- 1Food Quality Perception and Society Team, iSENSE Lab, Department of Food Science, Aarhus University, Aarhus, Denmark
- 2Sino-Danish College (SDC), University of Chinese Academy of Sciences, Beijing, China
- 3Neuropsychology and Applied Cognitive Neuroscience Laboratory, CAS Key Laboratory of Mental Health, Institute of Psychology, Chinese Academy of Sciences, Beijing, China
- 4Department of Clinical Medicine, Center for Music in the Brain, Aarhus University, Aarhus, Denmark
- 5Department of Psychology, University of Chinese Academy of Sciences, Beijing, China
Our ability to evaluate long-term goals over immediate rewards is manifested in the brain’s decision circuit. Simplistically, it can be divided into a fast, impulsive, reward “system 1” and a slow, deliberate, control “system 2.” In a noisy eating environment, our cognitive resources may get depleted, potentially leading to cognitive overload, emotional arousal, and consequently more rash decisions, such as unhealthy food choices. Here, we investigated the combined impact of cognitive regulation and ambient noise on food cravings through neurophysiological activity. Thirty-seven participants were recruited for an adapted version of the Regulation of Craving (ROC) task. All participants underwent two sessions of the ROC task; once with soft ambient restaurant noise (∼50 dB) and once with loud ambient restaurant noise (∼70 dB), while data from electroencephalography (EEG), electrodermal activity (EDA), and self-reported craving were collected for all palatable food images presented in the task. The results indicated that thinking about future (“later”) consequences vs. immediate (“now”) sensations associated with the food decreased cravings, which were mediated by frontal EEG alpha power. Likewise, “later” trials also increased frontal alpha asymmetry (FAA) —an index for emotional motivation. Furthermore, loud (vs. soft) noise increased alpha, beta, and theta activity, but for theta activity, this was solely occurring during “later” trials. Similarly, EDA signal peak probability was also higher during loud noise. Collectively, our findings suggest that the presence of loud ambient noise in conjunction with prospective thinking can lead to the highest emotional arousal and cognitive load as measured by EDA and EEG, respectively, both of which are important in regulating cravings and decisions. Thus, exploring the combined effects of interoceptive regulation and exteroceptive cues on food-related decision-making could be methodologically advantageous in consumer neuroscience and entail theoretical, commercial, and managerial implications.
Introduction
Value-Based Decision-Making
Our ability to evaluate long-term goals over immediate rewards is encoded in an array of complex computational processes in the brain (Rangel et al., 2008; Levin et al., 2012). These include resisting the impulse of consuming palatable foods, foreseeing the future potential health consequences associated, and at the same time being able to delay one’s gratification by valuing the “rational” alternative despite temporal discounting (Volkow and Baler, 2015; Cai et al., 2019).
Indeed, our choices and decisions ought to fulfill both immediate needs and those that are better served for future gains (Motoki et al., 2019). To evolutionarily optimize such balanced utilitarian behaviors, the neural circuitry of human decision-making can simplistically be divided into two neuroanatomically and -functionally distinctive systems—an automatic, emotional, impulsive system (bottom-up) and a deliberate, reflective, control system (top-down)—popularly referred to as a fast “system 1” and a slow “system 2” (Evans, 2007; Chen et al., 2018). While the emotional and motivational behaviors of system 1 are manifested in deeper striatal brain structures, the prefrontal cortices govern the cognitive and prospective system 2 functions (Peng-Li et al., 2020c).
Without cognitive inhibition of system 2, the mere presence of appetitive and salient food cues reinforces anticipatory reward (“wanting”) responses through sensitized neural firing of dopamine, potentially leading to excess food consumption, weight gain, and even addictive behaviors (Burger and Stice, 2012; Schulte et al., 2016; Coccurello and Maccarrone, 2018; Nguyen et al., 2021).
Top-Down Cognitive Regulation
In fact, several cognitive strategies have been proposed to facilitate top-down self-regulatory eating behaviors, such as mental imagery (Petit et al., 2017; Zorjan et al., 2020) or episodic future thinking (Dassen et al., 2016; Sun and Kober, 2020). These self-managerial strategies are important components in cognitive-behavioral treatments for treating obesity, eating disorders and addictions (Grilo et al., 2011; Gearhardt et al., 2012) and have been instrumentalized in experimental paradigms (Sun and Kober, 2020).
The Regulation of Craving (ROC) task, originally developed by Kober et al. (2010a) attempts to measure the specific causal effect of regulation strategies on craving for cigarette, alcohol, and/or foods (Kober et al., 2010b; Boswell et al., 2018; Suzuki et al., 2020). The ROC task enables quantification and casual inferences of the underlying neural mechanisms of cue-induced cravings from an immediate “now” perspective (anticipatory reward) and a future “later” decision perspective (delayed gratification). For instance, using functional Magnetic Resonance Imaging (fMRI), Kober et al. (2010b) demonstrated that cravings for both cigarettes and food decreased when thinking about long-term consequences vs. immediate sensations. These subjective ratings were reflected in the blood-oxygen-level-dependent (BOLD) signal which showed that later (vs. now) -trials increased activation in the dorsomedial prefrontal cortex (dmPFC), dorsolateral prefrontal cortex (dlPFC), and ventrolateral prefrontal cortex (vlPFC)—all a part of the reflective system 2—whereas they decreased activity in brain regions associated with emotion and reward valuation (system 1), i.e., ventral striatum and amygdala.
Similarly, an electroencephalogram (EEG) study focusing on event-related potentials (ERPs), showed that a later (vs. now) mindset reduced cravings for high-caloric foods as well as evoked larger late positive potential (LPP) compared to remaining conditions, suggesting that a cognitive focus on negative long-term consequences increases arousal (Meule et al., 2013).
Bottom-Up Auditory Manipulation
In commercial contexts, consumer researchers and behavioral economists have explored more bottom-up avenues for alleviating the “obesogenic” environment. Such sensory marketing strategies entail changing the so-called choice architecture by nudging consumers toward healthier behaviors through multisensory cues in the environment (Krishna, 2012; Bucher et al., 2016; Seo, 2020). Particularly, auditory contributions to this field have in the past decade emerged with numerous studies highlighting the often underestimated power of sound and noise on food choice (Huang and Labroo, 2019), liking (Alamir and Hansen, 2021), attention (Peng-Li et al., 2020b), and perception (Woods et al., 2011).
Louder (vs. softer) ambient noise has consistently shown adverse effects on psychophysiological mechanisms, including increased arousal states (Alvarsson et al., 2010) and cognitive load (Mehta et al., 2012), potentially resulting in poorer decisions and unhealthier food choices (Biswas et al., 2019; Volz et al., 2021; Peng-Li et al., 2022). These phenomena can be explained through the lenses of attentional processes and sensory overload (Doucé and Adams, 2020), whereby “louder noise may diminish the ability to attend to specific elements of the experience” (Bravo-Moncayo et al., 2020). In fact, attentional distractions have been associated with decreased functional brain connectivity between the inferior frontal gyrus (part of system 2) and the putamen (part of system 1) during goal-directed effort for food rewards (Duif et al., 2020). A complementary mechanism can be reasoned through evidence of sensation transference (Spence and Gallace, 2011), affective priming (Tay and Ng, 2019), or embodied cognition (Zhu and Meyers-Levy, 2005), all in which the ambient sounds physiologically change consumers’ interoceptive, reward, and emotional responses (Salimpoor et al., 2011; Liu et al., 2018; Kantono et al., 2019).
Conceptual Framework
The evidence highlighted thus far conveys that our food cravings are driven by how we internally are able to regulate our valuation and decisions processes (system 1 or system 2), but at the same time, sensory distractions, such as ambient noise, are also influencing our cognitive resources and emotional states necessary for controlling and managing these behaviors. This implies that the underlying mechanisms of food-related decision-making are based on an integration of exteroceptive sensory inputs and interoceptive bodily states (Petit et al., 2016; Papies et al., 2020), that translate our somatic signals into feelings of anticipation, desires, or cravings (Bechara et al., 2005; He et al., 2019).
To understand these different, yet possibly interacting factors, on a behavioral as well as neural level, the employment of implicit psychophysiological measures can be advantageous. One approach to assess this is through EEG. In addition to the measurement of electrophysiological activity response to a specific single time-locked stimulus or event as in ERP research (Shang et al., 2018), longer-lasting and continuous functional indices of neural activity are also possible via EEG (Fernandez Rojas et al., 2020; Firestone et al., 2020; Diao et al., 2021). Here, the EEG signal can be decomposed into various frequency spectra representing the oscillatory dynamics in the brain and correlated with certain mental processes (Barlaam et al., 2011; Diao et al., 2017; Aoh et al., 2019). In fact, the power spectral density (PSD) in specific frequency bands, e.g., theta (4–8), alpha (8–12 Hz), and beta (12–25 Hz), have been associated with various distinct cognitive and emotional states during food viewing (Tashiro et al., 2019; Biehl et al., 2020) and music/noise listening (Gleiss and Kayser, 2014; Chabin et al., 2020).
In the decision and cognitive science literature, both theta and alpha activity in frontal and parietal regions are commonly linked to measures of cognitive load, i.e., the used amount of working memory recourses (Stipacek et al., 2003; Antonenko et al., 2010; Brouwer et al., 2012), including focused attention and sensory processing (Cabañero et al., 2019). Particularly, spectral theta power has been found to increase with sustained concentration and task difficulty (Gevins and Smith, 2003), while alpha oscillatory activity has been associated with alertness (Kamzanova et al., 2014) and cognitive fatigue (Borghini et al., 2012). Likewise, a large body of evidence suggests that augmented PSD in the beta frequency band is related to active and analytical thinking (Zhang et al., 2008) as well as short-term memory (Palva et al., 2011) and mental workload (Coelli et al., 2015). Of course, delta and gamma band power have also been explored in the context of human behavior (Posada-Quintero et al., 2019), yet they are less related to cognitive and mental workload in decision research (Fernandez Rojas et al., 2020).
Instead, frontal lateralization, commonly referred to as frontal asymmetry (FA; Ramsøy et al., 2018), especially in the alpha frequency range, FA has been employed as an index of mental engagement, reward anticipation, and incentive salience and shown to converge with BOLD activity in frontal cortices (Gorka et al., 2015). In particular, greater right (vs. left) frontal hemispheric alpha power is indexed by a positive frontal alpha asymmetry (FAA) score, denoting emotional motivation and approach, whereas a negative FAA score is linked to avoidance and withdrawal behavior (van Bochove et al., 2016; Fischer et al., 2018). Preliminary evidence even suggests that FAA functions as a potential biomarker for affective neuromodulation (Sun et al., 2017). FAA might therefore be a useful measure for studying affective states and cognitive processes in response to multisensory stimuli.
In short, EEG frequency patterns can be an excellent tool and for measuring the underlying brain dynamics of food-related and managerial decision-making processes. Through spectral analyses, it offers an implicit, objective, and nuanced quantification of cognitive load and related emotional processes, which is not restrained by introspection, verbalization, or any other subjective and self-report limitations.
Similarly, measurements based on the sympathetic activity in the peripheral nervous system, including electrodermal activity (EDA), also referred to as galvanic skin response (GSR) can generate complementary biometric information of these affective processes. That is, EDA amplitude amplification, thereby higher EDA peak probability has been used to capture increased emotional arousal states. With increased sympathetic activity due to interoceptive or exteroceptive triggers, sweat production is elevated, leading to heightened/lowered skin conductance/resistance as an indication of elevated arousal (Kytö et al., 2019; Verastegui-Tena et al., 2019; Pedersen et al., 2021), as determined by the circumplex model of affect (Russell, 1980).
In light of the empirical framework, we here investigated the influences of self-regulatory decision strategy and ambient noise level on cue-induced food cravings by means of neurophysiological activity. We adapted an EEG-based ROC task (Kober et al., 2010b; Meule et al., 2013) in which participants should either focus on the long-term consequences or the immediate rewards of eating high-caloric palatable foods while listening to either soft or loud levels of restaurant noise. We hypothesized that both noise level and decision perspective would affect subjective food cravings as well as objective measures, including EDA and EEG, as measures of emotional arousal/motivation and cognitive load (Figure 1). Specifically, we expected, as a result of increased emotional arousal and motivation as well as cognitive load, that loud noise would potentially diminish the cognitive resources requisite for more top-down processing, important for especially thinking about future consequences associated with the food. To test this, we examined the PSD in the theta, alpha, and beta frequency bands in the fronto-cortical areas, FAA, as well as EDA during cognitive regulation in the presence of ambient noise and visual food presentation.
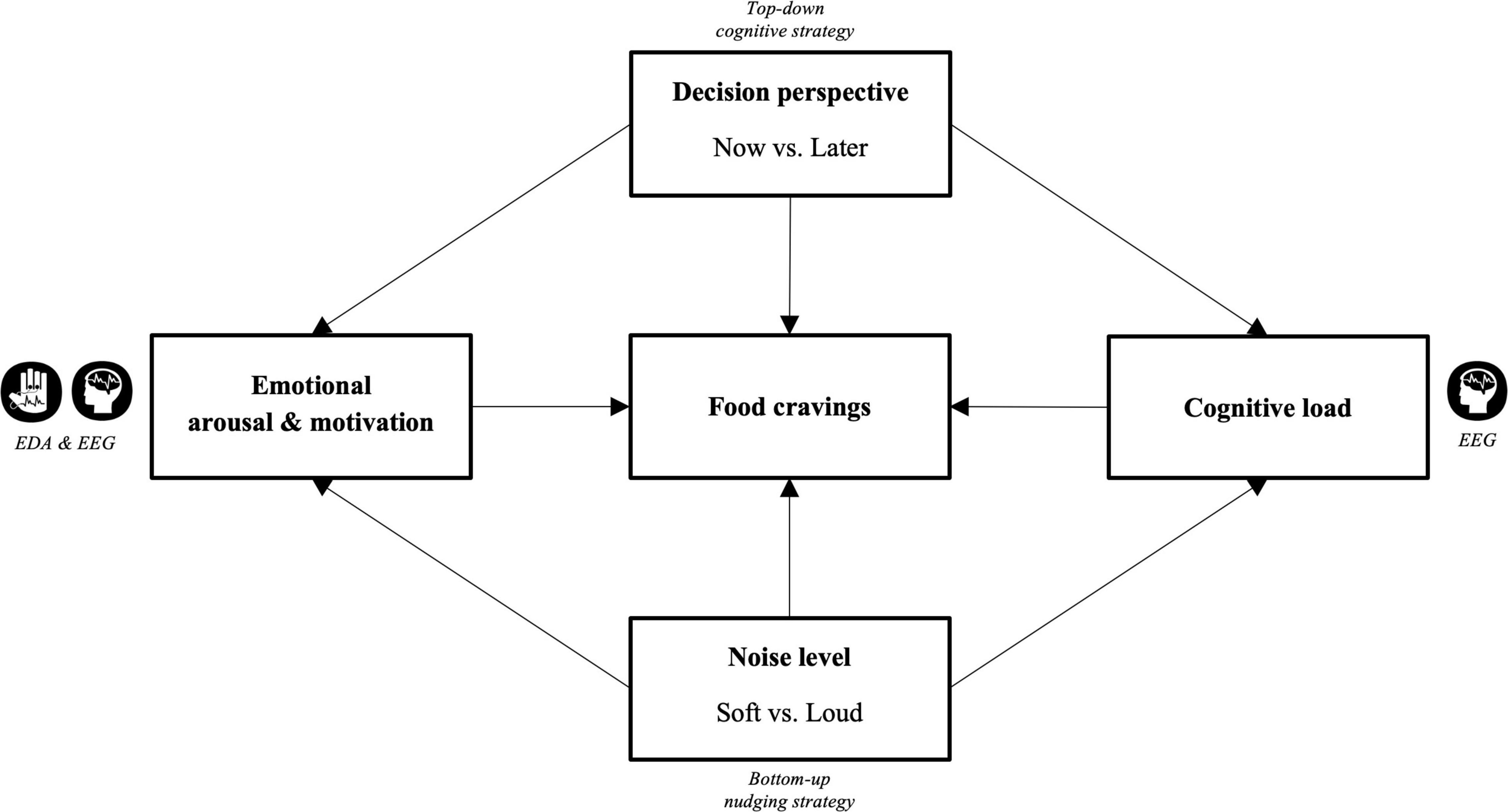
Figure 1. Conceptual framework. Exploring the effects of top-down cognitive strategy (now vs. later decision perspective) and bottom-up nudging strategy (soft vs. loud ambient restaurant noise) on food cravings by means of cognitive load (EEG), emotional motivation (EEG), and emotional arousal (EDA).
Materials and Methods
Participants
Thirty-seven healthy Danish university students aged 18–35 years were recruited through the Sona recruitment system at the Cognition and Behavior (COBE) Lab, Aarhus University, Denmark.1 The choice of sample size was based on previous EEG literature employing similar designs (n = 25; Meule et al., 2013; n = 28; Biehl et al., 2020; n = 19; Tashiro et al., 2019). As this is the first study implementing these conditions/manipulations, we computed a hypothetical power calculation in G*power (Faul et al., 2009). This yielded a required sample size of at least 28 participants at a power of 0.95, effect size of 0.1, and α of 0.05. All participants fulfilled the screening criteria and reported having a normal or corrected-to-normal hearing, normal or corrected-to-normal vision without color blindness, no food allergies, no dietary restraints, and no cardiovascular or neurological diseases. One participant was omitted from the analysis due to unacceptable data quality, resulting in a valid sample size of 36 (mean age ± SD = 24.22 ± 3.59 years; mean BMI ± SD = 23.52 ± 3.90 kg/m2; 50% females) all of whom provided written informed consent. The study was approved by the Aarhus University Ethics Committee (approval number: 2020-0184772) and conducted in accordance with the ethical standards laid out in the Declaration of Helsinki. All participants were compensated monetarily for their participation (250 DKK).
Regulation of Craving Task
The ROC task experimentally measures the specific causal effect of regulation strategies and self-management on craving, as well as allows to study its underlying neural mechanisms. The original ROC used images of cigarettes and unhealthy foods to induce cravings among cigarette smokers (Kober et al., 2010a). In our adapted version, we exclusively focused on high-calorie food items as craving cues. During each trial of the adapted ROC task (Figure 2), participants were exposed to one of these cues, preceded by the instruction to follow one of two decision perspectives: “now”—focus on the immediate sensations and feelings associated with consuming the food (e.g., it will taste good and satisfy my cravings), or “later”—focus on the long-term negative consequences associated with repeated consumption (e.g., it will increase my risk for weight gain and heart disease). Participants were then asked to rate their craving for the specific food they just saw (“how much do you crave this food?”), using a 1 (not at all) to 5 (very much) visual analog scale (VAS). The now or later instructions were presented for 3,000 ms and the subsequent food image for 5,000 ms. Between each trial, a jittered 2,000–2,400 ms fixation cross was inserted. We implemented 60 different trials (30 now-trials and 30 later-trials) per experimental block, which was repeated for each of the two sound conditions (soft noise vs. loud noise), resulting in a total of 120 trials in the experiment. Trials were presented in a randomized order and blocks were counterbalanced across participants. The adapted ROC task was programmed in the iMotions software (Copenhagen, Denmark)2.
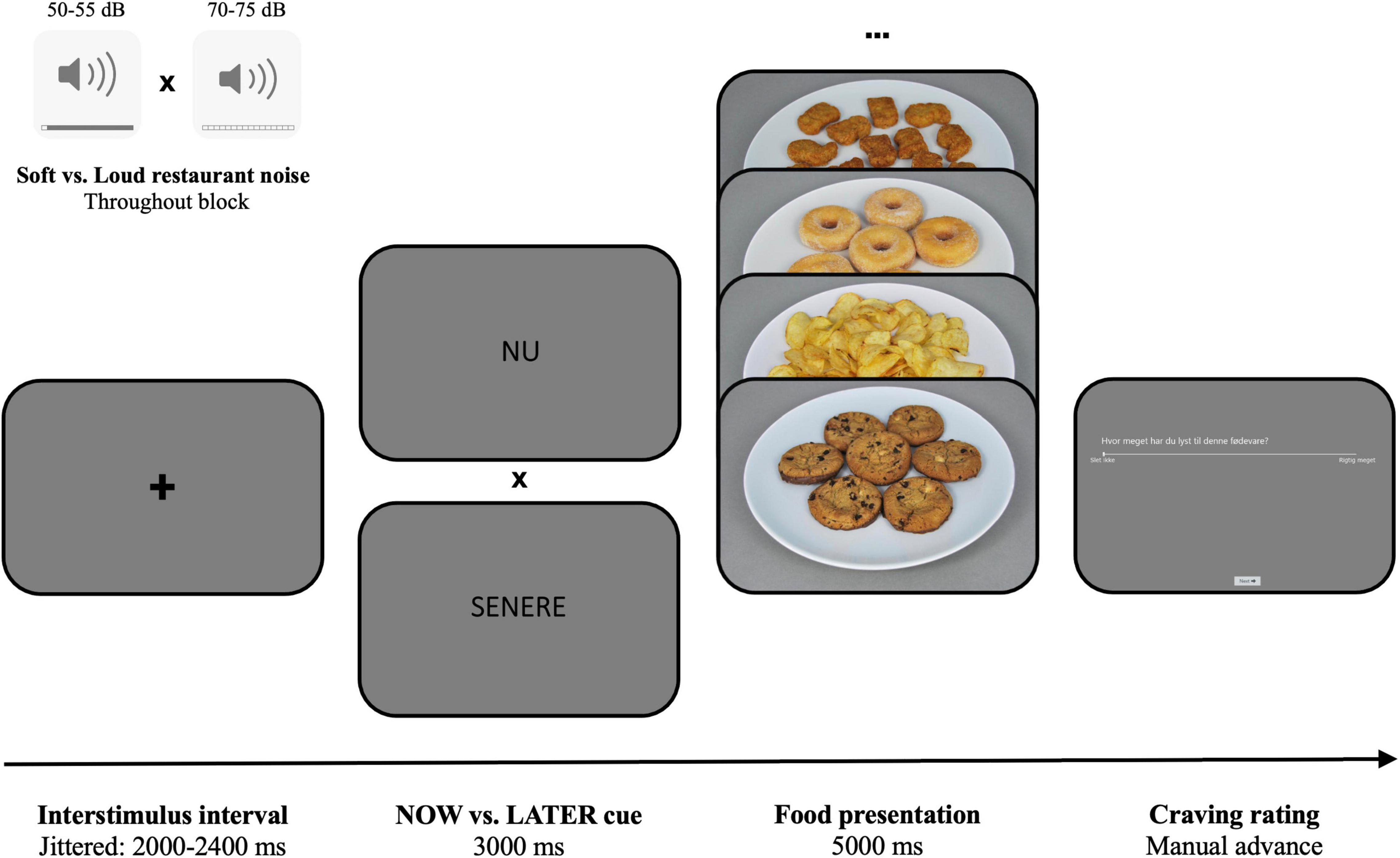
Figure 2. The adapted ROC task. Before each trial, a jittered inter-trial interval (fixation cross) is presented for 2–2.4 s. Then either a now or later cue (nu or senere in Danish) is shown for 3 s, followed by 5 s exposure of a high-calorie food item. Finally, participants rate how much they want the presented food on a VAS from “not at all” to “very much.” Either soft or loud noise is played in the background throughout the entire block.
Self-Regulation of Eating Behavior Questionnaire
The 5-item Self-Regulation of Eating Behavior Questionnaire (SREBQ) is a measure of eating self-regulatory capacity (Kliemann et al., 2016). The SREBQ assesses people’s capacity to control and manage their eating behavior in order to achieve and/or maintain their eating intentions. We adapted the original SREBQ into a Danish version using back-translation. The total score cut-off points include < 2.8 = low self-regulation, 2.8–3.6 = medium self-regulation > 3.6 = high self-regulation.
Visual Stimuli
Thirty high-resolution standardized high-caloric food images from the Full4Health Image Collection (Charbonnier et al., 2016) were selected for the current study (meancalorie = 384 kcal/100 g; meanfat = 21 g/100 g). The images were balanced in terms of taste, such that 15 images were categorized as sweet food items and 15 as savory food items (Table 1). The images were taken in a closed 60 × 60 × 60 cm cubic photo tent. Two daylight lamps (E27/55W) were used to create optimal lighting conditions. The lens angle was approximately 45°, the distance from center plate to center tripod was 39.5 cm, and the height of the center of the camera on the tripod was 38 cm to resemble the viewing of a plate of food on a table during mealtime. Each food was presented on a white plate with a diameter of 17.0 cm. A light gray background was chosen to ensure sufficient contrast between plate and background. To standardize the background, MeVisLab (MeVis Medical Solutions AG, Bremen, Germany) and the open-source registration software Elastix3 were used (Klein et al., 2010). Each plate was segmented, registered on a standardized background from one image, and smoothened on the plate edges. The complete photographing protocol is described in Charbonnier et al. (2016).
Auditory Stimuli
Two versions of a restaurant noisescape (chattering and tableware noises) retrieved from Freesound4 were used for the study. The volume level of the noisescape was manipulated based on the Loudness Unit Full Scale (LUFS) by the European Broadcast Union (EBU) standards (European Broadcast Union, 2016). To attain a soft volume version, the noisescape was decreased to approximately –30 LUFS, while the loud version was increased to approximately –4 LUFS via Logic Pro Version 10.6.1 (Apple Inc.). This was done to ensure the sound intensity (dB) matched 50–55 dB (soft) and 70–75 dB (loud) after audio calibration. The volume levels were chosen based on prior research, which has indicated sound at 80 dB leads to negative affect and even loss of hearing, and sound below 50 dB is often not detected (Witt, 2008). Furthermore, previous food-sound studies have used sound/noise levels in similar ranges (Woods et al., 2011; Biswas et al., 2019). The two noisescapes were first validated in a separate online test (N = 91) in which participants listened to each version and rated them in terms of relaxation/arousal on a VAS from 1 to 9. Soft restaurant noise (mean rating ± SD = 4.27 ± 2.25) was expectably perceived as being more relaxing (vs. arousing) compared to loud restaurant noise (mean rating ± SD = 7.49 ± 1.04). The final noisescapes used for the study can be heard at: https://soundcloud.com/danni-peng-li/sets/eeg-roc-t-sound-study.
Design and Procedure
To control for possible hunger effects, participants were asked to fast for 2 h (i.e., no food intake but water intake was allowed) and refrain from consuming alcoholic drinks for 24 h prior to the study (Frank et al., 2010; Hume et al., 2015; Zhang and Seo, 2015). On testing days (between 9 am to 5 pm), participants arrived at the laboratory for a 1.5 h session where they were informed about the study procedure and provided written informed consent. Participants were seated 70 cm from the HP EliteDisplay E243i, 24,” 16:10 monitor (screen resolution of 1,920 × 1,080 pixels), while EEG and EDA electrodes were applied while checking signal quality in the iMotions software. No natural light entered the room (i.e., only artificial LED light). To reduce movement artifacts participants rested their heads on a chinrest attached to the table. During the paradigm introduction, participants were instructed to minimize head movements throughout the recordings. They also rated how hungry they were on a 9-point VAS. They then completed 4 practice trials to familiarize themselves with the task. After ensuring that participants understood the procedure, they initiated the two counterbalanced experimental blocks (conditions) of the adapted ROC-task—one block with soft ambient restaurant noise and one block with loud ambient restaurant noise—with a 5 min break between blocks and an optional break within each block. The adapted ROC task was followed by a manipulation check, i.e., arousal, valence, and distraction ratings of the noisescapes on a 9-point VAS, as well as completion of the SREBQ. Finally, demographic information was collected.
Signal Processing
EEG data were collected from 32 Ag/AgCl electrodes (Fp1, Fz, F3, F4, FT9, FC5, FC7, C3, T7, TP9, CP5, CP1, Pz, P3, P7, O1, Oz, O2, P4, P9, TP10, CP6, CP2, Cz, C4, T8, FT10, FC6, FC2, F4, F8, Fp2) placed according to the 10–20 system using actiCap (Brain Products GmbH, Gilching, Germany) with a sampling rate of 500 Hz. Raw EEG data were filtered (Butterworth) with a zero phase-lag band-pass filter [0.5–100 Hz] and a zero phase-lag notch filter (50 Hz), re-referenced to the mastoid reference electrode placed at TP9. Artifacts were then rejected using an artifact threshold [120 μV] based on the absolute signal value. Power spectra analysis was computed using Fast Fourier Transform (FFT; Welch method; Welch, 1967), by splitting pre-processed data into 1-s time windows with an overlap of 50% and submitted to the FFT, resulting in one power spectrum per 0.5 s. Finally, theta, alpha, and beta activities were calculated by averaging the power spectral density within the standard power bands: theta [4–8 Hz], alpha [8–12 Hz], and beta [12–25 Hz] (Figure 3B). We focused on a hypothesis-based region of interest (ROI) by clustering the frontal electrodes (Fp1, Fz, F3, F4, FT9, FC5, FC7, FT10, FC6, FC2, F4, F8, Fp2; Figure 3A). This electrode clustering was chosen based on previous literature showing various cognitive processes related to the multiple frontal regions as described in the “Introduction” section as well as to avoid loss in statistical power (Moazami-Goudarzi et al., 2008). Furthermore, FAA scores were computed using two frontal electrodes (F3 and F4) on each hemisphere using the formula according to Allen et al. (2004):
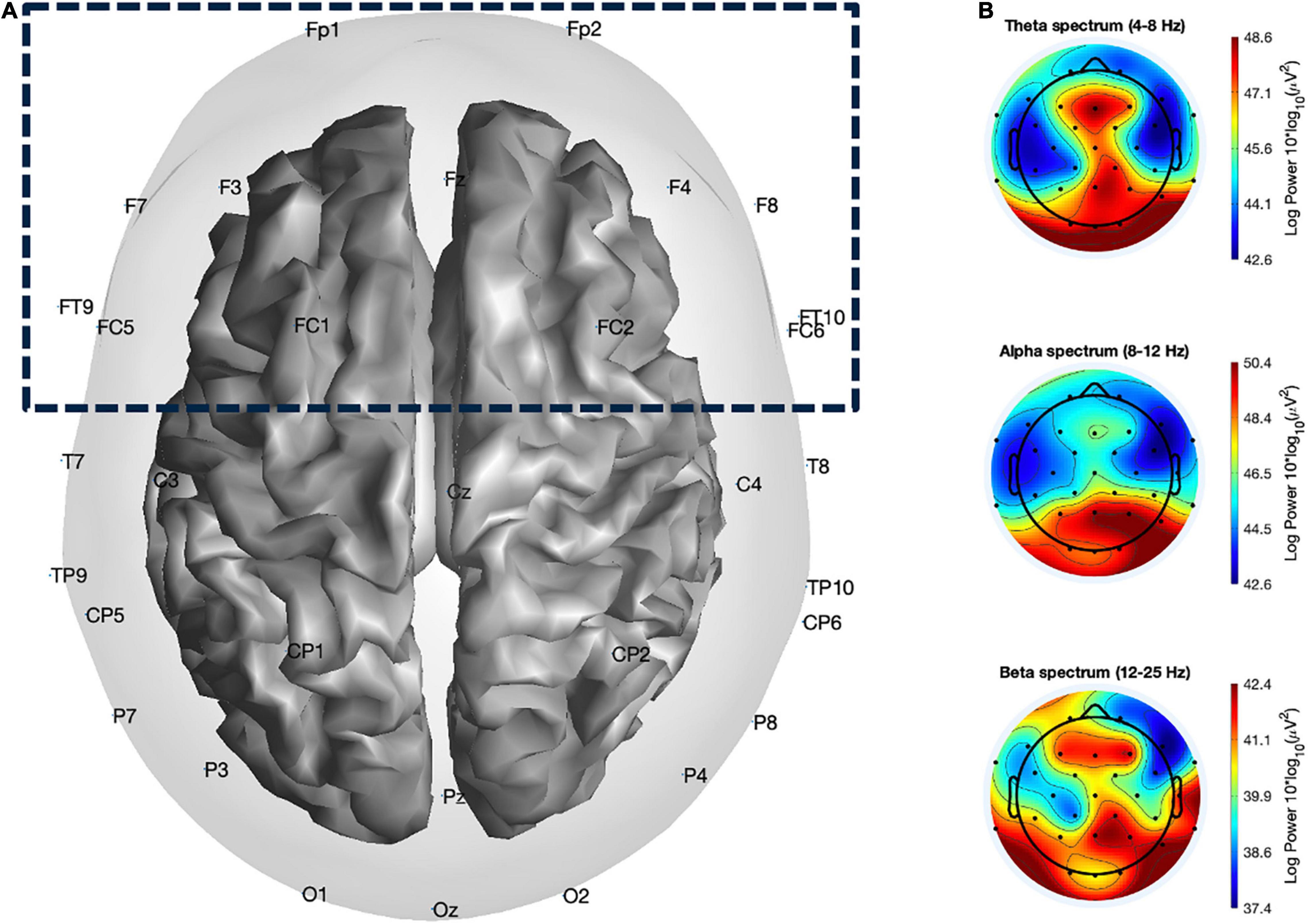
Figure 3. Illustration of (A) channel locations of the 32 electrodes with the frontal ROI highlighted, including Fp1, Fz, F3, F4, FT9, FC5, FC7, FT10, FC6, FC2, F4, F8, Fp2, and (B) example topographical maps of theta (4–8 Hz), alpha (8–12 Hz), and beta (12–25 Hz) power band activity across conditions.
EDA data was collected from two analog electrode channels placed on the tip of the fingers using a Shimmer3 GSR+ (Shimmer Sensing, Dublin, Ireland). The phasic signal was extracted using a median filter over a time window of 8,000 ms, and a low-pass Butterworth filter with a cutoff frequency of 5 Hz was applied to the phasic signal. Peak onset thresholds [0.01 μS] and offset thresholds [0 μS] were then detected on the phasic signal. EDA peak amplitude threshold was set at 0.005 μS with a minimum peak duration of 500 ms. All physiological measures were enclosed to a time window of 5 s, i.e., during food presentation in order to capture audiovisual stimulations of food and noise. Signal processing steps for EEG and EDA were carried out in iMotions through an integrated R algorithm.
Data Analysis
All physiological and behavioral data were imported and analyzed in R version 4.0.2 for Mac OS. A manipulation check was performed using a pairwise t-test based on VAS ratings to ensure that the two soundscapes were in fact perceived differently in terms of arousal (1 = very relaxing; 9 = very arousing), valence (1 = very pleasant; 9 = very unpleasant), and distraction (1 = not distracting at all; 9 = very distracting).
To investigate the effects of ambient noise and cognitive regulation strategy on EEG, EDA, and self-reported cravings, we carried out generalized linear mixed models (GLMMs) via the glmer()-function of the lme4 package. The GLMMs account for the hierarchical structure, non-independence of observations from individual participants in the repeated measure design, and to satisfy the normality assumptions without transformation. EEG and craving data were fitted using a Gaussian distribution with the restricted maximum likelihood (REML) method (Heller et al., 2016), while EDA peak detection was fitted using Poisson distribution (Bolker et al., 2009). In all models, the independent variables were noise level (soft vs. loud) and decision perspective (now vs. later), which were coded as fixed effects. Participant ID entered the model as a random effect. Furthermore, we controlled for possible confounds by adding BMI, hunger status, and SREBQ scores as covariates to the models. However, none of the covariates contributed significantly to any of the models, and as we did not have any a priori hypotheses regarding these factors, they were therefore removed from the analyses [BMItheta: F(1, 34) = 0.39; p = 0.538; BMIalpha: F(1, 34) = 0.26; p = 0.614; BMIbeta: F(1, 34) = 0.21; p = 0.653; Hungertheta: F(1, 34) = 1.45; p = 0.237; Hungeralpha: F(1, 34) = 0.33; p = 0.567; Hungerbeta: F(1, 34) = 3.35; p = 0.076; SREBQtheta: F(1, 34) = 0.11; p = 0.738; SREBQalpha: F(1, 34) = 0.53; p = 0.470; SREBQbeta: F(1, 34) = 0.46; p = 0.502]. The dependent variables of interest included frontal theta power, frontal alpha power, frontal beta power, FAA, EDA peaks, and food craving. Omnibus tests were carried out to test the main effects and interactions between the fixed independent variables. If a significant interaction was indicated by the GLMM, Tukey’s HSD post hoc tests were performed to explore the corrected pairwise comparisons.
Finally, to theorize our conceptual model, we computed four conjoint multiple mediation analyses using the lavaan structural equation modeling package (Rosseel, 2012). Noise level and decision perspective, respectively, entered the models as the binary independent/exogenous variables, craving as the dependent/endogenous variable, and measures of cognitive load (frontal theta power, frontal alpha power, and frontal beta power) as well as emotional arousal (EDA) and emotional motivation (FAA) as the mediators. The multiple mediation analyses were carried out using bootstrapping procedure with the DWLS estimator for 1,000 bootstrapped samples.
Results
Manipulation Check
In terms of arousal, the loud noise (mean rating ± SD = 7.22 ± 1.37) compared to the soft noise (mean rating ± SD = 3.99 ± 1.71) was perceived as being significantly more arousing [vs. relaxing; t(35) = 10.98; p < 0.001]. For valence, the soft noise (mean rating ± SD = 4.03 ± 1.60) compared to the loud noise (mean rating ± SD = 6.88 ± 1.64) was likewise perceived as being significantly more pleasant [vs. unpleasant; t(35) = 8.53; p < 0.001]. Finally, with regard to distraction, the loud noise (mean rating ± SD = 7.57 ± 1.34) compared to the soft noise (mean rating ± SD = 3.73 ± 1.81) was perceived as being significantly more distracting [t(35) = 12.86; p < 0.001].
Behavioral Analysis
The GLMM did not detect any significant interaction, but a main effect of decision perspective was observed with food cravings being reportedly significantly stronger in now (vs. later) -trials [F(1, 4222) = 1,032.92; p < 0.001; Table 2 and Figure 4].
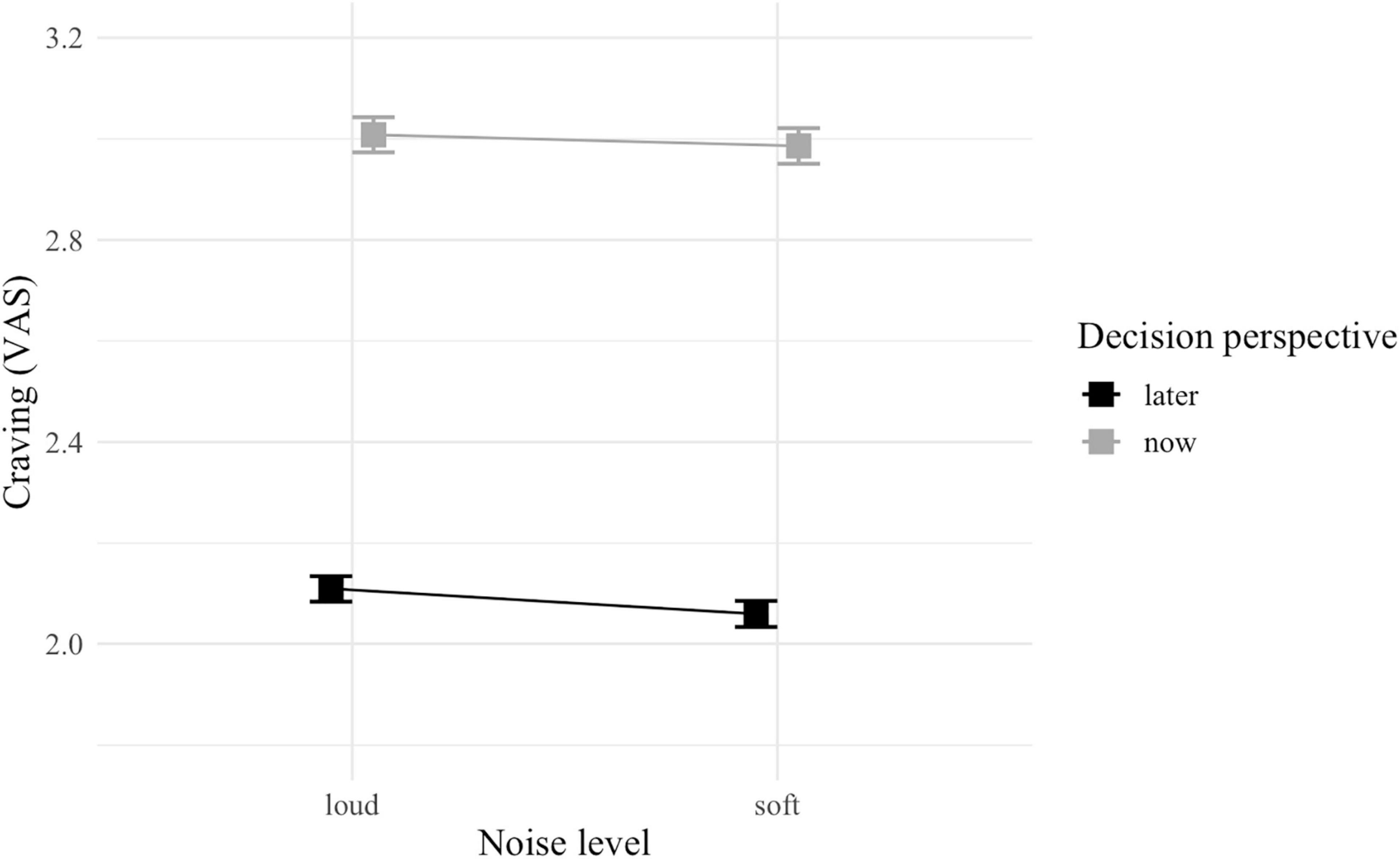
Figure 4. Interaction plot of self-reported cravings between noise level (soft vs. loud) and decision perspective (now vs. later). Error bars represent standard error.
Electroencephalography Power Spectral Analysis
For frontal theta power, the GLMM indicated a significant interaction effect between noise level and decision perspective [F(1, 4222) = 5.49; p = 0.019; Table 3 and Figure 5A]. Post hoc analyses showed that only in the loud noise condition, the theta band power was stronger for later (vs. now) decisions [z(1609) = 2.72; p = 0.033]. The GLMM for frontal alpha power did not detect any significant interaction but, a main effect of both noise level and decision perspective was observed with alpha band power being stronger during the loud noise [F(1, 4222) = 10.59; p = 0.001] and later decision perspective [F(1, 4222) = 16.49; p < 0.001] conditions (Table 3 and Figure 5B). Similarly, the GLMM for frontal beta power did not detect any significant interaction, but a main effect of noise level was observed with beta band power being stronger in the loud noise condition [F(1, 4222) = 12.86; p < 0.001; Table 3 and Figure 5C]. Finally, for FAA, the GLMM did not detect any significant interaction, but a main effect of decision perspective was observed with FAA being higher in the later decision perspective condition [F(1, 4222) = 6.08; p = 0.014; Table 3 and Figure 5D].
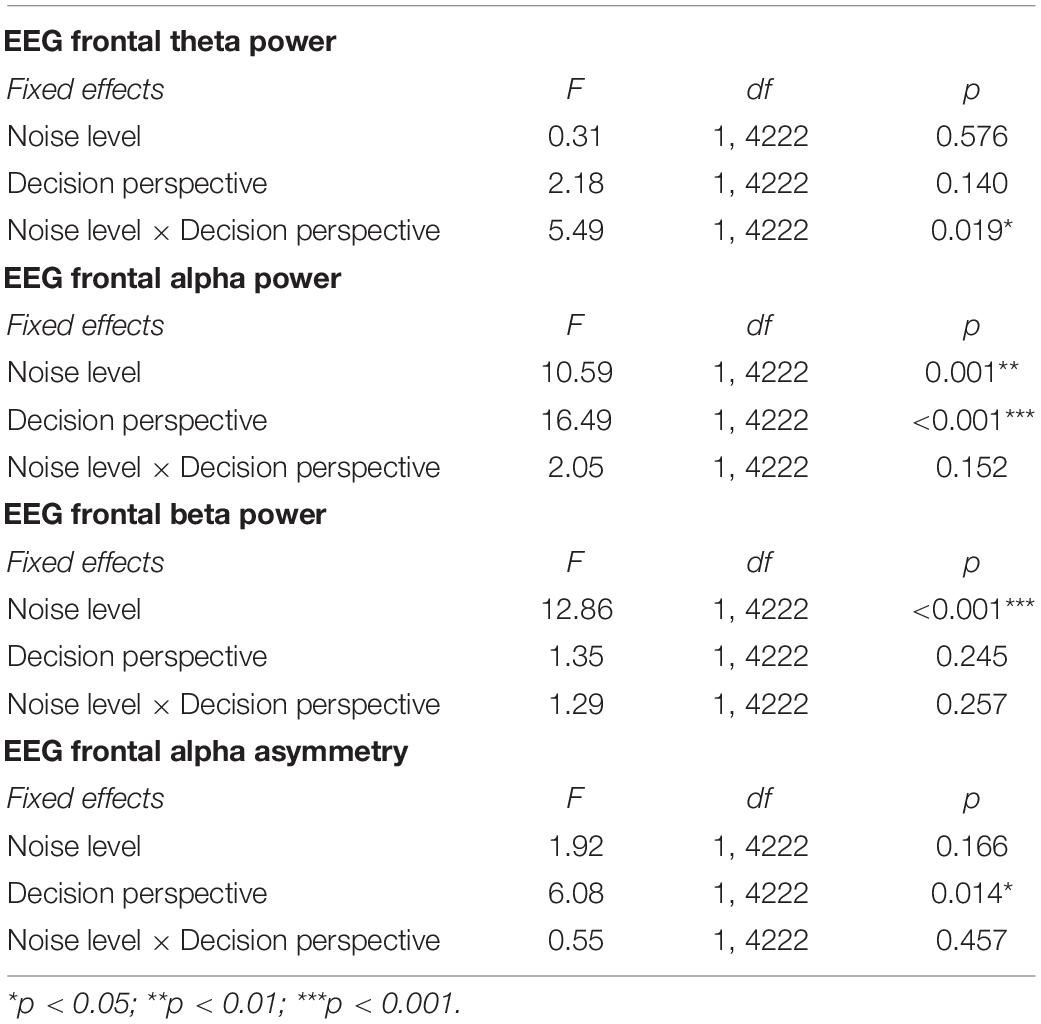
Table 3. Overview of the GLMM omnibus tests for frontal theta power, frontal alpha power, frontal beta power, and frontal alpha asymmetry.
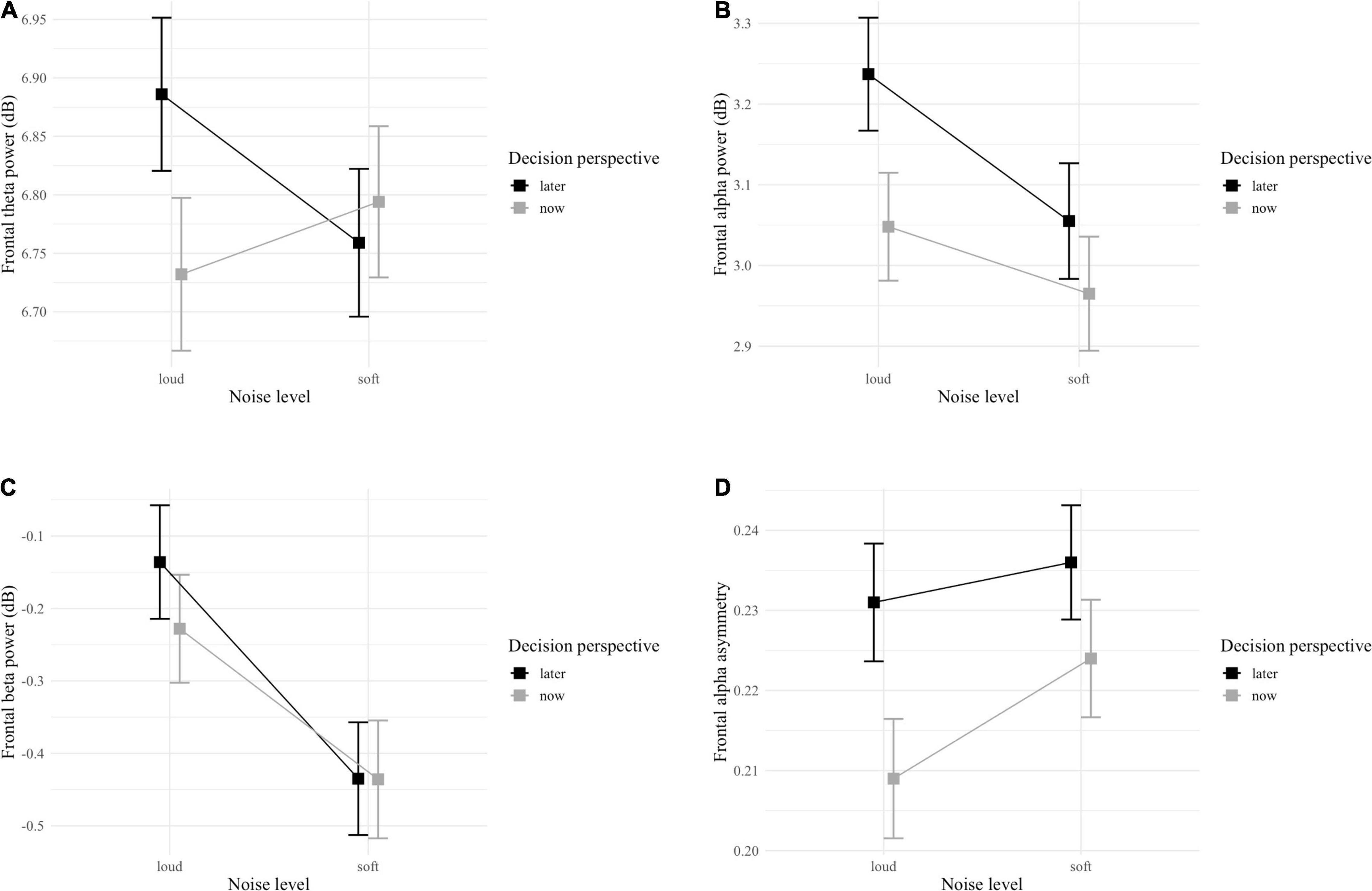
Figure 5. Interaction plots of (A) frontal theta power, (B) frontal alpha power, (C) frontal beta power frontal, and (D) alpha asymmetry between noise level (soft vs. loud) and decision perspective (now vs. later). Error bars represent standard error.
Biometric Analysis
The EDA-based GLMM did not detect any significant interaction, but a main effect of noise level was observed with a higher probability of EDA peak threshold during loud (vs. soft) noise [z(4122) = 3.27; p = 0.001; Table 4 and Figure 6].
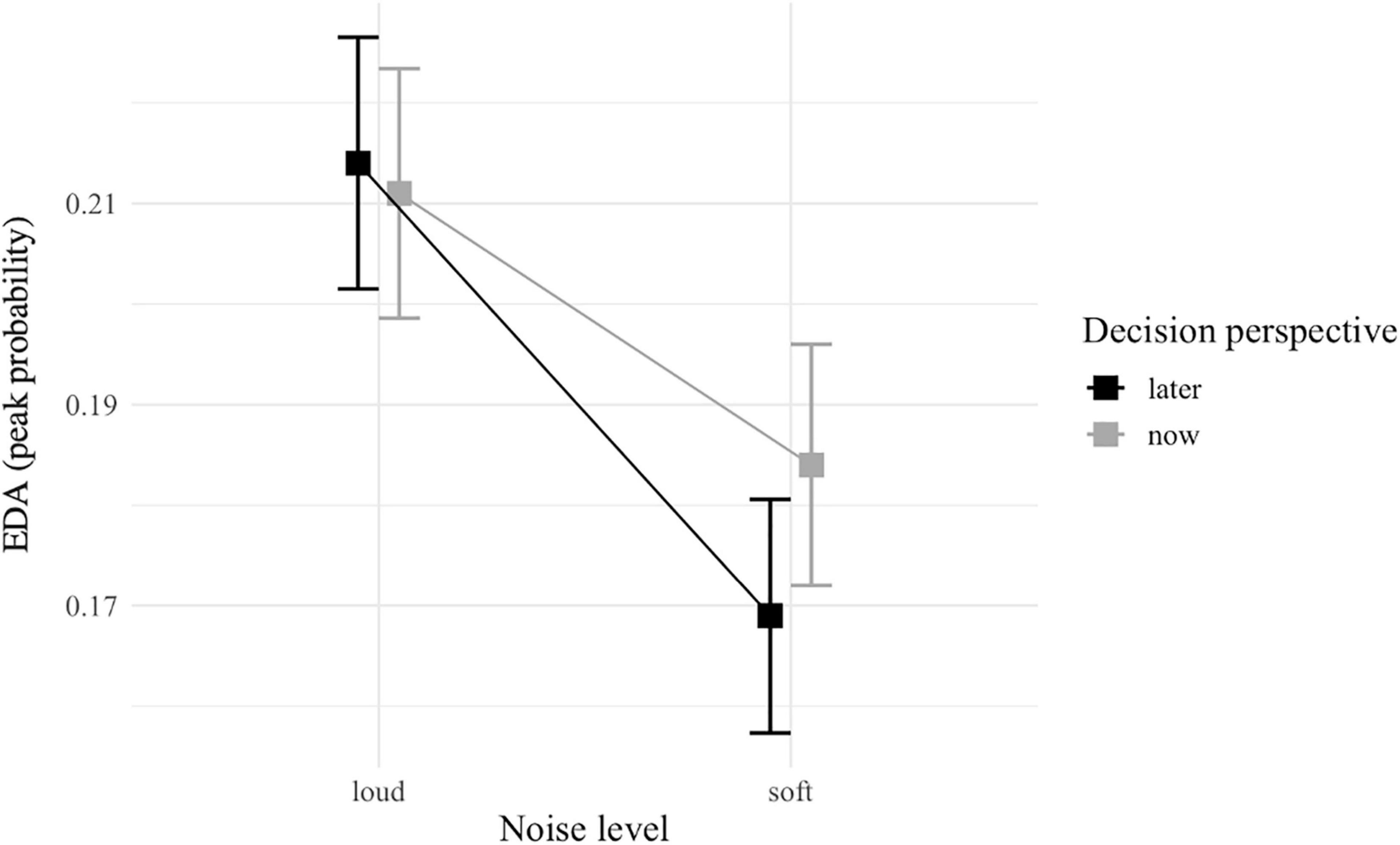
Figure 6. Interaction plot of EDA peaks between noise level (soft vs. loud) and decision perspective (now vs. later). Error bars represent standard error.
Multiple Mediation Analysis
Figure 7 illustrates all of the regression coefficients between independent variables and the mediators as well as the pathways from the mediators onto the dependent variable. With noise level (NL) as the independent variable, the mediation analysis indicated that the standardized indirect effects of neither cognitive load measures (frontal theta power, frontal alpha power, and frontal beta power) nor emotional measures (EDA and FAA) were significant, although frontal alpha power denoted a trend (aNL2*b2; β = 0.01; z = 1.76; p = 0.079). Similarly, the direct effect of noise level on cravings was insignificant (cNL’; β = 0.02; z = –1.34; p = 0.179). With decision perspective (DP) as the independent variable, the mediation analysis signified that the standardized indirect effects of frontal alpha power were significant (aDP2*b2; β = 0.01; z = 1.95; p = 0.050), while the remaining mediators were not. Once this mediator was accounted for, there was still a significant direct effect of decision perspective on cravings (cDP’; β = –0.41; z = 30.69; p < 0.001), suggesting a partial mediation effect of the frontal EEG alpha power on self-reported food cravings.
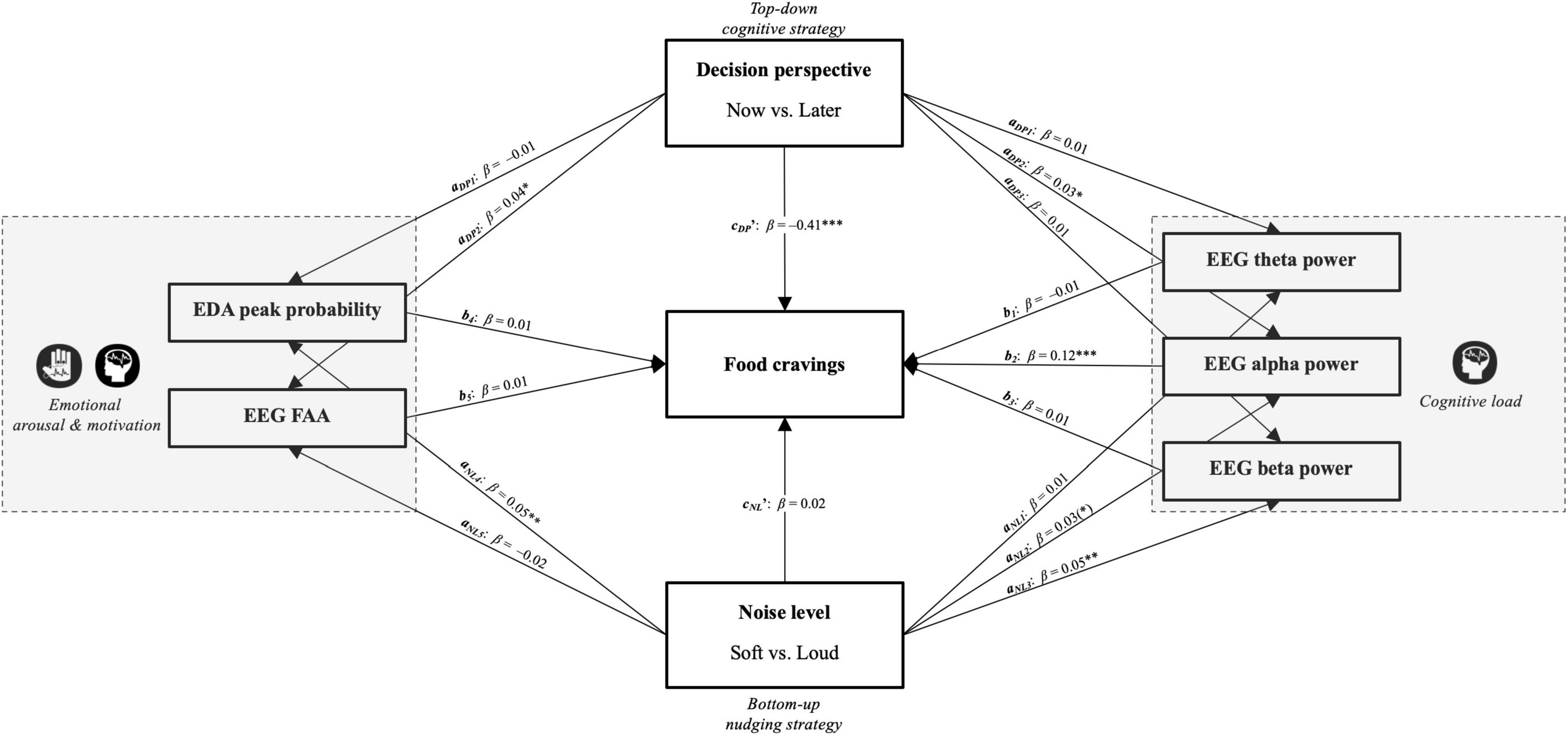
Figure 7. Diagram of the multiple mediation analyses based on our conceptual framework in Figure 1 with noise level (NL) and decision perspective (DP) as the independent variables, cravings as the dependent variable, and frontal theta power, frontal alpha power, frontal beta power and EDA as the mediators. Paths are shown with standardized regression coefficients and p-values (*p < 0.05; **p < 00.01; ***p < 0.001).
Discussion
While a body of psychiatric and neuroscientific research has investigated the impact of top-down cognitive strategies, self-regulation, and managerial decision-making on the neurophysiological underpinnings of food cravings, empirical findings in sensory and consumer science have shown that bottom-up auditory nudging strategies can also influence eating motivation and food valuation. In the current study, we explored both avenues in a single experimental paradigm employing an adapted version of the ROC task.
Our findings do not only provide direct support for our hypothesis that prospectively thinking about long-term consequences can effectively reduce food cravings as demonstrated in Kober et al. (2010a), but simultaneously our results suggest that the underlying causal mechanisms of these self-regulated cravings may at least partially be explained through frontal brain oscillations. That is, the multiple mediation analysis signified a partial mediation effect of decision perspective on self-reported cravings through frontal alpha power. This denotes that in particular augmented activity in the alpha frequency range is associated with increased cravings of high-calorie foods and potentially unhealthy eating behavior. Additionally, irrespectively of behavioral ratings, we found that during delayed (vs. immediate) gratification of food rewards, i.e., in later-trials, the PSD in both the theta and alpha frequency spectra as well as FAA were increased.
This is in line with previous neuroimaging research using the ROC, which has shown increased BOLD activation in frontal regions associated with cognitive control, including the dmPFC, dlPFC, and vlPFC (Kober et al., 2010b). A hyperactivation of these regions might therefore denote cognitive overload (Matsuo et al., 2007). In fact, structural MRI studies have consistently reported reduced gray matter volume in these frontal regions (Horstmann et al., 2011; He et al., 2013) as well as lower structural connectivity between frontal and limbic structures associated with decision-making, reward, and interoceptive awareness (Gupta et al., 2015; Peng-Li et al., 2020c) in individuals with elevated impulsivity and poorer self-regulation abilities. An EEG study by (Meule et al., 2013) also found larger LPP amplitude (350–550 ms after onset)—an ERP component commonly linked to attention capture (Zorjan et al., 2020) and emotion regulation (Hajcak et al., 2010).
Likewise, empirical findings in consumer neuroscience, popularly referred to as neuromarketing, have utilized FA and FAA to objectively quantify consumer behaviors (Bazzani et al., 2020), such as willingness to pay (Ramsøy et al., 2018), hedonic food valuation (van Bochove et al., 2016), and attention biases (McGeown and Davis, 2018). This suggests that FAA cannot only be used as a measure of cognitive engagement but also as an emotional valence marker denoting affective and reward processes, including anticipatory pleasure and incentive salience (“wanting”). Although, one might expect that the FAA ought to be greater during now-trials due to closer reward proximity and delayed discounting, the manifestation of the opposite pattern can be reasoned through higher incentive salience and valuation of health benefits. That is, participants may have considered the future rewards of controlling their consumption of unhealthy foods in the presence. Nevertheless, this evidence, across different neuroimaging modalities and metrics, suggests increased cognitive demand and emotional engagement, especially when actively deliberating on long-term consequences (system 2) rather than simply evaluating immediate rewards in the present (system 1).
Importantly, these psychophysiological processes may be even more intensified during exteroceptive sensory inputs and distractions including ambient noise, as the increased theta activity in the later-trials was only occurring in the presence of loud (vs. soft) ambient noise. Correspondingly, alpha activity was also augmented during the loud noise condition, yet serving as a main (and not interaction) effect. As theta and alpha waves are arguably the power spectra mostly associated with cognitive/work load and attention (Klimesch, 1996; O’Keefe and Burgess, 1999; Stipacek et al., 2003; Antonenko et al., 2010; Brouwer et al., 2012; Wang et al., 2019), a combination of reflective system 2 thinking during prospective thinking and environmental auditory disturbances requires the most cognitive resources.
However, the power of the cerebral oscillations in the higher beta frequency spectra was not affected by decision perspective but solely augmented in the loud noise condition. Salisbury et al. (2002) similarly observed that background noise increased the latency of the P300 component, even while performance was unaffected. In an EEG review, Blume et al. (2019) have highlighted the elevated resting-state beta activity in fronto-central regions in individuals with obesity and binge-eating disorder. The authors argued that this increased beta activity may be the manifestation of the hyper-awareness of food cues and maladaptive eating behavior. Through deductive reasoning and in light of these collateral findings in combination with the results from the present study, it can be inferred that excessively loud noise indeed has neurophysiological impacts. This is measured by means of augmented beta activity, which in turn may provoke adverse effects on food-seeking behavior, even though we did not establish that link between beta activity (only alpha) and behavior (cravings) in the mediation analysis.
In addition, we found that the probability for EDA peak detection was also higher during the exposure to loud noise, indicating elevated arousal state (Salimpoor et al., 2011; Kantono et al., 2019). Louder noise may lead to a more stressful mindset that in turn diminishes the cognitive resources requisite for processing and making more rational and healthy decisions (Caviola et al., 2021). In contrast, when consumers are not interrupted by loud restaurant noises, they are in a more relaxed psychological state, which places them in a better position of restraining and managing their irrational and unhealthy food choices (Peng-Li et al., 2021). In fact, fast tempo and high volume of sound, both of which elevate physiological arousal (Liu et al., 2018; Biswas et al., 2019), have been reported to reduce one’s cognitive abilities, such as decision accuracy (Day et al., 2009), task performance (Nagar and Pandey, 1987), and creative thinking (Mehta et al., 2012).
Altogether, the findings are partly in line with our hypothesis that both noise level and decision perspective would influence subjective food cravings and objective measures, including EDA and EEG. However, we did not observe that the manipulations of both noise level and decision perspective had an impact on all measures. Indeed, alpha activity was affected by both loud noise and prospective thinking and could even predict food cravings based on the mediation analysis. Theta activity was influenced by the interaction of these, i.e., only loud noise and later decision perspective. Yet, beta activity and EDA peak probability were solely determined by noise level, while FAA and food cravings were influenced by decision perspective only. Hence, it can be inferred that louder noise and prospective thinking strategy can at least to some degree elevate neurophysiological constructs of emotional arousal and motivation as well as cognitive load, but will not necessarily help consumers regulate and manage their ultimate subjective food cravings.
Managerial Implications
Due to the interdisciplinary nature and methodological novelty of our study, the results have several translational implications both clinically and commercially. First, we have demonstrated that food cravings could be restrained effectively merely via a single cognitive strategy involving deliberately devaluing the immediate rewards and delaying one’s gratification for future and long-term health benefits. Thus, we build on the previous literature that has incorporated cognitive strategies to highlight the use of interoceptive regulation and managerial decision-making in food (Kober et al., 2010b; Meule et al., 2013; Boswell et al., 2018) and other substance (Kober et al., 2010a; Naqvi et al., 2015; Suzuki et al., 2020) cravings, which collectively reinforces the theoretical foundation for practically implementing these measures in clinical contexts to help individuals who exhibit maladaptive eating behaviors.
Secondly, the identified underlying neurophysiological mechanisms by which top-down self-regulation alleviates cravings, are essential for understanding people’s subconscious and at times suboptimal eating behaviors. In addition, by applying exteroceptive auditory manipulations that analogously affect these fronto-cortical brain oscillations, we emphasize the importance of the power of a well-engineered acoustic environment. Hence, managers and other practitioners, who are at least partly responsible for the consumer, could try to establish eating atmospheres that reinforce healthier eating behavior by reducing stress, arousal and mental load (Doucé and Adams, 2020). Especially, in the times of COVID-19, in which several patients have suffered from anosmia (i.e., loss of smell) and/or ageusia (i.e., loss of taste), focusing on other attributes of the food, such as the texture, could help regaining the hedonic eating experience (Høier et al., 2021). Broaden out, one could also imagine that auditory cues, both intrinsic (i.e., the inherent sound of the food) and extrinsic/contextual (e.g., background music), might sensorily compensate for the loss of olfactory and gustatory perception.
Finally, with the current study being a cross-over between sensory and consumer science and cognitive neuroscience, the framework of the experiment in itself advocates the relevance of robust multidisciplinary research in decision sciences. Particularly, there has been increasing employment of neuroimaging procedures and biometric measurements in (food) market research (Knutson et al., 2007; Plassmann et al., 2008; Clement et al., 2013; Motoki and Suzuki, 2020), and neuromarketing and neuroeconomics have received considerable attention in both the scientific community and the media (Platt and Huettel, 2008; Ariely and Berns, 2010; Plassmann et al., 2012; Zhang et al., 2019). Thus, with the implementation of both EEG and EDA measurements, the study is of commercial and managerial interest. These tools can offer objective quantitative insights beyond traditional subjective and explicit methods that may be constrained by introspection and verbalization. From an industrial management perspective, consistent utilization of such multimodal methods might enable valid forecasting about consumers’ intentions, behaviors, and ultimately purchases. At the same time, it would at least to some degree increase reproducibility and circumvent the consequences of the replication crisis (Chives, 2019). Yet, to optimally exploit this attention and potential, while preventing it from becoming a mere marketing gimmick, academics in the respective fields should exploit their experience and ask relevant questions that can in fact provide useful inputs to marketers and managers in addition to conventional marketing research.
Limitations
Despite these abovementioned implications, our study involves several limitations. First, it should be noted that the physiological signal analyses were based on rather conservative pre-processing procedures due to the employment of the integrated R algorithm of iMotions. This implicates inflexible parameter adjustments during data pre-processing of EEG and EDA. The EEG signal was referenced to a single mastoid instead of e.g., two mastoids or an average reference, but lateralized metrics, such as FAA can be prone to confounds (Lei and Liao, 2017). Analogously, we could not carry out scrutinized eye-blink detection, manual removal of single trials or events, nor independent component analysis (ICA), but only rely on the simple automated algorithm. Notably, according to a methodological review by Allen et al. (2004), for some spectral computations (e.g., FAA), artifact thresholding alone might be as adequate as using other manual accessorial procedures, such as electrooculography (EOG) and electromyography (EMG).
Secondly, due to the nature of our controlled experimental setup, our findings cannot necessarily be directly generalized to naturalistic food choice settings (Andrade, 2018) in which multiple other external factors (including price, labeling, and social factors) may affect the consumers’ emotional states, cognitive processing, and behaviors (Sørensen et al., 2013; Spence et al., 2014; Petit et al., 2015). Besides, albeit food cravings are strong predictors of eating behavior and food choice (Boswell et al., 2018; Chen et al., 2018; Sun and Kober, 2020), we cannot assure that these independent results encompass ecological validity and are applicable in a real-life managerial decision context.
Thirdly, we did not incorporate any neutral/silent condition, which could have strengthened the comparability within the study, as done in some previous food, sound, and decision research (Alamir et al., 2020; Peng-Li et al., 2020a). However, the longer design could have been time-consuming and fatiguing for the participants. Besides, one could argue that the soft noise condition would serve as a control condition since complete silence is highly unlikely in a normal eating situation.
Finally, we simply confined our EEG analyses to the frontal part of the brain through theta, alpha, and beta activity based on our conceptual framework. While, several studies have investigated the oscillatory power in other or smaller ROIs (Tashiro et al., 2019; Biehl et al., 2020) as well as other frequency bands (i.e., delta and gamma; Colrain et al., 2009; Dimigen et al., 2009) during mental operations, we chose not to, as the analyses would be unreasonably extensive and outside the scope of our framework.
Conclusion
To conclude, the present study has underlined the combined effects of cognitive regulation and ambient restaurant noise on food cravings through EDA peak probability as well as fronto-cortical brain oscillations as quantitative measures of emotional arousal, motivation, and cognitive load. More broadly, we have highlighted the prospect of and need for considering both interoceptive states and exteroceptive cues, while employing different physiological measurements to more holistically, objectively, and optimally study food-related decision-making that can provoke an actual societal and managerial impact. This is not solely confined to the field of sensory and consumer neuroscience, but for any decision sciences, this seems applicable and highly pertinent.
Data Availability Statement
The raw data supporting the conclusions of this article will be made available by the authors, without undue reservation.
Ethics Statement
The studies involving human participants were reviewed and approved by the Aarhus University Ethics Committee (approval number: 2020-0184772). The patients/participants provided their written informed consent to participate in this study.
Author Contributions
DP-L: conceptualization, methodology, formal analysis, investigation, resources, data curation, project administration, writing—original draft, writing—review and editing, and visualization. PA: conceptualization and writing—review and editing. CC: investigation and writing—review and editing. RC: writing—review and editing and supervision. DB and QW: conceptualization, writing—review and editing, and supervision. All authors contributed to the article and approved the submitted version.
Funding
The research was supported by the Graduate School of Science and Technology, Aarhus University and the Sino-Danish College, University of Chinese Academy of Sciences (DP-L) Project/funding number: 30367.
Conflict of Interest
The authors declare that the research was conducted in the absence of any commercial or financial relationships that could be construed as a potential conflict of interest.
Publisher’s Note
All claims expressed in this article are solely those of the authors and do not necessarily represent those of their affiliated organizations, or those of the publisher, the editors and the reviewers. Any product that may be evaluated in this article, or claim that may be made by its manufacturer, is not guaranteed or endorsed by the publisher.
Acknowledgments
Data was generated though accessing research infrastructure at AU, including FOODHAY (Food and Health Open Innovation Laboratory, Danish Roadmap for Research Infrastructure). We would furthermore like to thank Kiara Heide and Tue Hvass from the iMotions Client Success Team for facilitating the EEG procedure and Camilla Andersen for helping with the data collection.
Footnotes
- ^ https://aucobe.sona-systems.com/default.aspx?logout=Y
- ^ https://imotions.com
- ^ http://elastix.isi.uu.nl/
- ^ https://freesound.org
References
Alamir, M. A., AlHares, A., Hansen, K. L., and Elamer, A. (2020). The effect of age, gender and noise sensitivity on the liking of food in the presence of background noise. Food Qual. Prefer. 84:103950. doi: 10.1016/j.foodqual.2020.103950
Alamir, M. A., and Hansen, K. (2021). The effect of type and level of background noise on food liking: a laboratory non-focused listening test. Appl. Acoust. 172:107600. doi: 10.1016/j.apacoust.2020.107600
Allen, J. J. B., Coan, J. A., and Nazarian, M. (2004). Issues and assumptions on the road from raw signals to metrics of frontal EEG asymmetry in emotion. Biol. Psychol. 67, 183–218. doi: 10.1016/j.biopsycho.2004.03.007
Alvarsson, J. J., Wiens, S., and Nilsson, M. E. (2010). Stress recovery during exposure to nature sound and environmental noise. Int. J. Environ. Res. Public Health 7, 1036–1046. doi: 10.3390/ijerph7031036
Andrade, C. (2018). Internal, external, and ecological validity in research design, conduct, and evaluation. Indian J. Psychol. Med. 40, 498–499. doi: 10.4103/IJPSYM.IJPSYM_334_18
Antonenko, P., Paas, F., Grabner, R., and van Gog, T. (2010). Using electroencephalography to measure cognitive load. Educ. Psychol. Rev. 22, 425–438. doi: 10.1007/s10648-010-9130-y
Aoh, Y., Hsiao, H.-J., Lu, M.-K., Macerollo, A., Huang, H.-C., Hamada, M., et al. (2019). Event-related desynchronization/synchronization in spinocerebellar ataxia type 3. Front. Neurol. 10:822. doi: 10.3389/fneur.2019.00822
Ariely, D., and Berns, G. S. (2010). Neuromarketing: the hope and hype of neuroimaging in business. Nat. Rev. Neurosci. 11, 284–292. doi: 10.1038/nrn2795
Barlaam, F., Descoins, M., Bertrand, O., Hasbroucq, T., Vidal, F., Assaiante, C., et al. (2011). Time-frequency and ERP analyses of EEG to characterize anticipatory postural adjustments in a bimanual load-lifting task. Front. Hum. Neurosci. 5:163. doi: 10.3389/fnhum.2011.00163
Bazzani, A., Ravaioli, S., Trieste, L., Faraguna, U., and Turchetti, G. (2020). Is EEG suitable for marketing research? A systematic review. Front. Neurosci. 14:594566. doi: 10.3389/fnins.2020.594566
Bechara, A., Damasio, H., Tranel, D., and Damasio, A. R. (2005). The Iowa Gambling Task and the somatic marker hypothesis: some questions and answers. Trends Cogn. Sci. 9, 159–162. doi: 10.1016/j.tics.2005.02.002
Biehl, S. C., Keil, J., Naumann, E., and Svaldi, J. (2020). ERP and oscillatory differences in overweight/obese and normal-weight adolescents in response to food stimuli. J. Eat. Disord. 8, 1–11. doi: 10.1186/s40337-020-00290-8
Biswas, D., Lund, K., and Szocs, C. (2019). Sounds like a healthy retail atmospheric strategy: effects of ambient music and background noise on food sales. J. Acad. Market. Sci. 47, 37–55. doi: 10.1007/s11747-018-0583-8
Blume, M., Schmidt, R., and Hilbert, A. (2019). Abnormalities in the EEG power spectrum in bulimia nervosa, binge-eating disorder, and obesity: a systematic review. Eur. Eat. Disord. Rev. 27, 124–136. doi: 10.1002/erv.2654
Bolker, B. M., Brooks, M. E., Clark, C. J., Geange, S. W., Poulsen, J. R., Stevens, M. H. H., et al. (2009). Generalized linear mixed models: a practical guide for ecology and evolution. Trends Ecol. Evol. 24, 127–135. doi: 10.1016/j.tree.2008.10.008
Borghini, G., Vecchiato, G., Toppi, J., Astolfi, L., Maglione, A., Isabella, R., et al. (2012). “Assessment of mental fatigue during car driving by using high resolution EEG activity and neurophysiologic indices,” in Proceedings of the 2012 Annual International Conference of the IEEE Engineering in Medicine and Biology Society, San Diego, CA, 6442–6445. doi: 10.1109/EMBC.2012.6347469
Boswell, R. G., Sun, W., Suzuki, S., and Kober, H. (2018). Training in cognitive strategies reduces eating and improves food choice. Proc. Natl. Acad. Sci. U.S.A. 115, E11238–E11247. doi: 10.1073/pnas.1717092115
Bravo-Moncayo, L., Reinoso-Carvalho, F., and Velasco, C. (2020). The effects of noise control in coffee tasting experiences. Food Qual. Prefer. 86:104020. doi: 10.1016/j.foodqual.2020.104020
Brouwer, A.-M., Hogervorst, M. A., van Erp, J. B. F., Heffelaar, T., Zimmerman, P. H., and Oostenveld, R. (2012). Estimating workload using EEG spectral power and ERPs in the n-back task. J. Neural Eng. 9:045008. doi: 10.1088/1741-2560/9/4/045008
Bucher, T., Collins, C., Rollo, M. E., McCaffrey, T. A., De Vlieger, N., Van Der Bend, D., et al. (2016). Nudging consumers towards healthier choices: a systematic review of positional influences on food choice. Br. J. Nutr. 115, 2252–2263. doi: 10.1017/S0007114516001653
Burger, K. S., and Stice, E. (2012). Frequent ice cream consumption is associated with reduced striatal response to receipt of an ice cream–based milkshake. Am. J. Clin. Nutr. 95, 810–817. doi: 10.3945/ajcn.111.027003.2
Cabañero, Hervás, R., González, Fontecha, Mondéjar, T., and Bravo, J. (2019). Analysis of cognitive load using EEG when interacting with mobile devices. Proceedings 31:70. doi: 10.3390/proceedings2019031070
Cai, X. l., Weigl, M., Liu, B. H., Cheung, E. F. C., Ding, J. H., and Chan, R. C. K. (2019). Delay discounting and affective priming in individuals with negative schizotypy. Schizophr. Res. 210, 180–187. doi: 10.1016/j.schres.2018.12.040
Caviola, S., Visentin, C., Borella, E., Mammarella, I., and Prodi, N. (2021). Out of the noise: effects of sound environment on maths performance in middle-school students. J. Environ. Psychol. 73:101552. doi: 10.1016/j.jenvp.2021.101552
Chabin, T., Gabriel, D., Chansophonkul, T., Michelant, L., Joucla, C., Haffen, E., et al. (2020). Cortical patterns of pleasurable musical chills revealed by high-density EEG. Front. Neurosci. 14:565815. doi: 10.3389/fnins.2020.565815
Charbonnier, L., van Meer, F., van der Laan, L. N., Viergever, M. A., and Smeets, P. A. M. (2016). Standardized food images: a photographing protocol and image database. Appetite 96, 166–173. doi: 10.1016/j.appet.2015.08.041
Chen, R., Peng-Li, D., Turel, O., Sørensen, T. A., Bechara, A., Li, Y., et al. (2018). Decision making deficits in relation to food cues influence obesity: a triadic neural model of problematic eating. Front. Psychiatry 9:264. doi: 10.3389/fpsyt.2018.00264
Chives, T. (2019). A Theroy in Crisis. Nature. Available online at: https://media.nature.com/original/magazine-assets/d41586-019-03755-2/d41586-019-03755-2.pdf (accessed December 1, 2021).
Clement, J., Kristensen, T., and Grønhaug, K. (2013). Understanding consumers’ in-store visual perception: the influence of package design features on visual attention. J. Retail. Consum. Serv. 20, 234–239. doi: 10.1016/j.jretconser.2013.01.003
Coccurello, R., and Maccarrone, M. (2018). Hedonic eating and the “delicious circle”: from lipid-derived mediators to brain dopamine and back. Front. Neurosci. 12:271. doi: 10.3389/fnins.2018.00271
Coelli, S., Sclocco, R., Barbieri, R., Reni, G., Zucca, C., and Bianchi, A. M. (2015). “EEG-based index for engagement level monitoring during sustained attention,” in Proceedings of the 2015 37th Annual International Conference of the IEEE Engineering in Medicine and Biology Society (EMBC), Milan, 1512–1515. doi: 10.1109/EMBC.2015.7318658
Colrain, I. M., Turlington, S., and Baker, F. C. (2009). Impact of alcoholism on sleep architecture and EEG power spectra in men and women. Sleep 32, 1341–1352. doi: 10.1093/sleep/32.10.1341
Dassen, F. C. M., Jansen, A., Nederkoorn, C., and Houben, K. (2016). Focus on the future: episodic future thinking reduces discount rate and snacking. Appetite 96, 327–332. doi: 10.1016/j.appet.2015.09.032
Day, R.-F., Lin, C.-H., Huang, W.-H., and Chuang, S.-H. (2009). Effects of music tempo and task difficulty on multi-attribute decision-making: an eye-tracking approach. Comput. Hum. Behav. 25, 130–143. doi: 10.1016/j.chb.2008.08.001
Diao, L., Li, W., Zhang, W., Ma, Q., and Jin, J. (2021). Electroencephalographic theta-band oscillatory dynamics represent attentional bias to subjective preferences in value-based decisions. Psychol. Res. Behav. Manag. 14, 149–158. doi: 10.2147/PRBM.S292172
Diao, L., Qi, S., Xu, M., Fan, L., and Yang, D. (2017). Electroencephalographic theta oscillatory dynamics reveal attentional bias to angry faces. Neurosci. Lett. 656, 31–36. doi: 10.1016/j.neulet.2017.06.047
Dimigen, O., Valsecchi, M., Sommer, W., and Kliegl, R. (2009). Human microsaccade-related visual brain responses. J. Neurosci. 29, 12321–12331. doi: 10.1523/JNEUROSCI.0911-09.2009
Doucé, L., and Adams, C. (2020). Sensory overload in a shopping environment: not every sensory modality leads to too much stimulation. J. Retail. Consum. Serv. 57:102154. doi: 10.1016/j.jretconser.2020.102154
Duif, I., Wegman, J., de Graaf, K., Smeets, P. A. M., and Aarts, E. (2020). Distraction decreases rIFG-putamen connectivity during goal-directed effort for food rewards. Sci. Rep. 10, 1–15. doi: 10.1038/s41598-020-76060-y
European Broadcast Union (2016). Guidelines for Production of Programmes in Accordance With EBU R 128. Available online at: https://tech.ebu.ch/docs/tech/tech3343.pdf (accessed December 1, 2021).
Evans, J. S. B. T. (2007). On the resolution of conflict in dual process theories of reasoning. Think. Reason. 13, 321–339. doi: 10.1080/13546780601008825
Faul, F., Erdfelder, E., Buchner, A., and Lang, A.-G. (2009). Statistical power analyses using G*Power 3.1: tests for correlation and regression analyses. Behav. Res. Methods 41, 1149–1160. doi: 10.3758/BRM.41.4.1149
Fernandez Rojas, R., Debie, E., Fidock, J., Barlow, M., Kasmarik, K., Anavatti, S., et al. (2020). Electroencephalographic workload indicators during teleoperation of an unmanned aerial vehicle shepherding a swarm of unmanned ground vehicles in contested environments. Front. Neurosci. 14:40. doi: 10.3389/fnins.2020.00040
Firestone, G. M., McGuire, K., Liang, C., Zhang, N., Blankenship, C. M., Xiang, J., et al. (2020). A preliminary study of the effects of attentive music listening on cochlear implant users’ speech perception, quality of life, and behavioral and objective measures of frequency change detection. Front. Hum. Neurosci. 14:110. doi: 10.3389/fnhum.2020.00110
Fischer, N. L., Peres, R., and Fiorani, M. (2018). Frontal alpha asymmetry and theta oscillations associated with information sharing intention. Front. Behav. Neurosci. 12:166. doi: 10.3389/fnbeh.2018.00166
Frank, S., Laharnar, N., Kullmann, S., Veit, R., Canova, C., Hegner, Y. L., et al. (2010). Processing of food pictures: influence of hunger, gender and calorie content. Brain Res. 1350, 159–166. doi: 10.1016/j.brainres.2010.04.030
Gearhardt, A. N., White, M. A., Masheb, R. M., Morgan, P. T., Crosby, R. D., and Grilo, C. M. (2012). An examination of the food addiction construct in obese patients with binge eating disorder. Int. J. Eat. Disord. 45, 657–663. doi: 10.1002/eat.20957
Gevins, A., and Smith, M. E. (2003). Neurophysiological measures of cognitive workload during human-computer interaction. Theor. Issues Ergon. Sci. 4, 113–131. doi: 10.1080/14639220210159717
Gleiss, S., and Kayser, C. (2014). Acoustic noise improves visual perception and modulates occipital oscillatory states. J. Cogn. Neurosci. 26, 699–711. doi: 10.1162/jocn_a_00524
Gorka, S. M., Phan, K. L., and Shankman, S. A. (2015). Convergence of EEG and fMRI measures of reward anticipation. Biol. Psychol. 112, 12–19. doi: 10.1016/j.biopsycho.2015.09.007
Grilo, C. M., Masheb, R. M., Wilson, G. T., Gueorguieva, R., and White, M. A. (2011). Cognitive–behavioral therapy, behavioral weight loss, and sequential treatment for obese patients with binge-eating disorder: a randomized controlled trial. J. Consult. Clin. Psychol. 79, 675–685. doi: 10.1037/a0025049
Gupta, A., Mayer, E. A., Sanmiguel, C. P., Van Horn, J. D., Woodworth, D., Ellingson, B. M., et al. (2015). Patterns of brain structural connectivity differentiate normal weight from overweight subjects. Neuroimage 7, 506–517. doi: 10.1016/j.nicl.2015.01.005
Hajcak, G., MacNamara, A., and Olvet, D. M. (2010). Event-related potentials, emotion, and emotion regulation: an integrative review. Dev. Neuropsychol. 35, 129–155. doi: 10.1080/87565640903526504
He, Q., Chen, C., Dong, Q., Xue, G., Chen, C., Lu, Z. L., et al. (2013). Gray and white matter structures in the midcingulate cortex region contribute to body mass index in Chinese young adults. Brain Struct. Funct. 220, 319–329. doi: 10.1007/s00429-013-0657-9
He, Q., Huang, X., Zhang, S., Turel, O., Ma, L., and Bechara, A. (2019). Dynamic causal modeling of insular, striatal, and prefrontal cortex activities during a food-specific Go/NoGo task. Biol. Psychiatry 4, 1080–1089. doi: 10.1016/j.bpsc.2018.12.005
Heller, G. Z., Manuguerra, M., and Chow, R. (2016). How to analyze the visual analogue scale: myths, truths and clinical relevance. Scand. J. Pain 13, 67–75. doi: 10.1016/j.sjpain.2016.06.012
Høier, A. T. Z. B., Chaaban, N., and Andersen, B. V. (2021). Possibilities for maintaining appetite in recovering COVID-19 patients. Foods 10:464. doi: 10.3390/foods10020464
Horstmann, A., Busse, F. P., Mathar, D., Müller, K., Lepsien, J., Schlögl, H., et al. (2011). Obesity-related differences between women and men in brain structure and goal-directed behavior. Front. Hum. Neurosci. 5:58. doi: 10.3389/fnhum.2011.00058
Huang, X., and Labroo, A. A. (2019). Cueing morality: the effect of high-pitched music on healthy choice. J. Market. 84:002224291881357. doi: 10.1177/0022242918813577
Hume, D. J., Howells, F. M., Rauch, H. G. L., Kroff, J., and Lambert, E. V. (2015). Electrophysiological indices of visual food cue-reactivity. Differences in obese, overweight and normal weight women. Appetite 85, 126–137. doi: 10.1016/j.appet.2014.11.012
Kamzanova, A. T., Kustubayeva, A. M., and Matthews, G. (2014). Use of EEG workload indices for diagnostic monitoring of vigilance decrement. Hum. Fact. 56, 1136–1149. doi: 10.1177/0018720814526617
Kantono, K., Hamid, N., Shepherd, D., Lin, Y. H. T., Skiredj, S., and Carr, B. T. (2019). Emotional and electrophysiological measures correlate to flavour perception in the presence of music. Physiol. Behav. 199, 154–164. doi: 10.1016/j.physbeh.2018.11.012
Klein, S., Staring, M., Murphy, K., Viergever, M. A., and Pluim, J. (2010). elastix: a toolbox for intensity-based medical image registration. IEEE Trans. Med. Imag. 29, 196–205. doi: 10.1109/TMI.2009.2035616
Kliemann, N., Beeken, R. J., Wardle, J., and Johnson, F. (2016). Development and validation of the self-regulation of eating behaviour questionnaire for adults. Int. J. Behav. Nutr. Phys. Act. 13, 1–11. doi: 10.1186/s12966-016-0414-6
Klimesch, W. (1996). Memory processes, brain oscillations and EEG synchronization. Int. J. Psychophysiol. 24, 61–100. doi: 10.1016/S0167-8760(96)00057-8
Knutson, B., Rick, S., Wimmer, G. E., Prelec, D., and Loewenstein, G. (2007). Neural predictors of purchases. Neuron 53, 147–156. doi: 10.1016/j.neuron.2006.11.010
Kober, H., Kross, E. F., Mischel, W., Hart, C. L., and Ochsner, K. N. (2010a). Regulation of craving by cognitive strategies in cigarette smokers. Drug Alcoh. Depend. 106, 52–55. doi: 10.1016/j.drugalcdep.2009.07.017
Kober, H., Mende-Siedlecki, P., Kross, E. F., Weber, J., Mischel, W., Hart, C. L., et al. (2010b). Prefrontal-striatal pathway underlies cognitive regulation of craving. Proc. Natl. Acad. Sci. U.S.A. 107, 14811–14816. doi: 10.1073/pnas.1007779107
Krishna, A. (2012). An integrative review of sensory marketing: engaging the senses to affect perception, judgment and behavior. J. Consum. Psychol. 22, 332–351. doi: 10.1016/j.jcps.2011.08.003
Kytö, E., Bult, H., Aarts, E., Wegman, J., Ruijschop, R. M. A. J., and Mustonen, S. (2019). Comparison of explicit vs. implicit measurements in predicting food purchases. Food Qual. Prefer. 78:103733. doi: 10.1016/j.foodqual.2019.103733
Lei, X., and Liao, K. (2017). Understanding the influences of EEG reference: a large-scale brain network perspective. Front. Neurosci. 11:205. doi: 10.3389/fnins.2017.00205
Levin, I. P., Xue, G., Weller, J. A., Reimann, M., Lauriola, M., and Bechara, A. (2012). A neuropsychological approach to understanding risk-taking for potential gains and losses. Front. Neurosci. 6:15. doi: 10.3389/fnins.2012.00015
Liu, Y., Liu, G., Wei, D., Li, Q., Yuan, G., Wu, S., et al. (2018). Effects of musical tempo on musicians’ and non-musicians’ emotional experience when listening to music. Front. Psychol. 9:2118. doi: 10.3389/fpsyg.2018.02118
Matsuo, K., Glahn, D. C., Peluso, M. A. M., Hatch, J. P., Monkul, E. S., Najt, P., et al. (2007). Prefrontal hyperactivation during working memory task in untreated individuals with major depressive disorder. Mol. Psychiatry 12, 158–166. doi: 10.1038/sj.mp.4001894
McGeown, L., and Davis, R. (2018). Frontal EEG asymmetry moderates the association between attentional bias towards food and body mass index. Biol. Psychol. 136, 151–160. doi: 10.1016/j.biopsycho.2018.06.001
Mehta, R., Zhu, R., and Cheema, A. (2012). Is noise always bad? Exploring the effects of ambient noise on creative cognition. J. Consum. Res. 39, 784–799. doi: 10.1086/665048
Meule, A., Kübler, A., and Blechert, J. (2013). Time course of electrocortical food-cue responses during cognitive regulation of craving. Front. Psychol. 4:669. doi: 10.3389/fpsyg.2013.00669
Moazami-Goudarzi, M., Sarnthein, J., Michels, L., Moukhtieva, R., and Jeanmonod, D. (2008). Enhanced frontal low and high frequency power and synchronization in the resting EEG of parkinsonian patients. Neuroimage 41, 985–997. doi: 10.1016/j.neuroimage.2008.03.032
Motoki, K., Sugiura, M., and Kawashima, R. (2019). Common neural value representations of hedonic and utilitarian products in the ventral striatum: an fMRI study. Sci. Rep. 9:15630. doi: 10.1038/s41598-019-52159-9
Motoki, K., and Suzuki, S. (2020). Extrinsic factors underlying food valuation in the human brain. Front. Behav. Neurosci. 14:131. doi: 10.3389/fnbeh.2020.00131
Nagar, D., and Pandey, J. (1987). Affect and performance on cognitive task as a function of crowding and noise1. J. Appl. Soc. Psychol. 17, 147–157. doi: 10.1111/j.1559-1816.1987.tb00306.x
Naqvi, N. H., Ochsner, K. N., Kober, H., Kuerbis, A., Feng, T., Wall, M., et al. (2015). Cognitive regulation of craving in alcohol-dependent and social drinkers. Alcoholism 39, 343–349. doi: 10.1111/acer.12637
Nguyen, D., Naffziger, E. E., and Berridge, K. C. (2021). Positive affect: nature and brain bases of liking and wanting. Curr. Opin. Behav. Sci. 39, 72–78. doi: 10.1016/j.cobeha.2021.02.013
O’Keefe, J., and Burgess, N. (1999). Theta activity, virtual navigation and the human hippocampus. Trends Cogn. Sci. 3, 403–406. doi: 10.1016/S1364-6613(99)01396-0
Palva, S., Kulashekhar, S., Hamalainen, M., and Palva, J. M. (2011). Localization of cortical phase and amplitude dynamics during visual working memory encoding and retention. J. Neurosci. 31, 5013–5025. doi: 10.1523/JNEUROSCI.5592-10.2011
Papies, E. K., Barsalou, L. W., and Rusz, D. (2020). Understanding desire for food and drink: a grounded-cognition approach. Curr. Dir. Psychol. Sci. 29, 193–198. doi: 10.1177/0963721420904958
Pedersen, H., Quist, J. S., Jensen, M. M., Clemmensen, K. K. B., Vistisen, D., Jørgensen, M. E., et al. (2021). Investigation of eye tracking, electrodermal activity and facial expressions as biometric signatures of food reward and intake in normal weight adults. Food Qual. Prefer. 93:104248. doi: 10.1016/j.foodqual.2021.104248
Peng-Li, D., Andersen, T., Finlayson, G., Byrne, D. V., and Wang, Q. J. (2022). The impact of environmental sounds on food reward. Physiol. Behav. 245:113689. doi: 10.1016/j.physbeh.2021.113689
Peng-Li, D., Byrne, D. V., Chan, R. C. K., and Wang, Q. J. (2020a). The influence of taste-congruent soundtracks on visual attention and food choice: a cross-cultural eye-tracking study in Chinese and Danish consumers. Food Qual. Prefer. 85:103962. doi: 10.1016/j.foodqual.2020.103962
Peng-Li, D., Chan, R. C. K., Byrne, D. V., and Wang, Q. J. (2020b). The effects of ethnically congruent music on eye movements and food choice—a cross-cultural comparison between Danish and Chinese consumers. Foods 9:1109. doi: 10.3390/foods9081109
Peng-Li, D., Sørensen, T. A., Li, Y., and He, Q. (2020c). Systematically lower structural brain connectivity in individuals with elevated food addiction symptoms. Appetite 155:104850. doi: 10.1016/j.appet.2020.104850
Peng-Li, D., Mathiesen, S. L., Chan, R. C. K., Byrne, D. V., and Wang, Q. J. (2021). Sounds healthy: modelling sound-evoked consumer food choice through visual attention. Appetite 164:105264. doi: 10.1016/j.appet.2021.105264
Petit, O., Basso, F., Merunka, D., Spence, C., Cheok, A. D., and Oullier, O. (2016). Pleasure and the control of food intake: an embodied cognition approach to consumer self-regulation. Psychol. Market. 33, 608–619. doi: 10.1002/mar.20903
Petit, O., Spence, C., Velasco, C., Woods, A. T., and Cheok, A. D. (2017). Changing the influence of portion size on consumer behavior via imagined consumption. J. Bus. Res. 75, 240–248. doi: 10.1016/j.jbusres.2016.07.021
Petit, O., Velasco, C., Cheok, A. D., and Spence, C. (2015). “Consumer sensory neuroscience in the context of food marketing,” in Proceedings of the ACM International Conference Proceeding Series, 3–6. (New York, NY: Association for Computing Machinery). doi: 10.1145/2832932.2856226
Plassmann, H., O’Doherty, J., Shiv, B., and Rangel, A. (2008). Marketing actions can modulate neural representations of experienced pleasantness. Proc. Natl. Acad. Sci. U.S.A. 105, 1050–1054. doi: 10.1073/pnas.0706929105
Plassmann, H., Ramsøy, T. Z., and Milosavljevic, M. (2012). Branding the brain: a critical review and outlook. J. Consum. Psychol. 22, 18–36. doi: 10.1016/j.jcps.2011.11.010
Platt, M. L., and Huettel, S. A. (2008). Risky business: the neuroeconomics of decision making under uncertainty. Nat. Neurosci. 11, 398–403. doi: 10.1038/nn2062
Posada-Quintero, H. F., Reljin, N., Bolkhovsky, J. B., Orjuela-Cañón, A. D., and Chon, K. H. (2019). Brain activity correlates with cognitive performance deterioration during sleep deprivation. Front. Neurosci. 13:1001. doi: 10.3389/fnins.2019.01001
Ramsøy, T. Z., Skov, M., Christensen, M. K., and Stahlhut, C. (2018). Frontal brain asymmetry and willingness to pay. Front. Neurosci. 12:138. doi: 10.3389/fnins.2018.00138
Rangel, A., Camerer, C., and Montague, P. R. (2008). A framework for studying the neurobiology of value-based decision making. Nat. Rev. Neurosci. 9, 545–556. doi: 10.1038/nrn2357
Rosseel, Y. (2012). lavaan: an R package for structural equation modeling. J. Stat. Softw. 48, 1–36. doi: 10.18637/jss.v048.i02
Russell, J. A. (1980). A circumplex model of affect. J. Pers. Soc. Psychol. 39, 1161–1178. doi: 10.1037/h0077714
Salimpoor, V. N., Benovoy, M., Larcher, K., Dagher, A., and Zatorre, R. J. (2011). Anatomically distinct dopamine release during anticipation and experience of peak emotion to music. Nat. Neurosci. 14, 257–262. doi: 10.1038/nn.2726
Salisbury, D. F., Desantis, M. A., Shenton, M. E., and McCarley, R. W. (2002). The effect of background noise on P300 to suprathreshold stimuli. Psychophysiology 39, 111–115. doi: 10.1111/1469-8986.3910111
Schulte, E. M., Grilo, C. M., and Gearhardt, A. N. (2016). Shared and unique mechanisms underlying binge eating disorder and addictive disorders. Clin. Psychol. Rev. 44, 125–139. doi: 10.1016/j.cpr.2016.02.001
Seo, H.-S. (2020). Sensory nudges: the influences of environmental contexts on consumers’ sensory perception, emotional responses, and behaviors toward foods and beverages. Foods 9:509. doi: 10.3390/foods9040509
Shang, Q., Pei, G., Jin, J., Zhang, W., Wang, Y., and Wang, X. (2018). ERP evidence for consumer evaluation of copycat brands. PLoS One 13:e0191475. doi: 10.1371/journal.pone.0191475
Sørensen, H. S., Holm, L., Møgelvang-Hansen, P., Barratt, D., Qvistgaard, F., and Smith, V. (2013). Consumer understanding of food labels: toward a generic tool for identifying the average consumer. Int. Rev. Retail Distrib. Consum. Res. 23, 291–304. doi: 10.1080/09593969.2013.764339
Spence, C., and Gallace, A. (2011). Multisensory design: reaching out to touch the consumer. Psychol. Market. 28, 267–308. doi: 10.1002/mar.20392
Spence, C., Puccinelli, N. M., Grewal, D., and Roggeveen, A. L. (2014). Store atmospherics: a multisensory perspective. Psychol. Market. 31, 472–488. doi: 10.1002/mar.20709
Stipacek, A., Grabner, R. H., Neuper, C., Fink, A., and Neubauer, A. C. (2003). Sensitivity of human EEG alpha band desynchronization to different working memory components and increasing levels of memory load. Neurosci. Lett. 353, 193–196. doi: 10.1016/j.neulet.2003.09.044
Sun, L., Peräkylä, J., and Hartikainen, K. M. (2017). Frontal Alpha asymmetry, a potential biomarker for the effect of neuromodulation on brain’s affective circuitry—preliminary evidence from a deep brain stimulation study. Front. Hum. Neurosci. 11:584. doi: 10.3389/fnhum.2017.00584
Sun, W., and Kober, H. (2020). Regulating food craving: from mechanisms to interventions. Physiol. Behav. 222:112878. doi: 10.1016/j.physbeh.2020.112878
Suzuki, S., Mell, M. M., O’Malley, S. S., Krystal, J. H., Anticevic, A., and Kober, H. (2020). Regulation of craving and negative emotion in alcohol use disorder. Biol. Psychiatry 5, 239–250. doi: 10.1016/j.bpsc.2019.10.005
Tashiro, N., Sugata, H., Ikeda, T., Matsushita, K., Hara, M., Kawakami, K., et al. (2019). Effect of individual food preferences on oscillatory brain activity. Brain Behav. 9, 1–7. doi: 10.1002/brb3.1262
Tay, R. Y. L., and Ng, B. C. (2019). Effects of affective priming through music on the use of emotion words. PLoS ONE 14, 1–26. doi: 10.1371/journal.pone.0214482
van Bochove, M. E., Ketel, E., Wischnewski, M., Wegman, J., Aarts, E., de Jonge, B., et al. (2016). Posterior resting state EEG asymmetries are associated with hedonic valuation of food. Int. J. Psychophysiol. 110, 40–46. doi: 10.1016/j.ijpsycho.2016.10.006
Verastegui-Tena, L., van Trijp, H., and Piqueras-Fiszman, B. (2019). Heart rate, skin conductance, and explicit responses to juice samples with varying levels of expectation (dis)confirmation. Food Qual. Prefer. 71, 320–331. doi: 10.1016/j.foodqual.2018.08.011
Volkow, N. D., and Baler, R. D. (2015). NOW vs LATER brain circuits: implications for obesity and addiction. Trends Neurosci. 38, 345–352. doi: 10.1016/j.tins.2015.04.002
Volz, S., Ward, A., and Mann, T. (2021). Eating up cognitive resources: does attentional consumption lead to food consumption? Appetite 162:105165. doi: 10.1016/j.appet.2021.105165
Wang, X., Duan, H., Kan, Y., Wang, B., Qi, S., and Hu, W. (2019). The creative thinking cognitive process influenced by acute stress in humans: an electroencephalography study. Stress 22, 472–481. doi: 10.1080/10253890.2019.1604665
Welch, P. (1967). The use of fast Fourier transform for the estimation of power spectra: a method based on time averaging over short, modified periodograms. IEEE Trans. Audio Electroacoust. 15, 70–73. doi: 10.1109/TAU.1967.1161901
Witt, C. L. (2008). Turn down the noise. Adv. Neonat. Care 8, 137–138. doi: 10.1097/01.ANC.0000324331.63851.46
Woods, A. T., Poliakoff, E., Lloyd, D. M., Kuenzel, J., Hodson, R., Gonda, H., et al. (2011). Effect of background noise on food perception. Food Qual. Prefer. 22, 42–47. doi: 10.1016/j.foodqual.2010.07.003
Zhang, B., and Seo, H.-S. (2015). Visual attention toward food-item images can vary as a function of background saliency and culture: an eye-tracking study. Food Qual. Prefer. 41, 172–179. doi: 10.1016/j.foodqual.2014.12.004
Zhang, W., Jin, J., Wang, A., Ma, Q., and Yu, H. (2019). Consumers’ implicit motivation of purchasing luxury brands: an EEG study. Psychol. Res. Behav. Manag. 12, 913–929. doi: 10.2147/PRBM.S215751
Zhang, Y., Chen, Y., Bressler, S. L., and Ding, M. (2008). Response preparation and inhibition: the role of the cortical sensorimotor beta rhythm. Neuroscience 156, 238–246. doi: 10.1016/j.neuroscience.2008.06.061
Zhu, R. Meyers-Levy, J. (2005). Distinguishing between the Meanings of Music: when background music affects product perceptions. J. Market. Res. 42, 333–345. doi: 10.1509/jmkr.2005.42.3.333
Keywords: EEG, EDA, cognitive load, emotions, self-regulation, restaurant noise, decision-making, consumer behavior
Citation: Peng-Li D, Alves Da Mota P, Correa CMC, Chan RCK, Byrne DV and Wang QJ (2022) “Sound” Decisions: The Combined Role of Ambient Noise and Cognitive Regulation on the Neurophysiology of Food Cravings. Front. Neurosci. 16:827021. doi: 10.3389/fnins.2022.827021
Received: 01 December 2021; Accepted: 17 January 2022;
Published: 16 February 2022.
Edited by:
Wuke Zhang, Ningbo University, ChinaReviewed by:
Yijie Lai, Shanghai Jiao Tong University, ChinaMark Selikowitz, Sydney Developmental Clinic, Australia
Debo Dong, University of Electronic Science and Technology of China, China
Copyright © 2022 Peng-Li, Alves Da Mota, Correa, Chan, Byrne and Wang. This is an open-access article distributed under the terms of the Creative Commons Attribution License (CC BY). The use, distribution or reproduction in other forums is permitted, provided the original author(s) and the copyright owner(s) are credited and that the original publication in this journal is cited, in accordance with accepted academic practice. No use, distribution or reproduction is permitted which does not comply with these terms.
*Correspondence: Danni Peng-Li, ZGFubmlwZW5nbGlAb3V0bG9vay5jb20=