- 1Department of Neurosurgery, The Affiliated Brain Hospital of Nanjing Medical University, Nanjing, China
- 2Department of Functional Neurosurgery, The Affiliated Brain Hospital of Nanjing Medical University, Nanjing, China
Objective: To identify whether tumor invasion of the temporal lobe induces functional compensation of the hippocampal-subregion (HIPsub) network connectivity before surgery, and to further validate the stability of this functional compensation within the HIPsub network in patients with temporal glioma tumor (TTumor) after surgical resection of the tumor.
Methods: In the first cohort, analysis of HIPsub functional connectivity (FC) was conducted to identify the functional compensation of the altered HIPsub connectivity pattern in TTumor through a pattern classification approach. Then, the second cohort investigated whether functional compensation in TTumor patients changed after surgical resection of the tumor.
Results: In the first cohort, this study identified altered HIPsub network connectivity patterns and its functional compensation regions (i.e., left parahippocampal gyrus and bilateral cerebellum anterior lobe) in TTumor patients. Second, the altered HIPsub network connectivity patterns had the power to discriminate TTumor patients from healthy controls (CN) on an individual subject basis, with an AUC of 97.0%, sensitivity of 93.5%, and specificity of 90.3%. Finally, in the second cohort, we found that functional connectivities of functional compensation regions within the HIPsub network in TTumor patients did not change between before and after surgery.
Conclusion: This study provides novel evidence regarding functional compensation within the HIPsub network in TTumor patients. It has been suggested that the fine hippocampal subregion was more sensitive, which reveals functional compensation induced by tumor invasion of the temporal lobe. Furthermore, this study verified the stability and persistence of this functional compensation in TTumor patients after surgical resection of the tumor.
Introduction
Gliomas account for approximately 81% of malignant primary brain tumors (Ostrom et al., 2014), with the second-highest proportion of temporal lobe invasion (Ostrom et al., 2019). Damage to the temporal lobe, known to be located in the hippocampal memory network, can alter its function including memory processing, emotional processing, and sensory processing (Chen et al., 2016, 2020, 2022). Interestingly, clinical observations have discovered that memory function remains intact among patients with temporal glioma. In recent years, a large number of studies have demonstrated that local tumor invasion could brain neural remodeling in the form of a network in order to maintain normal cognitive function (Liu et al., 2019, 2020b). However, little is known about whether tumor invasion of the temporal lobe can induce functional compensation of the hippocampal network connectivity. In particular, it is unknown whether this functional compensation can be retained after surgical resection of the tumor. Therefore, it is critical to understand the stability of the functional compensatory mechanism, which facilitates the preoperative planning of the compensatory mechanism of the protective function.
In recent years, neuroimaging studies have suggested functional heterogeneity and different FC patterns within the hippocampal subregion (Davachi, 2006; Robinson et al., 2015; Chen et al., 2016, 2020, 2022). Neuroimaging studies indicated that the hippocampus could be separated into three functional subregions: the anterior emotional region (HIPe), the middle cognitive region (HIPc), and the posterior perceptual region (HIPp) (Robinson et al., 2015; Chen et al., 2020, 2022). Different hippocampal subregions are involved in different cognitive processing (Robinson et al., 2015; Chen et al., 2016, 2020, 2022; Bai et al., 2019). Therefore, it is reasonable to speculate that tumor invasion of the temporal lobe can have different effects on different hippocampal subnetworks. In particular, the hippocampal subnetwork can more accurately describe the compensatory mechanisms that are induced by tumors.
In recent years, a large number of studies have consistently demonstrated that tumor invasion of the brain or lesion of a certain brain region within the network induces different forms of network compensation, and tumors in the different brain regions induce various forms of compensation (Bartolomei et al., 2006; He et al., 2007; Guggisberg et al., 2008; Alstott et al., 2009; Pravata et al., 2011; Liu et al., 2019, 2020b; Yuan et al., 2019). Based on the fact that the temporal lobe is located within the hippocampus network, we hypothesized that tumor invasion of the temporal lobe induces functional compensation of the hippocampal subnetwork. However, whether compensation induced by tumor invasion of the brain can be eliminated by tumor resection is unknown. In order to address these issues, a preoperative and postoperative study design was utilized to identify the stability of this functional compensation in patients with temporal glioma tumor (TTumor) after undergoing surgical resection of the tumor.
The objective of this study was to identify functional compensatory mechanisms of the hippocampal subnetwork in a cohort of TTumor patients through the use of a pattern classification approach (i.e., SVM). After identifying the compensatory mechanisms, we further investigated the stability of functional compensation in TTumor patients after undergoing surgical resection of the tumor in a separate cohort. Figure 1 shows the data analysis pipeline performed in this study.
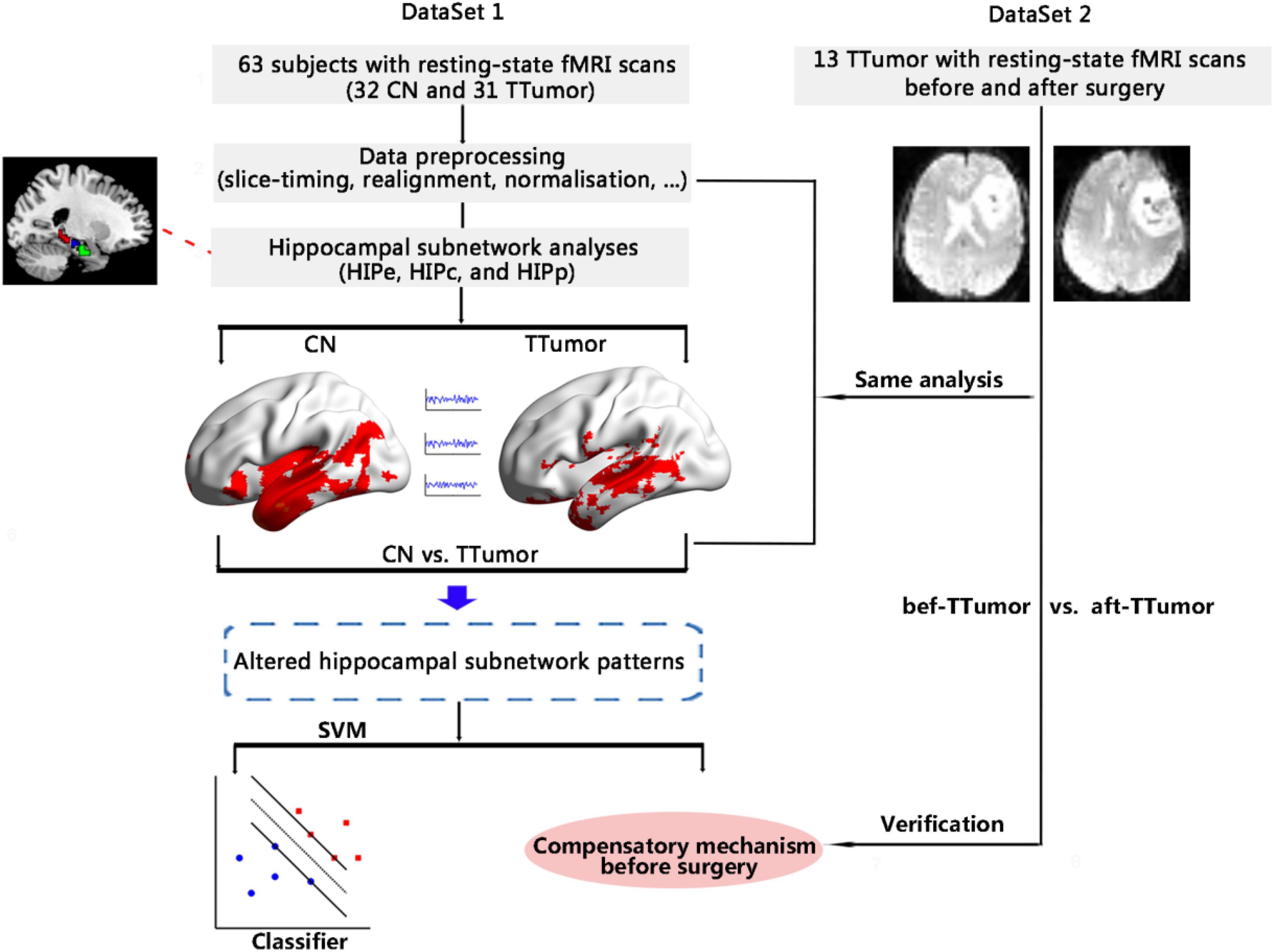
Figure 1. Schematic of the data analysis pipeline. Firstly, we measured functional connectivity (FC) within hippocampal subnetworks to identify any altered hippocampal subnetwork patterns in 32 CN and 31 TTumor subjects from the first cohort, as well as to identify preoperative compensatory brain areas. Secondly, we further applied a pattern classification approach (SVM) to evaluate whether altered hippocampal subnetwork patterns were able to distinguish TTumor from CN subjects. Finally, to test the stability of brain compensation mechanisms in preoperative TTumor glioma patients, we compared abnormal patterns of the hippocampal subnetwork before and after surgery in the second cohort. CN, healthy controls; TTumor, patients with temporal tumor (glioma); HIPe, hippocampal emotional region; HIPc, hippocampal cognitive region; HIPp, hippocampal perceptual region; fMRI, functional magnetic resonance imaging; SVM, support vector machine; bef-TTumor, patients with temporal tumor (glioma) before surgery; aft-TTumor, patients with temporal tumor (glioma) after surgery.
Materials and methods
Subjects
Overall, this study recruited 44 patients with temporal tumors (TTumor) from two cohorts (31 patients from the first cohort and 13 patients from the second cohort). The entire cohort comprised of inpatients at the Department of Neurosurgery at the Affiliated Brain Hospital of Nanjing Medical University. In total, 32 healthy control (CN) volunteers were recruited through advertisements. Written informed consent was obtained from all participants. The study was approved by the Human Participants Ethics Committee of the Affiliated Brain Hospital of Nanjing Medical University, Nanjing, China.
Detailed inclusion and exclusion criteria are provided in our previously published studies (Hu et al., 2020; Liu et al., 2020a,c) and in Supplementary Methods 1.
MRI data acquisition
The MRI data of TTumor patients were scanned between 2013 and 2022. The MRI images of TTumor patients were acquired before and 1 week after surgery using a 3.0 Tesla Verio Siemens scanner with an 8-channel head-coil that was provided by the department of radiology at the Affiliated Brain Hospital of Nanjing Medical University. The scanning parameters are provided in our previously published studies (Liu et al., 2019, 2020a,b) and in Supplementary Methods 2.
Image preprocessing
The preprocessing steps in this study refer to previously published studies (Chen et al., 2020, 2022; Liu et al., 2020b) and are provided in SI Methods 3. MATLAB R2015b1 and SPM122 were used to preprocess the MRI data. In brief, we conducted the following fMRI preprocessing steps: removing the first 10 images, slice-timing, head motion corrections, spatial normalization, spatial smoothing, nuisance covariates regression, and temporal filtering (0.01–0.1 Hz). Framewise displacement (FD) in the head motion parameter was not significantly different between the groups (T = −0.974, p = 0.334) (Power et al., 2012; Van Dijk et al., 2012). Furthermore, we calculated total intracranial volumes (TIV) using gray matter (GM), white matter (WM), and cerebrospinal fluid (CSF) partitions that were normalized and segmented using the Diffeomorphic Anatomical Registration Through Exponentiated Lie Algebra (DARTEL) method (Ashburner and Friston, 2009).
Definition of hippocampal subregions
We defined our HIPsub based on recent studies by Robinson et al. (2015); Bai et al. (2019), and Chen et al. (2020, 2022). In brief, we defined the hippocampus as the following three subregions (Supplementary Figure 1): HIPe, HIPc, and HIPp. A detailed definition of the hippocampal subregion is provided in previous publications (Chen et al., 2020, 2022) and provided in Supplementary Methods 4.
Hippocampal-subregion functional connectivity analyses
First, we created a group GM mask in the tumor-free region based on prior studies (Zhang et al., 2018; Di et al., 2022), and then the FC analysis of the HIPsub was conducted under the GM mask. The details regarding the construction of the GM mask in the tumor-free region are described in Supplementary Methods 5.
The steps of FC analysis of HIPsub are as follows. Firstly, the average time course for all voxels within HIPe, HIPc, and HIPp was extracted as the reference time course. Secondly, a voxel-wise cross-correlation analysis was conducted between the average time courses of all voxels within HIPe, HIPc, and HIPp and each voxel in the remainder of the whole brain within the group-specific GM mask. We defined the correlation coefficients obtained from this voxel-wise cross-correlation analysis as FC correlation coefficients. Finally, a Fisher’s z-transform analysis was conducted to improve the normality of the FC correlation coefficients.
Pattern classification based on the altered functional connectivity within hippocampal subnetworks before surgery in the first cohort
In reference to prior studies (Chen et al., 2020, 2022), we applied an support vector machine (SVM) method in order to investigate the extent to which FC within the HIPsub network was able to distinguish TTumor from CN subjects. The LIBSVM software (Software available at http://www.csie.ntu.edu.tw/∼cjlin/libsvm) was then utilized to perform a linear SVM classifier. A leave-one-out cross-validation (LOOCV) strategy was then utilized to assess the generalization of this SVM classifier, and its sensitivity and specificity. We evaluated the power of this SVM classifier in order to discriminate TTumor from CN subjects with the use of receiver operating characteristic (ROC) curves. The area under the ROC (AUC) value was employed to evaluate the power.
Changes of altered hippocampal-subregion networks related to temporal glioma tumor before and after surgery in in the second cohort
Based on the abnormal patterns that were present within the HIPsub networks before surgery (the first cohort), we compared the changes between these abnormal patterns before and after surgery in the second cohort using a paired t-test. Specifically, we tested the stability of brain compensation mechanisms among preoperative TTumor (glioma) patients.
Statistical analysis
Demographics data
We conducted a two-sample independent t-test and chi-square tests (only applied in gender comparisons) to compare differences in demographic data, head movement parameters, and TIV between CN and TTumor groups. The level of statistical significance is set at p < 0.05.
Altered hippocampal-subregion network functional connectivity patterns related to temporal glioma tumor patients
In order to characterize the HIPsub network FC patterns at a group level, we carried out a random-effect analysis through the use of one-sample t-tests in the spatial maps of FC in CN and TTumor subjects with a stringent threshold of p < 0.05 using a permutation test with TFCE and the family-wise error (FWE) correction together with a cluster extent k > 100 voxels (2,700 mm3). Then we created masks based on brain regions most robustly correlated with each HIPsub seed in TTumor and CN. FC data were extracted from the brain regions within these masks.
We conducted a general liner model (GLM) analysis to investigate differences in the FCs of HIPsub between the TTumor subjects and CN before surgery after controlling for age, sex, education, TIV, and mean FD (TFCE-FDR-corrected p < 0.05 and cluster size > 810 mm3). Then we developed masks based on brain regions that demonstrated differences in the FCs of HIPsub in TTumor compared to CN. These masks were utilized for the analysis before vs. after surgery fMRI data.
Results
Demographic and neuropsychological characteristics
As shown in Table 1, no significant differences in age or gender were observed between the TTumor group and the CN group (all p > 0.05). TTumor group had lower education level than the CN subjects (p < 0.05).

Table 1. Demographics, head movement parameters, and total intracranial volumes of CN and TTumor subjects before surgery in the first cohort.
Identification of altered hippocampal-subregion networks related to temporal glioma tumor patients
As shown in Supplementary Figure 2, TTumor subjects displayed distinctly altered FC patterns of the three HIPsub networks (i.e., HIPe, HIPc, and HIPp networks) compared to CN (PTFCE–FWE < 0.05, cluster size > 2,700 mm3).
Figure 2A and Table 2 show that, in the HIPe network, compared to CN, TTumor patients had significantly decreased FC in the right amygdala, right parahippocampal gyrus, right superior temporal gyrus, and right thalamus. Additionally, Figure 2B and Table 2 depict that, in the HIPc network, compared to CN, TTumor patients showed significantly decreased FC in the right parahippocampal gyrus, but increased FC in the left parahippocampal gyrus. Figure 2C and Table 2 indicate that, in the HIPp network, compared to CN, TTumor patients revealed significantly decreased FC in the left posterior cingulate cortex and right thalamus, but increased FC in bilateral cerebellum anterior lobes. All the results were controlled for age, sex, education, TIV, and FD.
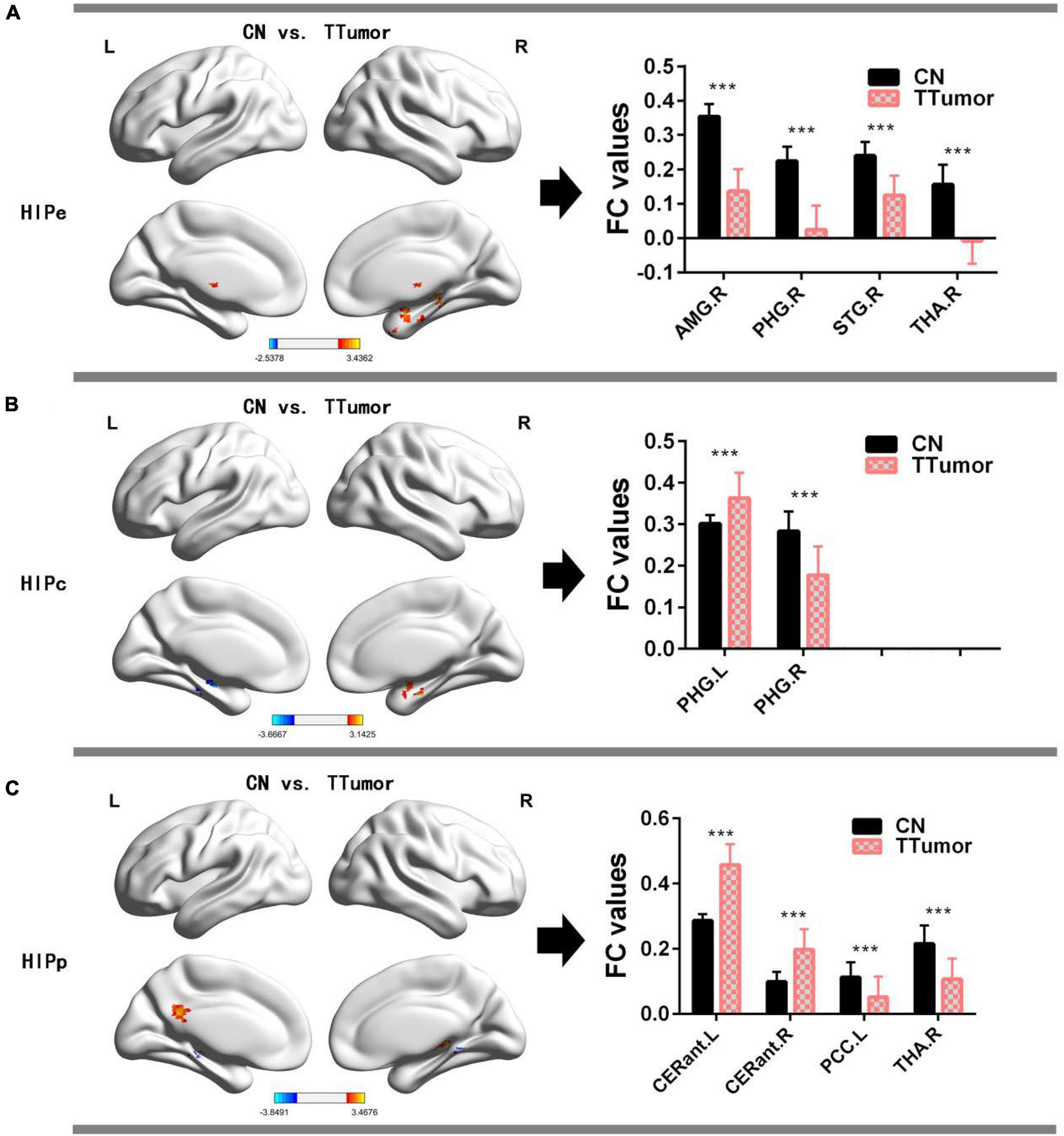
Figure 2. Comparison of FC within the hippocampal subnetworks between CN and TTumor subjects before surgery in the first cohort. (A) Altered regions of FC within the HIPe subnetwork in TTumor patients compared to CN. The bar chart on the right shows the quantitative comparison of FC within these altered regions; ***p < 0.001. (B) Altered regions of FC within the HIPc subnetwork in TTumor patients compared to CN. The bar chart on the right shows a quantitative comparison of FC within these altered regions; ***p < 0.001. (C) Altered regions of FC within the HIPp subnetwork in TTumor patients compared to CN. The bar chart on the right shows the quantitative comparison of FC within these altered regions; ***p < 0.001. CN, healthy controls; TTumor, patients with temporal tumor (glioma); HIPe, hippocampal emotional region; HIPc, hippocampal cognitive region; HIPp, hippocampal perceptual region; AMG.R, right amygdala; PHG.R, right parahippocampal gyrus; STG.R, right superior temporal gyrus; THA.R, right thalamus; PHG.L, left parahippocampal gyrus; CERant.L, left cerebellum anterior lobe; CERant.R, right cerebellum anterior lobe; PCC.L, left posterior cingulate cortex; L, left hemisphere; R, right hemisphere; FC, functional connectivity.
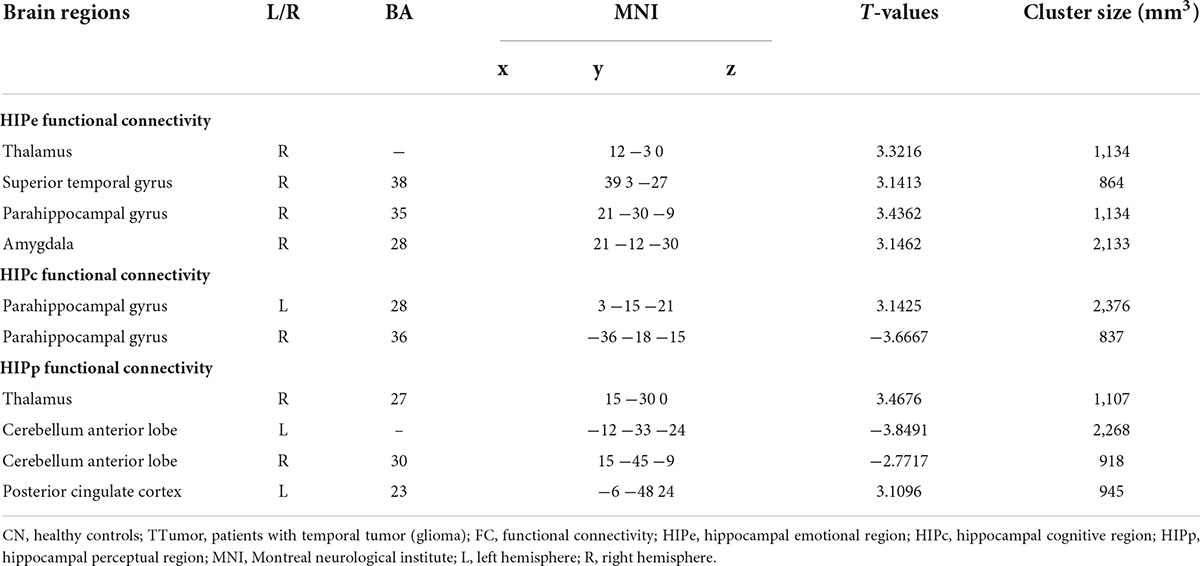
Table 2. Comparison of FC in the hippocampal subnetwork between CN and TTumor subjects before surgery in the first cohort.
Classification of temporal glioma tumor patients based on the altered hippocampal-subregion network connectivity
The SVM classifier’s ROC curve revealed a high power to discriminate TTumor patients from CN on an individual subject basis, with an AUC of 97.0%, 93.5% sensitivity, and 90.3% specificity (Figure 3).
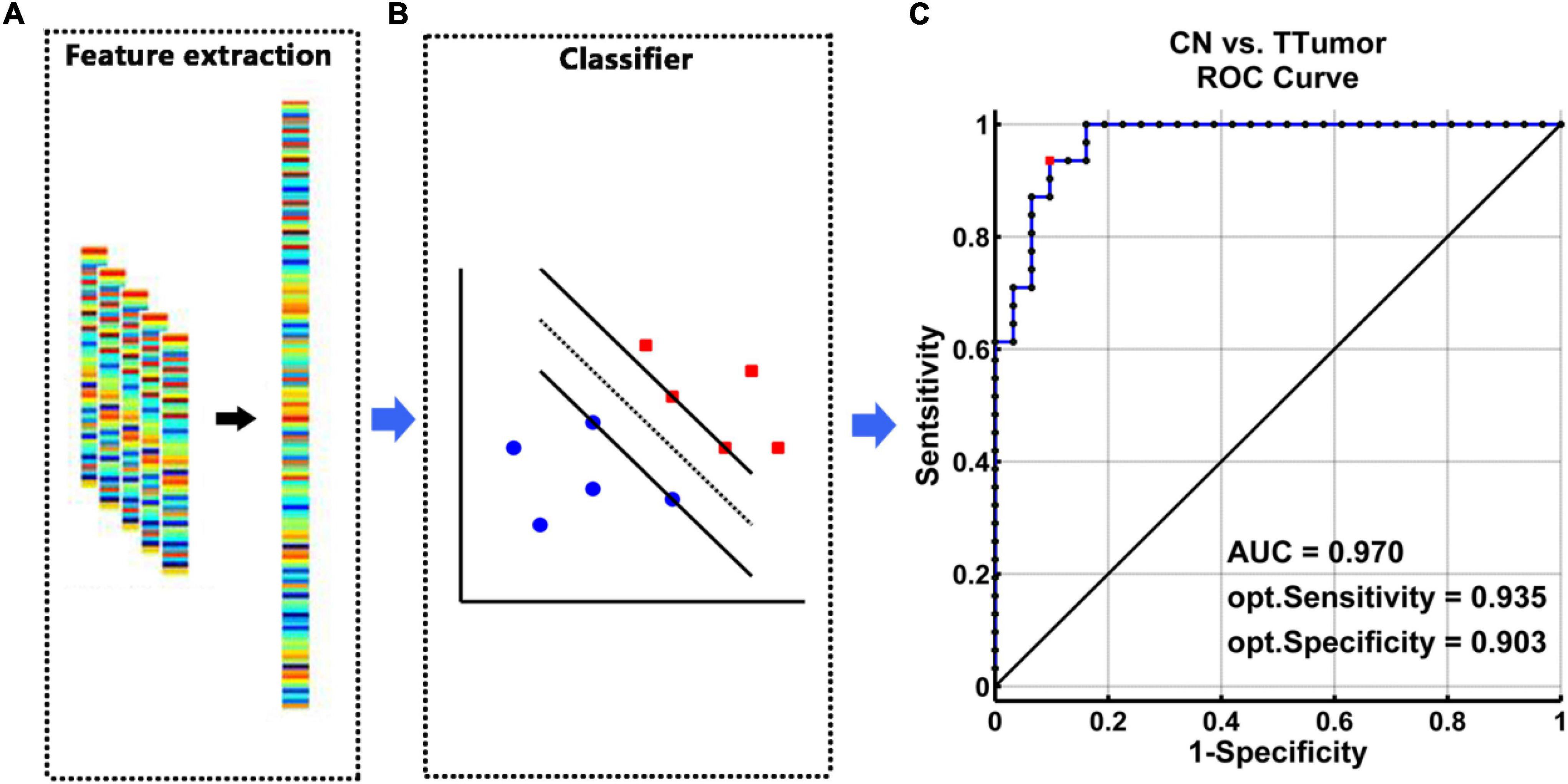
Figure 3. The classification power of an MRI-based “classifier” in distinguishing individuals with TTumor from CN before surgery in the first cohort. (A) We extracted features based on FC of the altered hippocampal subnetworks in order to train the “classifier” model. (B) The schematic diagram indicated a support vector machine “classifier,” based on altered functional characteristics within the HIPe, HIPc, and HIPp subnetworks. (C) The ROC curve showed the classification power of the MRI-based “classifier.” The values of AUC, sensitivity, and specificity are marked in the lower right of the figure. CN, healthy controls; TTumor, patients with temporal tumor (glioma); HIPe, hippocampal emotional region; HIPc, hippocampal cognitive region; HIPp, hippocampal perceptual region; AUC, area under the curve; FC, functional connectivity; opt, optimal.
Validation of stable compensatory mechanisms within hippocampal-subregion network in temporal glioma tumor patients
Figure 4A show that, in the HIPe network, compared to bef-TTumor, aft-TTumor patients demonstrated no differences in FC in the right amygdala and right superior temporal gyrus. They significantly decreased FC in the right parahippocampal gyrus and right thalamus. Figure 4B show that, in the HIPc network, compared to bef-TTumor, aft-TTumor patients showed no differences in FC in the left parahippocampal gyrus and significantly decreased FC in the right parahippocampal gyrus. Figure 4C show that, in the HIPp network, compared to bef-TTumor, aft-TTumor patients demonstrated no significant differences in FC in the bilateral cerebellum anterior lobe and significantly decreased FC in the left posterior cingulate cortex and right thalamus. All the results were controlled for age, sex, education, TIV, and FD.
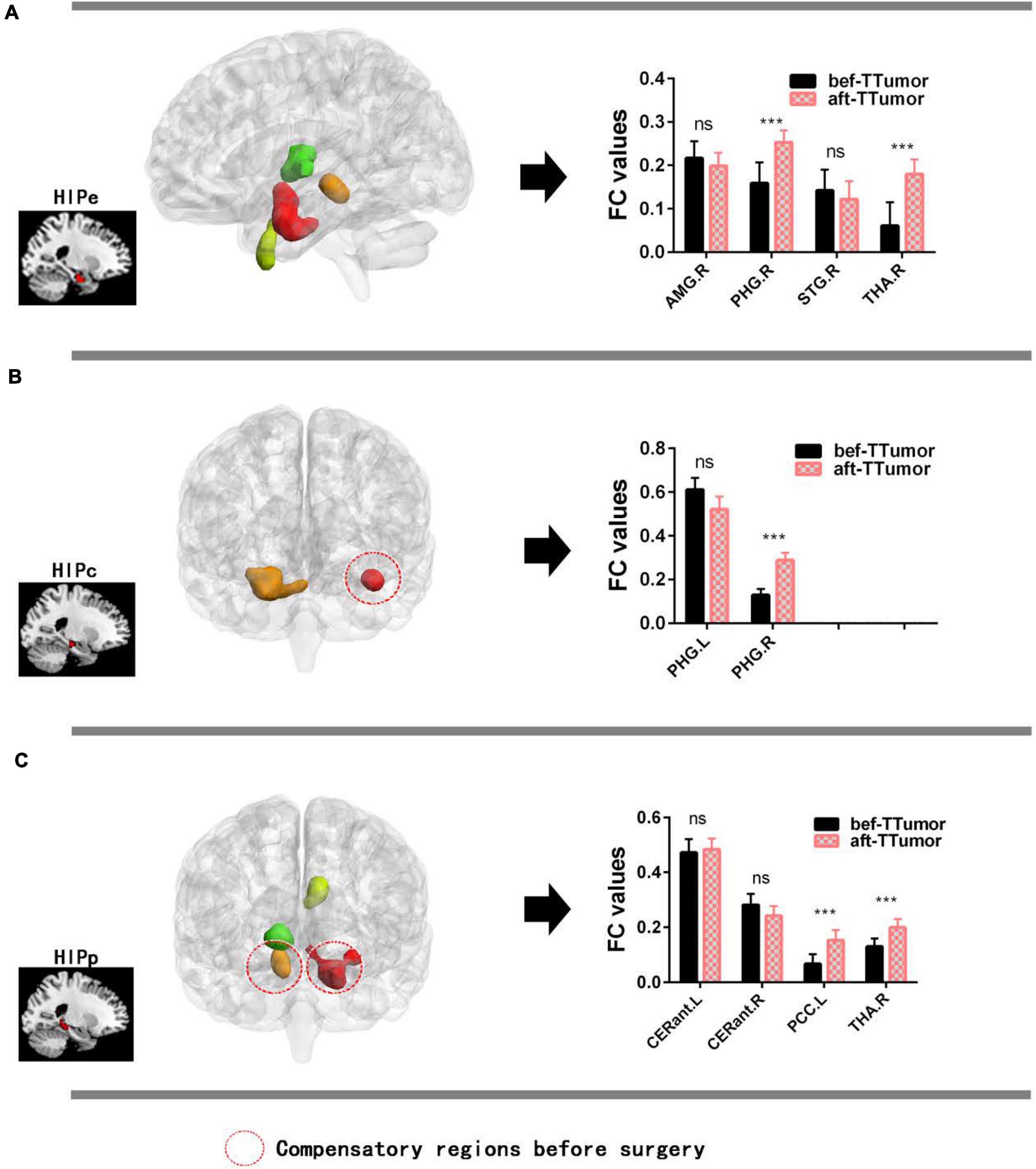
Figure 4. Changes on FC of altered hippocampal subnetworks in TTumor patients between before and after surgery in the second cohort controlling for age, sex, and education. (A) Bar chart showing quantitative effects on the HIPe subnetwork of TTumor patients after surgery after controlling for age, sex, and education. (B) Bar chart shows the quantitative effects on the HIPc subnetwork of TTumor patients after surgery after controlling for age, sex, and education. (C) Bar chart demonstrating the quantitative effects on HIPp subnetwork of TTumor patients post-surgery after controlling for age, sex, and education; ns, not significant. ***p < 0.001. Red circle indicates compensatory regions before surgery. CN, healthy controls; TTumor, patients with temporal tumor (glioma); HIPe, hippocampal emotional region; HIPc, hippocampal cognitive region; HIPp, hippocampal perceptual region; FC, functional connectivity; bef-TTumor, patients with temporal tumor (glioma) before surgery; aft-TTumor, patients with temporal tumor (glioma) after surgery; AMG.R, right amygdala; PHG.R, right parahippocampal gyrus; STG.R, right superior temporal gyrus; THA.R, right thalamus; PHG.L, left parahippocampal gyrus; CERant.L, left cerebellum anterior lobe; CERant.R, right cerebellum anterior lobe; PCC.L, left posterior cingulate cortex.
Discussion
To the best of our knowledge, particularly, the three most fascinating findings need to be emphasized. Firstly, tumor invasion of the temporal lobe resulted in abnormal HIPsub network connectivity and induced functional compensation of the HIPsub networks in the first cohort. Secondly, the altered HIPsub network connectivity patterns had a high power for discriminating TTumor patients from CN on an individual subject basis. Finally, this study discovered that the FC of functional compensation regions in TTumor patients did not change before and after surgery in the second cohort. Therefore, the unique contribution of this study was to identify functional compensation within the HIPsub network among patients with preoperative temporal glioma, which does not appear to be decompensated with tumor resection.
A highlight of this work is that when there is tumor invasion of the temporal lobe within HIPsub networks, TTumor patients demonstrated significantly different abnormal patterns in three HIPsub networks, which had the high power to discriminate TTumor patients from CN on an individual subject basis. On the one hand, these results validate this view on functional heterogeneity and different FC patterns present in the hippocampal subregion (Davachi, 2006; Robinson et al., 2015; Chen et al., 2016, 2020, 2022). On the other hand, this result also suggests that there is tumor invasion of the temporal lobe, which can affect brain function in a network form, and is consistent with prior studies (Bartolomei et al., 2006; He et al., 2007; Guggisberg et al., 2008; Alstott et al., 2009; Pravata et al., 2011; Liu et al., 2019, 2020b; Yuan et al., 2019). Furthermore, these results can enhance effective tools for early screening of tumors, and develop neurorehabilitation strategies for TTumor patients. Interestingly, we discovered that FC decreased in brain regions of the functional hippocampal subnetwork caused by tumor invasion of the temporal lobe. However, it increased after tumor resection. Therefore, it is reasonable to speculate that the decrease in FC of these subhippocampal networks caused by tumor invasion of the temporal lobe may be a temporary brain functional abnormality that is caused by the tumor mass. These results can further provide some theoretical basis for surgical planning of the resection range in clinical temporal lobe patients.
The unique contribution of this study is that tumor invasion of the temporal lobe was found toinduce functional compensation of the HIPsub networks in TTumor patients. This study found a spatial distance between these compensatory areas (i.e., left parahippocampal gyrus and bilateral cerebellum anterior lobes) and tumor areas. Indeed, many studies have reported that tumor invasion affects not only the tumor area but also the functional reorganization of the distal brain regions of the lesion (Thiel et al., 2001; Duffau, 2006; Desmurget et al., 2007). This may be because the effects of tumor invasion are network-based forms, rather than isolated brain regions (Vigneau et al., 2006; Xiang et al., 2010; Briganti et al., 2012; Liu et al., 2020b). These results suggest that tumor invasion of the temporal lobe helps induce functional compensation in the distal and proximal parts of the lesion in a network-based form. Furthermore, and most importantly, we discovered that functional compensation of the hippocampal subnetwork caused by tumor invasion of the temporal lobe in TTumor patients remained after tumor resection. This suggests that the compensatory mechanism formed during tumor invasion of the temporal lobe is stable, and not caused by tumor mass. Instead, it is a new mechanism to compensate for brain function, which will not be decompensated with tumor resection. One of the explanations may be that prolonged tumor invasion induces neuroplasticity of the central nervous system, which sustains cognitive function within the brain (Payne and Lomber, 2001; Voytek et al., 2010; Duffau, 2014). However, we need to be careful in coming to this conclusion as our observation point was only 1 week after surgery, and so we only confirmed the stability of this short-term compensation. Future long-term follow-up studies should be used to investigate and assess the stability of this compensation over time.
This study also has several limitations. Firstly, this study looked at only one point in time, 1 week after surgery, and this cannot verify long-term compensation for functional compensation. In the future, longitudinal studies are needed to confirm the stability and persistence of functional compensation within the HIPsub networks in TTumor patients. Secondly, the second cohort used in this study was a relatively small pre- and post-surgical sample. A larger sample study is required in the future to validate this compensatory mechanism.
Conclusion
This study identifies that tumor invasion of the temporal lobe led to abnormal HIPsub network connectivity, and induced functional compensation of the HIPsub networks in TTumor patients, which can discriminate TTumor patients from CN on an individual subject basis. It has been suggested that the fine hippocampal subregion was more sensitive to reveal the functional compensation induced by tumor invasion of the temporal lobe. This study further validated the stability and persistence of this functional compensation in TTumor patients after surgical resection of the tumor. This study has significant implications that provide a novel avenue that avoids damage to the compensation of HIPsub networks, may protect against a brain function decline after surgery, and can improve the quality of life of patients.
Data availability statement
The datasets presented in this study can be found in online repositories. The names of the repository/repositories and accession number(s) can be found in the article/Supplementary material.
Ethics statement
The studies involving human participants were reviewed and approved by the Human Participants Ethics Committee of the Affiliated Brain Hospital of Nanjing Medical University. The patients/participants provided their written informed consent to participate in this study.
Author contributions
YHZ and HX undertook the data analysis and wrote the manuscript. YL, KY, and YJZ acquired the data. HYL designed the study and provided infrastructure. YHZ, HX, and HYL supervised the data analysis. All authors contributed to and approved the final manuscript.
Funding
This study was supported by the National Natural Science Foundation of China (No. 81972350), the Medical Scientific Project of Jiangsu Provincial Commission of Health and Family Planning (No. M2021003), and the Medical Scientific and Technologic Development Project of Nanjing (No. YKK20097).
Conflict of interest
The authors declare that the research was conducted in the absence of any commercial or financial relationships that could be construed as a potential conflict of interest.
The reviewer Y-CC declared a shared affiliation with the authors to the handling editor at the time of review.
Publisher’s note
All claims expressed in this article are solely those of the authors and do not necessarily represent those of their affiliated organizations, or those of the publisher, the editors and the reviewers. Any product that may be evaluated in this article, or claim that may be made by its manufacturer, is not guaranteed or endorsed by the publisher.
Supplementary material
The Supplementary Material for this article can be found online at: https://www.frontiersin.org/articles/10.3389/fnins.2022.991406/full#supplementary-material
Footnotes
References
Alstott, J., Breakspear, M., Hagmann, P., Cammoun, L., and Sporns, O. (2009). Modeling the impact of lesions in the human brain. PLoS Comput. Biol. 5:e1000408. doi: 10.1371/journal.pcbi.1000408
Ashburner, J., and Friston, K. J. (2009). Computing average shaped tissue probability templates. NeuroImage 45, 333–341. doi: 10.1016/j.neuroimage.2008.12.008
Bai, T., Wei, Q., Xie, W., Wang, A., Wang, J., Ji, G. J., et al. (2019). Hippocampal-subregion functional alterations associated with antidepressant effects and cognitive impairments of electroconvulsive therapy. Psychol. Med. 49, 1357–1364. doi: 10.1017/S0033291718002684
Bartolomei, F., Bosma, I., Klein, M., Baayen, J. C., Reijneveld, J. C., Postma, T. J., et al. (2006). How do brain tumors alter functional connectivity? A magnetoencephalography study. Ann. Neurol. 59, 128–138. doi: 10.1002/ana.20710
Briganti, C., Sestieri, C., Mattei, P. A., Esposito, R., Galzio, R. J., Tartaro, A., et al. (2012). Reorganization of functional connectivity of the language network in patients with brain gliomas. AJNR. Am. J. Neuroradiol. 33, 1983–1990. doi: 10.3174/ajnr.A3064
Chen, J., Chen, R., Xue, C., Qi, W., Hu, G., Xu, W., et al. (2022). Hippocampal-Subregion Mechanisms of Repetitive Transcranial Magnetic Stimulation Causally Associated with Amelioration of Episodic Memory in Amnestic Mild Cognitive Impairment. J. Alzheimer’s Dis. 85, 1329–1342. doi: 10.3233/JAD-210661
Chen, J., Duan, X., Shu, H., Wang, Z., Long, Z., Liu, D., et al. (2016). Differential contributions of subregions of medial temporal lobe to memory system in amnestic mild cognitive impairment: Insights from fMRI study. Sci. Rep. 6:26148. doi: 10.1038/srep26148
Chen, J., Ma, N., Hu, G., Nousayhah, A., Xue, C., Qi, W., et al. (2020). rTMS modulates precuneus-hippocampal subregion circuit in patients with subjective cognitive decline. Aging 13, 1314–1331. doi: 10.18632/aging.202313
Davachi, L. (2006). Item, context and relational episodic encoding in humans. Curr. Opin. Neurobiol. 16, 693–700. doi: 10.1016/j.conb.2006.10.012
Desmurget, M., Bonnetblanc, F., and Duffau, H. (2007). Contrasting acute and slow-growing lesions: A new door to brain plasticity. Brain 130, 898–914. doi: 10.1093/brain/awl300
Di, G., Tan, M., Xu, R., Zhou, W., Duan, K., Hu, Z., et al. (2022). Altered Structural and Functional Patterns Within Executive Control Network Distinguish Frontal Glioma-Related Epilepsy. Front. Neurosci. 16:916771. doi: 10.3389/fnins.2022.916771
Duffau, H. (2006). New concepts in surgery of WHO grade II gliomas: Functional brain mapping, connectionism and plasticity–a review. J. Neurooncol. 79, 77–115. doi: 10.1007/s11060-005-9109-6
Duffau, H. (2014). Diffuse low-grade gliomas and neuroplasticity. Diagn. Interv. Imaging 95, 945–955. doi: 10.1016/j.diii.2014.08.001
Guggisberg, A. G., Honma, S. M., Findlay, A. M., Dalal, S. S., Kirsch, H. E., Berger, M. S., et al. (2008). Mapping functional connectivity in patients with brain lesions. Ann. Neurol. 63, 193–203. doi: 10.1002/ana.21224
He, B. J., Snyder, A. Z., Vincent, J. L., Epstein, A., Shulman, G. L., and Corbetta, M. (2007). Breakdown of functional connectivity in frontoparietal networks underlies behavioral deficits in spatial neglect. Neuron 53, 905–918. doi: 10.1016/j.neuron.2007.02.013
Hu, G., Hu, X., Yang, K., Liu, D., Xue, C., Liu, Y., et al. (2020). Restructuring of contralateral gray matter volume associated with cognition in patients with unilateral temporal lobe glioma before and after surgery. Hum. Brain Mapp. 41, 1786–1796. doi: 10.1002/hbm.24911
Liu, Y., Hu, G., Yu, Y., Jiang, Z., Yang, K., Hu, X., et al. (2020b). Structural and Functional Reorganization Within Cognitive Control Network Associated With Protection of Executive Function in Patients With Unilateral Frontal Gliomas. Front. Oncol. 10:794. doi: 10.3389/fonc.2020.00794
Liu, D., Chen, J., Hu, X., Hu, G., Liu, Y., Yang, K., et al. (2020a). Contralesional homotopic functional plasticity in patients with temporal glioma. J. Neurosurg. 10:794. doi: 10.3171/2019.11.JNS191982
Liu, Y., Yang, K., Hu, X., Xiao, C., Rao, J., Li, Z., et al. (2020c). Altered Rich-Club Organization and Regional Topology Are Associated With Cognitive Decline in Patients With Frontal and Temporal Gliomas. Front. Hum. Neurosci. 14:23. doi: 10.3389/fnhum.2020.00023
Liu, D., Hu, X., Liu, Y., Yang, K., Xiao, C., Hu, J., et al. (2019). Potential Intra- or Cross-Network Functional Reorganization of the Triple Unifying Networks in Patients with Frontal Glioma. World Neurosurg. 128:e732–e743. doi: 10.1016/j.wneu.2019.04.248
Ostrom, Q. T., Bauchet, L., Davis, F. G., Deltour, I., Fisher, J. L., Langer, C. E., et al. (2014). The epidemiology of glioma in adults: A “state of the science” review. Neuro Oncol. 16, 896–913.
Ostrom, Q. T., Cioffi, G., Gittleman, H., Patil, N., Waite, K., Kruchko, C., et al. (2019). Primary Brain and Other Central Nervous System Tumors Diagnosed in the United States in 2012-2016. Neuro Oncol. 21:v1–v100. doi: 10.1093/neuonc/noz150
Payne, B. R., and Lomber, S. G. (2001). Reconstructing functional systems after lesions of cerebral cortex. Nat. Rev. Neurosci. 2, 911–919. doi: 10.1038/35104085
Power, J. D., Barnes, K. A., Snyder, A. Z., Schlaggar, B. L., and Petersen, S. E. (2012). Spurious but systematic correlations in functional connectivity MRI networks arise from subject motion. NeuroImage 59, 2142–2154. doi: 10.1016/j.neuroimage.2011.10.018
Pravata, E., Sestieri, C., Mantini, D., Briganti, C., Colicchio, G., Marra, C., et al. (2011). Functional connectivity MR imaging of the language network in patients with drug-resistant epilepsy. AJNR. Am. J. Neuroradiol. 32, 532–540.
Robinson, J. L., Barron, D. S., Kirby, L. A., Bottenhorn, K. L., Hill, A. C., Murphy, J. E., et al. (2015). Neurofunctional topography of the human hippocampus. Hum. Brain Mapp. 36, 5018–5037.
Thiel, A., Herholz, K., Koyuncu, A., Ghaemi, M., Kracht, L. W., and Habedank, B. (2001). Plasticity of language networks in patients with brain tumors: A positron emission tomography activation study. Ann. Neurol. 50, 620–629. doi: 10.1002/ana.1253
Van Dijk, K. R., Sabuncu, M. R., and Buckner, R. L. (2012). The influence of head motion on intrinsic functional connectivity MRI. NeuroImage 59, 431–438.
Vigneau, M., Beaucousin, V., Herve, P. Y., Duffau, H., Crivello, F., Houde, O., et al. (2006). Meta-analyzing left hemisphere language areas: Phonology, semantics, and sentence processing. NeuroImage 30, 1414–1432. doi: 10.1016/j.neuroimage.2005.11.002
Voytek, B., Davis, M., Yago, E., Barcelo, F., Vogel, E. K., and Knight, R. T. (2010). Dynamic neuroplasticity after human prefrontal cortex damage. Neuron 68, 401–408.
Xiang, H. D., Fonteijn, H. M., Norris, D. G., and Hagoort, P. (2010). Topographical functional connectivity pattern in the perisylvian language networks. Cereb. Cortex 20, 549–560. doi: 10.1093/cercor/bhp119
Yuan, B., Zhang, N., Yan, J., Cheng, J., Lu, J., and Wu, J. (2019). Resting-state functional connectivity predicts individual language impairment of patients with left hemispheric gliomas involving language network. Neuroimage Clin. 24:102023. doi: 10.1016/j.nicl.2019.102023
Zhang, N., Xia, M., Qiu, T., Wang, X., Lin, C. P., Guo, Q., et al. (2018). Reorganization of cerebro-cerebellar circuit in patients with left hemispheric gliomas involving language network: A combined structural and resting-state functional MRI study. Hum. Brain Mapp. 39, 4802–4819. doi: 10.1002/hbm.24324
Keywords: temporal glioma, hippocampal-subregion, functional connectivity, pattern classification, functional compensation
Citation: Zhang Y, Xu H, Liu Y, Yang K, Zou Y and Liu H (2022) Stable functional compensation within hippocampal-subregion networks in patients with temporal glioma before and after surgery. Front. Neurosci. 16:991406. doi: 10.3389/fnins.2022.991406
Received: 11 July 2022; Accepted: 08 August 2022;
Published: 01 September 2022.
Edited by:
Jiaojian Wang, Kunming University of Science and Technology, ChinaReviewed by:
Yu-Chen Chen, Nanjing Medical University, ChinaRui Liu, Capital Medical University, China
Meng Zhang, Xinxiang Medical University, China
Copyright © 2022 Zhang, Xu, Liu, Yang, Zou and Liu. This is an open-access article distributed under the terms of the Creative Commons Attribution License (CC BY). The use, distribution or reproduction in other forums is permitted, provided the original author(s) and the copyright owner(s) are credited and that the original publication in this journal is cited, in accordance with accepted academic practice. No use, distribution or reproduction is permitted which does not comply with these terms.
*Correspondence: Hongyi Liu, aHlsaXUxOEAxMjYuY29t
†These authors have contributed equally to this work and share first authorship