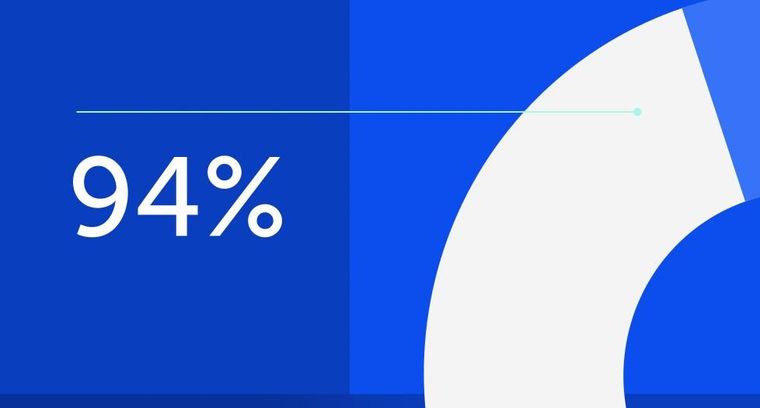
94% of researchers rate our articles as excellent or good
Learn more about the work of our research integrity team to safeguard the quality of each article we publish.
Find out more
EDITORIAL article
Front. Neurosci., 13 April 2022
Sec. Brain Imaging Methods
Volume 16 - 2022 | https://doi.org/10.3389/fnins.2022.854471
This article is part of the Research TopicFrom raw MEG/EEG to publication: how to perform MEG/EEG group analysis with free academic softwareView all 26 articles
Editorial of the Research Topic
From Raw MEG/EEG to Publication: How to Perform MEG/EEG Group Analysis With Free Academic Software
Free and open-source academic toolboxes have gained increasing prominence in the field of MEG/EEG research to disseminate cutting-edge methods, share best practices between different research groups, and pool resources for developing essential tools for the MEG/EEG community. Large and vibrant research communities have emerged around several of these toolboxes in recent years. Training events are regularly held around the world where the basics of each toolbox are explained by its respective developers and experienced power users. However, most training material and tutorials only show analysis of a single “typical best” subject, whereas most real MEG/EEG studies involve group data analysis. It is then left to the researchers to figure out how to make the transition and obtain group results. This special Research Topic addresses this gap by publishing detailed descriptions of complete group analyses for which code and data are also shared. The level of detail of the description should be such that the readers will be able to fully reproduce the analysis and results and port the analysis to their own data.
A total of 25 articles, summarized in Table 1, were accepted for this special issue. In particular to foster comparable analysis with different tools and strategies, we encouraged authors to reuse a dataset containing responses to face stimuli acquired by Richard Henson and Daniel Wakeman (Wakeman and Henson, 2015; https://openfmri.org/dataset/ds000117/) (HW dataset). This dataset is formatted following the Brain Imaging Data Structure specification (Gorgolewski et al., 2016), which has become increasingly popular in the MEG (Niso et al., 2018), EEG (Pernet et al., 2019) and iEEG fields (Holdgraf et al., 2019). The specific dataset contains multiple modalities, including EEG (with digitized electrode positions), MEG, fMRI, and anatomical MRI, making it suitable for demonstrating multimodal analysis pipelines. Out of the 25 published articles, 10 are using this data (Table 1). All other articles used data that is also publicly available.
The articles in this special issue focus on different aspects of MEEG data processing. Some articles processed EEG data (n = 9), MEG data (n = 8), joint EEG/MEG data (n = 7), or even EEG/MEG/fMRI data (n = 1). Four articles focused on automated processing of EEG data, 10 dealt
with source localization, 3 with connectivity analysis, 3 with statistical analysis, 2 with EEG data classification. Other topics included microstates and Bayesian modeling. Submissions were based on existing MEEG software, in particular EEGLAB (n = 7), FieldTrip (n = 7), MNE (n = 4), SPM (n = 3), Brainstorm (n = 2), and NUTMEG (n = 1). Of the 25 articles, 21 are using MATLAB, 4 are using Python, and 1 is partially using R. Most scripts and tools were released under the GNU/GPL license (n = 10), BSD or MIT commercial friendly license (n = 2), no specific license (n = 11), or a combination of licenses (n = 2).
For researchers starting to process MEG/EEG data, we would recommend downloading the HW dataset (https://doi.org/10.18112/openneuro.ds000117.v1.0.5) and trying the methods described in this special issue. A simplified BIDS version of this dataset with EEG only is also available (https://doi.org/10.18112/openneuro.ds002718.v1.0.5). Furthermore, we recommend researchers to format their own data to BIDS to facilitate the application of some of the tools in this special issue and help the field move toward better tool integration centered on the BIDS framework.
Overall, there is tremendous potential in using different tools to process the same datasets. First, it forces tool developers to use a standard data format (BIDS) and increases interoperability between tools. Second, these tools offer common features, so the community may compare and check the numerical validity of each approach. Validity checking of MEEG signal processing approaches is important for open-source software, which often has limited resources assigned for testing purposes. Being able to process the same dataset using different tools also makes it simpler for users to compare them and see which one fits their style best, whether it is mixed GUI/script tools like EEGLAB, Brainstorm, SPM and NUTMEG or pure scripting tools such as Fieldtrip or MNE. Finally, making it possible to combine the signal processing pipelines of different tools allows users to develop approaches, leading to new methodological developments.
AD wrote the manuscript. RO, FT, AG, SN, and VL edited the manuscript. All authors contributed to the article and approved the submitted version.
The authors declare that the research was conducted in the absence of any commercial or financial relationships that could be construed as a potential conflict of interest.
All claims expressed in this article are solely those of the authors and do not necessarily represent those of their affiliated organizations, or those of the publisher, the editors and the reviewers. Any product that may be evaluated in this article, or claim that may be made by its manufacturer, is not guaranteed or endorsed by the publisher.
Gorgolewski, K.J., Auer, T., Calhoun, V.D., Craddock, R.C., Das, S., Duff, E.P., et al. (2016). The brain imaging data structure, a format for organizing and describing outputs of neuroimaging experiments. Sci. Data. 3, 160044. doi: 10.1038/sdata.2016.44
Holdgraf, C., Appelhoff, S., Bickel, S., Bouchard, K., D'Ambrosio, S., David, O., et al. (2019). iEEG-BIDS, extending the Brain Imaging Data Structure specification to human intracranial electrophysiology. Sci. Data. 6, 102. doi: 10.1038/s41597-019-0105-7
Niso, G., Gorgolewski, K.J., Bock, E., Brooks, T.L., Flandin, G., Gramfort, A., et al. (2018). MEG-BIDS, the brain imaging data structure extended to magnetoencephalography. Sci. Data. 5, 180110. doi: 10.1038/sdata.2018.110
Pernet, C.R., Appelhoff, S., Gorgolewski, K.J., Flandin, G., Phillips, C., Delorme, A., et al. (2019). EEG-BIDS, an extension to the brain imaging data structure for electroencephalography. Sci. Data. 6, 103. doi: 10.1038/s41597-019-0104-8
Keywords: EEG, MEG, iEEG, BIDS, pipeline
Citation: Delorme A, Oostenveld R, Tadel F, Gramfort A, Nagarajan S and Litvak V (2022) Editorial: From Raw MEG/EEG to Publication: How to Perform MEG/EEG Group Analysis With Free Academic Software. Front. Neurosci. 16:854471. doi: 10.3389/fnins.2022.854471
Received: 14 January 2022; Accepted: 25 February 2022;
Published: 13 April 2022.
Edited and reviewed by: Vince D. Calhoun, Georgia State University, United States
Copyright © 2022 Delorme, Oostenveld, Tadel, Gramfort, Nagarajan and Litvak. This is an open-access article distributed under the terms of the Creative Commons Attribution License (CC BY). The use, distribution or reproduction in other forums is permitted, provided the original author(s) and the copyright owner(s) are credited and that the original publication in this journal is cited, in accordance with accepted academic practice. No use, distribution or reproduction is permitted which does not comply with these terms.
*Correspondence: Arnaud Delorme, YXJub2RlbG9ybWVAZ21haWwuY29t
Disclaimer: All claims expressed in this article are solely those of the authors and do not necessarily represent those of their affiliated organizations, or those of the publisher, the editors and the reviewers. Any product that may be evaluated in this article or claim that may be made by its manufacturer is not guaranteed or endorsed by the publisher.
Research integrity at Frontiers
Learn more about the work of our research integrity team to safeguard the quality of each article we publish.