- 1Department of Radiology and Tianjin Key Laboratory of Functional Imaging, Tianjin Medical University General Hospital, Tianjin, China
- 2Tianjin Medical University, Tianjin, China
There are many factors that influence the academic achievements of medical students, but how personality and brain modulate the academic achievements of medical students remains unclear. The study collected the personality, brain imaging, and academic data from 448 medical students at Tianjin Medical University with admission time between 2008 and 2017. Four types of academic achievements, including behavioral and social sciences, clinical sciences and skills, basic biomedical sciences, and scientific methods, were assessed by the academic records of 58 courses. Personality was evaluated by Tridimensional Personality Questionnaire and Neuroticism Extraversion Openness Personality Inventory. Brain structural and functional properties, including gray matter volume, spontaneous brain activity and functional connectivity, were computed based on magnetic resonance imaging (MRI). Linear regression was used to evaluate the associations between personality and academic achievements. A voxel-wise correlation was used to identify areas of the brain where structural and functional properties were associated with academic achievements. Mediation analysis was used to test whether brain properties and personality independently contribute to academic achievements. Our results showed that novelty seeking (NS) was negatively correlated, and conscientiousness was positively correlated with all types of academic achievements. Brain functional properties showed negatively correlated with academic achievement in basic biomedical sciences. However, we did not find any mediation effect of the brain functional properties on the association between personality (NS and conscientiousness) and academic achievement in basic biomedical sciences, nor mediation effect of the personality (NS and conscientiousness) on the association between brain functional properties and academic achievement in basic biomedical sciences. These findings suggest that specific personality (NS and conscientiousness) and brain functional properties independently contribute to academic achievements in basic biomedical sciences, and that modulation of these properties may benefit academic achievements among medical students.
Introduction
Academic achievements of medical students represent the mastery of knowledge of medical science and related disciplines and are of great significance to the professional development of medical students. A variety of factors may influence the academic achievements of college students, such as age, sex, socioeconomic status, physical and mental health, intelligence, personality, interests, attitudes, behaviors and motivations, extracurricular activities, high school grades, study habits and methods, study time, teaching methods, teaching, and learning environments (De Luca et al., 2016; Partido and Stafford, 2018; Ahmady et al., 2019; Dzubur et al., 2020; Kusnierz et al., 2020; Al-Momani, 2021). However, only a few of these factors, such as study skills, attitudes, behaviors and motivations, time management, physical activity, personality, and coping strategies, were investigated and associated with the academic achievements of medical students (Ahmady et al., 2019; Hou et al., 2020; Lateef Junaid et al., 2020; Al-Momani, 2021).
Personality refers to individual differences in characteristic patterns of thinking, feeling, and behavior. The PPKI theory, which postulates intelligence as process, personality, knowledge and interest (Ackerman, 1996), proposes that personality plays a critical role in knowledge development, guiding a student’s choice and adherence to learning. Indeed, many studies have reported associations between personality traits and academic achievements, some of which have found associations between personality traits and overall or specific academic achievements (Hoschl and Kozeny, 1997; Vedel, 2014; Marcela, 2015; Bhagat et al., 2016; Mccredie and Kurtz, 2019; Dzubur et al., 2020; Kusnierz et al., 2020). Medical students must study different types of courses, which may be related to different personality traits. However, among medical students, the associations between personality traits and different types of academic achievements remain unknown.
Many factors that influence on academic achievements of medical students have been associated with human brain structural and functional properties. For example, understanding patterns of brain dominance could help improve how medical students teach and learn (Suresh et al., 2020); functional connectivity is associated with academic achievement in reading and is regulated through cognitive control abilities (Jolles et al., 2020); working memory is related to activity in frontal and parietal regions (Eriksson et al., 2015); and long-term memory is associated with the hippocampus (Sawangjit et al., 2018); the motivation to learn is related to the activity in the putamen (Gao et al., 2021). However, few studies have investigated the relationship between structural and functional properties of the human brain and the academic achievements of medical students. Besides, neural substrates are correlated with underlying personality traits and brain properties may help to predict one’s personality (Cai et al., 2020; Kabbara et al., 2020). Since both personality and brain may influence the academic achievements in medical students, and brain properties are associated with personality, it remains unclear how personality and brain structural and functional properties affect different types of academic achievements, and whether there are mediation effects among brain properties, personality, and academic achievements.
In this study, we aimed to answer three questions: (a) whether the academic achievements in different types of courses are correlated with different personality traits; (b) the structural and/or functional properties of which brain regions are correlated with different types of academic achievements; and (c) whether brain properties and personality independently contribute to academic achievements.
To answer these questions, we categorized 58 courses into four types (behavioral and social sciences, clinical sciences and skills, basic biomedical sciences, and scientific method) and calculated the grade point average (GPA) for each course as its academic achievement. The personality traits of each student were assessed using two commonly used questionnaires: the tridimensional personality questionnaire (TPQ) and Neuroticism Extraversion Openness (NEO) Personality Inventory (Cloninger et al., 1991; Costa and Mccrae, 1992; Caprara et al., 1993). The brain structural and functional properties were evaluated by grey matter volume (GMV), regional homogeneity (ReHo), and amplitude of low frequency fluctuation (ALFF) of spontaneous brain activity, and functional connectivity density and strength (FCD and FCS) that were derived from multi-modality MRI data. In 448 medical students with personality, academic achievements and brain MRI data, we investigated correlations of different personality traits and brain imaging measures with different types of academic achievements. For personality traits and brain imaging measures that were correlated with academic achievements, we performed mediation analysis to clarify whether there were mediation effects among brain properties, personality and academic achievements. The system flow of the study design is shown in Figure 1.
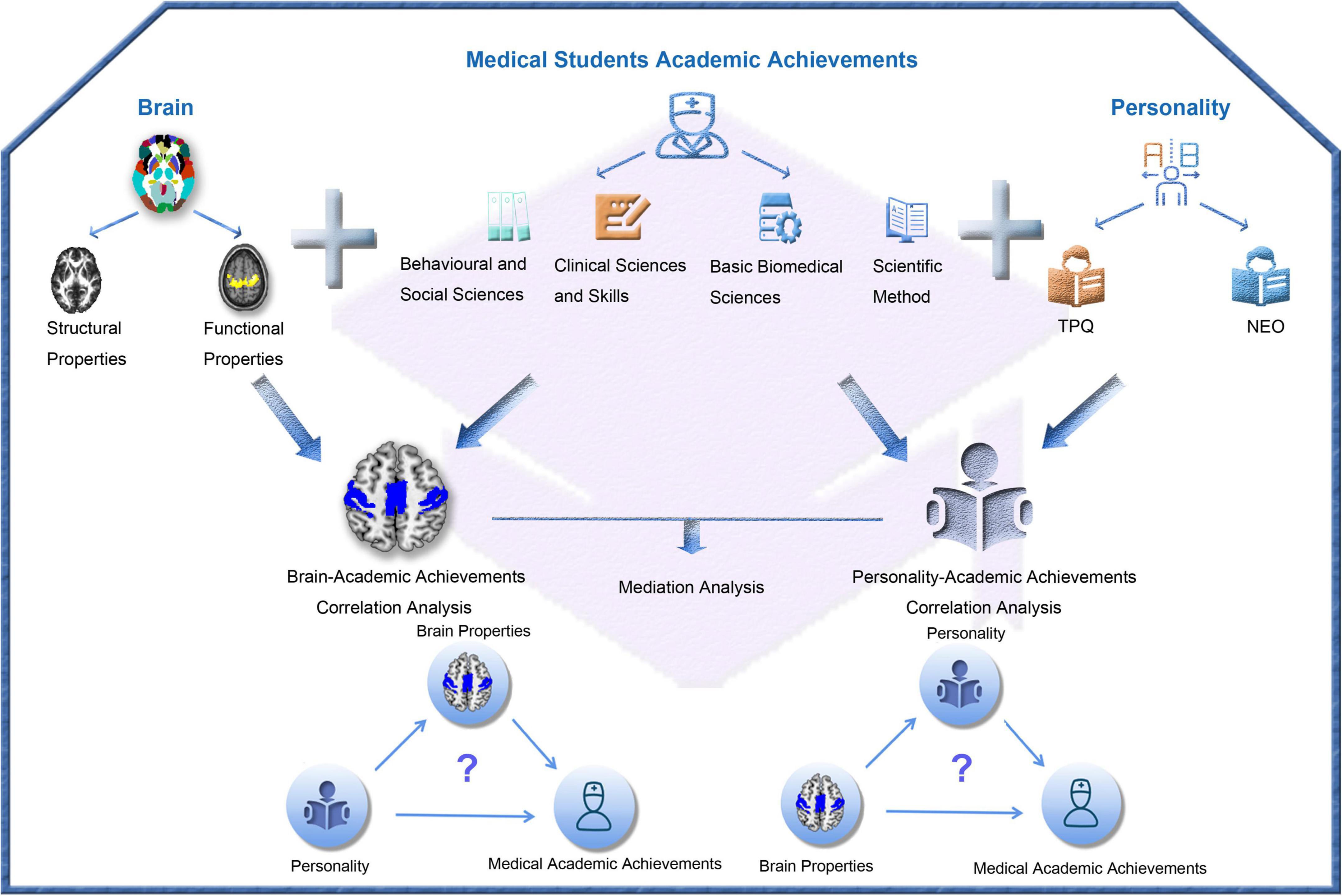
Figure 1. Flowchart of the study design. TPQ, tridimensional personality questionnaire; NEO, neuroticism Extraversion openness personality inventory.
Materials and methods
Participants
A total of 448 medical students (153 males, 295 females; age: 22.2 ± 1.8 years, range: 18–29°years; education: 15.6 ± 1.6°years) were recruited from Tianjin Medical University with admission time from 2008 to 2017, with different degrees (Master, N = 71; Bachelor, N = 375; Doctor, N = 2), and the major of all students were Clinical Medicine according to the Undergraduate Specialty Catalog of Higher Institutions (the 2020 revision) which was released by the National Educational Commission of China. Detailed inclusion and exclusion criteria can be found in Supplementary Table 1. This study was approved by the ethics committee of Tianjin Medical University General Hospital and all participants signed written informed consent before the experiment. The assessment time for each subject is detailed in Supplementary Figure 1 and Supplementary Table 2.
Academic achievements
Firstly, 58 courses were included based on the following criteria: (a) only compulsory courses and restrictive courses are included; (b) students complete courses at Tianjin Medical University and obtain valid scores; and (c) the number of students enrolled in each course is no less than 5% of the total number of students, taking into account the bias caused by the number of students in each course and reliability of academic achievements. Since then, 58 courses have been classified into four types of academic achievements (calculated from the scores of courses), including behavioral and social sciences (11 courses), clinical sciences and skills (24 courses), basic biomedical sciences (17 courses), and scientific method (6 courses), according to the Basic Medical Education WFME Global Standards for Quality Improvement (the 2015 revision) (WFME, 2015). Different academic achievements could represent different kinds of medical abilities. The detailed courses for each academic achievement and the number of students in each course are shown in Supplementary Table 3.
According to the Academic Grading System of Tianjin Medical University, 100 points per course was converted into Grade Point (GP). The detailed conversion criterion is shown in Supplementary Table 4. On this basis, the course credit (C) for each course is taken into account and the Course Grade Point (CGP) was derived from equation [1].
Finally, the GPA for each type of academic achievement was calculated based on equation [2], with N representing the number of courses in each type of academic achievement. The GPA for each kind of academic achievement in each student was calculated based on the courses they take.
Personality
Personality data of each participant were evaluated by the Tridimensional Personality Questionnaire (TPQ) and Neuroticism Extraversion Openness (NEO) Personality Inventory (Cloninger, 1987; Cloninger et al., 1991; Caprara et al., 1993). The TPQ is a self-reported personality inventory which evaluates three major personality dimensions, including avoidance of harm (HA), novelty seeking (NS), and reward dependence (RD). Besides, the NEO personality inventory was used to evaluate the big five personality traits which are commonly used models of personality in academic psychology, including extroversion, neuroticism, agreeableness, conscientiousness, and openness to experience. The detailed assessment contents and scientific significance of the two questionnaires were contained in Supplementary Table 5.
Magnetic resonance imaging data acquisition
Magnetic resonance imaging data were acquired using 3.0-Tesla MR scanners (Discovery MR750, General Electric, Milwaukee, WI, United States). The high-resolution structural T1-weighted images were acquired with the following parameters: repetition time (TR) = 8.16 ms; echo time (TE) = 3.18 ms; inversion time (TI) = 450 ms; matrix = 256 × 256; field of view (FOV) = 256 mm × 256 mm; flip angle (FA) = 12°; slice thickness = 1 mm; and 188 sagittal slices. The resting-state fMRI data were obtained using single shot gradient-echo echo-planar imaging (SS-GRE-EPI): TR = 2,000 ms; TE = 30 ms; matrix = 64 × 64; FOV = 220 mm × 220 mm; FA = 90°; slice thickness = 3 mm; number of slices = 36. During scanning, all participants were asked to stay as motionless as possible, and not to fall asleep.
fMRI data preprocessing
Statistical Parametric Mapping (SPM121) was used for the resting-state fMRI data preprocessing. The first five volumes with unstable signals were discarded. The collection time delay between slices is corrected by sinc-interpolation so that the collection time of all voxels is consistent within a repetition time. The head movements of each subject were assessed and corrected using rigid body transformations. All subjects satisfied the requirement for head motion (translation < 2 mm and rotation < 2°). The structural images were segmented and coregistered to the Montreal Neurological Institute (MNI) space. The parameters from structural images were used to normalize the fMRI images into the MNI space. The framewise displacement (FD) was also calculated for fMRI images, which indexes volume-to-volume changes in head position. The normalized fMRI images were resampled to 3-mm cubic voxels and smoothed using an 8-mm full-width at half-maximum (FWHM) Gaussian kernel. Spurious variances of the fMRI data were removed by regressing out the average blood oxygenation level-dependent (BOLD) signals of white matter (WM), cerebral spinal fluid (CSF), and 24 head motion parameters. Finally, the fMRI images were filtered using the frequency range of 0.01 to 0.08 Hz.
ReHo calculation
ReHo and ALFF are both reliable and reproducible parameters of functional MRI to reflect the level of regional functional neural activity. ReHo reflects the coherence of regional brain activity. For a given voxel, ReHo was defined as Kendall’s coefficient of concordance (KCC) of the time series of this voxel with its nearest neighbors (26 voxels) (Zang et al., 2004). ReHo of each grey matter (GM) voxel was calculated based on the normalized fMRI data, and the resulting ReHo map was further standardized, with each individual ReHo map divided by its mean ReHo of all GM voxels. A Gaussian kernel of 8 mm × 8 mm × 8 mm FWHM was used to smooth the data to reduce noise after calculating the ReHo.
Amplitude of low frequency fluctuation calculation
Amplitude of low frequency fluctuation represents the amplitude of the low-frequency BOLD signal fluctuation. The preprocessed time series were transformed to a frequency domain with a fast Fourier transform (FFT) and the power spectrum was then obtained. Because the power of a given frequency is proportional to the square of the amplitude of this frequency component of the original time series in the time domain, the square root was calculated at each frequency of the power spectrum, and the averaged square root was obtained across 0.01–0.08 Hz at each voxel. This averaged square root was taken as the ALFF. For standardization purposes, the ALFF of each voxel was divided by the global mean ALFF value of each subject.
Functional connectivity density and strength functional connectivity strength calculation
The FCD and FCS of each voxel were calculated to represent brain function at the connectivity level (Tomasi and Volkow, 2011). A GM mask was used to restrict the voxels in the GM regions with a probability of voxels within the mask belonging to GM > 50%. Pearson’s correlation coefficients were calculated between the BOLD time courses of all pairs of voxels and a whole GM functional connectivity matrix was obtained for each subject. Two voxels with a correlation coefficient of R > 0.6 were considered functionally connected for FCD, which was suggested to be the most optimal threshold for calculating FCD (Tomasi and Volkow, 2011). For FCS analysis, to eliminate weak correlations possibly arising from background noise, we restricted our analysis to positive correlations above a threshold of 0.2. The total number of functional connections was defined as the FCD for a voxel and the average strength of functional connections was defined as the FCS. This calculation was performed in each voxel throughout the brain and Fisher’s r-to-z transformation was performed to increase the normality of the distribution. Finally, the FCD and FCS maps were smoothed with an 8 mm × 8 mm × 8 mm Gaussian kernel.
Grey matter volume calculation
CAT12 toolbox (version r13642) was used for GMV calculation. The structural images were segmented into GM, WM, and CSF after the bias-field inhomogeneity was corrected, and then affine registration to standard space. The diffeomorphic anatomical registration through the exponentiated lie algebra (DARTEL) technique was used in normalization and the normalized images were resampled to a voxel size of 1.5 mm × 1.5 mm × 1.5 mm (Ashburner, 2007). The modulation was performed on the normalized GM images to preserve the absolute volume of the GM tissue. Finally, the images were smoothed with an 8 mm × 8 mm × 8 mm Gaussian kernel.
Association analysis between personality traits and academic achievements
Linear regression estimate was performed between the scores of HA, NS, and RD calculated from TPQ, five personality scores calculated from the NEO personality inventory and the four types of academic achievements. Specially, age, gender, and education years were considered as nuisance variables and regressed out in the analysis. The β values and P values were obtained and the significance threshold was defined as P < 0.05.
Association analysis between brain properties and academic achievements
Statistical parametric mapping [SPM12(see text footnote 1)] was used to explore which voxels were correlated with academic achievements. Age, gender, education years, and total intracranial volume were regressed out in the association analysis. Multiple comparisons were corrected by a false discovery rate (FDR) method (the threshold was voxel-wise FDR: q < 0.05 and a cluster size of at least 50 voxels).
Multivariate mediation analysis
The PROCESS macro (v3.5) for SPSS was used in multivariate mediation analysis (Hayes, 2013). Only personality traits and brain properties with significant correlations with academic achievements were included in the mediation analysis. On the one hand, the personality score was defined as an independent variable, the brain properties were defined as mediator variables, and the academic achievement was defined as a dependent variable. On the other hand, the brain property was defined as the independent variable, the personalities were defined as mediator variables, and academic achievement was defined as a dependent variable. Age, gender, and education years were regarded as covariates. In multivariate mediation analysis, all indirect effects are estimated in one multiple regression analysis with independent variable and all mediators as predictor variables. We used bootstrapping to assess the significance of the mediation effect. After 5,000 bias-corrected bootstrapping, we estimated the distribution of the indirect effects and calculate their 95% confidence intervals (CI). If zero does not fall between the resulting 95% confidence interval of the bootstrapping method, we confirmed the existence of a significant mediation effect (P < 0.05).
Results
Academic achievements associated with personality traits
The 58 courses were included and divided into four types of academic achievements, followed by the GPA distribution for each of the four types, as shown in Figure 2. In addition, to further validate the robustness of our calculations, we also calculated the GPA based on a “point to point” theory, which means that when the scale of the score was between 60 and 89, the higher the score, the higher GPA available based on linear transformation (Supplementary Table 6). Spearman correlations were performed between the GPA used in our work and the new GPA calculated based on the “point to point” theory, as shown in Supplementary Figure 2, significant correlations were found in all four academic achievements, which further suggests that the GPA we used is robust.
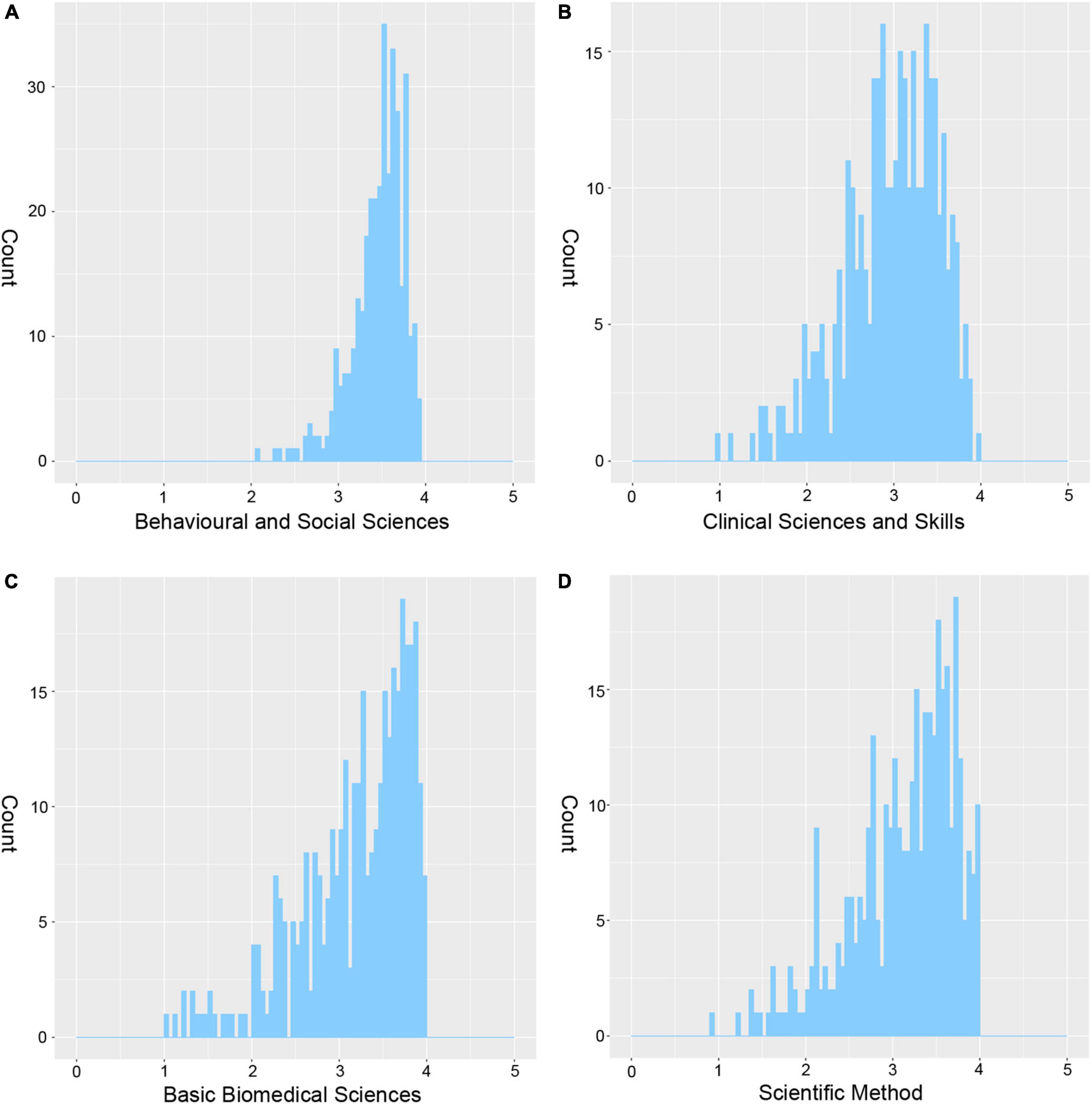
Figure 2. The distribution of grade point average in each type of academic achievements. (A–D) The distribution of GPA scores in behavioral and social sciences, clinical sciences and skills, basic biomedical sciences, and scientific method, respectively. The x-axis shows the GPA scores and the y-axis shows the number of subjects.
Meanwhile, the distribution of GPA scores for four types of academic achievements in master and bachelor were shown in Supplementary Figure 3. A higher score was found in the master group in each kind of academic achievement and a two-sample t-test was performed to make a comparison between the master group and bachelor group (PScientific method = 0.04, PBehavioral and social sciences = 0.04, PBasic biomedical sciences = 0.02, PClinical sciences and skills = 8.92E-5). In addition, we calculated the total score based on the four kinds of academic achievements (the sum of the GPA from four kinds of academic achievements), and the distribution of which was shown in Supplementary Figure 4. Furthermore, Spearman correlations were performed among the four kinds of academic achievements. The rho values were shown in Supplementary Table 7, and there were significant correlations among different kinds of academic achievements (P < 0.001).
Based on the HA, NS, and RD scores calculated from TPQ, linear regression analyses found that the NS scores showed significantly negative association with all the four types of academic achievements (behavioral and social sciences: P = 3.86E-5, β = −3.03; clinical sciences and skills: P = 0.02, β = −0.89; basic biomedical sciences: P = 1.60E-4, β = −1.31; and scientific method: P = 5.85E-4, β = −1.14). Besides, the conscientiousness scores calculated from the NEO personality inventory showed significantly positive association with all the four types of academic achievements (behavioral and social sciences: P = 4.59E-4, β = 4.19; clinical sciences and skills: P = 7.41E-3, β = 1.73; basic biomedical sciences: P = 4.61E-3, β = 1.61; and scientific method: P = 1.37E-4, β = 2.06), the results are shown in Tables 1, 2. Besides, the total GPA also showed a significant negative association with the NS scores and showed a significant positive association with the conscientiousness scores, which led to the same conclusion as the four kinds of academic achievements (Supplementary Table 8). These findings informed that NS and conscientiousness personality traits may exert different influences on the academic achievements of medical students.
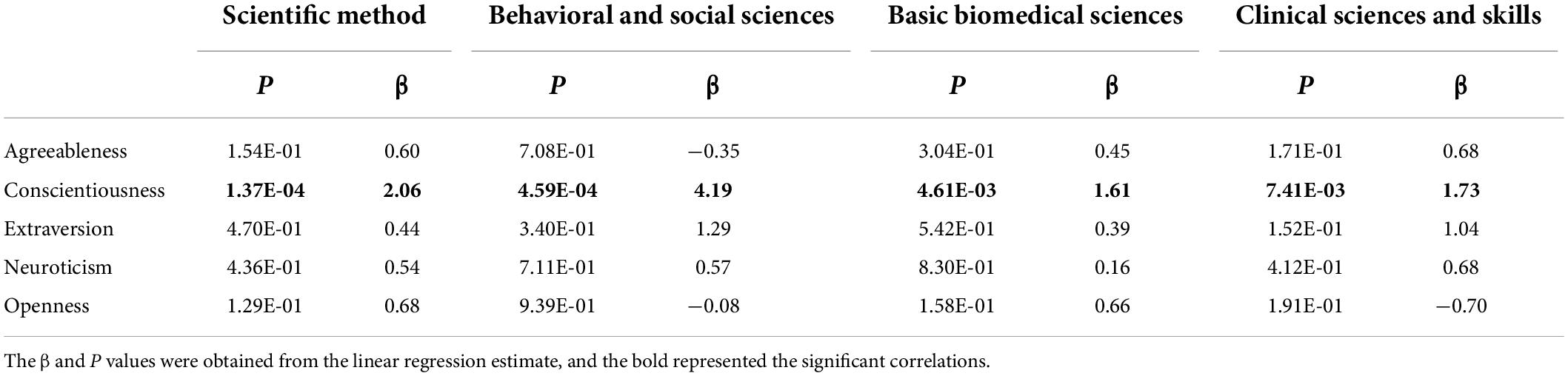
Table 2. The association analysis between the neuroticism extraversion openness personality inventory and academic achievements.
Taking into account the time gap between MRI/personality assessment and academic achievement assessment, we calculated the time gap between the two time points [i.e., time gap (calendar year) = timeacademic assessment−timeMRI/personality assessment], with most participants receiving two evaluations within 2 years. The distribution of the time gap was shown in Supplementary Figure 5. Besides, we regarded the time gap as one of the co-variables and re-performed the association analyses. The NS scores were still significantly negatively correlated with four academic achievements, and the conscientiousness scores were still significantly positively correlated with four academic achievements. The results were presented in Supplementary Tables 9, 10.
Brain properties associated with academic achievements
For each brain imaging measure (i.e., GMV, ReHo, ALFF, FCD, and FCS), voxel-based correlation analyses were performed to explore which brain regions were significantly associated with academic achievements. While we did not find a significant correlation between GMV and academic achievement, we found a significant negative correlation between regional ReHo, ALFF, FCD, and FCS and the GPA scores in basic biomedical sciences. Specifically, the GPA scores of Basic Biomedical Sciences were correlated with ReHo values in the bilateral precentral and postcentral gyri, left superior parietal lobule, right paracentral lobule, and right supplementary motor area; ALFF values in the bilateral occipital gyri, lingual gyri, cuneus, calcarine fissures and surrounding cortices, precentral and postcentral gyri, paracentral lobules, and left superior parietal gyrus; FCD values in the bilateral precentral and postcentral gyri, paracentral lobules, right superior occipital gyrus, cuneus, calcarine fissure and surrounding cortex, and right supplementary motor area; and FCS values in the right precentral gyrus, postcentral gyrus and paracentral lobule (Figure 3). Furthermore, we did not find a significant correlation between each brain imaging measure and total academic achievement.
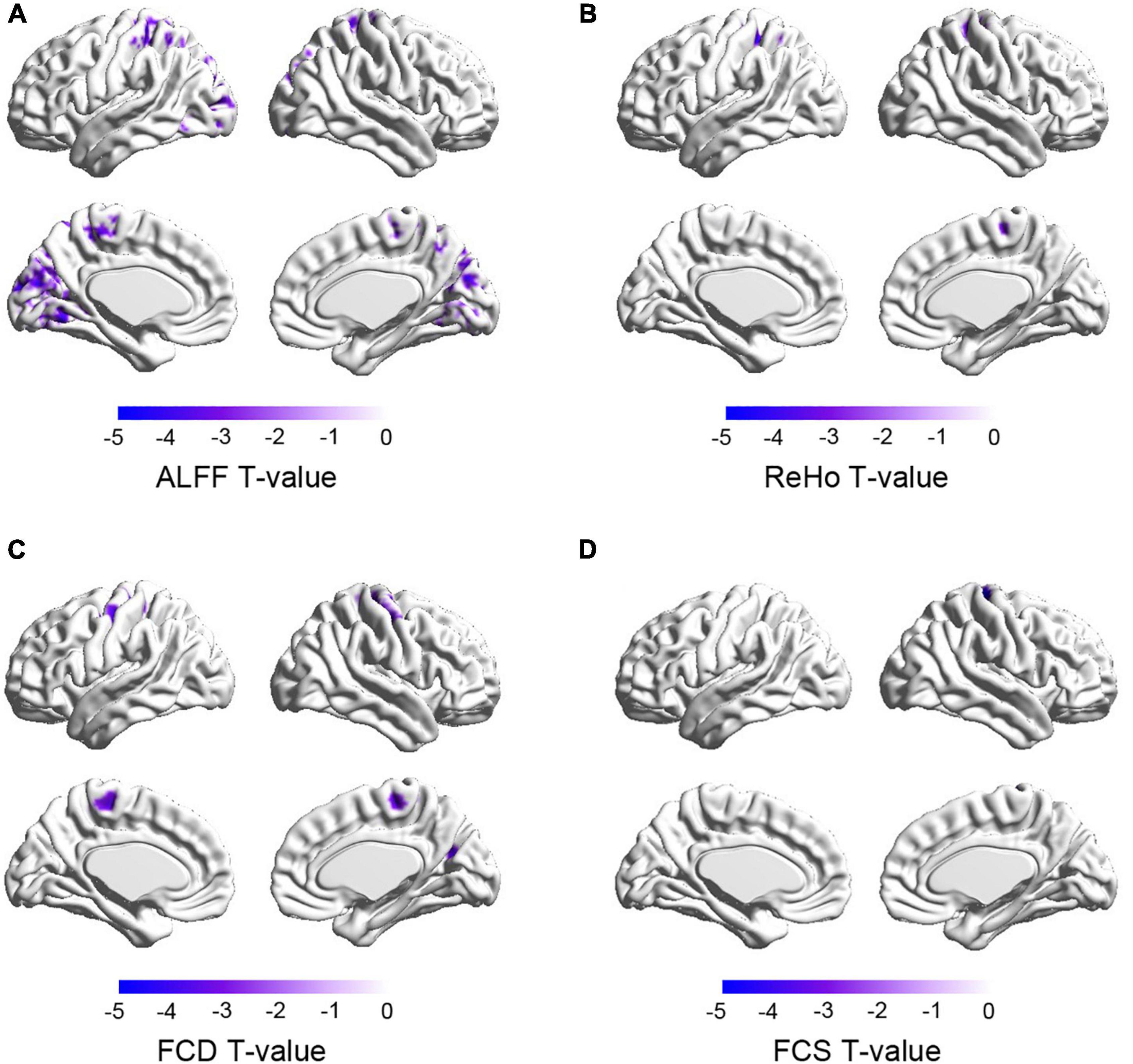
Figure 3. (A–D) Functional properties of brain areas correlated with academic achievement in basic biomedical sciences. The color demonstrates the T-values of the correlation analyses. ReHo, regional homogeneity; ALFF, amplitude of low frequency fluctuation; FCD, functional connectivity density; FCS, functional connectivity strength.
Mediation analysis
In the mediation analysis, the mediator variables were first defined as the regional ReHo, ALFF, FCD, and FCS values, which were significantly associated with academic achievement in basic biomedical sciences. The values were calculated by the mask which was derived from the significantly correlated brain regions in each brain functional property, respectively. The independent variable was the NS score or the conscientiousness score which showed a significant correlation with academic achievement of basic biomedical sciences, and the dependent variable was defined as the academic achievement of basic biomedical sciences that was correlated with both personality and brain functional properties. The multivariate mediation model aimed to explore whether brain functional properties mediated the associations between personality traits and academic achievements, and we did not find any significant indirect effects (NS: 95% CI of the indirect effect,−0.0083 to 0.0010; Conscientiousness: 95% CI of the indirect effect, −0.0022 to 0.0050) (Figures 4A,B). Similarly, we changed the independent variable to regional ReHo, ALFF, FCD, and FCS value, respectively, and the mediator variables were defined as the NS score and the conscientiousness score. However, we also did not find any significant indirect effects (ReHo: 95% CI of the indirect effect,−1.9722 to 0.9736; ALFF: 95% CI of the indirect effect, −0.8983 to 1.3128); FCS: 95% CI of the indirect effect, −0.8410 to 1.4899; FCD: 95% CI of the indirect effect, −0.0003 to 0.0007 (Figure 4F).
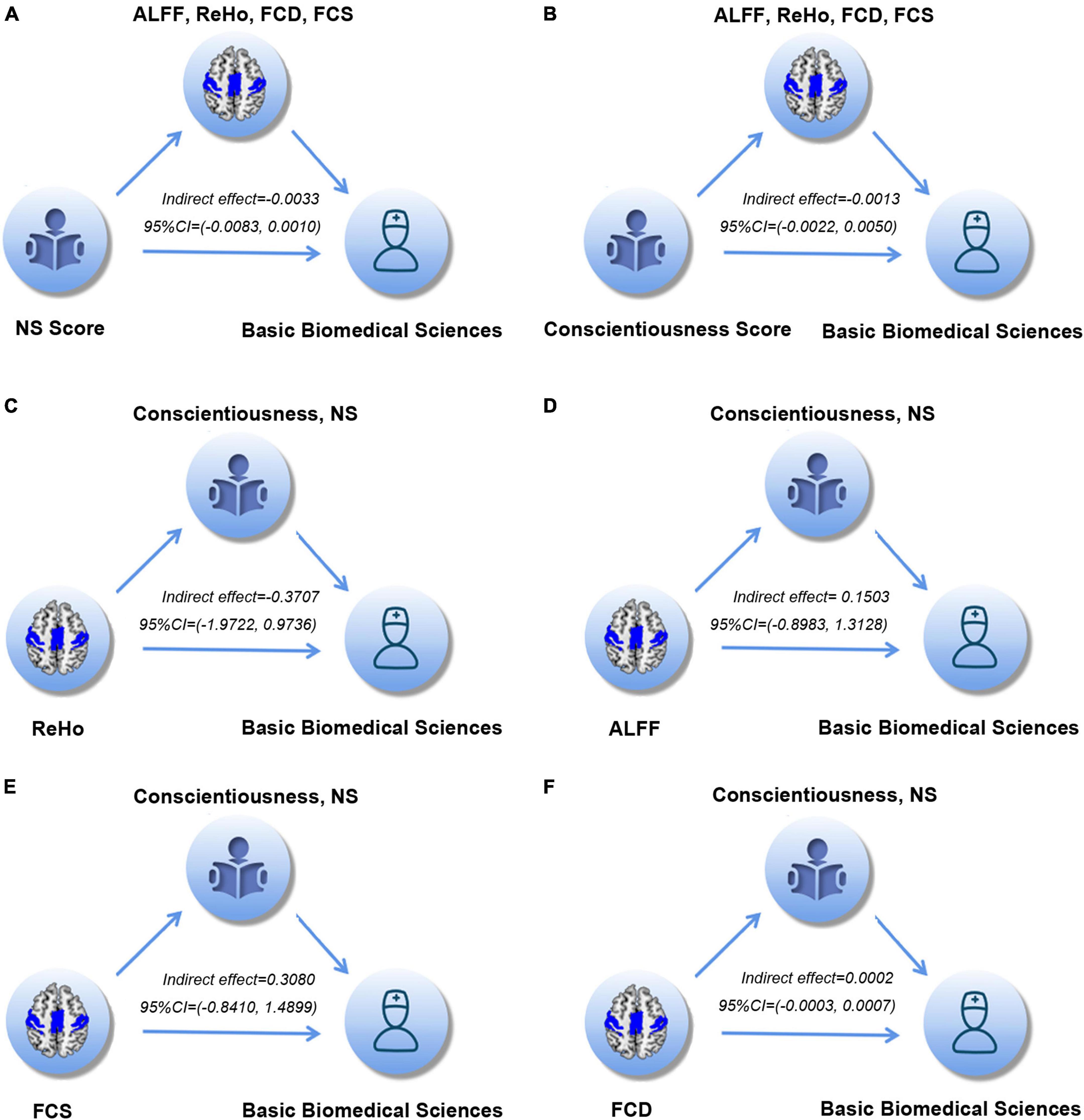
Figure 4. (A–F) The multivariate mediation analyses of the associations among personality, brain functional properties and academic achievement of basic biomedical sciences. The indirect effects and their 95%CI were provided. NS, novelty seeking; ReHo, regional homogeneity; ALFF, amplitude of low frequency fluctuation; FCD, functional connectivity density; FCS, functional connectivity strength.
Furthermore, we performed medication analyses based on the brain mask to further validate the mediation results. The mean values of ReHo, ALFF, FCD, and FCS were calculated based on the 90 non-cerebellar regions derived from the Automatic Anatomical Labeling (AAL) mask (Tzourio-Mazoyer et al., 2002). After that, the mediation analysis was performed on each functional property and each brain area. On the one hand, the independent variable was defined as the NS score or the conscientiousness score and the dependent variable was defined as academic achievement of basic biomedical sciences. The mediator variable was defined as the regional ReHo, ALFF, FCD, and FCS value in each brain area, respectively. On the other hand, the independent variable was defined as the regional ReHo, ALFF, FCD, and FCS value in each brain area, respectively. The dependent variable was defined as the academic achievement in basic biomedical sciences. The mediator variable was defined as the NS score or the conscientiousness score. The results showed no significant indirect effects were found (Supplementary Tables 11, 12), further supporting the conclusion that the personality (NS and conscientiousness) and brain properties (functional and connectivity) independently contribute to academic achievements in basic biomedical sciences.
Discussion
In this study, we investigated the associations between personality traits and brain structural and functional properties of medical students and different types of academic achievements. We found that the NS was negatively correlated with academic achievements, while conscientiousness was positively correlated with academic achievements. In addition, the time gap had little effect on the correlations, and the total GPA score reached the same conclusion, suggesting a stable relationship between medical students’ NS or conscientiousness and academic achievements. Moreover, we found that brain functional (ReHo and ALFF) and connectivity (FCD and FCS) properties rather than structural property (GMV) were negatively correlated with the academic achievement in basic biomedical sciences. However, there was no significant mediation effect among brain functional properties, personality traits (NS and conscientiousness) and academic achievements in basic biomedical sciences. These findings indicate that personality traits (NS and conscientiousness) and brain properties (functional and connectivity) contribute independently to the academic achievements of medical students in basic biomedical sciences, which may help design the promotion plan of medical education.
The tridimensional personality traits were associated with different neurotransmitter systems, such as dopamine-related NS (Benjamin et al., 1996; Ebstein et al., 1996; Stuettgen et al., 2005); 5-transerotonin-associated HA (Hansenne and Ansseau, 1999; Becker et al., 2007); and norepinephrine-associated RD (Garvey et al., 1996). They can predict the adaptive responses of individuals to environmental stimuli. NS reflects the tendency of humans to explore novel and unfamiliar stimuli and environments (Costa et al., 2014), and a higher NS score has been associated with greater impulsivity, sensation seeking, orientation toward independence, openness to experience, risk-taking, and extraversion (Wills et al., 1994; Mallet and Vignoli, 2007; Gordon and Luo, 2011). Consistent with previous studies reporting a negative correlation of NS with overall academic achievements (El Sheikh et al., 2014; Jang and Park, 2016), we found a significant negative correlation of NS with all four kinds of academic performances in medical students, which indicates a stable relationship between NS and academic achievements in medical students. Since NS is a personality trait associated with exploration activities, this negative correlation maybe related to individuals with lower NS scores being more academically oriented and less exposed to outside environmental interference.
Consistent with studies reporting positive correlations between conscientiousness and academic achievements (Vedel, 2014; Marcela, 2015; Ahmady et al., 2019), we also found significant positive correlations between conscientiousness and all four types of academic achievements in medical students. Conscientiousness reflects the overall motivational tendencies (Kanfer and Heggestad, 1997; Martocchio and Judge, 1997), and higher conscientiousness has been associated with more self-efficient, hard-working, and dedicated (Mount and Ba Rrick, 1995; Chen et al., 2001). High conscientious individuals are more willing to succeed and work harder than low conscientious ones (Gellatly, 2013; Kun et al., 2020). It has been reported that motivation and self-control may be the two neural pathways underlying the impacts of conscientiousness on learning (Park et al., 2017; Gao et al., 2021). These findings indicate that individuals with high conscientiousness have a better ability to control, manage, and regulate, which may contribute to better academic performance.
In this study, we found that brain functional (ReHo and ALFF) and connectivity (FCD and FCS) properties rather than structural property (GMV) were correlated with the academic achievement of basic biomedical sciences, indicating that brain functional activity and connectivity have closer relationships with academic achievements than brain structural property of GMV. However, we did not find any significant correlations of brain functional and connectivity properties with other types of academic achievements, suggesting that brain function and connectivity may only selectively affect a specific domain of academic performance in medical students. More importantly, we found negative correlations between the academic achievement of basic biomedical sciences with brain functional and connectivity properties in the sensorimotor areas, including the somatosensory areas, motor areas, and visual areas. These may represent an indirect association between brain and academic performance. Long-term training has been reported to lead to functional and morphological changes in primary motor and somatosensory cortices (Seidler and Carson, 2017). Besides, based on the idea of “Sensorimotor Learning,” during learning, the brain first converts primal sensory input into potential sensory variables, generating potential motor variables related to behavior, and ultimately driving muscle activations (Sohn et al., 2021). Thus, the somatosensory brain areas may play an important role in learning. Furthermore, increased local functional homogeneity was reported to be associated with reduced complexity of information processing and hierarchies of functional integration or separation within the ventral visual stream (Jiang et al., 2015). In addition, the reduction in ReHo was reported to be associated with stressful tasks (Zhang et al., 2019). These may help to explain the negative correlations between academic achievements and functional properties of sensorimotor areas in the brain.
The multivariate mediation analyses showed the brain functional and connectivity properties that were correlated with academic performance did not show any mediation effects on the associations between personality (NS and conscientiousness) and academic achievement of basic biomedical sciences, and the personality (NS and conscientiousness) also did not show any mediation effects on the associations between the brain functional and connectivity properties and academic achievement of basic biomedical sciences. In addition, brain mask-based mediation analyses did not show significant indirect effects. These findings indicate that personality of NS or conscientiousness, as well as brain functional and connectivity properties, independently contribute to academic achievements in basic biomedical sciences for medical students, informing neurological changes, and personality influences that may affect academic performance through independent pathways.
Limitations
In interpreting our findings, several limitations should be mentioned. First, the degrees of the included subjects were different (including Bachelor’s, Master’s, and Doctor’s degrees), which can lead to bias on degree differences. Besides, while the time gap has little effect on the results, there was a time gap between the time of MRI/personality assessment and the time of academic achievement assessment for each participant. Future studies should therefore also consider these biases.
Conclusion
In this study, we found a significant correlation between medical students’ personality traits and academic achievement, suggesting that personality assessment may be useful in student counseling and guidance. We also found that brain functional properties correlate with academic performance; however, no significant mediation effect was found among brain functional properties, personality traits (NS and conscientiousness) and academic achievements in basic biomedical sciences, suggesting that personality (NS and conscientiousness) and brain functional properties independently contribute to the academic performance in basic biomedical sciences for medical students.
Data availability statement
The raw data supporting the conclusions of this article will be made available by the corresponding authors.
Ethics statement
The studies involving human participants were reviewed and approved by Tianjin Medical University General Hospital. The patients/participants provided their written informed consent to participate in this study.
Author contributions
YX and CSY designed research and wrote the manuscript. YX, CCY, MS, and JS performed research. YX, CCY, MS, HX, and SW were involved in the assessment. NZ, WQ, FL, HD, JH, LH, XL, and CSY provided guidance and advice. All authors contributed to the article and approved the submitted version.
Funding
This work was supported by the National Key Research and Development Program of China (2018YFC1314300); National Natural Science Foundation of China (82030053 and 82071907); Wu Jieping Medical Foundation-Special Fund for Clinical Research (320.6750.2022-3-5); Scientific Research Planning Project of Tianjin Education Commisson (2021KJ219); and Tianjin Key Medical Discipline (Specialty) Construction Project (TJYXZDXK-001A).
Conflict of interest
The authors declare that the research was conducted in the absence of any commercial or financial relationships that could be construed as a potential conflict of interest.
Publisher’s note
All claims expressed in this article are solely those of the authors and do not necessarily represent those of their affiliated organizations, or those of the publisher, the editors and the reviewers. Any product that may be evaluated in this article, or claim that may be made by its manufacturer, is not guaranteed or endorsed by the publisher.
Supplementary material
The Supplementary Material for this article can be found online at: https://www.frontiersin.org/articles/10.3389/fnins.2022.964904/full#supplementary-material
Footnotes
References
Ackerman, P. L. (1996). “Intelligence as process and knowledge: An integration for adult development and application,” in Aging and skilled performance: Advances in theory and applications, eds W. A. Rogers, A. D. Fisk, and N. Walker (Mahwah, NJ: Lawrence Erlbaum Associates, Inc), 139–156.
Ahmady, S., Khajeali, N., Sharifi, F., and Mirmoghtadaei, Z. S. (2019). Factors related to academic failure in preclinical medical education: A systematic review. J. Adv. Med. Educ. Prof. 7, 74–85.
Al-Momani, M. M. (2021). Health-promoting lifestyle and its association with the academic achievements of medical students in Saudi Arabia. Pak. J. Med. Sci. 37, 561–566. doi: 10.12669/pjms.37.2.3417
Ashburner, J. (2007). A fast diffeomorphic image registration algorithm. Neuroimage 38, 95–113. doi: 10.1016/j.neuroimage.2007.07.007
Becker, K., El-Faddagh, M., Schmidt, M. H., and Laucht, M. (2007). Is the serotonin transporter polymorphism (5-Httlpr) associated with harm avoidance and internalising problems in childhood and adolescence? J Neural Transm (Vienna) 114, 395–402. doi: 10.1007/s00702-006-0577-4
Benjamin, J., Li, L., Patterson, C., Greenberg, B. D., Murphy, D. L., and Hamer, D. H. (1996). Population and familial association between the D4 dopamine receptor gene and measures of novelty seeking. Nat. Genet. 12, 81–84. doi: 10.1038/ng0196-81
Bhagat, V., Haque, M., Simbak, N. B., and Jaalam, K. (2016). Study on personality dimension negative emotionality affecting academic achievement among Malaysian medical students studying in Malaysia and overseas. Adv. Med. Educ. Pract. 7, 341–346. doi: 10.2147/AMEP.S108477
Cai, H., Zhu, J., and Yu, Y. (2020). Robust prediction of individual personality from brain functional connectome. Soc. Cogn. Affect Neurosci. 15, 359–369. doi: 10.1093/scan/nsaa044
Caprara, G. V., Barbaranelli, C., Borgogni, L., and Perugini, M. (1993). The “Big Five Questionnaire”: A new questionnaire to assess the Five Factor Model. Pers. Individ. Dif. 15, 281–288. doi: 10.1016/0191-8869(93)90218-R
Chen, G., Ca Sper, W. J., and Cortina, J. M. (2001). The roles of self-efficacy and task complexity in the relationships among cognitive ability, conscientiousness, and work-related performance: A meta-analytic examination.. Hum. Perform. 14, 209–230. doi: 10.1207/S15327043HUP1403_1
Cloninger, C. R. (1987). A systematic method for clinical description and classification of personality variants. A proposal. Arch. Gen. Psychiatry 44, 573–588. doi: 10.1001/archpsyc.1987.01800180093014
Cloninger, C. R., Przybeck, T. R., and Svrakic, D. M. (1991). The tridimensional personality questionnaire: U.S. normative data. Psychol. Rep. 69, 1047–1057. doi: 10.2466/pr0.1991.69.3.1047
Costa, P. T., and Mccrae, R. R. (1992). Revised Neo Personality Inventory (Neo Pi-R) and Neo Five-Factor Inventory (Neo-Ffi). NewYork: Springer.
Costa, V. D., Tran, V. L., Turchi, J., and Averbeck, B. B. (2014). Dopamine modulates novelty seeking behavior during decision making. Behav. Neurosci. 128, 556–566. doi: 10.1037/a0037128
De Luca, S. M., Franklin, C., Yueqi, Y., Johnson, S., and Brownson, C. (2016). The relationship between suicide ideation, behavioral health, and college academic performance. Commun. Ment. Health J. 52, 534–540. doi: 10.1007/s10597-016-9987-4
Dzubur, A., Lisica, D., Abdulahovic, D., and Ejubovic, M. (2020). Impact of social and psychological factors on academic achievement of university students. Med. Glas (Zenica) 17, 234–238.
Ebstein, R. P., Novick, O., Umansky, R., Priel, B., Osher, Y., Blaine, D., et al. (1996). Dopamine D4 receptor (D4dr) exon Iii polymorphism associated with the human personality trait of Novelty Seeking. Nat. Genet. 12, 78–80. doi: 10.1038/ng0196-78
El Sheikh, M. M., Shaker, N. M., Hussein, H., and Ramy, H. A. (2014). Impact of personality temperaments and characters on academic performance and specialty selection among a group of Egyptian medical graduates. Int. J. Soc. Psychiatry 60, 499–507. doi: 10.1177/0020764013501485
Eriksson, J., Vogel, E. K., Lansner, A., Bergstrom, F., and Nyberg, L. (2015). Neurocognitive architecture of working memory. Neuron 88, 33–46. doi: 10.1016/j.neuron.2015.09.020
Gao, K., Zhang, R., Xu, T., Zhou, F., and Feng, T. (2021). The effect of conscientiousness on procrastination: The interaction between the self-control and motivation neural pathways. Hum. Brain Mapp. 42, 1829–1844. doi: 10.1002/hbm.25333
Garvey, M. J., Noyes, R. Jr., Cook, B., and Blum, N. (1996). Preliminary confirmation of the proposed link between reward-dependence traits and norepinephrine. Psychiatry Res. 65, 61–64. doi: 10.1016/0165-1781(96)02954-X
Gellatly, I. R. (2013). Conscientiousness and task performance: Test of cognitive process model. J. Appl. Psychol. 81, 474–482. doi: 10.1037/0021-9010.81.5.474
Gordon, C. L., and Luo, S. (2011). The personal expansion questionnaire: Measuring one’s tendency to expand through novelty and augmentation. Pers. Individ. Dif. 51, 89–94. doi: 10.1016/j.paid.2011.03.015
Hansenne, M., and Ansseau, M. (1999). Harm avoidance and serotonin. Biol. Psychol. 51, 77–81. doi: 10.1016/S0301-0511(99)00018-6
Hayes, A. F. (2013). Introduction to mediation, moderation, and conditional process analysis: A regression-based approach. New York, NY: The Guilford Press.
Hoschl, C., and Kozeny, J. (1997). Predicting academic performance of medical students: The first three years. Am. J. Psychiatry 154, 87–92. doi: 10.1176/ajp.154.6.87
Hou, Y., Mei, G., Liu, Y., and Xu, W. (2020). physical fitness with regular lifestyle is positively related to academic performance among chinese medical and dental students. Biomed. Res. Int. 2020:5602395. doi: 10.1155/2020/5602395
Jang, H. W., and Park, S. W. (2016). Effects of personality traits on collaborative performance in problem-based learning tutorials. Saudi Med. J. 37, 1365–1371. doi: 10.15537/smj.2016.12.15708
Jiang, L., Xu, T., He, Y., Hou, X. H., Wang, J., Cao, X. Y., et al. (2015). Toward neurobiological characterization of functional homogeneity in the human cortex: Regional variation, morphological association and functional covariance network organization. Brain Struct. Funct. 220, 2485–2507. doi: 10.1007/s00429-014-0795-8
Jolles, D. D., Mennigen, E., Gupta, M. W., Hegarty, C. E., Bearden, C. E., and Karlsgodt, K. H. (2020). Relationships between intrinsic functional connectivity, cognitive control, and reading achievement across development. Neuroimage 221:117202. doi: 10.1016/j.neuroimage.2020.117202
Kabbara, A., Paban, V., Weill, A., Modolo, J., and Hassan, M. (2020). Brain network dynamics correlate with personality traits. Brain Connect 10, 108–120. doi: 10.1089/brain.2019.0723
Kanfer, R., and Heggestad, E. D. (1997). Motivational traits and skills: A person-centered approach to work motivation. Res. Organ. Behav. 19, 1–56.
Kun, B., Takacs, Z. K., Richman, M. J., Griffiths, M. D., and Demetrovics, Z. (2020). Work addiction and personality: A meta-analytic study. J. Behav. Addict. 9, 945–966. doi: 10.1556/2006.2020.00097
Kusnierz, C., Rogowska, A. M., and Pavlova, I. (2020). Examining gender differences, personality traits, academic performance, and motivation in ukrainian and polish students of physical education: A Cross-Cultural Study. Int. J. Environ. Res. Public Health 17:5729. doi: 10.3390/ijerph17165729
Lateef Junaid, M. A., Auf, A., Shaikh, K., Khan, N., and Abdelrahim, S. A. (2020). Correlation between academic performance and anxiety in medical students of Majmaah University–Ksa. J. Pak. Med. Assoc. 70, 865–868. doi: 10.5455/JPMA.19099
Mallet, P., and Vignoli, E. (2007). Intensity seeking and novelty seeking: Their relationship to adolescent risk behavior and occupational interests. Pers. Individ. Dif. 43, 2011–2021. doi: 10.1016/j.paid.2007.06.018
Marcela, V. (2015). Learning strategy, personality traits and academic achievement of university students. Procedia Soc. Behav. Sci. 174, 3473–3478. doi: 10.1016/j.sbspro.2015.01.1021
Martocchio, J. J., and Judge, T. A. (1997). Relationship between conscientiousness and learning in employee training: Mediating influences of self-deception and self-efficacy. J. Appl. Psychol. 82, 764–773. doi: 10.1037/0021-9010.82.5.764
Mccredie, M. N., and Kurtz, J. E. (2019). Prospective prediction of academic performance in college using self- and informant-rated personality traits. J. Res. Pers. 85:103911. doi: 10.1016/j.jrp.2019.103911
Mount, M. K., and Ba Rrick, M. R. (1995). The big five personality dimensions: Implications for research and practice in human resources management. Res. Pers. Hum. Resour. Manag. 13, 153–200.
Park, D., Tsukayama, E., Goodwin, G. P., Patrick, S., and Duckworth, A. L. (2017). A tripartite taxonomy of character: Evidence for intrapersonal, interpersonal, and intellectual competencies in children. Contemp. Educ. Psychol. 48, 16–27. doi: 10.1016/j.cedpsych.2016.08.001
Partido, B. B., and Stafford, R. (2018). association between emotional intelligence and academic performance among dental hygiene students. J. Dent. Educ. 82, 974–979. doi: 10.21815/JDE.018.094
Sawangjit, A., Oyanedel, C. N., Niethard, N., Salazar, C., Born, J., and Inostroza, M. (2018). The hippocampus is crucial for forming non-hippocampal long-term memory during sleep. Nature 564, 109–113. doi: 10.1038/s41586-018-0716-8
Seidler, R. D., and Carson, R. G. (2017). Sensorimotor learning: Neurocognitive mechanisms and individual differences. J. Neuroeng. Rehabil. 14:74. doi: 10.1186/s12984-017-0279-1
Sohn, H., Meirhaeghe, N., Rajalingham, R., and Jazayeri, M. (2021). A network perspective on sensorimotor learning. Trends Neurosci. 44, 170–181. doi: 10.1016/j.tins.2020.11.007
Stuettgen, M. C., Hennig, J., Reuter, M., and Netter, P. (2005). Novelty seeking but not bas is associated with high dopamine as indicated by a neurotransmitter challenge test using mazindol as a challenge substance. Pers. Individ. Dif. 38, 1597–1608. doi: 10.1016/j.paid.2004.09.025
Suresh, V. C., Poornima, C., Anjana, K. K., and Debata, I. (2020). Assessment of brain dominance and its correlation with academic achievement among medical students: A cross-sectional study. Arch. Ment. Health 21:25. doi: 10.4103/AMH.AMH_3_20
Tomasi, D., and Volkow, N. D. (2011). Functional connectivity hubs in the human brain. Neuroimage 57, 908–917. doi: 10.1016/j.neuroimage.2011.05.024
Tzourio-Mazoyer, N., Landeau, B., Papathanassiou, D., Crivello, F., Etard, O., Delcroix, N., et al. (2002). Automated anatomical labeling of activations in Spm using a macroscopic anatomical parcellation of the Mni Mri single-subject brain. Neuroimage 15, 273–289. doi: 10.1006/nimg.2001.0978
Vedel, A. (2014). The Big Five and tertiary academic performance: A systematic review and meta-analysis. Pers. Individ. Dif. 71, 66–76. doi: 10.1016/j.paid.2014.07.011
WFME (2015). Basic medical education wfme global standards for quality improvement. Copenhagen: World Federation for Medical Education.
Wills, T. A., Vaccaro, D., and Mcnamara, G. (1994). Novelty seeking, risk taking, and related constructs as predictors of adolescent substance use: An application of Cloninger’s theory. J. Subst. Abuse 6, 1–20. doi: 10.1016/S0899-3289(94)90039-6
Zang, Y., Jiang, T., Lu, Y., He, Y., and Tian, L. (2004). Regional homogeneity approach to fmri data analysis. Neuroimage 22, 394–400. doi: 10.1016/j.neuroimage.2003.12.030
Keywords: brain function, brain structural, medical education, academic achievements, personality, mediation analysis
Citation: Xie Y, Yuan C, Sun M, Sun J, Zhang N, Qin W, Liu F, Xue H, Ding H, Wang S, He J, Hu L, Li X and Yu C (2022) Personality and brain contribute to academic achievements of medical students. Front. Neurosci. 16:964904. doi: 10.3389/fnins.2022.964904
Received: 09 June 2022; Accepted: 04 August 2022;
Published: 06 September 2022.
Edited by:
Xin Di, New Jersey Institute of Technology, United StatesReviewed by:
Qinghua He, Southwest University, ChinaSihua Xu, Shanghai International Studies University, China
Copyright © 2022 Xie, Yuan, Sun, Sun, Zhang, Qin, Liu, Xue, Ding, Wang, He, Hu, Li and Yu. This is an open-access article distributed under the terms of the Creative Commons Attribution License (CC BY). The use, distribution or reproduction in other forums is permitted, provided the original author(s) and the copyright owner(s) are credited and that the original publication in this journal is cited, in accordance with accepted academic practice. No use, distribution or reproduction is permitted which does not comply with these terms.
*Correspondence: Xiaoxia Li, bGl4eEB0bXUuZWR1LmNu; Chunshui Yu, Y2h1bnNodWl5dUB0bXUuZWR1LmNu
†These authors have contributed equally to this work