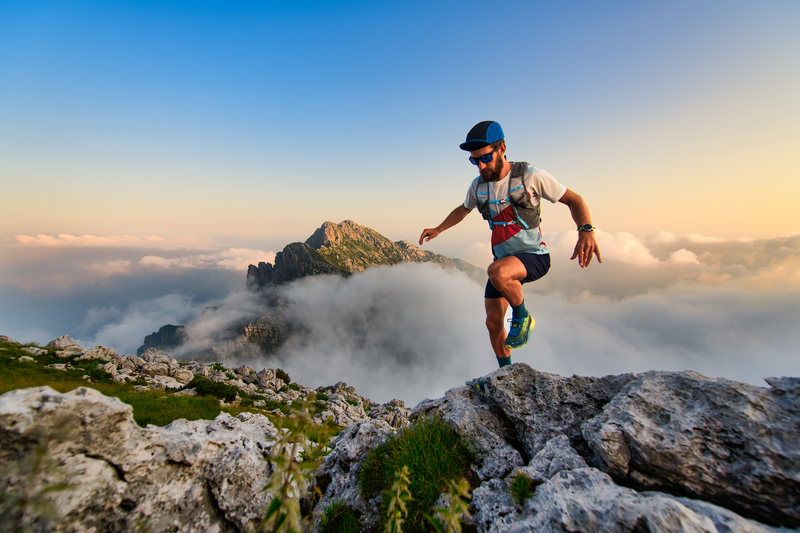
94% of researchers rate our articles as excellent or good
Learn more about the work of our research integrity team to safeguard the quality of each article we publish.
Find out more
SYSTEMATIC REVIEW article
Front. Neurosci. , 02 February 2023
Sec. Auditory Cognitive Neuroscience
Volume 17 - 2023 | https://doi.org/10.3389/fnins.2023.1046669
This article is part of the Research Topic Hearing Loss Rehabilitation and Higher-Order Auditory and Cognitive Processing View all 16 articles
Background: Cochlear implants (CIs) are considered an effective treatment for severe-to-profound sensorineural hearing loss. However, speech perception outcomes are highly variable among adult CI recipients. Top-down neurocognitive factors have been hypothesized to contribute to this variation that is currently only partly explained by biological and audiological factors. Studies investigating this, use varying methods and observe varying outcomes, and their relevance has yet to be evaluated in a review. Gathering and structuring this evidence in this scoping review provides a clear overview of where this research line currently stands, with the aim of guiding future research.
Objective: To understand to which extent different neurocognitive factors influence speech perception in adult CI users with a postlingual onset of hearing loss, by systematically reviewing the literature.
Methods: A systematic scoping review was performed according to the PRISMA guidelines. Studies investigating the influence of one or more neurocognitive factors on speech perception post-implantation were included. Word and sentence perception in quiet and noise were included as speech perception outcome metrics and six key neurocognitive domains, as defined by the DSM-5, were covered during the literature search (Protocol in open science registries: 10.17605/OSF.IO/Z3G7W of searches in June 2020, April 2022).
Results: From 5,668 retrieved articles, 54 articles were included and grouped into three categories using different measures to relate to speech perception outcomes: (1) Nineteen studies investigating brain activation, (2) Thirty-one investigating performance on cognitive tests, and (3) Eighteen investigating linguistic skills.
Conclusion: The use of cognitive functions, recruiting the frontal cortex, the use of visual cues, recruiting the occipital cortex, and the temporal cortex still available for language processing, are beneficial for adult CI users. Cognitive assessments indicate that performance on non-verbal intelligence tasks positively correlated with speech perception outcomes. Performance on auditory or visual working memory, learning, memory and vocabulary tasks were unrelated to speech perception outcomes and performance on the Stroop task not to word perception in quiet. However, there are still many uncertainties regarding the explanation of inconsistent results between papers and more comprehensive studies are needed e.g., including different assessment times, or combining neuroimaging and behavioral measures.
Systematic review registration: https://doi.org/10.17605/OSF.IO/Z3G7W.
Cochlear implants (CIs) are considered an effective treatment for severe-to-profound sensorineural hearing loss, when hearing aids provide insufficient benefits. However, speech perception performance outcomes of this treatment are highly variable among adult CI listeners (Holden et al., 2013). Different biological and audiological factors, such as residual hearing before implantation and duration of hearing loss, only contribute to a small extent when explaining this variation (Zhao et al., 2020). A multicentre study using data from 2,735 adult CI users investigated how much variance in word perception outcomes in quiet could be explained by previously identified factors. When including 17 predictive factors (e.g., duration of hearing loss, etiology, being a native speaker, age at implantation, and preoperative hearing performance) in a linear regression model, the variance explained was only 0.12–0.21 (Goudey et al., 2021).
To decrease uncertainty, other factors, such as (neuro)cognition need to be considered. Neurocognitive factors are skills used to acquire knowledge and manipulate information and reasoning. In addition to bottom-up factors, top-down neurocognitive factors have been proposed to contribute to variation in postoperative speech perception (Baskent et al., 2016; Moberly et al., 2016a). In this context, top-down processing means that higher-order cognitive processes drive lower-order systems. For example, prior knowledge is used for processing incoming information from the senses such as speech (bottom-up information). Bottom-up processes are lower-order mechanisms that, in turn, can trigger additional higher-order processing (Breedlove and Watson, 2013). Interactions of top-down processes and neurocognitive functions with the incoming speech signal, have been shown to be highly important for distorted speech recognition (Davis and Johnsrude, 2007; Stenfelt and Rönnberg, 2009; Mattys et al., 2012). Given that speech signal output from a CI is distorted, neurocognitive mechanisms are needed for active and effortful decoding of this speech. This is thought to enable CI listeners to compensate for the loss of spectro-temporal resolution (Baskent et al., 2016; Moberly et al., 2016a). Several studies have investigated the association of neurocognitive factors and brain activation patterns with CI performance. These studies did not only use varying designs and methods, but also observed varying results. A literature review may help interpret and summarize these outcomes. After a preliminary search for existing reviews in PROSPERO and PubMed (June 2020) showed that these studies were not collected and evaluated in a review before, this scoping review was initiated.
The objective of this scoping review is to gain understanding of which brain activation patterns and top-down neurocognitive factors are associated with speech perception outcomes in hearing-impaired adults after cochlear implantation. This is also done by exploring differences between poorer and better performers. When referring to top-down neurocognitive factors or mechanisms, we refer to the ones that can be classified under one of six neurocognitive domains, defined in the Diagnostic Statistical Manual of Mental Disorders, Fifth Edition (DSM-5); (1) complex attention, (2) executive function, (3) social cognition, (4) learning and memory, (5) perceptual-motor function, and (6) language (Figure 1; Sachdev et al., 2014).
Figure 1. Key cognitive domains defined by the Diagnostic Statistical Manual of Mental Disorders, Fifth Edition (DSM-5). [Source: Sachdev et al. (2014)].
1. Complex attention involves sustained attention, divided attention, selective attention, and processing speed. Attention is a state or condition of selective awareness or perceptual receptivity by which a single stimulus or task (sustained), or several (divided) are selected for enhanced processing, while possibly other irrelevant stimuli, thoughts, and actions are ignored (selective). Cortical regions that play an important role in attentional processes are the posterior parietal lobe and cingulate cortex (Breedlove and Watson, 2013).
2. Executive function includes planning, decision-making, working memory, responding to feedback, inhibition, flexibility, and non-verbal intelligence – all high-level control processes that manage other cognitive functions important for generating meaningful goal-oriented behavior. The frontal lobe is mainly involved in these processes (Breedlove and Watson, 2013).
3. Social cognition refers to cognitive processes involved in social behavior (Hogg and Vaughan, 2018). In other words, how people think about themselves and others and how these processes affect judgment and behavior in a social context, leading to socially appropriate or less appropriate behavior. These behaviors include the recognition of emotions, having theory of mind and insight (Sachdev et al., 2014).
4. Learning and memory include short-term memory, measured by free and cued recall, recognition memory, semantic and autobiographical long-term memory, and implicit learning. Learning is acquiring new and relatively enduring information, behavior patterns or abilities, because of practice or experience. Memory is the ability to store learned information and retrieve or reactivate it over time. Structures of the limbic system, the temporal and frontal cortex are mainly involved in memory formation, but plasticity within the brain also indicates learning (Breedlove and Watson, 2013).
5. Perceptual-motor function includes visual perception, visuoconstructional reasoning and perceptual-motor coordination (Sachdev et al., 2014). These are processes involved in movement and being able to interact with the environment.
6. Language, the most sophisticated structured system for communicating (Breedlove and Watson, 2013), encompasses skills needed for both language production (object naming, word finding, fluency, grammar and syntax) and language comprehension (receptive language and grammar and syntax). Areas involved in language processing are Broca’s area in the frontal lobe, along with the primary motor cortex, the supramarginal gyrus in the parietal cortex, and Wernicke’s area, primary auditory cortex and angular gyrus in the temporal cortex (Breedlove and Watson, 2013).
These domains are not mutually exclusive, meaning that some cognitive functions might be part of processes underlying other cognitive functions. For example, social cognitive skills involve executive functions, such as decision-making. In the same way, this review will explore which cognitive factors are involved in or part of speech perception processing in adult CI users, which can be classified as a neurocognitive factor under the language domain. Furthermore, CI users might recruit several alternative brain regions during auditory and speech perception. Identifying these activation patterns could pinpoint neurocognitive mechanisms that facilitate or constrain speech perception outcomes (Lazard et al., 2010). Therefore, in addition to studies including behavioral cognitive measures, studies using neuroimaging metrics will be explored.
In this review, speech perception outcomes encompass word or sentence perception in quiet and noise. Besides assessing CI performance, some studies use these speech perception outcome metrics to classify patients as good or poor performers (e.g., Suh et al., 2015; Kessler et al., 2020; Völter et al., 2021). However, there are no general guidelines for classifying good and poor performers, resulting in varying performance classification between studies. See for example, Kessler et al. (2020), divided good and poor performers based on sentence perception in noise. Other examples with respect to word perception in quiet are Völter et al. (2017), who used as cut-off scores >30 and <70% for, respectively poor and good performers, while Mortensen et al. (2006), opted for >60 and <96% limits. Suh et al. (2015) used 80% speech perception score to split between poor and good performers. Therefore, when discussing studies having implemented performance classification, their participants will be referred to as “better” and “poorer” performers in this review.
Discussing and summarizing the wide variety of studies investigating the association between neurocognitive factors and CI performance in a systematic scoping review might provide new insights and guide new research on this topic. Research in this field helps understand CI outcome variation and could be particularly valuable to improve care for poorer performing adult CI listeners. Being able to more accurately predict performance outcomes will facilitate managing their expectations. Furthermore, identification of the root causes of poorer performance could lead to the development of individualized aftercare.
The Preferred Reporting Items for Systematic Reviews and Meta-Analysis (PRISMA) was used for this systematic scoping review (Moher et al., 2009). A systematic scoping review was performed instead of a systematic literature review because of the variability in methods between the included studies. Therefore, this review does not include any meta-analysis or risk of bias assessment. Furthermore, Population, Concept, and Context (PCC) was used as the research question framework (Peters et al., 2015). The population being postlingually deaf adult CI users, the concept being speech perception outcomes, in the context of neurocognition. The protocol of this review was registered in the open science registries 10.17605/OSF.IO/Z3G7W.
This review encompasses studies investigating the influence of one or more neurocognitive factors on speech perception after cochlear implantation. Word and sentence perception in quiet and noise were included as speech perception outcome metrics. Studies including participants listening in both unimodal (CI-only), bimodal (CI and hearing aid) and bilateral (CI both ears) conditions were eligible. To provide a complete overview, the six key neurocognitive domains as defined by the DSM-5 were covered during the literature search. No limitations on cognitive measures were implemented. Included study designs were cross-sectional studies, non-randomized control trials, quasi-experimental studies, longitudinal studies, prospective and retrospective cohort studies and meta-analysis performed in a clinical setting. Studies from publication year 2000 and onward were included. Furthermore, all studies involving children and adults with a prelingual onset of deafness were excluded. There were no restrictions on publication status or language of publication (Figure 2B).
Figure 2. (A) Preferred reporting items for systematic reviews and meta-analysis (PRISMA) flowchart of the literature search and study selection. Last date of first search June 2020, numbers are indicated with n1. Last date of second search April 2022, numbers are indicated with n2. (B) Inclusion and exclusion criteria of the articles.
Four scientific databases: PubMed, Embase, PsychInfo and Web of Science were searched. BioRxiv and medRxiv were used to search for any preprints. Terms and their synonyms related to the outcomes, predictive factors based on the DSM-5 neurocognitive domains and patient population were included in the search strategy. Thesauruses like MeSH and Emtree were used besides free-text terms in titles and abstracts. The search strategies for each database can be found in Supplementary Material Part A. Reference lists of articles were scanned for additional suitable studies. Systematic searches were conducted up to July 2020 and assisted by a trained librarian. In April 2022 a second search was performed using the same protocol.
Literature screening was performed in two steps. First, the results of all databases were merged. Duplicates were removed using Rayyan QCRI systematics review app (Ouzzani et al., 2016) and Endnote (EndNote X9, 2013, Clarivate, Philadelphia, PA, USA). Second, two authors (LB and NT) blindly selected relevant studies by screening titles and abstracts based on the eligibility criteria in the same app. In case it was unclear from the title and abstract if an article should be included, the decision was made based on full-text. Any study selection conflict was resolved by discussion between two authors (LB and NT).
After screening all included publications, a custom data extraction form was used for data capturing, which was piloted before data collection commencement. The final form included details relating to study design, participants, eligibility criteria, hearing device, speech perception measurement, cognitive measurement, relation between cognitive measurement and outcome, analysis method, limitations, possible biases and the conclusion of the author.
A total of 5,652 unique articles were retrieved. After screening titles, abstracts of 150 articles remained for full-text screening. Of these 150 articles, 96 were excluded based on reading the full-text. In 26 studies, there was no speech perception outcome reported or used in the relevant analysis (Giraud et al., 2000, 2001a,b; Gfeller et al., 2003; Oba et al., 2013; Berding et al., 2015; Finke et al., 2015; Jorgensen and Messersmith, 2015; Song et al., 2015b; Wang et al., 2015; McKay et al., 2016; Shafiro et al., 2016; Perreau et al., 2017; Amichetti et al., 2018; Butera et al., 2018; Bönitz et al., 2018; Cartocci et al., 2018; Lawrence et al., 2018; Moberly et al., 2018b; Patro and Mendel, 2018, 2020; Dimitrijevic et al., 2019; Chari et al., 2020; Zaltz et al., 2020; Schierholz et al., 2021; Abdel-Latif and Meister, 2022). Twenty-two studies were excluded based on population criteria, studies testing children and adults with prelingual onset of hearing loss (El-Kashlan et al., 2001; Most and Adi-Bensaid, 2001; Lyxell et al., 2003; Rönnberg, 2003; Middlebrooks et al., 2005; Doucet et al., 2006; Heydebrand et al., 2007; Rouger et al., 2007; Hafter, 2010; Li et al., 2013; Lazard et al., 2014; Bisconti et al., 2016; Anderson et al., 2017; Finke et al., 2017; Miller et al., 2017; Moradi et al., 2017; Purdy et al., 2017; McKee et al., 2018; Verhulst et al., 2018; Winn and Moore, 2018; Lee et al., 2019; Smith et al., 2019). In 22 studies, no neurocognitive measure was present (Meyer et al., 2000; Vitevitch et al., 2000; Wable et al., 2000; Giraud and Truy, 2002; Lachs et al., 2002; Lonka et al., 2004; Kelly et al., 2005; Debener et al., 2008; Tremblay et al., 2010; Winn et al., 2013; Moberly et al., 2014; Turgeon et al., 2014; Ramos-Miguel et al., 2015; Collett et al., 2016; Purdy and Kelly, 2016; Sterling Wilkinson Sheffield et al., 2016; Harris et al., 2017; Alemi and Lehmann, 2019; Balkenhol et al., 2020; Crowson et al., 2020; Naples and Berryhill McCarty, 2020; Lee et al., 2021). Fifteen reviews were excluded as they did not include an original study (Wilson et al., 2003, 2011; Mitchell and Maslin, 2007; Peterson et al., 2010; Aggarwal and Green, 2012; Anderson and Kraus, 2013; Lazard et al., 2013; Anderson and Jenkins, 2015; Baskent et al., 2016; Pisoni et al., 2016, 2017; Wallace, 2017; Oxenham, 2018; Bortfeld, 2019; Glennon et al., 2020). Two articles were excluded because they focused on a reversed hypothesis (the influence of CI on cognition) (Anderson and Jenkins, 2015; Nagels et al., 2019) and nine articles were excluded because no abstract and/or full-text paper was available. Fifty-four articles remained after full-text screening. From scanning the references lists of these papers, 28 abstracts were considered. After reading four full-text papers (Lee et al., 2001; Suh et al., 2009; Tao et al., 2014; Wagner et al., 2017), none of the articles were included, leading to 54 included articles (Figure 2A).
The selected articles were grouped into three categories: (1) Studies investigating brain activation patterns in CI users in relation to speech perception performance (N = 18), this includes articles assessing cross-modal activation, (2) Studies investigating performance on cognitive tests in relation to performance on speech perception tests (N = 17), and (3) Studies investigating the use of linguistic skills and information and the relationship with speech perception performance (N = 5). Note that some studies investigated both brain activation and cognitive and linguistic functions (N = 1), or cognitive and linguistic skills (N = 13). Each category of studies will be discussed below. An overview of these studies is shown in Supplementary Tables 1–4.
Three of the 15 studies observed activation patterns during auditory or speech perception, whereas nine focused on cross-modal activation. Three papers used speech imagery tasks preoperatively instead of speech perception tasks. These studies are discussed below. To better understand the brain areas involved, data are visualized in Figure 3.
Figure 3. Regions of the cortex found to be activated in the papers related to speech perception outcomes. +, –, and 0 indicates a positive correlation, negative correlation, or null results, respectively found in an included paper. Accuracy of the depiction depends on accuracy of the reports, neuroimaging and analysis technique used in the papers. Top: left hemisphere, Bottom: right hemisphere. (A) The parts of the cortex found to be activated during auditory perception related to speech perception outcomes. Blue areas are found to be positively correlated and orange areas negative. (B) The parts of the cortex found to be activated during auditory perception, and visual perception. Blue areas are found to be mostly positively correlated and orange areas mostly negative. Yellow areas show conflicting results. The right amygdala (+), cingulate sulcus (+) and bilateral thalami (–) are not depicted because they are not located on the outside cortex. (C) The parts of the cortex found to be activated during speech imagery tasks preoperatively. Blue areas are found to be positively correlated and orange areas negative. Yellow areas show conflicting results. Since most of this data is from the same participant group, no signs are used to indicate findings per paper. Left and right medial temporal lobes including hippocampal gyrus are not depicted because they are not located on the outside cortex. (D) The lobes of the cortex (frontal, parietal, temporal, and occipital lobe) and the cerebellum. This image can be used as a guidance to read the text and interpret part (A–C) of the figure. The outline of the brain was drawn by Patrick J. Lynch, medical illustrator and C. Carl Jaffe, MD, cardiologist, https://creativecommons.org/licenses/by/2.5/.
Three studies divided their participants into better and poorer performers based on speech perception performance and explored the differences in brain activation while listening. These are Mortensen et al. (2006); Suh et al. (2015), Kessler et al. (2020) (see Table 1 for an overview) and are summarized below:
First, Mortensen and colleagues showed alternative patterns of activation between better performers (96–100% word score in quiet) and poorer performers (<60% word score in quiet), while listening passively to a range of speech and non-speech stimuli. Better performers showed increased activity in the left inferior prefrontal, left and right anterior and posterior temporal cortex (auditory cortex), and the right cerebellum. Poorer performers only showed increased activity in the left temporal areas (p < 0.05) (Mortensen et al., 2006).
Second, Suh et al. (2015) measured preoperative brain activation during listening to noise and compared the results of a group of postoperative poorer and better (cutoff: 80%-word score) performers. Participants with higher activity in the inferior temporal gyrus and premotor areas (part of frontal cortex) became better performers (p = 0.005), and participants with higher activation in the occipital lobe (visual cortex) became poorer performers (p = 0.01) (Suh et al., 2015).
In the third study, Kessler et al. (2020), examined brain activation during a speech discrimination task consisting of correct and incorrect sentences. When dividing the group of participants into better and poorer performers [median split with cutoff +7.6 dB Signal to Noise Ratio (SNR) on a sentence test in noise], better performers showed significantly higher activity in the right parietal and temporal area and left occipital area (p < 0.001, uncorrected for multiple comparisons), and poorer performers significantly higher activation in the superior frontal areas (p < 0.001, uncorrected for multiple comparisons). Activity during resting state revealed that poorer performers had a higher activity in the right motor and premotor cortex and right parietal cortex, whereas better performers had higher activity in the left hippocampal area, left inferior frontal areas and left inferior temporal cortex (p < 0.001, uncorrected for multiple comparisons). The differences in activity between better and poorer performers in the bilateral temporal, frontal, parietal, and bilateral motor cortex were significantly positively correlated with performance on a monosyllabic word test and the MWT-B verbal intelligence test (p > 0.001, uncorrected and p < 0.05 FWE). There were also small positive correlations between this activity in the left temporal, parietal and occipital regions with working memory span scores, and activity in the left temporal lobe with a verbal learning task (only in testing without correction for multiple comparisons and not in tests including FWE) (Kessler et al., 2020).
In individuals with hearing loss cross-modal activation occurs when two things are at play. (1) The visual cortex is involved in auditory perception. (2) The auditory cortex is also recruited and used to process visual stimuli instead of or in addition to auditory stimuli to understand speech (Bavelier and Neville, 2002). Several studies have investigated whether such reorganization occurs in postlingually deaf participants and whether it is related to postoperative speech perception performance, as this reorganization might limit these areas to return to their original functioning (see Table 2 for an overview). These studies are summarized below (* indicates whether the study reported sufficient power):
Six of the ten studies observed activation in the temporal lobe (auditory cortex) in response to visual stimuli and activation in the occipital lobe (visual cortex) in response to auditory stimuli [they used ROI (Regions Of Interest)] (Buckley and Tobey, 2010; Sandmann et al., 2012; Chen et al., 2016, 2017; Kim et al., 2016; Zhou et al., 2018). Buckley and Tobey (2010) did not find any significant correlation between activation in the temporal lobe in response to visual stimuli and word and sentence perception in noise (r = 0.1618, p = 0.6155*). On the contrary, Sandmann et al. (2012) did find activation in the right temporal cortex evoked by visual stimuli to significantly negatively correlate with word perception in quiet (r = –0.75, p < 0.05) and positively correlate with sentence perception in noise (r = 0.72, p < 0.05). Kim et al. (2016), also found that better performers (>60% word score in quiet) showed a significantly smaller P1 amplitude in response to visual stimuli compared to poorer performers (<40% word score) (p = 0.002*). Additionally, better performers showed larger P1 amplitudes in the occipital cortex (p = 0.013*). Both effects showed a correlation with a word intelligibility test (occipital: r = 0.755, p = 0.001; temporal: r = –0.736, p = 0.003*) (Kim et al., 2016). Zhou et al. (2018) confirmed these results and found a significant negative correlation between temporal cortex activation and word perception in quiet and sentences in quiet and noise (r = –0.668, p = 0.009). Along the same lines, correlations to sentence perception in quiet and noise revealed a higher activation in the visual cortex to be positively correlated, as opposed to higher activation in the auditory cortex induced by visual stimuli (r = 0.518, p = 0.027). It was found that if the beneficial activation in the visual cortex was higher than the activation in the auditory cortex induced by visual stimuli, speech perception was better (Chen et al., 2016). A follow-up analysis calculated the correlations of the continuous input streams of the different areas. It was found that CI users with significantly higher connectivity for auditory than visual stimuli performed better on a word perception test in quiet (r = 0.525, p = 0.021), but no correlation was found for a sentence perception test in quiet or noise. This might have facilitated auditory speech perception learning processes by supporting visual cues, such as lip reading (Chen et al., 2017).
Three out of ten studies analyzed whole brain activation in response to auditory, visual and audiovisual stimuli (Strelnikov et al., 2013; Song et al., 2015a; Layer et al., 2022). Strelnikov et al. (2013) also found significant negative correlations of temporal lobe activity with word perception in quiet (rest: r = 0.9, visual: r = 0.77, audiovisual: r = 0.7, p < 0.05) and positive correlations with posterior temporal cortex and occipital lobe activation (rest: r = 0.9, visual: r = 0.8, audiovisual: r = 0.5, p < 0.05). However, Song et al. (2015a) found a negative correlation between occipital lobe activation and word perception in quiet (left: rho = –0.826, p = 0.013, right: rho = –0.777, p = 0.019). Similarly, Layer et al. (2022) did not find a correlation between activation in the left temporal cortex in response to audiovisual stimuli with word perception in quiet (r = 0.27, p = 0.29). While the whole brain was observed in these studies, Strelnikov et al. (2013) found activation in the inferior frontal area to be positively correlated with word perception in quiet (rest: r = 0.809, visual: r = 0.77, audiovisual: r = 0.90, p < 0.05). This is in line with results from the previous section “3.1.1 Brain responses to auditory stimuli”. Song et al. (2015a) also observed activation in the right amygdala to be positively correlated with word perception in quiet (rho = –0.888, p = 0.008).
Lastly, one paper by Han et al. (2019) measured activity pre-implantation and found a significant negative correlation between activity in the superior occipital gyrus and postoperative word score in quiet (r = –0.538, p < 0.001), as well as a positive correlation with the dorsolateral and dorsomedial frontal cortex (r = 0.595, p > 0.001). No significant correlation was found with activity in the auditory pathway areas, the inferior colliculus, and the bilateral superior temporal gyrus.
Another way to consider cortical reorganization, focusing more on altered cortical structure than brain activity, is analyzing gray matter probabilities using Magnetic Resonance Imaging (MRI) pre-implantation. Researchers found that gray matter probability in the left superior middle temporal cortex (r = 0.42) and bilateral thalamus (r = –0.049, p < 0.05) significantly predicted postoperative word recognition in quiet (Sun et al., 2021). Similarly, Knopke et al. (2021) demonstrated that white matter lesions (captured using the Fazekas Score) predicted word perception scores in quiet after implantation in 50–70 year-old CI users, but not in older users. The white matter score explained 27.4% of the speech perception variance in quiet (p < 0.05, df = 24 and 21), but was not replicated for a sentence test in noise.
A group of studies by Lazard et al. (2010, 2011) and Lazard and Giraud (2017) used “mental auditory tasks” to overcome the negative impact of hearing impairment pre-implantation. The tasks involved imagining words or sounds without auditory input. It was hypothesized that performance on these tasks would involve brain areas similar to the ones involved in auditory processing and therefore show good correlations with speech perception outcomes postoperatively. These studies are summarised below (see Table 3 for an overview):
Lazard et al. (2010) found preoperative imaging data can be used to distinguish future better (>70% word score in quiet) and poorer (<50% word score in quiet) performers based on a rhyming task recruiting phonological strategies during reading. Better performers relied on a dorsal phonological route (dynamic stimulus combination) during a written rhyming task, while poorer performers involved a ventral temporo-frontal route (global) and additionally recruited the right supramarginal gyrus. More specifically, they found a significant positive correlation between brain activation during the phonological task and post-CI word recognition in quiet in the left frontal, parietal, posterior temporal and bilateral occipital cortices. A negative correlation was found in the bilateral anterior temporal, inferior frontal cortex and right supramarginal gyrus. This indicates that poorer performers rely more on semantic information, bypassing the phoneme identification and better performers rely more on visual input (P < 0.001 uncorrected).
The same research group correlated preoperative imaging data measured during an auditory imagery task with post-CI word scores in quiet. This showed a decline in activity in the dorsal and frontoparietal cortex and an increase in the ventral cortical regions, right anterior temporal pole and hippocampal gyrus. Activation levels of the right posterior temporal cortex and the left insula were not significantly correlated, but activation levels of the inferior frontal gyrus were positively correlated with word scores in quiet (r = 0.94, p = 0.0001) (Figure 3C; Lazard et al., 2011).
Lastly, Lazard and Giraud (2017) used a visual phonological rhyming task, including non-words that are pronounced as words, and measured brain activity preoperatively. They correlated this with postoperative word scores in quiet and found that response time on the task (r = 0.60, p = 0.008) and reorganized connectivity across the bilateral visual, right superior temporal sulcus and the left superior parietal cortex/postcentral gyrus correlated significantly with poorer CI performance (p < 0.001). Slower response times were associated with increased activity in the frontoparietal regions and better CI performance. Based on these papers, the group of Lazard concluded that poorer performers use more semantic concepts of sounds instead of phoneme identification, even when not confronted with auditory input. Better performers seemed to be able to utilize additional visual input to support speech perception, as also seen in the studies investigating cross-modal plasticity.
In this review, 31 studies used cognitive tests to assess one or more neurocognitive function(s) and related these outcomes to speech perception outcomes. The studies are described below. Table 4 summarizes time, type of speech perception measurements and related cognitive domain of the papers. Additionally, the sample size and whether a power analysis is reported are noted down. Note that most studies performed cognitive testing postoperatively. If a study performed cognitive assessment preoperatively this will be explicitly mentioned. All speech perception measures were performed postoperatively.
Three of the included papers used general (diagnostic) cognitive measures, not specifying which of the cognitive domains were measured by the task. These four more clinical tests, mostly used to detect early signs of Dementia (see Table 5a for an overview), are: (1) The Mini-Mental State Examination (MMSE) which did not significantly correlate with word perception in quiet (r2 = 0.061, p = 0.280, N = 15) (Zucca et al., 2022). (2) The Self-Administered Gerocognitive Examination (SAGE), where preoperative screening of cognitive functions significantly positively correlated with word recognition in quiet [r2(32) = 0.1955, p = 0.0025] and sentence perception in quiet [r2(32) = 0.1564, p = 0.0067] and noise [r2(32) = 0.1543, p = 0.007] (Wazen et al., 2020). (3) The Montreal Cognitive Assessment (MoCA), which was included in a multivariate model explaining variance in sentence perception performance in noise. Together with neuronal health measures, MoCA explained 64.5% of this variance (β = 2.06, p < 0.05, df = 29) (Walia et al., 2022). (4) The clock drawing test, a short subtest of SAGE, which did not show a significant relationship with word perception in quiet (r2 = 0.177, p = 0.058, N = 15) (Zucca et al., 2022).
Table 5. Overview of included papers (a) using general cognitive measures and (b) studying complex attention.
Attention is a state of selective awareness by which a single stimulus is selected for enhanced processing. Tasks assessing attention mainly involve target selection. For the papers included in this review, the “Leiter-3 sustained attention task,” “Woodcock-Johnson IV (WJ-IV) letter and number pattern matching task and the pair cancelation task”, and the “ALAcog M3 attentional task” are used (see Table 5b for an overview). These tests involve targets like figures, letters, numbers or repeated patterns on paper among a set of distractors (Moberly et al., 2016b, 2017b; Hillyer et al., 2019; Völter et al., 2021). Of these tasks, only performance on the ALAcog attentional task was significantly different between better and poorer performers on a word test in quiet (Cohen’s d = 1.12, p = 0.003) (Völter et al., 2021). The other tests showed no significant relationship with sentences in quiet or noise (Moberly et al., 2016b, 2017b; Hillyer et al., 2019).
Another task used in several studies to investigate attention is the Trail Making Task B (TMT-B). For this task the participant has to draw a “trail” of consecutive numbers and letters: 1-A-2-B-3-C etc. Across the studies that this task was used in, the results were inconsistent showing both significant negative and positive correlations with words in quiet (WQ: CI only: r = –0.52, p < 0.05, corrected for age: r = –0.53, p < 0.05, bimodal r = 0.75, p > 0.01 corrected for age: r = –0.67, p < 0.01; Cohen’s d = 0.96, p = 0.018) and positive (r = 0.55, p > 0.05) or non-significant correlations (when measured preoperatively: p = 0.087) with sentences in noise (Hua et al., 2017; Völter et al., 2021; Zucca et al., 2022).
Processing speed is the time required to complete a mental task (Kail and Salthouse, 1994). To test this, one task is used that also assesses attention: the “WJ-IV letter and number pattern matching task and the pair cancelation task.” Furthermore, the “Wechsler Adult Intelligence Scale III (WAIS-III) symbol search.” the “NIH toolbox pattern comparison processing speed test”, the “WAIS-III coding test” and the “WJ-IV numbers reversed test” are used (see Table 5b for an overview) (Hillyer et al., 2019; Mussoi and Brown, 2019; Tinnemore et al., 2020). Of all of these tests only the pattern comparison test showed a significant relationship with sentences in quiet (p = 0.006) (Tinnemore et al., 2020).
Another task used to measure processing speed is the TMT-A. For this task the participant has to draw a trail from 1 to 25. When measured preoperatively, performance on TMT-A was a significant factor in regression analysis for words in quiet postoperatively (r2 = 0.236, p = 0.035) (Zucca et al., 2022). However, when TMT-A was measured postoperatively, performance was in general not significantly related to perception of words in quiet or sentences in noise (WQ: CI only non-significant r = –0.27, Bimodal r = –0.60, p > 0.05, corrected for age non-significant r = –0.48, SN r = 0.19; Cohen’s d = 0.8, p = 0.053) (Hua et al., 2017; Völter et al., 2021).
Non-verbal intelligence is an executive function that relates to thinking skills and problem-solving abilities that do not require language. “Ravens progressive matrices task,” the “WAIS-III matrix reasoning test,” “Leiter-3 visual pattern task,” “Test of Non-verbal Intelligence–3 (TONI-3) pointing to pictures” and the “Leiter-3 figure ground and form completion” were used to measure this (see Table 6 for an overview).
In this review, the Ravens task is used most frequently to measure non-verbal intelligence (Moberly et al., 2017c, 2018a,c, 2021; Mattingly et al., 2018; Pisoni et al., 2018; Moberly and Reed, 2019; O’Neill et al., 2019; Skidmore et al., 2020; Zhan et al., 2020; Tamati and Moberly, 2021; Tamati et al., 2021). The task is to pick the piece that fits within the pattern of a visual geometric matrix. A significant relationship between performance on the Ravens task and word perception in quiet was found in five out of six included papers (r = 0.35, p < 0.05; r2 = 0.325, p < 0.001; r2 = 0.64, p < 0.05; r = 0.196, p = 0.421) (Moberly et al., 2018a,c, 2021; Pisoni et al., 2018; Zhan et al., 2020; Tamati et al., 2021). However, it should be noted, that in a study by Tamati and Moberly (2021) this positive correlation was found after 10 trials of word perception, when the listener was adapted to the talker (r = 0.68, p = 0.009, df = 10). In a study by Moberly et al. (2021) this positive correlation was found for participants with low auditory sensitivity (r = 0.52, p = 0.02) (where auditory sensitivity was determined by Spectral-Temporally Modulated Ripple Test performance). Eight out of ten studies (some using the same group of participants) using this task reported a significant relationship between performance and sentence perception in quiet [r(26) = 0.53, p < 0.01; PRESTO words: r = 0.45, p < 0.01; r = 0.62, p < 0.05; r = 0.41, p < 0.01, and sentences: r = 0.47, p < 0.01; r = 0.68, p < 0.05; r = 0.47, p < 0.01; Harvard words: r = 0.71, p < 0.05; r = 0.35, p < 0.05 and sentences r = 0.39, p < 0.05; r = 0.60, p < 0.05; r = 0.46, p < 0.01; Adding Ravens score to a blockwise multiple linear regression analysis to predict anomalous sentences p = 0.08, df = 32] (Moberly et al., 2017c, 2018a,b, 2021; Mattingly et al., 2018; Pisoni et al., 2018; Moberly and Reed, 2019; O’Neill et al., 2019; Skidmore et al., 2020; Zhan et al., 2020). For one of these studies, Ravens task performance discriminated highly between two groups of better and poorer performers on sentences in quiet (Matrix coefficient = 0.35, rank 2, df = 10) (Tamati et al., 2020). In another study, Moberly et al. (2021) found that there was only a significant positive correlation between Ravens score and sentence perception in participants with high auditory sensitivity (r = 0.52, p = 0.01). Lastly, in another paper they found that there was only a predictive value of Ravens score with anomalous sentences and not meaningful sentences (p = 0.008, df = 32) (Moberly and Reed, 2019). The other tasks used to assess non-verbal intelligence did not show any significant results when related to speech perception performance (r = –0.16 to 0.33) (Collison et al., 2004; Holden et al., 2013; Moberly et al., 2016b).
Working memory is a buffer that holds memories accessible while a task is performed (Breedlove and Watson, 2013). It has been suggested that a linear relationship exists between the ambiguity of the speech stimulus and the working memory capacity needed, to decide what words were perceived (Rönnberg, 2003). Working memory can be assessed in different ways and using different modalities; visual (see Table 7 for an overview), auditory, audio-visual and verbal (see Table 8 for an overview).
The “visual digit span task,” “Leiter-3 forward and reversed memory test, letters, and symbols,” “ALAcog 2-back test” and “Operation Span” (OSPAN) are used to assess visual working memory (Moberly et al., 2016b, 2017c, 2018c, 2021; Mattingly et al., 2018; Hillyer et al., 2019; Moberly and Reed, 2019; Skidmore et al., 2020; Tamati et al., 2020; Zhan et al., 2020; Tamati and Moberly, 2021; Völter et al., 2021; Luo et al., 2022).
The most used in the included literature is the visual digit span. Scores on this task showed no significant correlations with word perception in quiet (r = –0.14 to 0.448, p = 0.32–0.704) (Moberly et al., 2018a,c, 2021; Pisoni et al., 2018; Skidmore et al., 2020; Zhan et al., 2020; Tamati and Moberly, 2021). For sentence perception in quiet and noise, three out of nine papers found a significant correlation (Moberly et al., 2017c, 2018a,c, 2021; Hillyer et al., 2019; Moberly and Reed, 2019; Skidmore et al., 2020; Tamati et al., 2020; Zhan et al., 2020). More specifically, Moberly et al. (2017c) found a positive correlation with one of two sentence perception tasks in quiet [r(26) = 0.40, p = 0.035] and Hillyer et al. (2019) a positive correlation when corrected for age (r = 0.539, p = 0.016). Furthermore, digit span did not significantly discriminate between better and poorer performers on sentence perception in quiet (Matrix coefficient = 0.00, rank 10, df = 10) (Tamati et al., 2020). Lastly, Moberly et al. (2021), found a positive correlation with one of two sentence perception tasks in quiet for participants with an intermediate degree of auditory sensitivity (rho = 0.49, p = 0.03).
For similar span tests using pictures or objects, like in the forward and reversed memory test, letters, and symbols, performance showed a significant relationship with word and sentence perception in quiet and noise in one of seven papers (Moberly et al., 2016b, 2017c; Hillyer et al., 2019; Skidmore et al., 2020; Zhan et al., 2020; Luo et al., 2022). This paper showed a positive relationship between symbol and object span measured preoperatively and word perception in quiet and sentence perception in quiet and noise postoperatively (words: r = 0.599, p = 0.007, sentences in quiet r = 0.504, p = 0.028 and noise: r = 0.486, p = 0.035) (Zhan et al., 2020).
Lastly, the OPSAN and 2-back task were applied in one paper each. Performance on the 2-back did not differ significantly between better and poorer performers on a word task in quiet (Cohen’s d = 0.5, p = 0.22), but the OSPAN score was significantly worse for poorer performers (Cohen’s d = 1.01, p = 0.0068) (Völter et al., 2021).
Similar tasks are used to measure working memory capacity with auditory stimuli instead of visual stimuli (Table 8). The digit span task is used in four of the included studies (r = –0.27, p = 0.35) (Holden et al., 2013; Moberly et al., 2017a; Bosen et al., 2021; Luo et al., 2022). Scores on these tasks were not significantly correlated to words nor sentences in quiet and noise when administered preimplantation (measured once) or postimplantation (Holden et al., 2013; Moberly et al., 2017b). Only one paper reported a significant correlation with sentence perception in quiet, but this effect disappeared when corrected for auditory sensitivity based on spectral or temporal resolution thresholds (r = 0.51, p = 0.03, after correcting for auditory resolution: r = 0.39, p = 0.08) (Bosen et al., 2021).
Lastly, stimuli can be presented in the auditory and visual modality at the same time (Hillyer et al., 2019; Mussoi and Brown, 2019; Zucca et al., 2022; Table 8). The audiovisual digit span test was applied in three included studies, with no significant results when related to words in quiet (e.g., when measured preoperatively forward: r2 = 0.003, p = 0.826, backward: r2 = 0.036, p = 0.410), but a significant positive correlation with sentences in quiet (r = 0.539 p = 0.016) and noise (r = 0.573, p = 0.018) (Hillyer et al., 2019; Mussoi and Brown, 2019; Zucca et al., 2022).
Interestingly, Luo et al. (2022) used a cued modality working memory task, where participants needed to remember auditory digits and visual letters and recall one of them or both. In the cued conditions they were instructed beforehand what they needed to recall after the stimuli were presented, but no instruction was given in the uncued condition. They found that for both the cued and uncued auditory condition, the score on the task was significantly negatively correlated with sentence (cued: r = –0.66, p = 0.01, uncued: r = –0.54, p = 0.045) and word perception in noise (cued: r = –0.54, p = 0.047, uncued: r = –0.60, p = 0.02). However, after correcting for spectral and temporal resolution, only the significant negative correlation between auditory uncued performance on the working memory task and word perception in noise remained (r = –0.65, p = 0.03). The authors suggest this might be because the same underlying strategies are used, and because top-down correction using semantic information is not possible, unlike for sentence perception and cued working memory. However, this paper had small sample sizes and results should be interpreted with caution (Luo et al., 2022).
Other working memory tasks involve more language perception skills. These measures are thought to assess verbal working memory more specifically, using both auditory and visual stimuli. Examples of such tasks are the “Reading Span task,” the “Size Comparison Span” (SicSpan) task and the “Listening span task” (Table 8; Finke et al., 2016; Hua et al., 2017; Kaandorp et al., 2017; Moberly et al., 2017b; Dingemanse and Goedegebure, 2019; O’Neill et al., 2019; Kessler et al., 2020). For the Reading or Listening span, participants must decide for each sentence they see or hear whether the sentence is semantically true or false, while retaining items that are presented in memory and recalling them after the true/false task.
Reading span was used most frequently in the included papers. For these studies, performance did not significantly correlate with word perception scores in quiet and noise in three papers (Kaandorp et al., 2017; Dingemanse and Goedegebure, 2019; Luo et al., 2022). A significant positive correlation was, however, found for bimodal listening to words in quiet (r = 0.71, p > 0.01) (Hua et al., 2017). In two out of six included papers there was a significant positive correlation between Reading span and sentence perception in quiet or noise (r = 0.430, p = 0.018; r = 0.38, p = 0.009) (Hua et al., 2017; Moberly et al., 2017b; Dingemanse and Goedegebure, 2019; O’Neill et al., 2019; Luo et al., 2022) and one paper showed a significant negative correlation (r = –0.57, p = 0.009) (Kaandorp et al., 2017).
Furthermore, only one paper implemented the Listening span and found performance to be significantly positively correlated with sentence perception in quiet and noise (quiet: r = 0.64, non-word quiet: r = 0.68, noise: r = 0.57, p < 0.01) (Moberly et al., 2017b). For SicSpan the two included studies found no significant relationship with sentence or word perception in quiet and noise (Finke et al., 2016; Kessler et al., 2020).
Cognitive inhibition is the ability to suppress goal-irrelevant information. For example, being able to ignore background noise or lexical competitors. In the included papers two tasks were used to assess inhibitory control: the “Flanker task” and the “Stroop task” (see Table 9 for an overview). Both tasks contain congruent, incongruent, and neutral conditions. In the congruent condition, the participant must respond to a target where the rest of the properties of the trial are aligned with the required response. In the incongruent condition, the participant must respond to a target where the rest of the properties of the trial are opposite to the required response and in the neutral condition the rest of properties of the trial do not have the ability to evoke a response conflict (Zelazo et al., 2014; Knight and Heinrich, 2017).
Eleven included studies used the Stroop task, where response time was measured, and a lower value represented better performance on the task. Four of four studies show that performance on this task, both preoperatively and postoperatively, did not significantly correlate with or predict word perception in quiet (Moberly et al., 2018c; Skidmore et al., 2020; Zhan et al., 2020). Two exceptions exist which showed a significant negative correlation with word perception in quiet: (1) in a group having high auditory sensitivity (rho = –0.49, p = 0.02) (Moberly et al., 2021) (2) with word perception after ten trials of a task, when the listener has adapted to the speech (r = –0.50, p = 0.044) (Tamati and Moberly, 2021). Three of seven studies showed a significant negative relation with sentence perception tests in quiet (adding Stroop to the model to predict meaningful SQ p = 0.008, β = –0.259; r = –0.43, p < 0.05; r = –0.41, p < 0.05) (Moberly et al., 2016b, 2017b, 2018a,c; Moberly and Reed, 2019; Tamati et al., 2020; Zhan et al., 2020). Additionally, a significant negative correlation was found between Stroop task performance and sentence perception in quiet, for a group having high auditory sensitivity (rho = –0.047, p = 0.03) (Moberly et al., 2021). Furthermore, preoperative Stroop was significantly negatively correlated with postoperative sentence perception in quiet and noise (AzBio: Q r = –0.484, p = 0.042, N r = –0.412, p = 0.09, Harvard: standard r = –0.321, p = 0.193, anomalous r = –0.319, p = 0.197, PRESTO r = –0.301, p = 0.224) (Zhan et al., 2020) (Note that many of these studies including the Stroop task were performed in the same lab, some using the same participants, which might hamper generalizability).
Additionally, two included studies used the Flanker task. They showed that performance on this task significantly differed between better and poorer performers on a word task in quiet (Cohen’s d = 0.58, p = 0.037) (Völter et al., 2021). However, the performance did not significantly predict performance for sentence perception in quiet (Tinnemore et al., 2020).
Flexibility, often referred to as executive control, encompasses functions related to planning and task switching. It is mostly found to be supported by the frontal lobe (Gilbert and Burgess, 2007), which seems to be more activated in better performers. The TMT-B is not only used to measure attention, but also executive control or flexibility. Additionally, the “NIH Toolbox Dimensional Change Card Sort Test” (DCCS) is used (see Table 9 for an overview). As discussed before, the TMT-B score showed inconsistent correlations with word or sentence perception in quiet (Hua et al., 2017; Völter et al., 2021; Zucca et al., 2022). The other task was only applied in one paper. Performance on the DCCS, which asks participants to match cards with a target card based on different properties, was found to be significant in a general linear model with sentences in quiet (p = 0.006) (Tinnemore et al., 2020).
None of the included studies contained measures of social cognition related to speech perception outcomes.
Memory is the ability to store learned information and retrieve it over time. In the brain activation section, it was observed that the temporal and frontal cortex are recruited in better performers. Those areas are mainly involved in memory formation, and together with brain plasticity indicate learning (Breedlove and Watson, 2013). These skills can be measured in different ways. In the included papers this is done using recall tasks (Moberly et al., 2017a; Hillyer et al., 2019; Völter et al., 2021), learning tasks (Zucca et al., 2022), or both (Holden et al., 2013; Pisoni et al., 2018; Kessler et al., 2020; Skidmore et al., 2020; Tamati et al., 2020; Ray et al., 2022), mostly in the verbal domain (see Table 10a for an overview).
In five papers recall tasks were used (Moberly et al., 2017a; Hillyer et al., 2019; Kessler et al., 2020; Völter et al., 2021; Zucca et al., 2022). Performance scores did not significantly correlate with, predict or dissociate better and poorer performers on sentence perception in quiet or noise. However, there was a significant difference in the “ALAcog delay recall score” between CI users that had higher and lower performance on a word perception task (Cohen’s d = 0.88, p = 0.04) (Völter et al., 2021) as opposed to Zucca et al. (2022) who did not find a significant result when it was measured preoperatively (p = 0.343, p = 0.445).
Furthermore, the CVLT test battery was used in five included papers to assess verbal learning and recall (Holden et al., 2013; Pisoni et al., 2018; Skidmore et al., 2020; Tamati et al., 2020; Ray et al., 2022). The CVLT includes various short- and long-term recall tasks, and calculates several scores reflecting word recall strategies. In two out of five papers, scores on this task did not significantly correlate with word and sentence perception in quiet or noise when administered pre- or post-implantation (Holden et al., 2013; Pisoni et al., 2018; Skidmore et al., 2020). If a relationship was found, it was always a subtest of the battery. In these studies, (1) recall on list B, was positively correlated with words and sentence perception in quiet (WQ: r = 0.47 SQ: r = 0.56, r = 0.52, P < 0.05) (Pisoni et al., 2018), (2) sub-scores short delay cued recall, semantic clustering, subjective clustering, primacy recall and recall consistency were important predictors of sentence perception in quiet and noise (Ray et al., 2022) and (3) list B and Y/N discriminability could discriminate very little between better and poorer performers on a sentences in quiet task, and T1/T4 not at all (Tamati et al., 2020).
Perceptual motor skills allow individuals to interact with the environment by combining the use of senses and motor skills. These skills are involved in many of the tasks discussed above. Only two papers used tasks that explicitly measure these skills (see Table 10b for an overview) (Hillyer et al., 2019; Zucca et al., 2022). Tasks used to measure this skill are the “WJ-IV visualization parts A and B,” the “corsi block tapping test” and the “block rotation task.” These tasks were only applied in one paper each and did not show any significant relation with speech perception performance (e.g., r = 0.081–0.103, p = 0.156–0.588) (Hillyer et al., 2019; Zucca et al., 2022).
Many of the cognitive tasks mentioned above already include verbal ability assessments, for example verbal working memory, learning and recall. Although these different cognitive functions do not seem to correlate consistently with speech perception outcomes, it is valuable to explore what the included literature says about language skills in CI users and the relationship with speech perception outcomes. An overview of the studies including language assessments can be found in Table 4.
Vocabulary is the language user’s knowledge of words. In the included papers vocabulary is assessed by picture naming tasks (Collison et al., 2004; Holden et al., 2013; Kaandorp et al., 2015; Völter et al., 2021), choosing synonym tasks (Collison et al., 2004; Kaandorp et al., 2015, 2017), discriminating real words from pseudowords (Kaandorp et al., 2017; Moberly et al., 2017a; Kessler et al., 2020; Tamati et al., 2020; Völter et al., 2021), by reporting the degree of familiarity with words (Pisoni et al., 2018; Skidmore et al., 2020; Bosen et al., 2021; Tamati et al., 2021), or describing the similarity or difference between two words (Holden et al., 2013) (see Table 11 for an overview). In three out of ten papers there was an indication of a relationship between vocabulary and speech perception outcomes. First, there was a difference in score on the Rapid Automatic Naming (RAN) task and a lexical decision task between a group of poorer and better performing CI users on a word perception task in quiet, where better performers had significantly higher RAN task scores (Cohen’s d = –0.82 to –1.34, p = 0.0021–0.031) and non-word discrimination task scores (Cohen’s d = –1.27, p = 0.0021) (Völter et al., 2021). Secondly, performance on a lexical decision task was found to be a significant predictor of the average score of both word and sentence perception in noise (r = 0.45, p = 0.047) (Kaandorp et al., 2017). Third, there was a significant correlation between performance on a word recognition test and sentences in quiet (r = 0.45, p < 0.05) (Pisoni et al., 2018).
Verbal fluency is the readiness in which words are accessed and produced from one’s own long-term lexical knowledge. Four of the papers address verbal fluency (Finke et al., 2016; Kessler et al., 2020; Völter et al., 2021; Zucca et al., 2022) (see Table 11 for an overview). Performance on the verbal fluency tasks was assessed in four papers. In three out of four no significant relationship with word and sentence perception in quiet was found (Finke et al., 2016; Kessler et al., 2020; Zucca et al., 2022). Performance did significantly differ between better and poorer performers on a word perception task in quiet (Cohen’s d = 0.8, p = 0.025) (Völter et al., 2021).
Speed of lexical and phonological access represents how fast written text is generated into phonemes or meaningful speech. Speed of lexical and phonological access is assessed in ten of the included papers. Speed reading tasks of real and non-words and sentences, such as the “Test Of Word Reading Efficiency” (TOWRE) and the “Wide Range Achievement Test” (WRAT), are mostly used for this (Hay-McCutcheon et al., 2005; Moberly et al., 2018a,c, 2021; Pisoni et al., 2018; Moberly and Reed, 2019; Skidmore et al., 2020; Tamati et al., 2020, 2021) (see Table 12 for an overview).
Table 12. Overview of included papers studying speed of lexical and phonological access and degraded receptive language.
In eight papers, TOWRE was used. Participants had to read aloud as many words or non-words in a list in 45 seconds for this task. Some studies included the same participants, therefore, in three out of five study populations there were significant positive correlations between performance on the TOWRE word and non-word scores and word and sentence perception in quiet (r = 0.41–0.49, p < 0.05; adding model to predict anomalous sentences p = 0.010, df = 32) (Moberly et al., 2018a,c, 2021; Pisoni et al., 2018; Moberly and Reed, 2019; Skidmore et al., 2020; Tamati et al., 2021). More specifically, when performing a regression analysis, Moberly et al. (2018a) found that only TOWRE word and non-word score was related to word and sentence perception in quiet (WQ: r2 = 0.312, p = 0.001, non-words: r2 = 0.173, p = 0.014, SQ: Harvard: r2 = 0.175, p = 0.015, PRESTO: r2 = 0.187, p = 0.011). Lastly, Moberly et al. (2021), found a significant positive correlation with word perception in quiet for participants with an intermediate and high degree of auditory sensitivity (intermediate: rho = 0.48, p = 0.03, high: rho = 0.42, p = 0.05).
The remaining tasks used to study speed of lexical and phonological access: the WRAT, “preoperative speechreading of sentences,” “Lexical Model Oriented (LEMO) subtest of internal homophonic word reading” and non-word repetition task were only applied in one study each. The results varied and showed significant positive (Cohen’s d = –1.23, p = 0.0039) (Völter et al., 2021), negative (r = –0.872, p = 0.002) (Hay-McCutcheon et al., 2005) and non-significant relationships (Moberly et al., 2017a; Skidmore et al., 2020).
Degraded receptive language is captured with the Text Reception Threshold (TRT) task. This is a visual analog of the Speech Reception Threshold (SRT) task, where sentences are masked using different visual patterns. The participant needs to try and read the sentences and is scored based on the degree of masking at which they are able to repeat 50% of the words correctly. This task was used in three included studies (Haumann et al., 2012; Kaandorp et al., 2017; Völter et al., 2021) (see Table 12 for an overview). In one of the three papers the TRT was measured preoperatively (Haumann et al., 2012). Results of these papers assessing performance the TRT and a very similar fragmented sentences test task were highly variable: both non-significant results (Kaandorp et al., 2017), significantly positive (Cohen’s d = –0.94 to 1.57, p = 0.0021–0.0002; r2 = 0.157, p < 0.001) (Moberly et al., 2018c; Völter et al., 2021) and negative relations (SN modulated: TRT random dots r = –0.23, r2 = 0.05, p = 0.036, TRT random bars r = –0.27, r2 = 0.07, p = 0.012, SN modulated: TRT random dots r = –0.29, r2 = 0.09, p = 0.007, TRT random bars r = –0.28, r2 = 0.08, p = 0.009) (Haumann et al., 2012) were found.
This scoping review aimed to provide a comprehensive overview of the current literature on the relationship between both neurocognitive factors and brain activation patterns, with speech perception outcomes in postlingually deafened adult CI users. Fifty-four papers were included and divided into three categories: (1) literature discussing different brain activation patterns in better and poorer CI performers, (2) literature relating performance on cognitive tasks to speech perception outcomes, and (3) literature relating performance on cognitive language tasks to speech perception outcomes.
Overall, literature studying brain activation patterns in CI listeners demonstrated that better performers in quiet or noise showed increased activation in the left frontal areas and temporal cortex when passively listening to noise, speech and non-speech stimuli, and actively to semantically correct and incorrect sentences. The frontal lobe is thought to be involved in several speech-related functions, such as semantic generation, decision making and short-term memory (Mortensen et al., 2006; Strelnikov et al., 2013), while the temporal cortex is the main hub for auditory and speech processing. However, activity in the premotor cortex and parietal cortex showed less consistent links with performance. These areas are involved in planning movement and spatial attention, respectively (Breedlove and Watson, 2013).
Moreover, cross-modal activation in the visual occipital cortex during speech perception was seen in better performers. Conversely, visual stimuli activating the auditory temporal cortex was observed in poorer performers. This suggests that learning auditory speech perception with a CI is facilitated by visual cues, yet visual cues should not be the main input for the auditory cortex. In practice, provided the beneficial activation in the visual cortex is higher than the activation induced by visual stimuli in the auditory cortex, speech perception in quiet is more successful. This occurrence of cross-modal activation might be related to duration of deafness and plasticity postimplantation (Buckley and Tobey, 2010; Sandmann et al., 2012; Song et al., 2015a; Chen et al., 2016, 2017; Han et al., 2019).
Subsequently, Lazard et al. (2010, 2011) and Lazard and Giraud (2017) investigated both the involvement of the visual cortex during listening and cross-modal activation in the auditory cortex in CI candidates. They found that performance after implantation depended on activation of either the dorsal route or the ventral route during sound imagery tasks, indicating the use of phonological and speech sound properties, or the use of lexico-semantic properties, respectively. This confirmed the importance of maintaining both the temporal and occipital cortex for normal sound or language processing [such as phonological processing and the integration of visual cues (visemes) with phonological properties] even if the input is not auditory. It seems that if only fast semantic- or lexical-based strategies become the default during the time of deafness, it is hard to return to incorporating original slow speech sound-based strategies once implanted, which contributes to poorer performance. Future research may provide further insights into what causes CI listeners to use different speech perception strategies engaging different brain areas, leading to better or poorer outcomes.
Several observations were made from the literature studying cognitive performance in CI listeners and its association with speech perception outcomes.
First, non-verbal intelligence, assessed using the Ravens Matrices task, was positively related to word or sentence perception in quiet in most studies (9 out of 13) (Moberly et al., 2017c, 2018c, 2021; Mattingly et al., 2018; Pisoni et al., 2018; Moberly and Reed, 2019; O’Neill et al., 2019; Skidmore et al., 2020; Zhan et al., 2020; Tamati and Moberly, 2021; Tamati et al., 2021). The Ravens task is thought to, amongst other things, involve the ability of inducing abstract relations as well as working memory (Carpenter et al., 1990). Since it is suggested that several basic cognitive functions are involved in performing the Ravens task, it is unclear whether one of these cognitive functions underlie the observed relationship with speech perception performance (Mattingly et al., 2018). Studies that used other tasks to measure the same domain failed to provide additional evidence for the association of the cognitive subdomain non-verbal intelligence with speech perception outcomes (Collison et al., 2004; Holden et al., 2013; Moberly et al., 2016b).
Second, performance on auditory and visual working memory tasks was unrelated to speech perception outcomes in most studies (11 of 15) (Holden et al., 2013; Moberly et al., 2016b, 2017a,b,c, 2018c, 2021; Moberly and Reed, 2019; Skidmore et al., 2020; Tamati et al., 2020; Zhan et al., 2020; Bosen et al., 2021; Tamati and Moberly, 2021; Luo et al., 2022). When both modalities were combined in the working memory task, or more verbal aspects were added, significant correlations with word and sentence perception, both in quiet and noise, appeared to be more prevalent. However, there were only a limited number of studies assessing these types of working memory and thus more data is required to draw any conclusions. Interestingly, Luo et al. (2022), performed a more extensive verbal working memory task, including a cued and uncued working memory condition. When corrected for temporal and spectral resolution, only a significant positive correlation remained between performance in the uncued condition and word perception in noise, but not sentence perception in noise. This suggests that while top-down information is less available, as in the uncued condition of the working memory task (compared to the cued condition), similar working memory processes are at play as during word perception in noise (compared to sentence perception in noise). More research is needed to confirm whether a specific type of working memory is involved in particular speech perception tasks, in the same way that working memory is thought to be modality-specific (Park and Jon, 2018). Working memory processes would enable the listener to retain relevant information while listening to speech [as suggested by the ease of language understanding model (ELU) (Rönnberg et al., 2013)].
Third, cognitive inhibition was generally unrelated to word perception in quiet; a negative relationship was only observed in people with a high degree of auditory sensitivity or after adaptation to speech (Moberly et al., 2021; Tamati and Moberly, 2021). The relationship with sentence perception in quiet was less clear and in several papers negative relationships were observed (3 of 7) (Moberly et al., 2016b, 2017b, 2018a,c; Moberly and Reed, 2019; Tamati et al., 2020; Zhan et al., 2020). This possibly indicates that inhibiting information is engaged more in sentence perception compared to word perception. In theory, sentences contain more information than single words, and interfering information needs to be suppressed while items are retained in working memory (Rönnberg, 2003). It should be noted, however, that since most of these studies were performed in the same lab within the same participant sample, the results should be considered carefully. Additionally, only one main task, the Stroop task, was used to assess cognitive inhibition. It is possible that by implementing the Flanker task more often, different results might be observed, as both tasks measure different facets of inhibitory control (Knight and Heinrich, 2017).
Fourth, performance on standard recall tasks assessing the cognitive domain learning and memory in general did not to correlate with speech perception performance (Holden et al., 2013; Moberly et al., 2017a; Pisoni et al., 2018; Hillyer et al., 2019; Kessler et al., 2020; Skidmore et al., 2020; Tamati et al., 2020; Völter et al., 2021; Ray et al., 2022; Zucca et al., 2022). This is contrary to expectations, as the relevance of these skills is often emphasized in speech perception models (Rönnberg et al., 2013). One explanation for this discrepancy could be that these tasks are not reflective of the use of memory and learning for everyday speech perception. The fact that scores on subtests of the CVLT showed significant positive correlations with sentence perception in quiet and noise (Pisoni et al., 2018; Ray et al., 2022), might already give an example of a test or scoring method more representative of memory and learning skills involved in speech perception. Compared to simple word and picture recall tasks, this test calculates specific scores on, for example, semantic clustering or recall consistency.
Although these studies provide some indications, for many of the cognitive functions there is no or insufficient data to make any inferences. Often, one task is applied only within a single study or results are inconsistent. This is true for social cognition, the general cognitive measures, attention, processing speed, flexibility, audio-visual and verbal working memory, and perceptual-motor function. Moreover, most studies do not report any power analysis, which further increases the unreliability of results should the power be insufficient. One example where a greater sample size seemed to lead to clearer outcomes was for the general cognitive measures. These did not predict word perception in quiet with a sample size of 15 (Zucca et al., 2022). However, research including a larger sample size (df = 32) (Wazen et al., 2020) did show the effectiveness of a quick cognitive assessment for predicting sentence perception in quiet and noise preoperatively. Furthermore, it might be beneficial to consider social cognition, the only cognitive domain currently not covered in the literature. This domain might be of value to CI listeners, as better social skills might lead to more social exposure and therefore more listening practice (Knickerbocker et al., 2021). Therefore, it seems that it would be worthwhile to include this cognitive domain in future research.
Language is the most interesting cognitive domain in the context of the current paper, as speech perception is part of this domain. According to the outcomes of the included papers, vocabulary was not associated with speech perception performance (7 out of 10 papers) (Collison et al., 2004; Kaandorp et al., 2015, 2017; Shafiro et al., 2016; Moberly et al., 2017a; Kessler et al., 2020; Tamati et al., 2020; Völter et al., 2021). For both verbal fluency and degraded language perception, only a few papers were included, which did not allow to make any inferences about these cognitive skills (Haumann et al., 2012; Finke et al., 2016; Kaandorp et al., 2017; Moberly et al., 2018c; Kessler et al., 2020; Völter et al., 2021; Zucca et al., 2022). The last skill, speed of lexical and phonological access, was often shown to be significantly positively correlated with word and sentence perception when assessed using TOWRE (3 of 5 study populations) (Moberly et al., 2018a,c, 2021; Pisoni et al., 2018; Moberly and Reed, 2019; Skidmore et al., 2020; Tamati et al., 2021). Overall, it seems that in adult CI users, rather than lexical knowledge, the ability to form words quickly and efficiently from phonemes or written text is crucial for speech perception outcomes (even if bottom-up information is incomplete).
This literature overview points toward some cognitive factors predicting or failing to predict speech perception performance. Unfortunately, a considerable number of reviewed studies showed inconsistent results. As more studies are needed to validate the conclusions above, possible reasons for inconsistency and suggestions to improve future studies are provided:
First, tasks capture scores in different manners when evaluating a cognitive skill. For example, a significant positive correlation was found when measuring response time per trial in an attention task, but not when measuring accuracy within a prespecified time frame (Moberly et al., 2016b; Hillyer et al., 2019; Völter et al., 2021). Similarly, some tasks are more engaging compared to others which aim to assess the same cognitive skills. This might lead to differences in validity between these tests. For example, of two measures assessing attention, the TMT-B requires more use of semantic knowledge compared to pattern matching. Future studies might consider using different measures assessing the same cognitive skills, or one task under different conditions, to determine what feature of the task is relevant for assessing a certain cognitive skill in relation to speech perception outcomes.
Second, the time of assessment might influence results. Significant positive correlations of performance on the TMT-A with speech perception were found when measured preoperatively (Zucca et al., 2022), but similar measures performed postoperatively did not show such a relationship with speech perception outcomes (Hua et al., 2017; Völter et al., 2021). Performing the same cognitive test before and after implantation could provide more insight in this respect. Furthermore, it might provide more granular information on causal relationships, which is valuable for clinical purposes.
Third, the speech perception measures used and the mode of presentation might explain inconsistent findings. Many studies reported cognitive measures to be related to sentence perception outcomes (in noise), rather than word perception outcomes (in quiet). However, it is unclear whether adding noise to words or sentences causes particular cognitive skills to be engaged, as many studies measure words in quiet and sentences in noise only. Measuring all four possible conditions might also be important to create a general classification system for better and poorer performers, which in turn can help to better generalize results. For example, it has been observed that poorer performers in quiet are poorer performers in noise, but better performers in quiet might be poorer performers in noise (Walia et al., 2022). Understanding the underlying causes leading to either poor performance in quiet or noise is needed, as this might lead toward different treatment options. In addition to the speech perception task, the extent to which bottom-up information during this task is not accessible, might also lead to the use of different cognitive strategies. Therefore, including measures of auditory sensitivity (as in Moberly et al., 2021) might be valuable. Furthermore, presentation mode (whether speech is presented in CI alone, or best aided condition) should be clearly stated. Unfortunately, this is overlooked in many of the included papers. Therefore, based on the included literature, it is impossible to make any inferences regarding the influence of listening condition on the use of specific cognitive skills. Indicating the test conditions in detail, or even including different testing conditions in future studies, like Hua et al. (2017), might be insightful.
Fourth, as mentioned in the introduction, the different cognitive domains and factors are not independent of each other. In fact, some tasks are used specifically to measure two different cognitive factors. Therefore, results based on correlation analysis, whereby each of the cognitive task scores are correlated separately with speech perception outcomes, should be interpreted with caution. It might be more informative to more often use alternative statistical analyses, such as regression analysis, instead. This could reveal any mediation of specific cognitive factors or cognitive scores explaining more of the variance in speech perception outcomes. Furthermore, as discussed before, many of the included studies do not report their power calculations, nor do they provide all statistical values. Ensuring sufficient power and consistently reporting statistical values (including effect sizes and values of non-significant results) will improve interpretation of results.
Lastly, while it is useful to look at papers which investigate either brain activation patterns or performance on cognitive and language tasks, it could be highly valuable to combine both neuroimaging techniques and behavioral measures within studies. We believe this could be beneficial as these measures could validate each other, as well as provide information on underlying neurocognitive processes involved in the observed behavior.
The main limitation of this scoping review is that the number of papers for several neurocognitive domains is limited and their methods and dependent/independent variables are highly variable. Conclusions, therefore, are only based on a limited number of papers that cover the same cognitive domain or function. Secondly, as stressed previously, many questions remain as to why results of different papers do not agree. Furthermore, no risk of bias assessment was performed. This makes the conclusions drawn prone to being influenced by biases, whether coming from the authors of the included literature or from interpretation by the authors of this scoping review. Implementing the above-mentioned suggestions could improve evidence in future research and bring more clarity on the topics discussed in this review.
In this scoping review, a comprehensive overview of literature on the relationship between cognitive factors and speech perception outcomes in adult CI users was given. This literature showed that the use of higher-order cognitive functions, recruiting the frontal cortex, the use of visual cues, recruiting the occipital cortex, and the temporal cortex still available for auditory processing, are beneficial for postlingually deafened adult CI users in relation to speech perception outcomes. Cognitive assessments indicate that performance on non-verbal intelligence tasks positively correlated with speech perception outcomes. Performance on auditory or visual working memory, learning, memory and vocabulary tasks were unrelated to speech perception outcomes and performance on the Stroop task unrelated to word perception in quiet. However, many uncertainties regarding the explanation of inconsistent results and the small number of studies limit the extent of these conclusions. Additional research is needed to validate current findings. Only then will they potentially be used as a guide for counseling and rehabilitating adult CI users.
LB, BP, EM, and WH contributed to the conception and design of the study. LB and NT collected the data. LB, NT, BP, and WH curated and interpreted the data. LB wrote the first draft of the manuscript. NT, BP, EM, and WH revised the manuscript critically. All authors read and approved the submitted version.
This funding was received from the European Union’s Horizon 2020 Research and Innovation Programme under the Marie Skłodowska-Curie grant agreement No. 860718.
The authors thank Dr. Isabelle Mosnier her support and insightful comments while writing this manuscript.
LB and BP were employed by Cochlear Ltd. NT was affiliated with Cochlear Ltd.
The remaining authors declare that the research was conducted in the absence of any commercial or financial relationships that could be construed as a potential conflict of interest.
All claims expressed in this article are solely those of the authors and do not necessarily represent those of their affiliated organizations, or those of the publisher, the editors and the reviewers. Any product that may be evaluated in this article, or claim that may be made by its manufacturer, is not guaranteed or endorsed by the publisher.
The Supplementary Material for this article can be found online at: https://www.frontiersin.org/articles/10.3389/fnins.2023.1046669/full#supplementary-material
CI, Cochlear Implant; CVLT, California Verbal Learning Test; DSM-5, Diagnostic Statistical Manual of Mental Disorders, Fifth Edition; EEG, electroencephalography; fNIRS, functional near-infrared spectroscopy; (f)MRI, (functional) magnetic resonance imaging; MoCA, Montreal Cognitive Assessment; OSPAN, Operation Span; PET, positron emission tomography; PRISMA, Preferred Reporting Items for Systematic Reviews and Meta-Analysis; RAN, Rapid Automatic Naming; SAGE, Self-Administered Gerocognitive Examination; SicSpan, Size comparison Span; TMT (–A/B), Trail Making Task (version A or B); TOWRE, Test of Word Reading Efficiency; TRT, Text Reception Threshold; VEP, visual evoked potential; WAIS-III, Wechsler Adult Intelligence Scale III; WJ-IV, Woodcock-Johnson IV (test battery); WRAT, Wide Range Achievement Test.
Abdel-Latif, K. H. A., and Meister, H. (2022). Speech recognition and listening effort in cochlear implant recipients and normal-hearing listeners. Front. Neurosci. 15:725412. doi: 10.3389/fnins.2021.725412
Aggarwal, R., and Green, K. M. J. (2012). Cochlear implants and positron emission tomography. J. Laryngol. Otol. 126, 1200–1203. doi: 10.1017/S0022215112002241
Alemi, R., and Lehmann, A. (2019). Middle latency responses to optimized chirps in adult cochlear implant users. J. Am. Acad. Audiol. 30, 396–405. doi: 10.3766/jaaa.18014
Amichetti, N. M., Atagi, E., Kong, Y. Y., and Wingfield, A. (2018). Linguistic context versus semantic competition in word recognition by younger and older adults with cochlear implants. Ear Hear. 39, 101–109. doi: 10.1097/AUD.0000000000000469
Anderson, C. A., Wiggins, I. M., Kitterick, P. T., and Hartley, D. E. H. (2017). Adaptive benefit of cross-modal plasticity following cochlear implantation in deaf adults. Proc. Natl. Acad. Sci. U.S.A. 114, 10256–10261. doi: 10.1073/pnas.1704785114
Anderson, S., and Jenkins, K. (2015). Electrophysiologic assessment of auditory training benefits in older adults. Semin. Hear. 36, 250–262. doi: 10.1055/s-0035-1564455
Anderson, S., and Kraus, N. (2013). Auditory training: Evidence for neural plasticity in older adults. Perspect. Hear. Hear. Disord. Res. Diagn. 17, 37–57. doi: 10.1044/hhd17.1.37
Balkenhol, T., Wallhäusser-Franke, E., Rotter, N., and Servais, J. J. (2020). Changes in speech-related brain activity during adaptation to electro-acoustic hearing. Front. Neurol. 11:161. doi: 10.3389/fneur.2020.00161
Baskent, D., Clarke, J., Pals, C., Benard, M. R., Bhargava, P., Saija, J., et al. (2016). Cognitive compensation of speech perception with hearing impairment, cochlear implants, and aging. Trends Hear. 20:233121651667027. doi: 10.1177/2331216516670279
Bavelier, D., and Neville, H. J. (2002). Cross-modal plasticity: Where and how? Nat. Rev. Neurosci. 3, 443–452. doi: 10.1038/nrn848
Berding, G., Wilke, F., Rode, T., Haense, C., Joseph, G., Meyer, G. J., et al. (2015). Positron emission tomography imaging reveals auditory and frontal cortical regions involved with speech perception and loudness adaptation. PLoS One 10:e0128743. doi: 10.1371/journal.pone.0128743
Bisconti, S., Shulkin, M., Hu, X., Basura, G. J., Kileny, P. R., and Kovelman, I. (2016). Functional near-infrared spectroscopy brain imaging investigation of phonological awareness and passage comprehension abilities in adult recipients of cochlear implants. J. Speech Lang. Hear. Res. 59, 239–253. doi: 10.1044/2015_JSLHR-L-14-0278
Bönitz, H., Kopp, B., Büchner, A., Lunner, T., Lyxell, B., and Finke, M. (2018). Event-related neuronal responses to acoustic novelty in single-sided deaf cochlear implant users: Initial findings. Clin. Neurophysiol. 129, 133–142. doi: 10.1016/j.clinph.2017.10.025
Bortfeld, H. (2019). Functional near-infrared spectroscopy as a tool for assessing speech and spoken language processing in pediatric and adult cochlear implant users. Dev. Psychobiol. 61, 430–443. doi: 10.1002/dev.21818
Bosen, A. K., Sevich, V. A., and Cannon, S. A. (2021). Forward digit span and word familiarity do not correlate with differences in speech recognition in individuals with cochlear implants after accounting for auditory resolution. J. Speech Lang. Hear. Res. 64, 3330–3342. doi: 10.1044/2021_JSLHR-20-00574
Breedlove, S. M., and Watson, N. V. (2013). Biological psychology: An introduction to behavioral, cognitive, and clinical neuroscience, 7th Edn. Sunderland, MA: Sinauer Associates.
Buckley, K. A., and Tobey, E. A. (2010). Cross-modal plasticity and speech perception in pre-and postlingually deaf cochlear implant users. Ear Hear. 32, 2–15.
Butera, I. M., Stevenson, R. A., Mangus, B. D., Woynaroski, T. G., Gifford, R. H., and Wallace, M. T. (2018). Audiovisual temporal processing in postlingually deafened adults with cochlear implants. Sci. Rep. 8:11345. doi: 10.1038/s41598-018-29598-x
Carpenter, P. A., Just, M. A., Shell, P., Klahr, D., Kotovsky, K., Newell, A., et al. (1990). Psychological review. Washington, DC: American Psychological Association.
Cartocci, G., Maglione, A. G., Vecchiato, G., Modica, E., Rossi, D., Malerba, P., et al. (2018). Frontal brain asymmetries as effective parameters to assess the quality of audiovisual stimuli perception in adult and young cochlear implant users. Acta Otorhinolaryngol. Ital. 38, 346–360. doi: 10.14639/0392-100X-1407
Chari, D. A., Barrett, K. C., Patel, A. D., Colgrove, T. R., Jiradejvong, P., Jacobs, L. Y., et al. (2020). Impact of auditory-motor musical training on melodic pattern recognition in cochlear implant users. Otol. Neurotol. 41, e422–e431. doi: 10.1097/MAO.0000000000002525
Chen, L. C., Puschmann, S., and Debener, S. (2017). Increased cross-modal functional connectivity in cochlear implant users. Sci. Rep. 7:10043. doi: 10.1038/s41598-017-10792-2
Chen, L. C., Sandmann, P., Thorne, J. D., Bleichner, M. G., and Debener, S. (2016). Cross-modal functional reorganization of visual and auditory cortex in adult cochlear implant users identified with fNIRS. Neural Plast. 2016:4382656. doi: 10.1155/2016/4382656
Collett, E., Marx, M., Gaillard, P., Roby, B., Fraysse, B., Deguine, O., et al. (2016). Categorization of common sounds by cochlear implanted and normal hearing adults. Hear. Res. 335, 207–219. doi: 10.1016/j.heares.2016.03.007
Collison, E. A., Munson, B., and Carney, A. E. (2004). Relations among linguistic and cognitive skills and spoken word recognition in adults with cochlear implants. J. Speech Lang. Hear. Res. 47, 469–508. doi: 10.1044/1092-4388(2004/039)
Crowson, M. G., Dixon, P., Mahmood, R., Lee, J. W., Shipp, D., Le, T., et al. (2020). Predicting postoperative cochlear implant performance using supervised machine learning. Otol. Neurotol. 41, e1013–e1023. doi: 10.1097/MAO.0000000000002710
Davis, M. H., and Johnsrude, I. S. (2007). Hearing speech sounds: Top-down influences on the interface between audition and speech perception. Hear. Res. 229, 132–147. doi: 10.1016/j.heares.2007.01.014
Debener, S., Hine, J., Bleeck, S., and Eyles, J. (2008). Source localization of auditory evoked potentials after cochlear implantation. Psychophysiology 45, 20–24. doi: 10.1111/j.1469-8986.2007.00610.x
Dimitrijevic, A., Smith, M. L., Kadis, D. S., and Moore, D. R. (2019). Neural indices of listening effort in noisy environments. Sci. Rep. 9:11278. doi: 10.1038/s41598-019-47643-1
Dingemanse, G. J., and Goedegebure, A. (2019). The important role of contextual information in speech perception in cochlear implant users and its consequences in speech tests. Trends Hear. 23:2331216519838672. doi: 10.1177/2331216519838672
Doucet, M. E., Bergeron, F., Lassonde, M., Ferron, P., and Lepore, F. (2006). Cross-modal reorganization and speech perception in cochlear implant users. Brain 129, 3376–3383. doi: 10.1093/brain/awl264
El-Kashlan, H. K., Boerst, A., and Telian, S. A. (2001). Multichannel cochlear implantation in visually impaired patients. Otol. Neurotol. 22, 53–56.
Finke, M., Büchner, A., Ruigendijk, E., Meyer, M., and Sandmann, P. (2016). On the relationship between auditory cognition and speech intelligibility in cochlear implant users: An ERP study. Neuropsychologia 87, 169–181. doi: 10.1016/j.neuropsychologia.2016.05.019
Finke, M., Sandmann, P., Bönitz, H., Kral, A., and Büchner, A. (2017). Consequences of stimulus type on higher-order processing in single-sided deaf cochlear implant users. Audiol. Neurotol. 21, 305–315. doi: 10.1159/000452123
Finke, M., Sandmann, P., Kopp, B., Lenarz, T., and Büchner, A. (2015). Auditory distraction transmitted by a cochlear implant alters allocation of attentional resources. Front. Neurosci. 9:68. doi: 10.3389/fnins.2015.00068
Gfeller, K., Miller, R. F. W., Christ, A., Knutson, J., Witt, S., Ccc-A, M. A., et al. (2003). The effects of familiarity and complexity on appraisal of complex songs by cochlear implant recipients and normal hearing adults. J. Music Ther. 40, 78–112.
Gilbert, S. J., and Burgess, P. W. (2007). Executive function. Curr. Biol. 18, 110–114. doi: 10.1016/j.cub.2007.12.014
Giraud, A. L., Price, C. J., Graham, J. M., and Frackowiak, R. S. J. (2001a). Functional plasticity of language-related brain areas after cochlear implantation. Brain 124, 1307–1316. doi: 10.1093/brain/124.7.1307
Giraud, A.-L., Price, C., Graham, J., Truy, E., and Frackowiak, R. (2001b). Cross-modal plasticity underpins language recovery after cochlear implantation. Neuron 30, 657–663. doi: 10.1016/s0896-6273(01)00318-x
Giraud, A. L., and Truy, E. (2002). The contribution of visual areas to speech comprehension: A PET study in cochlear implants patients and normal-hearing subjects. Neuropsychologia 40, 1562–1569. doi: 10.1016/s0028-3932(02)00023-4
Giraud, A.-L., Truy, E., Frackowiak, R. S. J., Grégoire, M.-C., Pujol, J.-F., Collet, L., et al. (2000). Differential recruitment of the speech processing system in healthy subjects and rehabilitated cochlear implant patients. Brain 123, 1391–1402. doi: 10.1093/brain/123.7.1391
Glennon, E., Svirsky, M. A., and Froemke, R. C. (2020). Auditory cortical plasticity in cochlear implant users. Curr. Opin. Neurobiol. 60, 108–114. doi: 10.1016/j.conb.2019.11.003
Goudey, B., Plant, K., Kiral, I., Jimeno-Yepes, A., Swan, A., Gambhir, M., et al. (2021). A multicenter analysis of factors associated with hearing outcome for 2,735 adults with cochlear implants. Trends Hear. 25:23312165211037525. doi: 10.1177/23312165211037525
Hafter, E. R. (2010). Is there a hearing aid for the thinking person? J. Am. Acad. Audiol. 21, 594–600. doi: 10.3766/jaaa.21.9.5
Han, J. H., Lee, H. J., Kang, H., Oh, S. H., and Lee, D. S. (2019). Brain plasticity can predict the cochlear implant outcome in adult-onset deafness. Front. Hum. Neurosci. 13:38. doi: 10.3389/fnhum.2019.00038
Harris, M. S., Boyce, L., Pisoni, D. B., Shafiro, V., and Moberly, A. C. (2017). The relationship between environmental sound awareness and speech recognition skills in experienced cochlear implant users. Otol. Neurotol. 38, e308–e314. doi: 10.1097/MAO.0000000000001514
Haumann, S., Hohmann, V., Meis, M., Herzke, T., Lenarz, T., and Büchner, A. (2012). Indication criteria for cochlear implants and hearing aids: Impact of audiological and non-audiological findings. Audiol. Res. 2:e12. doi: 10.4081/audiores.2012.e12
Hay-McCutcheon, M. J., Pisoni, D. B., and Kirk, K. I. (2005). Audiovisual speech perception in elderly cochlear implant recipients. Laryngoscope 115, 1887–1894. doi: 10.1097/01.mlg.0000173197.94769.ba
Heydebrand, G., Hale, S., Potts, L., Gotter, B., and Skinner, M. (2007). Cognitive predictors of improvements in adults’ spoken word recognition six months after cochlear implant activation. Audiol. Neurotol. 12, 254–264. doi: 10.1159/000101473
Hillyer, J., Elkins, E., Hazlewood, C., Watson, S. D., Arenberg, J. G., and Parbery-Clark, A. (2019). Assessing cognitive abilities in high-performing cochlear implant users. Front. Neurosci. 13:1056. doi: 10.3389/fnins.2018.01056
Hogg, M. A., and Vaughan, G. M. (2018). Social psychology, 8th Edn. New York, NY: Pearson Education Limited.
Holden, L. K., Finley, C. C., Firszt, J. B., Holden, T. A., Brenner, C., Potts, L. G., et al. (2013). Factors affecting open-set word recognition in adults with cochlear implants. Ear Hear. 34, 342–360. doi: 10.1097/AUD.0b013e3182741aa7
Hua, H., Johansson, B., Magnusson, L., Lyxell, B., and Ellis, R. J. (2017). Speech recognition and cognitive skills in bimodal cochlear implant users. J. Speech Lang. Hear. Res. 60, 2752–2763. doi: 10.1044/2017_JSLHR-H-16-0276
Jorgensen, L. E., and Messersmith, J. J. (2015). Impact of aging and cognition on hearing assistive technology use. Semin. Hear. 36, 162–174. doi: 10.1055/s-0035-1555119
Kaandorp, M. W., Smits, C., Merkus, P., Festen, J. M., and Goverts, S. T. (2017). Lexical-access ability and cognitive predictors of speech recognition in noise in adult cochlear implant users. Trends Hear. 21:2331216517743887. doi: 10.1177/2331216517743887
Kaandorp, M. W., Smits, C., Merkus, P., Goverts, S. T., and Festen, J. M. (2015). Assessing speech recognition abilities with digits in noise in cochlear implant and hearing aid users. Int. J. Audiol. 54, 48–57. doi: 10.3109/14992027.2014.945623
Kail, R., and Salthouse, T. A. (1994). Processing speed as a mental capacity. Acta Psychol. 86, 199–225.
Kelly, A. S., Purdy, S. C., and Thorne, P. R. (2005). Electrophysiological and speech perception measures of auditory processing in experienced adult cochlear implant users. Clin. Neurophysiol. 116, 1235–1246. doi: 10.1016/j.clinph.2005.02.011
Kessler, M., Schierholz, I., Mamach, M., Wilke, F., Hahne, A., Büchner, A., et al. (2020). Combined brain-perfusion SPECT and EEG measurements suggest distinct strategies for speech comprehension in CI users with higher and lower performance. Front. Neurosci. 14:787. doi: 10.3389/fnins.2020.00787
Kim, M. B., Shim, H. Y., Jin, S. H., Kang, S., Woo, J., Han, J. C., et al. (2016). Cross-Modal and intra-modal characteristics of visual function and speech perception performance in postlingually deafened, cochlear implant users. PLoS One 11:e0148466. doi: 10.1371/journal.pone.0148466
Knickerbocker, A., Bourn, S., Goldstein, M. R., and Jacob, A. (2021). Cochlear implant outcomes in elderly recipients during the COVID-19 pandemic. Otol. Neurotol. 42, e1256–e1262. doi: 10.1097/MAO.0000000000003291
Knight, S., and Heinrich, A. (2017). Different measures of auditory and visual stroop interference and their relationship to speech intelligibility in noise. Front. Psychol. 8:230. doi: 10.3389/fpsyg.2017.00230
Knopke, S., Bauknecht, H. C., Gräbel, S., Häußler, S. M., Szczepek, A. J., and Olze, H. (2021). White matter lesions as possible predictors of audiological performance in adults after cochlear implantation. Brain Sci. 11:600. doi: 10.3390/brainsci11050600
Lachs, L., Weiss, J. W., and Pisoni, D. B. (2002). Use of partial stimulus information by cochlear implant users and listeners with normal hearing in identifying spoken words: Some preliminary analyses. Volta Rev. 102, 303–320.
Lawrence, B. J., Jayakody, D. M. P., Henshaw, H., Ferguson, M. A., Eikelboom, R. H., Loftus, A. M., et al. (2018). Auditory and cognitive training for cognition in adults with hearing loss: A systematic review and meta-analysis. Trends Hear. 22:2331216518792096. doi: 10.1177/2331216518792096
Layer, N., Weglage, A., Müller, V., Meister, H., Lang-Roth, R., Walger, M., et al. (2022). The timecourse of multisensory speech processing in unilaterally stimulated cochlear implant users revealed by ERPs. Neuroimage Clin. 34:102982. doi: 10.1016/j.nicl.2022.102982
Lazard, D. S., and Giraud, A. L. (2017). Faster phonological processing and right occipito-temporal coupling in deaf adults signal poor cochlear implant outcome. Nat. Commun. 8:14872. doi: 10.1038/ncomms14872
Lazard, D. S., Giraud, A.-L., and Barone, P. (2013). “Multisensory interactions in auditory cortex and auditory rehabilitation in deafness,” in Springer handbook of auditory research, Vol. 47, eds A. Kral, A. Popper, and R. Fay (New York, NY: Springer), 217–236. doi: 10.1007/2506_2013_8
Lazard, D. S., Giraud, A. L., Truy, E., and Lee, H. J. (2011). Evolution of non-speech sound memory in postlingual deafness: Implications for cochlear implant rehabilitation. Neuropsychologia 49, 2475–2482. doi: 10.1016/j.neuropsychologia.2011.04.025
Lazard, D. S., Innes-Brown, H., and Barone, P. (2014). Adaptation of the communicative brain to post-lingual deafness. Evidence from functional imaging. Hear. Res. 307, 136–143. doi: 10.1016/j.heares.2013.08.006
Lazard, D. S., Lee, H. J., Gaebler, M., Kell, C. A., Truy, E., and Giraud, A. L. (2010). Phonological processing in post-lingual deafness and cochlear implant outcome. Neuroimage 49, 3443–3451. doi: 10.1016/j.neuroimage.2009.11.013
Lee, D. S., Lee, J. S., Oh, H., Kim, S.-K., Kim, J.-W., Chung, J.-K., et al. (2001). Cross-modal plasticity and cochlear implants. J. P. Trends Neurosci. 117, 526–528.
Lee, H. J., Lee, J. M., Choi, J. Y., and Jung, J. (2021). The effects of preoperative audiovisual speech perception on the audiologic outcomes of cochlear implantation in patients with postlingual deafness. Audiol. Neurotol. 26, 149–156. doi: 10.1159/000509969
Lee, S., Lucks Mendel, L., and Bidelman, G. M. (2019). Predicting speech recognition using the speech intelligibility index and other variables for cochlear implant users. J. Speech Lang. Hear. Res. 62, 1517–1531.
Li, Y., Booth, J. R., Peng, D., Zang, Y., Li, J., Yan, C., et al. (2013). Altered intra- and inter-regional synchronization of superior temporal cortex in deaf people. Cereb. Cortex 23, 1988–1996. doi: 10.1093/cercor/bhs185
Lonka, E., Kujala, T., Lehtokoski, A., Johansson, R., Rimmanen, S., Alho, K., et al. (2004). Mismatch negativity brain response as an index of speech perception recovery in cochlear-implant recipients. Audiol. Neurootol. 9, 160–162. doi: 10.1159/000077265
Luo, X., Azuma, T., Kolberg, C., and Pulling, K. R. (2022). The effects of stimulus modality, task complexity, and cuing on working memory and the relationship with speech recognition in older cochlear implant users. J. Commun. Disord. 95:106170. doi: 10.1016/j.jcomdis.2021.106170
Lyxell, B., Andersson, U., Borg, E., and Ohlsson, I. S. (2003). Working-memory capacity and phonological processing in deafened adults and individuals with a severe hearing impairment. Int. J. Audiol. 42, S86–S89. doi: 10.3109/14992020309074628
Mattingly, J. K., Castellanos, I., and Moberly, A. C. (2018). Nonverbal reasoning as a contributor to sentence recognition outcomes in adults with cochlear implants. Otol. Neurotol. 39, e956–e963. doi: 10.1097/MAO.0000000000001998
Mattys, S. L., Davis, M. H., Bradlow, A. R., and Scott, S. K. (2012). Speech recognition in adverse conditions: A review. Lang. Cogn. Process. 27, 953–978. doi: 10.1080/01690965.2012.705006
McKay, C. M., Shah, A., Seghouane, A. K., Zhou, X., Cross, W., and Litovsky, R. (2016). Connectivity in language areas of the brain in cochlear implant users as revealed by fNIRS. Adv. Exp. Med. Biol. 894, 327–335. doi: 10.1007/978-3-319-25474-6_34
McKee, M. M., Lin, F. R., and Zazove, P. (2018). State of research and program development for adults with hearing loss. Disabil. Health J. 11, 519–524. doi: 10.1016/j.dhjo.2018.07.010
Meyer, T. A., Frisch, S., Svirsky, M. A., Pisoni, D. B., Otol, A., Laryngol, R., et al. (2000). Modeling phoneme and open-set word recognition by cochlear implant users: A preliminary report. Ann. Otol. Rhinol. Laryngol. Suppl. 185, 68–70. doi: 10.1177/0003489400109s1229
Middlebrooks, J. C., Bierer, J. A., and Snyder, R. L. (2005). Cochlear implants: The view from the brain. Curr. Opin. Neurobiol. 15, 488–493. doi: 10.1016/j.conb.2005.06.004
Miller, C. W., Stewart, E. K., Wu, Y. H., Bishop, C., Bentler, R. A., and Tremblay, K. (2017). Working memory and speech recognition in noise under ecologically relevant listening conditions: Effects of visual cues and noise type among adults with hearing loss. J. Speech Lang. Hear. Res. 60, 2310–2320. doi: 10.1044/2017_JSLHR-H-16-0284
Mitchell, T. V., and Maslin, M. T. (2007). How vision matters for individuals with hearing loss. Int. J. Audiol. 46, 500–511. doi: 10.1080/14992020701383050
Moberly, A. C., Bates, C., Harris, M. S., and Pisoni, D. B. (2016a). The enigma of poor performance by adults with cochlear implants. Otol. Neurotol. 37, 1522–1528. doi: 10.1097/MAO.0000000000001211
Moberly, A. C., Houston, D. M., and Castellanos, I. (2016b). Non-auditory neurocognitive skills contribute to speech recognition in adults with cochlear implants. Laryngoscope Investig. Otolaryngol. 1, 154–162. doi: 10.1002/lio2.38
Moberly, A. C., Castellanos, I., Vasil, K. J., Adunka, O. F., and Pisoni, D. B. (2018a). “product” versus “process” measures in assessing speech recognition outcomes in adults with cochlear implants. Otol. Neurotol. 39, e195–e202. doi: 10.1097/MAO.0000000000001694
Moberly, A. C., Vasil, K., Baxter, J., and Ray, C. (2018b). What to do when cochlear implant users plateau in performance: A pilot study of clinician-guided aural rehabilitation. Otol. Neurotol. 39, E794–E802. doi: 10.1097/MAO.0000000000001964
Moberly, A. C., Vasil, K. J., Wucinich, T. L., Safdar, N., Boyce, L., Roup, C., et al. (2018c). How does aging affect recognition of spectrally degraded speech? Laryngoscope 128, S1–S16. doi: 10.1002/lary.27457
Moberly, A. C., Harris, M. S., Boyce, L., and Nittrouer, S. (2017a). Speech recognition in adults with cochlear implants: The effects of working memory, phonological sensitivity, and aging. J. Speech Lang. Hear. Res. 60, 1046–1061. doi: 10.1044/2016_JSLHR-H-16-0119
Moberly, A. C., Houston, D. M., Harris, M. S., Adunka, O. F., and Castellanos, I. (2017b). Verbal working memory and inhibition-concentration in adults with cochlear implants. Laryngoscope Investig. Otolaryngol. 2, 254–261. doi: 10.1002/lio2.90
Moberly, A. C., Pisoni, D. B., and Harris, M. S. (2017c). Visual working memory span in adults with cochlear implants: Some preliminary findings. World J. Otorhinolaryngol. Head Neck Surg. 3, 224–230. doi: 10.1016/j.wjorl.2017.12.003
Moberly, A. C., Lewis, J. H., Vasil, K. J., Ray, C., and Tamati, T. N. (2021). Bottom-up signal quality impacts the role of top-down cognitive-linguistic processing during speech recognition by adults with cochlear implants. Otol. Neurotol. 42, S33–S41. doi: 10.1097/MAO.0000000000003377
Moberly, A. C., Lowenstein, J. H., Tarr, E., Caldwell-Tarr, A., Welling, D. B., Shahin, A. J., et al. (2014). Do adults with cochlear implants rely on different acoustic cues for phoneme perception than adults with normal hearing? J. Speech Lang. Hear. Res. 57, 566–582. doi: 10.1044/2014_JSLHR-H-12-0323
Moberly, A. C., and Reed, J. (2019). Making sense of sentences: Top-down processing of speech by adult cochlear implant users. J. Speech Lang. Hear. Res. 62, 2895–2905. doi: 10.1044/2019_JSLHR-H-18-0472
Moher, D., Liberati, A., Tetzlaff, J., Altman, D. G., Altman, D., Antes, G., et al. (2009). Preferred reporting items for systematic reviews and meta-analyses: The PRISMA statement. PLoS Med. 6:e1000097. doi: 10.1371/journal.pmed.1000097
Moradi, S., Lidestam, B., Danielsson, H., Ng, E. H. N., and Rönnberg, J. (2017). Visual cues contribute differentially to audiovisual perception of consonants and vowels in improving recognition and reducing cognitive demands in listeners with hearing impairment using hearing aids. J. Speech Lang. Hear. Res. 60, 2687–2703. doi: 10.1044/2016_JSLHR-H-16-0160
Mortensen, M. V., Mirz, F., and Gjedde, A. (2006). Restored speech comprehension linked to activity in left inferior prefrontal and right temporal cortices in postlingual deafness. Neuroimage 31, 842–852. doi: 10.1016/j.neuroimage.2005.12.020
Most, T., and Adi-Bensaid, L. (2001). The influence of contextual information on the perception of speech by postlingually and prelingually profoundly hearing-impaired hebrew-speaking adolescents and adults. Ear Hear. 22, 252–263. doi: 10.1097/00003446-200106000-00008
Mussoi, B. S. S., and Brown, C. J. (2019). Age-related changes in temporal resolution revisited: Electrophysiological and behavioral findings from cochlear implant users. Ear Hear. 40, 1328–1344. doi: 10.1097/AUD.0000000000000732
Nagels, L., Bastiaanse, R., Başkent, D., and Wagner, A. (2019). Individual differences in lexical access among cochlear implant users. J. Speech Lang. Hear. Res. 63, 286–304.
Naples, J. G., and Berryhill McCarty, E. (2020). Olfaction and smell identification tests: A novel test that may correlate with cochlear implant outcomes. Med. Hypotheses 135:109446. doi: 10.1016/j.mehy.2019.109446
Oba, S. I., Galvin, J. J., and Fu, Q.-J. (2013). Minimal effects of visual memory training on the auditory performance of adult cochlear implant users. J. Rehabil. Res. Dev. 50, 99–110. doi: 10.1682/jrrd.2011.12.0229
O’Neill, E. R., Kreft, H. A., and Oxenham, A. J. (2019). Cognitive factors contribute to speech perception in cochlear-implant users and age-matched normal-hearing listeners under vocoded conditions. J. Acoust. Soc. Am. 146, 195–210. doi: 10.1121/1.5116009
Ouzzani, M., Hammady, H., Fedorowicz, Z., and Elmagarmid, A. (2016). Rayyan-a web and mobile app for systematic reviews. Syst. Rev. 5:210. doi: 10.1186/s13643-016-0384-4
Oxenham, A. J. (2018). Loudness context effects and auditory enhancement in normal, impaired, and electric hearing. Acta Acust. United Acust. 104, 839–843. doi: 10.3813/AAA.919254
Park, E. H., and Jon, D. I. (2018). Modality-specific working memory systems verified by clinical working memory tests. Clin. Psychopharmacol. Neurosci. 16, 489–493. doi: 10.9758/cpn.2018.16.1.489
Patro, C., and Mendel, L. L. (2018). Gated word recognition by postlingually deafened adults with cochlear implants: Influence of semantic context. J. Speech Lang. Hear. Res. 61, 145–158. doi: 10.1044/2017_JSLHR-H-17-0141
Patro, C., and Mendel, L. L. (2020). Semantic influences on the perception of degraded speech by individuals with cochlear implants. J. Acoust. Soc. Am. 147, 1778–1789. doi: 10.1121/10.0000934
Perreau, A. E., Wu, Y. H., Tatge, B., Irwin, D., and Corts, D. (2017). Listening effort measured in adults with normal hearing and cochlear implants. J. Am. Acad. Audiol. 28, 685–697. doi: 10.3766/jaaa.16014
Peters, M. D. J., Godfrey, C. M., Khalil, H., McInerney, P., Parker, D., and Soares, C. B. (2015). Guidance for conducting systematic scoping reviews. Int. J. Evid. Based Healthc. 13, 141–146. doi: 10.1097/XEB.0000000000000050
Peterson, N. R., Pisoni, D. B., and Miyamoto, R. T. (2010). Cochlear implants and spoken language processing abilities: Review and assessment of the literature. Restor. Neurol. Neurosci. 28, 237–250. doi: 10.3233/RNN-2010-0535
Pisoni, D. B., Broadstock, A., Wucinich, T., Safdar, N., Miller, K., Hernandez, L. R., et al. (2018). Verbal learning and memory after cochlear implantation in postlingually deaf adults: Some new findings with the CVLT-II. Ear Hear. 39, 720–745. doi: 10.1097/AUD.0000000000000530
Pisoni, D. B., Kronenberger, W. G., Chandramouli, S. H., and Conway, C. M. (2016). Learning and memory processes following cochlear implantation: The missing piece of the puzzle. Front. Psychol. 7:493. doi: 10.3389/fpsyg.2016.00493
Pisoni, D. B., Kronenberger, W. G., Harris, M. S., and Moberly, A. C. (2017). Three challenges for future research on cochlear implants. World J. Otorhinolaryngol. Head Neck Surg. 3, 240–254. doi: 10.1016/j.wjorl.2017.12.010
Purdy, S. C., and Kelly, A. S. (2016). Change in speech perception and auditory evoked potentials over time after unilateral cochlear implantation in postlingually deaf adults. Semin. Hear. 37, 62–73. doi: 10.1055/s-0035-1570329
Purdy, S. C., Welch, D., Giles, E., Morgan, C. L. A., Tenhagen, R., and Kuruvilla-Mathew, A. (2017). Impact of cognition and noise reduction on speech perception in adults with unilateral cochlear implants. Cochlear Implants Int. 18, 162–170. doi: 10.1080/14670100.2017.1299393
Ramos-Miguel, A., Perez-Zaballos, T., Perez, D., Falconb, J. C., and Ramosb, A. (2015). Use of data mining to predict significant factors and benefits of bilateral cochlear implantation. Eur. Arch. Otorhinolaryngol. 272, 3157–3162. doi: 10.1007/s00405-014-3337-3
Ray, C., Pisoni, D. B., Lu, E., Kronenberger, W. G., and Moberly, A. C. (2022). Preoperative visual measures of verbal learning and memory and their relations to speech recognition after cochlear implantation. Ear Hear. 43, 993–1002. doi: 10.1097/AUD.0000000000001155
Rönnberg, J. (2003). Cognition in the hearing impaired and deaf as a bridge between signal and dialogue: A framework and a model. Int. J. Audiol. 42, S68–S76. doi: 10.3109/14992020309074626
Rönnberg, J., Lunner, T., Zekveld, A., Sörqvist, P., Danielsson, H., Lyxell, B., et al. (2013). The ease of language understanding (ELU) model: Theoretical, empirical, and clinical advances. Front. Syst. Neurosci. 7:31. doi: 10.3389/fnsys.2013.00031
Rouger, J., Lagleyre, S., Fraysse, B., Denever, S., Deguine, O., and Barone, P. (2007). Evidence that cochlear-implanted deaf patients are better multisensory integrators. Proc. Natl. Acad. Sci. U.S.A. 104, 7295–7300. doi: 10.1073/pnas.0609419104
Sachdev, P. S., Blacker, D., Blazer, D. G., Ganguli, M., Jeste, D. V., Paulsen, J. S., et al. (2014). Classifying neurocognitive disorders: The DSM-5 approach. Nat. Rev. Neurol. 10, 634–642. doi: 10.1038/nrneurol.2014.181
Sandmann, P., Dillier, N., Eichele, T., Meyer, M., Kegel, A., Pascual-Marqui, R. D., et al. (2012). Visual activation of auditory cortex reflects maladaptive plasticity in cochlear implant users. Brain 135, 555–568. doi: 10.1093/brain/awr329
Schierholz, I., Schönermark, C., Ruigendijk, E., Kral, A., Kopp, B., and Büchner, A. (2021). An event-related brain potential study of auditory attention in cochlear implant users. Clin. Neurophysiol. 132, 2290–2305. doi: 10.1016/j.clinph.2021.03.055
Shafiro, V., Sheft, S., Norris, M., Spanos, G., Radasevich, K., Formsma, P., et al. (2016). Toward a nonspeech test of auditory cognition: Semantic context effects in environmental sound identification in adults of varying age and hearing abilities. PLoS One 11:e0167030. doi: 10.1371/journal.pone.0167030
Skidmore, J. A., Vasil, K. J., He, S., and Moberly, A. C. (2020). Explaining speech recognition and quality of life outcomes in adult cochlear implant users: Complementary contributions of demographic, sensory, and cognitive factors. Otol. Neurotol. 41, e795–e803. doi: 10.1097/MAO.0000000000002682
Smith, G. N. L., Pisoni, D. B., and Kronenberger, W. G. (2019). High-variability sentence recognition in long-term cochlear implant users: Associations with rapid phonological coding and executive functioning. Ear Hear. 40, 1149–1161. doi: 10.1097/AUD.0000000000000691
Song, J. J., Lee, H. J., Kang, H., Lee, D. S., Chang, S. O., and Oh, S. H. (2015a). Effects of congruent and incongruent visual cues on speech perception and brain activity in cochlear implant users. Brain Struct. Funct. 220, 1109–1125. doi: 10.1007/s00429-013-0704-6
Song, J. J., Vanneste, S., Lazard, D. S., van de Heyning, P., Park, J. H., Oh, S. H., et al. (2015b). The role of the salience network in processing lexical and nonlexical stimuli in cochlear implant users: An ALE meta-analysis of PET studies. Hum. Brain Mapp. 36, 1982–1994. doi: 10.1002/hbm.22750
Stenfelt, S., and Rönnberg, J. (2009). The signal-cognition interface: Interactions between degraded auditory signals and cognitive processes. Scand. J. Psychol. 50, 385–393. doi: 10.1111/j.1467-9450.2009.00748.x
Sterling Wilkinson Sheffield, B., Gifford, R. H., Ashmead, D. H., Rogers, B., and Christopher Stecker, G. (2016). The effects of auditory stimulus level and speech recognition performance on fNIRS measured cortical activation in adults with normal hearing and adults with cochlear implants. Ph.D. dissertation. Nashville, TN: Vanderbilt University.
Strelnikov, K., Rouger, J., Demonet, J. F., Lagleyre, S., Fraysse, B., Deguine, O., et al. (2013). Visual activity predicts auditory recovery from deafness after adult cochlear implantation. Brain 136, 3682–3695. doi: 10.1093/brain/awt274
Suh, M. W., Lee, H. J., Kim, J. S., Chung, C. K., and Oh, S. H. (2009). Speech experience shapes the speechreading network and subsequent deafness facilitates it. Brain 132, 2761–2771. doi: 10.1093/brain/awp159
Suh, M. W., Park, K. T., Lee, H. J., Lee, J. H. O., Chang, S. O., and Oh, S. H. A. (2015). Factors contributing to speech performance in elderly cochlear implanted patients: An FDG-PET study: A preliminary study. J. Int. Adv. Otol. 11, 98–103. doi: 10.5152/iao.2015.424
Sun, Z., Seo, J. W., Park, H. J., Lee, J. Y., Kwak, M. Y., Kim, Y., et al. (2021). Cortical reorganization following auditory deprivation predicts cochlear implant performance in postlingually deaf adults. Hum. Brain Mapp. 42, 233–244. doi: 10.1002/hbm.25219
Tamati, T. N., and Moberly, A. C. (2021). Talker adaptation and lexical difficulty impact word recognition in adults with cochlear implants. Audiol. Neurotol. 27, 260–270. doi: 10.1159/000518643
Tamati, T. N., Ray, C., Vasil, K. J., Pisoni, D. B., and Moberly, A. C. (2020). High- and low-performing adult cochlear implant users on high-variability sentence recognition: Differences in auditory spectral resolution and neurocognitive functioning. J. Am. Acad. Audiol. 31, 324–335. doi: 10.3766/jaaa.18106
Tamati, T. N., Vasil, K. J., Kronenberger, W. G., Pisoni, D. B., Moberly, A. C., and Ray, C. (2021). Word and nonword reading efficiency in postlingually deafened adult cochlear implant users. Otol. Neurotol. 42, e272–e278. doi: 10.1097/MAO.0000000000002925
Tao, D., Deng, R., Jiang, Y., Galvin, J. J., Fu, Q. J., and Chen, B. (2014). Contribution of auditory working memory to speech understanding in Mandarin-speaking cochlear implant users. PLoS One 9:e99096. doi: 10.1371/journal.pone.0099096
Tinnemore, A. R., Gordon-Salant, S., and Goupell, M. J. (2020). Audiovisual speech recognition with a cochlear implant and increased perceptual and cognitive demands. Trends Hear. 24:2331216520960601. doi: 10.1177/2331216520960601
Tremblay, C., Champoux, F., Lepore, F., and Théoret, H. (2010). Audiovisual fusion and cochlear implant proficiency. Restor. Neurol. Neurosci. 28, 283–291. doi: 10.3233/RNN-2010-0498
Turgeon, C., Lazzouni, L., Lepore, F., and Ellemberg, D. (2014). An objective auditory measure to assess speech recognition in adult cochlear implant users. Clin. Neurophysiol. 125, 827–835. doi: 10.1016/j.clinph.2013.09.035
Verhulst, S., Ernst, F., Garrett, M., and Vasilkov, V. (2018). Suprathreshold psychoacoustics and envelope-following response relations: Normal-hearing, synaptopathy and cochlear gain loss. Acta Acust. United Acust. 104, 800–803. doi: 10.3813/AAA.919227
Vitevitch, M. S., Pisoni, D. B., Kirk, K. I., Hay-Mccutcheon, M., Yount, S. L., Michael, M. A., et al. (2000). Effects of phonotactic probabilities on the processing of spoken words and nonwords by adults with cochlear implants who were postlingually deafened. Volta Rev. 102, 283–302.
Völter, C., Götze, L., Falkenstein, M., Dazert, S., and Thomas, J. P. (2017). Application of a computer-based neurocognitive assessment battery in the elderly with and without hearing loss. Clin. Interv. Aging 12, 1681–1690. doi: 10.2147/CIA.S142541
Völter, C., Oberländer, K., Carroll, R., Dazert, S., Lentz, B., Martin, R., et al. (2021). Nonauditory functions in low-performing adult cochlear implant users. Otol. Neurotol. 42, e543–e551. doi: 10.1097/MAO.0000000000003033
Wable, J., van den Abbeele, T., Âphane, S., Âgo, G., and Frachet, B. (2000). Mismatch negativity: A tool for the assessment of stimuli discrimination in cochlear implant subjects. Clin. Neurophysiol. 111, 743–751.
Wagner, F., Weder, S., Caversaccio, M. D., Federspiel, A., Wiest, R., and Senn, P. (2017). Functional magnetic resonance imaging in seven borderline cochlear implant candidates: A preliminary research study. Clin. Otolaryngol. 42, 1053–1057. doi: 10.1111/coa.12806
Walia, A., Shew, M. A., Kallogjeri, D., Wick, C. C., Durakovic, N., Lefler, S. M., et al. (2022). Electrocochleography and cognition are important predictors of speech perception outcomes in noise for cochlear implant recipients. Sci. Rep. 12:3083. doi: 10.1038/s41598-022-07175-7
Wallace, M. T. (2017). Cooperation between hearing and vision in people with cochlear implants. Proc. Natl. Acad. Sci. U.S.A. 114, 10003–10005. doi: 10.1073/pnas.1712810114
Wang, S., Dorman, M., Berisha, V., and Liss, J. (2015). Audiovisual sentence recognition in bimodal and bilateral cochlear implant users. Ph.D. thesis. Tempe, AZ: Arizona State University.
Wazen, J. J., Kellermeyer, B., Lange, L., Rende, S., Ortega, C., and Rosenberg, S. (2020). Predicting speech outcomes after cochlear implantation in older adults using the self-administered gerocognitive examination test. Otol. Neurotol. 41, E28–E35. doi: 10.1097/MAO.0000000000002425
Wilson, B. S., Dorman, M. F., Woldorff, M. G., and Tucci, D. L. (2011). Cochlear implants. Matching the prosthesis to the brain and facilitating desired plastic changes in brain function. Prog. Brain Res. 194, 117–129. doi: 10.1016/B978-0-444-53815-4.00012-1
Wilson, B. S., Lawson, D. T., Müller, J. M., Tyler, R. S., and Kiefer, J. (2003). Cochlear implants: Some likely next steps. Annu. Rev. Biomed. Eng. 5, 207–249. doi: 10.1146/annurev.bioeng.5.040202.121645
Winn, M. B., and Moore, A. N. (2018). Pupillometry reveals that context benefit in speech perception can be disrupted by later-occurring sounds, especially in listeners with cochlear implants. Trends Hear. 22:2331216518808962. doi: 10.1177/2331216518808962
Winn, M. B., Rhone, A. E., Chatterjee, M., and Idsardi, W. J. (2013). The use of auditory and visual context in speech perception by listeners with normal hearing and listeners with cochlear implants. Front. Psychol. 4:824. doi: 10.3389/fpsyg.2013.00824
Zaltz, Y., Bugannim, Y., Zechoval, D., Kishon-Rabin, L., and Perez, R. (2020). Listening in noise remains a significant challenge for cochlear implant users: Evidence from early deafened and those with progressive hearing loss compared to peers with normal hearing. J. Clin. Med. 9:1381. doi: 10.3390/jcm9051381
Zelazo, P. D., Anderson, J. E., Richler, J., Wallner-Allen, K., Beaumont, J. L., Conway, K. P., et al. (2014). NIH toolbox cognition battery (CB): Validation of executive function measures in adults. J. Int. Neuropsychol. Soc. 20, 620–629. doi: 10.1017/S1355617714000472
Zhan, K. Y., Lewis, J. H., Vasil, K. J., Tamati, T. N., Harris, M. S., Pisoni, D. B., et al. (2020). Cognitive functions in adults receiving cochlear implants: Predictors of speech recognition and changes after implantation. Otol. Neurotol. 41, e322–e329. doi: 10.1097/MAO.0000000000002544
Zhao, E. E., Dornhoffer, J. R., Loftus, C., Nguyen, S. A., Meyer, T. A., Dubno, J. R., et al. (2020). Association of patient-related factors with adult cochlear implant speech recognition outcomes: A meta-analysis. JAMA Otolaryngol. Head Neck Surg. 146, 613–620. doi: 10.1001/jamaoto.2020.0662
Zhou, X., Seghouane, A. K., Shah, A., Innes-Brown, H., Cross, W., Litovsky, R., et al. (2018). Cortical speech processing in postlingually deaf adult cochlear implant users, as revealed by functional near-infrared spectroscopy. Trends Hear. 22:2331216518786850. doi: 10.1177/2331216518786850
Keywords: cochlear implants, neurocognition, scoping review, sensorineural hearing loss, postlingual, speech perception
Citation: Beckers L, Tromp N, Philips B, Mylanus E and Huinck W (2023) Exploring neurocognitive factors and brain activation in adult cochlear implant recipients associated with speech perception outcomes—A scoping review. Front. Neurosci. 17:1046669. doi: 10.3389/fnins.2023.1046669
Received: 16 September 2022; Accepted: 05 January 2023;
Published: 02 February 2023.
Edited by:
Helen Henshaw, University of Nottingham, United KingdomReviewed by:
Chi Yhun Lo, Toronto Metropolitan University, CanadaCopyright © 2023 Beckers, Tromp, Philips, Mylanus and Huinck. This is an open-access article distributed under the terms of the Creative Commons Attribution License (CC BY). The use, distribution or reproduction in other forums is permitted, provided the original author(s) and the copyright owner(s) are credited and that the original publication in this journal is cited, in accordance with accepted academic practice. No use, distribution or reproduction is permitted which does not comply with these terms.
*Correspondence: Loes Beckers, bGphLmJlY2tlcnNAZ21haWwuY29t
Disclaimer: All claims expressed in this article are solely those of the authors and do not necessarily represent those of their affiliated organizations, or those of the publisher, the editors and the reviewers. Any product that may be evaluated in this article or claim that may be made by its manufacturer is not guaranteed or endorsed by the publisher.
Research integrity at Frontiers
Learn more about the work of our research integrity team to safeguard the quality of each article we publish.