- 1Department of Biomedical Engineering, North-Eastern Hill University, Shillong, India
- 2Biomedical Applications Unit, Central Scientific Instruments Organisation, Chandigarh, India
- 3Department of Neurology, North Eastern Indira Gandhi Regional Institute of Health and Medical Sciences, Shillong, India
The study of human movement and biomechanics forms an integral part of various clinical assessments and provides valuable information toward diagnosing neurodegenerative disorders where the motor symptoms predominate. Conventional gait and postural balance analysis techniques like force platforms, motion cameras, etc., are complex, expensive equipment requiring specialist operators, thereby posing a significant challenge toward translation to the clinics. The current manuscript presents an overview and relevant literature summarizing the umbrella of factors associated with neurodegenerative disorder management: from the pathogenesis and motor symptoms of commonly occurring disorders to current alternate practices toward its quantification and mitigation. This article reviews recent advances in technologies and methodologies for managing important neurodegenerative gait and balance disorders, emphasizing assessment and rehabilitation/assistance. The review predominantly focuses on the application of inertial sensors toward various facets of gait analysis, including event detection, spatiotemporal gait parameter measurement, estimation of joint kinematics, and postural balance analysis. In addition, the use of other sensing principles such as foot-force interaction measurement, electromyography techniques, electrogoniometers, force-myography, ultrasonic, piezoelectric, and microphone sensors has also been explored. The review also examined the commercially available wearable gait analysis systems. Additionally, a summary of recent progress in therapeutic approaches, viz., wearables, virtual reality (VR), and phytochemical compounds, has also been presented, explicitly targeting the neuro-motor and functional impairments associated with these disorders. Efforts toward therapeutic and functional rehabilitation through VR, wearables, and different phytochemical compounds are presented using recent examples of research across the commonly occurring neurodegenerative conditions [viz., Parkinson’s disease (PD), Alzheimer’s disease (AD), multiple sclerosis, Huntington’s disease (HD), and amyotrophic lateral sclerosis (ALS)]. Studies exploring the potential role of Phyto compounds in mitigating commonly associated neurodegenerative pathologies such as mitochondrial dysfunction, α-synuclein accumulation, imbalance of free radicals, etc., are also discussed in breadth. Parameters such as joint angles, plantar pressure, and muscle force can be measured using portable and wearable sensors like accelerometers, gyroscopes, footswitches, force sensors, etc. Kinetic foot insoles and inertial measurement tools are widely explored for studying kinematic and kinetic parameters associated with gait. With advanced correlation algorithms and extensive RCTs, such measurement techniques can be an effective clinical and home-based monitoring and rehabilitation tool for neuro-impaired gait. As evident from the present literature, although the vast majority of works reported are not clinically and extensively validated to derive a firm conclusion about the effectiveness of such techniques, wearable sensors present a promising impact toward dealing with neurodegenerative motor disorders.
Introduction
Human gait refers to the way an individual walks. It is a cyclical process with various phases, and each step contributes to one of the significant tasks responsible for locomotion, viz., weight acceptance, balance, and limb advancement. Human gait has been widely studied in healthy individuals and various pathologies to understand the mechanisms of movement and balance disorders. Alteration of synchronous coordination of multiple muscles and the neuro-motor system can cause atypical gait generation. Factors like accidents, aging, and neurological impairments cause degeneration of the musculoskeletal system, resulting in gait abnormalities. In turn, a pathological gait can significantly reduce the quality of life in terms of mobility and other psychological factors. One of the leading causes for gait impairment is neurodegenerative disorders like Parkinson’s disease (PD), multiple sclerosis (MS), Alzheimer’s disease (AD), Huntington’s disease (HD), Amyotrophic Lateral Sclerosis (ALS), along with certain forms of dementia. Although, as per the prediction of the World Health Organization (2006), neurological disorders were to contribute as the second leading cause of worldwide deaths, this figure was surpassed almost one and half decades ahead of its predicted time frame (Feigin et al., 2017, 2019). PD is one of the major neurodegenerative disorders of the central nervous system (CNS), affecting motor and non-motor functions, including gait and posture (Emamzadeh and Surguchov, 2018). Hip and knee are the two major contributors to non-neurological gait abnormalities (Pirker and Katzenschlager, 2017).
Gait events like heel strike (HS) of the foot [often denoted as Initial Contact (IC)] as well as the toe off (TO) signify the phase shift between stance and swing phase. Although these events are general indicators of typical gait phases and appear sequentially in gait timelines, these might be missing in some pathological cases. Figure 1 shows the conventional events associated with walking and different phases of the human gait cycle. Previous literature has reported gait kinetics and kinematics, spatiotemporal, mobility, balance, rhythm, etc., as potential inputs for classifying gait patterns of healthy controls from PD (Wahid et al., 2015; Papavasileiou et al., 2017; Suppa et al., 2017), HD (Kegelmeyer et al., 2017; Purcell et al., 2019), Hemiplegia (Lemoyne and Mastroianni, 2020, 2021), and stroke (Mannini et al., 2016; Papavasileiou et al., 2017). Gait parameters are a practical input in monitoring and quantifying therapeutic progress (Badaru et al., 2012). An in-depth study of any individual’s gait can give information varying from kinetic and kinematic aspects to different musculoskeletal functions (Li et al., 2016a). Hence, gait analysis finds application in clinical diagnosis, rehabilitation, sports, and biometric security. This article presents a review of the latest advances in wearable sensors and techniques for ambulatory gait analysis, focusing on clinical aspects of neurodegenerative gait disorders. The role of different sensors in studying various facets of clinical gait analysis, including event detection, spatiotemporal parameter measurement, joint kinematics analysis, gait investigation, and postural balance analysis, have been methodically reported. A total of 48 original research articles published from 2005 to 2021 have been thoroughly discussed, along with several other technical and review papers. In addition, four commercially available products and their application have also been outlined. Section 2 presents an overview of the most prevalent neurodegenerative gait disorders and their neuromuscular motor implications. Section “Gait Analysis: Terminologies and Techniques” gives an overview of various practiced modalities of a conventional clinical gait analysis over the years and how the advancement of wearable technology has led to the shift from stationary ambient sensor-based measurement setups to body-mounted techniques. Section “Wearable Sensors for Gait Parameter Estimation” offers a detailed review of the sensing techniques for general and neurodegenerative gait disorders. This section predominately highlights the role and application of inertial sensors in almost all spectrums of gait analysis. Other methods such as force sensor-based insole and electromyography are also discussed in detail. Apart from these conventional methods, different miscellaneous sensors that have been attempted or show promising performances are also highlighted. In addition, the review also discusses some commercially available measurement systems. Section “Advances in Therapeutic Intervention” introduces the recent progress toward a non-pharmacological intervention to mitigate the challenges of neurodegenerative motor and functional impairments. Section “Discussion and Future Direction” includes a discussion and the authors’ comments about the reported techniques and the future trends. Each unit is presented with a short concluding remark by authors emphasizing relevant context. The review concludes with a summary of the article.
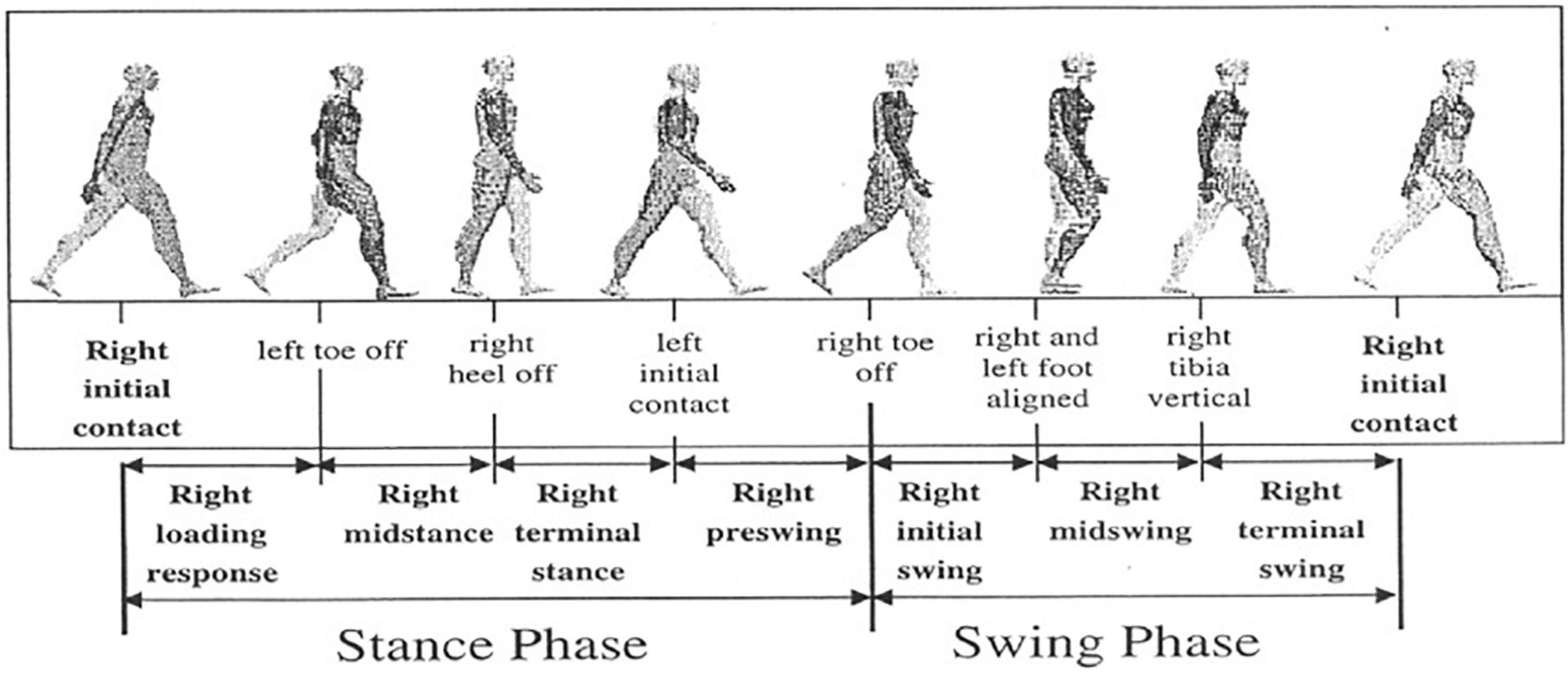
Figure 1. Gait phases and used terminologies to partition the phases. For the representational purpose, the gait segmentation of the right leg (ipsilateral) is described w.r.t. the left (contralateral) leg. [Image source: Li et al. (2016a), the open-access article under the CC BY-NC-ND license].
Neurodegenerative Gait Disorders
Neurodegenerative diseases are a heterogeneous group of progressive disorders associated with degeneration of the central or peripheral nervous system. They alter the nervous system’s structural, biochemical, electrical, and functional activities. This results in a loss of coordination of the neuromuscular system, causing gait and balance disorders (Cicirelli et al., 2021).
Alzheimer’s Disease
Alzheimer’s disease is the most prevalent neurodegenerative disorder that affects the patient’s memory and comprehension early and eventually leads to neuromotor impairments. Although the exact pathology behind AD isn’t ascertained, studies have reported mitochondrial dysfunction (Correia et al., 2012; Carvalho et al., 2015; Rahman and Rhim, 2017) and alteration of axonal transport (AT) (Wang Z. X. et al., 2015) as contributing agents for neurodegenerative diseases like AD. In the late stages, the most common motor impairments include bradykinesia (slow movement), extrapyramidal rigidity, and gait disorders. Such patients exhibit reduced gait speed and step length, decreased gait frequency (cadence) and increased variability (Pieruccini-Faria et al., 2021). A recent study (Tian et al., 2021) has shown that older persons with slow gait speed and less fragmented activity are at higher risk of developing AD, thereby presenting gait as a potential early indicator for predicting the disease.
Parkinson’s Disease
Parkinson’s disease is the second most common neurodegenerative disease, resulting from neuronal cell loss in the mid-brain substantia nigra pars compacta region and dopamine (DA) depletion in the striatum (ST) (Bastide et al., 2015) with multiple neurotransmitters deficits (Marinus et al., 2018). In addition, AD associated pathology like mitochondrial dysfunction is also commonly present (Carvalho et al., 2015; Rahman and Rhim, 2017). Accumulation of misfolded α-synuclein, often related to different neurotoxin pathways, is another distinct hallmark of PD (Chen et al., 2019) and can cause neuroinflammation, oxidative stress, and induced endoplasmic reticulum (ER) stress in such patients. This neuro-disorder affects up to 10 million people worldwide (Emamzadeh and Surguchov, 2018). Oxidative stress caused due to imbalance of free radicals’ homeostasis in the body causes cellular and tissue damage. PD involves the primary type of hypokinetic movement disorder resulting in bradykinesia, hypertonia (rigidity), tremor, and flexed posture, marked by reduced gait speed and step length, festination (Vallabhajosula et al., 2013). A typical condition during severe stages of the disease is the freezing of gait (FOG). It is defined as a brief, episodic absence or marked reduction of forwarding progression of the feet while walking, turning, or initiating gait, despite the intention to walk. FOG episodes are one of the primary reasons for losing balance and increased risk of falls in such patients.
Huntington’s Disease
The Huntingtin (HTT) gene mutation, due to expansion of CAG triplet (cytosine, adenine, and guanine), leads to polyglutamine tract elongation is often linked to HD (Xu et al., 2017). In addition, the formation of Reactive Oxygen Species (ROS) due to oxidative stress is also considered a significant trigger for it (Manoharan et al., 2016). Increased ROS generation is also related to the accumulation of proteins such as α-synuclein in PD (Follett et al., 2016). HD is clinically characterized by involuntary movements such as chorea, psychiatric signs, and progressive dementia. Such patients struggle with uncontrolled movements and loss of cognitive abilities. With disease progression, poorly coordinated body movements and unsteady gait become more visible. Their gait is characterized by slow speed, reduced stride length, variable stepping pattern, and increased stance-to-swing duration ratio. All these factors, in turn, increase the risk of falls in HD patients, thereby further limiting their functional capacity (Georgiou et al., 2020).
Amyotrophic Lateral Sclerosis
Amyotrophic lateral sclerosis is pathogenically characterized by enormous oxidative stress and mitochondrial dysfunction. It affects the motor neurons of the cerebral cortex, brain stem, and spinal cord. This disruption of communication between the cerebrum and muscle results in muscle atrophy improper limb functioning, resulting in altered gait patterns. Gait dysfunction in ALS is distinguished by increased inter-stride fluctuation, small duration on a single limb, small step length, and decreased cadence (Garcia-Gancedo et al., 2019).
Multiple Sclerosis
Multiple sclerosis is a demyelinating, chronic, and progressive disease-causing episodic deterioration of neuromuscular functions caused due to autoimmune-mediated loss of myelin and axonal damage (Jones et al., 2017). Clinical symptoms of MS include sensory, cognitive, and motor impairment. With progression over time, the gait shows distinguishable signs of decreased gait speed, step length, cadence, and joint range of motion (ROM) with increased inter-stride gait variability. Such patients also exhibit poor postural control (Kalron et al., 2016).
To summarize, the literature shows that gait and functional impairments are prevalent in subjects with neurodegenerative disorders. So, gait and balance analysis as a clinical tool is helpful for better diagnosing and managing such impairments. In many cases, locomotor impairments are the earliest manifestation of the disease, such as PD. Also, different neurological disorders often appear with overlapping clinical symptoms, such as dementia. However, assessment of movement and balance-related parameters can help diagnose the exact underlying cause. Thus, gait and postural analysis are increasingly used in clinical setups for diagnosis, progression monitoring, and providing targeted therapy.
Gait Analysis: Terminologies and Techniques
Gait analysis refers to the systematic measurement, description, and assessment of parameters significant to human motion. These parameters can vary from ground reaction force (GRF) and joint torque to joint kinematics like range of motion (ROM) and segment acceleration. A systematic gait analysis includes structuring information, observation of a strict pre-assigned protocol, and a method for data interpretation. General gait analysis is performed from the perspectives of extracting kinematic, kinetic, or Spatiotemporal gait parameters. During the clinical gait study, two effective practices were followed: semi-subjective analysis and objective analysis. Specialists and experts usually perform semi-subjective measurements based on a predefined set of observations and questionnaires. The subjects must perform certain activities in a pre-determined walking circuit with clear markings, and the clinician records the parameters of interest with simple tools like a stopwatch. Although such methods are practical in settings with no access to sophisticated gait measurement systems, such measurements are primarily inaccurate, require expertise, and are highly subjective processes. It is almost impossible to observe/record multiple contrasting parameters simultaneously. On the other hand, the objective-based analysis uses sophisticated devices and equipment to measure a wide range of parameters simultaneously. These devices can be based on image processing techniques, floor-mounted measurement systems, or body-mounted instruments.
Objective assessments based on quantitative gait measurements are desired for practical gait analysis and rehabilitation planning. However, 3D gait analysis, which is conventionally conducted using motion capture cameras and force platforms, requires technical expertise and is inaccessible to most clinics and hospitals in rural settings due to high cost. Gait data outputs can include many variables, which may be overwhelming for clinicians to interpret, adding to the challenges of using gait analysis in clinical settings. Therefore, a low-cost, portable, user-friendly instrumented method that quantifies gait has broad clinical application for monitoring individuals’ gait parameters and physical activities in outpatient clinics, community, and home settings. An objective, automated process of quantifying and assessing gait pathology can allow clinicians to invest their resources and time in prescribing effective and more targeted treatments. User-friendly systems for measuring and tracking gait can also aid in assessing the quality of life.
The earliest modern studies on human walking kinematic were performed by Marey and Muybridge using still cameras in the 1870s (Whittle, 1996). With the use of television cameras during the seventies, the process became quicker and more convenient since these cameras were linked directly into computers (Whittle, 1982). Several research groups developed a kinematic gait measurement system using this approach, and several of these early systems evolved into commercial equipment.
Gait kinetic measurement-based studies have mainly dealt with the forces generating during the ground-foot interactions, primarily measured using force platforms. The force platforms have evolved from purely mechanical one-dimensional design (Amar, 1920) to more accurate 3D instruments with digital outputs. Although, until recently, most research on gait kinetic analysis was focused on ground reaction forces, modern gait analysis systems also provide a measure of joint moments and joint powers.
Based on the requirement and technique of measurement, a clinical gait analysis is targeted either at gait event detection and segmentation, spatiotemporal parameter estimation, or gait classification. A wide range of sensors and systems are developed and used for capturing these signals. These devices and techniques used for gait investigation can be classified based on the placement and positioning of the measurement system: Wearable sensors (WS) and Non-wearable sensors (NWS). A WS system is mandatorily placed on different body segments like the foot, shank, pelvis, etc. For example, data from an inertial sensor (accelerometer and gyroscope) set around the ankle joint can be used for distinguishing a healthy gait from a person with Friedrich’s ataxia (Lemoyne et al., 2016). State-of-the-art NWS systems, colloquially also referred to as ambient sensors, consist of two distinct technological approaches: (a) optical motion capture systems that track targeted joints and orientations while walking, either in 2D or 3D and (b) floor sensor-based plantar profile measurement systems. Again, the optical motion capture systems have two families: reflective markers (Roy et al., 2020; Wang et al., 2021) and markerless camera systems (Zago et al., 2020; Hazra et al., 2021). The marker-based systems compute the position of joints and the orientation body segments through the 3D localization of the body markers using multi-camera stereophotogrammetric video systems. A markerless camera system uses the human body model and image features to determine the shape, pose, and joint orientations. The floor sensor-based measurement method relies on pressure sensing technology. Force plates, consisting of load cells, measure the 3D GRF and moments involved during human locomotion. On the other hand, a pressure platform records the plantar pressure profile variation during gait, revealing critical information regarding foot loading pattern and CoP progression. Such a platform consists of arrays of capacitive/resistive sensitive cells that measure pressure acting on each cell due to foot-ground interaction. Both force plate and pressure platform are usually floor mounted, and thus several gait steps can be recorded from them depending on the size of the same. However, a class of treadmill-mounted force/pressure measurement systems allows a larger volume of gait data recording (Wiik, 2016). All NWS systems are operated in controlled facilities, and subjects must follow a predefined protocol. Some measurement methods involve a combination (hybridization) and are essentially a laboratory confined practice. However, the current gold standards for gait analysis are NWS-based measurement tools and consist of either a force plate, an instrumented walkway, or a motion capture system. These measurement methods provide excellent quality data with high accuracy and repeatability. However, high-cost setups provide limited capture volume and a specialized workforce to operate the instruments. Work on compact and ambulatory gait sensors (WS) has picked up the pace at different research groups to overcome these limitations. Such a measurement system provides an alternative to conventional laboratory-based measurements at the cost of reduced accuracy and reliability. Table 1 highlights some of the characteristics associated with both techniques.
The distinct advantages possessed by WS in terms of proving almost natural agility over long-term measurements have made them a popular choice for use in ambulatory gait analysis. Such a method allows researchers and clinicians to record physiological features constantly. Therefore, the demand for such technology, which measures gait characteristics either for activity recognition or gait event classification, has risen significantly in the last two decades.
Literature shows that gait analysis is a multidimensional approach depending on the parameter of interest. The gait and balance features can vary from kinematic kinetic to physiological aspects, presenting a vast gait feature set. However, a current technique to extract these features is broadly grouped into two classes, based on the position of the measurement device. The ambient or non-wearable sensors, although being gold standards for gait measurements, possesses specific challenges, thereby limiting their clinical translation. On the other hand, wearable devices show promising performance for future clinical gait analysis. Therefore, this review presents an overview of advancements in wearable technologies aiming to mitigate the challenges associated with ambient sensors.
Wearable Sensors for Gait Parameter Estimation
Wearable gait analysis tools generally comprise different sensing principles, mainly inertial, force, flexible goniometers, and myoelectric. Few additional sensors, such as electromagnetic tracking systems, sensing fabric, ultrasonic sensors, etc., have also been reported. A single type or combined multiple sensor system (multimodal) may be used based on the target application. However, the wearable gait analysis-based technique is still in its infancy. There is no consensus regarding a set of derived gait characteristics to be assessed and their clinical relevance (Beauchet et al., 2017). The basic principles, features, and overview of reported works around these sensors and systems are described in this section.
Inertial Sensors
Inertial sensors (IS) or Inertial Measurement Units (IMU), comprising accelerometers and gyroscopes and sometimes magnetometers, measure an object’s motion dynamics, viz., orientation, velocity, acceleration, and gravitational forces. An accelerometer measures acceleration along its sensitive axis and effectively measures the gait motion. The acceleration/velocity of the feet or legs in the gait has been determined to perform the gait analysis (Zeng and Zhao, 2011; Hsu et al., 2014). A gyroscope measures the angular rate and can be incorporated to measure the motion and body segment orientation (Catalfamo et al., 2010; Ayrulu-Erdem and Barshan, 2011). The commercial availability of MEMS-based IS, small form factor, and accessible electronic integration is the critical factor that truly opened up new perspectives in human movement analysis, which justifies the significant literature-related exploitation during the last two decades (Tao et al., 2012; Muro-De-La-Herran et al., 2014; Caldas et al., 2017; Bowman et al., 2021).
Morris (1973) marked the beginning of wearable sensor-based ambulatory gait analysis using accelerometers. The author used six uniaxial accelerometers mounted on a rigid bar for solving the equation governing the motion of a rotating rigid body and determining its angular acceleration. IS-based gait analysis since then has been used for a wide range of measurements: from event detection, kinematic, and kinetic parameter estimation to gait classification. Inertial sensors, placed at different limb segments such as foot, shank, hip, etc., produce a repeatable pattern of signals that signify specific gait events. Many algorithms and methods like thresholding (Ledoux, 2018), peak detection (Lee and Park, 2011; Maqbool et al., 2016; O’brien et al., 2019), zero-crossing (Hundza et al., 2013; Formento et al., 2014; Gouwanda et al., 2016; Maqbool et al., 2016; O’brien et al., 2019), angular rate reversal (Hundza et al., 2013; Schülein et al., 2017), Linear Discriminant Analysis (LDA) (Jiang et al., 2018) have been proposed and developed to identify gait events like HS, TO, and flat foot (FF) on real-time as well as offline mode. For example, the TO and HS detection work using a single gyroscope placed at shank by Hundza et al. (2013) uses rate reversals and zero crossings of the angular rate pattern. Figure 2 shows typical acceleration and angular rate trajectories of foot-mounted IMUs (Schülein et al., 2017). The algorithm satisfactorily identifies events with 100% accuracy in the case of healthy controls and PD patients. However, the method needs manual adjustment of parameters to process data with varied angular rates to reject any false peaks.
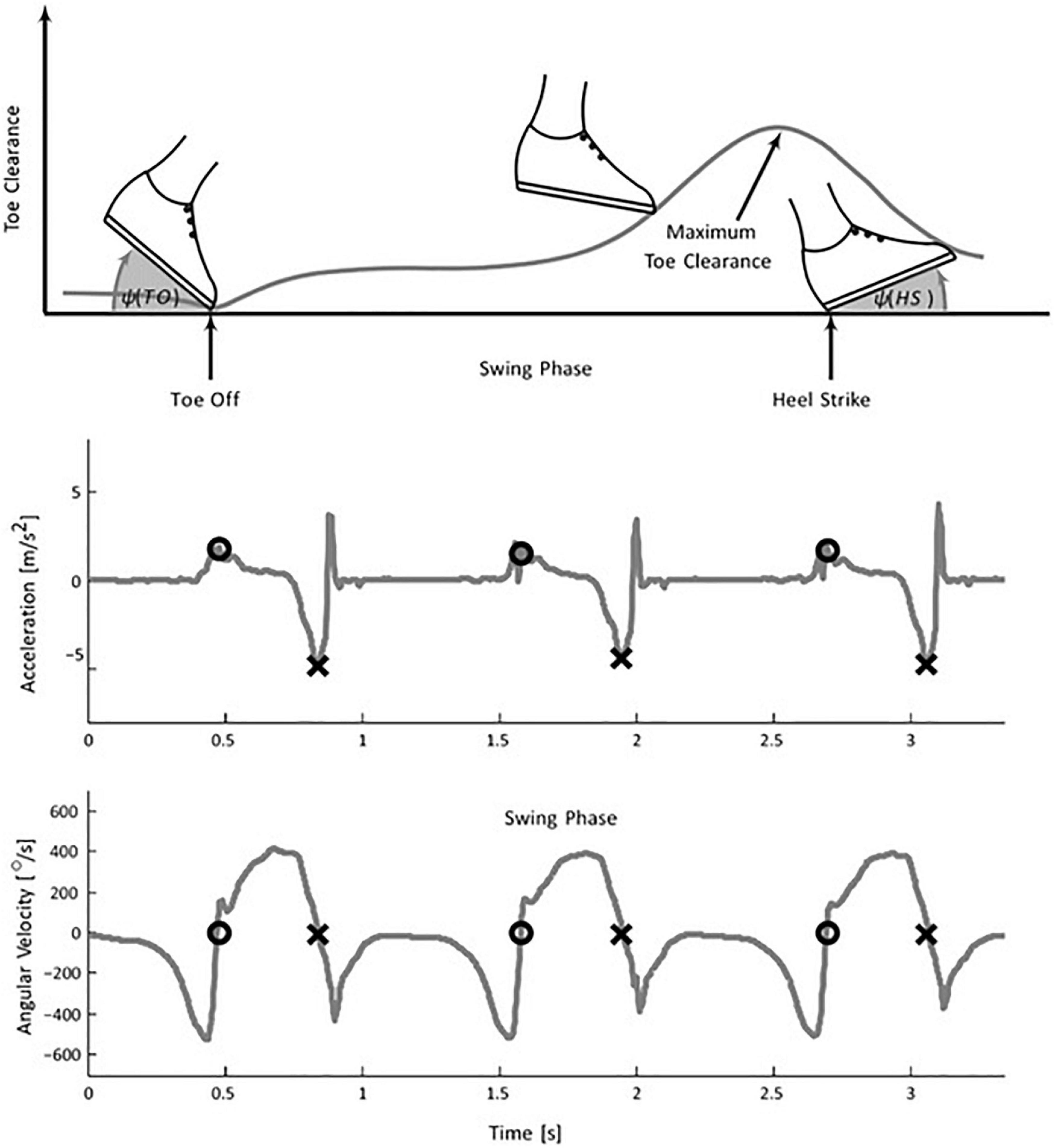
Figure 2. Foot mounted inertial gait pattern in the sagittal plane. (Top) the plot shows a variation of foot angle (w.r.t. ground) at TO, HS, and toes clearance during the swing phase. The negative peak of the acceleration signal determines the HS (middle plot) whereas (lower plot) TO is extracted using the zero crossing of the angular velocity signal [Image source: Schülein et al. (2017), under Creative Commons Attribution 4.0 International License].
Rampp et al. (2015) presented a method for detecting gait events and subsequently computing clinically relevant temporal and spatial parameters like stride length, stride time, swing duration, and stance time using an accelerometer and gyroscope. The stride length was calculated by double integrating the acceleration data obtained from an accelerometer. A work on TO detection for level ground and transition on-ramps was reported by Joshi et al. (2016) using a tri-axial accelerometer placed at the dorsum of the foot. The method uses the wavelet decomposition technique of foot acceleration data to derive a unique feature in a particular frequency band, yielding estimated TO occurrence. For repeatable reference of TO, foot switches were placed below the foot. The work also reported the detection of a transition from a level ground walk to ramps. However, as noted, the algorithm is limited to the identification of TO alone and has not been validated for detecting HS. Das et al. (2019) proposed a method based on the variation of foot inclination angle, measured using an inertial sensor-based wireless foot sensor module (WFSM) placed at the dorsum of the foot during walking to identify HS TO. The detection algorithm uses a peak detection and heuristic approach to mark these events.
The choice of the detection algorithm is based on the position of the sensor attachment as the signal patterns are strictly a function of the sensor placement on limb segments. The methods discussed above have the advantage of being easy to implement and require less computation power and tools. However, inherent noise in raw data, like multiple peaks and thresholds from inertial sensors, yields poor detection when gait is altered (Cuesta-Vargas et al., 2010; Chalmers et al., 2014). Techniques such as Fuzzy Logic (Alaqtash et al., 2011), Machine Learning (Mannini et al., 2016; Hannink et al., 2017), Neural Networks (Sun et al., 2012; Dehzangi et al., 2017) have also been used to overcome this. Such practices show improved accuracy and can handle complex signals. However, they require high computation time and power and thus are not always apt for real-time applications.
Although the earliest application of inertial sensors, especially accelerometers, was for gait event detection, most recent reports targeted kinematic gait features (Seel et al., 2014; Picerno, 2017; Dorschky et al., 2019). The first study that formalized estimating joint kinematics using inertial sensors (accelerometers) dates to 1990 (Willemsen et al., 1990). Accelerometers have been widely used for static tilt calculation, while a gyroscope has frequently been used for estimating rotational angles. A fusion of both these sensor inputs can yield information regarding joint angles and positions. Figure 3 shows the typical functional block for inertial-based estimation of parameters such as linear acceleration, position, tilt angle, etc.
Measurement of position and range of motion (ROM) of lower limb joints, viz., knee (Willemsen et al., 1990; Tong and Granat, 1999; Dejnabadi et al., 2005; Cooper et al., 2009; Favre et al., 2009; Takeda et al., 2009; Djurić-Jovičić et al., 2011; Seel et al., 2014), hip (Cutti et al., 2010; Horenstein et al., 2019; Teufl et al., 2019b), ankle (Kwakkel et al., 2007; O’donovan et al., 2007; Chang et al., 2016), and entire lower limbs (Findlow et al., 2008; Picerno et al., 2008; Sun et al., 2016), is one of the leading reported methods toward gait kinematics. Such methods rely on either calculation of individual segment kinematics (mainly in the hip and ankle) or the relative orientation between the proximal and distal sensor-embedded frames (in the case of knee joints).
Dejnabadi et al. (2005) presented a novel method for measuring the absolute angle of knee rotation by estimating the joint center of rotation acceleration. Two accelerometers were placed at the shank and thigh region, and their projections (virtual sensors) were set to adjacent segments at the center of rotation. As a result, the absolute value of knee joint rotation could be measured rather than the relative angle (commonly measured using information about adjacent limb inclination). The method thus eliminates the need for integration or cyclical nature to compute the angle, thereby minimizing integration or drift errors. However, subject-specific modeling with prior anatomical information is required.
A recent application of IMUs in movement analysis is toward gait and postural balance study, especially for patients with balance disorders like AD, PD, mild cognitive impairment (MCI), etc. Hsu et al. (2014) proposed an automated algorithm with input from a hip-mounted accelerometer for studying gait balance in AD and healthy controls (HC). The acceleration data of the waist was used to compute the signal vector magnitude and subsequently the 3-axis directional angle. The projection displacement of each step, estimated using the inclination angle, is translated in postural sway in the anterior-posterior and medial-lateral directions. The method was validated on a cohort of 21 AD and 50 HC under eight standard balance test protocols like Eyes open/close, single foot tandem stand, etc.
Wang Z. X. et al. (2015) presented an automated algorithm for performing the time-up and go test (TUGT) using inputs from 3 IMUs, one each placed at hip and both feet. A unique motion signature pattern, based on a pre-set threshold, recorded from the coronal plane is segregated to identify the start point and endpoint of the stand-up period and sit-down. The time required by an individual to perform, sit, stand, and finally complete the TUGT is calculated based on these events. A study with 46 subjects (21 AD + 25 HC) was performed to validate the proposed methodology.
One of the most detailed works on balance analysis using IS-based measurement is reported by O’brien et al. (2019) using a single IS placed at the hip. Gait events, namely HS and TO, were detected by finding the local minima and local maxima of the continuous wavelet transformed vertical acceleration component. The angular velocity along the vertical axis filtered through a fourth-order low pass Butterworth filter identifies the left and right foot. The lower limbs were modeled using an inverse pendulum model (IPM) for spatial feature estimation. The change in the height of CoM (obtained by double integration of vertical component of acceleration) was scaled in terms of step length. The frequency-domain features were extracted using the Bergs Balance Scale (BBS) for the static postural balance study. In contrast, the time-domain features were evaluated from acceleration and its differential and integral outputs. Table 2 tabulates significant inertial sensor-based gait analysis targeted at event detection, parameter estimation, and balance analysis.
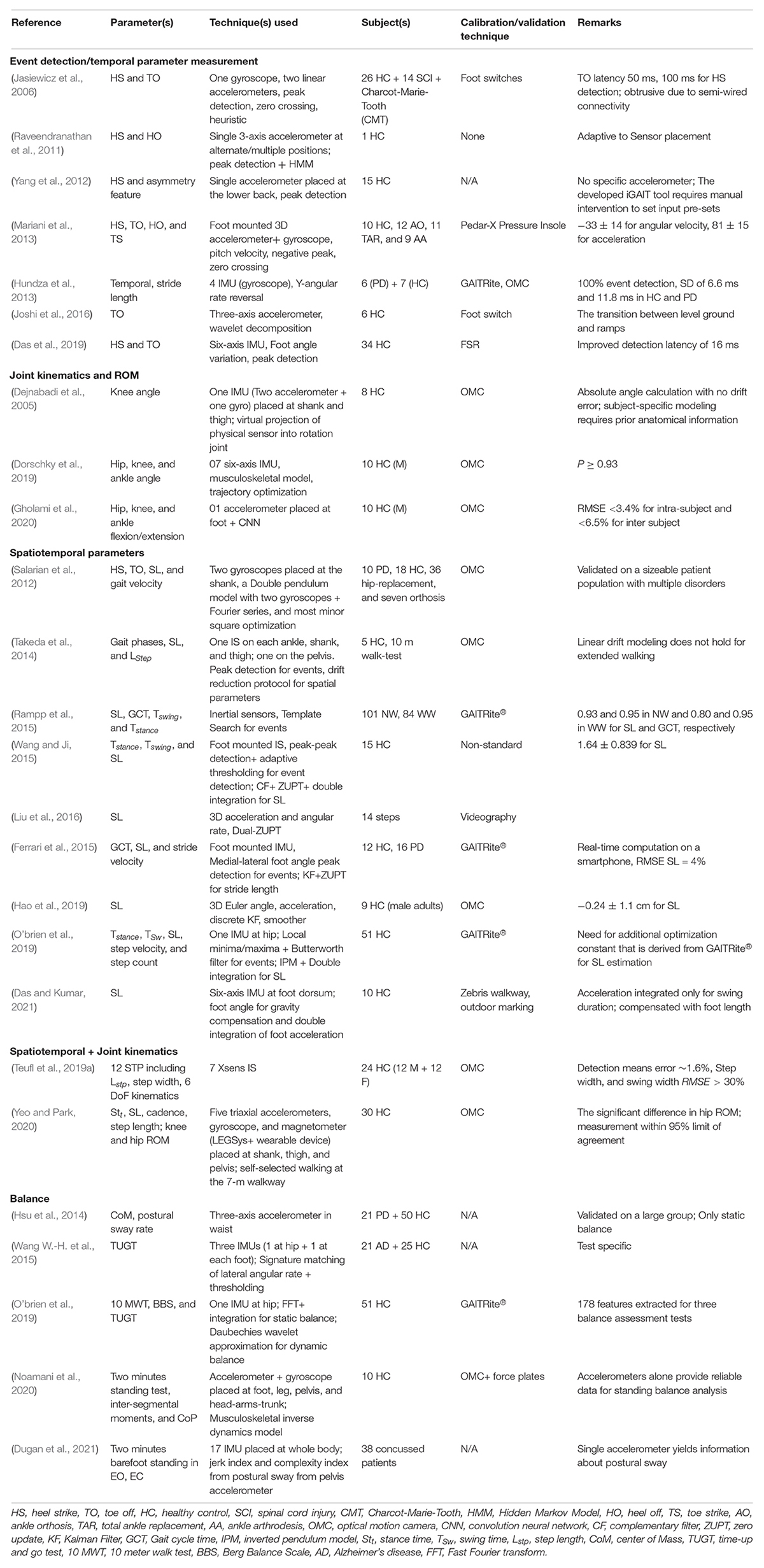
Table 2. Inertial sensor-based gait and balance analysis: from the prospect of event detection, spatiotemporal parameter estimation, joint ROM, and balance analysis.
To recapitulate, inertial sensors, including accelerometers, gyroscopes, and magnetometers owing to distinct advantages, presents probably the most promising alternative to laboratory-based gait analysis. The measurement from IMU-based methods has been reported for all the domains of gait measurement, i.e., from the very initial step of gait clinical gait assessment (event detection) to its use for automated quantification of disease severity (PD and balance grading). The integration of IMU sensor chips in modern smart devices and their widespread penetration all across society [smartphones (Lemoyne et al., 2010a,b; Mcguire, 2012; Kashihara et al., 2013; Susi et al., 2013; Lemoyne and Mastroianni, 2015, 2018; Sprager and Juric, 2015), wearable bands, etc.] are a positive aspect to look forward to the translation of such technologies for clinical benefit. However, there are still specific challenges to the domain that need to maximize the benefit and make IMU-based devices a conventional gait analysis tool. One of the definite challenges toward fulfilling it is the non-uniformity of technology and use. There is no consensus and guidelines for inertial sensor-based clinical gait evaluation, making it a technology-specific and subjective method. Subject preparation for trials also depends on the specific nature of the targeted parameter, which again is a significant challenge for deployment in clinical settings. Also, from a technology point of use, most of the reported work toward neuro-disorder diagnosis relies on certain aspects and characteristics of the disease. This limits the general use for evaluating other disorders.
Instrumented Foot Insoles for Gait Kinematics
Floor mounted plantar pressure measurement systems/force plates provide accurate and repeatable measurements. Such systems are widely used for clinical gait assessment and studying gait biomechanics worldwide. However, such stationary platforms have certain demerits, primarily due to the high cost and number of steps that could be recorded. To overcome these limitations, various research groups worked toward developing instrumented force insole that can measure specific plantar kinetic parameters. However, the initially reported methods (Hausdorff et al., 1995; Fraser et al., 2007; Yong et al., 2011) were based on wired communication that often disturbs the natural walk. However, with the advancement of material technology, components of standard plantar force and pressure measurement devices have become readily available in compact and robust forms. As a result, many novel wirelesses-based plantar gait measurement systems have been developed to study gait in naturalistic scenarios over a longer duration (Wahab et al., 2008; Yang et al., 2009; Liu et al., 2010; Crea et al., 2012, 2014; De Rossi et al., 2012; Donati et al., 2013; Howell et al., 2013; Mn, 2014; Qin et al., 2015; Bark et al., 2017; Arafsha et al., 2018; Zhang et al., 2019; Sorrentino et al., 2020; Tahir et al., 2020). While most studies reported the application of commercially available force sensors for their development (Howell, 2012; Howell et al., 2013; Mn, 2014; Qin et al., 2015; Bark et al., 2017; Arafsha et al., 2018; Tahir et al., 2020), few groups designed and fabricated the sensors as well (Wahab et al., 2008; Yang et al., 2009; Zhang et al., 2019; Sorrentino et al., 2020).
One of the detailed and earliest works toward a whole plantar pressure profile measurement system was reported by Howell (2012) and Howell et al. (2013). They reported an instrumented insole with 32 FSRs to cover the entire footprint area with more concentration in pressure hotspots. Each sensor was calibrated for force measurement using a load cell, and the contribution of each calibrated sensor was taken into consideration for measuring vGRF and ankle moment. The loading patterns were studied at each sensor during walking to sort an adequate number of sensors and their best locations. Subsequently, a second prototype was developed with 12 FSRs to measure vGRF, ankle, and knee moments. Using the developed insole and data recorded from the motion analysis laboratory, a database was generated and validated on HC hemiplegic patients using regular ankle-foot orthosis on stroke patients. A linear regression model with inputs from the database was used to develop each subject’s gait models. Testing data for each subject were used to predict the GRF and ankle and knee motion moments with appreciable correlations. Several FSR-based force insoles for gait analysis and related studies have been reported with the interplay of the number and location of sensors, parameters estimated from it, and application area (Liu et al., 2010; Qin et al., 2015; Tahir et al., 2020).
A novel and well-reported system for real-time foot pressure measurement based on silicon cell-based pressure-sensitive pad is reported by the research group of De Rossi et al. (2011, 2012), Crea et al. (2012, 2014), and Donati et al. (2013). The device, consisting of 64 pressure-sensitive elements, is embedded in an insole. The integrated signal conditioning electronic board with wireless communication and power source (battery) is placed inside a casing along the shoe’s lateral side. The authors calculated the vGRF, CoP, and a set of temporal parameters based on the amplitude and activation profile of the sensitive cell and validated against an AMTI force plate. The method thus reported has few distinct advantages in providing high spatial resolution plantar force data, insensitivity to temperature variation, and the need for only calibration. Pressure insoles currently are extensively used for studying gait and balance disorders associated with neurodegenerative motor symptoms, such as detection of FOG episodes in PD (Marcante et al., 2021; Pardoel et al., 2021; Shalin et al., 2021).
Electromyography
The muscles in a human compose about half of the total body weight. These are composed of bundles of specialized cells capable of contraction and relaxation in response to the stimuli received from the cerebral cortex. The contraction and expansion of skeletal muscles provide the force required to perform various actions in electrical signals. These signals range from a few hertz to 400 Hz and voltages ranging from approximately 10 μV to a few millivolts. The underlying chemical process produces a shortening of the contractile elements of the muscle cell. Electromyography (EMG) is a technique to record and measure muscle activities. Based on the placement of EMG electrodes on the human body, it can be either invasive or non-invasive (also known as surface-EMG).
Surface-EMG (sEMG), in particular, has been exploited for studying muscle activity during dynamic activities such as walking, particularly in gait events and phase recognition for healthy as well as neuro-impaired gait (Ryu and Kim, 2017; Ziegier et al., 2018; Morbidoni et al., 2019; Nazmi et al., 2019; Di Nardo et al., 2020; Keloth et al., 2021; Zahra, 2021; Rezaee et al., 2022). Nazmi et al. (2019) proposed a classification approach for the segregation of stance and swing phase (HS and TO detection) from feeding EMG signal to an artificial neural network. EMG has also been used for intent, like sit to stand detection (Rasool et al., 2012; Chorin et al., 2016; Li B. et al., 2016; Roldán Jiménez et al., 2019; Bhardwaj et al., 2021) and quantitative localized muscle fatigue estimation (Boudarham et al., 2014; Ropars et al., 2016; Roldán Jiménez et al., 2019; Parent et al., 2019) during gait. Although fatigue is considered a multidimensional concept involving both physiological and psychological implications, the former dimension of fatigue can be observed in both the central and peripheral system domains (Zwarts et al., 2008) and is a widely accepted tool for fatigue estimation (Al-Mulla et al., 2011). Analysis of the EMG frequency spectrum (mean, median) is the most widely explored technique for fatigue estimation, and localized muscle fatigue often results in a downward shift of the frequency content of the EMG signals (Naik, 2012). Besides being an everyday activity humans perform in their daily lives, a sit-to-stand task is also commonly used in clinics to evaluate lower limb muscle function. Moreover, it is immensely practiced for therapeutic rehabilitation exercises targeting muscle strengthening, balance improvement, and gait therapy.
Chorin et al. (2016) analyzed EMG and force data from 40 subjects (10 elderly fallers, 30 non-fallers) and concluded that the gastrocnemius lateralis muscle activity differs significantly between fallers and non-faller group. Similarly, Li B. et al. (2016) detected sit-to-stand transitions from the quadriceps EMG data integrated with upper Trunk kinematic data. Roldán Jiménez et al. (2019) evaluated the fatigue during the 30-second sit-to-stand (30-STS) test on a young obese and sedentary woman to ensure fatigue during the trial. The subject was otherwise free from cognitive disorders, musculoskeletal, bone, or joint injury. The muscle activity was measured at six locations of the dominant side (GM, BF, VM, AR, ES, RF, SO, and TA) at a frequency of 1,000 Hz. Muscle fatigue estimation for neurological disorders such as hemiplegia (Boudarham et al., 2013, 2014; Wang et al., 2017; Mazzoli et al., 2018; Fujita et al., 2020, 2021), PD (Huang et al., 2017; Palinkas et al., 2019; Cao et al., 2021), MS (Octavia et al., 2015; Porcaro et al., 2019; Stańczyk et al., 2019) has been reported. The approach for EMG-to-muscle force has also been analyzed in Bogey et al. (2005). Furthermore, EMG signals have been reported to be used to measure other gait characteristics like joint kinematic plots. The joint angular motion recorded simultaneously with EMG data is correlated.
Miscellaneous Sensors
In addition to the widely used sensing methods discussed above, researchers have explored the possibility of the novel application of alternate sensors/techniques for gait analysis. A few such measurement techniques are discussed below.
Force Myography
Force myography measures the external muscle force/pressure generated during human activity. When strapped around a limb circumference using a bracelet/socket, force Sensors measure the outward force developed due to the volumetric changes resulting from the displacement of muscles, tendons, and the skin (Castellini et al., 2014). Research groups have proposed and demonstrated the application of different sensors like piezoelectric (Li et al., 2016b; Ha et al., 2018), capacitive (Truong et al., 2018), flexible fabric (Rasouli et al., 2015), and optical sensors (Fujiwara and Suzuki, 2018). However, the most commonly used sensor for FMG application is FSR, and FlexiForce Sensors, made out of Resistive polymer thick film material (Xiao and Menon, 2019). These methods have been widely used (almost 90% of work-related to FMG) to study and control an upper limb, especially arm-related movements (Xiao and Menon, 2019). Lukowicz et al. (2006) first presented the use of FMG to segregate four types of walking. Signal patterns from FSRs strapped at the thigh were used for classifying locomotion patterns. Yungher et al. (2011) demonstrated the correlation of FMG (surface muscle pressure) with surface muscle electrical activity during gait. FMG signal patterns showed better stride-to-stride consistency than sEMG signals.
Similarly, the study reported in Belyea et al. (2018) also supported the correlation of FMG and sEMG signals, with FMG signals being reported as a better indicator for understanding the fatigue label. Although these studies show potential for application toward gait analysis, the objectives/outcomes of these reported works do not precisely meet the requirement of clinical gait analysis with targeted parameter evaluation. Two recent results (Godiyal et al., 2018; Jiang et al., 2018), using FMG, have been reported for gait event/phase detection.
The work reported by Godiyal et al. (2018) presented a method for detecting HS and TO for overground and ramp walking, including transition. Eight FSRs, evenly placed in a bracelet was, strapped around the subject’s thigh such that each FSR aligned corresponding to prominent thigh muscle. A foot insole system for ground truth/reference data synchronized with the FMG measurement system. A classifier was trained with reference signatures extracted during the training phase that consisted of two signature patterns (one each for HS and TO) for each locomotion mode. Hence, the system could successfully match any of the three-locomotion modes for a test FMG signature. However, the detection framework is subject-dependent as a separate database is created for every trial subject.
Another subsequent work on gait phase detection is reported by Jiang et al. (2018) and Jiang (2018) for event detection in treadmill walking. During gait, periodic contraction and relaxation of the extensor and flexor muscles at the ankle alter the pressure distribution. An FMG band, consisting of eight equispaced instrumented FSRs, was strapped around the ankle to record these distinctive FMG patterns (Figure 4). A high-speed motion camera was used for referencing four instances: HS, MSt, PSw, and Sw. A 125 ms sliding window with 93 ms overlap was used to extract a set of 14 distinguishable features, and a Linear Discriminant Analysis (LDA) technique was implemented to classify the gait phases further.
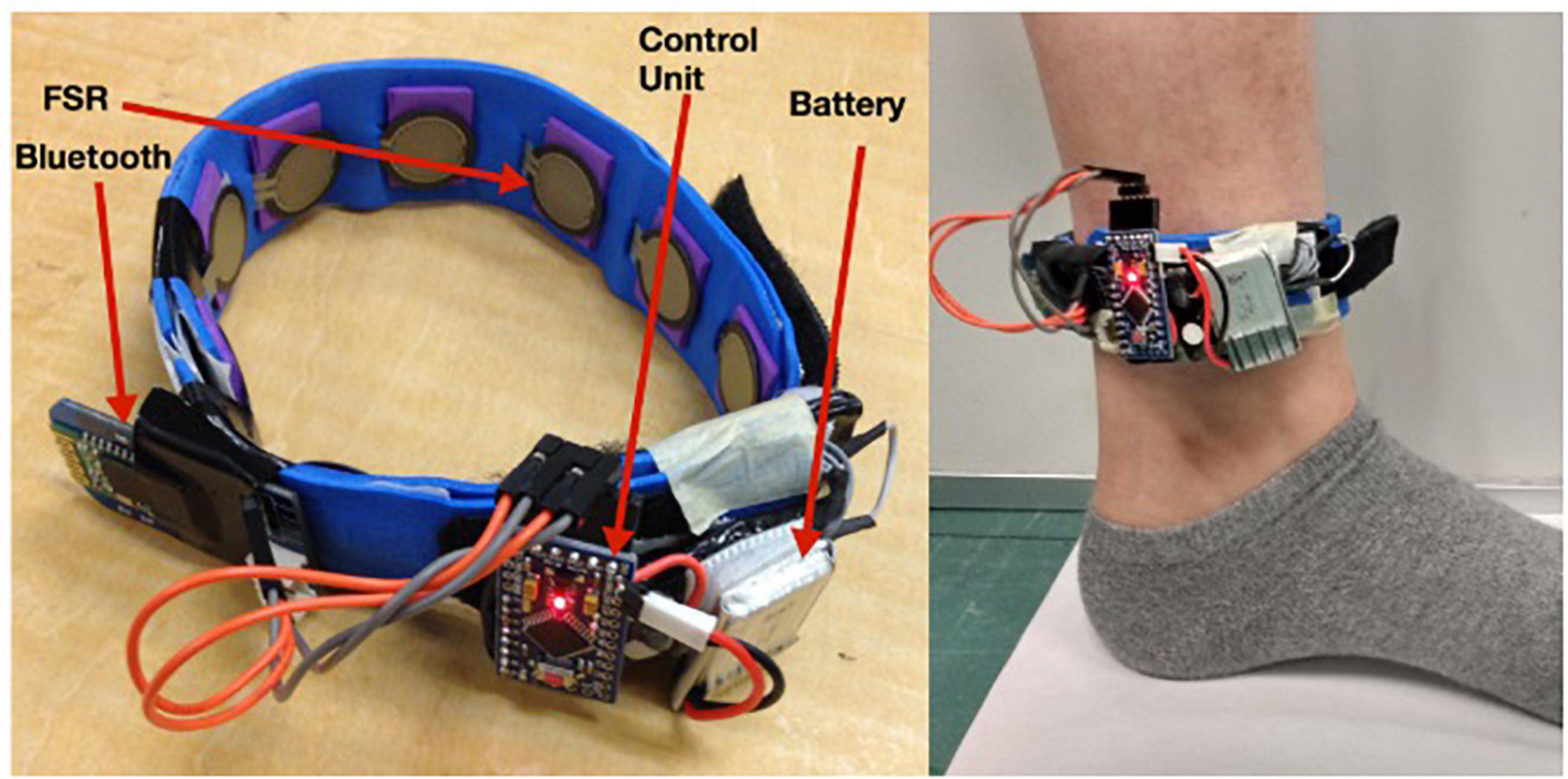
Figure 4. An FSR sensor-based force myography band for gait application [Reproduced with permission from Jiang (2018), Memorial University of Newfoundland].
Piezoelectric Sensors
Piezoelectric sensors, when stressed, generate electric potential and are often used for dynamic pressure or vibration measurement. The most common piezoelectric sensors are made of either ceramic-based or MEMS-based material. Due to the parasitic effect of piezo materials, the ceramic piezoelectric sensors are not suitable for static or low-frequency measurements (Tahir et al., 2020). However, the MEMS sensors possess distinct advantages: they can be used for 3-axis measurements they generate positive or negative (amplitude) electrical impulses depending on the direction of force acting around each axis. They help detect human motion primarily due to their flexibility, wide frequency range (0.001 Hz to 10 MHz) (Tahir et al., 2020).
Balbinot et al. (2014) designed a footswitch system for HS and TO detection using a piezoelectric transducer out of a buzzer. The Piezo sensors, calibrated for force measurement, were attached beneath a rubber insole at the hallux and heel region. HS and TO were defined as the instances when the force signal exceeded or fell from a threshold of 5N, respectively. These events, thus detected, were further used for deriving a set of temporal gait parameters. The results were validated with a standard force plate (AMTI Inc., United States).
A gait recognition based on flexible piezoelectric sensors [polyvinylidene fluoride (PVDF)] is reported by Cha et al. (2018). Four flexible sensors, one each at the knee and hip of both legs, were attached on an item of loose clothing. The PVDF sensor generates an electric voltage that corresponds to the bending of the corresponding limb joint. The periodic motion component, extracted from the FFT of the time-varying signal, correlates to the gait speed. The system was also able to detect different postural transitions between standing and walking.
Although explored widely, the majority of the piezoelectric sensor-based gait measurement systems focus on analyzing vertical components of pressure/force. However, the shear stress provides vital information especially involving a pathological gait (Hamatani et al., 2016; Jeong et al., 2021). Moreover, crosstalk arising due to the co-existence of piezoelectric coefficients significantly impacts the measurement accuracy (Dai et al., 2020). To address these challenges, recent works (Chen et al., 2020; Dai et al., 2020; Gao et al., 2020) have proposed using multilayer structure with piezoelectric films in distinct polarization orientations (Gao et al., 2020), lamination technique for assembling electrodes with functional films (Dai et al., 2020), and signal decomposition technique to separate the raw signal into several intrinsic mode functions (Chen et al., 2020).
Wearable Microphone
The foot is the anatomical structure that is in contact with the ground during walking, and exploration of foot-ground interaction (footstep sound) can reveal important aspects of gait. Microphone sensors, being tiny, low-cost, portable, and readily configurable with embedded electronics, has promising application for capturing footstep sounds generated due to ground-foot impact. Although very limited, footstep sound or gait acoustics have also been explored for gait analysis (Makela et al., 2003; Altaf et al., 2015; Hwang and Gim, 2015). The first wearable acoustic sensor-based gait parameter estimation method was reported by Wang et al. (2016). They presented an event detection method using the wearable microphone to collect footstep sound signals during walking. One microphone attached at the ankle of each foot, such that the microphone holes face toward the ground, was used to collect the sound resulting from different foot parts. Based on the spectral analysis of the sound, a set of 36 features were extracted that included correlation coefficients, energy bands, zero-crossing rate, linear prediction, and mel-frequency coefficients. A support vector machine (SVM) based approach was used to identify and classify step detection, HS, and toe-on, and a set of 9 STP were estimated by fusing both feet sound data. The study was validated on 15 healthy subjects with a detection accuracy of nearly 95%. Jesus et al. (2019) presented a case report on estimation of gait cadence, speed, and stride/step duration for a PD subject (stage 3 on Hoehn and Yahr’s scale) from the gait acoustic signal captured using a microphone. The parameters thus recorded were validated against a motion capture system.
A very recent and exciting feasibility study for using an ear-worn wearable (Earable) microphone for gait identification is proposed by Ferlini et al. (2021). Their work (called EarGate) demonstrates the feasibility of gait identification from walking sound generated and propagated through the musculoskeletal system in the body. The EarGate system employs an inward-facing earphone to leverage the occlusion effect (low-frequency components of a bone-propagated sound when the ear canal orifice is sealed) during the wearer’s movement from inside the ear canal. The proposed method identified 31 healthy subjects with an overwhelming balance accuracy of over 97%. Chiang et al. (2019, 2020) explored the self-doppler effect using a microphone coupled with three buzzers (mounted on a shoe) to detect gait events and compute step length, orientation, and posture.
Ultrasonic Sensor
An ultrasonic sensor computes the distance of a target object by emitting short, high-frequency sound waves at regular intervals. When the sound pulse encounters an object in its path, it has reflected the transmitter as an echo signal. This signal reflects a measurement of the distance of the object/target from the source based on flight time. Such time-of-flight-based measurements possess a distinct advantage in suppressing background interference compared to intensity-based measurements. Recent ultrasonic sensors can measure very minute distance change, showing potential for gait analysis application.
Huitema et al. (2002) presented a semi-wearable (hybrid) system for measuring spatiotemporal parameters using ultrasonic sensors. An ultrasonic transmitter, fixed at a stationary position in the room, sends bursts of periodic pulses to the two receivers attached at both shoes of the subject. The propagation delay recorded between transmission and reception is used to localize the position of the subjects’ feet. Information about the dynamic position of the foot and zero foot-velocity phase was used to calculate step length and stride length. Gait events, namely HS and TO, were calculated from the speed plot variation within a single step by applying pre-set thresholds. The study was validated on four healthy subjects.
Another work on the semi-wearable approach by Qi et al. (2014) reported the measurement of 3D foot trajectory using a wireless ultrasonic sensor network. Contrary to Huitema et al. (2002), the ultrasonic transmission unit (mobile) was fixed at the shoe. At the same time, there were four receiving units (anchor), set at 3D planes across the room, all interconnected and synchronized wirelessly. The system records the time-of-flight of the ultrasonic sound waves from mobile to anchors and measures the distance covered by the foot. The 3D foot trajectories thus computed were validated using eight motion camera-based systems while tracking three reflective markers, systematically fixed at the subject’s foot with an RMS error of 4.2%.
Multimodal In-Shoe Approaches
Researchers all across have been trying to integrate/embed one or more of these techniques, as mentioned earlier, to develop a wearable gait analysis system (especially an in-shoe based) that can deliver the majority of clinically relevant kinetic and kinematic gait parameters. The shoe-based measurement offers distinct advantages in requiring the least preparation time, providing a surface for embedding electronics and hardware with minimal or no aesthetic change. One of the earliest and most comprehensive (and highly referred) works on multimodal shoe-based gait system development is the GaitShoe by Bamberg et al. (2008) from the Massachusetts Institute of Technology (MIT). GaitShoe includes 3-axis (orthogonal) accelerometers and gyroscopes, four foot-force sensors, two bidirectional bend sensors, two dynamic pressure sensors (PVDF strips), as well as electric field height sensors. The accelerometers estimate SL, SV, and displacement, whereas Gyroscopes measure the foot orientation. The force-sensitive resistors were used for two targets: first to analyze the force distribution under the foot and second to detect gait events, namely HS and TO. The PVDF strips were used as redundant event detection while the electric field sensor analyzed the foot clearance from the floor. The bend sensor records the foot plantar flexion/dorsiflexion variation at metatarsal points. The GaitShoe system physically consists of an instrumented insole that houses FSR, PVDF, bend sensor, and field sensor with an attachment housing the accelerometers, gyroscopes, and hardware electronics. The system performance was validated on healthy and pathological (Parkinsonian) gait. However, this system still needs a high level of customization for regular use.
A similar recent system (eSHOE) based on integrated sensors packaged in a shoe is reported by Jagos et al. (2017). The developed wireless eSHOE includes a six-axis IMU with four FSR sensors used for calculating eight temporal parameters: gait cycle time, stance time, swing duration, step time, single support duration, initial double support, double terminal support, and cadence. The system’s performance was validated against GAITRite® walkway, a standard plantar pressure and gait analysis system used widely for computing STP. The measured parameters collected during a study of 10 subjects with a proximal femur fracture from eSHOE showed a significant correlation with the parameter recorded from GAITRite®. However, this system functionality is limited to measuring temporal feature sets only, emphasizing spatial features, essential outcomes of clinical gait analysis. Similar in-shoe-based multimodal measurement systems have been reported for application in rehabilitation tasks (Benocci et al., 2009; Edgar et al., 2010; Wada et al., 2010).
Commercial Ambulatory Wearable Gait Sensors
Research in WS-based gait analysis system development has been ongoing for the last two decades. However, there are minimal commercial WS-based gait analysis systems available in clinics and research setups. One prominent WS system is the Xsens Motion System that uses inertial trackers to capture full-body kinematics of the body with a wireless communication suit. It is widely used in gait motion and biomechanics study (Zhang et al., 2013; Loose and Orlowski, 2015; Schepers et al., 2018) and also serves as a referencing system for validating custom-developed gait measurement methods and protocols (Cutti et al., 2008; Ferrari et al., 2010; Wouda et al., 2016).
Another very recent product based on inertial sensor-based for gait and biomechanics study is “Mobility Lab” from APDM Wearable technologies (Eftekharsadat et al., 2015). The system can perform a standardized test based on clinical protocol, and a patient report is generated for assessment by the clinicians. The system can report performance regarding gait STP, arm kinematics, lumbar postural sway, and sit-stand parameters. Many experimental studies have been reported for studying gait pathologies (Horak et al., 2016; Pal et al., 2016; De Souza Fortaleza et al., 2017; El-Gohary et al., 2017).
An in-shoe plantar pressure measurement system called F-Scan (Júlio et al., 2020) provides dynamic pressure, force, and timing information for foot function and gait analysis. The system targets two user bases: Researchers and Clinicians with additional research software that provides researchers access to raw and processed data in-depth. For medical practitioners, the system generates automated reports. This system is often used to optimize and customize therapy and understand foot biomechanics (Mueller et al., 2003; Thies et al., 2007; Fourchet et al., 2015). Another insole-based plantar force measurement system with integrated IMU is available from Moticon (Moticon ReGo AG, Munich, Germany) (Barratt et al., 2021). It is an entirely wireless device such that the insoles can be embedded into any pair of shoes for continuous and unobtrusive data collection. It also has onboard data recording for outdoor and more extended data recording.
Advances in Therapeutic Intervention
Despite the widespread prevalence of neurodegenerative disorders, the current treatment (pharmacological) options focus on providing symptomatic relief and slowing down the progression. Presently, no effective drug is available to cure or prevent neurodegenerative disorders. They are also reported to produce adverse health effects. For example, Levodopa (L-dopa) is the most commonly prescribed drug for managing early-stage motor symptoms of PD (Chagraoui et al., 2020). Still, it is also reported to cause high dyskinesia and relapse of PD symptoms (Turcano et al., 2018). Also, several disorders exhibit similar symptoms but vary in terms of mechanisms of their pathogenesis. These challenges demand an effective therapeutic intervention to slow down or halt the progression and present a permanent cure. Thus, researchers and clinicians have been looking for promising alternate, personalized, targeted therapeutic strategies to combat such neurodegenerative gait/postural impairments. This section highlights three current paradigms of neurodegenerative disease therapy design: Wearables, virtual reality (VR), and phytochemicals to improve neuro-motor functions.
Wearable and Virtual Reality Technologies for Gait and Balance Rehabilitation
Taking a step forward from gait analysis, wearable devices are also being explored to assist/rehabilitate to tackle neurological gait disorders (Bowman et al., 2021). These devices perform real-time computation/detection based on kinematic, kinetic, and other physiological parameters and provide desired biofeedback (auditory, visual, vibratory, etc., cues) on detecting gait or postural anomaly (Mazilu et al., 2015; Lazarou et al., 2016; Pacilli et al., 2016; Pereira et al., 2016; Mendoza et al., 2017; Suppa et al., 2017; Amato et al., 2018; Rossi et al., 2020; Saif et al., 2020; Muurling et al., 2021; Stavropoulos et al., 2021). Such an approach influences motor learning in patients by engaging them toward continuous and real-time rehabilitation. Wearables, often compact and lightweight, possess a distinct advantage of being suitable for long-term and outdoor use, thereby enabling the user to experience and learn during realistic scenarios.
Virtual reality (VR) is another technology-driven paradigm shift in therapeutic intervention toward physical and cognitive rehabilitation of neurological disorders (Voinescu et al., 2021). VR-based rehabilitation technique creates a realistic experience by projecting virtual environments (through immersive/non-immersive displays). Physiotherapy is one of the earliest and most effective forms of therapy for gait and balance rehabilitation. However, it is a monotonous task that requires individuals to perform specific tasks repeatedly, and the outcome for such activity is not available for immediate realization. As a result, often, the individual loses interest in the activity. The VR-based intervention addresses this challenge by translating the standard therapeutic exercise protocols into interactive games (actions). The real-time measurement from the body segments (hands, leg, head, etc.) is used as biofeedback signals to update the therapy (game) environments. This results in better patient engagement in the physiotherapy session, ensuring a more effective outcome (Voinescu et al., 2021).
Phytochemicals and Its Neuroprotective Roles
With the significant shortcomings associated with available drugs for the management of neurological disorders, researchers have been investigating the discovery of molecules that can effectively cure/prevent the pathology. The major pathological features associated with neuro-disorders are oxidative stress, neuro-inflammation, and aggregated proteins. Naturally, derived compounds from the plant (phytochemicals) have been widely used for extracting clinically valuable compounds. For example, the common flowering quince (FQ) has been used traditionally to treat migraine, neuralgia, depression, tremors, and dyskinesia (Zhao et al., 2008). Over the recent years, researchers have been exploring various phytochemicals like Berberine (BBR), Curcumin, Ginsenoside, Puerarin, etc., with potential application toward the management of neurodegenerative disorders and symptoms (Price et al., 2012; Lin et al., 2018; Corpas et al., 2019; Nair et al., 2019; Wang et al., 2019; Calfio et al., 2020; Balakrishnan et al., 2021). For example, phytochemical like Resveratrol (a bioactive component of red wine) (Price et al., 2012; Lin et al., 2018; Corpas et al., 2019; Nair et al., 2019) and Melatonin (Wang et al., 2019) have shown reported benefit, both in vitro and vivo conditions, toward improving the mitochondrial function believed to be one primary cause for diseases like AD and PD. Resveratrol, at a very low concentration, has also demonstrated its benefit toward preventing α-synuclein aggregation (Eschbach et al., 2015; Gautam et al., 2017). Apigenin (AGN), a flavone class phytochemical known for anti-inflammatory and free radical scavenging activities, is also reported to alleviate α-synuclein accumulation significantly and mitochondrial dysfunction in rotenone-induced PD rats (Anusha et al., 2017). Many studies reported promising results toward effective management of cognitive and non-motor functions (Balakrishnan et al., 2021). However, compounds such as BBR, quercetin, ferulic acid (FA), etc., have also shown promising motor functions in neuro-disorder induced rat and mice models (Chen et al., 2002; Van Kampen et al., 2003; Zhao et al., 2008; Kim et al., 2014; Jiang et al., 2015; Yadav et al., 2016; Askar et al., 2019; Madiha et al., 2021). For example, blueberry supplementation (rich in polyphenols) in the geriatric population effectively checks the decline of functional mobility and improves the performance of activities of daily living (Schrager et al., 2015). BBR showed effectiveness toward preventing memory loss in PD (Kim et al., 2014) and alleviating motor dysfunction in PD (Kim et al., 2014) and HD (Jiang et al., 2015) in mice models. Quercetin stands as a strong potential candidate for application in PD as it enhances antioxidant enzyme activity, thereby improving motor function (Madiha et al., 2021). When subjected to FA doses, rotenone-induced PD mice improved neuromotor function and muscle exercises (Askar et al., 2019). Curcumin, a polyphenol in turmeric, is one of the most promising natural compound against AD (Serafini et al., 2017; Reddy et al., 2018) owing to its β-amyloid inhibition and antioxidant solid property (Tang and Taghibiglou, 2017; Su et al., 2020; Utomo et al., 2021). An established ROS scavenger (Cao et al., 2008; Barzegar and Moosavi-Movahedi, 2011), curcumin also safeguards mitochondria against peroxynitrite in nigrostriatal, making it a strong therapeutic agent for AD and PD (Ramires Júnior et al., 2021). It is also reported to induce neuroplasticity in rats (Choi et al., 2017; Fan et al., 2018). Another widely extracted Phyto-compound, Ginseng, was reported to have improved dopaminergic neuronal loss and gait disturbance in PD mice models (Van Kampen et al., 2003). Table 3 summarizes the motor and biomechanical implications of common neurodegenerative gait disorders and the ongoing novel therapeutic approaches to mitigate the common gait and balance impairments accompanying them.
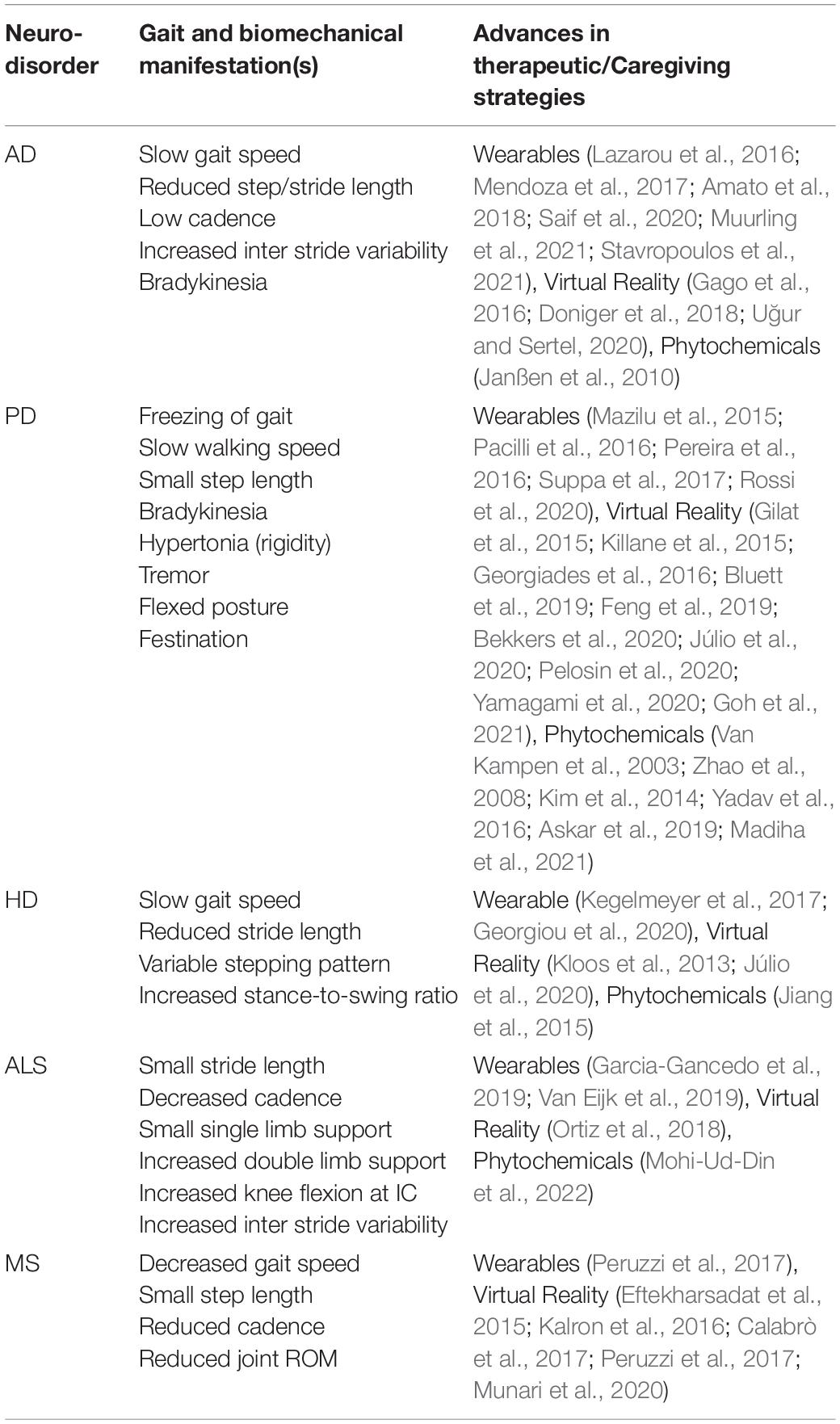
Table 3. Current progress in non-pharmacological therapeutic and rehabilitation measures for neurodegenerative gait and motor functions.
Discussion
Gait and balance analysis offer a medium to not only understand the locomotor/functional impairments but a way to accurately diagnose neurodegenerative disorders like PD, AD, MS, etc., that otherwise have almost similar non-motor symptoms. Despite being accurate and reliable, conventional gait laboratory-based measurement setups suffer from the limitation of being costly, bulky, and requires specialists to operate. Various research groups have researched WS-based gait analysis globally to address these challenges. We present a detailed review of all such recent progress (from 2005) in WS-based gait analysis based on the application of IMU, pressure sensor, EMG, FMG, Piezoelectric sensor, Microphone, and ultrasonic sensors along with available commercial systems. The review was performed covering certain clinically relevant aspects (parameters) of neurodegenerative gait disorders, and below, we summarize the role, applicability, and technique of the aforementioned sensors toward them. In addition, a summary of ongoing efforts toward mitigation of neurodegenerative gait disorders through application of wearable and VR technologies and the potential role of phytochemicals for alleviating such conditions have also been presented.
Event Detection
Gait events like HS, TO, and FF are critical to gait partitioning and signify the phase shift between various walking states. Almost all reported sensors from IMUs to EMG are explored to detect such events in both healthy and impaired gait. Commonly used detection techniques include thresholding (in case of IMU, footswitches, pressure sensors, wearable microphone, and piezoelectric sensors), rate reversal/peak detection/zero-crossing (IMUs) (Jasiewicz et al., 2006; Raveendranathan et al., 2011; Yang et al., 2012; Hundza et al., 2013; Mariani et al., 2013; Joshi et al., 2016; Montero-Odasso and Perry, 2019), PSD and frequency analysis (for EMG and FMG) (Ryu and Kim, 2017; Ziegier et al., 2018; Morbidoni et al., 2019; Nazmi et al., 2019; Di Nardo et al., 2020; Keloth et al., 2021; Zahra, 2021; Rezaee et al., 2022). Researchers have focused on applying machine learning too for handling complex signals (from IMUs) associated with impaired gait for such event recognition.
Kinematic Analysis
Joint and limb trajectories offer a proper way to understand the biomechanics of walking. Among all the reported sensors, IMU contributes to an overwhelming majority of research into understanding the kinematics of locomotion. From head to toe and range of motion to pose estimation, IMUs have been extensively used (Dorschky et al., 2019; Teufl et al., 2019a; Gholami et al., 2020; Yeo and Park, 2020). Recent effort toward the analysis of joint angles using instrumented force insole has also been reported using an artificial neural network (Li G. et al., 2016).
Kinetic Analysis
Understanding of forces and torques associated with walking is equally important. The most common kinematic parameter explored in gait analysis is ground reaction forces. The force and pressure sensors embedded into footwear have been investigated by most researchers for healthy and impaired gait (Marcante et al., 2021; Pardoel et al., 2021; Shalin et al., 2021). Recently, the application of inertial sensors has also been studied for the estimation of GRF and joint moments (Kodama and Watanabe, 2016). EMG and FMG are the other two techniques commonly used for measuring muscle forces.
Spatiotemporal Analysis
Gait presents a series of time and length domain data crucial for quantitative and qualitative gait evaluation. Although almost all the mentioned sensors capable of event detection are useful for temporal parameter measurement, IMUs integrated with a human model are reported widely toward estimation of spatial parameters such as step/stride length and step width (Salarian et al., 2012; Takeda et al., 2014; Ferrari et al., 2015; Rampp et al., 2015; Wang and Ji, 2015; Liu et al., 2016; Hao et al., 2019; O’brien et al., 2019; Teufl et al., 2019a; Yeo and Park, 2020; Das and Kumar, 2021).
Balance Analysis
Center of mass, CoG, and postural sway are the most common indicator of postural balance corresponding to gait and stance. Instrumented foot insoles are the most commonly used technique for measuring (Marcante et al., 2021; Pardoel et al., 2021; Shalin et al., 2021), along with a few reports using IMUs (Hsu et al., 2014; Noamani et al., 2020; Dugan et al., 2021). In addition, such methods are also reportedly used for automated and quantified measures of standard balance scales such as 10 MWT, BBS, 2 min standing test, and TUGT (Wang Z. X. et al., 2015; Kodama and Watanabe, 2016; Noamani et al., 2020; Dugan et al., 2021). However, gait and balance analysis have already been commonly adopted for physiological and functional monitoring; limited reliable and straightforward wearable devices are used in non-specialized clinical settings. Realistic implementation of WS in the clinical setting demands limiting the number of sensor devices for long-term monitoring. Although a more significant number of sensors placed at different body segments yields higher spatial features, at the same time, it increases computational as well as data analysis complexity along with system cost. Moreover, the outcome/output from a gait analysis system should contain clinically meaningful and comprehensive gait features. To minimize clinicians’ temporal, physical, and cognitive burdens, it is highly desirable to have the fewest number of devices possible to assess performance. Although the state-of-art commercially available systems provide widely accepted gait parameters, they lack objectivity in data interpretation. Clinicians analyze the data based on their expertise and experience to draw inferences for disorder correlation. This is a time-consuming and burdensome process and often suffers from subjectivity limitation. An added “intelligence” to the WS-based measurement to automatically correlate normal and pathological gait would assist the clinicians in better evaluation of the derived gait features. Progress has been made in research in automatic neurodegenerative disorder classification using gait features during the last few years. However, most reported research on gait impairment identification algorithms involves input features from laboratory-based measurement (NWS) systems.
Therapeutic Advances
Although the exact pathogenesis of most neurodegenerative disorders like PD, AD, MS, ALS, etc., couldn’t be ascertained yet, some distinctive hallmarks have been associated with them. The common pathologies reported includes mitochondrial dysfunction (common in AD, PD, and MS) (Correia et al., 2012; Carvalho et al., 2015; Rahman and Rhim, 2017), accumulation of misfolded α-synuclein (Chen et al., 2019), aggregation of Amyloid-β (Aβ) senile plaques (Malafaia et al., 2021), up of dopamine (DA) depletion (Bastide et al., 2015), neuroinflammation, oxidative stress, and induced endoplasmic reticulum (ER) stress, formation of reactive oxygen species (ROS) (Manoharan et al., 2016), to autoimmune-mediated loss of myelin and axonal damage (Jones et al., 2017), etc. Numerous bioactive phytochemicals have gained special attention as potential neuroprotective agents that help in mitigating such associated pathologies. For example, Curcumin (present in turmeric) exhibits multitude of benefits including antioxidant, β-amyloid inhibition property (Tang and Taghibiglou, 2017; Su et al., 2020; Utomo et al., 2021), ROS scavenging (Cao et al., 2008; Barzegar and Moosavi-Movahedi, 2011), alleviating mitochondrial damage (Ramires Júnior et al., 2021). Similarly, AGN has shown usefulness through anti-inflammatory and free radical scavenging activities, which in turn clear α-synuclein accumulation (Anusha et al., 2017). Mitochondrial function improvement has been reported using Resveratrol (Price et al., 2012; Lin et al., 2018; Corpas et al., 2019; Nair et al., 2019), AGN (Wiik, 2016), Curcumin (Ramires Júnior et al., 2021), Apigenin (Anusha et al., 2017), Curcumin (Cao et al., 2008; Barzegar and Moosavi-Movahedi, 2011), Quercetin (Madiha et al., 2021) are the most commonly investigated compounds showing strong anti-oxidant properties.
Other than pharmacological therapeutic interventions, efforts are also underway to provide a solution to tackle some of the significant motor challenges associated with neuro-disorders through VR and wearable devices. Both this class of devices performs real-time analysis of body movements (including gait) and generates various forms of countermeasure signals. The major benefit such approach offers is it provides a way for motor and cognitive learning, thereby ensuring effective and long-term rehabilitation.
Future Direction
For over two decades, tremendous efforts in wearable gait analysis have shown some fruitful results and translation to commercial products like XSENS F-Scan. Currently, such systems are parallelly used along with the gold standards like force and motion capture systems. However, these systems’ broad and consensual clinical applicability demands further work and improvements. As a takeaway from the literature review performed, below are some of the critical suggestions presented by the authors toward effective, easy-to-use WS devices for regular use in clinical practice.
Firstly, the non-uniformity of WS-based measurement methods and outcome measures needs to be addressed. There is no consensus concerning the number of sensors, position, placement on the body, resultant gait feature set, etc. Such an approach hinders scaling up for general and broad applicability. Measurement devices need to generate reliable and reproducible output for clinical and research use.
Secondly, there is a need to develop intelligent and intelligent algorithms, especially concerning the umbrella of disorders diagnosis. Most reported works (algorithms) exploit certain traits associated with a particular disease, making them disease-specific and less efficient with other disorders. However, the system needs to cater to the broader population group for clinical applicability. Moreover, most of the reported literature deals with binary (sometimes 3–4) classification, i.e., targeted to identifying a selected disorder. Classification of conditions based on the severity, i.e., grading the disease, is still an area that needs broader investigation for acceptance in clinical usability. An objective, automated process of quantifying and classifying gait data can allow clinicians to invest their resources and time in prescribing better, more effective, and more targeted treatments in actual clinical practice. More penetration of AI, ML, and deep learning techniques can bridge the gap.
And thirdly, the exploitation of smartphones as a tool for movement analysis requires more focus. Given the broad reach of smartphone devices for extensive use, such mobile-based technologies can cater to a large population with limited access to quantified gait analysis. Moreover, accurate life data from all daily activities can open up newer avenues in this direction.
Conclusion
This article highlights significant recent works toward assessing neurodegenerative gait disorders using wearable sensing techniques. Some recent progress reported toward non-pharmacological therapeutic intervention toward mitigating gait and balance disorders originating from neuro-degeneration. Reported sensing methods for studying gait kinematics, kinetics, spatiotemporal, and postural balance through inertial, footswitches, pressure sensors, ultrasound sensors, proximity sensors, plantar pressure sensors, electromyography, etc., have extensively been discussed. The IS-based measurements correlate well with kinematics and other qualitative and quantitative measures corresponding to gait and activity monitoring. IMUs, owing to distinct advantages, offer the most promising alternative to laboratory-based gait analysis covering almost all spectrum of clinical gait analysis. The wide availability of IMU integrated devices is undoubtedly a positive aspect of translating such technologies for clinical benefit. However, specific challenges still need to be addressed toward achieving that goal, mainly deriving a common technology consensus and guideline conforming to its use. The wearable biofeedback systems and VR-based technology offer a promising solution toward rehabilitation and assistance of neuro-impaired gait symptoms, such as in the case of freezing of gait episodes postural imbalance. Similarly, various phytochemical compounds such as BBR FA have shown few positive outcomes in mitigating movement and balance-related impairments in cellular and rodent models. However, research in this domain is still pre-infancy and demands a widescale effort to reach a firm conclusion concerning their benefit in tackling neurodegenerative gait disorders in humans.
Author Contributions
RD performed the literature search article scrutiny and wrote the first draft of the manuscript. All authors contributed to the conception and design of the study, and reviewed, read, and approved the submitted version.
Funding
This work was jointly supported by the Twinning Program of the Department of Biotechnology, Government of India, and IMPRINT-2, DST-SERB, Government of India.
Conflict of Interest
The authors declare that the research was conducted in the absence of any commercial or financial relationships that could be construed as a potential conflict of interest.
Publisher’s Note
All claims expressed in this article are solely those of the authors and do not necessarily represent those of their affiliated organizations, or those of the publisher, the editors and the reviewers. Any product that may be evaluated in this article, or claim that may be made by its manufacturer, is not guaranteed or endorsed by the publisher.
Acknowledgments
We acknowledge the support of the Department of Biotechnology, and Department of Science and Technology, Government of India, for providing the fund to carry out the work through their DBT-NER Twinning project grant. We wholeheartedly thank Prof. Xianta Jiang of Memorial University of Newfoundland for very kindly agreeing to use his copyrighted image (Figure 4). We express their sincere heartfulness to Dr. Dinesh Bhatia (Associate Professor, Department of BME, NEHU Shillong) for initiating and providing his valuable input during the project proposal submission.
Abbreviations
10 MWT, 10 meter walk test; 2D, two dimensional; 3D, three dimensional; AA, ankle arthrodesis; AD, Alzheimer’s disease; ALS, amyotrophic lateral sclerosis; AO, ankle orthosis; BBS, Bergs Balance Scale; BF, biceps femoris; CF, complementary Filter; CMT, Charcot-Marie-Tooth; CNN, convolution neural network; CoG, center of gravity; CoM, center of mass; CoP, center of pressure; EMG, electromyography; ES, erector spinae; FF, flat foot; FFT, Fast Fourier transform; FMG, force myography; FSR, force sensitive resistors; GCT, gait cycle time; GM, gastrocnemius medialis; GRF, ground reaction force; HC, healthy controls; HD, Huntington’s disease; HMM, Hidden Markov Model; HO, heel off; HS, heel strike; IC, initial contact; IMU, Inertial Measurement Unit; IPM, inverse pendulum model; IS, inertial sensors; KF, Kalman Filter; LDA, linear discriminant analysis; Lstp, step length; MEMS, micro-electromechanical systems; MS, multiple sclerosis; ms, millisecond; MSt, mid-stance; NWS, non-wearable sensors; OMC, optical motion camera; PD, Parkinson’s disease; PSw, pre-swing; PVDF, polyvinylidene fluoride; RF, rectus femoris; ROM, range of motion; SCI, spinal cord injury; sEMG, surface-electromyography; SL, stride length; STP, spatiotemporal parameter; Stt, stance time; SV, stride velocity; SVM, support vector machine; Sw, swing; TA, tibialis anterior; TAR, total ankle replacement; TO, toe off; TS, toe strike; TSw, swing time; TUGT, time-up and go test; vGRF, vertical ground reaction force; VM, vastus medialis; WFSM, wireless foot sensor module; WHO, World Health Organization; WS, wearable sensors; ZUPT, zero update; μ V, micro volts.
References
Alaqtash, M., Yu, H., Brower, R., Abdelgawad, A., and Sarkodie-Gyan, T. (2011). Application of wearable sensors for human gait analysis using fuzzy computational algorithm. Eng. Appl. Artif. Intell. 24, 1018–1025.
Al-Mulla, M. R., Sepulveda, F., and Colley, M. J. S. (2011). A review of non-invasive techniques to detect and predict localised muscle fatigue. Sensors 11, 3545–3594. doi: 10.3390/s110403545
Altaf, M. U. B., Butko, T., and Juang, B.-H. F. (2015). Acoustic gaits: gait analysis with footstep sounds. IEEE Trans. Biomed. Eng. 62, 2001–2011. doi: 10.1109/TBME.2015.2410142
Amar, J. (1920). The Human Motor: or, The Scientific Foundations of Labour and Industry. London, UK: Routledge.
Amato, F., Bianchi, S., Comai, S., Crovari, P., Pasquarelli, M. G. G., Imtiaz, A., et al. (2018). “CLONE: a promising system for the remote monitoring of Alzheimer’s patients: an experimentation with a wearable device in a village for Alzheimer’s care,” in Proceedings of the 4th EAI International Conference on Smart Objects and Technologies for Social Good, New York, NY: Association for Computing Machinery 255–260.
Anusha, C., Sumathi, T., and Joseph, L. D. (2017). Protective role of apigenin on rotenone induced rat model of Parkinson’s disease: suppression of neuroinflammation and oxidative stress mediated apoptosis. Chemico Biolo. Interact. 269, 67–79. doi: 10.1016/j.cbi.2017.03.016
Arafsha, F., Hanna, C., Aboualmagd, A., Fraser, S., and El Saddik, A. (2018). Instrumented wireless smartinsole system for mobile gait analysis: a validation pilot study with tekscan strideway. J. Sens. Actuator Netw. 36, 1–16.
Askar, M. H., Hussein, A. M., Al-Basiony, S. F., Meseha, R. K., Metias, E. F., Salama, M. M., et al. (2019). Effects of exercise and ferulic acid on alpha synuclein and neuroprotective heat shock protein 70 in an experimental model of Parkinsonism disease. CNS Neurol. Disord. Drug Targets 18, 156–169. doi: 10.2174/1871527317666180816095707
Ayrulu-Erdem, B., and Barshan, B. (2011). Leg motion classification with artificial neural networks using wavelet-based features of gyroscope signals. Sensors 11, 1721–1743. doi: 10.3390/s110201721
Badaru, U., Alonge, V., Adeniyi, A., and Ogwumike, O. (2012). Relative Therapeutic Efficacy of the Treadmill and Step Bench in Gait Rehabilitation of Hemiparetic Stroke Patients. African J. Physiother. Rehabil. Sci. 4, 45–50.
Balakrishnan, R., Azam, S., Cho, D.-Y., Su-Kim, I., and Choi, D.-K. (2021). Natural Phytochemicals as Novel Therapeutic Strategies to Prevent and Treat Parkinson’s Disease: current Knowledge and Future Perspectives. Oxidative Med. Cell. Longev. 2021:1–32. doi: 10.1155/2021/6680935
Balbinot, G., Schuch, C. P., Zaro, M. A., and Vaz, M. (2014). Low-cost piezoelectric footswitch system for measuring temporal parameters during walking. Int. J. Eng. Technol. 3, 75–81. doi: 10.14419/ijet.v3i1.1733
Bamberg, S. J. M., Benbasat, A. Y., Scarborough, D. M., Krebs, D. E., and Paradiso, J. A. (2008). Gait analysis using a shoe-integrated wireless sensor system. IEEE Trans. Inform. Technol. Biomed. 12, 413–423. doi: 10.1109/TITB.2007.899493
Bark, C., Chaccour, K., Darazi, R., El Hassani, A. H., and Andres, E. (2017). “Design and development of a force-sensing shoe for gait analysis and monitoring,” in 2017 Fourth International Conference on Advances in Biomedical Engineering (ICABME): IEEE, (New Jersey: IEEE), 1–4.
Barratt, G. K., Bellenger, C., Robertson, E. Y., Lane, J., and Crowther, R. G. (2021). Validation of Plantar Pressure and Reaction Force Measured by Moticon Pressure Sensor Insoles on a Concept2 Rowing Ergometer. Sensors 21:2418. doi: 10.3390/s21072418
Barzegar, A., and Moosavi-Movahedi. (2011). Intracellular ROS protection efficiency and free radical-scavenging activity of curcumin. PLoS One 6:e26012. doi: 10.1371/journal.pone.0026012
Bastide, M. F., Meissner, W. G., Picconi, B., Fasano, S., Fernagut, P.-O., Feyder, M., et al. (2015). Pathophysiology of L-dopa-induced motor and non-motor complications in Parkinson’s disease. Prog. Neurobiol. 132, 96–168.
Beauchet, O., Allali, G., Sekhon, H., Verghese, J., Guilain, S., Steinmetz, J.-P., et al. (2017). Guidelines for assessment of gait and reference values for spatiotemporal gait parameters in older adults: the biomathics and canadian gait consortiums initiative. Front. Hum. Neurosci. 11:353. doi: 10.3389/fnhum.2017.00353
Bekkers, E. M., Mirelman, A., Alcock, L., Rochester, L., Nieuwhof, F., Bloem, B. R., et al. (2020). Do patients with Parkinson’s disease with freezing of gait respond differently than those without to treadmill training augmented by virtual reality? Neurorehabil. Neural Repair 34, 440–449. doi: 10.1177/1545968320912756
Belyea, A. T., Englehart, K. B., and Scheme, E. J. (2018). A proportional control scheme for high density force myography. J. Neural Eng. 15:046029. doi: 10.1088/1741-2552/aac89b
Benocci, M., Rocchi, L., Farella, E., Chiari, L., and Benini, L. (2009). “A wireless system for gait and posture analysis based on pressure insoles and Inertial Measurement Units,” in 2009 3rd International Conference on Pervasive Computing Technologies for Healthcare: IEEE, (New Jersey: IEEE), 1–6.
Bhardwaj, S., Khan, A. A., Muzammil, M. J. D., and Technology, R. A. (2021). Lower limb rehabilitation using multimodal measurement of sit-to-stand and stand-to-sit task. Disabil. Rehabil. Assist. Technol. 6, 438–445. doi: 10.1080/17483107.2019.1629701
Bluett, B., Bayram, E., and Litvan, I. (2019). The virtual reality of Parkinson’s disease freezing of gait: a systematic review. Park. Relat. Disord. 61, 26–33. doi: 10.1016/j.parkreldis.2018.11.013
Bogey, R., Perry, J., and Gitter, A. (2005). An EMG-to-force processing approach for determining ankle muscle forces during normal human gait. Neural Syst. Rehabil. Eng. IEEE Trans. 13, 302–310. doi: 10.1109/TNSRE.2005.851768
Boudarham, J., Hameau, S., Pradon, D., Bensmail, D., Roche, N., and Zory, R. (2013). Changes in electromyographic activity after botulinum toxin injection of the rectus femoris in patients with hemiparesis walking with a stiff-knee gait. J. Electromyogr. Kinesiol. 23, 1036–1043. doi: 10.1016/j.jelekin.2013.07.002
Boudarham, J., Roche, N., Pradon, D., Delouf, E., Bensmail, D., and Zory, R. (2014). Effects of quadriceps muscle fatigue on stiff-knee gait in patients with hemiparesis. PLoS One 9:e94138. doi: 10.1371/journal.pone.0094138
Bowman, T., Gervasoni, E., Arienti, C., Lazzarini, S. G., Negrini, S., Crea, S., et al. (2021). Wearable devices for biofeedback rehabilitation: a systematic review and meta-analysis to design application rules and estimate the effectiveness on balance and gait outcomes in neurological diseases. Sensors 21:3444. doi: 10.3390/s21103444
Calabrò, R. S., Russo, M., Naro, A., De Luca, R., Leo, A., and Tomasello, P. (2017). Robotic gait training in multiple sclerosis rehabilitation: can virtual reality make the difference? Findings from a randomized controlled trial. J. Neurol. Sci. 377, 25–30. doi: 10.1016/j.jns.2017.03.047
Caldas, R., Mundt, M., Potthast, W., De Lima Neto, F. B., and Markert, B. (2017). A systematic review of gait analysis methods based on inertial sensors and adaptive algorithms. Gait posture 57, 204–210. doi: 10.1016/j.gaitpost.2017.06.019
Calfio, C., Gonzalez, A., Singh, S. K., Rojo, L. E., and Maccioni, R. (2020). The emerging role of nutraceuticals and phytochemicals in the prevention and treatment of Alzheimer’s disease. J. Alzheimer’s Dis. 77, 33–51. doi: 10.3233/JAD-200443
Cao, J., Liu, Y., Jia, L., Jiang, L.-P., Geng, C.-Y., Yao, X.-F., et al. (2008). Curcumin attenuates acrylamide-induced cytotoxicity and genotoxicity in HepG2 cells by ROS scavenging. J. Agric. Food Chem. 56, 12059–12063. doi: 10.1021/jf8026827
Cao, X.-Y., Zhang, J.-R., Shen, Y., Mao, C.-J., Shen, Y.-B., Cao, Y.-L., et al. (2021). Fatigue correlates with sleep disturbances in Parkinson disease. Chinese Med. J. 134, 668–674. doi: 10.1097/CM9.0000000000001303
Carvalho, C., Correia, S. C., Cardoso, S., Placido, A. I., Candeias, E., Duarte, A. I., et al. (2015). The role of mitochondrial disturbances in Alzheimer, Parkinson and Huntington diseases. Expert Rev. Neurother. 15, 867–884. doi: 10.1586/14737175.2015.1058160
Castellini, C., Artemiadis, P., Wininger, M., Ajoudani, A., Alimusaj, M., Bicchi, A., et al. (2014). Proceedings of the first workshop on peripheral machine interfaces: going beyond traditional surface electromyography. Front. Neurorobot. 8:22. doi: 10.3389/fnbot.2014.00022
Catalfamo, P., Ghoussayni, S., and Ewins, D. (2010). Gait event detection on level ground and incline walking using a rate gyroscope. Sensors 10, 5683–5702. doi: 10.3390/s100605683
Cha, Y., Kim, H., and Kim, D. (2018). Flexible piezoelectric sensor-based gait recognition. Sensors 18:468. doi: 10.3390/s18020468
Chagraoui, A., Boulain, M., Juvin, L., Anouar, Y., and Barrière, G. (2020). L-DOPA in parkinson’s disease: looking at the “false” neurotransmitters and their meaning. Int. J. Mol. Sci. 21:294. doi: 10.3390/ijms21010294
Chalmers, E., Le, J., Sukhdeep, D., Watt, J., Andersen, J., and Lou, E. (2014). Inertial sensing algorithms for long-term foot angle monitoring for assessment of idiopathic toe-walking. Gait posture 39, 485–489. doi: 10.1016/j.gaitpost.2013.08.021
Chang, H.-C., Hsu, Y.-L., Yang, S.-C., Lin, J.-C., and Wu, Z.-H. (2016). A wearable inertial measurement system with complementary filter for gait analysis of patients with stroke or Parkinson’s disease. IEEE Access 4, 8442–8453. doi: 10.1109/access.2016.2633304
Chen, B., Wen, X., Jiang, H., Wang, J., Song, N., and Xie, J. (2019). Interactions between iron and α-synuclein pathology in Parkinson’s disease. Lancet Neurol. 141, 253–260. doi: 10.1016/j.freeradbiomed.2019.06.024
Chen, J., Zhang, M., Dai, Y., Xie, Y., Tian, W., and Xu, L. (2020). A force–voltage responsivity stabilization method for piezoelectric-based insole gait analysis for high detection accuracy in health monitoring. Int. J. Distrib. Sens. Netw. 16:155014772090544. doi: 10.1177/1550147720905441
Chen, J.-F., Steyn, S., Staal, R., Petzer, J. P., Xu, K., Van Der Schyf, C. J., et al. (2002). 8-(3-Chlorostyryl) caffeine may attenuate MPTP neurotoxicity through dual actions of monoamine oxidase inhibition and A2A receptor antagonism. J. Biol. Chem. 277, 36040–36044. doi: 10.1074/jbc.M206830200
Chiang, T.-H., Ou, K.-Y., Qiu, J.-W., and Tseng, Y.-C. (2019). Pedestrian tracking by acoustic Doppler effects. IEEE Sens. J. 19, 3893–3901. doi: 10.3390/s19112458
Chiang, T.-H., Su, Y.-J., Shiu, H.-R., and Tseng, Y.-C. (2020). “3D Gait Tracking by Acoustic Doppler Effects,” in 2020 42nd Annual International Conference of the IEEE Engineering in Medicine & Biology Society (EMBC): IEEE, (New Jersey: IEEE), 3146–3149. doi: 10.1109/EMBC44109.2020.9175663
Choi, G.-Y., Kim, H.-B., Hwang, E.-S., Lee, S., Kim, M.-J., Choi, J.-Y., et al. (2017). Curcumin alters neural plasticity and viability of intact hippocampal circuits and attenuates behavioral despair and COX-2 expression in chronically stressed rats. Mediators Inflamm. 2017:6280925 doi: 10.1155/2017/6280925
Chorin, F., Cornu, C., Beaune, B., Frère, J., and Rahmani, A. (2016). Sit to stand in elderly fallers vs non-fallers: new insights from force platform and electromyography data. Aging clin. Exp. Res. 28, 871–879. doi: 10.1007/s40520-015-0486-1
Cicirelli, G., Impedovo, D., Dentamaro, V., Marani, R., Pirlo, G., and D’orazio, T. (2021). Human gait analysis in neurodegenerative diseases: a review. IEEE J. Biomed. Health Inform. 26, 229–242. doi: 10.1109/JBHI.2021.3092875
Cooper, G., Sheret, I., Mcmillian, L., Siliverdis, K., Sha, N., Hodgins, D., et al. (2009). Inertial sensor-based knee flexion/extension angle estimation. J. Biomech. 42, 2678–2685. doi: 10.1016/j.jbiomech.2009.08.004
Corpas, R., Griñán-Ferré, C., Rodríguez-Farré, E., Pallàs, M., and Sanfeliu, C. J. (2019). Resveratrol induces brain resilience against Alzheimer neurodegeneration through proteostasis enhancement. Mol. Neurobiol. 56, 1502–1516. doi: 10.1007/s12035-018-1157-y
Correia, S. C., Santos, R. X., Cardoso, S., Carvalho, C., Candeias, E., Duarte, A. I., et al. (2012). Alzheimer disease as a vascular disorder: where do mitochondria fit? Exp. Gerontol. 47, 878–886. doi: 10.1016/j.exger.2012.07.006
Crea, S., De Rossi, S. M. M., Donati, M., Reberšek, P., Novak, D., Vitiello, N., et al. (2012). “Development of gait segmentation methods for wearable foot pressure sensors,” in 2012 Annual International Conference of the IEEE Engineering in Medicine and Biology Society: IEEE, (New Jersey: IEEE), 5018–5021. doi: 10.1109/EMBC.2012.6347120
Crea, S., Donati, M., De Rossi, S. M. M., Oddo, C. M., and Vitiello, N. (2014). A wireless flexible sensorized insole for gait analysis. Sensors 14, 1073–1093. doi: 10.3390/s140101073
Cuesta-Vargas, A. I., Galán-Mercant, A., and Williams, J. M. (2010). The use of inertial sensors system for human motion analysis. Phys. Ther. Rev. 15, 462–473. doi: 10.1179/1743288X11Y.0000000006
Cutti, A. G., Ferrari, A., Garofalo, P., Raggi, M., Cappello, A., and Ferrari, A. (2010). ‘Outwalk’: a protocol for clinical gait analysis based on inertial and magnetic sensors. Med. Biol. Eng. comput. 48:17. doi: 10.1007/s11517-009-0545-x
Cutti, A. G., Giovanardi, A., Rocchi, L., Davalli, A., and Sacchetti, R. (2008). Ambulatory measurement of shoulder and elbow kinematics through inertial and magnetic sensors. Med. Biol. Eng. comput. 46, 169–178. doi: 10.1007/s11517-007-0296-5
Dai, Y., Xie, Y., Chen, J., Kang, S., and Xu, L. (2020). A lamination-based piezoelectric insole gait analysis system for massive production for Internet-of-health things. Int. J. Distrib. Sens. Netw. 16:155014772090543. doi: 10.1177/1550147720905431
Das, R., Hooda, N., and Kumar, N. J. I. S. L. (2019). A novel approach for real-time gait events detection using developed wireless foot sensor module. IEEE Sens. Lett. 3, 1–4. doi: 10.1109/lsens.2019.2914719
Das, R., and Kumar, N. (2021). A Novel Method for Stride Length Estimation Using Wireless Foot Sensor Module. IETE J. Res. 2021, 1–8. doi: 10.1080/03772063.2021.1905565
De Rossi, S., Lenzi, T., Vitiello, N., Donati, M., Persichetti, A., Giovacchini, F., et al. (2011). “Development of an in-shoe pressure-sensitive device for gait analysis,” in 2011 Annual International Conference of the IEEE Engineering in Medicine and Biology Society: IEEE, (New Jersey IEEE), 5637–5640. doi: 10.1109/IEMBS.2011.6091364
De Rossi, S. M., Crea, S., Donati, M., Reberšek, P., Novak, D., Vitiello, N., et al. (2012). “Gait segmentation using bipedal foot pressure patterns,” in 2012 4th IEEE RAS & EMBS International Conference on Biomedical Robotics and Biomechatronics (BioRob): IEEE, (New Jersey IEEE), 361–366.
De Souza Fortaleza, A. C., Mancini, M., Carlson-Kuhta, P., King, L. A., Nutt, J. G., Chagas, E. F., et al. (2017). Dual task interference on postural sway, postural transitions and gait in people with Parkinson’s disease and freezing of gait. Gait posture 56, 76–81. doi: 10.1016/j.gaitpost.2017.05.006
Dehzangi, O., Taherisadr, M., and Changalvala, R. (2017). IMU-based gait recognition using convolutional neural networks and multi-sensor fusion. Sensors 17:2735.
Dejnabadi, H., Jolles, B. M., and Aminian, K. (2005). A new approach to accurate measurement of uniaxial joint angles based on a combination of accelerometers and gyroscopes. IEEE Trans. Biomed. Eng. 52, 1478–1484. doi: 10.1109/TBME.2005.851475
Di Nardo, F., Morbidoni, C., Mascia, G., Verdini, F., and Fioretti, S. (2020). Intra-subject approach for gait-event prediction by neural network interpretation of EMG signals. Biomed. Eng. Online 19, 1–20. doi: 10.1186/s12938-020-00803-1
Djurić-Jovičić, M. D., Jovičić, N. S., and Popović, D. B. (2011). Kinematics of gait: new method for angle estimation based on accelerometers. Sensors 11, 10571–10585. doi: 10.3390/s111110571
Donati, M., Vitiello, N., De Rossi, S. M. M., Lenzi, T., Crea, S., Persichetti, A., et al. (2013). A flexible sensor technology for the distributed measurement of interaction pressure. Sensors 13, 1021–1045. doi: 10.3390/s130101021
Doniger, G. M., Beeri, M. S., Bahar-Fuchs, A., Gottlieb, A., Tkachov, A., Kenan, H., et al. (2018). Virtual reality-based cognitive-motor training for middle-aged adults at high Alzheimer’s disease risk: a randomized controlled trial. Alzheimer’s Dementia Trans. Res. Clin. Int. 4, 118–129. doi: 10.1016/j.trci.2018.02.005
Dorschky, E., Nitschke, M., Seifer, A.-K., Van Den Bogert, A. J., and Eskofier, B. M. (2019). Estimation of gait kinematics and kinetics from inertial sensor data using optimal control of musculoskeletal models. J. Biomech. 95:109278. doi: 10.1016/j.jbiomech.2019.07.022
Dugan, E. L., Shilt, J. S., Masterson, C. M., and Ernest, K. M. (2021). The use of inertial measurement units to assess gait and postural control following concussion. Gait Posture 83, 262–267. doi: 10.1016/j.gaitpost.2020.10.004
Edgar, S. R., Swyka, T., Fulk, G., and Sazonov, E. S. (2010). “Wearable shoe-based device for rehabilitation of stroke patients,” in 2010 Annual International Conference of the IEEE Engineering in Medicine and Biology: IEEE, (New Jersey: IEEE), 3772–3775. doi: 10.1109/IEMBS.2010.5627577
Eftekharsadat, B., Babaei-Ghazani, A., Mohammadzadeh, M., Talebi, M., Eslamian, F., and Azari, E. J. N. R. (2015). Effect of virtual reality-based balance training in multiple sclerosis. Neurol. Res. 37, 539–544. doi: 10.1179/1743132815Y.0000000013
El-Gohary, M., Peterson, D., Gera, G., Horak, F. B., and Huisinga, J. M. (2017). Validity of the instrumented push and release test to quantify postural responses in persons with multiple sclerosis. Arch. phys. Med. Rehabil. 98, 1325–1331. doi: 10.1016/j.apmr.2017.01.030
Emamzadeh, F. N., and Surguchov, A. J. (2018). Parkinson’s disease: biomarkers, treatment, and risk factors. Front. Neurosci. 12:612. doi: 10.3389/fnins.2018.00612
Eschbach, J., Von Einem, B., Müller, K., Bayer, H., Scheffold, A., Morrison, B. E., et al. (2015). Mutual exacerbation of peroxisome proliferator-activated receptor γ coactivator 1α deregulation and α-synuclein oligomerization. Ann. Neurol. 77, 15–32. doi: 10.1002/ana.24294
Fan, C., Song, Q., Wang, P., Li, Y., Yang, M., Liu, B., et al. (2018). Curcumin protects against chronic stress-induced dysregulation of neuroplasticity and depression-like behaviors via suppressing IL-1β pathway in rats. Neuroscience 392, 92–106. doi: 10.1016/j.neuroscience.2018.09.028
Favre, J., Aissaoui, R., Jolles, B. M., De Guise, J. A., and Aminian, K. (2009). Functional calibration procedure for 3D knee joint angle description using inertial sensors. J. Biomech. 42, 2330–2335. doi: 10.1016/j.jbiomech.2009.06.025
Feigin, V. L., Abajobir, A. A., Abate, K. H., Abd-Allah, F., Abdulle, A. M., Abera, S. F., et al. (2017). Global, regional, and national burden of neurological disorders during 1990–2015: a systematic analysis for the Global Burden of Disease Study 2015. Lancet Neurol. 16, 877–897.
Feigin, V. L., Nichols, E., Alam, T., Bannick, M. S., Beghi, E., Blake, N., et al. (2019). Global, regional, and national burden of neurological disorders, 1990–2016: a systematic analysis for the Global Burden of Disease Study 2016. Lancet Neurol. 18, 459–480. doi: 10.1016/S1474-4422(18)30499-X
Feng, H., Li, C., Liu, J., Wang, L., Ma, J., and Li, G. (2019). Virtual reality rehabilitation versus conventional physical therapy for improving balance and gait in Parkinson’s disease patients: a randomized controlled trial. Med. Sci. Monit. 25, 4186–4192. doi: 10.12659/MSM.916455
Ferlini, A., Ma, D., Harle, R., and Mascolo, C. (2021). “EarGate: gait-based user identification with in-ear microphones,” in Proceedings of the 27th Annual International Conference on Mobile Computing and Networking, (Piscataway: IEEE), 337–349.
Ferrari, A., Cutti, A. G., Garofalo, P., Raggi, M., Heijboer, M., Cappello, A., et al. (2010). First in vivo assessment of “Outwalk”: a novel protocol for clinical gait analysis based on inertial and magnetic sensors. Med. Biol. Eng. Comput. 48:1. doi: 10.1007/s11517-009-0544-y
Ferrari, A., Ginis, P., Hardegger, M., Casamassima, F., Rocchi, L., and Chiari, L. (2015). A mobile Kalman-filter based solution for the real-time estimation of spatio-temporal gait parameters. IEEE Trans. Neural Syst. Rehabil. Eng. 24, 764–773. doi: 10.1109/TNSRE.2015.2457511
Findlow, A., Goulermas, J., Nester, C., Howard, D., and Kenney, L. (2008). Predicting lower limb joint kinematics using wearable motion sensors. Gait posture 28, 120–126. doi: 10.1016/j.gaitpost.2007.11.001
Follett, J., Bugarcic, A., Yang, Z., Ariotti, N., Norwood, S. J., Collins, B. M., et al. (2016). Parkinson disease-linked Vps35 R524W mutation impairs the endosomal association of retromer and induces α-synuclein aggregation. J. Biol. Chem. 291, 18283–18298. doi: 10.1074/jbc.M115.703157
Formento, P. C., Acevedo, R., Ghoussayni, S., and Ewins, D. (2014). Gait event detection during stair walking using a rate gyroscope. Sensors 14, 5470–5485. doi: 10.3390/s140305470
Fourchet, F., Kelly, L., Horobeanu, C., Loepelt, H., Taiar, R., and Millet, G. (2015). High-intensity running and plantar-flexor fatigability and plantar-pressure distribution in adolescent runners. J. Athletic Train. 50, 117–125. doi: 10.4085/1062-6050-49.3.90
Fraser, S. A., Li, K. Z., Demont, R. G., and Penhune, V. B. (2007). Effects of balance status and age on muscle activation while walking under divided attention. J. Gerontol. Series B Psychol. Sci. Soc. Sci. 62, 171–178. doi: 10.1093/geronb/62.3.p171
Fujita, K., Kobayashi, Y., and Hitosugi, M. (2021). Temporal Changes in Electromyographic Activity and Gait Ability During Extended Walking in Individuals Post-Stroke: a Pilot Stud. Healthcare 9:444. doi: 10.3390/healthcare9040444
Fujita, K., Kobayashi, Y., Miaki, H., Hori, H., Tsushima, Y., Sakai, R., et al. (2020). Pedaling improves gait ability of hemiparetic patients with stiff-knee gait: fall prevention during gait. J. Stroke Cerebrovasc. Dis. 29:105035. doi: 10.1016/j.jstrokecerebrovasdis.2020.105035
Fujiwara, E., and Suzuki, C. K. (2018). Optical fiber force myography sensor for identification of hand postures. J. Sens. 2018:8940373.
Gago, M. F., Yelshyna, D., Bicho, E., Silva, H. D., Rocha, L., Rodrigues, M. L., et al. (2016). Compensatory postural adjustments in an Oculus virtual reality environment and the risk of falling in Alzheimer’s disease. Dement. Geriatr. Cogn. Disord. Extra 6, 252–267. doi: 10.1159/000447124
Gao, S., Chen, J.-L., Dai, Y.-N., Wang, R., Kang, S.-B., and Xu, L.-J. (2020). Piezoelectric-Based Insole Force Sensing for Gait Analysis in the Internet of Health Things. IEEE Consumer Electron. Magaz. 10, 39–44. doi: 10.1109/mce.2020.2986828
Garcia-Gancedo, L., Kelly, M. L., Lavrov, A., Parr, J., Hart, R., Marsden, R., et al. (2019). Objectively monitoring amyotrophic lateral sclerosis patient symptoms during clinical trials with sensors: observational study. JMIR mHealth uHealth 7:e13433. doi: 10.2196/13433
Gautam, S., Karmakar, S., Batra, R., Sharma, P., Pradhan, P., Singh, J., et al. (2017). Polyphenols in combination with β-cyclodextrin can inhibit and disaggregate α-synuclein amyloids under cell mimicking conditions: a promising therapeutic alternative. Biochim. Biophys. Acta Proteins Proteom 1865, 589–603. doi: 10.1016/j.bbapap.2017.02.014
Georgiades, M. J., Gilat, M., Martens, K., Walton, C. C., Bissett, P. G., Shine, J. M., et al. (2016). Investigating motor initiation and inhibition deficits in patients with Parkinson’s disease and freezing of gait using a virtual reality paradigm. Neuroscience 337, 153–162. doi: 10.1016/j.neuroscience.2016.09.019
Georgiou, T., Islam, R., Holland, S., Van Der Linden, J., Price, B., Mulholland, P., et al. (2020). Rhythmic haptic cueing using wearable devices as physiotherapy for huntington disease: case study. JMIR Rehabil. Assist. Technol. 7:e18589. doi: 10.2196/18589
Gholami, M., Napier, C., and Menon, C. (2020). Estimating Lower Extremity Running Gait Kinematics with a Single Accelerometer: a Deep Learning Approach. Sensors 20:2939. doi: 10.3390/s20102939
Gilat, M., Shine, J. M., Walton, C. C., O’callaghan, C., Hall, J. M., and Lewis, S. (2015). Brain activation underlying turning in Parkinson’s disease patients with and without freezing of gait: a virtual reality fMRI study. Npj Park. Dis. 1:15020. doi: 10.1038/npjparkd.2015.20
Godiyal, A. K., Verma, H. K., Khanna, N., and Joshi, D. (2018). A force myography-based system for gait event detection in overground and ramp walking. IEEE Trans. Instrum. Meas. 67, 2314–2323. doi: 10.1109/tim.2018.2816799
Goh, L., Allen, N. E., Ahmadpour, N., Martens, K., Song, J., Clemson, L., et al. (2021). A Video Self-Modeling Intervention Using Virtual Reality Plus Physical Practice for Freezing of Gait in Parkinson Disease: feasibility and Acceptability Study. JMIR Form. Res. 5:e28315. doi: 10.2196/28315
Gouwanda, D., Gopalai, A. A., and Khoo, B. H. (2016). A low cost alternative to monitor human gait temporal parameters–wearable wireless gyroscope. IEEE Sens. J. 16, 9029–9035. doi: 10.1109/jsen.2016.2616163
Ha, N., Withanachchi, G. P., and Yihun, Y. (2018). “Force myography signal-based hand gesture classification for the implementation of real-time control system to a prosthetic hand,” in Proceedings of the 2018 Design of Medical Devices Conference DMD2018, Minneapolis, MN, USA: The American Society of Mechanical Engineers 1–4.
Hamatani, M., Mori, T. O. M., Noguchi, H., Takehara, K., Amemiya, A., Ohashi, Y., et al. (2016). Factors associated with callus in patients with diabetes, focused on plantar shear stress during gait. J. Diabetes Sci. Technol. 10, 1353–1359. doi: 10.1177/1932296816648164
Hannink, J., Kautz, T., Pasluosta, C. F., Gaßmann, K.-G., Klucken, J., and Eskofier, B. M. (2017). Sensor-based gait parameter extraction with deep convolutional neural networks. IEEE J. Biomed. Health Inf. 21, 85–93.
Hao, M., Chen, K., and Fu, C. (2019). Smoother-based 3D Foot Trajectory Estimation Using Inertial Sensors. IEEE Trans. Biomed. Eng. 66, 3534–3542. doi: 10.1109/TBME.2019.2907322
Hausdorff, J. M., Ladin, Z., and Wei, J. Y. (1995). Footswitch system for measurement of the temporal parameters of gait. J. Biomech. 28, 347–351. doi: 10.1016/0021-9290(94)00074-e
Hazra, S., Pratap, A. A., Tripathy, D., and Nandy, A. (2021). Novel data fusion strategy for human gait analysis using multiple kinect sensors. Biomed. Signal. Process. Control 67:102512. doi: 10.1016/j.bspc.2021.102512
Horak, F. B., Mancini, M., Carlson-Kuhta, P., Nutt, J. G., and Salarian, A. (2016). Balance and gait represent independent domains of mobility in Parkinson disease. Phys. Ther. 96, 1364–1371. doi: 10.2522/ptj.20150580
Horenstein, R. E., Lewis, C. L., Yan, S., Halverstadt, A., and Shefelbine, S. J. (2019). Validation of magneto-inertial measuring units for measuring hip joint angles. J. Biomech. 91, 170–174. doi: 10.1016/j.jbiomech.2019.05.029
Howell, A. M. (2012). Insole-Based Gait Analysis. University of Utah: Department of Mechanical Engineering
Howell, A. M., Kobayashi, T., Hayes, H. A., Foreman, K. B., and Bamberg, S. J. (2013). Kinetic gait analysis using a low-cost insole. Biomed. Eng. IEEE Trans. 60, 3284–3290. doi: 10.1109/TBME.2013.2250972
Hsu, Y.-L., Chung, P.-C., Wang, W.-H., Pai, M.-C., Wang, C.-Y., Lin, C.-W., et al. (2014). Gait and balance analysis for patients with Alzheimer’s disease using an inertial-sensor-based wearable instrument. IEEE J. Biomed. Health Inform. 18, 1822–1830. doi: 10.1109/JBHI.2014.2325413
Huang, Y.-Z., Chang, F.-Y., Liu, W.-C., Chuang, Y.-F., Chuang, L.-L., and Chang, Y.-J. (2017). Fatigue and muscle strength involving walking speed in Parkinson’s disease: insights for developing rehabilitation strategy for PD. Neural plasticity 2017:1941980. doi: 10.1155/2017/1941980
Huitema, R. B., Hof, A. L., and Postema, K. (2002). Ultrasonic motion analysis system—measurement of temporal and spatial gait parameters. J. Biomech. 35, 837–842. doi: 10.1016/s0021-9290(02)00032-5
Hundza, S. R., Hook, W. R., Harris, C. R., Mahajan, S. V., Leslie, P. A., Spani, C. A., et al. (2013). Accurate and reliable gait cycle detection in Parkinson’s disease. IEEE Trans. Neural Syst. Rehabil. Eng. 22, 127–137. doi: 10.1109/TNSRE.2013.2282080
Hwang, S., and Gim, J. (2015). “Listen to your footsteps: Wearable device for measuring walking quality,” in Proceedings of the 33rd Annual ACM Conference Extended Abstracts on Human Factors in Computing Systems, (New Jersey: IEEE), 2055–2060.
Jagos, H., Pils, K., Haller, M., Wassermann, C., Chhatwal, C., Rafolt, D., et al. (2017). Mobile gait analysis via eSHOEs instrumented shoe insoles: a pilot study for validation against the gold standard GAITRite®. J. Med. Eng. Technol. 41, 375–386. doi: 10.1080/03091902.2017.1320434
Janßen, I. M., Sturtz, S., Skipka, G., Zentner, A., Garrido, M. V., and Busse, R. (2010). Ginkgo biloba in Alzheimer’s disease: a systematic review. Wien. Med. Wochenschr. 160, 539–546.
Jasiewicz, J. M., Allum, J. H., Middleton, J. W., Barriskill, A., Condie, P., Purcell, B., et al. (2006). Gait event detection using linear accelerometers or angular velocity transducers in able-bodied and spinal-cord injured individuals. Gait Posture 24, 502–509. doi: 10.1016/j.gaitpost.2005.12.017
Jeong, H., Johnson, A. W., Feland, J. B., Petersen, S. R., and Staten, J. M. (2021). Added body mass alters plantar shear stresses, postural control, and gait kinetics: implications for obesity. PLoS One 16:e0246605. doi: 10.1371/journal.pone.0246605
Jesus, J., Ramos, H., Chiementin, X., Taiar, R., Sá-Caputo, D., Bernardo-Filho, M., et al. (2019). A Microphone-Based Gait Assessment Method (MGAM) in a patient with Parkinson disease. A case report. Series Biomech. 33, 25-33
Jiang, W., Wei, W., Gaertig, M. A., Li, S., and Li, X.-J. (2015). Therapeutic effect of berberine on Huntington’s disease transgenic mouse model. PLoS One 10:e0134142. doi: 10.1371/journal.pone.0134142
Jiang, X. (2018). Gait Analysis with an Ankle Band: Novel Force Myography-Based System to Monitor and Analyse Gait. Available online at: https://ispgr.org/gait-analysis-with-an-ankle-band-novel-force-myography-based-system-to-monitor-and-analyse-gait/ (accessed September 23, 2021).
Jiang, X., Chu, K. H., Khoshnam, M., and Menon, C. (2018). A wearable gait phase detection system based on force myography techniques. Sensors 18:1279. doi: 10.3390/s18041279
Jones, A. P., Kermode, A. G., Lucas, R. M., Carroll, W. M., Nolan, D., and Hart, P. (2017). Circulating immune cells in multiple sclerosis. Clin. Exp. Immunol. 187, 193–203. doi: 10.1111/cei.12878
Joshi, D., Nakamura, B. H., and Hahn, M. E. (2016). A novel approach for toe off estimation during locomotion and transitions on ramps and level ground. IEEE J. Biomed. Health Inform. 20, 153–157. doi: 10.1109/JBHI.2014.2377749
Júlio, F., Ribeiro, M. J., Morgadinho, A., Sousa, M., Van Asselen, M., Simões, M. R., et al. (2020). Cognition, function and awareness of disease impact in early Parkinson’s and Huntington’s disease. Disabil. Rehabil. 44, 921-939. doi: 10.1080/09638288.2020.1783001
Kalron, A., Fonkatz, I., Frid, L., Baransi, H., and Achiron, A. (2016). The effect of balance training on postural control in people with multiple sclerosis using the CAREN virtual reality system: a pilot randomized controlled trial. J. Neuroeng. Rehabil. 13:13. doi: 10.1186/s12984-016-0124-y
Kashihara, H., Shimizu, H., Houchi, H., Yoshimi, M., Yoshinaga, T., and Irie, H. (2013). “A real-time gait improvement tool using a smartphone,” in Proceedings of the 4th Augmented Human International Conference, (New Jersey: IEEE), 243–243.
Kegelmeyer, D. A., Kostyk, S. K., Fritz, N. E., Fiumedora, M. M., Chaudhari, A., Palettas, M., et al. (2017). Quantitative biomechanical assessment of trunk control in Huntington’s disease reveals more impairment in static than dynamic tasks. J. Neurol. Sci. 376, 29–34. doi: 10.1016/j.jns.2017.02.054
Keloth, S. M., Arjunan, S. P., Raghav, S., and Kumar, D. K. (2021). Muscle activation strategies of people with early-stage Parkinson’s during walking. J. NeuroEng. Rehabil. 18:133. doi: 10.1186/s12984-021-00932-1
Killane, I., Fearon, C., Newman, L., Mcdonnell, C., Waechter, S. M., Sons, K., et al. (2015). Dual motor-cognitive virtual reality training impacts dual-task performance in freezing of gait. IEEE J. Biomed. Health Inform 19, 1855–1861. doi: 10.1109/JBHI.2015.2479625
Kim, M., Cho, K.-H., Shin, M.-S., Lee, J.-M., Cho, H.-S., Kim, C.-J., et al. (2014). Berberine prevents nigrostriatal dopaminergic neuronal loss and suppresses hippocampal apoptosis in mice with Parkinson’s disease. Int. J. Mol. Med. 33, 870–878. doi: 10.3892/ijmm.2014.1656
Kloos, A. D., Fritz, N. E., Kostyk, S. K., Young, G. S., and Kegelmeyer, D. (2013). Video game play (Dance Dance Revolution) as a potential exercise therapy in Huntington’s disease: a controlled clinical trial. Clin. Rehabil. 27, 972–982. doi: 10.1177/0269215513487235
Kodama, J., and Watanabe, T. (2016). Examination of inertial sensor-based estimation methods of lower limb joint moments and ground reaction force: results for squat and sit-to-stand movements in the sagittal plane. Sensors 16:1209. doi: 10.3390/s16081209
Kwakkel, S., Godha, S., and Lachapelle, G. (2007). “Foot and ankle kinematics during gait using foot mounted inertial system,” in Proceedings of the 2007 National Technical Meeting of The Institute of Navigation, San Diego, CA, (Virginia: The Institute of Navigation).
Lazarou, I., Karakostas, A., Stavropoulos, T. G., Tsompanidis, T., Meditskos, G., Kompatsiaris, I., et al. (2016). A novel and intelligent home monitoring system for care support of elders with cognitive impairment. J. Alzheimer’s Dis. 54, 1561–1591. doi: 10.3233/JAD-160348
Ledoux, E. (2018). Inertial Sensing for Gait Event Detection and Transfemoral Prosthesis Control Strategy. IEEE Trans. Biomed. Eng. 65, 2704–2712. doi: 10.1109/TBME.2018.2813999
Lee, J. K., and Park, E. J. (2011). Quasi real-time gait event detection using shank-attached gyroscopes. Med. Biol. Eng. Comput. 49, 707–712. doi: 10.1007/s11517-011-0736-0
Lemoyne, R., Heerinckx, F., Aranca, T., De Jager, R., Zesiewicz, T., and Saal, H. J. (2016). “Wearable body and wireless inertial sensors for machine learning classification of gait for people with Friedreich’s ataxia,” in 2016 IEEE 13th International Conference on Wearable and Implantable Body Sensor Networks (BSN): IEEE, (New Jersey: IEEE), 147–151.
Lemoyne, R., and Mastroianni, T. (2015). Use of smartphones and portable media devices for quantifying human movement characteristics of gait, tendon reflex response, and Parkinson’s disease hand tremor. Mobile Health Technol. 1256, 335–358. doi: 10.1007/978-1-4939-2172-0-23
Lemoyne, R., and Mastroianni, T. (2018). Implementation of a smartphone as a wireless accelerometer platform for quantifying hemiplegic gait disparity in a functionally autonomous context. J. Mech. Med. Biol. 18:1850005. doi: 10.1142/s0219519418500057
Lemoyne, R., and Mastroianni, T. (2020). “Network centric therapy for machine learning classification of hemiplegic gait through conformal wearable and wireless inertial sensors,” in 2020 International Conference on e-Health and Bioengineering (EHB): IEEE, (New Jersey IEEE), 1–4.
Lemoyne, R., and Mastroianni, T. (2021). Implementation of Machine Learning Classification Regarding Hemiplegic Gait Using an Assortment of Machine Learning Algorithms with Quantification from Conformal Wearable and Wireless Inertial Sensor System. J. Biomed. Sci. Eng. 14, 415–425. doi: 10.4236/jbise.2021.1412035
Lemoyne, R., Mastroianni, T., Cozza, M., Coroian, C., and Grundfest, W. (2010a). “Implementation of an iPhone as a wireless accelerometer for quantifying gait characteristics,” in 2010 Annual International Conference of the IEEE Engineering in Medicine and Biology: IEEE, (New Jersey: IEEE), 3847–3851. doi: 10.1109/IEMBS.2010.5627699
Lemoyne, R., Mastroianni, T., Cozza, M., and Coroian, C. (2010b). Quantification of gait characteristics through a functional iPhone wireless accelerometer application mounted to the spine. Front. Biomed. Dev. 87–88. doi: 10.1115/BioMed2010-32043
Li, X., Xu, H., and Cheung, J. T. (2016a). Gait-force model and inertial measurement unit-based measurements: a new approach for gait analysis and balance monitoring. J. Exerc. Sci. Fit. 14, 60–66. doi: 10.1016/j.jesf.2016.07.002
Li, X., Zhuo, Q., Zhang, X., Samuel, O. W., Xia, Z., Zhang, X., et al. (2016b). “FMG-based body motion registration using piezoelectret sensors,” in 2016 38th Annual International Conference of the IEEE Engineering in Medicine and Biology Society (EMBC): IEEE, (New Jersey: IEEE), 4626–4629. doi: 10.1109/EMBC.2016.7591758
Li, B., Gui, Q., Ali, H. B., Li, H., and Jin, Z. (2016). “A wearable sit-to-stand detection system based on angle tracking and lower limb EMG,” in 2016 IEEE Signal Processing in Medicine and Biology Symposium (SPMB): IEEE, (Piscataway: IEEE), 1–6.
Li, G., Liu, T., Yi, J., Wang, H., and Li, J. (2016). The lower limbs kinematics analysis by wearable sensor shoes. IEEE Sens. J. 16, 2627–2638. doi: 10.1109/jsen.2016.2515101
Lin, K.-L., Lin, K.-J., Wang, P.-W., Chuang, J.-H., Lin, H.-Y., Chen, S.-D., et al. (2018). Resveratrol provides neuroprotective effects through modulation of mitochondrial dynamics and ERK1/2 regulated autophagy. Free Rad. Res. 52, 1371–1386. doi: 10.1080/10715762.2018.1489128
Liu, T., Inoue, Y., and Shibata, K. (2010). A wearable ground reaction force sensor system and its application to the measurement of extrinsic gait variability. Sensors 10, 10240–10255. doi: 10.3390/s101110240
Liu, Y., Li, S., Mu, C., and Wang, Y. (2016). Step length estimation based on D-ZUPT for pedestrian dead-reckoning system. Electron. Lett. 52, 923–924. doi: 10.1049/el.2016.0702
Loose, H., and Orlowski, K. (2015). “Gait patterns in standard scenarios: Using Xsens MTw inertial measurement units,” in 2015 16th International Conference on Research and Education in Mechatronics (REM): IEEE, (Piscataway: IEEE), 296–300.
Lukowicz, P., Hanser, F., Szubski, C., and Schobersberger, W. (2006). “Detecting and interpreting muscle activity with wearable force sensors,” in International Conference on Pervasive Computing: Springer, (New Jersey: IEEE), 101–116. doi: 10.1007/11748625_7
Madiha, S., Batool, Z., Tabassum, S., Liaquat, L., Sadir, S., Shahzad, S., et al. (2021). Quercetin exhibits potent antioxidant activity, restores motor and non-motor deficits induced by rotenone toxicity. PLoS One 16:e0258928. doi: 10.1371/journal.pone.0258928
Makela, K., Hakulinen, J., and Turunen, M. (2003). The use of Walking Sounds in Supporting Awareness. Georgia: Georgia Institute of Technology.
Malafaia, D., Albuquerque, H. M., and Silva, A. (2021). Amyloid-β and tau aggregation dual-inhibitors: a synthetic and structure-activity relationship focused review. Eur. J. Med. Chem. 214:113209. doi: 10.1016/j.ejmech.2021.113209
Mannini, A., Trojaniello, D., Cereatti, A., and Sabatini, A. M. (2016). A machine learning framework for gait classification using inertial sensors: application to elderly, post-stroke and huntington’s disease patients. Sensors 16:134. doi: 10.3390/s16010134
Manoharan, S., Guillemin, G. J., Abiramasundari, R. S., Essa, M. M., Akbar, M., and Akbar, M. (2016). The role of reactive oxygen species in the pathogenesis of Alzheimer’s disease, Parkinson’s disease, and Huntington’s disease: a mini review. Oxid. Med. cell. Longev. 2016:8590578. doi: 10.1155/2016/8590578
Maqbool, H. F., Husman, M., Awad, M. I., Abouhossein, A., Mehryar, P., Iqbal, N., et al. (2016). “Real-time gait event detection for lower limb amputees using a single wearable sensor,” in 2016 38th Annual International Conference of the IEEE Engineering in Medicine and Biology Society (EMBC): IEEE, (New Jersey: IEEE), 5067–5070. doi: 10.1109/EMBC.2016.7591866
Marcante, A., Di Marco, R., Gentile, G., Pellicano, C., Assogna, F., Pontieri, F. E., et al. (2021). Foot pressure wearable sensors for freezing of gait detection in Parkinson’s disease. Sensors 21:128 doi: 10.3390/s21010128
Mariani, B., Rouhani, H., Crevoisier, X., and Aminian, K. (2013). Quantitative estimation of foot-flat and stance phase of gait using foot-worn inertial sensors. Gait posture 37, 229–234. doi: 10.1016/j.gaitpost.2012.07.012
Marinus, J., Zhu, K., Marras, C., Aarsland, D., and Van Hilten, J. (2018). Risk factors for non-motor symptoms in Parkinson’s disease. Lancet Neurol. 17, 559–568.
Mazilu, S., Blanke, U., Dorfman, M., Gazit, E., Mirelman, A., Hausdorff, M., et al. (2015). A wearable assistant for gait training for Parkinson’s disease with freezing of gait in out-of-the-lab environments. ACM Trans. Interact. Intell. Syst. 5, 5:31.
Mazzoli, D., Giannotti, E., Manca, M., Longhi, M., Prati, P., Cosma, M., et al. (2018). Electromyographic activity of the vastus intermedius muscle in patients with stiff-knee gait after stroke. A retrospective observational study. Gait Posture 60, 273–278. doi: 10.1016/j.gaitpost.2017.07.002
Mcguire, M. L. (2012). “An overview of gait analysis and step detection in mobile computing devices,” in 2012 Fourth International Conference on Intelligent Networking and Collaborative Systems: IEEE, (New Jersey: IEEE), 648–651.
Mendoza, M. B., Bergado, C. A., De Castro, J. L. B., and Siasat, R. G. T. (2017). “Tracking system for patients with Alzheimer’s disease in a nursing home,” in TENCON 2017-2017 IEEE Region 10 Conference: IEEE, (New Jersey: IEEE), 2566–2570.
Mn, A. (2014). An Instrumented Insole System for Gait Monitoring and Analysis. Int. J. Online Eng. 10, 30–34. doi: 10.3991/ijoe.v10i6.3971
Mohi-Ud-Din, R., Mir, R. H., Shah, A. J., Sabreen, S., Wani, T. U., Masoodi, M. H., et al. (2022). Plant-derived natural compounds for the treatment of amyotrophic lateral sclerosis: an update. Curr. Neuropharmacol. 20, 179–193. doi: 10.2174/1570159X19666210428120514
Montero-Odasso, M., and Perry, G. (2019). Gait disorders in Alzheimer’s disease and other dementias: there is something in the way you walk. J. Alzheimer’s Dis. 71, S1-S4. doi: 10.3233/JAD-190790
Morbidoni, C., Cucchiarelli, A., Fioretti, S., and Di Nardo, F. J. E. (2019). A deep learning approach to EMG-based classification of gait phases during level ground walking. Electronics 8, 894–908. doi: 10.3390/electronics8080894
Morris, J. (1973). Accelerometry a technique for the measurement of human body movements. J. Biomech. 6, 729–736. doi: 10.1016/0021-9290(73)90029-8
Mueller, M. J., Hastings, M., Commean, P. K., Smith, K. E., Pilgram, T. K., Robertson, D., et al. (2003). Forefoot structural predictors of plantar pressures during walking in people with diabetes and peripheral neuropathy. J. Biomech. 36, 1009–1017. doi: 10.1016/s0021-9290(03)00078-2
Munari, D., Fonte, C., Varalta, V., Battistuzzi, E., Cassini, S., Montagnoli, A. P., et al. (2020). Effects of robot-assisted gait training combined with virtual reality on motor and cognitive functions in patients with multiple sclerosis: a pilot, single-blind, randomized controlled trial. Restor. Neurol. Neurosci. 38, 151–164. doi: 10.3233/RNN-190974
Muro-De-La-Herran, A., Garcia-Zapirain, B., and Mendez-Zorrilla, A. (2014). Gait analysis methods: an overview of wearable and non-wearable systems, highlighting clinical applications. Sensors 14, 3362–3394. doi: 10.3390/s140203362
Muurling, M., De Boer, C., Kozak, R., Religa, D., Koychev, I., Verheij, H., et al. (2021). Remote monitoring technologies in Alzheimer’s disease: design of the RADAR-AD study. Alzheimer’s Res. Ther. 13:89. doi: 10.1186/s13195-021-00825-4
Naik, G. R. (2012). Computational Intelligence in Electromyography Analysis: a Perspective on Current Applications and Future Challenges. Germany: BoD–Books on Demand.
Nair, A. T., Vadivelan, R., Ramachandran, R., and Ahamed, H. (2019). Resveratrol with an adjunct for improved maintenance of mitochondrial homeostasis and dopamine neuronal rescue in neurodegeneration. J. Pharm. Sci. Res. 11, 1210–1215.
Nazmi, N., Rahman, M. A. A., Yamamoto, S.-I., and Ahmad, S. A. (2019). Walking gait event detection based on electromyography signals using artificial neural network. Biomed. Signal. Process. Control 47, 334–343. doi: 10.1016/j.bspc.2018.08.030
Noamani, A., Nazarahari, M., Lewicke, J., Vette, A. H., and Rouhani, H. (2020). Validity of using wearable inertial sensors for assessing the dynamics of standing balance. Med. Eng. phys. 77, 53–59. doi: 10.1016/j.medengphy.2019.10.018
O’brien, M. K., Hidalgo-Araya, M. D., Mummidisetty, C. K., Vallery, H., Ghaffari, R., Rogers, J. A., et al. (2019). Augmenting clinical outcome measures of gait and balance with a single inertial sensor in age-ranged healthy adults. Sensors 19:4537. doi: 10.3390/s19204537
Octavia, J. R., Feys, P., and Coninx, K. (2015). Development of activity-related muscle fatigue during robot-mediated upper limb rehabilitation training in persons with multiple sclerosis: a pilot trial. Mult. Scler. Int. 2015:650431. doi: 10.1155/2015/650431
O’donovan, K. J., Kamnik, R., O’keeffe, D. T., and Lyons, G. M. (2007). An inertial and magnetic sensor based technique for joint angle measurement. J. Biomech. 40, 2604–2611. doi: 10.1016/j.jbiomech.2006.12.010
Ortiz, J. S., Palacios-Navarro, G., Carvajal, C. P., and Andaluz, V. H. (2018). “3D virtual path planning for people with amyotrophic lateral sclerosis through standing wheelchair,” in International Conference on Social Robotics: Springer, (Germany: Springer), 181–191. doi: 10.1007/978-3-030-05204-1_18
Pacilli, A., Mileti, I., Germanotta, M., Di Sipio, E., Imbimbo, I., Aprile, I., et al. (2016). “A wearable setup for auditory cued gait analysis in patients with Parkinson’s Disease,” in 2016 IEEE International Symposium on Medical Measurements and Applications (MeMeA): IEEE, (New Jersey: IEEE), 1–6.
Pal, G., O’keefe, J., Robertson-Dick, E., Bernard, B., Anderson, S., and Hall, D. (2016). Global cognitive function and processing speed are associated with gait and balance dysfunction in Parkinson’s disease. J. Neuroeng. Rehabil. 13:94. doi: 10.1186/s12984-016-0205-y
Palinkas, M., Pagnano, L., Da Siva, G. P., Hallak, J. E. C., Da Silva, N. S., Regalo, I. H., et al. (2019). Does Parkinson’s disease interfere with electromyographic fatigue of masticatory muscles. Arch. Ital. Biol. 157, 105–110. doi: 10.12871/00039829202041
Papavasileiou, I., Zhang, W., Wang, X., Bi, J., Zhang, L., and Han, S. (2017). “Classification of neurological gait disorders using multi-task feature learning,” in Connected Health: Applications, Systems and Engineering Technologies (CHASE), 2017 IEEE/ACM International Conference on: IEEE, (New Jersey: IEEE), 195–204.
Pardoel, S., Shalin, G., Nantel, J., Lemaire, E. D., and Kofman, J. J. S. (2021). Early detection of freezing of gait during walking using inertial measurement unit and plantar pressure distribution data. Sensors 21:2246. doi: 10.3390/s21062246
Parent, A., Pouliot-Laforte, A., Dal Maso, F., Cherni, Y., Marois, P., and Ballaz, L. (2019). Muscle fatigue during a short walking exercise in children with cerebral palsy who walk in a crouch gait. Gait posture 72, 22–27. doi: 10.1016/j.gaitpost.2019.05.021
Pelosin, E., Cerulli, C., Ogliastro, C., Lagravinese, G., Mori, L., Bonassi, G., et al. (2020). A multimodal training modulates short afferent inhibition and improves complex walking in a cohort of faller older adults with an increased prevalence of Parkinson’s disease. J. Gerontol. Series A 75, 722–728. doi: 10.1093/gerona/glz072
Pereira, M. P., Gobbi, L. T., and Almeida, Q. (2016). Freezing of gait in Parkinson’s disease: evidence of sensory rather than attentional mechanisms through muscle vibration. Park. Relat. Disord. 29, 78–82. doi: 10.1016/j.parkreldis.2016.05.021
Peruzzi, A., Zarbo, I. R., Cereatti, A., Della Croce, U., and Mirelman, A. (2017). An innovative training program based on virtual reality and treadmill: effects on gait of persons with multiple sclerosis. Disabil. Rehabil. 39, 1557–1563. doi: 10.1080/09638288.2016.1224935
Picerno, P. (2017). 25 years of lower limb joint kinematics by using inertial and magnetic sensors: a review of methodological approaches. Gait posture 51, 239–246. doi: 10.1016/j.gaitpost.2016.11.008
Picerno, P., Cereatti, A., and Cappozzo, A. (2008). Joint kinematics estimate using wearable inertial and magnetic sensing modules. Gait posture 28, 588–595. doi: 10.1016/j.gaitpost.2008.04.003
Pieruccini-Faria, F., Black, S. E., Masellis, M., Smith, E. E., Almeida, Q. J., Li, K. Z., et al. (2021). Gait variability across neurodegenerative and cognitive disorders: results from the Canadian Consortium of Neurodegeneration in Aging (CCNA) and the Gait and Brain Study. Alzheimer’s Dement. 17, 1317–1328. doi: 10.1002/alz.12298
Pirker, W., and Katzenschlager, R. (2017). Gait disorders in adults and the elderly. Wien. Klin. Wochenschr. 129, 81–95. doi: 10.1007/s00508-016-1096-4
Porcaro, C., Cottone, C., Cancelli, A., Rossini, P. M., Zito, G., and Tecchio, F. (2019). Cortical neurodynamics changes mediate the efficacy of a personalized neuromodulation against multiple sclerosis fatigue. Sci. Rep. 9:18213. doi: 10.1038/s41598-019-54595-z
Price, N. L., Gomes, A. P., Ling, A. J., Duarte, F. V., Martin-Montalvo, A., North, B. J., et al. (2012). SIRT1 is required for AMPK activation and the beneficial effects of resveratrol on mitochondrial function. Cell Metab. 15, 675–690. doi: 10.1016/j.cmet.2012.04.003
Purcell, N. L., Goldman, J. G., Ouyang, B., Bernard, B., and O’keefe, J. (2019). The effects of dual-task cognitive interference and environmental challenges on balance in Huntington’s disease. Mov. Disord. Clin. Pract. 6, 202–212. doi: 10.1002/mdc3.12720
Qi, Y., Soh, C. B., Gunawan, E., and Low, K.-S. (2014). Ambulatory measurement of three-dimensional foot displacement during treadmill walking using wearable wireless ultrasonic sensor network. IEEE J. Biomed. Health Inform. 19, 446–452. doi: 10.1109/JBHI.2014.2316998
Qin, L.-Y., Ma, H., and Liao, W.-H. (2015). “Insole plantar pressure systems in the gait analysis of post-stroke rehabilitation,” in 2015 IEEE International Conference on Information and Automation: IEEE, (New Jersey: IEEE), 1784–1789.
Rahman, M. A., and Rhim, H. (2017). Therapeutic implication of autophagy in neurodegenerative diseases. BMB Rep. 50:345. doi: 10.5483/bmbrep.2017.50.7.069
Ramires Júnior, O. V., Alves, B. D. S., Barros, P., Rodrigues, J. L., Ferreira, S. P., Monteiro, L. K. S., et al. (2021). Nanoemulsion Improves the Neuroprotective Effects of Curcumin in an Experimental Model of Parkinson’s Disease. Neurotox. Res. 39, 787–799. doi: 10.1007/s12640-021-00362-w
Rampp, A., Barth, J., Schülein, S., Gassmann, K.-G., Klucken, J., and Eskofier, B. M. (2015). Inertial sensor-based stride parameter calculation from gait sequences in geriatric patients. Biomed. Eng. IEEE Trans. 62, 1089–1097. doi: 10.1109/TBME.2014.2368211
Rasool, G., Iqbal, K., White, G. A. J. C., and Applications, M. W. (2012). Myoelectric activity detection during a Sit-to-Stand movement using threshold methods. Comput. Math. Appl. 64, 1473–1483. doi: 10.1016/j.camwa.2012.03.094
Rasouli, M., Ghosh, R., Lee, W. W., Thakor, N. V., and Kukreja, S. (2015). “Stable force-myographic control of a prosthetic hand using incremental learning,” in 2015 37th Annual International Conference of the IEEE Engineering in Medicine and Biology Society (EMBC): IEEE, (New Jersey: IEEE), 4828–4831. doi: 10.1109/EMBC.2015.7319474
Raveendranathan, N., Galzarano, S., Loseu, V., Gravina, R., Giannantonio, R., Sgroi, M., et al. (2011). From modeling to implementation of virtual sensors in body sensor networks. IEEE Sens. J. 12, 583–593. doi: 10.1109/jsen.2011.2121059
Reddy, P. H., Manczak, M., Yin, X., Grady, M. C., Mitchell, A., Tonk, S., et al. (2018). Protective effects of Indian spice curcumin against amyloid-β in Alzheimer’s disease. J. Alzheimer’s Dis. 61, 843–866. doi: 10.3233/JAD-170512
Rezaee, K., Savarkar, S., Yu, X., and Zhang, J. (2022). A hybrid deep transfer learning-based approach for Parkinson’s disease classification in surface electromyography signals. Biomed. Signal. Process. Control 71:103161. doi: 10.1016/j.bspc.2021.103161
Roldán Jiménez, C., Bennett, P., Ortiz García, A., and Cuesta Vargas, A. (2019). Fatigue detection during sit-to-stand test based on surface electromyography and acceleration: a case study. Sensors 19:4202. doi: 10.3390/s19194202
Ropars, J., Lempereur, M., Vuillerot, C., Tiffreau, V., Peudenier, S., Cuisset, J.-M., et al. (2016). Muscle activation during gait in children with Duchenne muscular dystrophy. PLoS One 11:e0161938. doi: 10.1371/journal.pone.0161938
Rossi, S., Lisini Baldi, T., Aggravi, M., Ulivelli, M., Cioncoloni, D., Niccolini, V., et al. (2020). Wearable haptic anklets for gait and freezing improvement in Parkinson’s disease: a proof-of-concept study. Neurol. Sci. 41, 3643–3651. doi: 10.1007/s10072-020-04485-4
Roy, G., Jacob, T., Bhatia, D., and Bhaumik, S. (2020). “Optical Marker- and Vision-Based Human Gait Biomechanical Analysis”,” in Hybrid Machine Intelligence for Medical Image Analysis. Studies in Computational Intelligence, eds S. Bhattacharyya, D. Konar, J. Platos, C. Kar, and K. Sharma (Berlin: Springer), 841. doi: 10.1007/978-981-13-8930-6-11
Ryu, J., and Kim, D.-H. (2017). Real-time gait subphase detection using an EMG signal graph matching (ESGM) algorithm based on EMG signals. Exp. Syst. Appl. 85, 357–365. doi: 10.1016/j.eswa.2017.05.006
Saif, N., Yan, P., Niotis, K., Scheyer, O., Rahman, A., Berkowitz, M., et al. (2020). Feasibility of using a wearable biosensor device in patients at risk for Alzheimer’s disease dementia. J. prevent. Alzheimer’s Dis. 7, 104–111. doi: 10.14283/jpad.2019.39
Salarian, A., Burkhard, P. R., Vingerhoets, F. J., Jolles, B. M., and Aminian, K. (2012). A novel approach to reducing number of sensing units for wearable gait analysis systems. IEEE Trans. Biomed. Eng. 60, 72–77. doi: 10.1109/TBME.2012.2223465
Schepers, M., Giuberti, M., and Bellusci, G. (2018). Xsens mvn: consistent tracking of human motion using inertial sensing. Xsens Technol. 2018:1–8.
Schrager, M. A., Hilton, J., Gould, R., and Kelly, V. (2015). Effects of blueberry supplementation on measures of functional mobility in older adults. Appl. Physiol. Nutr. Metab. 40, 543–549. doi: 10.1139/apnm-2014-0247
Schülein, S., Barth, J., Rampp, A., Rupprecht, R., Eskofier, B. M., Winkler, J., et al. (2017). Instrumented gait analysis: a measure of gait improvement by a wheeled walker in hospitalized geriatric patients. J. Neuroeng. Rehabil. 14:18. doi: 10.1186/s12984-017-0228-z
Seel, T., Raisch, J., and Schauer, T. (2014). IMU-based joint angle measurement for gait analysis. Sensors 14, 6891–6909. doi: 10.3390/s140406891
Serafini, M. M., Catanzaro, M., Rosini, M., Racchi, M., and Lanni, C. (2017). Curcumin in Alzheimer’s disease: can we think to new strategies and perspectives for this molecule? Pharmacol. Res. 124, 146–155. doi: 10.1016/j.phrs.2017.08.004
Shalin, G., Pardoel, S., Lemaire, E. D., Nantel, J., Kofman, J. J. J. O. N., and Rehabilitation. (2021). Prediction and detection of freezing of gait in Parkinson’s disease from plantar pressure data using long short-term memory neural-networks. J. NeuroEng. Rehabil. 18:16718. doi: 10.1186/s12984-021-00958-5
Sorrentino, I., Andrade Chavez, F. J., Latella, C., Fiorio, L., Traversaro, S., Rapetti, L., et al. (2020). A Novel Sensorised Insole for Sensing Feet Pressure Distributions. Sensors 20:747. doi: 10.3390/s20030747
Sprager, S., and Juric, M. B. (2015). Inertial sensor-based gait recognition: a review. Sensors 15, 22089–22127. doi: 10.3390/s150922089
Stańczyk, K., Poświata, A., Roksela, A., Mikulski, M. (2019). “Assessment of muscle fatigue, strength and muscle activation during exercises with the usage of robot luna EMG, among patients with multiple sclerosis,” in Information Technology in Biomedicine. ITIB 2019. Advances in Intelligent Systems and Computing, Vol. 1011, eds Pietka, E., Badura, P., Kawa, J., and Wieclawek, W. (Cham: Springer), 117–128. doi: 10.1007/978-3-030-23762-2_11
Stavropoulos, T. G., Lazarou, I., Diaz, A., Gove, D., Georges, J., Manyakov, N. V., et al. (2021). Wearable devices for assessing function in Alzheimer’s disease: a european public involvement activity about the features and preferences of patients and caregivers. Front. Aging Neurosci. 13:643135. doi: 10.3389/fnagi.2021.643135
Su, I.-J., Chang, H.-Y., Wang, H.-C., and Tsai, K. (2020). A curcumin analog exhibits multiple biologic effects on the pathogenesis of Alzheimer’s disease and improves behavior, inflammation, and β-amyloid accumulation in a mouse model. Int. J. Mol. Sci. 21:5459. doi: 10.3390/ijms21155459
Sun, H., Yuan, T., Li, X., and Hu, Y. (2012). Accelerometer-based gait authentication via neural network. Chin. J. Electron. 21, 481–484.
Sun, T., Liu, Q., Li, W., Lu, Z., Chen, H., Chen, P., et al. (2016). “Hip, knee and ankle motion angle detection based on inertial sensor,” in 2016 IEEE International Conference on Information and Automation (ICIA): IEEE, (Piscataway: IEEE), 1612–1617.
Suppa, A., Kita, A., Leodori, G., Zampogna, A., Nicolini, E., Lorenzi, P., et al. (2017). L-DOPA and freezing of gait in Parkinson’s disease: objective assessment through a wearable wireless system. Front. Neurol. 8:406. doi: 10.3389/fneur.2017.00406
Susi, M., Renaudin, V., and Lachapelle, G. (2013). Motion mode recognition and step detection algorithms for mobile phone users. Sensors 13, 1539–1562. doi: 10.3390/s130201539
Tahir, A. M., Chowdhury, M. E., Khandakar, A., Al-Hamouz, S., Abdalla, M., Awadallah, S., et al. (2020). A systematic approach to the design and characterization of a smart insole for detecting vertical ground reaction force (vGRF) in gait analysis. Sensors 20:957. doi: 10.3390/s20040957
Takeda, R., Lisco, G., Fujisawa, T., Gastaldi, L., Tohyama, H., and Tadano, S. (2014). Drift removal for improving the accuracy of gait parameters using wearable sensor systems. Sensors 14, 23230–23247. doi: 10.3390/s141223230
Takeda, R., Tadano, S., Todoh, M., Morikawa, M., Nakayasu, M., and Yoshinari, S. (2009). Gait analysis using gravitational acceleration measured by wearable sensors. J. Biomech. 42, 223–233. doi: 10.1016/j.jbiomech.2008.10.027
Tang, M., and Taghibiglou, C. (2017). The mechanisms of action of curcumin in Alzheimer’s disease. J. Alzheimer’s Dis. 58, 1003–1016. doi: 10.3233/jad-170188
Tao, W., Liu, T., Zheng, R., and Feng, H. (2012). Gait analysis using wearable sensors. Sensors 12, 2255–2283. doi: 10.3390/s120202255
Teufl, W., Lorenz, M., Miezal, M., Taetz, B., Fröhlich, M., and Bleser, G. (2019a). Towards inertial sensor based mobile gait analysis: event-detection and spatio-temporal parameters. Sensors 19:38. doi: 10.3390/s19010038
Teufl, W., Taetz, B., Miezal, M., Lorenz, M., Pietschmann, J., Jöllenbeck, T., et al. (2019b). Towards an Inertial Sensor-Based Wearable Feedback System for Patients after Total Hip Arthroplasty: validity and Applicability for Gait Classification with Gait Kinematics-Based Features. Sensors 19:5006. doi: 10.3390/s19225006
Thies, S. B., Ashton-Miller, J. A., and Richardson, J. K. (2007). What causes a crossover step when walking on uneven ground?: a study in healthy young women. Gait posture 26, 156–160. doi: 10.1016/j.gaitpost.2006.08.011
Tian, Q., Studenski, S. A., An, Y., Kuo, P.-L., Schrack, J. A., Wanigatunga, A. A., et al. (2021). Association of Combined Slow Gait and Low Activity Fragmentation With Later Onset of Cognitive Impairment. JAMA Netw. Open 4:e2135168. doi: 10.1001/jamanetworkopen.2021.35168
Tong, K., and Granat, M. H. (1999). A practical gait analysis system using gyroscopes. Med. Eng. phys. 21, 87–94. doi: 10.1016/s1350-4533(99)00030-2
Truong, H., Zhang, S., Muncuk, U., Nguyen, P., Bui, N., Nguyen, A., et al. (2018). “Capband: Battery-free successive capacitance sensing wristband for hand gesture recognition,” in Proceedings of the 16th ACM Conference on Embedded Networked Sensor Systems, (Piscataway: IEEE), 54–67.
Turcano, P., Mielke, M. M., Bower, J. H., Parisi, J. E., Cutsforth-Gregory, J. K., Ahlskog, J. E., et al. (2018). Levodopa-induced dyskinesia in Parkinson disease: a population-based cohort study. Neurology 91, 2238–2243. doi: 10.1212/WNL.0000000000006643
Uğur, F., and Sertel, M. (2020). The Effect of Virtual Reality Applications on Balance and Gait Speed in Individuals With Alzheimer Dementia: a Pilot Study. Top. Geriatr. Rehabil. 36, 221–229. doi: 10.1097/tgr.0000000000000285
Utomo, R. Y., Asawa, Y., Okada, S., Ban, H. S., Yoshimori, A., Bajorath, J., et al. (2021). Development of curcumin-based amyloid β aggregation inhibitors for Alzheimer’s disease using the SAR matrix approach. Bioorg. Med. Chem. 46:116357. doi: 10.1016/j.bmc.2021.116357
Vallabhajosula, S., Buckley, T. A., Tillman, M. D., and Hass, C. (2013). Age and Parkinson’s disease related kinematic alterations during multi-directional gait initiation. Gait posture 37, 280–286. doi: 10.1016/j.gaitpost.2012.07.018
Van Eijk, R., Bakers, J. N., Bunte, T. M., De Fockert, A. J., Eijkemans, M. J., and Van Den Berg, L. (2019). Accelerometry for remote monitoring of physical activity in amyotrophic lateral sclerosis: a longitudinal cohort study. J. Neurol. 266, 2387–2395. doi: 10.1007/s00415-019-09427-5
Van Kampen, J., Robertson, H., Hagg, T., and Drobitch, R. J. (2003). Neuroprotective actions of the ginseng extract G115 in two rodent models of Parkinson’s disease. Exp. Neurol. 184, 521–529. doi: 10.1016/j.expneurol.2003.08.002
Voinescu, A., Sui, J., and Fraser, S. (2021). Virtual Reality in Neurorehabilitation: An Umbrella Review of Meta-Analyses. J. Clin. Med. 10:1478. doi: 10.3390/jcm10071478
Wada, C., Sugimura, Y., Wada, F., Hachisuka, K., Ienaga, T., Kimuro, Y., et al. (2010). “Development of a rehabilitation support system with a shoe-type measurement device for walking,” in Proceedings of SICE Annual Conference 2010: IEEE, (New Jersey: IEEE), 2534–2537.
Wahab, Y., Zayegh, A., Begg, R. K., and Veljanovski, R. (2008). “Design of MEMS biomedical pressure sensor for gait analysis,” in 2008 IEEE International Conference on Semiconductor Electronics: IEEE, (New Jersey: IEEE), 166–169.
Wahid, F., Begg, R. K., Hass, C. J., Halgamuge, S., and Ackland, D. C. (2015). Classification of Parkinson’s disease gait using spatial-temporal gait features. IEEE J. Biomed. Health Inform. 19, 1794–1802. doi: 10.1109/JBHI.2015.2450232
Wang, C., Song, C., Wang, X., Huang, L., Ding, M., Yang, H., et al. (2019). Protective effects of melatonin on mitochondrial biogenesis and mitochondrial structure and function in the HEK293-APPswe cell model of Alzheimer’s disease. Eur. Rev. Med. Pharmacol. Sci. 23, 3542–3550. doi: 10.26355/eurrev_201904_17723
Wang, C., Wang, X., Long, Z., Yuan, J., Qian, Y., and Li, J. (2016). Estimation of temporal gait parameters using a wearable microphone-sensor-based system. Sensors 16:2167. doi: 10.3390/s16122167
Wang, S., Zeng, X., Huangfu, L., Xie, Z., Ma, L., Huang, W., et al. (2021). Validation of a portable marker-based motion analysis system. J. Orthop. surg. Res. 16:425. doi: 10.1186/s13018-021-02576-2
Wang, W., Li, K., Yue, S., Yin, C., and Wei, N. (2017). Associations between lower-limb muscle activation and knee flexion in post-stroke individuals: a study on the stance-to-swing phases of gait. PLoS One 12:e0183865. doi: 10.1371/journal.pone.0183865
Wang, W.-H., Chung, P.-C., Yang, G.-L., Lin, C.-W., Hsu, Y.-L., and Pai, M.-C. (2015). “An inertial sensor based balance and gait analysis system,” in 2015 IEEE International Symposium on Circuits and Systems (ISCAS): IEEE, (Piscataway: IEEE), 2636–2639.
Wang, Z., and Ji, R. (2015). “Estimate spatial-temporal parameters of human gait using inertial sensors,” in 2015 IEEE International Conference on Cyber Technology in Automation, Control, and Intelligent Systems (CYBER): IEEE, (Piscataway: IEEE), 1883–1888.
Wang, Z.-X., Tan, L., and Yu, J.-T. (2015). Axonal transport defects in Alzheimer’s disease. Mol. Neurobiol. 51, 1309–1321. doi: 10.1007/s12035-014-8810-x
Whittle, M. W. (1982). Calibration and performance of a 3-dimensional television system for kinematic analysis. J. Biomech. 15, 185–196. doi: 10.1016/0021-9290(82)90251-2
Whittle, M. W. (1996). Clinical gait analysis: a review. Hum. Move. sci. 15, 369–387. doi: 10.1016/j.clinbiomech.2008.07.012
Willemsen, A. T. M., Van Alste, J. A., and Boom, H. (1990). Real-time gait assessment utilizing a new way of accelerometry. J. Biomech. 23, 859–863. doi: 10.1016/0021-9290(90)90033-y
Wouda, F. J., Giuberti, M., Bellusci, G., and Veltink, P. H. (2016). Estimation of full-body poses using only five inertial sensors: an eager or lazy learning approach? Sensors 16:2138. doi: 10.3390/s16122138
World Health Organization (2006). Neurological Disorders: Public Health Challenges. Geneva: World Health Organization.
Xiao, Z. G., and Menon, C. (2019). A review of force myography research and development. Sensors 19:4557. doi: 10.3390/s19204557
Xu, X., Tay, Y., Sim, B., Yoon, S.-I., Huang, Y., Ooi, J., et al. (2017). Reversal of phenotypic abnormalities by CRISPR/Cas9-mediated gene correction in Huntington disease patient-derived induced pluripotent stem cells. Stem cell Rep. 8, 619–633. doi: 10.1016/j.stemcr.2017.01.022
Yadav, S. K., Rai, S. N., and Singh, S. P. (2016). Mucuna pruriens shows neuroprotective effect by inhibiting apoptotic pathways of dopaminergic neurons in the paraquat mouse model of parkinsonism. Eur. J. Pharmaceut. Med. Res. 3, 441–451.
Yamagami, M., Imsdahl, S., Lindgren, K., Bellatin, O., Nhan, N., Burden, S. A., et al. (2020). Effects of virtual reality environments on overground walking in people with Parkinson disease and freezing of gait. Disabil. Rehabil. Assist. Technol. 6, 1–8. doi: 10.1080/17483107.2020.1842920
Yang, C.-M., Chou, C.-M., Hu, J.-S., Hung, S.-H., Yang, C.-H., Wu, C.-C., et al. (2009). “A wireless gait analysis system by digital textile sensors,” in 2009 Annual International Conference of the IEEE Engineering in Medicine and Biology Society: IEEE, (New Jersey: IEEE), 7256–7260. doi: 10.1109/IEMBS.2009.5334738
Yang, M., Zheng, H., Wang, H., Mcclean, S., and Newell, D. (2012). iGAIT: an interactive accelerometer based gait analysis system. Comput. Methods Prog. Biomed. 108, 715–723. doi: 10.1016/j.cmpb.2012.04.004
Yeo, S. S., and Park, G. Y. (2020). Accuracy verification of spatio-temporal and kinematic parameters for gait using inertial measurement unit system. Sensors 20:1343. doi: 10.3390/s20051343
Yong, F., Yunjian, G., and Quanjun, S. (2011). “A human identification method based on dynamic plantar pressure distribution,” in Proceeding of 2011 IEEE International Conference on Information and Automation (ICIA), (New Jersey: IEEE), 329–332. doi: 10.1016/s0168-6054(02)00186-1
Yungher, D. A., Wininger, M. T., Barr, J., Craelius, W., and Threlkeld, A. J. (2011). Surface muscle pressure as a measure of active and passive behavior of muscles during gait. Med. Eng. phys. 33, 464–471. doi: 10.1016/j.medengphy.2010.11.012
Zago, M., Luzzago, M., Marangoni, T., De Cecco, M., Tarabini, M., and Galli, M. (2020). 3D tracking of human motion using visual skeletonization and stereoscopic vision. Front. Bioeng. Biotechnol. 8:181. doi: 10.3389/fbioe.2020.00181
Zahra, N. (2021). Application of Wavelet in Quantitative Evaluation of Gait Events of Parkinson’s Disease. Appl. Bionics Biomech. 2021:7199007. doi: 10.1155/2021/7199007
Zeng, H., and Zhao, Y. (2011). Sensing movement: microsensors for body motion measurement. Sensors 11, 638–660. doi: 10.3390/s110100638
Zhang, J.-T., Novak, A. C., Brouwer, B., and Li, Q. (2013). Concurrent validation of Xsens MVN measurement of lower limb joint angular kinematics. Physiol. Meas. 34, N63-9. doi: 10.1088/0967-3334/34/8/N63
Zhang, Q., Wang, Y. L., Xia, Y., Wu, X., Kirk, T. V., and Chen, X. D. (2019). A low-cost and highly integrated sensing insole for plantar pressure measurement. Sens. BioSensing Res. 26:100298. doi: 10.1016/j.sbsr.2019.100298
Zhao, G., Jiang, Z.-H., Zheng, X.-W., Zang, S.-Y., and Guo, L.-H. (2008). Dopamine transporter inhibitory and antiparkinsonian effect of common flowering quince extract. Pharmacol. Biochem. Behav. 90, 363–371. doi: 10.1016/j.pbb.2008.03.014
Ziegier, J., Gattringer, H., and Mueller, A. (2018). “Classification of gait phases based on bilateral emg data using support vector machines,” in 2018 7th IEEE International Conference on Biomedical Robotics and Biomechatronics (Biorob): IEEE, (New Jersey: IEEE), 978–983.
Keywords: gait, inertial sensor, myography, plantar pressure, postural balance, wearable sensors, neurological disorder, phytochemical
Citation: Das R, Paul S, Mourya GK, Kumar N and Hussain M (2022) Recent Trends and Practices Toward Assessment and Rehabilitation of Neurodegenerative Disorders: Insights From Human Gait. Front. Neurosci. 16:859298. doi: 10.3389/fnins.2022.859298
Received: 21 January 2022; Accepted: 01 March 2022;
Published: 15 April 2022.
Edited by:
Gaurav Kumar, Galgotias University, IndiaReviewed by:
Ravi Prakash Tewari, Motilal Nehru National Institute of Technology Allahabad, IndiaSachchida Nand Rai, University of Allahabad, India
Robert LeMoyne, Northern Arizona University, United States
Copyright © 2022 Das, Paul, Mourya, Kumar and Hussain. This is an open-access article distributed under the terms of the Creative Commons Attribution License (CC BY). The use, distribution or reproduction in other forums is permitted, provided the original author(s) and the copyright owner(s) are credited and that the original publication in this journal is cited, in accordance with accepted academic practice. No use, distribution or reproduction is permitted which does not comply with these terms.
*Correspondence: Sudip Paul, sudip.paul.bhu@gmail.com