- 1State Key Laboratory of Medical Neurobiology, Department of Radiology, Huashan Hospital, Fudan University, Shanghai, China
- 2Department of Radiology, Ningbo First Hospital, Ningbo, China
- 3Department of Neurology, Huashan Hospital, Fudan University, Shanghai, China
- 4National Center for Neurological Disorders, Shanghai, China
- 5Center for Shanghai Intelligent Imaging for Critical Brain Diseases Engineering and Technology Research, Huashan Hospital, Fudan University, Shanghai, China
Objectives: To identify preoperative prognostic factors for acute ischemic stroke (AIS) patients receiving mechanical thrombectomy (MT) and compare the performance of quantitative collateral score (qCS) and visual collateral score (vCS) in outcome prediction.
Methods: Fifty-five patients with AIS receiving MT were retrospectively enrolled. qCS was defined as the percentage of the volume of collaterals of both hemispheres. Based on the dichotomous outcome assessed using a 90-day modified Rankin Scale (mRS), we compared qCS, vCS, age, sex, National Institute of Health stroke scale score, etiological subtype, platelet count, international normalized ratio, glucose levels, and low-density lipoprotein cholesterol (LDL-C) levels between favorable and unfavorable outcome groups. Logistic regression analysis was performed to determine the effect on the clinical outcome. The discriminatory power of qCS, vCS, and their combination with cofounders for determining favorable outcomes was tested with the area under the receiver-operating characteristic curve (AUC).
Results: vCS, qCS, LDL-C, and age could all predict clinical outcomes. qCS is superior over vCS in predicting favorable outcomes with a relatively higher AUC value (qCS vs. vCS: 0.81 vs. 0.74) and a higher sensitivity rate (qCS vs. vCS: 72.7% vs. 40.9%). The prediction power of qCS + LDL-C + age was best with an AUC value of 0.91, but the accuracy was just increased slightly compared to that of qCS alone.
Conclusion: Collateral scores, LDL-C and age were independent prognostic predictors for patients with AIS receiving MT; qCS was a better predictor than vCS. Furthermore, qCS + LDL-C + age offers a strong prognostic prediction power and qCS alone was another good choice for predicting clinical outcome.
Introduction
The use of mechanical thrombectomy (MT) has increased dramatically since several large randomized clinical trials have confirmed that neurological outcomes of patients with acute ischemic stroke (AIS) were better when treated with MT than when treated with other methods (Albers et al., 2018; Nogueira et al., 2018; Powers et al., 2019). However, in clinical practice, the clinical outcome remains unsatisfying with higher rate of moderate to severe disability at discharge (79%) and higher 3-month mortality (29%) (Wollenweber et al., 2019; Qureshi et al., 2020). In addition, the cost of MT can be prohibitively expensive; therefore, it is particularly important to identify preoperative factors for the prediction of postoperative outcome in patients eligible for MT treatment; this shall help to avoid over-treatment as well.
Previous studies have shown that collateral circulation plays an important role in the prognosis of patients with AIS, and patients with good collaterals have a high rate of functional independence (Berkhemer et al., 2016; Lu et al., 2019). However, de Havenon et al. (2019) reported that collateral circulation only affected the infarct core volume and infarct rate and was not a predictor of neurological prognosis or mortality after MT. Collateral circulation was most commonly evaluated by manually performed visual grading of computed tomography angiography (CTA) images; these findings are reflected as the visual collateral score (vCS). This method requires experienced neuroradiologists and has frequent intra-observer disagreement (McVerry et al., 2012; Cui et al., 2021). Such inaccurate methods may limit the prognostic efficacy of collateral circulation, and a reliable quantitative collateral score (qCS) method is needed (Shi et al., 2019). Furthermore, the robustness of qCS in indicating the prognosis of patients with AIS receiving MT remains unclear. Low-density lipoprotein cholesterol (LDL-C) is known as bad cholesterol and is associated with increased inflammatory response, which reflects as increased incidences of vascular diseases and worsened stroke outcomes (Kim and Cho, 2021). Therefore, whether LDL-C can predict the stroke outcome needs to be investigated.
In this study, we quantified the collateral volume and acquired qCS using the ITK-SNAP software. The study was aimed at identifying preoperative prognostic factors for patients with AIS receiving MT, and demonstrating whether collaterals assessed by qCS could predict clinical outcomes better than vCS.
Materials and methods
Study population
From January 2018 to May 2021, we retrospectively selected consecutive patients who presented with symptoms of AIS within 24 h of onset and those who underwent MT from our single-institution stroke center. This study was reviewed and approved by the local institutional review board, and the need for written informed consent was waived owing to the retrospective design of the study and anonymization of the data. The inclusion criteria were as follows: (1) patient age > 18 years; (2) premorbid modified Rankin Scale (mRS) score ≤ 2; (3) occlusions in the M1 segment of the middle cerebral artery (MCA) and/or intracranial internal carotid artery (ICA); and (4) preinterventional computed tomography perfusion (CTP) performed for patients within 6–24 h from the time last known well with an MIStar-determined ischemic core volume < 70 mL, mismatch ratio ≥ 1.8, and mismatch volume ≥ 15 mL. The exclusion criteria were as follows: (1) intracranial hemorrhage identified by non-contrast computed tomography (NCCT); (2) a low-density area greater than 1/3rd of the MCA territory on NCCT; (3) occlusion of other intracranial arteries or stenosis of MCA; (4) a history of a moderate to large stroke in the contralateral hemisphere resulting in a measurable decrease in vasculature; and (5) CTA or CTP images with obvious motion artifacts or improper phase; (6) Moyamoya disease; (7) incomplete clinical data.
Image acquisition
Patients with symptoms of AIS underwent the imaging protocol according to the early patient management guidelines (Powers et al., 2019). CTP was not required for selecting suitable patients within 6 h from onset but was required for selecting suitable patients within 6–24 h from onset. Images were obtained using a 64-multislice CT scanner (Discovery CT750 HD, GE Healthcare) in the order of NCCT, CTP, and CTA. Single-phase CTA was performed during the administration of 80 mL of non-ionic iodine contrast agent (ioversol 320 mgI/mL, Jiangsu Hengrui Medicine Co., Ltd., China) at the rate of 4 mL/s, followed by the administration of 40 mL of saline at the same rate. The parameters were as follows: tube voltage 140 kVp, tube current 630 mA, rotation time 0.5 s, and slice thickness 0.625 mm; the area covered was from the aortic arch to the top of the cranium. CTP was obtained using volume shuttle scanning started with a 5–10-s delay after contrast adjustment. Acquisition parameters for CTP were tube voltage 100 kVp, tube current 400 mA, rotation time 0.4 s, and slice thickness 5 mm; the area covered was from the base to the top of the skull.
Image analysis
Axial, coronal, and sagittal 20-mm-thick maximum intensity projection images were reformatted from CTA images. Two experienced neuroradiologists who were blinded to the clinical and CTP data assessed the vCS independently using a previously reported, predefined scoring system (Tan et al., 2007). For disputed cases, an experienced senior neuroradiologist who was also blinded to the data made the final decision. Considering the contralateral side as the reference, the scoring system was divided into four points according to the filling proportion of collaterals in the occluded territory: 0 = absent collaterals (0%), 1 = poor collateral circulation (≤ 50%), 2 = moderate collateral circulation (50--100%, not including 100%), and 3 = good collateral circulation (100%). qCS was defined as the percentage of the volume of collaterals in the occluded area divided by the volume of vessels in the corresponding area on the unaffected side. The vessel volume was obtained by manual segmentation on CTA images using the open-source software ITK-SNAP (version 3.8.0),1 and the annotation was performed by the first author of this paper and another experienced neuroradiologist under the supervision of the senior neuroradiologist. Different segmental arteries in the MCA territories were labeled by user-defined colors based on the anatomy (Shapiro et al., 2020). Then, the vessel volume of each segment was automatically calculated (Figure 1). The M3-4 segment and pial collaterals share the same label “M3-distal,” and qCS (M3-distal) represents qCS of the segment of M3 to pial collaterals. The qCS of the whole vessel was presented as qCS (total). The segmental volume and qCS were compared. A commercial software, MIStar (Apollo Medical Imaging Technology, Melbourne, Australia), was used to obtain CTP parameters, including infarct core, ischemic volume, mismatch volume (ischemic volume–infarct core), and mismatch ratio (ischemic volumes/infarct core).
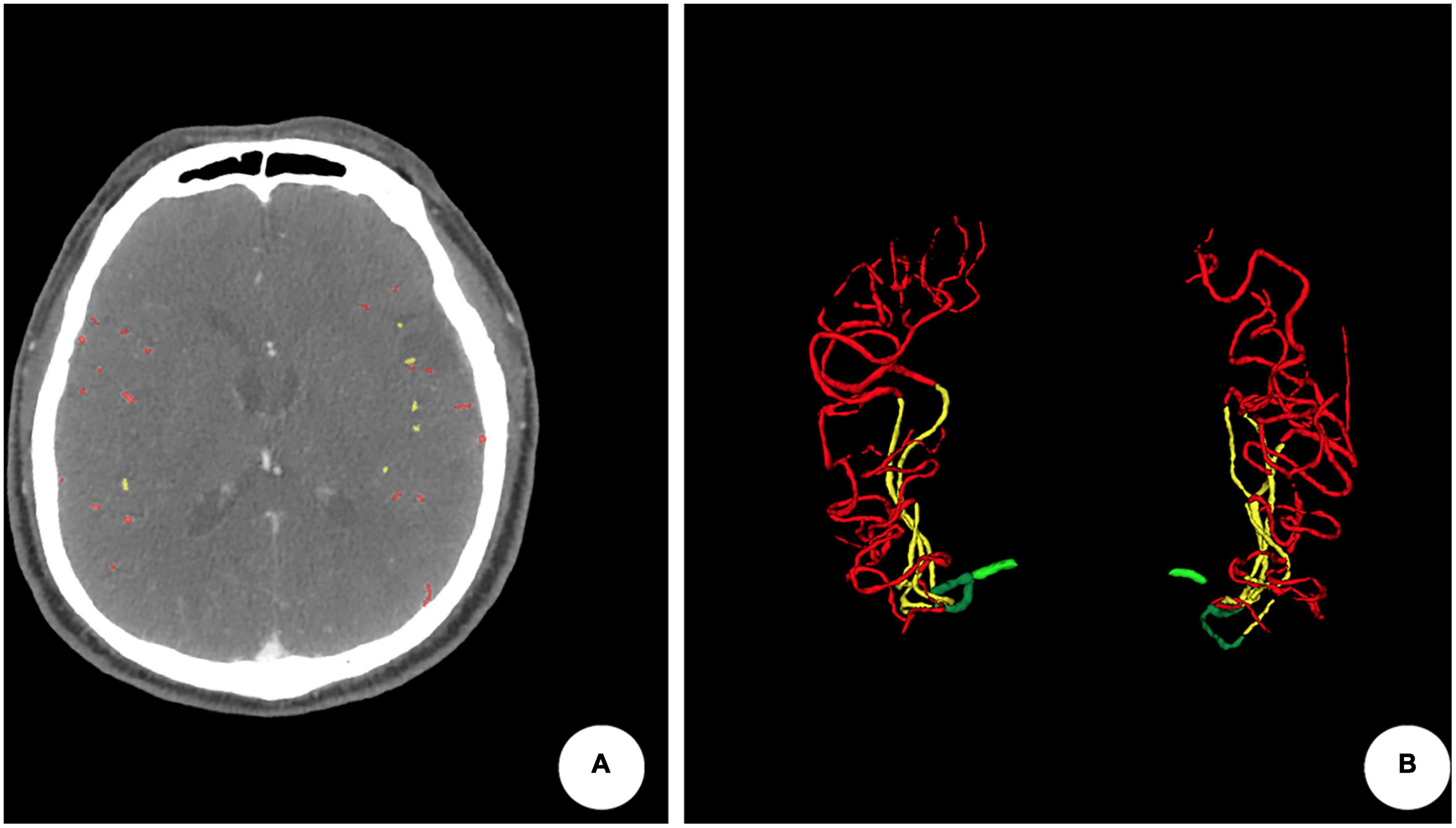
Figure 1. An example of quantitative collateral scoring using the ITK-SNAP software. (A) Segmentation of arteries in the middle cerebral artery territories using different labels. The quantitative collateral score was 98.74%. (B) 3D representation of the segmented vasculature with occlusion of the left M1 segment.
Clinical evaluation
Baseline clinical data were collected by a neurologist specializing in stroke. Age, sex, admission National Institute of Health stroke scale (NIHSS) score, etiological subtype [trial of ORG 10172 in acute stroke treatment (TOAST) classification], and related laboratory markers (platelet, international normalized ratio, glucose levels, and LDL-C) on admission were recorded. Reperfusion was rated according to the modified Treatment In Cerebral Ischemia (mTICI) score (Goyal et al., 2014) during operation, and successful reperfusion was defined as an mTICI score of ≥ 2b. The patients were divided into two groups according to their 90-day clinical outcome: the favorable outcome group (mRS ≤ 2) and the unfavorable outcome group (mRS > 2).
Statistical analysis
Statistical analysis was performed using the IBM SPSS Statistics software (Version: 26.0.0.0). The Shapiro–Wilk test was used for normality test. Continuous variables were presented as mean ± standard deviation or median (interquartile range). Categorical variables were expressed as frequency and percentage. Spearman correlation coefficients and intraclass correlation efficient (ICC) were performed to compare qCS and vCS. The Chi-square or Fisher’s exact test and the independent samples t-test or Mann–Whitney U-test was used to compare the differences between favorable and unfavorable outcome groups. Binary logistic regression analysis was performed to determine the effect on the clinical outcome. The variables with P < 0.1 at univariable analysis were included in the multivariable logistic regression analysis. Since both qCS and vCS measured the same factor, they were, respectively, put into the model with other statistically significant parameters. Receiver operating characteristic (ROC) analysis was performed to test the performance of qCS, vCS, and their combination with cofounders in determining favorable outcomes. A P-value of < 0.05 was considered statistically significant.
Results
Cohort characterization
A total of 55 patients (median age, 74 years; 38 men) with acute M1 and/or ICA occlusion who underwent MT were enrolled (Figure 2). The median baseline NIHSS score of the patients was 13; 41 patients were evaluated as having moderate-to-good collateral circulation by vCS (Tan score ≥ 2). Herein, 22/55 patients belonged to the favorable outcome group, and 33/55 patients belonged to the unfavorable outcome group; among these 33 patients, 25 had to live dependently and 8 died. The clinical characteristics of all enrolled patients are presented in Table 1. The comparison reveals that patients in the unfavorable outcome group were significantly older and had higher baseline NIHSS score, higher glucose levels, lower LDL-C levels, and worse collateral circulation than patients in the favorable outcome group (Table 1).
Agreement between visual collateral score and quantitative collateral score
The distribution of qCS (total) and qCS (M3-distal) per vCS is presented in Figure 3. The results of correlation analysis are illustrated in Table 2. There was merely a moderate correlation between qCS (M2) and vCS; while, qCS (total) and qCS (M3-distal) had substantial correlation with vCS (r = 0.78, r = 0.76, respectively; all P < 0.001). And qCS (M3-distal) produced a little higher agreement with the consensus vCS than qCS (total) did [ICC: 0.69 (0.52–0.80) vs. 0.50 (0.28–0.68), all P < 0.001]. Although the reliability was of only moderate degree between qCS and vCS, the agreement for identifying favorable collateral scores (Tan score ≥ 2) compared to consensus were 97.56% for qCS (M3-distal) and 92.68% for qCS (total), respectively (Figure 4).
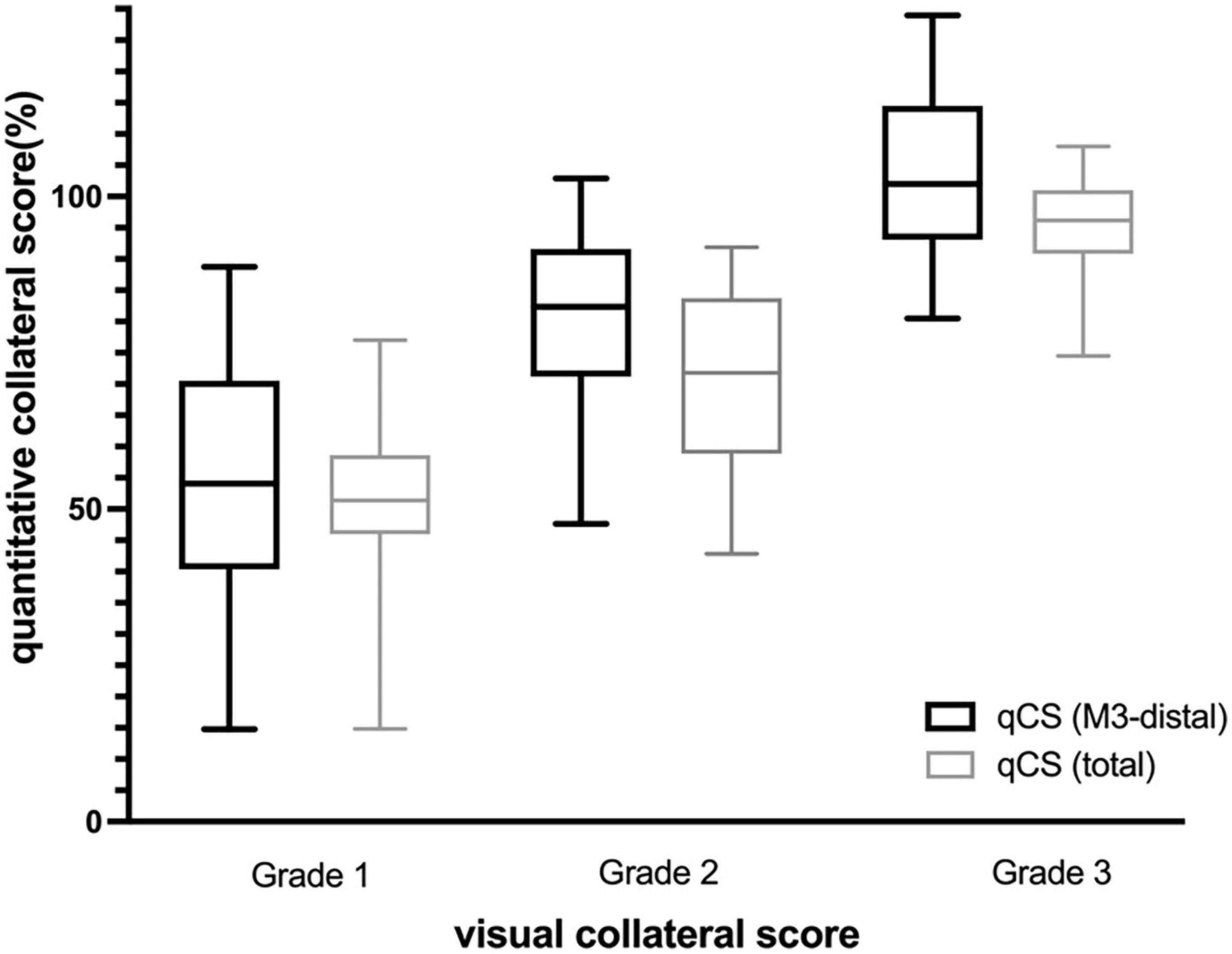
Figure 3. Distribution of qCS per visual collateral score (Box- and-whisker plot). qCS, quantitative collateral score.
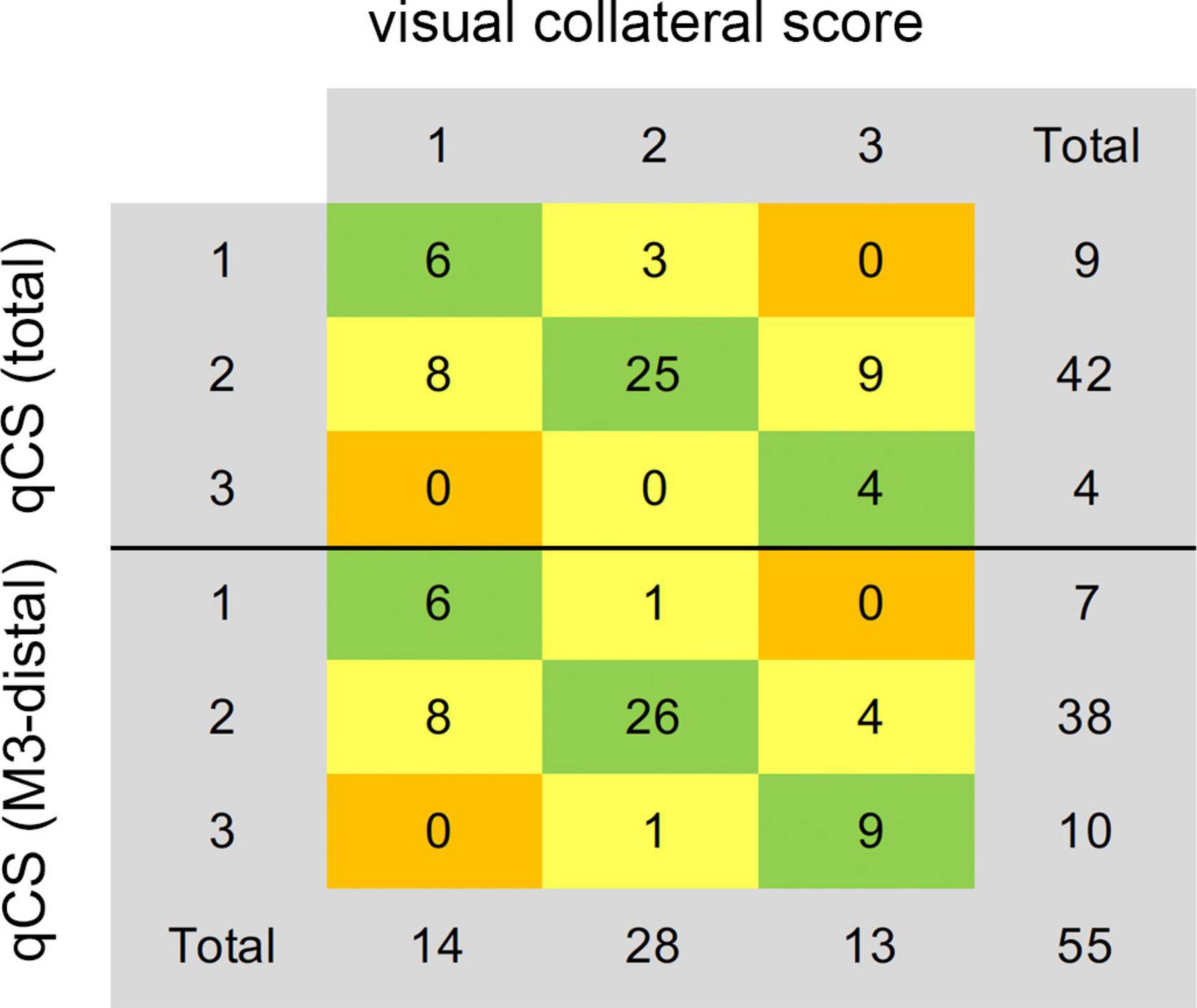
Figure 4. Agreement of the consensus visual collateral score (Tan score) with qCS (total) and qCS (M3-distal). qCS was divided into four points according to the classification criteria of vCS. qCS, quantitative collateral score.
Quantitative collateral score (M3-distal) is superior over visual collateral score in predicting clinical outcome
The Spearman correlation analysis implied that qCS (M3-distal) correlated a slightly stronger with 90-day mRS than vCS did (r = –0.50, P < 0.001; r = –0.47, P < 0.001; respectively) (Table 2). The predictive power of qCS (M3-distal) was superior to that of vCS [qCS (M3-distal): area under the receiver-operating characteristic curve (AUC), 0.81 (0.69–0.92), P < 0.001; vCS: AUC, 0.74 (0.61–0.87), P = 0.003] with a higher sensitivity [qCS (M3-distal) vs. vCS: 72.7% vs. 40.9%]. And the optimal cutoff point was 88.29% (72.7% sensitivity, 81.8% specificity) with qCS (M3-distal), and 1.5 (95.5% sensitivity, 39.4% specificity) with vCS.
Identification of outcome-related risk factors
Based on the results of agreement and correlation analysis, qCS (M3-distal) rather than qCS (total) was preferred. Age, baseline NIHSS score, glucose levels, LDL-C levels, and collateral circulation were statistically significant in the univariate analysis (Supplementary Table 1). These factors, then, were integrated into the multivariable logistic regression models. The analysis showed that qCS, vCS, LDL-C, and age were independent predictors of clinical outcome receiving MT (Table 3). After adjusting for LDL-C and age, a 1% increase in qCS (M3-distal) corresponded to a 10% better outcome of patients with AIS. The adjusted odds ratio (OR) for vCS in predicting favorable outcome was 3.36.
Prognosis prediction model
The performances of different models are presented in Table 4. Herein, qCS (M3-distal) and vCS predicted favorable outcomes with accuracy of 78.2 and 69.1%, respectively. And the models based on qCS (M3-distal) to measure collaterals are generally more sensitive than those models based on vCS. Although the largest AUC value of 0.91 was achieved in the model of a combination of qCS (M3-distal), LDL-C and age, the accuracy was just increased slightly with 3.6% compared to that of qCS (M3-distal) alone.
Discussion
In this study, we identified that collateral scores, LDL-C and age were independent preoperative predictors of the outcome of patients with AIS receiving MT and demonstrated that qCS performed better than vCS (Tan score) in predicting the clinical outcome.
Many predictors of the clinical outcome of patients with AIS receiving MT have been reported so far, including age, stroke severity, 24-h blood pressure after MT, 24-h NIHSS score after interventional therapy, and final infarct volume (Cernik et al., 2019; Wollenweber et al., 2019; Brugnara et al., 2020). However, some of these factors are postoperative indicators that cannot help with or provide timely advice before clinical decision-making. Our study reported that higher collateral scores, higher LDL-C and younger age were the independent preoperative predictors for a better neurologic outcome. It has been widely accepted that LDL-C has an atherogenic effect on large vessels; however, the protective effect of hyperlipidemia on clinical outcome in patients with AIS is not widely acknowledged. Cuadrado-Godia et al. (2009) reported that higher total cholesterol and LDL-C levels were associated with better outcomes in male patients after the first AIS. There could be underlying mechanisms to this effect: antioxidation and endothelial protection, namely neutralizing free radicals and down-regulation of the vascular endothelial growth factor, respectively (Yeramaneni et al., 2017). Higher but within the normal range LDL-C was also found in the 90-day favorable outcome group in our current study, which adds to the existing evidence of the protective effect of lipid paradox (Cheng et al., 2018). Another prognostic factor with statistical significance in the logistic regression model was age, which was consistent with previous studies that younger age was associated with a good outcome with OR 1.06 (Wollenweber et al., 2019; Yao et al., 2020).
Our study also confirmed that patients with AIS having good collaterals would have lesser neurological impairment, which was in line with many previous studies (Berkhemer et al., 2016; Anadani et al., 2021; Uniken Venema et al., 2022). And a patient with a qCS (M3-distal) of < 88.29% would be predicted to have an ominous prognosis, with a cutoff higher than the cutoff vCS (50%) (Lu et al., 2019), even if the tissue perfusion parameters met the criteria for MT. In predicting clinical outcome, qCS (M3-distal) is superior over vCS with a higher AUC value and a higher sensitivity. The reason for the discrepancy may lie in the nature of evaluation methods, namely quantitative method vs. categorical method. The conventional vCS systems, which are based on traditional or dynamic CTA, depend on manual visual assessment, making them prone to both intra- and inter-observer variations (McVerry et al., 2012; Aktar et al., 2020; Cui et al., 2021). Several vCS systems have been proposed. The prognostic power of different scoring systems varied in different studies, and was far from satisfactory with AUCs ranging from 0.59 to 0.77 (Berkhemer et al., 2016; Lu et al., 2019; Seker et al., 2020; Cui et al., 2021). No consensus has been reached yet in terms of the evaluation criteria.
qCS is the ratio of the collateral volume on the affected side to that on the unaffected side, which quantifies collateral circulation and may reduce the effect of observers’ experience using visual methods. Boers et al. (2018) acquired qCS using Hessian-based enhancement filters and revealed that qCS had substantial correlation with vCS (r = 0.75, P < 0.001), a similar result with our study (r = 0.78, P < 0.001). In addition, they also found that with an AUC of 0.71, qCS was slightly superior to vCS in predicting functional independence; but the difference was not statistically significant. In the subsequent study, a moderate ICC of 0.60 between qCS and vCS and an agreement of 81% in collaterals dichotomization were obtained in the agreement analysis (Wolff et al., 2022). Su et al. (2020) applied convolutional neural networks to quantify collateral circulation; the model achieved an accuracy of 80% in comparison to the vCS, and the value was increased to 90% for collaterals dichotomization. Similar results—a moderate ICC but a high agreement for identifying favorable collateral circulation (Tan score ≥ 2)—were also observed in our study. These interesting results revealed that the disagreement mainly occurred within the sets of Tan score 0 and 1 (0–50%) or Tan score 2 and 3 (50–100%). Recently, another quantitative measurement of the collateral circulation (VCCq) was proposed—the method calculating vessel volumes using the identified CT values at three selected layers on time maximum intensity projection (tMIP) CTA images (Cao et al., 2021). The study showed that both VCCq and Tan score were moderately negatively correlated with final infarct volume; however, unlike Tan score, VCCq was an independent predictive factor of the clinical outcome (OR = 0.14, P = 0.009). In addition, VCCq had a better value than Tan score in predicting the clinical outcome [VCCq: AUC, 0.93 (0.85–1.00); Tan score: AUC, 0.79 (0.68–0.89); all P < 0.001], revealing that the quantitative method is superior to vCS in predicting the prognosis, which was also verified in our current study. The adjusted OR value (3.36) of vCS in this study was relatively too large, as 1 point increase in vCS corresponded to a 236% better outcome of patients with AIS. The large span, also found in a recent study (Uniken Venema et al., 2022), reinforced the point that vCS was inferior to qCS due to its unrefined categorical method.
Although a statistically significant difference in baseline NIHSS score and admission glucose levels was observed between favorable and unfavorable outcome groups, neither of them could predict clinical outcomes. Hyperglycemia on admission in patients with AIS was usually transient and frequently caused by temporary stress (Ntaios et al., 2011). In our current study, post-stroke hyperglycemia (defined as admission glucose levels ≥ 7.8 mmol/L; Zuurbier et al., 2016; Zonneveld et al., 2017) may have mainly resulted from stress as only thirteen patients had a history of diabetes mellitus. Tziomalos et al. (2017) reported that stress hyperglycemia was not an independent predictor of poor outcome but was rather associated with a more severe stroke (admission NIHSS). In regard to NIHSS score, early neurological change or the change of NIHSS score, rather than baseline score, was significantly and independently associated with 90-day outcomes (Heitsch et al., 2021. While, some studies found that a lower baseline score was associated with a favorable outcome (Sojka et al., 2021) and the performance of NIHSS score in predicting functional outcome was time-dependent (Wu et al., 2019). Therefore, the prognostic prediction power of glucose levels and baseline NIHSS score remains controversial.
Numerous attempts have been made to accurately predict the AIS treatment outcome, and several stroke outcome prediction scores have been developed to help physicians make better therapy decisions; however, difficulties and challenges still exist. Brugnara et al. (2020) developed a machine learning-based predictive model with an AUC score of 0.86; however, it combined twelve input variables from clinical, imaging, and angiographic aspects and was inconvenient for rapid clinical evaluation. Conversely, even though the present study had only three variables involved in the prediction model, namely qCS (M3-distal) + LDL-C + age, our model offered strong prognostic prediction power with an AUC value of 0.91, which was better than that of stroke outcome prediction scores (AUC 0.70–0.86) (Matsumoto et al., 2020). Furthermore, qCS alone could also predict favorable outcome with an AUC value of 0.81 and an accuracy of 78.2%. Although the VCCq mentioned above had a superior prognostic value for clinical outcome than qCS (M3-distal), the parameter was derived from CTA images of 19 phases, which were not available for some hospitals. Our model, from the single-phase CTA, is simple and effective and has the potential to be applied in the acute clinical setting.
Our study had some limitations. First, artificial bias may be introduced in the annotation of collateral vessels. To minimize bias, repeated training and practice were conducted before annotation until the approval from the senior neuroradiologist. Second, the sample size was small at only 55 cases; however, the power values of our study were above 0.8 (ranging from 0.81 to 1.00), suggesting that the results obtained with the present sample size were reliable (Serdar et al., 2021). Prospective multicenter studies will be conducted in the future to further validate the results and address the issue of potential data bias. Although, in present study, the vessels segmentation is labor- and time-consuming which is not suited for the scenario of acute stroke management now, our results prove that collateral quantification is feasible and also widen the application range of artificial intelligence in the future.
Conclusion
Collateral scores, LDL-C and age were independent prognostic predictors in patients with AIS receiving MT, and the performance of qCS (M3-distal) was superior to that of vCS. Furthermore, qCS + LDL-C + age offers a strong prognostic prediction power and qCS (M3-distal) alone was another good choice for predicting clinical outcome.
Data availability statement
The data that support the findings of this study are available from the corresponding authors upon reasonable request.
Ethics statement
The studies involving human participants were reviewed and approved by the Ethics Committee of Huashan Hospital. Written informed consent for participation was not required for this study in accordance with the national legislation and the institutional requirements.
Author contributions
QL: conceptualization, methodology, investigation, data curation, formal analysis, and writing—original draft. HZ and JF: methodology, investigation, and data curation. XC: methodology, investigation, and formal analysis. YP: resources and formal analysis. XZ: investigation and data curation. JW: conceptualization, investigation, and data curation. DG: resources, writing—review and editing, supervision, and funding acquisition. JZ: conceptualization, formal analysis, writing—review and editing, supervision, and funding acquisition. All authors contributed to the article and approved the submitted version.
Funding
This work was supported by the Shanghai Municipal Commission of Science and Technology (grant no. 22S31905300), the Shanghai Municipal Commission of Health (grant no. 20224Z0002), the Greater Bay Area Institute of Precision Medicine (Guangzhou) (grant no. KCH2310094), the Shanghai Shuguang Program (grant no. 19SG06), and the Ningbo Municipal Bureau of Science and Technology (grant no. 2021S188).
Conflict of interest
The authors declare that the research was conducted in the absence of any commercial or financial relationships that could be construed as a potential conflict of interest.
Publisher’s note
All claims expressed in this article are solely those of the authors and do not necessarily represent those of their affiliated organizations, or those of the publisher, the editors and the reviewers. Any product that may be evaluated in this article, or claim that may be made by its manufacturer, is not guaranteed or endorsed by the publisher.
Supplementary material
The Supplementary Material for this article can be found online at: https://www.frontiersin.org/articles/10.3389/fnins.2022.980135/full#supplementary-material
Abbreviations
MT, mechanical thrombectomy; AIS, acute ischemic stroke; CTA, computed tomography angiography; vCS, visual collateral score; qCS, quantitative collateral score; LDL-C, Low-density lipoprotein cholesterol; mRS, modified Rankin Scale; MCA, middle cerebral artery; ICA, internal carotid artery; CTP, computed tomography perfusion; NCCT, non-contrast computed tomography; NIHSS, National Institute of Health stroke scale; TOAST, trial of ORG 10,172 in acute stroke treatment; mTICI, the modified Treatment In Cerebral Ischemia; ICC, intraclass correlation efficient; ROC, receiver operating characteristic; AUC, area under the curve; OR, odds ratio.
Footnotes
References
Aktar, M., Tampieri, D., Rivaz, H., Kersten-Oertel, M., and Xiao, Y. (2020). Automatic collateral circulation scoring in ischemic stroke using 4D CT angiography with low-rank and sparse matrix decomposition. Int. J. Comput. Assist. Radiol. Surg. 15, 1501–1511. doi: 10.1007/s11548-020-02216-w
Albers, G. W., Marks, M. P., Kemp, S., Christensen, S., Tsai, J. P., Ortega-Gutierrez, S., et al. (2018). Thrombectomy for Stroke at 6 to 16 hours with selection by perfusion imaging. N. Engl. J. Med. 378, 708–718.
Anadani, M., Finitsis, S., Clarençon, F., Richard, S., Marnat, G., Bourcier, R., et al. (2021). Collateral status reperfusion and outcomes after endovascular therapy: Insight from the endovascular treatment in ischemic stroke (ETIS) registry. J. Neurointerv. Surg. 14, 551–557. doi: 10.1136/neurintsurg-2021-017553
Berkhemer, O. A., Jansen, I. G., Beumer, D., Fransen, P. S., van den Berg, L. A., Yoo, A. J., et al. (2016). Collateral status on baseline computed tomographic angiography and intra-arterial treatment effect in patients with proximal anterior circulation stroke. Stroke 47, 768–776. doi: 10.1161/STROKEAHA.115.011788
Boers, A. M. M., Sales Barros, R., Jansen, I. G. H., Berkhemer, O. A., Beenen, L. F. M., Menon, B. K., et al. (2018). Value of quantitative collateral scoring on CT angiography in patients with acute ischemic stroke. AJNR Am. J. Neuroradiol. 39, 1074–1082. doi: 10.3174/ajnr.A5623
Brugnara, G., Neuberger, U., Mahmutoglu, M. A., Foltyn, M., Herweh, C., Nagel, S., et al. (2020). Multimodal predictive modeling of endovascular treatment outcome for acute ischemic stroke using machine-learning. Stroke 51, 3541–3551.
Cao, R., Qi, P., Jiang, Y., Hu, S., Ye, G., Zhu, Y., et al. (2021). Preliminary application of a quantitative collateral assessment method in acute ischemic stroke patients with endovascular treatments: A single-center study. Front. Neurol. 12:714313. doi: 10.3389/fneur.2021.714313
Cernik, D., Sanak, D., Divisova, P., Kocher, M., Cihlar, F., Zapletalova, J., et al. (2019). Impact of blood pressure levels within first 24 hours after mechanical thrombectomy on clinical outcome in acute ischemic stroke patients. J. Neurointerv. Surg. 11, 735–739. doi: 10.1136/neurintsurg-2018-014548
Cheng, K. H., Lin, J. R., Anderson, C. S., Lai, W. T., and Lee, T. H. (2018). Lipid paradox in statin-naïve acute ischemic stroke but not hemorrhagic stroke. Front. Neurol. 9:541. doi: 10.3389/fneur.2018.00541
Cuadrado-Godia, E., Jiménez-Conde, J., Ois, A., Rodríguez-Campello, A., García-Ramallo, E., and Roquer, J. (2009). Sex differences in the prognostic value of the lipid profile after the first ischemic stroke. J. Neurol. 256, 989–995. doi: 10.1007/s00415-009-5059-9
Cui, C., Hong, Y., Bao, J., and He, L. (2021). The diagnostic reliability and validity of noninvasive imaging modalities to assess leptomeningeal collateral flow for ischemic stroke patients: A systematic review and meta-analysis. Medicine (Baltimore) 100:e25543. doi: 10.1097/MD.0000000000025543
de Havenon, A., Mlynash, M., Kim-Tenser, M. A., Lansberg, M. G., Leslie-Mazwi, T., Christensen, S., et al. (2019). Results From DEFUSE 3: Good Collaterals Are Associated With Reduced Ischemic Core Growth but Not Neurologic Outcome. Stroke 50, 632–638.
Goyal, M., Fargen, K. M., Turk, A. S., Mocco, J., Liebeskind, D. S., Frei, D., et al. (2014). 2C or not 2C: Defining an improved revascularization grading scale and the need for standardization of angiography outcomes in stroke trials. J. Neurointerv. Surg. 6, 83–86.
Heitsch, L., Ibanez, L., Carrera, C., Binkley, M. M., Strbian, D., Tatlisumak, T., et al. (2021). Early neurological change after ischemic stroke is associated with 90-day outcome. Stroke 52, 132–141.
Kim, E., and Cho, S. (2021). CNS and peripheral immunity in cerebral ischemia: partition and interaction. Exp. Neurol. 335:113508.
Lu, S. S., Zhang, X., Xu, X. Q., Cao, Y. Z., Zhao, L. B., Liu, Q. H., et al. (2019). Comparison of CT angiography collaterals for predicting target perfusion profile and clinical outcome in patients with acute ischemic stroke. Eur. Radiol. 29, 4922–4929. doi: 10.1007/s00330-019-06027-9
Matsumoto, K., Nohara, Y., Soejima, H., Yonehara, T., Nakashima, N., and Kamouchi, M. (2020). Stroke prognostic scores and data-driven prediction of clinical outcomes after acute ischemic stroke. Stroke 51, 1477–1483.
McVerry, F., Liebeskind, D. S., and Muir, K. W. (2012). Systematic review of methods for assessing leptomeningeal collateral flow. AJNR Am. J. Neuroradiol. 33, 576–582.
Nogueira, R. G., Jadhav, A. P., Haussen, D. C., Bonafe, A., Budzik, R. F., Bhuva, P., et al. (2018). Thrombectomy 6 to 24 hours after stroke with a mismatch between deficit and infarct. N. Engl. J. Med. 378, 11–21.
Ntaios, G., Abatzi, C., Alexandrou, M., Lambrou, D., Chatzopoulos, S., Egli, M., et al. (2011). Persistent hyperglycemia at 24-48 h in acute hyperglycemic stroke patients is not associated with a worse functional outcome. Cerebrovasc. Dis. 32, 561–566. doi: 10.1159/000331924
Powers, W. J., Rabinstein, A. A., Ackerson, T., Adeoye, O. M., Bambakidis, N. C., Becker, K., et al. (2019). Guidelines for the early management of patients with acute ischemic stroke: 2019 Update to the 2018 0Guidelines for the early management of acute ischemic stroke: A guideline for healthcare professionals from the american heart association/american stroke association. Stroke 50, e344–e418.
Qureshi, A. I., Singh, B., Huang, W., Du, Z., Lobanova, I., Liaqat, J., et al. (2020). Mechanical thrombectomy in acute ischemic stroke patients performed within and outside clinical trials in the united states. Neurosurgery 86, E2–E8.
Seker, F., Pereira-Zimmermann, B., Pfaff, J., Purrucker, J., Gumbinger, C., Schönenberger, S., et al. (2020). Collateral scores in acute ischemic stroke : A retrospective study assessing the suitability of collateral scores as standalone predictors of clinical outcome. Clin. Neuroradiol. 30, 789–793. doi: 10.1007/s00062-019-00858-1
Serdar, C. C., Cihan, M., Yücel, D., and Serdar, M. A. (2021). Sample size, power and effect size revisited: Simplified and practical approaches in pre-clinical, clinical and laboratory studies. Biochem. Med. (Zagreb) 31:010502. doi: 10.11613/BM.2021.010502
Shapiro, M., Raz, E., Nossek, E., Chancellor, B., Ishida, K., and Nelson, P. K. (2020). Neuroanatomy of the middle cerebral artery: Implications for thrombectomy. J. Neurointerv. Surg. 12, 768–773. doi: 10.1136/neurintsurg-2019-015782
Shi, F., Gong, X., Liu, C., Zeng, Q., Zhang, M., Chen, Z., et al. (2019). Acute stroke: Prognostic value of quantitative collateral assessment at perfusion CT. Radiology 290, 760–768.
Sojka, M., Szmygin, M., Pyra, K., Tarkowski, P., Luchowski, P., Wojczal, J., et al. (2021). Predictors of outcome after mechanical thrombectomy for acute ischemic stroke in patients aged = 90 years. Clin. Neurol. Neurosurg. 200:106354.
Su, J., Wolff, L., van Es, A. C. G. M., van Zwam, W., Majoie, C., Dippel, D. W. J., et al. (2020). Automatic collateral scoring from 3D CTA images. IEEE Trans. Med. Imaging 39, 2190–2200. doi: 10.1109/TMI.2020.2966921
Tan, J. C., Dillon, W. P., Liu, S., Adler, F., Smith, W. S., and Wintermark, M. (2007). Systematic comparison of perfusion-CT and CT-angiography in acute stroke patients. Ann. Neurol. 61, 533–543.
Tziomalos, K., Dimitriou, P., Bouziana, S. D., Spanou, M., Kostaki, S., Angelopoulou, S. M., et al. (2017). Stress hyperglycemia and acute ischemic stroke in-hospital outcome. Metabolism 67, 99–105.
Uniken Venema, S. M., Wolff, L., van den Berg, S. A., Reinink, H., Luijten, S., Lingsma, H. F., et al. (2022). Time since stroke onset, quantitative collateral score and functional outcome after endovascular treatment for acute ischemic stroke. Neurology 53:AWM92.
Wolff, L., Uniken Venema, S. M., Luijten, S. P. R., Hofmeijer, J., Martens, J. M., Bernsen, M. L. E., et al. (2022). Diagnostic performance of an algorithm for automated collateral scoring on computed tomography angiography. Eur. Radiol. 32, 5711–5718.
Wollenweber, F. A., Tiedt, S., Alegiani, A., Alber, B., Bangard, C., Berrouschot, J., et al. (2019). Functional outcome following stroke thrombectomy in clinical practice. Stroke 50, 2500–2506.
Wu, Z., Zeng, M., Li, C., Qiu, H., Feng, H., Xu, X., et al. (2019). Time-dependence of NIHSS in predicting functional outcome of patients with acute ischemic stroke treated with intravenous thrombolysis. Postgrad. Med. J. 95, 181–186. doi: 10.1136/postgradmedj-2019-136398
Yao, S., Liu, X., Luo, Y., Liu, Y., Huang, L., and Zhao, J. (2020). How strong the predictive ability of the variable age and stroke severity for patients presented late. J. Stroke Cerebrovasc. Dis. 29:104538. doi: 10.1016/j.jstrokecerebrovasdis.2019.104538
Yeramaneni, S., Kleindorfer, D. O., Sucharew, H., Alwell, K., Moomaw, C. J., Flaherty, M. L., et al. (2017). Hyperlipidemia is associated with lower risk of poststroke mortality independent of statin use: A population-based study. Int. J. Stroke 12, 152–160. doi: 10.1177/1747493016670175
Zonneveld, T. P., Nederkoorn, P. J., Westendorp, W. F., Brouwer, M. C., van de Beek, D., Kruyt, N. D., et al. (2017). Hyperglycemia predicts poststroke infections in acute ischemic stroke. Neurology 88, 1415–1421. doi: 10.1212/WNL.0000000000003811
Keywords: quantitative collateral score, visual collateral score, acute ischemic stroke, mechanical thrombectomy, low-density lipoprotein cholesterol, independent prognostic predictor
Citation: Lu Q, Zhang H, Cao X, Fu J, Pan Y, Zheng X, Wang J, Geng D and Zhang J (2022) Quantitative collateral score for the prediction of clinical outcomes in stroke patients: Better than visual grading. Front. Neurosci. 16:980135. doi: 10.3389/fnins.2022.980135
Received: 28 June 2022; Accepted: 04 October 2022;
Published: 25 October 2022.
Edited by:
Antonio Colantuoni, University of Naples Federico II, ItalyReviewed by:
Dominga Lapi, University of Naples Federico II, ItalyMariarosaria Santillo, University of Naples Federico II, Italy
Copyright © 2022 Lu, Zhang, Cao, Fu, Pan, Zheng, Wang, Geng and Zhang. This is an open-access article distributed under the terms of the Creative Commons Attribution License (CC BY). The use, distribution or reproduction in other forums is permitted, provided the original author(s) and the copyright owner(s) are credited and that the original publication in this journal is cited, in accordance with accepted academic practice. No use, distribution or reproduction is permitted which does not comply with these terms.
*Correspondence: Jianhong Wang, wangjianhong@huashan.org.cn; Daoying Geng, gdy_2019@163.com; Jun Zhang, zhangjun_zj@fudan.edu.cn