk speed increases, oxyHB in the Supplementary Motor Area increases.
5. Discussion
In this section, the configurations of locomotive and cognitive demands, the research interests regarding neurological and musculoskeletal drivers, and the observational constraints from the sensing techniques are discussed concerning the impact of brain-body imaging sensors on the design methodology of experimental protocols for measuring dynamics of brain, body, and behavior. Since few papers in the field have adopted a conceptual framework to evaluate the quality of the neurological and musculoskeletal correlates of human locomotion extracted using various methods, it is not easy to compare these state-of-the-art methods. Therefore, in this section, we focus on establishing the elements of the proposed conceptual framework and discuss how to assess the neurological and musculoskeletal measures extracted from brain-body imaging sensors for further clinical use.
5.1. Neurological and musculoskeletal drivers
According to our systematic review results, most studies followed the philosophy of medical diagnosis that is rooted in conducting statistical analysis between different groups (e.g., young vs. old, control vs. patient). These groups were asked to perform specific behavioral protocols under which their performances were supposed to show neurological and musculoskeletal differences reflected by the brain-body imaging data. Therefore, various experimental protocols were explored to capture the differences in statistical norms among groups (Kashuba et al., 2020; Warmerdam et al., 2020). The typical statistical analysis approaches include comparisons of statistical norms (e.g., p-value, effect size) and classifications based on machine learning (e.g., accuracy, precision) (Figueiredo et al., 2018; Hatami et al., 2019; Patil et al., 2019; Hausmann et al., 2021). Few studies adopted representation learning methods based on deep neural networks to explore patterns and features of brain and motion signals to show the group differences (Vásquez-Correa et al., 2018; Talo et al., 2019; Song et al., 2021). Furthermore, specific quantitative assessments, such as clinical outcomes or symptoms, were identified as ground truth so that the same reference could examine both groups. However, no consensus on experimental protocols caused challenges in determining reliable ground truth or references. Otherwise, few studies provided explanations and rationales for the design methodology of behavioral experiments.
Although it lacks standardization of experimental protocols, existing studies still generated consistent conclusions on neurological and musculoskeletal correlates of human locomotion. For example, most studies revealed that the active brain regions during various walking protocols include the motor, sensory, and prefrontal cortexes. The brain areas involved in walking behavior span SMA, premotor cortex, sensorimotor, M1, and left and right prefrontal cortex. Notably, the age-related changes showed that the involvement of PFC increased among old adults, especially during high-speed walking. When the subjects need to adjust their postures for cycling, the involvement of PFC decreases in order to keep mental resources for the maintenance of these additional requirements during exercise. The PFC has been implicated in planning complex cognitive behavior, especially in the resting status. Few studies explored the running protocols; therefore, there is little consistent knowledge regarding neurological and musculoskeletal correlates of human running. It is noteworthy that when subjects are required to conduct spontaneous cognitive tasks, the PFC activation increases in all age populations, and its increase is more significant in older adults with and without cognitive impairment. These conclusions have motivated many hypothesis-driven research projects on disease-related changes in neurological and musculoskeletal correlates of human locomotion.
Besides the consistent understanding of neurological correlates of human locomotion, mixed results and conclusions exist due to the heterogeneity of participants' neurological expressions, musculoskeletal variance under imprecise experimental protocols, and observation constraints by the sensing techniques. For instance, some studies concluded that the cerebral cortex controls multiple muscles hierarchically through a few synergies during walking. In contrast, few studies argued that the role of cortical control during walking might not be valid due to the motion artifacts of EEG. Furthermore. Subjects that conducted actual walking were more accurately classified than subjects with imaginary walking. Subjects that walked on a treadmill showed different band activities captured by EEG compared to subjects who walked over the ground. These inconsistent results require further investment in establishing protocols, standardization, and benchmarking tools, which also motivated this research work.
5.2. Locomotive and cognitive demands
The primary assumption underlying the experimental protocols is that the locomotive and cognitive demands could stimulate changes and patterns in neural activity, which the brain-body sensors could pick up (Ladouce et al., 2019). Thus, the knowledge of the simulation mechanisms further guides the treatment and rehabilitation strategies for potential clinical outcomes. However, most experimental protocols involve low-intensity locomotion tasks, such as walking and cycling, while few studies conducted high-intensity locomotion tasks, such as running. In addition, little research explained the design methodology of the experimental protocol, especially the research hypothesis of which types of neural activities might be expected under the experimental configurations, such as duration, intervals, and frequency. Also, conducting behavioral studies on human subjects is always challenging, even more, if considering psychological factors such as state of mind, concentration, and technical dexterity. Therefore, some studies integrated cognitive demands into locomotive tasks, such as avoiding obstacles, determining walking directions, or following an avatar. Other studies developed a dual-task paradigm to examine the involvement of cognition in human locomotion.
As illustrated in Figure 1, the conceptual framework argued that more work is needed to examine the simulation mechanisms, thus developing a better design methodology for experimental protocols. The systematic review results showed that several challenging questions remain in the research field. First, benchmarking the locomotive and cognitive demands will be needed. Locomotive tasks have several configurable parameters, such as duration, intervals, and frequency, which need to divide into several levels or intensities. Cognitive tasks also have configurable parameters, such as type and complexity, depending on working memory and executive functions. For instance, for a specific subpopulation's demographic, locomotion and cognition capacity, researchers in designing experimental protocol need a basic understanding of which levels of demands might stimulate the anticipated brain activity. Knowledge of locomotion disorder patterns and corresponding brain areas has helped establish experimental protocols for several neurological diseases, such as parkinsonian gait for Parkinson disease (Ghai et al., 2018) and NIH cognitive toolbox for cognitive impairment (Gershon et al., 2010). Second, manipulation of the tasks needs more research investment. Existing studies rarely consider how the sequential configuration of the tasks stimulates neural activity. Most protocols conducted a heuristic-designed sequence of locomotive tasks and anticipated the brain-body sensors could capture the subtle changes or patterns of neural activities. However, the loop from demands to brain and musculoskeletal activities to sensors, in Figure 1, shows that manipulation of the demands could generate richer information than the heuristic-designed protocol. Third, the experimental protocols should be easily administered to avoid confusion and distraction for participants. Few studies gave a clear description of how the protocols are being instructed. Cueing the participants toward specific tasks could have influenced the expected neural activity and cognitive performance. Therefore, most researches argued that when the experiment protocol is administered and instructed by assistance of a computer is less likely that neural activity and cognitive performance get adversely affected compared to the case that the experiment is controlled and instructed by interference of a human. Hence, replication of previous experiment protocols that were controlled by a human as examiner with a computer-assisted administration provides a high-quality data with removal of the human interference affects (Vrana and Vrana, 2017; Dror, 2020; Young et al., 2022). Moreover, computer assistance in the cognitive load assessment could make it feasible to conduct the experiment at places out of the clinics and laboratories. These places (e.g., participants' home or a local clinic) are accessible to the participants and individuals are comfortable to perform the experiment with assistance of a computer without interference of an examiner. As positive side effects of the computer-assisted experiment administration, high-quality and cost-effective patient care could be provided (Porrselvi, 2022; Young et al., 2022).
5.3. Observation constraints by the sensing techniques
Another dimension of our systematic review results is illustrating the impacts of the observation constraints by the brain-body sensing techniques. Previous work has reviewed and discussed the advantages and disadvantages of these techniques, including tolerance of motion artifacts and spatial and temporal resolutions. However, most studies reviewed in this work did not explain the rationale for selecting specific sensing techniques in experimental protocol design. According to our review results, it is obvious to see the impacts of sensing techniques on the research results and generated knowledge. Therefore, we summarize these impacts for enabling guidelines for future researchers to design experimental standards and benchmarks.
5.3.1. Tolerance of motion artifacts
Prior knowledge of sensing techniques has concluded that among three of them, MRI has the lowest tolerance to motion artifacts, EEG less, and fNIRS the highest. Despite efforts in signal processing and denoising to improve each technique's tolerance to motion artifacts, most studies still followed the knowledge and showed different experimental results. Studies that adopted MRI has considered its limited tolerance of motion artifacts and mainly designed imaginary locomotion or simulated surrogate tasks rather than actual locomotion in their experiments (Stolbkov et al., 2019; Amemiya et al., 2021). The central assumption of these studies is that neurological correlates of these imaginary or surrogate tasks are closely related to neurological activation during actual locomotion. However, our summarized results in locomotion tables and Figure 9 showed that these imaginary or surrogate tasks could not simulate the complex dynamics the brain must execute to adjust and maintain the musculoskeletal patterns during actual locomotion. Especially studies that utilized EEG have shown that actual walking stimulated brain activation patterns that could be classified with higher precision than imagery walking. Therefore, studies that adopted EEG sensors designed a more comprehensive range of locomotion tasks, from imaginary walking and cycling to running. Furthermore, the fNIRS studies are preferred in high-intensity locomotion experiments. More than 50% of studies reviewed in this work that conducted cycling and running tasks adopted fNIRS sensors.
Sensitivity of EEG data to motion artifacts is a research concern and the results of some previous published research due to not considering an extensive removal of motion artifacts has been questioned. For instance, the reported EEG results indicating the changes in high-gamma frequency band during walking (Gwin et al., 2011) could be caused by motion artifacts as well (Castermans et al., 2014). To address the considerable drawback, different denoising methods to employ during or after data collection have been developed. During data collection, artifact removal is associated with hardware modifications. In this respect, one effective way has been introduced to separate electrophysiological signals from non-neural signals. To this end, in one approach, two layers are used below the EEG cap. A silicone layer is used on top of the scalp to block electrophysiological signal. Then, a simulated conductive scalp with similar impedance to human scalp is used to measure the voltage differences generated by gait dynamics (Snyder et al., 2015). In another approach, well-known as a EEG dual electrode design, simultaneously EEG data and isolated motion artifacts are recorded by pairs of the electrodes that are electrically independent and mechanically coupled (Nordin et al., 2018; Clark et al., 2020). After the data collection, denoising is coupled with software data processing. In this regard, Independent Component Analysis (ICA), low, high, and band band pass filter are routinely applied. Besides these remedies, ICA-based methods such as adaptive ICA mixture model algorithm (Palmer et al., 2006), extended infomax ICA (Lee et al., 1999) and multiple mixture ICA (Allen et al., 2000) approaches are utilized (Gwin et al., 2010). Moreover, a developed conductive head phantom and robotic motion platform has emerged as a powerful tool to analyze the artifact removal methods through generating a ground truth for EEG signal. This device is used to evaluate artifact removal methods such as dual-layer EEG and Artifact Subspace Reconstruction (Richer et al., 2020). Also, this device is used to show that electrodes with larger surface reduces the electrodes vulnerability to motion artifacts (Symeonidou et al., 2018). In addition, this device is employed to assess the effect of the motion artifacts parameters such as frequency and amplitude (Oliveira et al., 2016).
5.3.2. Spatial and temporal resolutions
MRI has the highest spatial resolution among the three sensing techniques while fNIRS has the lowest (only centimeters under the skull) (Li et al., 2022b). Accordingly, MRI studies discussed their results with a detailed description of the brain cortex, such as SMA and the dorsal premotor cortex. Nonetheless, lacking the portability feature has significantly limited the application of the MRI in the brain-body imaging of locomotion studies mostly to imaginary task and thus, this scope of study has been deprived from the high spatial resolution of the MRI imaging technique. On the other hand, although EEG has lower spatial resolution than MRI, appropriate source localization approaches could improve its spatial resolution at a cortical level. When source localization is used, the spatial resolution of the fNIRS and EEG are comparable. However, EEG has the highest temporal resolution among the three methods and permits the highest and the most precise data brain investigation compared to the fNIRS and MRI methods. Thus, Looking into the results summarized in our work, we can see that studies that adopted EEG sensors provided the neurological and musculoskeletal correlates on a fine-grained temporal scale, such as neural activation patterns during different movement periods (e.g., swing, stance). These features has made the EEG the most popular technique in the explorations of the brain-body imaging of human locomotion.
5.3.3. Miscellaneous constraints
We also observed other constraints in the design methodology of experimental protocols, such as imbalanced ages of participants. For example, most studies recruited participants aged below 40, while fewer recruited participants older than 60. A significant age gap (40–60) among the participants involved in these studies existed. However, the adults within this age range are baby boomers in the U.S., a large sector of the population, a group deemed by the Centers for Disease Control and Prevention (CDC) as mid-life stage adults. This age population is facing an increase in clinical and other preventive services to support maintaining good health into older age (Pearson-Stuttard et al., 2019; Doubeni et al., 2021), which means this age group should be a critical population for studies on neurological and musculoskeletal correlates of human locomotion. Although there might exist real-world challenges to recruiting this specific age population, additional investment in balancing the age distribution of the participants is needed to fulfill a cross-lifespan understanding of human locomotion and its neurological and musculoskeletal correlates.
6. Conclusion
This paper explores the topic of the design methodology of experimental protocols that aim to study neurological and musculoskeletal correlates of human locomotion using brain-body sensing techniques. The review of many types of neural activities stimulated by human locomotion demonstrates the importance of quantitative analysis using brain-body sensors in potential healthcare applications. By reviewing the current design methodology of experimental protocols, this paper illustrates that the protocol design significantly impacts the experimental results due to the heterogeneity of participants' neurological expressions, musculoskeletal variance under the imprecise locomotive and cognitive demands, and observation constraints by the sensing techniques. Finally, the impacts of the experimental protocols are discussed by reviewing the practical issues to provide implications and guidelines for future researchers to design experimental standards and benchmarks.
Brain-body imaging of human locomotion is a vast area of research. This paper focused on a significant research issue: how to reproduce human locomotion experiments. Therefore, we conducted a systematic review of existing experiment protocols to examine various settings and conditions, such as locomotion intensity, locomotion duration, locomotion distance, brain sensing technologies, and corresponding brain activation expressions. In future work, technologies for locomotion sensing and their advantages and disadvantages will be further examined and discussed. Also, upper-limb locomotion, such as shoulder, elbow, wrist, and finger movement, is a broad study that will be examined in another work. Finally, as the participants of this systematic review are healthy, similar brain-body imaging experiment exploration for neuromechanical disorders would be a valuable work to extend.
Data availability statement
The original contributions presented in the study are included in the article/supplementary material, further inquiries can be directed to the corresponding author.
Author contributions
JG designed the study, created the research question, and finalized the manuscript. SK implemented the study process, collected and analyzed the data, and initiated the manuscript. NJ developed the study motivation and significance, guided the data analysis, and revised the manuscript. All authors contributed to the article and approved the submitted version.
Conflict of interest
The authors declare that the research was conducted in the absence of any commercial or financial relationships that could be construed as a potential conflict of interest.
Publisher's note
All claims expressed in this article are solely those of the authors and do not necessarily represent those of their affiliated organizations, or those of the publisher, the editors and the reviewers. Any product that may be evaluated in this article, or claim that may be made by its manufacturer, is not guaranteed or endorsed by the publisher.
References
Abtahi, M., Borgheai, S. B., Jafari, R., Constant, N., Diouf, R., Shahriari, Y., et al. (2020). Merging fnirs-eeg brain monitoring and body motion capture to distinguish parkinsons disease. IEEE Trans. Neural Syst. Rehabil. Eng. 28, 1246–1253. doi: 10.1109/TNSRE.2020.2987888
Alchalabi, B., Faubert, J., and Labbe, D. R. (2019). “EEG can be used to measure embodiment when controlling a walking self-avatar,” in 2019 IEEE Conference on Virtual Reality and 3D User Interfaces (VR) (Osaka: IEEE), 776–783.
Allali, G., Blumen, H. M., Devanne, H., Pirondini, E., Delval, A., and Van De Ville, D. (2018). Brain imaging of locomotion in neurological conditions. Neurophysiol. Clin. 48, 337–359. doi: 10.1016/j.neucli.2018.10.004
Allen, P. J., Josephs, O., and Turner, R. (2000). A method for removing imaging artifact from continuous eeg recorded during functional mri. Neuroimage 12, 230–239. doi: 10.1006/nimg.2000.0599
Al-Yahya, E., Dawes, H., Smith, L., Dennis, A., Howells, K., and Cockburn, J. (2011). Cognitive motor interference while walking: a systematic review and meta-analysis. Neurosci. Biobehav. Rev. 35, 715–728. doi: 10.1016/j.neubiorev.2010.08.008
Amemiya, K., Morita, T., Hirose, S., Ikegami, T., Hirashima, M., and Naito, E. (2021). Neurological and behavioral features of locomotor imagery in the blind. Brain Imaging Behav. 15, 656–676. doi: 10.1007/s11682-020-00275-w
Andonian, B. J., and Huffman, K. M. (2020). Skeletal muscle disease in rheumatoid arthritis: the center of cardiometabolic comorbidities? Curr. Opin. Rheumatol. 32, 297–306. doi: 10.1097/B.O.R.0000000000000697
Artoni, F., Fanciullacci, C., Bertolucci, F., Panarese, A., Makeig, S., Micera, S., et al. (2017). Unidirectional brain to muscle connectivity reveals motor cortex control of leg muscles during stereotyped walking. Neuroimage 159, 403–416. doi: 10.1016/j.neuroimage.2017.07.013
Bassiri, Z., Austin, C., Cousin, C., and Martelli, D. (2022). Subsensory electrical noise stimulation applied to the lower trunk improves postural control during visual perturbations. Gait Posture 96, 22–28. doi: 10.1016/j.gaitpost.2022.05.010
Berger, A., Horst, F., Steinberg, F., Thomas, F., Müller-Eising, C., Schöllhorn, W. I., et al. (2019). Increased gait variability during robot-assisted walking is accompanied by increased sensorimotor brain activity in healthy people. J. Neuroeng. Rehabil. 16, 1–13. doi: 10.1186/s12984-019-0636-3
Beurskens, R., and Bock, O. (2012). Age-related deficits of dual-task walking: a review. Neural Plast. 2012, 608. doi: 10.1155/2012/131608
Billaut, F., Kerris, J. P., Rodriguez, R. F., Martin, D. T., Gore, C. J., and Bishop, D. J. (2013). Interaction of central and peripheral factors during repeated sprints at different levels of arterial O2 saturation. PLoS ONE 8, e77297. doi: 10.1371/journal.pone.0077297
Blyth, F. M., Briggs, A. M., Schneider, C. H., Hoy, D. G., and March, L. M. (2019). The global burden of musculoskeletal pain–where to from here? Am. J. Public Health 109, 35–40. doi: 10.2105/AJPH.2018.304747
Bonnal, J., Monnet, F., Le, B.-T., Pila, O., Grosmaire, A.-G., Ozsancak, C., et al. (2022). Relation between cortical activation and effort during robot-mediated walking in healthy people: a functional near-infrared spectroscopy neuroimaging study (fnirs). Sensors 22, 5542. doi: 10.3390/s22155542
Bradford, J. C., Lukos, J. R., and Ferris, D. P. (2016). Electrocortical activity distinguishes between uphill and level walking in humans. J. Neurophysiol. 115, 958–966. doi: 10.1152/jn.00089.2015
Brantley, J. A., Luu, T. P., Ozdemir, R., Zhu, F., Winslow, A. T., Huang, H., et al. (2016). “Noninvasive eeg correlates of overground and stair walking,” in 2016 38th Annual International Conference of the IEEE Engineering in Medicine and Biology Society (EMBC) (Orlando, FL: IEEE), 5729–5732.
Bruijn, S. M., Van Dieën, J. H., and Daffertshofer, A. (2015). Beta activity in the premotor cortex is increased during stabilized as compared to normal walking. Front. Hum. Neurosci. 9, 593. doi: 10.3389/fnhum.2015.00593
Buckley, C., Alcock, L., McArdle, R., Rehman, R. Z. U., Del Din, S., Mazzà, C., et al. (2019). The role of movement analysis in diagnosing and monitoring neurodegenerative conditions: Insights from gait and postural control. Brain Sci. 9, 34. doi: 10.3390/brainsci9020034
Budde, H., Wegner, M., Soya, H., Voelcker-Rehage, C., and McMorris, T. (2016). Neuroscience of exercise: neuroplasticity and its behavioral consequences. Neural Plast. 2016, 3643879. doi: 10.1155/2016/3643879
Bulea, T. C., Kim, J., Damiano, D. L., Stanley, C. J., and Park, H.-S. (2015). Prefrontal, posterior parietal and sensorimotor network activity underlying speed control during walking. Front. Hum. Neurosci. 9, 247. doi: 10.3389/fnhum.2015.00247
Calyx (2023). ©Calyx. Available online at: https://www.calyx.ai/ (accessed February 14, 2023).
Castermans, T., Duvinage, M., Cheron, G., and Dutoit, T. (2014). About the cortical origin of the low-delta and high-gamma rhythms observed in eeg signals during treadmill walking. Neurosci. Lett. 561, 166–170. doi: 10.1016/j.neulet.2013.12.059
Castermans, T., Duvinage, M., Petieau, M., Hoellinger, T., De Saedeleer, C., Seetharaman, K., et al. (2011). Optimizing the performances of a p300-based brain-computer interface in ambulatory conditions. IEEE J. Emerg. Select. Top. Circ. Syst. 1, 566–577. doi: 10.1109/JETCAS.2011.2179421
Centers for Medicare & Medicaid Services. (2023). CMS.gov. Available online at: https://www.cms.gov/ (accessed February 14, 2023).
Chenausky, K. V., and Tager-Flusberg, H. (2022). The importance of deep speech phenotyping for neurodevelopmental and genetic disorders: a conceptual review. J. Neurodev. Disord. 14, 1–14. doi: 10.1186/s11689-022-09443-z
Clark, D. J., Manini, T. M., Ferris, D. P., Hass, C. J., Brumback, B. A., Cruz-Almeida, Y., et al. (2020). Multimodal imaging of brain activity to investigate walking and mobility decline in older adults (mind in motion study): hypothesis, theory, and methods. Front. Aging Neurosci. 11, 358. doi: 10.3389/fnagi.2019.00358
Cortney Bradford, J., Lukos, J. R., Passaro, A., Ries, A., and Ferris, D. P. (2019). Effect of locomotor demands on cognitive processing. Sci. Rep. 9, 1–12. doi: 10.1038/s41598-019-45396-5
Dai, Y., Wang, J., and Gao, S. (2022). Advanced electronics and artificial intelligence: must-have technologies toward human body digital twins. Adv. Intell. Syst. 2022, 2100263. doi: 10.1007/978-3-031-14054-9
De Bartolo, D., Morone, G., Giordani, G., Antonucci, G., Russo, V., Fusco, A., et al. (2020). Effect of different music genres on gait patterns in parkinson's disease. Neurol. Sci. 41, 575–582. doi: 10.1007/s10072-019-04127-4
De Sanctis, P., Butler, J. S., Malcolm, B. R., and Foxe, J. J. (2014). Recalibration of inhibitory control systems during walking-related dual-task interference: a mobile brain-body imaging (mobi) study. Neuroimage 94, 55–64. doi: 10.1016/j.neuroimage.2014.03.016
De Sanctis, P., Wagner, J., Molholm, S., Foxe, J. J., Blumen, H. M., and Horsthuis, D. J. (2023). Neural signature of mobility-related everyday function in older adults at-risk of cognitive impairment. Neurobiol. Aging 122, 1–11. doi: 10.1016/j.neurobiolaging.2022.11.005
de Tommaso, M., Vecchio, E., Ricci, K., Montemurno, A., De Venuto, D., and Annese, V. F. (2015). “Combined EEG/EMG evaluation during a novel dual task paradigm for gait analysis,” in 2015 6th International Workshop on Advances in Sensors and Interfaces (IWASI) (Gallipoli: IEEE), 181–186.
Divya, R., and Peter, J. D. (2022). Smart healthcare system-a brain-like computing approach for analyzing the performance of detectron2 and posenet models for anomalous action detection in aged people with movement impairments. Complex Intell. Syst. 8, 3021–3040. doi: 10.1007/s40747-021-00319-8
do Nascimento, O. F., Nielsen, K. D., and Voigt, M. (2005). Influence of directional orientations during gait initiation and stepping on movement-related cortical potentials. Behav. Brain Res. 161, 141–154. doi: 10.1016/j.bbr.2005.02.031
Doubeni, C. A., Simon, M., and Krist, A. H. (2021). Addressing systemic racism through clinical preventive service recommendations from the us preventive services task force. JAMA 325, 627–628. doi: 10.1001/jama.2020.26188
Dror, I. E. (2020). Cognitive and human factors in expert decision making: six fallacies and the eight sources of bias. Anal. Chem. 92, 7998–8004. doi: 10.1021/acs.analchem.0c00704
Eggenberger, P., Wolf, M., Schumann, M., and De Bruin, E. D. (2016). Exergame and balance training modulate prefrontal brain activity during walking and enhance executive function in older adults. Front. Aging Neurosci. 8, 66. doi: 10.3389/fnagi.2016.00066
Enders, H., Cortese, F., Maurer, C., Baltich, J., Protzner, A. B., and Nigg, B. M. (2016). Changes in cortical activity measured with eeg during a high-intensity cycling exercise. J. Neurophysiol. 115, 379–388. doi: 10.1152/jn.00497.2015
Figueiredo, J., Santos, C. P., and Moreno, J. C. (2018). Automatic recognition of gait patterns in human motor disorders using machine learning: a review. Med. Eng. Phys. 53, 1–12. doi: 10.1016/j.medengphy.2017.12.006
Fontes, E. B., Bortolotti, H., da Costa, K. G., de Campos, B. M., Castanho, G. K., Hohl, R., et al. (2020). Modulation of cortical and subcortical brain areas at low and high exercise intensities. Br. J. Sports Med. 54, 110–115. doi: 10.1136/bjsports-2018-100295
Fraser, S. A., Dupuy, O., Pouliot, P., Lesage, F., and Bherer, L. (2016). Comparable cerebral oxygenation patterns in younger and older adults during dual-task walking with increasing load. Front. Aging Neurosci. 8, 240. doi: 10.3389/fnagi.2016.00240
Fumoto, M., Oshima, T., Kamiya, K., Kikuchi, H., Seki, Y., Nakatani, Y., et al. (2010). Ventral prefrontal cortex and serotonergic system activation during pedaling exercise induces negative mood improvement and increased alpha band in eeg. Behav. Brain Res. 213, 1–9. doi: 10.1016/j.bbr.2010.04.017
GE HealthCare. (2023). ©General Electric HealthCare. Available online at: https://www.gehealthcare.com/ (accessed February 14, 2023).
Gershon, R. C., Cella, D., Fox, N. A., Havlik, R. J., Hendrie, H. C., and Wagster, M. V. (2010). Assessment of neurological and behavioural function: the nih toolbox. Lancet Neurol. 9, 138–139. doi: 10.1016/S1474-4422(09)70335-7
Ghai, S., Ghai, I., Schmitz, G., and Effenberg, A. O. (2018). Effect of rhythmic auditory cueing on parkinsonian gait: a systematic review and meta-analysis. Sci. Rep. 8, 1–19. doi: 10.1038/s41598-017-16232-5
Giles, G. E., Cantelon, J. A., Eddy, M. D., Brunyé, T. T., Urry, H. L., Taylor, H. A., et al. (2018). Cognitive reappraisal reduces perceived exertion during endurance exercise. Motiv. Emot. 42, 482–496. doi: 10.1007/s11031-018-9697-z
Gramann, K., Gwin, J. T., Bigdely-Shamlo, N., Ferris, D. P., and Makeig, S. (2010). Visual evoked responses during standing and walking. Front. Hum. Neurosci. 4, 202. doi: 10.3389/fnhum.2010.00202
Guayacán, L. C., and Martínez, F. (2021). Visualising and quantifying relevant parkinsonian gait patterns using 3d convolutional network. J. Biomed. Inform. 123, 103935. doi: 10.1016/j.jbi.2021.103935
Gwin, J. T., Gramann, K., Makeig, S., and Ferris, D. P. (2010). Removal of movement artifact from high-density EEG recorded during walking and running. J. Neurophysiol. 103, 3526–3534. doi: 10.1152/jn.00105.2010
Gwin, J. T., Gramann, K., Makeig, S., and Ferris, D. P. (2011). Electrocortical activity is coupled to gait cycle phase during treadmill walking. Neuroimage 54, 1289–1296. doi: 10.1016/j.neuroimage.2010.08.066
Hallett, M., DelRosso, L. M., Elble, R., Ferri, R., Horak, F. B., Lehericy, S., et al. (2021). Evaluation of movement and brain activity. Clin. Neurophysiol. 132, 2608–2638. doi: 10.1016/j.clinph.2021.04.023
Harada, T., Miyai, I., Suzuki, M., and Kubota, K. (2009). Gait capacity affects cortical activation patterns related to speed control in the elderly. Exp. Brain Res. 193, 445–454. doi: 10.1007/s00221-008-1643-y
Hasan, S. S., Siddiquee, M. R., and Bai, O. (2019). “Supervised classification of eeg signals with score threshold regulation for pseudo-online asynchronous detection of gait intention,” in 2019 18th IEEE International Conference on Machine Learning and Applications (ICMLA) (Boca Raton, F: IEEE), 1476–1479.
Hatami, T., Hamghalam, M., Reyhani-Galangashi, O., and Mirzakuchaki, S. (2019). “A machine learning approach to brain tumors segmentation using adaptive random forest algorithm,” in 2019 5th Conference on Knowledge Based Engineering and Innovation (KBEI) (Tehran: IEEE), 076–082.
Hausmann, S. B., Vargas, A. M., Mathis, A., and Mathis, M. W. (2021). Measuring and modeling the motor system with machine learning. Curr. Opin. Neurobiol. 70, 11–23. doi: 10.1016/j.conb.2021.04.004
Holtzer, R., Mahoney, J. R., Izzetoglu, M., Izzetoglu, K., Onaral, B., and Verghese, J. (2011). fnirs study of walking and walking while talking in young and old individuals. J. Gerontol. A Biomed. Sci. Med. Sci. 66, 879–887. doi: 10.1093/gerona/glr068
Hornby, T. G., Moore, J. L., Lovell, L., and Roth, E. J. (2016). Influence of skill and exercise training parameters on locomotor recovery during stroke rehabilitation. Curr. Opin. Neurol. 29, 677. doi: 10.1097/WCO.0000000000000397
Hülser, M., Spielmann, H., Oertel, J., and Sippl, C. (2022). Motor skills, cognitive impairment, and quality of life in normal pressure hydrocephalus: early effects of shunt placement. Acta Neurochir. 164, 1765–1775. doi: 10.1007/s00701-022-05149-2
Ikeda, T., Matsushita, A., Saotome, K., Hasegawa, Y., Matsumura, A., and Sankai, Y. (2016). Muscle activity during gait-like motion provided by MRI compatible lower-extremity motion simulator. Adv. Robot. 30, 459–475. doi: 10.1080/01691864.2015.1122552
Iseki, K., Hanakawa, T., Shinozaki, J., Nankaku, M., and Fukuyama, H. (2008). Neural mechanisms involved in mental imagery and observation of gait. Neuroimage 41, 1021–1031. doi: 10.1016/j.neuroimage.2008.03.010
Jahn, K., Deutschländer, A., Stephan, T., Strupp, M., Wiesmann, M., and Brandt, T. (2004). Brain activation patterns during imagined stance and locomotion in functional magnetic resonance imaging. Neuroimage 22, 1722–1731. doi: 10.1016/j.neuroimage.2004.05.017
Jain, S., Gourab, K., Schindler-Ivens, S., and Schmit, B. D. (2013). EEG during pedaling: evidence for cortical control of locomotor tasks. Clin. Neurophysiol. 124, 379–390. doi: 10.1016/j.clinph.2012.08.021
Kameli, N., Dragojlovic-Kerkache, A., Savelkoul, P., and Stassen, F. R. (2021). Plant-derived extracellular vesicles: current findings, challenges, and future applications. Membranes 11, 411. doi: 10.3390/membranes11060411
Kashuba, V., Stepanenko, O., Byshevets, N., Kharchuk, O., Savliuk, S., Bukhovets, B., et al. (2020). The formation of human movement and sports skills in processing sports-pedagogical and biomedical data in masters of sports. Int. J. Human Movement Sport Sci. 8, 249–257. doi: 10.13189/saj.2020.080513
Keramidas, M. E., Kounalakis, S. N., Eiken, O., and Mekjavic, I. B. (2011). Muscle and cerebral oxygenation during exercise performance after short-term respiratory work. Respiratory Physiol. Neurobiol. 175, 247–254. doi: 10.1016/j.resp.2010.11.009
Kerkman, J. N., Daffertshofer, A., Gollo, L. L., Breakspear, M., and Boonstra, T. W. (2018). Network structure of the human musculoskeletal system shapes neural interactions on multiple time scales. Sci. Adv. 4, eaat0497. doi: 10.1126/sciadv.aat0497
Khaksar, S., Pan, H., Borazjani, B., Murray, I., Agrawal, H., Liu, W., et al. (2021). Application of inertial measurement units and machine learning classification in cerebral palsy: randomized controlled trial. JMIR Rehabil. Assist. Technol. 8, e29769. doi: 10.2196/29769
Khan, R. A., Naseer, N., Qureshi, N. K., Noori, F. M., Nazeer, H., and Khan, M. U. (2018). fnirs-based neurorobotic interface for gait rehabilitation. J. Neuroeng. Rehabil. 15, 1–17. doi: 10.1186/s12984-018-0346-2
Klibaite, U., Kislin, M., Verpeut, J. L., Bergeler, S., Sun, X., Shaevitz, J. W., et al. (2022). Deep phenotyping reveals movement phenotypes in mouse neurodevelopmental models. Mol. Autism 13, 1–18. doi: 10.1186/s13229-022-00492-8
Kline, A., Pittman, D., Ronsky, J., and Goodyear, B. (2020). Differentiating the brain's involvement in executed and imagined stepping using fmri. Behav. Brain Res. 394, 112829. doi: 10.1016/j.bbr.2020.112829
Kumar, N., and Michmizos, K. P. (2022). A neurophysiologically interpretable deep neural network predicts complex movement components from brain activity. Sci. Rep. 12, 1–12. doi: 10.1038/s41598-022-05079-0
Kurz, M. J., Wilson, T. W., and Arpin, D. J. (2012). Stride-time variability and sensorimotor cortical activation during walking. Neuroimage 59, 1602–1607. doi: 10.1016/j.neuroimage.2011.08.084
Labriffe, M., Annweiler, C., Amirova, L. E., Gauquelin-Koch, G., Ter Minassian, A., Leiber, L.-M., et al. (2017). Brain activity during mental imagery of gait versus gait-like plantar stimulation: a novel combined functional mri paradigm to better understand cerebral gait control. Front. Hum. Neurosci. 11, 106. doi: 10.3389/fnhum.2017.00106
Lacerenza, M., Spinelli, L., Buttafava, M., Dalla Mora, A., Zappa, F., Pifferi, A., et al. (2021). Monitoring the motor cortex hemodynamic response function in freely moving walking subjects: a time-domain fnirs pilot study. Neurophotonics 8, 015006. doi: 10.1117/1.NPh.8.1.015006
Ladouce, S., Donaldson, D. I., Dudchenko, P. A., and Ietswaart, M. (2019). Mobile eeg identifies the re-allocation of attention during real-world activity. Sci. Rep. 9, 1–10. doi: 10.1038/s41598-019-51996-y
Lau, T. M., Gwin, J. T., and Ferris, D. P. (2014). Walking reduces sensorimotor network connectivity compared to standing. J. Neuroeng. Rehabil. 11, 1–10. doi: 10.1186/1743-0003-11-14
Lau, T. M., Gwin, J. T., McDowell, K. G., and Ferris, D. P. (2012). Weighted phase lag index stability as an artifact resistant measure to detect cognitive eeg activity during locomotion. J. Neuroeng. Rehabil. 9, 1–9. doi: 10.1186/1743-0003-9-47
Lee, T.-W., Girolami, M., and Sejnowski, T. J. (1999). Independent component analysis using an extended infomax algorithm for mixed subgaussian and supergaussian sources. Neural Comput. 11, 417–441. doi: 10.1162/089976699300016719
Lewis, R., Gómez Álvarez, C. B., Rayman, M., Lanham-New, S., Woolf, A., and Mobasheri, A. (2019). Strategies for optimising musculoskeletal health in the 21st century. BMC Musculoskeletal Disord. 20, 1–15. doi: 10.1186/s12891-019-2510-7
Li, C., Su, M., Xu, J., Jin, H., and Sun, L. (2020). A between-subject fnirs-bci study on detecting self-regulated intention during walking. IEEE Trans. Neural Syst. Rehabil. Eng. 28, 531–540. doi: 10.1109/TNSRE.2020.2965628
Li, J., Chen, G., Thangavel, P., Yu, H., Thakor, N., Bezerianos, A., et al. (2016). “A robotic knee exoskeleton for walking assistance and connectivity topology exploration in eeg signal,” in 2016 6th IEEE International Conference on Biomedical Robotics and Biomechatronics (BioRob) (Singapore: IEEE), 1068–1073.
Li, J., Dimitrakopoulos, G. N., Thangavel, P., Chen, G., Sun, Y., Guo, Z., et al. (2019). What are spectral and spatial distributions of eeg-emg correlations in overground walking? an exploratory study. IEEE Access 7, 143935–143946. doi: 10.1109/ACCESS.2019.2945602
Li, R., Wang, X., Lawler, K., Garg, S., Bai, Q., and Alty, J. (2022a). Applications of artificial intelligence to aid detection of dementia: a scoping review on current capabilities and future directions. J. Biomed. Inform. 2022, 104030. doi: 10.1016/j.jbi.2022.104030
Li, R., Yang, D., Fang, F., Hong, K.-S., Reiss, A. L., and Zhang, Y. (2022b). Concurrent FNIRS and EEG for brain function investigation: a systematic, methodology-focused review. Sensors 22, 5865. doi: 10.3390/s22155865
Lin, Y.-P., Wang, Y., and Jung, T.-P. (2014). Assessing the feasibility of online ssvep decoding in human walking using a consumer eeg headset. J. Neuroeng. Rehabil. 11, 1–8. doi: 10.1186/1743-0003-11-119
Lu, C.-F., Liu, Y.-C., Yang, Y.-R., Wu, Y.-T., and Wang, R.-Y. (2015). Maintaining gait performance by cortical activation during dual-task interference: a functional near-infrared spectroscopy study. PLoS ONE 10, e0129390. doi: 10.1145/2818302
Ludyga, S., Gronwald, T., and Hottenrott, K. (2016). Effects of high vs. low cadence training on cyclists' brain cortical activity during exercise. J. Sci. Med. Sport 19, 342–347. doi: 10.1016/j.jsams.2015.04.003
Luu, T. P., Brantley, J. A., Zhu, F., and Contreras-Vidal, J. L. (2017a). “Cortical features of locomotion-mode transitions via non-invasive EEG,” in 2017 IEEE International Conference on Systems, Man, and Cybernetics (SMC) (Banff, AB: IEEE), 2437–2441.
Luu, T. P., Nakagome, S., He, Y., and Contreras-Vidal, J. L. (2017b). Real-time eeg-based brain-computer interface to a virtual avatar enhances cortical involvement in human treadmill walking. Sci. Rep. 7, 1–12. doi: 10.1038/s41598-017-09187-0
Magrinelli, F., Balint, B., and Bhatia, K. P. (2021). Challenges in clinicogenetic correlations: one gene-many phenotypes. Mov. Disord. Clin. Pract. 8, 299–310. doi: 10.1002/mdc3.13165
Mahlknecht, P., Kiechl, S., Bloem, B. R., Willeit, J., Scherfler, C., Gasperi, A., et al. (2013). Prevalence and burden of gait disorders in elderly men and women aged 60-97 years: a population-based study. PLoS ONE 8, e69627. doi: 10.1371/journal.pone.0069627
Makeig, S., Gramann, K., Jung, T.-P., Sejnowski, T. J., and Poizner, H. (2009). Linking brain, mind and behavior. Int. J. Psychophysiol. 73, 95–100. doi: 10.1016/j.ijpsycho.2008.11.008
Makizako, H., Shimada, H., Park, H., Tsutsumimoto, K., Uemura, K., Suzuki, T., et al. (2013). Brain activation during dual-task walking and executive function among older adults with mild cognitive impairment: a fnirs study. Aging Clin. Exp. Res. 25, 539–544. doi: 10.1007/s40520-013-0119-5
Malcolm, B. R., Foxe, J. J., Butler, J. S., and De Sanctis, P. (2015). The aging brain shows less flexible reallocation of cognitive resources during dual-task walking: a mobile brain/body imaging (mobi) study. Neuroimage 117, 230–242. doi: 10.1016/j.neuroimage.2015.05.028
Malouin, F., Richards, C. L., Jackson, P. L., Dumas, F., and Doyon, J. (2003). Brain activations during motor imagery of locomotor-related tasks: a pet study. Hum. Brain Mapp. 19, 47–62. doi: 10.1002/hbm.10103
Martini, M. L., Oermann, E. K., Opie, N. L., Panov, F., Oxley, T., and Yaeger, K. (2020). Sensor modalities for brain-computer interface technology: a comprehensive literature review. Neurosurgery 86, E108-E117. doi: 10.1093/neuros/nyz286
Mazurek, K. A., Patelaki, E., Foxe, J. J., and Freedman, E. G. (2021). Using the mobi motion capture system to rapidly and accurately localize EEG electrodes in anatomic space. Eur. J. Neurosci. 54, 8396–8405. doi: 10.1111/ejn.15019
Meester, D., Al-Yahya, E., Dawes, H., Martin-Fagg, P., and Piñon, C. (2014). Associations between prefrontal cortex activation and h-reflex modulation during dual task gait. Front. Hum. Neurosci. 8, 78. doi: 10.3389/fnhum.2014.00078
Mehra, D., Tiwari, A., and Joshi, D. (2021). Investigating neural correlates of locomotion transition via temporal relation of eeg and eog-recorded eye movements. Comput. Biol. Med. 132, 104350. doi: 10.1016/j.compbiomed.2021.104350
Minetto, M. A., Giannini, A., McConnell, R., Busso, C., Torre, G., and Massazza, G. (2020). Common musculoskeletal disorders in the elderly: the star triad. J. Clin. Med. 9, 1216. doi: 10.3390/jcm9041216
Mirelman, A., Maidan, I., Bernad-Elazari, H., Nieuwhof, F., Reelick, M., Giladi, N., et al. (2014). Increased frontal brain activation during walking while dual tasking: an fnirs study in healthy young adults. J. Neuroeng. Rehabil. 11, 1–7. doi: 10.1186/1743-0003-11-85
Mirelman, A., Maidan, I., Bernad-Elazari, H., Shustack, S., Giladi, N., and Hausdorff, J. M. (2017). Effects of aging on prefrontal brain activation during challenging walking conditions. Brain Cogn. 115, 41–46. doi: 10.1016/j.bandc.2017.04.002
Miyai, I., Tanabe, H. C., Sase, I., Eda, H., Oda, I., Konishi, I., et al. (2001). Cortical mapping of gait in humans: a near-infrared spectroscopic topography study. Neuroimage 14, 1186–1192. doi: 10.1006/nimg.2001.0905
Muñoz-Lasa, S., de Silanes, C. L., Atín-Arratibel, M., Bravo-Llatas, C., Pastor-Jimeno, S., et al. (2019). Effects of hippotherapy in multiple sclerosis: pilot study on quality of life, spasticity, gait, pelvic floor, depression and fatigue. Med. Clin. (English Edition) 152, 55–58. doi: 10.1016/j.medcle.2018.11.012
Nakagome, S., Luu, T. P., Brantley, J. A., and Contreras-Vidal, J. L. (2017). “Prediction of emg envelopes of multiple terrains over-ground walking from eeg signals using an unscented kalman filter,” in 2017 IEEE International Conference on Systems, Man, and Cybernetics (SMC) (Banff, AB: IEEE), 3175–3178.
NIRx. (2023). ©NIRx Medical Technologies. Available online at: https://nirx.net/ (accessed February 14, 2023).
NordicNeuroLab. (2023). ©NordicNeuroLab. Available online at: https://www.nordicneurolab.com/ (accessed February 14, 2023).
Nojiri, K., and Iwane, F. (2014). “Motion direction estimation of walking base on eeg signal,” in 2014 IEEE/ASME International Conference on Advanced Intelligent Mechatronics (Besacon: IEEE), 542–547.
Norbye, A. D., Midgard, R., and Thrane, G. (2020). Spasticity, gait, and balance in patients with multiple sclerosis: a cross-sectional study. Physiother. Res. Int. 25, e1799. doi: 10.1002/pri.1799
Nordin, A. D., Hairston, W. D., and Ferris, D. P. (2018). Dual-electrode motion artifact cancellation for mobile electroencephalography. J. Neural Eng. 15, 056024. doi: 10.1088/1741-2552/aad7d7
Nordin, A. D., Hairston, W. D., and Ferris, D. P. (2019a). Faster gait speeds reduce alpha and beta eeg spectral power from human sensorimotor cortex. IEEE Trans. Biomed. Eng. 67, 842–853. doi: 10.1109/TBME.2019.2921766
Nordin, A. D., Hairston, W. D., and Ferris, D. P. (2019b). Human electrocortical dynamics while stepping over obstacles. Sci. Rep. 9, 1–12. doi: 10.1038/s41598-019-41131-2
Oliveira, A. S., Schlink, B. R., Hairston, W. D., König, P., and Ferris, D. P. (2016). Induction and separation of motion artifacts in EEG data using a mobile phantom head device. J. Neural Eng. 13, 036014. doi: 10.1088/1741-2560/13/3/036014
Palmer, J. A., Kreutz-Delgado, K., and Makeig, S. (2006). “Super-gaussian mixture source model for ICA,” in International Conference on Independent Component Analysis and Signal Separation. ICA 2006, eds J. Rosca, D. Erdogmus, J. C. Príncipe, and S. Haykin (Berlin; Heidelberg: Springer). doi: 10.1007/11679363_106
Parmentier, T., Monteith, G., Cortez, M. A., Wielaender, F., Fischer, A., Jokinen, T. S., et al. (2020). Effect of prior general anesthesia or sedation and antiseizure drugs on the diagnostic utility of wireless video electroencephalography in dogs. J. Vet. Internal Med. 34, 1967–1974. doi: 10.1111/jvim.15856
Patil, P., Kumar, K. S., Gaud, N., and Semwal, V. B. (2019). “Clinical human gait classification: extreme learning machine approach,” in 2019 1st International Conference on Advances in Science, Engineering and Robotics Technology (ICASERT) (Dhaka: IEEE), 1–6.
Pearson-Stuttard, J., Ezzati, M., and Gregg, E. W. (2019). Multimorbidity–a defining challenge for health systems. Lancet Public Health 4, e599-e600. doi: 10.1016/S2468-2667(19)30222-1
Peters, S., Lim, S. B., Louie, D. R., Yang, C.-L., and Eng, J. J. (2020). Passive, yet not inactive: robotic exoskeleton walking increases cortical activation dependent on task. J. Neuroeng. Rehabil. 17, 1–12. doi: 10.1186/s12984-020-00739-6
Petersen, T. H., Willerslev-Olsen, M., Conway, B. A., and Nielsen, J. B. (2012). The motor cortex drives the muscles during walking in human subjects. J. Physiol. 590, 2443–2452. doi: 10.1113/jphysiol.2012.227397
Pires, F. O., Dos Anjos, C. A., Covolan, R. J., Pinheiro, F. A., St Clair Gibson, A., Noakes, T. D., et al. (2016). Cerebral regulation in different maximal aerobic exercise modes. Front. Physiol. 7, 253. doi: 10.3389/fphys.2016.00253
Pizzamiglio, S., Naeem, U., Abdalla, H., and Turner, D. L. (2017). Neural correlates of single-and dual-task walking in the real world. Front. Hum. Neurosci. 11, 460. doi: 10.3389/fnhum.2017.00460
Pons, J. L., Moreno, J. C., Torricelli, D., and Taylor, J. (2013). “Principles of human locomotion: a review,” in 2013 35th Annual International Conference of the IEEE Engineering in Medicine and Biology Society (EMBC) (Dhaka: IEEE), 6941–6944.
Porrselvi, A. P. (2022). Tam battery: development and pilot testing of a tamil computer-assisted cognitive test battery for older adults. Clin. Neuropsychol. 15, 1–20. doi: 10.1080/13854046.2022.2156396
Presacco, A., Forrester, L. W., and Contreras-Vidal, J. L. (2012). Decoding intra-limb and inter-limb kinematics during treadmill walking from scalp electroencephalographic (EEG) signals. IEEE Trans. Neural Syst. Rehabil. Eng. 20, 212–219. doi: 10.1109/TNSRE.2012.2188304
Presacco, A., Goodman, R., Forrester, L., and Contreras-Vidal, J. L. (2011). Neural decoding of treadmill walking from noninvasive electroencephalographic signals. J. Neurophysiol. 106, 1875–1887. doi: 10.1152/jn.00104.2011
Quiroz, G., Espinoza-Valdez, A., Salido-Ruiz, R., and Mercado, L. (2017). Coherence analysis of eeg in locomotion using graphs. Revista mexicana de ingeniería biomédica 38, 235–246.
Racinais, S., Buchheit, M., and Girard, O. (2014). Breakpoints in ventilation, cerebral and muscle oxygenation, and muscle activity during an incremental cycling exercise. Front. Physiol. 5, 142. doi: 10.3389/fphys.2014.00142
Radel, R., Brisswalter, J., and Perrey, S. (2017). Saving mental effort to maintain physical effort: a shift of activity within the prefrontal cortex in anticipation of prolonged exercise. Cognit. Affect. Behav. Neurosci. 17, 305–314. doi: 10.3758/s13415-016-0480-x
Richer, N., Downey, R. J., Hairston, W. D., Ferris, D. P., and Nordin, A. D. (2020). Motion and muscle artifact removal validation using an electrical head phantom, robotic motion platform, and dual layer mobile eeg. IEEE Trans. Neural Syst. Rehabil. Eng. 28, 1825–1835. doi: 10.1109/TNSRE.2020.3000971
Runge, M., and Hunter, G. (2006). Determinants of musculoskeletal frailty and the risk of falls in old age. J. Musculoskeletal Neuronal Interact. 6, 167.
Sacco, K., Cauda, F., Cerliani, L., Mate, D., Duca, S., and Geminiani, G. C. (2006). Motor imagery of walking following training in locomotor attention. The effect of ‘the tango lesson'. Neuroimage 32, 1441–1449. doi: 10.1016/j.neuroimage.2006.05.018
Sahyoun, C., Floyer-Lea, A., Johansen-Berg, H., and Matthews, P. M. (2004). Towards an understanding of gait control: brain activation during the anticipation, preparation and execution of foot movements. Neuroimage 21, 568–575. doi: 10.1016/j.neuroimage.2003.09.065
Salazar-Varas, R., Costa, Á., Iáñez, E., Úbeda, A., Hortal, E., and Azorín, J. (2015). Analyzing eeg signals to detect unexpected obstacles during walking. J. Neuroeng. Rehabil. 12, 1–15. doi: 10.1186/s12984-015-0095-4
Schneider, S., Rouffet, D., Billaut, F., and Strüder, H. (2013). Cortical current density oscillations in the motor cortex are correlated with muscular activity during pedaling exercise. Neuroscience 228, 309–314. doi: 10.1016/j.neuroscience.2012.10.037
Seeber, M., Scherer, R., Wagner, J., Solis-Escalante, T., and Müller-Putz, G. R. (2014). Eeg beta suppression and low gamma modulation are different elements of human upright walking. Front. Hum. Neurosci. 8, 485. doi: 10.3389/fnhum.2014.00485
Seeber, M., Scherer, R., Wagner, J., Solis-Escalante, T., and Müller-Putz, G. R. (2015). High and low gamma eeg oscillations in central sensorimotor areas are conversely modulated during the human gait cycle. Neuroimage 112, 318–326. doi: 10.1016/j.neuroimage.2015.03.045
Sejdić, E., Godfrey, A., McIlroy, W., and Montero-Odasso, M. (2019). “Engineering human gait and the potential role of wearable,” in Falls and Cognition in Older Persons, eds M. Montero-Odasso, and R. Camicioli (Cham: Springer). doi: 10.1007/978-3-030-24233-6_22
Severens, M., Nienhuis, B., Desain, P., and Duysens, J. (2012). “Feasibility of measuring event related desynchronization with electroencephalography during walking,” in 2012 Annual International Conference of the IEEE Engineering in Medicine and Biology Society (San Diego, CA: IEEE), 2764–2767.
Severens, M., Perusquia-Hernandez, M., Nienhuis, B., Farquhar, J., and Duysens, J. (2014). Using actual and imagined walking related desynchronization features in a bci. IEEE Trans. Neural Syst. Rehabil. Eng. 23, 877–886. doi: 10.1109/TNSRE.2014.2371391
Shibuya, K., Tanaka, J., Kuboyama, N., Muraj, S., and Ogaki, T. (2004). supramaximal exhaustive exercise. J. Sports Med. Phys. Fitness 44, 2.
Sipp, A. R., Gwin, J. T., Makeig, S., and Ferris, D. P. (2013). Loss of balance during balance beam walking elicits a multifocal theta band electrocortical response. J. Neurophysiol. 110, 2050–2060. doi: 10.1152/jn.00744.2012
Sisters of Charity of Leavenworth Health Services Corporation. (2023). ©SCL Health Medical Group. Available online at: https://www.sclhealth.org/locations/medical-group/ (accessed February 14, 2023).
Skinner, J. W., Lee, H. K., and Hass, C. J. (2022). Evaluation of gait termination strategy in individuals with essential tremor and parkinson's disease. Gait Posture 92, 338–342. doi: 10.1016/j.gaitpost.2021.12.007
Smania, N., Bonetti, P., Gandolfi, M., Cosentino, A., Waldner, A., Hesse, S., et al. (2011). Improved gait after repetitive locomotor training in children with cerebral palsy. Am. J. Phys. Med. Rehabil. 90, 137–149. doi: 10.1097/PHM.0b013e318201741e
Smith, K. J., and Billaut, F. (2010). Influence of cerebral and muscle oxygenation on repeated-sprint ability. Eur. J. Appl. Physiol. 109, 989–999. doi: 10.1007/s00421-010-1444-4
Snyder, K. L., Kline, J. E., Huang, H. J., and Ferris, D. P. (2015). Independent component analysis of gait-related movement artifact recorded using EEG electrodes during treadmill walking. Front. Hum. Neurosci. 9, 639. doi: 10.3389/fnhum.2015.00639
Song, S., Kidziński, Ł., Peng, X. B., Ong, C., Hicks, J., Levine, S., et al. (2021). Deep reinforcement learning for modeling human locomotion control in neuromechanical simulation. J. Neuroeng. Rehabil. 18, 1–17. doi: 10.1186/s12984-021-00919-y
Stolbkov, Y., Moshonkina, T., Orlov, I., Tomilovskaya, E., Kozlovskaya, I., and Gerasimenko, Y. P. (2019). The neurophysiological correlates of real and imaginary locomotion. Hum. Physiol. 45, 104–114. doi: 10.1134/S0362119719010146
Storzer, L., Butz, M., Hirschmann, J., Abbasi, O., Gratkowski, M., Saupe, D., et al. (2016). Bicycling and walking are associated with different cortical oscillatory dynamics. Front. Hum. Neurosci. 10, 61. doi: 10.3389/fnhum.2016.00061
Stratus. (2023). ©Stratus. Available online at: https://stratusneuro.com/clinical-trials/ (accessed February 14, 2023).
Subudhi, A. W., Dimmen, A. C., and Roach, R. C. (2007). Effects of acute hypoxia on cerebral and muscle oxygenation during incremental exercise. J. Appl. Physiol. 103, 177–183. doi: 10.1152/japplphysiol.01460.2006
Subudhi, A. W., Miramon, B. R., Granger, M. E., and Roach, R. C. (2009). Frontal and motor cortex oxygenation during maximal exercise in normoxia and hypoxia. J. Appl. Physiol. 106, 1153–1158. doi: 10.1152/japplphysiol.91475.2008
Suzuki, M., Miyai, I., Ono, T., and Kubota, K. (2008). Activities in the frontal cortex and gait performance are modulated by preparation. An fnirs study. Neuroimage 39, 600–607. doi: 10.1016/j.neuroimage.2007.08.044
Suzuki, M., Miyai, I., Ono, T., Oda, I., Konishi, I., Kochiyama, T., et al. (2004). Prefrontal and premotor cortices are involved in adapting walking and running speed on the treadmill: an optical imaging study. Neuroimage 23, 1020–1026. doi: 10.1016/j.neuroimage.2004.07.002
Symeonidou, E.-R., Nordin, A. D., Hairston, W. D., and Ferris, D. P. (2018). Effects of cable sway, electrode surface area, and electrode mass on electroencephalography signal quality during motion. Sensors 18, 1073. doi: 10.3390/s18041073
Takahiro, I., Akira, M., Kousaku, S., Yasuhisa, H., and Yoshiyuki, S. (2013). “Preliminary report of brain activities during active and passive gait-like motion,” in 2013 6th International IEEE/EMBS Conference on Neural Engineering (NER) (San Diego, CA: IEEE), 1566–1569
Talamonti, D., Vincent, T., Fraser, S., Nigam, A., Lesage, F., and Bherer, L. (2021). The benefits of physical activity in individuals with cardiovascular risk factors: a longitudinal investigation using fnirs and dual-task walking. J. Clin. Med. 10, 579. doi: 10.3390/jcm10040579
Talo, M., Yildirim, O., Baloglu, U. B., Aydin, G., and Acharya, U. R. (2019). Convolutional neural networks for multi-class brain disease detection using mri images. Comput. Med. Imaging Graphics 78, 101673. doi: 10.1016/j.compmedimag.2019.101673
Thomas, R., and Stephane, P. (2008). Prefrontal cortex oxygenation and neuromuscular responses to exhaustive exercise. Eur. J. Appl. Physiol. 102, 153–163. doi: 10.1007/s00421-007-0568-7
Tortora, S., Ghidoni, S., Chisari, C., Micera, S., and Artoni, F. (2020). Deep learning-based bci for gait decoding from eeg with lstm recurrent neural network. J. Neural Eng. 17, 046011. doi: 10.1088/1741-2552/ab9842
Úbeda, A., Planelles, D., Costa, A., Hortal, E., Iáñez, E., and Azorín, J. M. (2014). “Decoding knee angles from EEG signals for different walking speeds,” in 2014 IEEE International Conference on Systems, Man, and Cybernetics (SMC) (San Diego, CA: IEEE), 1475–1478.
Vásquez-Correa, J. C., Arias-Vergara, T., Orozco-Arroyave, J. R., Eskofier, B., Klucken, J., and Nöth, E. (2018). Multimodal assessment of parkinson's disease: a deep learning approach. IEEE J. Biomed. Health Inform. 23, 1618–1630. doi: 10.1109/JBHI.2018.2866873
Velu, P. D., and de Sa, V. R. (2013). Single-trial classification of gait and point movement preparation from human EEG. Front. Neurosci. 7, 84. doi: 10.3389/fnins.2013.00084
Vitorio, R., Stuart, S., Gobbi, L. T., Rochester, L., Alcock, L., and Pantall, A. (2018). Reduced gait variability and enhanced brain activity in older adults with auditory cues: a functional near-infrared spectroscopy study. Neurorehabil. Neural Repair. 32, 976–987. doi: 10.1177/1545968318805159
Vrana, S. R., and Vrana, D. T. (2017). Can a computer administer a wechsler intelligence test? Profess. Psychol. Res. Pract. 48, 191. doi: 10.1037/pro0000128
Wagner, J., Makeig, S., Gola, M., Neuper, C., and Müller-Putz, G. (2016). Distinct β band oscillatory networks subserving motor and cognitive control during gait adaptation. J. Neurosci. 36, 2212–2226. doi: 10.1523/JNEUROSCI.3543-15.2016
Wagner, J., Martinez-Cancino, R., Delorme, A., Makeig, S., Solis-Escalante, T., Neuper, C., et al. (2019a). High-density eeg mobile brain/body imaging data recorded during a challenging auditory gait pacing task. Scientific Data 6, 1–9. doi: 10.1038/s41597-019-0223-2
Wagner, J., Martínez-Cancino, R., and Makeig, S. (2019b). Trial-by-trial source-resolved eeg responses to gait task challenges predict subsequent step adaptation. Neuroimage 199, 691–703. doi: 10.1016/j.neuroimage.2019.06.018
Wagner, J., Solis-Escalante, T., Grieshofer, P., Neuper, C., Müller-Putz, G., and Scherer, R. (2012). Level of participation in robotic-assisted treadmill walking modulates midline sensorimotor eeg rhythms in able-bodied subjects. Neuroimage 63, 1203–1211. doi: 10.1016/j.neuroimage.2012.08.019
Wagner, J., Solis-Escalante, T., Scherer, R., Neuper, C., and Müller-Putz, G. (2014). It's how you get there: walking down a virtual alley activates premotor and parietal areas. Front. Hum. Neurosci. 8, 93. doi: 10.3389/fnhum.2014.00093
Wang, J., Wai, Y., Weng, Y., Ng, K., Huang, Y.-Z., Ying, L., et al. (2009). Functional mri in the assessment of cortical activation during gait-related imaginary tasks. J. Neural Transm. 116, 1087–1092. doi: 10.1007/s00702-009-0269-y
Warmerdam, E., Hausdorff, J. M., Atrsaei, A., Zhou, Y., Mirelman, A., Aminian, K., et al. (2020). Long-term unsupervised mobility assessment in movement disorders. Lancet Neurol. 19, 462–470. doi: 10.1016/S1474-4422(19)30397-7
Weersink, J. B., Maurits, N. M., and de Jong, B. M. (2019). EEG time-frequency analysis provides arguments for arm swing support in human gait control. Gait Posture 70, 71–78. doi: 10.1016/j.gaitpost.2019.02.017
Wei, P., Zhang, J., Wang, B., and Hong, J. (2021). Surface electromyography and electroencephalogram-based gait phase recognition and correlations between cortical and locomotor muscle in the seven gait phases. Front. Neurosci. 15, 607905. doi: 10.3389/fnins.2021.607905
Wieser, M., Haefeli, J., Bütler, L., Jäncke, L., Riener, R., and Koeneke, S. (2010). Temporal and spatial patterns of cortical activation during assisted lower limb movement. Exp. Brain Res. 203, 181–191. doi: 10.1007/s00221-010-2223-5
Xiang, K., Liu, Y., and Sun, L. (2021). Motoric cognitive risk syndrome: symptoms, pathology, diagnosis, and recovery. Front. Aging Neurosci. 13, 728799. doi: 10.3389/fnagi.2021.728799
Xu, J., Li, C., Li, J., Zhang, H., Jin, H., Qu, W., et al. (2017a). “Classification of desired motion speed–a study based on cerebral hemoglobin information,” in 2017 2nd International Conference on Advanced Robotics and Mechatronics (ICARM) (Hefei and Tai'an: IEEE), 115–119.
Xu, J., Li, C., Zhang, H., Li, J., Jin, H., Qu, W., et al. (2017b). A pilot study based on cerebral hemoglobin information to classify the desired walking speed. IEEE Robot. Autom. Lett. 3, 532–536. doi: 10.1109/LRA.2017.2765002
Yokoyama, H., Kaneko, N., Ogawa, T., Kawashima, N., Watanabe, K., and Nakazawa, K. (2018). Cortical control of locomotor muscle activity through muscle synergies in humans: a neural decoding study. bioRxiv, 413567. doi: 10.1101/413567
Young, S. R., Maddocks, D. L., and Caemmerer, J. M. (2022). Computer-enhanced practice: The benefits of computer-assisted assessment in applied clinical practice. Profess. Psychol. Res. Pract. 2022, 449. doi: 10.1037/pro0000449
Zeto (2023). ©Zeto. Available online at: https://zeto-inc.com/ (accessed February 14, 2023).
Keywords: human locomotion, observational constraints, brain body imaging, experimental design methodology, cognitive demands, locomotive task, brain muscle functional connectivity
Citation: Korivand S, Jalili N and Gong J (2023) Experiment protocols for brain-body imaging of locomotion: A systematic review. Front. Neurosci. 17:1051500. doi: 10.3389/fnins.2023.1051500
Received: 22 September 2022; Accepted: 06 February 2023;
Published: 01 March 2023.
Edited by:
David M. A. Mehler, University Hospital RWTH Aachen, GermanyReviewed by:
Shanshan Yao, Stony Brook University, United StatesJohanna Wagner, University of California, San Diego, United States
Copyright © 2023 Korivand, Jalili and Gong. This is an open-access article distributed under the terms of the Creative Commons Attribution License (CC BY). The use, distribution or reproduction in other forums is permitted, provided the original author(s) and the copyright owner(s) are credited and that the original publication in this journal is cited, in accordance with accepted academic practice. No use, distribution or reproduction is permitted which does not comply with these terms.
*Correspondence: Jiaqi Gong, amlhcWkuZ29uZ0B1YS5lZHU=