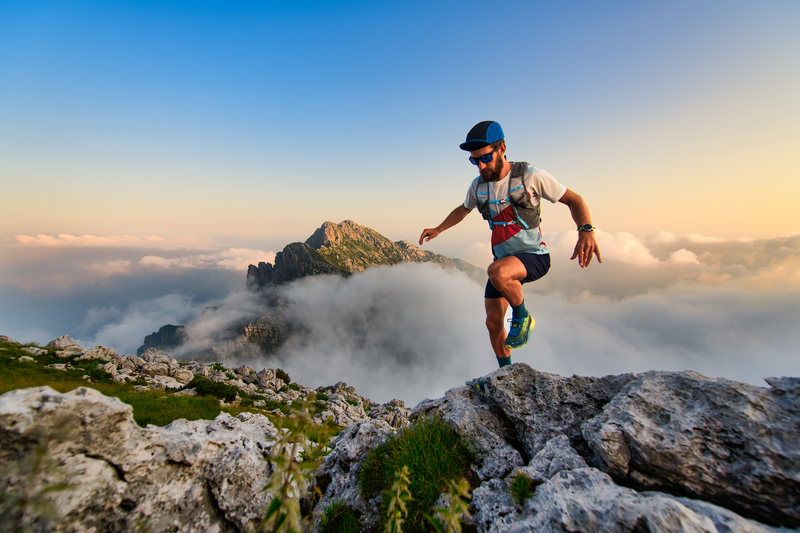
94% of researchers rate our articles as excellent or good
Learn more about the work of our research integrity team to safeguard the quality of each article we publish.
Find out more
REVIEW article
Front. Neurosci. , 25 March 2022
Sec. Brain Imaging Methods
Volume 16 - 2022 | https://doi.org/10.3389/fnins.2022.796129
This article is part of the Research Topic Advanced Imaging Techniques and Imaging Markers of Synaptic Density and Brain Connectivity in Animal Models of Neurological Diseases View all 9 articles
The brain is the central and most complex organ in the nervous system, comprising billions of neurons that constantly communicate through trillions of connections called synapses. Despite being formed mainly during prenatal and early postnatal development, synapses are continually refined and eliminated throughout life via complicated and hitherto incompletely understood mechanisms. Failure to correctly regulate the numbers and distribution of synapses has been associated with many neurological and psychiatric disorders, including autism, epilepsy, Alzheimer’s disease, and schizophrenia. Therefore, measurements of brain synaptic density, as well as early detection of synaptic dysfunction, are essential for understanding normal and abnormal brain development. To date, multiple synaptic density markers have been proposed and investigated in experimental models of brain disorders. The majority of the gold standard methodologies (e.g., electron microscopy or immunohistochemistry) visualize synapses or measure changes in pre- and postsynaptic proteins ex vivo. However, the invasive nature of these classic methodologies precludes their use in living organisms. The recent development of positron emission tomography (PET) tracers [such as (18F)UCB-H or (11C)UCB-J] that bind to a putative synaptic density marker, the synaptic vesicle 2A (SV2A) protein, is heralding a likely paradigm shift in detecting synaptic alterations in patients. Despite their limited specificity, novel, non-invasive magnetic resonance (MR)-based methods also show promise in inferring synaptic information by linking to glutamate neurotransmission. Although promising, all these methods entail various advantages and limitations that must be addressed before becoming part of routine clinical practice. In this review, we summarize and discuss current ex vivo and in vivo methods of quantifying synaptic density, including an evaluation of their reliability and experimental utility. We conclude with a critical assessment of challenges that need to be overcome before successfully employing synaptic density biomarkers as diagnostic and/or prognostic tools in the study of neurological and neuropsychiatric disorders.
Neurons are the fundamental units of the brain through which all bodily functions are coordinated. To achieve this level of global control, neurons communicate with each other through a network of synapses: the fundamental information processing units in the brain, which receive, process and transmit all the information (Stampanoni Bassi et al., 2019). In the central nervous system (CNS), the synapses can be classified as different types depending on: (1) the part of the presynaptic neuron connected to the postsynaptic neuron (axoaxonic, axosomatic, and axodendritic synapses), (2) the mechanism of information transmission between neurons (electrical or chemical synapses) or, in the case of chemical synapses, (3) the type of neurotransmitter involved in this transmission (excitatory and inhibitory synapses) (Torres and Varona, 2012; Caire et al., 2021). For the purpose of this review, we will exclusively focus on the (bipartite) chemical synapse — when a chemical (neurotransmitter) transduces an electrical impulse into chemical information (receptor binding) via a gap between a presynaptic and a postsynaptic neuron (synaptic cleft). This type of synapse is, by far, the most utilized in the CNS and, therefore, the main target of the existing methods to visualize or quantify synapses. Currently, these methods rely on detecting any one of the elements present in the presynaptic or the postsynaptic neuron, be it a morphological feature such as a change in dendritic spines (small dynamic protrusions located along the dendrites), or variations in the expression of a specific protein from the synaptic vesicle cycle or the postsynaptic density (PSD, an electron-dense structure located beneath the postsynaptic neuron’s membrane, usually at the tip of the dendritic spine) (see Figure 1).
Figure 1. Chemical synapse and targets for measuring synaptic density. (A) The main components of the bipartite synapse: a presynaptic and a postsynaptic neuron, separated by a synaptic cleft. In this synapse we have highlighted the two main targets involved in the methods currently available to quantify or assess synaptic density levels: (B) the dendritic spines (whose density and morphology are typically evaluated with different ex vivo methods) and (C) the proteins involved in synaptic transmission, including synaptic vesicle (SV), presynaptic and postsynaptic proteins present in excitatory and inhibitory synapses (which are quantified through ex vivo and in vivo methods). Adapted from “Synaptic Cleft (Horizontal),” by BioRender.com (2021). Retrieved from https://app.biorender.com/biorender-templates.
Recently, the interest in quantifying synaptic density has significantly increased. However, the concept of synaptic density has never been formally defined, which is reflected in the difficulties faced by researchers when measuring this parameter, and the controversy interpreting the results obtained with different methods. To understand the implications of measuring synaptic density, we should first consider how the number of synapses in the brain changes throughout life. During embryonic, neonatal, and adolescent brain development, new synapses are continually formed through a process known as synaptogenesis, after which they are either strengthened or, when no longer useful, eliminated through synaptic pruning (Oberman and Pascual-Leone, 2013; Power and Schlaggar, 2017). Although some synaptogenesis continues throughout life, the main assemblage of neural connections in an individual is likely completed by the end of adolescence, and this underpins the correct functioning of the nervous system (Peter, 1979; Paolicelli et al., 2011; Sakai, 2020). Thus, the concept of synaptic density is used to mean the net number of surviving synapses which changes very little in adulthood, except due to the influence of neurodevelopmental abnormalities, or during some neurodegenerative disorders (Lepeta et al., 2016).
The importance of correct synaptic organization is highlighted by emerging evidence that early life problems with either synaptogenesis or synaptic pruning may underpin many disorders of the nervous system (Cardozo et al., 2019). This includes disorders that are considered neurodevelopmental in origin (such as autism or schizophrenia), as well as psychiatric disorders (such as depression), and even neurodegenerative diseases that emerge later in life, despite having a proposed lengthy prodromal phase [such as Alzheimer’s disease (AD)]. Moreover, synapse loss is a hallmark of disorders that are traumatic in nature — such as stroke or brain injury — and even epilepsy (Murphy and Corbett, 2009; Rabiner, 2018; Jamjoom et al., 2021). More research in this field is needed to fully understand the importance of synapses, their number and organization, for proper function of the healthy CNS. What is unquestionable is that synaptic organization is dynamic and delicate, and that even small alterations at any developmental point may lead to profound imbalances and a variety of symptoms to sufferers. Research into autism, for example, has highlighted a possible relationship between abnormal brain development, symptomatology, and an excess of synapses via inadequate synaptic pruning (Neniskyte and Gross, 2017). Schizophrenia, on the other hand may be linked to excessive synaptic pruning within the prefrontal cortical brain circuitry (Sekar et al., 2016; Obi-Nagata et al., 2019; Keshavan et al., 2020; Germann et al., 2021). Synaptic loss in cortical and hippocampal brain areas in AD is well documented and robustly linked to cognitive symptoms of memory loss, and deficits in attention and thought organization (Selkoe, 2002; Knobloch and Mansuy, 2008; Scheff et al., 2014; Jackson et al., 2019; Colom-Cadena et al., 2020). This line of research resulted in the emergence of a novel theory positing that many, if not all neurodegenerative disorders are likely to be synaptopathies: disorders featuring disturbances in neuronal connectivity, in which the loss of synapses often features earlier than any other core symptoms (Grant, 2012; Lepeta et al., 2016; Luo et al., 2018). For example, synaptic pathology is present even in early stages of Huntington’s and Parkinson’s diseases (Li et al., 2003; Imbriani et al., 2018), as well as in related neurodegenerative disorders such as amyotrophic lateral sclerosis (ALS) and frontotemporal dementia (Taoufik et al., 2018; Fogarty, 2019).
Given the importance of appropriate synaptic numbers and connections in the living brain, accurate ways to determine their density are needed for research and diagnosis of psychiatric and neurological conditions. However, counting synapses and measuring their density is not a novel concept. Indeed, a plethora of validated methods exist, albeit the majority are performed ex vivo. The measurement of synaptic density in vivo is substantially more challenging and novel imaging methods are attempting to achieve this.
In this review, we first briefly summarize the existing ex vivo methods for measuring synaptic density and comment on their applications in neuroscience. Although all the methods and concepts outlined can and have been used in clinical as well as in preclinical research, here we focus on examples from preclinical (non-human) studies since the majority of ex vivo evaluations tend to be undertaken in experimental animal models. Furthermore, we provide a critique of these approaches, showcasing their strengths and weaknesses. We then discuss the current and emerging methods that attempt to measure synapses in vivo, and we comment on their utility in different settings, as well as the degree of their validation against gold standards. The overall aim of this review is to underline the importance of studying synaptic density in both the healthy brain and in neurological and neuropsychiatric disorders, as well as to help the reader select the most suitable tools with which to measure synaptic density for their own research.
To date, the gold standard methods for measuring synaptic density are conducted ex vivo (i.e., in post-mortem tissues) and involve either high resolution electron microscopy (EM), immunohistochemistry (IHC) or both. Although these methods preclude the longitudinal evaluation of the animals or patients under assessment — therefore unable to follow the development of a pathology or the response to treatment — they nevertheless tend to be more direct and specific, allowing more detailed analyses than in vivo methods due to their superior spatial resolution. These techniques focus predominantly on one of the three main aspects of the synapse: structure and spatial organization (EM), the morphology and density of dendritic spines (EM and histology), and the expression of proteins in the pre- and postsynaptic neurons, especially in the presynaptic active zone and the postsynaptic density (PSD) area (immuno-EM and IHC).
This section covers the assessment of synapses by EM and IHC, including some examples of applications in preclinical research. Although alternative, non-imaging ex vivo methods to measure parameters related to synaptic density exist (such as Western Blot and proteomics), they are beyond the scope of this review and are covered in other reviews such as (Ippolito and Eroglu, 2010; Patrizio and Specht, 2016).
Electron microscopy (EM) is the gold standard method to quantify changes in synaptic density, being the only technique that allows direct visualization of the synapse and its molecular organization due to its ultra-high spatial resolution (nanometer range) (Figure 2). Successful applications of EM have led to major breakthroughs in the field of neuroscience, such as the discovery of synaptic vesicles (Wells, 2005), the nature of dendrite spines as sites of synaptic contact (Gray, 1959), and the existence of cells other than neurons (such as astrocytes) involved in synaptic transmission (tripartite synapse) (Ventura and Harris, 1999).
Figure 2. Image of a cortical glutamatergic synapse of an adult C57BL/6 mouse. The image was obtained on a Jeol 1010 transmission electron microscope (Jeol, Tokyo, Japan) at 80,000× magnification. (A) Presynaptic neuron, with synaptic vesicles indicated with red arrows. (B) Synaptic cleft. (C) Dendritic spine of a postsynaptic neuron, with postsynaptic density (electron-dense zone juxtaposed to the postsynaptic membrane) indicated with a blue arrow. Image courtesy of Nuria García Font, see García-Font et al. (2019) for more information about the methodology.
There are two main types of electron microscopes, both employed to assess synaptic density: transmission (TEM) and scanning (SEM) electron microscopes. Although both use an electron source, they provide different information about the sample and are used for distinct purposes. In a TEM, the electrons pass through the tissue sample before they are collected. This system offers high spatial resolution (<50 pm) and provides valuable information about the inner structure of the synapse (e.g., area of active zone and number of docked presynaptic vesicles). However, the samples must be very thin, requiring complex preparation to avoid artifacts, and the images obtained are two-dimensional (2D) projections of the sample (micrographs). By contrast, SEMs scan the surface of the sample with a focused beam of electrons to provide a three-dimensional (3D) image, which can be used to study synapse morphology or measure dendritic spine volume and density within a brain region (Borczyk et al., 2019). However, the resolution of SEM (∼0.5 nm) is lower than TEM.
Based on the characteristics of these microscopes, variations have been developed to overcome their limitations (sample preparation, resolution, and 2D vs. 3D acquisition) and to allow combination with other techniques. Some examples of these derived methods are electron tomography (which uses TEM to generate a high-resolution 3D image), immuno-EM [which combines the use of gold-labeled antibodies with EM (D’Amico and Skarmoutsou, 2008; Liu et al., 2019)], array tomography [which combines the use of immunofluorescence (IF) with SEM to depict the specific components of the synapse (Micheva and Smith, 2007; Collman et al., 2015)], and focused ion beam milling-SEM (FIB-SEM, which allows the actual quantification of the density of synapses in a brain area through the automatization of the steps of sectioning and image acquisition (Merchan-Pérez, 2009; Santuy et al., 2020).
In preclinical research, EM has mainly been applied to map synaptic structures and regional densities in the brain of healthy wild type (WT) animals (Kaplan and Hinds, 1977; Harris and Weinberg, 2012; Santuy et al., 2018). Several studies have also explored the concept of synaptic plasticity through different paradigms such as: (1) evaluating how the PSD and dendritic spines modify their shape and structure after a chemically induced long-term potentiation (Borczyk et al., 2019), (2) studying synaptic reorganization and reactive synaptogenesis/synaptic loss in response to a lesion (DiFiglia et al., 1988; Marrone et al., 2004; Kim and Jones, 2010; Li Q. et al., 2018), or (3) analyzing the effect of maturation and aging in the synapse (Kaplan and Hinds, 1977; Harris and Weinberg, 2012; Fan et al., 2018; Santuy et al., 2018). These studies emphasize both the existence of synaptic remodeling (even during adulthood) and the presence of compensatory mechanisms for a decrease in synaptic density (such as the increase in the size and surface area of the remaining connections) (Scheff et al., 1991; Dawirs et al., 1992; Calì et al., 2018).
The use of EM to study synapses in the context of neurodevelopmental and neurodegenerative animal models remains limited, partially due to its spatial restrictions as well as laborious and lengthy analytical methods. Predominately, studies have focused on understanding changes in synaptic density in relation to AD, in mouse models such as Tg2576 (AD model expressing human mutant form of the amyloid precursor protein, APP). This research confirmed the expected decrease in synapses in regions known to be affected by AD pathology — such as the hippocampal dentate gyrus or the entorhinal cortex (Dong et al., 2007) — and showed improvements in synaptic deficits after experimental treatments (Pérez-González et al., 2014; Xiao et al., 2020). Most importantly, these findings served either to clarify previous inconsistencies regarding synaptic loss measured with other methods (such as synaptophysin IHC (Dong et al., 2007)) or to corroborate results obtained by using different techniques (Pérez-González et al., 2014; Fan et al., 2018).
Several other neurodevelopmental and neurodegenerative disease models have shown alterations in synaptic density. These include, for example, a decrease in the synapse-to-neuron ratio in the Ts65Dn mouse model of Down syndrome (Ayberk Kurt et al., 2004), an absence of life-long changes in total synaptic density (number of synapses and synapses onto spines) in the R6/2 mouse model of Huntington’s disease (Savage et al., 2020), and a decrease in spine density and ultrastructural spine abnormalities in the A53T-BAC-SNCA mouse model of Parkinson’s disease (Parajuli et al., 2020).
Despite the multiple possibilities of EM for the study synaptic density, its use in clinical and preclinical research is restricted due to the ex vivo nature of this technique, the high costs of purchasing and maintaining electron microscopes, and the complexity and skillset required for the data acquisition and analysis.
Furthermore, the brain tissue must be carefully processed, which typically involves the use of specific aldehyde fixatives and chemical compounds to preserve the ultra-structure of the synapse. Subsequently, the tissue is embedded in a resin to be sectioned in ultrathin sections (<150 nm) using an ultramicrotome. As these treatments themselves can induce artifacts or changes in the synaptic structure (Siksou et al., 2009), specialist training is required. Because of these barriers, the applications of EM and its combination with other ex vivo techniques remain limited.
Finally, the magnification power of electron microscopes comes with a trade-off of reduced field of view and sample size, hampering both global and multi-regional analyses of the brain. Nevertheless, recent improvements in EM methods for imaging large volumes may soon facilitate the visualization of larger brain areas (Kasthuri et al., 2015) or even the whole brain (Mikula et al., 2012).
While EM is arguably the best and most direct method to study synapses ex vivo, histology and IHC are the most common, owing to their wider accessibility, ease of use and lower cost. These techniques are employed with two main purposes: to evaluate the morphology and density of dendritic spines — present predominantly, but not exclusively, in excitatory synapses (Scheuss and Bonhoeffer, 2014) — and to detect changes in the expression of pre- and postsynaptic proteins with specific antibodies.
Histological quantification of dendritic spine morphology and density has been of much interest in the study of normal and pathological brain conditions, due to the important role of these structures in most excitatory synapses (Nimchinsky et al., 2002); their involvement in synaptic plasticity, learning and memory processes (Mahmmoud et al., 2015; Ma and Zuo, 2021); and the presence of abnormal spines in many brain disorders (Fiala et al., 2002; Blanpied and Ehlers, 2004; Herms and Dorostkar, 2016). The quantification of spines — the number of (visible) spines per micrometer of dendrite — is considered to represent an index of synaptic density and functionality, due to the presence of these structures in most synapses. Recent research has highlighted the existence of a bidirectional relationship between spine maturation/functionality and synapse stability. For example, it was reported that even subtle changes in spine structure or motility can affect synaptic transmission (Wong, 2005; Lordkipanidze et al., 2013; Obashi et al., 2021), and that an increase in synaptic strength promotes the emergence and maturation of spines (Hotulainen and Hoogenraad, 2010; Fortin et al., 2012).
Among the ex vivo methods used to visualize changes in spine morphology and density in brain sections, Golgi staining is the oldest and most commonly used. This impregnation method involves the accumulation of heavy metal ions (silver or mercury) on the surface of a small subset of neurons, allowing the visualization of their entire structure, including the dendritic spines. Since its inception, this method has been improved (Pilati et al., 2008), modified to make it faster (e.g., Golgi-Cox method (Ranjan and Mallick, 2010)), and even combined with other techniques such as EM (Fairén, 2005) or tissue clearing and fluorescence (Vints et al., 2019). Despite the relevance and utility of Golgi staining, it also presents disadvantages such as random and unpredictable cell staining (Mancuso et al., 2013). Therefore, alternative methods have been developed, such as staining with the carbocyanine dye DiI — a fluorescent dye whose insertion into the lipid membrane of the neurons allows the visualization of their architecture (Gan et al., 2000; Cheng et al., 2014; Ba̧czyńska et al., 2021) — or the use of Lucifer Yellow or XFP [green (GFP), yellow (YFP), cyan (CFP) and red (RFP)] fluorescent proteins that label the entire neuronal structure (Feng et al., 2000). All these techniques allow quantification of potential changes in spine morphology and density when combined with manual and automatic analysis of maximum intensity projection (2D images) or 3D and even 4D image reconstruction (Dickstein et al., 2016; Basu et al., 2018; Kashiwagi et al., 2019; Ba̧czyńska et al., 2021). Moreover, the in vivo visualization of spine dynamics is possible in freely moving animals, thanks to the combination of transgenic mice expressing genetically tagged fluorescent proteins (such as PSD-95:GFP, or Thy1-YFP mice) and new microscopy methods (such as two-photon microscopy) (Niell et al., 2004; Yang et al., 2014; Garré et al., 2017).
Immunohistochemistry and particularly IF (which involves the use of a secondary antibody chemically conjugated to a fluorescent dye) has been extensively used to evaluate changes in the expression of pre- and postsynaptic proteins as indices of synaptic density. One of the advantages of this technique is the possibility of differentiating between excitatory and inhibitory synapses, owing to the availability of multiple antibodies that target proteins predominantly expressed in these synapses (e.g., excitatory synapses express PSD-95 whereas inhibitory synapses express gephyrin) (van Spronsen and Hoogenraad, 2010; Sheng and Kim, 2011; McLeod et al., 2017; Favuzzi and Rico, 2018). Such differentiation of targets has been an important tool in the study of selective gains and losses of synapses in diseases such as AD (Lauterborn et al., 2021). Moreover, the ability to distinguish between synaptic proteins has also led to the discovery that PSD-95 and neuroligin-1 are among the key regulators of the ratio between the number of excitatory and inhibitory synapses in the brain, the imbalance of which has been hypothesized as an underpinning factor of some brain disorders (Prange et al., 2004; Keith, 2008). In contrast, most presynaptic proteins, including SV2A and synaptophysin, are expressed in both excitatory and inhibitory synapses, allowing a global quantification of synaptic density. Such quantification is mostly performed by first applying a background correction and then analyzing the distribution, number, or intensity of the immunoreactive puncta (Ippolito and Eroglu, 2010; McLeod et al., 2017; Guirado et al., 2018), or evaluating the mean fluorescence intensity within a region (Shihan et al., 2021).
A summary of the synaptic proteins most commonly used in the analysis of synaptic density is shown in Table 1.
Histological examination of synapses in preclinical research has hitherto contributed to many important scientific discoveries in animal models of different brain disorders. As an example, abnormal spine morphology, density, and function have been related to cognitive deficits and reported in neurodevelopmental disorders such as Down syndrome or autism (von Bohlen und Halbach, 2010; Varghese et al., 2017; Torres et al., 2018). For instance, the Ts65Dn mouse model of Down syndrome exhibits a decrease in spine density but an enlargement of these structures, which seems to correlate with the severity of cognitive impairment (Belichenko et al., 2004, 2007). In autism models, mutations in genes coding for postsynaptic proteins such as Shank3 result in modifications of dendritic spine morphology, suggesting a relationship between synaptic protein expression and spine morphology (Durand et al., 2012; Jiang and Ehlers, 2013; Zatkova et al., 2016; Guo et al., 2019).
However, not all the alterations in dendritic spines and synaptic protein concentration are associated with genetic factors: some of them are the result of internal changes (e.g., alterations in proteins such as Reelin), learning processes or environmental changes such as stress and isolation (Lieshoff and Bischof, 2003; Murmu et al., 2006; Bosch et al., 2016). Therefore, changes in spine density/morphology and in concentrations of different pre- and postsynaptic proteins have been extensively studied as markers and potential therapeutic targets of diverse pathologies and disorders (de Bartolomeis and Fiore, 2004; Dorostkar et al., 2015; Duman and Duman, 2015; Herms and Dorostkar, 2016; Ba̧czyńska et al., 2021).
Epilepsy is characterized by the presence of uncontrolled and recurrent seizures, which are bursts of electrical neuronal activity. As most of this excitatory activity affects the dendritic spines, multiple research groups have studied the relationship between seizures and changes in the morphology and density of these structures (Wong and Guo, 2013; Tiwari et al., 2020; Xie et al., 2020). Specifically, spine density of the hippocampal and cortical pyramidal neurons appears to be reduced immediately after acute seizures, whereas it is recovered during the transition phase (period without seizures) and reduced, again, during the later chronic phase (period with recurrent spontaneous seizures) (Isokawa, 1998; Guo et al., 2012; Xie et al., 2020).
Interestingly, this alteration in spine density seems to precede neuronal death and has been suggested as a potential reason behind the cognitive and memory deficits observed in this disease (Wong, 2005). However, the results of combining in vivo imaging (two-photon microscopy) of dendritic spines with electroencephalography (EEG) during focal neocortical seizures suggest that the presence of electrographic seizures only creates a predisposition toward, but not necessarily causes, dendritic spine degeneration (Rensing et al., 2005).
Another way to study changes in synaptic density during epileptogenesis is to quantify synaptic proteins such as SV2A, synaptophysin or synaptotagmin (see Figure 3). Indeed, these proteins are mostly decreased in preclinical models of epilepsy, which could be reversed by pharmacological treatments or by exposure to an enriched environment (van Vliet et al., 2009; Hanaya et al., 2012; Salaka et al., 2021), confirming the aforementioned utility of synaptic IHC as biomarker of epilepsy. On the other hand, reports of increases in the expression of the same markers (Contreras-García et al., 2018; Wang et al., 2018), highlight the possible presence of compensatory mechanisms including, for example, an increase in GABAergic neurotransmission (Ohno et al., 2012).
Figure 3. Representative hippocampal labeling of the synaptic vesicle protein synaptotagmin-1 in a control (A) and (B) epilepsy [kainic acid rat model of temporal lobe epilepsy (Levesque and Avoli, 2013)]. Both images were obtained using the polyclonal rabbit anti-Syt1 (Abcam, Cambridge, MA; Cat#ab131551); dilution 1:100 (overnight, 4°C). The secondary antibody was donkey anti-Rabbit Alexa Fluor488-conjugated (Thermo Fisher Scientific, Oregon, United States; Cat#A-21206); dilution 1:500 (45 min, RT). DAPI was used to counterstain (blue). The images were obtained with a scanning laser microscope (Leica TCS SP5 with AOBS, Leica Microsystems IR GmbH, Germany) with a 20 × magnification and similar exposure time. Note a decrease in synaptotagmin-1 labeling in the epileptic rat (3 months after kainic acid administration), compared to the control. Images were obtained at GIGA-CRC in vivo imaging and the GIGA-Imaging platform, ULiège (Belgium).
Changes in the morphology and density of dendritic spines have been postulated as main reasons for the synaptic and neuronal loss observed in AD. Therefore, dendritic spines are promising targets for new treatments (Knobloch and Mansuy, 2008; O’Neal et al., 2018; Ettcheto et al., 2020). Pathological extracellular deposits of amyloid-betaqq (A) protein, or Aβ plaques, seem to have a major role in the aberrant spine morphology and decrease in spine density observed in AD models (Dorostkar et al., 2015; Marttinen et al., 2018). Interestingly, A plaques were associated with elevated activity of calcineurin, which affects the morphology and density of dendritic spines through the inhibition of peptidyl-prolyl isomerase Pin1 signaling (Stallings et al., 2018; Reza-Zaldivar et al., 2020). This led to O’Neal et al. (2018) suggesting that an FDA approved calcineurin inhibitor be tested in AD patients. However, evidence from the Tg2576 mouse model of AD suggests that early decreases in spine density correlate with cognitive impairment and emerge before any measurable accumulation of insoluble A protein (Jacobsen et al., 2006).
Measurements of pre- and postsynaptic proteins have also been used as markers of synaptic density in AD models. Such investigations led to the discovery, in the 2xTg (APP/PS1) mouse, of an association between reduced SV2A and gephyrin in the nucleus accumbens (NAc), increased intracellular Aaccumulation, and decreased glycinergic (inhibitory) miniature synaptic currents (Fernández-Pérez et al., 2020).
Alterations in the morphology and density of dendritic spines, and in the expression of pre- and postsynaptic proteins have been associated not only with different neuropsychiatric disorders including depression and schizophrenia, but also with addiction to drugs of abuse such as psychostimulants (Robinson and Kolb, 1999; Blanpied and Ehlers, 2004).
In animal models of stress and depression, the reported changes in spine morphology and density seem to be strongly influenced by multiple parameters, including the model used, the sex and genetic strain of the animals, and the brain region analyzed (Murmu et al., 2006; Bock et al., 2011; Duman and Duman, 2015; Qiao et al., 2016). Most studies reported a decrease in spine density in the prefrontal cortex and hippocampus — regions typically atrophied in people suffering from depression and associated with the severity of the disease (Taylor et al., 2014)— but an increase in spine density in the amygdala and the NAc. Importantly, some of these alterations could be rescued by treatments with antidepressants such as ketamine (Krzystyniak et al., 2019; Ali et al., 2020). However, the existence of contradictory results — e.g., the absence of spine loss or even an increase in spine density in some animal models (Fox et al., 2020)— highlights the importance of creating standardized protocols and improving replicability between laboratories. Regarding the measurement of synaptic proteins, the evaluation of vesicular GABA transporter (vGAT) and gephyrin in mice that underwent chronic social defeat stress is more in agreement with the results observed in humans, where there was a significant decrease in both these synaptic markers in the NAc (Heshmati et al., 2020).
In schizophrenia, the research evaluating changes in dendritic spines is more consistent, with multiple laboratories reporting decreases in spine density and dendritic arborization in brain regions such as the primary visual cortex, the prefrontal cortex and the subiculum (Moyer et al., 2015; Tendilla-Beltrán et al., 2019; Chen P. et al., 2021). Despite some contradictory results, several studies have suggested that a reduction in microtubule-associated protein 2 (MAP2) — a constituent of the PSD — is one of the reasons for the observed spine density decrease (Gu et al., 2008; Jaworski et al., 2009). In agreement with the notion of decreased spine density in schizophrenia, lower levels of PSD-95 have also been found in the frontal cortex and ventral hippocampus of the sub-chronic phencyclidine (PCP) mouse model of schizophrenia (Gigg et al., 2020). A recent meta-analysis reviewed research quantifying multiple synaptic measures (dendritic spine density, PSD number and PSD protein expression levels) in post-mortem human brain tissue, from different methods (Golgi staining, IHC and EM) (Berdenis van Berlekom et al., 2020). The results of this meta-analysis are consistent with those obtained in preclinical research, with most studies highlighting a significant decrease in synaptic density in the brain of patients with schizophrenia compared to healthy controls.
There are several aspects to consider when using histological and IHC techniques to quantify synaptic density. Some of these are related to the nature of the target. For instance, the evaluation of spine density should be considered only as a partial biomarker of synaptic density, since not all synapses are formed on dendritic spines, which are absent in most inhibitory interneurons (Markram et al., 2004). The quantification of pre- and postsynaptic proteins, commonly present in chemical synapses, also disregards the existence of electric synapses and their important role in brain development (Szabo, 2004; Todd et al., 2010) and in the adult nervous system (Connors and Long, 2004; Pereda, 2014), especially in local inhibitory circuits (Galarreta and Hestrin, 2001; Vervaeke et al., 2012). Furthermore, it is important to carefully select which synaptic protein will be used as a synaptic density marker, since they are not only disease-specific but also have differential expressions across time and brain regions. For example, there is a selective, regional protein loss in AD patients, with some hippocampal postsynaptic proteins being less affected by the disease, while others present even an increase in their expression (Clare et al., 2010, de Wilde et al., 2016). Additionally, multiple studies have highlighted the presence of presynaptic proteins not only in neurons, but also in astrocytes. For instance, cultured astrocytes seem to express proteins such as synaptobrevin-2 (the so-called vesicle-associated membrane protein 2 or vAMP2), synaptotagmin-1, synaptophysin and SNAP-25 (Maienschein et al., 1999; Wilhelm et al., 2004; Singh et al., 2014), which is involved in the release (exocytosis) of glutamate-containing vesicles (Zhang et al., 2004; Crippa et al., 2006; Mielnicka and Michaluk, 2021). Recently, the vesicular glutamate transporter (vGLUT) has also been found in microglia (Brioschi et al., 2020). These studies highlight the importance of employing multiple antibodies (markers) and even different methods to measure synaptic structure and density before reaching any conclusion about how these are affected in neurological and neuropsychiatric disorders.
Other limitations of histology and IHC derive from the methodology itself: despite the existence of multiple antibodies to target pre- and postsynaptic proteins, some of them have poor labeling performance (e.g., lack of specificity and/or sensitivity) and, therefore, are not suitable to quantify synaptic density (Verstraelen et al., 2020). This specificity problem could explain the contradictory results obtained with some of these antibodies, which urges the development of new and more specific antibodies to precisely quantify synaptic density. In the case of IF, a bias in the measurement of synaptic density could also be introduced by the characteristics of the fluorophore and the fluorescence signal, which is expected to fade over time, precluding comparison of images obtained at different time points.
Finally, the intrinsic characteristics of the image acquisition and analysis are also a source of bias. In this regard, confocal laser-scanning microscopes often produce partial and distorted results due to their insufficient spatial resolution. A clear example is the bias in estimating spine density from 2D images, where many spines can be hidden from the field of view depending on their position on the dendrite. In recent years, the refinement of optical brain clearing techniques and tissue expansion-enabled imaging — extensively reviewed elsewhere (Gómez-Gaviro et al., 2020; Parra-Damas and Saura, 2020; Ueda et al., 2020) — have opened the door to a faster and more precise visualization of synapses, facilitating the study and the analysis of neural circuits even in deep brain structures (Hama et al., 2011) or living animals (Iijima et al., 2021). Additionally, the development of super-resolution fluorescence microscopy techniques — such as 3D stimulated emission depletion (STED) microscopy (Vicidomini et al., 2018; Sahl and Hell, 2019) or super-resolution shadow imaging (SUSHI) (Tønnesen et al., 2018)— has improved image resolution and decreased the presence of artifacts, enabling a more precise assessment of alterations in spine structure and density. However, these methods are not yet widely accessible and still require manual or semi-manual processing of images due to the absence of sufficient 3D data to properly implement machine learning algorithms (Ruszczycki et al., 2012; Belthangady and Royer, 2019).
Imaging synaptic density in post-mortem tissue is also possible with autoradiography, which involves imaging the distribution of molecules labeled with radioisotopes [e.g., hydrogen-3 (3H) or iodine-125 (125I)] in tissue sections (see Figure 4).
Figure 4. SV2A autoradiography with [3H]UCB-J, performed in an adult C57BL/6 mouse. (A,B) Two representative autoradiographs showing [3H]UCB-J labeling. (C) 3H standard for quantification (ART-123A American Radiolabeled Chemicals Inc., United States). Slices (20 μm) were mounted onto a glass slide (Superfrost™) and incubated with 3 nM [3H]UCB-J (Novandi Chemistry AB, Sweden). Once dried, the slide was placed into light-tight cassettes with the radioactive standard slide and a hyperfilm (Amersham 8 × 10 in Hyperfilm Scientific Laboratory Supplies, United Kingdom). Films were exposed for 2 weeks before being developed in a Protex Ecomax film developer (Protec GmbH & Co, Germany). Images acquired at BRAIN Centre, King’s College London, London, United Kingdom.
This technique presents two variations: in vitro autoradiography, which involves incubating mounted tissue with a radiolabeled ligand, and ex vivo autoradiography, which involves injecting a radiolabeled ligand at tracer concentrations (hence radiotracer) into a living animal before collecting, cutting, and mounting the tissue. In both forms, images of the radioligand distribution are acquired from the brains processed post-mortem (Maurer, 1984; Ishiwata et al., 1999; Griem-Krey et al., 2019). Autoradiography has proved useful for characterizing newly developed positron emission tomography (PET) tracers, due to its simplicity and relatively low cost, providing information about the metabolism of the radiotracers, ligand selectivity and target localization (Solon, 2012; Manuel et al., 2015; Griem-Krey et al., 2019). Furthermore, autoradiography provides higher spatial resolution images than PET (μm vs. mm), which enables the quantification and localization of binding in small anatomical structures of rodent brains (Schmidt and Smith, 2005). However, this method also has some limitations, such as the unsuitability for longitudinal studies, or the limited information that it provides about radiotracer kinetics (Kuhar and Unnerstall, 1985; Schmidt and Smith, 2005).
The use of autoradiography for quantification of synaptic density in preclinical research has been made possible by the development of SV2A radiotracers, described in more detail in the following section. These radiotracers have been used to map the expression of the presynaptic SV2A protein in the brain of WT and transgenic animals (Menten-Dedoyart et al., 2016; Varnäs et al., 2020), to evaluate changes in synaptic density in the 6-hydroxydopamine (6-OHDA) model of Parkinson’s disease and the quinolinic acid model of Huntington’s disease (Thomsen et al., 2021), and to study the effect of different drugs and treatments (Onwordi et al., 2020; Binda et al., 2021; Halff et al., 2021; Raval et al., 2021b).
Even though the spatial resolution of in vivo techniques is significantly poorer than that of ex vivo methods, measuring synaptic density in vivo presents many advantages, such as the possibility of quantifying the number of synapses longitudinally, which may result in earlier diagnosis of brain disorders. This characteristic has recently promoted the use of these techniques as important biomarkers in neurodegenerative and psychiatric disorders.
In this section we cover the most used in vivo techniques, which explore two different aspects of the synapse: the concentration of the SV2A protein (SV2A PET tracers), and the concentration of glutamate (gluCEST).
The last decade has seen a significant rise in the synthesis and use of SV2A PET tracers. These radioactive compounds are commonly designated as synaptic density radiotracers due to their affinity and specificity for the SV2A protein: a transmembrane presynaptic protein present in synaptic vesicles that plays an important role in synaptic transmission — specifically in the calcium-dependent release of neurotransmitters (through its interaction with synaptotagmin-1) — and in synaptic vesicle recycling (interacting with synaptotagmin-1 and stonin-2) (Mendoza-Torreblanca et al., 2013; Bartholome et al., 2017). Traditionally, this protein has been associated with epilepsy for three main reasons: (1) SV2A is the molecular target of the antiepileptic drug levetiracetam, (2) SV2A knockout mice suffer from seizures from postnatal day 7 and die 2 weeks later, and (3) the brain expression of SV2A in animal models and patients with epilepsy is lower than in healthy controls (Crowder et al., 1999; Lynch et al., 2004; Klitgaard and Verdru, 2007; van Vliet et al., 2009; Sills, 2010).
The discovery of the relationship between SV2A and epilepsy triggered the development of the first SV2A radiotracer, [3H]ucb 30889, with the aim to identify levetiracetam’s binding sites in the rat brain and spinal cord (Gillard et al., 2003; Lambeng et al., 2005). Despite its utility, the extremely long half-life (>12 years) of tritium precluded the use of this radiotracer in humans. In 2013, the creation of the first PET-suitable radiotracer, [18F]UCB-H, allowed the in vivo measurement of changes in SV2A brain levels in both humans and animals (Bretin et al., 2013; Warnock et al., 2014; Becker et al., 2017; Serrano et al., 2018). Since then, other PET radiotracers have been developed, such as [11C]levetiracetam (Cai et al., 2014), [11C]UCB-A (Estrada et al., 2016), and [11C]UCB-J (Nabulsi et al., 2016). The latter, [11C]UCB-J, displays higher specific binding capacity than [18F]UCB-H, with also a high brain uptake, fast in vivo kinetics and rapid metabolism. Nevertheless, the half-life of carbon-11 (11C, 20.4 min) is significantly shorter than that of fluorine-18 (18F, 109.8 min), which precludes the routine use of [11C]UCB-J as it requires an on-site cyclotron for its synthesis. New radiotracers, such as [18F]SynVesT-1 — previously called [18F]SDM-8 and [18F]MNI-1126 — and [18F]SynVesT-2 (also named [18F]SDM-2) are supposed to overcome this problem by combining the specificity for SV2A and the fast in vivo binding kinetics of [11C]UCB-J with the longer half-life of [18F]UCB-H (Li S. et al., 2018; Constantinescu et al., 2019; Cai et al., 2020; Patel et al., 2020; Sadasivam et al., 2021).
The development of these novel SV2A PET tracers occurred in parallel with the discovery of the important role of SV2A in the onset and development of multiple neurological and neuropsychiatric disorders, opening the door to a wide spectrum of applications (Mattheisen et al., 2012; Stockburger et al., 2015; Cortès-Saladelafont et al., 2016; Li and Kavalali, 2017; Heurling et al., 2019). Nevertheless, it was not until 2016 that the full potential of these radiotracers was unveiled: the possibility to detect changes in synaptic density in vivo (Finnema et al., 2016; Morris, 2016; Mercier et al., 2017) through regional quantification of brain SV2A expression.
The use of SV2A PET tracers in preclinical research has been key to improving their synthesis, specificity, and kinetics, as well as to enable the in vivo quantification of synaptic density in a wide spectrum of neurological and neuropsychiatric disorders (Rabiner, 2018; Cai et al., 2019; Constantinescu et al., 2019). For example, (Serrano et al., 2020) explored changes in synaptic density at different time-points of the rat lifespan (late puberty to adulthood) evaluating in parallel how epileptogenesis affects the brain. This study showed an increase in synaptic density throughout the lifespan of healthy animals, in line with the recently reported increases in gray and white matter volumes (MacNicol et al., 2022). These results support the idea of brain plasticity in which synapses are continuously being formed and strengthened, highlighting the potential of quantifying SV2A in vivo to detect aberrancies in brain development.
In the following subsections we give an overview of the use of SV2A PET tracers in preclinical research. Given the relative novelty of some of these tracers, relatively few preclinical studies have been published to date. Furthermore, SV2A PET tracers are increasingly used in clinical research, where they have been successfully used to map out synaptic changes in AD and Progressive Supranuclear Palsy (Passamonti et al., 2017; Holland et al., 2020, 2021; O’Dell et al., 2021; Tuncel et al., 2021) as well as in other conditions such as depression (Holmes et al., 2019), schizophrenia (Onwordi et al., 2020), cannabis use disorder (D’Souza et al., 2020), and human immunodeficiency virus (HIV) (Weiss et al., 2021).
Even though the development of SV2A tracers was motivated by epilepsy research, only three articles have been published to date in this area: two clinical proof-of-concept studies about the ability of [11C]UCB-J radiotracer to detect a decrease in synaptic density in patients with temporal lobe epilepsy and unilateral mesial temporal sclerosis (Finnema et al., 2016, 2020), and one preclinical study exploring in vivo changes in SV2A during the development of temporal lobe epilepsy (Serrano et al., 2020). The latter observed a progressive and region-dependent decrease in [18F]UCB-H binding (and, by extension, a decrease in synaptic density) in epileptic animals, with significant differences between groups even before the onset of seizures. Furthermore, the results obtained in vivo during the chronic phase of epilepsy were confirmed ex vivo with SV2A IF, highlighting not only the utility of SV2A PET as an epilepsy biomarker, but also the reliability of this in vivo technique.
[11C]UCB-J and [18F]SynVesT-1 have been used to study synaptic loss in two AD mouse models, ArcSwe and APP/PS1 (Sadasivam et al., 2021; Xiong et al., 2021), as well as to evaluate the potential effect of treating AD pathology with a Fyn kinase inhibitor, saracatinib (Toyonaga et al., 2019). Even though the methods employed to analyze radiotracer binding are different in these studies, they all reach the conclusion that there is a significant decrease in synaptic density in the hippocampus of AD animals, which can be rescued with saracatinib, and measured in vivo with SV2A PET radiotracers.
In psychiatric research, [11C]UCB-J has been used to measure synaptic density deficits in the Sapap3 knockout model of obsessive-compulsive disorder, through a longitudinal PET study in which an early decrease in radiotracer binding was reported in several brain regions of this model, including in the cortex, striatum, thalamus, and hippocampus (Glorie et al., 2020).
A general limitation of PET imaging is the difficulty in quantifying radiotracer binding, which usually requires drawing multiple blood samples at precise time-points to calculate the concentration of non-metabolized radiotracer in arterial plasma (arterial input function) (Laruelle, 2002; Acton et al., 2004). Even though non-invasive alternatives have been proposed — such as deriving the input function from the image, or the use of a reference region with no specific uptake — these methods also present associated problems, and their accuracy must be assessed before their routine use (Tomasi et al., 2012; Lammertsma, 2017; Serrano et al., 2018). With respect to the use of SV2A radiotracers, their results (although promising) must be taken with caution since they rely on three aspects: (1) the reliability of using SV2A as synaptic density marker in a specific disease, (2) the variability of SV2A expression due to external stimulus, and (3) the ability of the radiotracer to bind to the SV2A protein.
Regarding the first aspect, a selective loss and gain of different synaptic proteins has been reported in AD (Sze et al., 2000; de Wilde et al., 2016), with some contradictory results about changes in SV2A. For instance, several authors have reported unchanged SV2A levels in the middle frontal gyrus (Metaxas et al., 2019), the hippocampus, entorhinal cortex, caudate nucleus, and occipital cortex (Sze et al., 2000) in post-mortem samples from AD patients. In contrast, the in vivo comparison of healthy volunteers and AD patients with [11C]UCB-J PET showed a 41% reduction in hippocampal binding in the AD group (Chen M.K. et al., 2018). Interestingly, other synaptic proteins show a differential expression in AD, with the presynaptic proteins being more affected by the disease than the postsynaptic ones (de Wilde et al., 2016). These studies highlight the necessity of combining ex vivo and in vivo techniques and measuring different pre- and postsynaptic proteins to obtain a more reliable measure of synaptic density in a specific disease.
Concerning the second aspect, although SV2A expression is constant inside synaptic vesicles and presents a small intervesicle variation (Mutch et al., 2011), the number of synaptic vesicles in the presynapse is closely related to synaptic activity and functionality (Valtorta et al., 1990; Rizzoli and Betz, 2005). The number of synaptic vesicles, therefore, is expected to increase in the presence of a stimulus, raising the question whether the binding of SV2A radiotracers provides a stable measure of synaptic density or primarily reflects brain activity in the moment of scanning. This question has been recently tackled by Smart et al. (2021) where the effect of stimulating the visual cortex on the [11C]UCB-J binding was assessed through the measurement and comparison of three kinetic parameters: tissue influx (K1), volume of distribution (VT) and binding potential (BPND). The results highlighted the stability of VT and BPND during cortical stimulation and corroborated their utility as in vivo markers of SV2A levels and, for instance, as potential markers of synaptic density. On the contrary, K1 values increased during the visual stimulation and were significantly correlated with cerebral blood flow and fMRI BOLD signal assessed with the same paradigm. K1, therefore, reflects the radiotracer influx and should be considered an index of synaptic function rather than a synaptic density measure. In addition to the presence of a stimulus during the scan, a recent unpublished study (Miranda et al., 2021) has also suggested an effect of anesthesia on the measure provided by SV2A PET tracers. Specifically, the use of prolonged isoflurane anesthesia seems to produce a significant increase in [18F]SynVesT-1 uptake, compared with quickly anesthetized or awake animals. These results highlight the importance of maintaining similar and stable conditions between and within subjects to avoid potential bias in the quantification of SV2A levels.
Finally, the availability, conformation, and electrostatic properties of SV2A vary across the exocytosis process (Lynch et al., 2008; Shi et al., 2011; Correa-Basurto et al., 2015). Further studies should be carried out to determine how these changes affect the ability of the different SV2A PET radiotracers to recognize and bind to this presynaptic protein.
While PET is the gold standard for in vivo molecular and metabolic imaging, it has the disadvantages of ionizing radiation, limited accessibility, and high cost. There are several MRI methods that provide cheaper, less invasive alternatives. For example, structural MRI can measure changes in cortical thickness as well as whole brain morphometry and regional deformations resulting from abnormal development or disease. However, structural MRI is neither specific nor direct and it does not have a resolution high enough to detect synapses per se, although some authors have attempted to connect regional variation of brain structures by MRI to the underlying synaptic spine densities (Keifer et al., 2015) or to plastic changes related to synaptic remodeling (Lerch et al., 2017). MR-based molecular imaging methods that can measure glutamatergic function may provide more promising indices of synaptic density.
MRI most commonly involves imaging 1H nuclei (i.e., protons), which in biological tissue are predominately found in water molecules. In contrast, MR spectroscopy (MRS) is a technique that can detect and quantify concentrations of various metabolites: the different protons of each metabolite have slightly different resonant frequencies and thus produce different peaks in an MR spectrum. But because these metabolites are found in much lower abundance than water, they produce much lower signal, limiting the spatial and temporal resolution of MRS techniques.
Chemical exchange saturation transfer (CEST) MRI is a method that overcomes the problem of low metabolite concentrations by exploiting the phenomenon of chemical exchange, whereby the protons of certain molecules exchange with those of water. The sensitivity of CEST MRI is increased by up to two orders of magnitude compared to MRS via continuous magnetic saturation of metabolite protons and subsequent exchange with bulk water protons over a period of seconds. This enables spatial mapping of metabolite concentrations at resolutions comparable to conventional MR imaging techniques. The basic principles of CEST MRI are covered in greater detail in a review by Wu et al. (2016).
Among the different CEST MRI methods, glutamate CEST or GluCEST is sensitive to changes in levels of glutamate, the main excitatory neurotransmitter in the brain, which can be detected by targeting the amine proton at an offset frequency of 3 ppm (Cai et al., 2012).
Previous studies using EM have shown that the highest levels of glutamate in the rat hippocampus are in the axon terminals of excitatory neurons (Bramham et al., 1990) and that glutamate levels correlate strongly with synaptic vesicle density (r = 0.94) in rat spinocerebellar mossy fiber terminals (Ji et al., 1991). However, the relationship between glutamate levels and synaptic density is not simple and can be altered in pathological conditions. For instance, a recently published study (Onwordi et al., 2021) showed that the correlation between the glutamate-to-creatine ratio measured by MRS and the [11C]UCB-J distributed volume ratio is only significant in healthy volunteers (hippocampus and anterior cingulate cortex) and not in patients with schizophrenia.
The relationship between glutamate levels and synaptic density has been further explored in animal models of neurodegeneration through the GluCEST sequence. For example, the GluCEST signal was associated with synaptophysin IHC in the PS19 mouse model of tauopathy, with both showing a decrease in the CA3 hippocampal region and the thalamus but not in the dentate gyrus or the entorhinal cortex (Crescenzi et al., 2014). GluCEST signal was also decreased in different mouse models of AD, such as the APP/PS1 and the 5xFAD models. In the APP/PS1 mouse, the GluCEST signal correlated with the glutamate-to-creatine ratio measured by MRS (Haris et al., 2013), while in the 5xFAD model, the decrease in glutamate was correlated with ELISA-based synaptophysin measurements [see Figure 5, extracted from Igarashi et al. (2020)]. This result suggests a relationship between the in vivo levels of glutamate and the ex vivo levels of synaptophysin, a presynaptic protein typically used as synaptic density marker.
Figure 5. Representative images of GluCEST MRI of a 5xFAD and a WT mouse. (A) Structural T2-weighted image (T2WI) in the coronal plane. (B) GluCEST maps of the corresponding T2WI showing reduced GluCEST effects in an aged (7-month old) 5xFAD mouse compared with the WT. (C) Correlation between GluCEST and Synaptophysin concentration. Figure extracted and modified from Igarashi et al. (2020).
A technical limitation of GluCEST, as with many MRI methods, is its molecular specificity. Cai et al. estimated that 70–75% of the GluCEST signal comes from glutamate and the remaining 25–30% from creatine, GABA, and other molecules (Cai et al., 2012). More recently, a simulation study reported that glutamate contributes to about 60% of the GluCEST signal at 3.2 ppm at 7T and neutral pH, with this contribution increasing with decreasing pH and increasing field strength (Khlebnikov et al., 2019). Thus, the specificity of GluCEST can be maximized in preclinical studies conducted at ultra-high field strengths.
Additionally, the target selected to evaluate synaptic density is a limitation itself: the quantification of glutamate produces a bias in the measurement of synaptic density, since it only considers the number of glutamatergic synapses, disregarding the existence of other synapses in the brain, such as inhibitory or electric synapses. To date, and despite the ability of MRI to provide a measure of synaptic function (i.e., with functional MRI or fMRI), there is no other MRI sequence able to provide a better measure of synaptic density. Therefore, further improvements and different targets are necessary to obtain a reliable MRI biomarker of changes in synaptic density.
The brain changes constantly throughout the lifespan. The pursuit of understanding this plasticity has always been at the forefront of the neuroscientific community, considered as the path to neuroscience’s holy grail: to fathom the biological reason behind our singularity. In this quest, synaptic density is one of the gateways toward this goal, since the synapse is the functional unit of the brain (Mayford et al., 2012). Over the years, various ex vivo and in vivo methods have been developed to measure changes in synaptic density, structure, and function, each one of them targeting different aspects of the synapse. Importantly, although various studies often conflate synaptic density and synaptic function (Chen M.K. et al., 2021; Raval et al., 2021a; van Aalst et al., 2021), we must bear in mind that these two are different — although related — concepts that may sometimes lead to different results (see Figure 6). This distinction is also reflected in the methodology employed to quantify synaptic density, predominantly carried out in post-mortem tissue (e.g., EM and IHC), whereas synaptic function is by default linked to experiments in living tissues, using electrophysiology, electroencephalography, or brain imaging with [18F]FDG PET and fMRI. Moreover, synaptic density appears to be a more stable biomarker than synaptic function which, due to the nature of the target measured (i.e., electrical activity, glucose and blood oxygenation), can be affected by both internal and external stimuli, such as tissue preparation for ex vivo assessments (Kirov et al., 1999) or anesthesia used during PET or MRI scanning (Fueger et al., 2006; Aksenov et al., 2015; Spangler-Bickell et al., 2016; Paasonen et al., 2018; van der Linden and Hoehn, 2022), as well as physiological fluctuations during live animal imaging (Harris et al., 2018; Steiner et al., 2020).
Figure 6. Representative brain images comparing synaptic density and function in the same rat. The images represent the uptake of two radiotracers: (A) [18F]UCB-H (concentration of SV2A protein, synaptic density marker) and (B) [18F]FDG (glucose metabolism, synaptic function marker). Both images were acquired 40-60 min after intravenous radiotracer injection (36 and 20 MBq, respectively). Scans were performed 24h apart, followed by a T2-weighted MR image to allow a better comparison between both PET scans through manual rigid-body co-registration with PMOD software. The white arrows represent the main differences in uptake between both radiotracers. While synaptic density and function are similar in multiple brain areas, regions such as the prefrontal cortex and cerebellum seem to have relatively higher glucose metabolism (synaptic function) than concentration of SV2A (synaptic density). Images obtained at GIGA-CRC in vivo imaging, ULiège (Belgium).
In this review, we have briefly presented the most commonly used methods for quantifying synaptic density, focusing on their applications in preclinical studies of neurodevelopmental and neurodegenerative disorders. Even though many of these methods lead to the same conclusion — the existence of degeneration, synaptic density loss or dendritic spine abnormalities in models of neurological diseases — all of them also have limitations (e.g., resolution, specificity, sensitivity, and complexity) that should be considered when interpreting the results (see Table 2). To overcome some of these limitations, alternatives are being explored, such as the combination of existing techniques, or the development of brand-new methods to quantify synaptic density.
With respect to the combined use of existing techniques, array tomography marries the ex vivo methods of IF and volumetric EM, enabling the quantification of precise molecular targets and the direct visualization of structural changes in synapses with unprecedented specificity and spatial resolution (Micheva and Smith, 2007; Prieto and Cotman, 2017). An example of combining in vivo methods is the use of PET and MRI techniques [e.g., (11C)UCB-J PET and fMRI], which may help shed light on the relationship between synaptic density and synaptic function. For example, a reduction in [11C]UCB-J binding has recently been associated with aberrant neural network function and inversely correlated with the depressive symptomatology in patients with major depressive disorder (Holmes et al., 2019).
Regarding the development of new methods to measure synaptic density, the use of genetically encoded fluorescent molecules as indicators of neuronal activity is particularly promising (Hamel et al., 2015; Lin and Schnitzer, 2016). These molecules, when examined with multiphoton microscopes (e.g., two-photon microscopy) (Lecoq et al., 2019), allow imaging of different processes involved in synaptic transmission in freely behaving animals, such as vesicle release (Sankaranarayanan et al., 2000; Ferro et al., 2017; Bensussen et al., 2020) or intracellular calcium dynamics (Chen et al., 2013; Ziv et al., 2013; Sheffield and Dombeck, 2015; Yang et al., 2016). These techniques, even though they offer the possibility of obtaining a high-resolution visualization of synaptic transmission elements, also suffer from limitations such as a narrow depth of field (1.2 mm) (Dunn and Sutton, 2008; Benninger and Piston, 2013). New technologies are quickly emerging to overcome these limitations, such as gradient-index (GRIN) lenses (Meng et al., 2019; Chien et al., 2021) and two-photon miniscopes (miniaturized head-mounted microscopes) (Ghosh et al., 2011; Silva, 2017; Zong et al., 2017, 2021). These technologies provide deeper and fast high-resolution volumetric images of dendrites and spines in freely moving animals, helping to better understand the functioning of the living brain.
Throughout this review we have explored different methods for quantifying synaptic density in preclinical research, focusing on their potential as biomarkers of neurological and psychiatric disorders. However, we should highlight the translatability of most of these methods, which are currently being used in clinical research. In this regard, EM and IHC have been extensively used to examine synaptic density in post-mortem human brain samples, with the purpose of mapping neuronal connectivity in healthy donors (Glantz et al., 2007; Kay et al., 2013; Lewis et al., 2019; Sherwood et al., 2020), or in the framework of understanding the pathology behind some of the disorders tackled in this review (Fourie et al., 2014; Osimo et al., 2019; Lauterborn et al., 2021). Furthermore, the recent emergence of multiple SV2A PET tracers has opened the door for the study of synaptic density in the living human brain, with exciting implications for clinical practice (Finnema et al., 2016). These radiotracers have already been used to explore changes in synaptic density in patients with epilepsy, AD, depression, schizophrenia, and other diseases, with promising results (Bao et al., 2017; Chen M.K. et al., 2018; Holmes et al., 2019; Finnema et al., 2020; Onwordi et al., 2020; Radhakrishnan et al., 2021). Therefore, although there are still some challenges to successfully employ synaptic density as a diagnostic and/or prognostic biomarker for neurological and neuropsychiatric disorders, current methods of measuring synaptic density can help us understand both healthy and disordered brain development and function.
MS, MP, and DC contributed to the conception and design of the review. MS and EK wrote the first draft of the manuscript. MP, FT, and DC contributed to manuscript editing and revision. All authors read and approved the submitted version.
This research was funded by the Wellcome Trust (WT203148/Z/16/Z) to MS.
The authors declare that the research was conducted in the absence of any commercial or financial relationships that could be construed as a potential conflict of interest.
All claims expressed in this article are solely those of the authors and do not necessarily represent those of their affiliated organizations, or those of the publisher, the editors and the reviewers. Any product that may be evaluated in this article, or claim that may be made by its manufacturer, is not guaranteed or endorsed by the publisher.
We would like to thank Katarina Ilic and Nuria García Font for providing Figure 1 (created with BioRender software) and Figure 2, respectively. We are also grateful to Jean-Jacques Goval, and the GIGA-Imaging platform for their help and support in the process of obtaining Figure 3. Finally, we would like to acknowledge the contribution of Nucleis ULiège spin-off [(18F)FDG and (18F)UCB-H production] and the GIGA-CRC in vivo imaging (PET and MRI) facility in the obtention of Figure 5.
Acton, P. D., Zhuang, H., and Alavi, A. (2004). Quantification in PET. Radiol. Clin. North Am. 42, 1055–1062. doi: 10.1016/j.rcl.2004.08.010
Aksenov, D. P., Li, L., Miller, M. J., Iordanescu, G., and Wyrwicz, A. M. (2015). Effects of anesthesia on BOLD signal and neuronal activity in the somatosensory cortex. J. Cerebral Blood Flow Metab. 35, 1819–1826. doi: 10.1038/jcbfm.2015.130
Ali, F., Gerhard, D. M., Sweasy, K., Pothula, S., Pittenger, C., Duman, R. S., et al. (2020). Ketamine disinhibits dendrites and enhances calcium signals in prefrontal dendritic spines. Nat. Commun. 11:72. doi: 10.1038/s41467-019-13809-8
Antonucci, F., Corradini, I., Fossati, G., Tomasoni, R., Menna, E., and Matteoli, M. (2016). SNAP-25, a known presynaptic protein with emerging postsynaptic functions. Front. Syn. Neurosci. 8:e07. doi: 10.3389/fnsyn.2016.00007
Ayberk Kurt, M. Ilker Kafa, M., and Dierssen, M. Ceri Davies, D. (2004). Deficits of neuronal density in CA1 and synaptic density in the dentate gyrus, CA3 and CA1, in a mouse model of Down syndrome. Brain Res. 1022, 101–109. doi: 10.1016/j.brainres.2004.06.075
Ba̧czyńska, E., Pels, K. K., Basu, S., Włodarczyk, J., and Ruszczycki, B. (2021). Quantification of dendritic spines remodeling under physiological stimuli and in pathological conditions. Int. J. Mol. Sci. 22:4053. doi: 10.3390/ijms22084053
Bao, W., Jia, H., Finnema, S., Cai, Z., Carson, R. E., and Huang, Y. H. (2017). PET imaging for early detection of Alzheimer’s disease. PET Clin. 12, 329–350. doi: 10.1016/j.cpet.2017.03.001
Bartholome, O., van den Ackerveken, P., Sánchez Gil, J., de la Brassinne Bonardeaux, O., Leprince, P., Franzen, R., et al. (2017). Puzzling out synaptic vesicle 2 family members functions. Front. Mol. Neurosci. 10:148. doi: 10.3389/fnmol.2017.00148
Basu, S., Saha, P. K., Roszkowska, M., Magnowska, M., Baczynska, E., Das, N., et al. (2018). Quantitative 3-D morphometric analysis of individual dendritic spines. Sci. Rep. 8:3545. doi: 10.1038/s41598-018-21753-8
Becker, G., Warnier, C., Serrano, M. E., Bahri, M. A., Mercier, J., Lemaire, C., et al. (2017). Pharmacokinetic characterization of [18 F]UCB-H PET radiopharmaceutical in the rat brain. Mol. Pharmaceutics 14, 2719–2725. doi: 10.1021/acs.molpharmaceut.7b00235
Belichenko, P. V., Kleschevnikov, A. M., Salehi, A., Epstein, C. J., and Mobley, W. C. (2007). Synaptic and cognitive abnormalities in mouse models of down syndrome: exploring genotype-phenotype relationships. J. Comparat. Neurol. 504, 329–345. doi: 10.1002/cne.21433
Belichenko, P. V., Masliah, E., Kleschevnikov, A. M., Villar, A. J., Epstein, C. J., Salehi, A., et al. (2004). Synaptic structural abnormalities in the Ts65Dn mouse model of down syndrome. J. Comparat. Neurol. 480, 281–298. doi: 10.1002/cne.20337
Belthangady, C., and Royer, L. A. (2019). Applications, promises, and pitfalls of deep learning for fluorescence image reconstruction. Nat. Methods 16, 1215–1225. doi: 10.1038/s41592-019-0458-z
Benninger, R. K. P., and Piston, D. W. (2013). Two-photon excitation microscopy for the study of living cells and tissues. Curr. Protocols Cell Biol. 59:411. doi: 10.1002/0471143030.cb0411s59
Bensussen, S., Shankar, S., Ching, K. H., Zemel, D., Ta, T. L., Mount, R. A., et al. (2020). A viral toolbox of genetically encoded fluorescent synaptic tags. iScience 23:101330. doi: 10.1016/j.isci.2020.101330
Berdenis van Berlekom, A., Muflihah, C. H., Snijders, G. J. L. J., MacGillavry, H. D., Middeldorp, J., Hol, E. M., et al. (2020). Synapse pathology in schizophrenia: a meta-analysis of postsynaptic elements in postmortem brain studies. Schizophr. Bull. 46, 374–386. doi: 10.1093/schbul/sbz060
Binda, K. H., Lillethorup, T. P., Real, C. C., Bærentzen, S. L., Nielsen, M. N., Orlowski, D., et al. (2021). Exercise protects synaptic density in a rat model of Parkinson’s disease. Exp. Neurol. 342:113741. doi: 10.1016/j.expneurol.2021.113741
Blanpied, T. A., and Ehlers, M. D. (2004). Microanatomy of dendritic spines: emerging principles of synaptic pathology in psychiatric and neurological disease. Biol. Psychiatry 55, 1121–1127. doi: 10.1016/j.biopsych.2003.10.006
Bock, J., Murmu, M. S., Biala, Y., Weinstock, M., and Braun, K. (2011). Prenatal stress and neonatal handling induce sex-specific changes in dendritic complexity and dendritic spine density in hippocampal subregions of prepubertal rats. Neuroscience 193, 34–43. doi: 10.1016/j.neuroscience.2011.07.048
Bogen, I. L., Haug, K. H., Roberg, B., Fonnum, F., and Walaas, S. I. (2009). The importance of synapsin I and II for neurotransmitter levels and vesicular storage in cholinergic, glutamatergic and GABAergic nerve terminals. Neurochem. Int. 55, 13–21. doi: 10.1016/j.neuint.2009.02.006
Borczyk, M., Śliwińska, M. A., Caly, A., Bernas, T., and Radwanska, K. (2019). Neuronal plasticity affects correlation between the size of dendritic spine and its postsynaptic density. Sci. Rep. 9:1693. doi: 10.1038/s41598-018-38412-7
Bosch, C., Masachs, N., Exposito-Alonso, D., Martínez, A., Teixeira, C. M., Fernaud, I., et al. (2016). Reelin regulates the maturation of dendritic spines, synaptogenesis and glial ensheathment of newborn granule cells. Cerebral Cortex 26, 4282–4298. doi: 10.1093/cercor/bhw216
Bragina, L., Fattorini, G., Giovedí, S., Melone, M., Bosco, F., Benfenati, F., et al. (2012). Analysis of synaptotagmin, SV2, and Rab3 expression in cortical glutamatergic and GABAergic axon terminals. Front. Cell. Neurosci. 5:1–9. doi: 10.3389/fncel.2011.00032
Bramham, C. R., Torp, R., Zhang, N., Storm-Mathisen, J., and Ottersen, O. P. (1990). Distribution of glutamate-like immunoreactivity in excitatory hippocampal pathways: a semiquantitative electron microscopic study in rats. Neuroscience 39, 405–417. doi: 10.1016/0306-4522(90)90277-B
Bretin, F., Warnock, G., Bahri, M. A., Aerts, J., Mestdagh, N., Buchanan, T., et al. (2013). Preclinical radiation dosimetry for the novel SV2A radiotracer [18F]UCB-H. EJNMMI Res. 3:35. doi: 10.1186/2191-219X-3-35
Brioschi, S., D’Errico, P., Amann, L. S., Janova, H., Wojcik, S. M., Meyer-Luehmann, M., et al. (2020). Detection of synaptic proteins in microglia by flow cytometry. Front. Mol. Neurosci. 13:149. doi: 10.3389/fnmol.2020.00149
Cai, H., Mangner, T. J., Muzik, O., Wang, M.-W., Chugani, D. C., and Chugani, H. T. (2014). Radiosynthesis of 11C-levetiracetam: a potential marker for PET imaging of SV2A expression. ACS Med. Chem. Lett. 5, 1152–1155. doi: 10.1021/ml500285t
Cai, K., Haris, M., Singh, A., Kogan, F., Greenberg, J. H., Hariharan, H., et al. (2012). Magnetic resonance imaging of glutamate. Nat. Med. 18, 302–306. doi: 10.1038/nm.2615
Cai, Z., Li, S., Matuskey, D., Nabulsi, N., and Huang, Y. (2019). PET imaging of synaptic density: a new tool for investigation of neuropsychiatric diseases. Neurosci. Lett. 691, 44–50. doi: 10.1016/j.neulet.2018.07.038
Cai, Z., Li, S., Zhang, W., Pracitto, R., Wu, X., Baum, E., et al. (2020). Synthesis and preclinical evaluation of an 18 F-labeled synaptic vesicle glycoprotein 2A PET imaging probe: [18 F]SynVesT-2. ACS Chem. Neurosci. 11, 592–603. doi: 10.1021/acschemneuro.9b00618
Caire, M. J., Reddy, V., and Varacallo, M. (2021). Physiology, Synapse. Available online at: http://www.ncbi.nlm.nih.gov/pubmed/30252303 (accessed December 9, 2021).
Calì, C., Wawrzyniak, M., Becker, C., Maco, B., Cantoni, M., Jorstad, A., et al. (2018). The effects of aging on neuropil structure in mouse somatosensory cortex—A 3D electron microscopy analysis of layer 1. PLoS One 13:e0198131. doi: 10.1371/journal.pone.0198131
Cardozo, P. L., de Lima, I. B. Q., Maciel, E. M. A., Silva, N. C., Dobransky, T., and Ribeiro, F. M. (2019). Synaptic elimination in neurological disorders. Curr. Neuropharmacol. 17, 1071–1095. doi: 10.2174/1570159X17666190603170511
Cesca, F., Baldelli, P., Valtorta, F., and Benfenati, F. (2010). The synapsins: key actors of synapse function and plasticity. Prog. Neurobiol. 91, 313–348. doi: 10.1016/j.pneurobio.2010.04.006
Chaudhry, F. A., Reimer, R. J., Bellocchio, E. E., Danbolt, N. C., Osen, K. K., Edwards, R. H., et al. (1998). The vesicular GABA transporter, VGAT, localizes to synaptic vesicles in sets of glycinergic as well as GABAergic neurons. J. Neurosci. 18, 9733–9750. doi: 10.1523/JNEUROSCI.18-23-09733.1998
Chen, M.-K., Mecca, A. P., Naganawa, M., Finnema, S. J., Toyonaga, T., Lin, S., et al. (2018). Assessing synaptic density in alzheimer disease with synaptic vesicle glycoprotein 2A positron emission tomographic imaging. JAMA Neurol. 75:1215. doi: 10.1001/jamaneurol.2018.1836
Chen, M.-K., Mecca, A. P., Naganawa, M., Gallezot, J.-D., Toyonaga, T., Mondal, J., et al. (2021). Comparison of [11 C]UCB-J and [18 F]FDG PET in Alzheimer’s disease: a tracer kinetic modeling study. J. Cerebral Blood Flow Metab. 41, 2395–2409. doi: 10.1177/0271678X211004312
Chen, P., Jing, H., Xiong, M., Zhang, Q., Lin, D., Ren, D., et al. (2021). Spine impairment in mice high-expressing neuregulin 1 due to LIMK1 activation. Cell Death Dis. 12:403. doi: 10.1038/s41419-021-03687-8
Chen, T.-W., Wardill, T. J., Sun, Y., Pulver, S. R., Renninger, S. L., Baohan, A., et al. (2013). Ultrasensitive fluorescent proteins for imaging neuronal activity. Nature 499, 295–300. doi: 10.1038/nature12354
Cheng, C., Trzcinski, O., and Doering, L. C. (2014). Fluorescent labeling of dendritic spines in cell cultures with the carbocyanine dye “DiI. Front. Neuroanat. 8:30. doi: 10.3389/fnana.2014.00030
Chien, Y.-F., Lin, J.-Y., Yeh, P.-T., Hsu, K.-J., Tsai, Y.-H., Chen, S.-K., et al. (2021). Dual GRIN lens two-photon endoscopy for high-speed volumetric and deep brain imaging. Biomed. Optics Exp. 12:162. doi: 10.1364/BOE.405738
Choii, G., and Ko, J. (2015). Gephyrin: a central GABAergic synapse organizer. Exp. Mol. Med. 47:e158. doi: 10.1038/emm.2015.5
Clare, R., King, V. G., Wirenfeldt, M., and Vinters, H. V. (2010). Synapse loss in dementias. J. Neurosci. Res. 88, 2083–2090. doi: 10.1002/jnr.22392
Collman, F., Buchanan, J., Phend, K. D., Micheva, K. D., Weinberg, R. J., and Smith, S. J. (2015). Mapping synapses by conjugate light-electron array tomography. J. Neurosci. 35, 5792–5807. doi: 10.1523/JNEUROSCI.4274-14.2015
Colom-Cadena, M., Spires-Jones, T., Zetterberg, H., Blennow, K., Caggiano, A., DeKosky, S. T., et al. (2020). The clinical promise of biomarkers of synapse damage or loss in Alzheimer’s disease. Alzheimer’s Res. Ther. 12:21. doi: 10.1186/s13195-020-00588-4
Connors, B. W., and Long, M. A. (2004). Electrical synapses in the mammalian brain. Ann. Rev. Neurosci. 27, 393–418. doi: 10.1146/annurev.neuro.26.041002.131128
Constantinescu, C. C., Tresse, C., Zheng, M., Gouasmat, A., Carroll, V. M., Mistico, L., et al. (2019). Development and in vivo preclinical imaging of fluorine-18-labeled synaptic vesicle protein 2A (SV2A) PET tracers. Mol. Imag. Biol. 21, 509–518. doi: 10.1007/s11307-018-1260-5
Contreras-García, I. J., Pichardo-Macías, L. A., Santana-Gómez, C. E., Sánchez-Huerta, K., Ramírez-Hernández, R., Gómez-González, B., et al. (2018). Differential expression of synaptic vesicle protein 2A after status epilepticus and during epilepsy in a lithium-pilocarpine model. Epilepsy Behavior 88, 283–294. doi: 10.1016/j.yebeh.2018.08.023
Correa-Basurto, J., Cuevas-Hernández, R. I., Phillips-Farfán, B. V., Martínez-Archundia, M., Romo-Mancillas, A., Ramírez-Salinas, G. L., et al. (2015). Identification of the antiepileptic racetam binding site in the synaptic vesicle protein 2A by molecular dynamics and docking simulations. Front. Cell. Neurosci. 9:125. doi: 10.3389/fncel.2015.00125
Cortès-Saladelafont, E., Tristán-Noguero, A., Artuch, R., Altafaj, X., Bayès, A., and García-Cazorla, A. (2016). Diseases of the synaptic vesicle: a potential new group of neurometabolic disorders affecting neurotransmission. Seminars Pediatric Neurol. 23, 306–320. doi: 10.1016/j.spen.2016.11.005
Crescenzi, R., DeBrosse, C., Nanga, R. P. R., Reddy, S., Haris, M., Hariharan, H., et al. (2014). In vivo measurement of glutamate loss is associated with synapse loss in a mouse model of tauopathy. NeuroImage 101, 185–192. doi: 10.1016/j.neuroimage.2014.06.067
Crippa, D., Schenk, U., Francolini, M., Rosa, P., Verderio, C., Zonta, M., et al. (2006). Synaptobrevin2-expressing vesicles in rat astrocytes: insights into molecular characterization, dynamics and exocytosis. J. Physiol. 570, 567–582. doi: 10.1113/jphysiol.2005.094052
Crowder, K. M., Gunther, J. M., Jones, T. A., Hale, B. D., Zhang, H. Z., Peterson, M. R., et al. (1999). Abnormal neurotransmission in mice lacking synaptic vesicle protein 2A (SV2A). Proc. Natl. Acad. Sci. U.S.A. 96, 15268–15273. doi: 10.1073/pnas.96.26.15268
D’Amico, F., and Skarmoutsou, E. (2008). Quantifying immunogold labelling in transmission electron microscopy. J. Microscopy 230, 9–15. doi: 10.1111/j.1365-2818.2008.01949.x
D’Souza, D. C., Radhakrishnan, R., Naganawa, M., Ganesh, S., Nabulsi, N., Najafzadeh, S., et al. (2020). Preliminary in vivo evidence of lower hippocampal synaptic density in cannabis use disorder. Mol. Psychiatry 26, 3192–3200. doi: 10.1038/s41380-020-00891-4
Dawirs, R. R., Teuchert-Noodt, G., and Kacza, J. (1992). Naturally occurring degrading events in axon terminals of the dentate gyrus and stratum lucidum in the spiny mouse (acomys cahirinus) during maturation, adulthood and aging. Dev. Neurosci. 14, 210–220. doi: 10.1159/000111665
de Bartolomeis, A., and Fiore, G. (2004). Postsynaptic density scaffolding proteins at excitatory synapse and disorders of synaptic plasticity: implications for human behavior pathologies. Int. Rev Neurobiol. 59, 221–254. doi: 10.1016/S0074-7742(04)59009-8
de Wilde, M. C., Overk, C. R., Sijben, J. W., and Masliah, E. (2016). Meta-analysis of synaptic pathology in Alzheimer’s disease reveals selective molecular vesicular machinery vulnerability. Alzheimer’s Dementia 12, 633–644. doi: 10.1016/j.jalz.2015.12.005
Dickstein, D. L., Dickstein, D. R., Janssen, W. G. M., Hof, P. R., Glaser, J. R., Rodriguez, A., et al. (2016). Automatic dendritic spine quantification from confocal data with Neurolucida 360. Curr. Protoc. Neurosci. 77, 1.27.1–1.27.21. doi: 10.1002/cpns.16
Dieck, S., Sanmartí-Vila, L., Langnaese, K., Richter, K., Kindler, S., Soyke, A., et al. (1998). Bassoon, a novel zinc-finger CAG/glutamine-repeat protein selectively localized at the active zone of presynaptic nerve terminals. J. Cell Biol. 142, 499–509. doi: 10.1083/jcb.142.2.499
DiFiglia, M., Schiff, L., and Deckel, A. (1988). Neuronal organization of fetal striatal grafts in kainate- and sham- lesioned rat caudate nucleus: light- and electron-microscopic observations. J. Neurosci. 8, 1112–1130. doi: 10.1523/JNEUROSCI.08-04-01112.1988
Dong, H., Martin, M. V., Chambers, S., and Csernansky, J. G. (2007). Spatial relationship between synapse loss and β-amyloid deposition in Tg2576 mice. J. Comparat. Neurol. 500, 311–321. doi: 10.1002/cne.21176
Dorostkar, M. M., Zou, C., Blazquez-Llorca, L., and Herms, J. (2015). Analyzing dendritic spine pathology in Alzheimer’s disease: problems and opportunities. Acta Neuropathol. 130, 1–19. doi: 10.1007/s00401-015-1449-5
Duman, C. H., and Duman, R. S. (2015). Spine synapse remodeling in the pathophysiology and treatment of depression. Neurosci. Lett. 601, 20–29. doi: 10.1016/j.neulet.2015.01.022
Dunn, K. W., and Sutton, T. A. (2008). Functional studies in living animals using multiphoton microscopy. ILAR J. 49, 66–77. doi: 10.1093/ilar.49.1.66
Durand, C. M., Perroy, J., Loll, F., Perrais, D., Fagni, L., Bourgeron, T., et al. (2012). SHANK3 mutations identified in autism lead to modification of dendritic spine morphology via an actin-dependent mechanism. Mol. Psychiatry 17, 71–84. doi: 10.1038/mp.2011.57
Estrada, S., Lubberink, M., Thibblin, A., Sprycha, M., Buchanan, T., Mestdagh, N., et al. (2016). [11C]UCB-A, a novel PET tracer for synaptic vesicle protein 2 A. Nuclear Med. Biol. 43, 325–332. doi: 10.1016/j.nucmedbio.2016.03.004
Ettcheto, M., Busquets, O., Cano, A., Sánchez-Lopez, E., Manzine, P. R., Espinosa-Jimenez, T., et al. (2020). Pharmacological strategies to improve dendritic spines in Alzheimer’s disease. J. Alzheimer’s Dis. 82, S91–S107. doi: 10.3233/JAD-201106
Fairén, A. (2005). Pioneering a golden age of cerebral microcircuits: the births of the combined golgi–electron microscope methods. Neuroscience 136, 607–614. doi: 10.1016/j.neuroscience.2005.08.011
Fan, W.-J., Yan, M.-C., Wang, L., Sun, Y.-Z., Deng, J.-B., and Deng, J.-X. (2018). Synaptic aging disrupts synaptic morphology and function in cerebellar purkinje cells. Neural Regeneration Res. 13:1019. doi: 10.4103/1673-5374.233445
Favuzzi, E., and Rico, B. (2018). Molecular diversity underlying cortical excitatory and inhibitory synapse development. Curr. Opin. Neurobiol. 53, 8–15. doi: 10.1016/j.conb.2018.03.011
Feng, G., Mellor, R. H., Bernstein, M., Keller-Peck, C., Nguyen, Q. T., Wallace, M., et al. (2000). Imaging neuronal subsets in transgenic mice expressing multiple spectral variants of GFP. Neuron 28, 41–51. doi: 10.1016/S0896-6273(00)00084-2
Fernández-Pérez, E. J., Gallegos, S., Armijo-Weingart, L., Araya, A., Riffo-Lepe, N. O., Cayuman, F., et al. (2020). Changes in neuronal excitability and synaptic transmission in nucleus accumbens in a transgenic Alzheimer’s disease mouse model. Sci. Rep. 10:19606. doi: 10.1038/s41598-020-76456-w
Ferro, M., Lamanna, J., Ripamonti, M., Racchetti, G., Arena, A., Spadini, S., et al. (2017). Functional mapping of brain synapses by the enriching activity-marker SynaptoZip. Nat. Commun. 8:1229. doi: 10.1038/s41467-017-01335-4
Fiala, J. C., Spacek, J., and Harris, K. M. (2002). Dendritic spine pathology: cause or consequence of neurological disorders? Brain Res. Rev. 39, 29–54. doi: 10.1016/S0165-0173(02)00158-3
Finnema, S. J., Nabulsi, N. B., Eid, T., Detyniecki, K., Lin, S.-F., Chen, M.-K., et al. (2016). Imaging synaptic density in the living human brain. Sci. Trans. Med. 8:348ra96. doi: 10.1126/scitranslmed.aaf6667
Finnema, S. J., Toyonaga, T., Detyniecki, K., Chen, M., Dias, M., Wang, Q., et al. (2020). Reduced synaptic vesicle protein 2A binding in temporal lobe epilepsy: a [11 C]UCB-J positron emission tomography study. Epilepsia 61, 2183–2193. doi: 10.1111/epi.16653
Fogarty, M. (2019). Amyotrophic lateral sclerosis as a synaptopathy. Neural Regeneration Res. 14:189. doi: 10.4103/1673-5374.244782
Fortin, D. A., Srivastava, T., and Soderling, T. R. (2012). Structural modulation of dendritic spines during synaptic plasticity. Neuroscientist 18, 326–341. doi: 10.1177/1073858411407206
Fourie, C., Kim, E., Waldvogel, H., Wong, J. M., McGregor, A., Faull, R. L. M., et al. (2014). Differential changes in postsynaptic density proteins in postmortem huntington’s disease and Parkinson’s disease human brains. J. Neurodegener. Dis. 2014, 1–14. doi: 10.1155/2014/938530
Fox, M. E., Figueiredo, A., Menken, M. S., and Lobo, M. K. (2020). Dendritic spine density is increased on nucleus accumbens D2 neurons after chronic social defeat. Sci. Rep. 10:12393. doi: 10.1038/s41598-020-69339-7
Fremeau, R. T., Voglmaier, S., Seal, R. P., and Edwards, R. H. (2004). VGLUTs define subsets of excitatory neurons and suggest novel roles for glutamate. Trends Neurosci. 27, 98–103. doi: 10.1016/j.tins.2003.11.005
Fueger, B. J., Czernin, J., Hildebrandt, I., Tran, C., Halpern, B. S., Stout, D., et al. (2006). Impact of animal handling on the results of 18F-FDG PET studies in mice. J. Nuclear Med. Off. Publi. Soc. Nuclear Med. 47, 999–1006.
Galarreta, M., and Hestrin, S. (2001). Electrical synapses between gaba-releasing interneurons. Nat. Rev. Neurosci. 2, 425–433. doi: 10.1038/35077566
Gan, W.-B., Grutzendler, J., Wong, W. T., Wong, R. O. L., and Lichtman, J. W. (2000). Multicolor “DiOlistic” labeling of the nervous system using lipophilic dye combinations. Neuron 27, 219–225. doi: 10.1016/S0896-6273(00)00031-3
García-Font, N., Martín, R., Torres, M., Oset-Gasque, M. J., and Sánchez-Prieto, J. (2019). The loss of β adrenergic receptor mediated release potentiation in a mouse model of fragile X syndrome. Neurobiol. Dis. 130:104482. doi: 10.1016/j.nbd.2019.104482
Garré, J. M., Silva, H. M., Lafaille, J. J., and Yang, G. (2017). CX3CR1+ monocytes modulate learning and learning-dependent dendritic spine remodeling via TNF-α. Nat. Med. 23, 714–722. doi: 10.1038/nm.4340
Germann, M., Brederoo, S. G., and Sommer, I. E. C. (2021). Abnormal synaptic pruning during adolescence underlying the development of psychotic disorders. Curr. Opin. Psychiatry 34, 222–227. doi: 10.1097/YCO.0000000000000696
Ghosh, K. K., Burns, L. D., Cocker, E. D., Nimmerjahn, A., Ziv, Y., Gamal, A., et al. (2011). Miniaturized integration of a fluorescence microscope. Nat. Methods 8, 871–878. doi: 10.1038/nmeth.1694
Gigg, J., McEwan, F., Smausz, R., Neill, J., and Harte, M. K. (2020). Synaptic biomarker reduction and impaired cognition in the sub-chronic PCP mouse model for schizophrenia. J. Psychopharmacol. 34, 115–124. doi: 10.1177/0269881119874446
Gillard, M., Fuks, B., Michel, P., Vertongen, P., Massingham, R., and Chatelain, P. (2003). Binding characteristics of [3H]ucb 30889 to levetiracetam binding sites in rat brain. Eur. J. Pharmacol. 478, 1–9. doi: 10.1016/j.ejphar.2003.08.032
Glantz, L. A., Gilmore, J. H., Hamer, R. M., Lieberman, J. A., and Jarskog, L. F. (2007). Synaptophysin and postsynaptic density protein 95 in the human prefrontal cortex from mid-gestation into early adulthood. Neuroscience 149, 582–591. doi: 10.1016/j.neuroscience.2007.06.036
Glorie, D., Verhaeghe, J., Miranda, A., de Lombaerde, S., Stroobants, S., and Staelens, S. (2020). Sapap3 deletion causes dynamic synaptic density abnormalities: a longitudinal [11C]UCB-J PET study in a model of obsessive–compulsive disorder-like behaviour. EJNMMI Res. 10:140. doi: 10.1186/s13550-020-00721-2
Gómez-Gaviro, M. V., Sanderson, D., Ripoll, J., and Desco, M. (2020). Biomedical applications of tissue clearing and three-dimensional imaging in health and disease. iScience 23:101432. doi: 10.1016/j.isci.2020.101432
Grant, S. G. (2012). Synaptopathies: diseases of the synaptome. Curr. Opin. Neurobiol. 22, 522–529. doi: 10.1016/j.conb.2012.02.002
Gray, E. G. (1959). Electron microscopy of synaptic contacts on dendrite spines of the cerebral cortex. Nature 183, 1592–1593. doi: 10.1038/1831592a0
Griem-Krey, N., Klein, A. B., Herth, M., and Wellendorph, P. (2019). Autoradiography as a simple and powerful method for visualization and characterization of pharmacological targets. J. Vis. Exp. 145. doi: 10.3791/58879
Gu, J., Firestein, B. L., and Zheng, J. Q. (2008). Microtubules in dendritic spine development. J. Neurosci. 28, 12120–12124. doi: 10.1523/JNEUROSCI.2509-08.2008
Guirado, R., Carceller, H., Castillo-Gómez, E., Castrén, E., and Nacher, J. (2018). Automated analysis of images for molecular quantification in immunohistochemistry. Heliyon 4:e00669. doi: 10.1016/j.heliyon.2018.e00669
Gundelfinger, E. D., Reissner, C., and Garner, C. C. (2016). Role of bassoon and piccolo in assembly and molecular organization of the active zone. Front. Syn. Neurosci. 7:19. doi: 10.3389/fnsyn.2015.00019
Guo, B., Chen, J., Chen, Q., Ren, K., Feng, D., Mao, H., et al. (2019). Anterior cingulate cortex dysfunction underlies social deficits in Shank3 mutant mice. Nat. Neurosci. 22, 1223–1234. doi: 10.1038/s41593-019-0445-9
Guo, D., Arnspiger, S., Rensing, N. R., and Wong, M. (2012). Brief seizures cause dendritic injury. Neurobiol. Dis. 45, 348–355. doi: 10.1016/j.nbd.2011.08.020
Halff, E. F., Cotel, M.-C., Natesan, S., McQuade, R., Ottley, C. J., Srivastava, D. P., et al. (2021). Effects of chronic exposure to haloperidol, olanzapine or lithium on SV2A and NLGN synaptic puncta in the rat frontal cortex. Behav. Brain Res. 405:113203. doi: 10.1016/j.bbr.2021.113203
Hama, H., Kurokawa, H., Kawano, H., Ando, R., Shimogori, T., Noda, H., et al. (2011). Scale: a chemical approach for fluorescence imaging and reconstruction of transparent mouse brain. Nat. Neurosci. 14, 1481–1488. doi: 10.1038/nn.2928
Hamel, E. J. O., Grewe, B. F., Parker, J. G., and Schnitzer, M. J. (2015). Cellular level brain imaging in behaving mammals: an engineering approach. Neuron 86, 140–159. doi: 10.1016/j.neuron.2015.03.055
Hanaya, R., Hosoyama, H., Sugata, S., Tokudome, M., Hirano, H., Tokimura, H., et al. (2012). Low distribution of synaptic vesicle protein 2A and synaptotagimin-1 in the cerebral cortex and hippocampus of spontaneously epileptic rats exhibiting both tonic convulsion and absence seizure. Neuroscience 221, 12–20. doi: 10.1016/j.neuroscience.2012.06.058
Haris, M., Nath, K., Cai, K., Singh, A., Crescenzi, R., Kogan, F., et al. (2013). Imaging of glutamate neurotransmitter alterations in Alzheimer’s disease. NMR Biomed. 26, 386–391. doi: 10.1002/nbm.2875
Harris, K. M., and Weinberg, R. J. (2012). Ultrastructure of synapses in the mammalian brain. Cold Spring Harbor Perspect. Biol. 4:a005587. doi: 10.1101/cshperspect.a005587
Harris, S. S., Boorman, L. W., Das, D., Kennerley, A. J., Sharp, P. S., Martin, C., et al. (2018). Physiological and pathological brain activation in the anesthetized rat produces hemodynamic-dependent cortical temperature increases that can confound the BOLD fMRI signal. Front. Neurosci. 12:550. doi: 10.3389/fnins.2018.00550
Herms, J., and Dorostkar, M. M. (2016). Dendritic spine pathology in neurodegenerative diseases. Ann. Rev. Pathol. Mech. Dis. 11, 221–250. doi: 10.1146/annurev-pathol-012615-044216
Heshmati, M., Christoffel, D. J., LeClair, K., Cathomas, F., Golden, S. A., Aleyasin, H., et al. (2020). Depression and social defeat stress are associated with inhibitory synaptic changes in the nucleus accumbens. J. Neurosci. 40, 6228–6233. doi: 10.1523/JNEUROSCI.2568-19.2020
Heurling, K., Ashton, N. J., Leuzy, A., Zimmer, E. R., Blennow, K., Zetterberg, H., et al. (2019). Synaptic vesicle protein 2A as a potential biomarker in synaptopathies. Mol. Cell. Neurosci. 97, 34–42. doi: 10.1016/j.mcn.2019.02.001
Holland, N., Jones, P. S., Savulich, G., Wiggins, J. K., Hong, Y. T., Fryer, T. D., et al. (2020). Synaptic loss in primary tauopathies revealed by [11C]UCB-J positron emission tomography. Mov. Dis. 35, 1834–1842. doi: 10.1002/mds.28188
Holland, N., Malpetti, M., Rittman, T., Mak, E. E., Passamonti, L., Kaalund, S. S., et al. (2021). Molecular pathology and synaptic loss in primary tauopathies: an 18F-AV-1451 and 11C-UCB-J PET study. Brain. [Epub ahead of print], doi: 10.1093/brain/awab282
Holmes, S. E., Scheinost, D., Finnema, S. J., Naganawa, M., Davis, M. T., DellaGioia, N., et al. (2019). Lower synaptic density is associated with depression severity and network alterations. Nat. Commun. 10:1529. doi: 10.1038/s41467-019-09562-7
Hotulainen, P., and Hoogenraad, C. C. (2010). Actin in dendritic spines: connecting dynamics to function. J. Cell Biol. 189, 619–629. doi: 10.1083/jcb.201003008
Hu, X., Luo, J., and Xu, J. (2015). The interplay between synaptic activity and neuroligin function in the CNS. BioMed Res. Int. 2015, 1–13. doi: 10.1155/2015/498957
Hussain, S., Ringsevjen, H., Schupp, M., Hvalby, Ø, Sørensen, J. B., Jensen, V., et al. (2019). A possible postsynaptic role for SNAP-25 in hippocampal synapses. Brain Struct. Function 224, 521–532. doi: 10.1007/s00429-018-1782-2
Igarashi, H., Ueki, S., Kitaura, H., Kera, T., Ohno, K., Ohkubo, M., et al. (2020). Longitudinal GluCEST MRI changes and cerebral blood flow in 5xFAD mice. Contrast Med. Mol. Imaging 2020, 1–12. doi: 10.1155/2020/8831936
Iijima, K., Oshima, T., Kawakami, R., and Nemoto, T. (2021). Optical clearing of living brains with MAGICAL to extend in vivo imaging. iScience 24:101888. doi: 10.1016/j.isci.2020.101888
Imbriani, P., Schirinzi, T., Meringolo, M., Mercuri, N. B., and Pisani, A. (2018). Centrality of early synaptopathy in Parkinson’s disease. Front. Neurol. 9:103. doi: 10.3389/fneur.2018.00103
Ippolito, D. M., and Eroglu, C. (2010). Quantifying synapses: an immunocytochemistry-based assay to quantify synapse number. J. Vis. Exp. 45:2270. doi: 10.3791/2270
Irfan, M., Gopaul, K. R., Miry, O., Hökfelt, T., Stanton, P. K., and Bark, C. (2019). SNAP-25 isoforms differentially regulate synaptic transmission and long-term synaptic plasticity at central synapses. Sci. Rep. 9:6403. doi: 10.1038/s41598-019-42833-3
Ishiwata, K., Ogi, N., Tanaka, A., and Senda, M. (1999). Quantitative ex vivo and in vitro receptor autoradiography using 11C-labeled ligands and an imaging plate: a study with a dopamine D2-like receptor ligand [11C]nemonapride. Nuclear Med. Biol. 26, 291–296. doi: 10.1016/S0969-8051(98)00115-2
Isokawa, M. (1998). Remodeling dendritic spines in the rat pilocarpine model of temporal lobe epilepsy. Neurosci. Lett. 258, 73–76. doi: 10.1016/S0304-3940(98)00848-9
Jackson, J., Jambrina, E., Li, J., Marston, H., Menzies, F., Phillips, K., et al. (2019). Targeting the synapse in Alzheimer’s disease. Front. Neurosci. 13:735. doi: 10.3389/fnins.2019.00735
Jacobsen, J. S., Wu, C.-C., Redwine, J. M., Comery, T. A., Arias, R., Bowlby, M., et al. (2006). Early-onset behavioral and synaptic deficits in a mouse model of Alzheimer’s disease. Proc. Natl. Acad. Sci. U.S.A. 103, 5161–5166. doi: 10.1073/pnas.0600948103
Jamjoom, A. A. B., Rhodes, J., Andrews, P. J. D., and Grant, S. G. N. (2021). The synapse in traumatic brain injury. Brain 144, 18–31. doi: 10.1093/brain/awaa321
Jaworski, J., Kapitein, L. C., Gouveia, S. M., Dortland, B. R., Wulf, P. S., Grigoriev, I., et al. (2009). Dynamic microtubules regulate dendritic spine morphology and synaptic plasticity. Neuron 61, 85–100. doi: 10.1016/j.neuron.2008.11.013
Ji, Z., Aas, J.-E., Laake, J., Walberg, F., and Ottersen, O. P. (1991). An electron microscopic, immunogold analysis of glutamate and glutamine in terminals of rat spinocerebellar fibers. J. Comparat. Neurol. 307, 296–310. doi: 10.1002/cne.903070210
Jiang, Y., and Ehlers, M. D. (2013). Modeling autism by SHANK gene mutations in mice. Neuron 78, 8–27. doi: 10.1016/j.neuron.2013.03.016
Kaplan, M., and Hinds, J. (1977). Neurogenesis in the adult rat: electron microscopic analysis of light radioautographs. Science 197, 1092–1094. doi: 10.1126/science.887941
Kashiwagi, Y., Higashi, T., Obashi, K., Sato, Y., Komiyama, N. H., Grant, S. G. N., et al. (2019). Computational geometry analysis of dendritic spines by structured illumination microscopy. Nat. Commun. 10:1285. doi: 10.1038/s41467-019-09337-0
Kasthuri, N., Hayworth, K. J., Berger, D. R., Schalek, R. L., Conchello, J. A., Knowles-Barley, S., et al. (2015). Saturated reconstruction of a volume of neocortex. Cell 162, 648–661. doi: 10.1016/j.cell.2015.06.054
Kay, K. R., Smith, C., Wright, A. K., Serrano-Pozo, A., Pooler, A. M., Koffie, R., et al. (2013). Studying synapses in human brain with array tomography and electron microscopy. Nat. Protocols 8, 1366–1380. doi: 10.1038/nprot.2013.078
Keifer, O. P. Jr., Hurt, R. C., Gutman, D. A., Keilholz, S. D., Gourley, S. L., and Ressler, K. J. (2015). Voxel-based morphometry predicts shifts in dendritic spine density and morphology with auditory fear conditioning. Nat. Commun. 6:7582. doi: 10.1038/ncomms8582
Keith, D. (2008). Excitation control: balancing PSD-95 function at the synapse. Front. Mol. Neurosci. 1:2008. doi: 10.3389/neuro.02.004.2008
Keshavan, M., Lizano, P., and Prasad, K. (2020). The synaptic pruning hypothesis of schizophrenia: promises and challenges. World Psychiatry 19, 110–111. doi: 10.1002/wps.20725
Khlebnikov, V., van der Kemp, W. J. M., Hoogduin, H., Klomp, D. W. J., and Prompers, J. J. (2019). Analysis of chemical exchange saturation transfer contributions from brain metabolites to the Z-spectra at various field strengths and pH. Sci. Rep. 9:1089. doi: 10.1038/s41598-018-37295-y
Kiessling, V., Kreutzberger, A. J. B., Liang, B., Nyenhuis, S. B., Seelheim, P., Castle, J. D., et al. (2018). A molecular mechanism for calcium-mediated synaptotagmin-triggered exocytosis. Nat. Struct. Mol. Biol. 25, 911–917. doi: 10.1038/s41594-018-0130-9
Kim, S. Y., and Jones, T. A. (2010). Lesion size-dependent synaptic and astrocytic responses in cortex contralateral to infarcts in middle-aged rats. Synapse 64, 659–671. doi: 10.1002/syn.20777
Kirov, S. A., Sorra, K. E., and Harris, K. M. (1999). Slices have more synapses than perfusion-fixed hippocampus from both young and mature rats. J. Neurosci. 19, 2876–2886. doi: 10.1523/JNEUROSCI.19-08-02876.1999
Klitgaard, H., and Verdru, P. (2007). Levetiracetam: the first SV2A ligand for the treatment of epilepsy. Exp. Opin. Drug Dis. 2, 1537–1545. doi: 10.1517/17460441.2.11.1537
Knobloch, M., and Mansuy, I. M. (2008). Dendritic spine loss and synaptic alterations in Alzheimer’s disease. Mol. Neurobiol. 37, 73–82. doi: 10.1007/s12035-008-8018-z
Krzystyniak, A., Baczynska, E., Magnowska, M., Antoniuk, S., Roszkowska, M., Zareba-Koziol, M., et al. (2019). Prophylactic ketamine treatment promotes resilience to chronic stress and accelerates recovery: correlation with changes in synaptic plasticity in the CA3 subregion of the hippocampus. Int. J. Mol. Sci. 20:1726. doi: 10.3390/ijms20071726
Kuhar, M. J., and Unnerstall, J. R. (1985). Quantitative receptor mapping by autoradiography: some current technical problems. Trends Neurosci. 8, 49–53. doi: 10.1016/0166-2236(85)90025-6
Kwon, S. E., and Chapman, E. R. (2011). Synaptophysin regulates the kinetics of synaptic vesicle endocytosis in central neurons. Neuron 70, 847–854. doi: 10.1016/j.neuron.2011.04.001
Lambeng, N., Gillard, M., Vertongen, P., Fuks, B., and Chatelain, P. (2005). Characterization of [(3)H]ucb 30889 binding to synaptic vesicle protein 2A in the rat spinal cord. Eur. J. Pharmacol. 520, 70–76. doi: 10.1016/j.ejphar.2005.07.029
Lammertsma, A. A. (2017). Forward to the past: the case for quantitative PET imaging. J. Nuclear Med. Off. Publi. Soc. Nuclear Med. 58, 1019–1024. doi: 10.2967/jnumed.116.188029
Laruelle, M. (2002). Positron emission tomography: imaging and quantification of neurotransporter availability. Methods 27, 287–299. doi: 10.1016/S1046-2023(02)00085-3
Lauterborn, J. C., Scaduto, P., Cox, C. D., Schulmann, A., Lynch, G., Gall, C. M., et al. (2021). Increased excitatory to inhibitory synaptic ratio in parietal cortex samples from individuals with Alzheimer’s disease. Nat. Commun. 12:2603. doi: 10.1038/s41467-021-22742-8
Lecoq, J., Orlova, N., and Grewe, B. F. (2019). Wide. fast. deep: recent advances in multiphoton microscopy of in vivo neuronal activity. J. Neurosci. 39, 9042–9052. doi: 10.1523/JNEUROSCI.1527-18.2019
Lepeta, K., Lourenco, M. V., Schweitzer, B. C., Martino Adami, P. V., Banerjee, P., Catuara-Solarz, S., et al. (2016). Synaptopathies: synaptic dysfunction in neurological disorders - a review from students to students. J. Neurochem. 138, 785–805. doi: 10.1111/jnc.13713
Lerch, J. P., van der Kouwe, A. J. W., Raznahan, A., Paus, T., Johansen-Berg, H., Miller, K. L., et al. (2017). Studying neuroanatomy using MRI. Nat. Neurosci. 20, 314–326. doi: 10.1038/nn.4501
Levesque, M., and Avoli, M. (2013). The kainic acid model of temporal lobe epilepsy. Neurosci. Biobehav. Rev. 37, 2887–2899. doi: 10.1016/j.neubiorev.2013.10.011
Lewis, A. J., Genoud, C., Pont, M., van de Berg, W. D., Frank, S., Stahlberg, H., et al. (2019). Imaging of post-mortem human brain tissue using electron and X-ray microscopy. Curr. Opin. Struct. Biol. 58, 138–148. doi: 10.1016/j.sbi.2019.06.003
Li, J.-Y., Plomann, M., and Brundin, P. (2003). Huntington’s disease: a synaptopathy? Trends Mol. Med. 9, 414–420. doi: 10.1016/j.molmed.2003.08.006
Li, Q., Weiland, A., Chen, X., Lan, X., Han, X., Durham, F., et al. (2018). Ultrastructural characteristics of neuronal death and white matter injury in mouse brain tissues after intracerebral hemorrhage: coexistence of ferroptosis, autophagy, and necrosis. Front. Neurol. 9:581. doi: 10.3389/fneur.2018.00581
Li, S., Cai, Z., Wu, X., Holden, D., Pracitto, R., Kapinos, M., et al. (2018). Synthesis and in vivo evaluation of a novel PET radiotracer for imaging of synaptic vesicle glycoprotein 2A (SV2A) in nonhuman primates. ACS Chem. Neurosci. 10, 1544–1554. doi: 10.1021/acschemneuro.8b00526
Li, Y. C., and Kavalali, E. T. (2017). Synaptic vesicle-recycling machinery components as potential therapeutic targets. Pharmacol. Rev. 69, 141–160. doi: 10.1124/pr.116.013342
Lieshoff, C., and Bischof, H.-J. (2003). The dynamics of spine density changes. Behav. Brain Res. 140, 87–95. doi: 10.1016/S0166-4328(02)00271-1
Lin, M. Z., and Schnitzer, M. J. (2016). Genetically encoded indicators of neuronal activity. Nat. Neurosci. 19, 1142–1153. doi: 10.1038/nn.4359
Liu, Y.-T., Tao, C.-L., Lau, P.-M., Zhou, Z. H., and Bi, G.-Q. (2019). Postsynaptic protein organization revealed by electron microscopy. Curr. Opin. Struct. Biol. 54, 152–160. doi: 10.1016/j.sbi.2019.02.012
Lordkipanidze, T., Bikashvili, T., Japaridze, N., and Zhvania, M. (2013). The effect of kainic acid on hippocampal dendritic spine motility at the early and late stages of brain development. Micron 49, 28–32. doi: 10.1016/j.micron.2013.02.009
Luo, J., Norris, R. H., Gordon, S. L., and Nithianantharajah, J. (2018). Neurodevelopmental synaptopathies: Insights from behaviour in rodent models of synapse gene mutations. Prog. Neuro Psychopharmacol. Biol. Psychiatry 84, 424–439. doi: 10.1016/j.pnpbp.2017.12.001
Lynch, B. A., Lambeng, N., Nocka, K., Kensel-Hammes, P., Bajjalieh, S. M., Matagne, A., et al. (2004). The synaptic vesicle protein SV2A is the binding site for the antiepileptic drug levetiracetam. Proc. Natl. Acad. Sci. U.S.A. 101, 9861–9866. doi: 10.1073/pnas.0308208101
Lynch, B. A., Matagne, A., Brännström, A., von Euler, A., Jansson, M., Hauzenberger, E., et al. (2008). Visualization of SV2A conformations in situ by the use of protein tomography. Biochem. Biophys. Res. Commun. 375, 491–495. doi: 10.1016/j.bbrc.2008.07.145
Ma, S., and Zuo, Y. (2021). Synaptic modifications in learning and memory – a dendritic spine story. Seminars Cell Dev. Biol. [Epub ahead of print], doi: 10.1016/j.semcdb.2021.05.015
MacNicol, E., Wright, P., Kim, E., Brusini, I., Esteban, O., Simmons, C., et al. (2022). Age-specific adult rat brain MRI templates and tissue probability maps. Front. Neuroinform. 15:669049. doi: 10.3389/fninf.2021.669049
Mahmmoud, R. R., Sase, S., Aher, Y. D., Sase, A. Gröger, M., Mokhtar, M., et al. (2015). Spatial and working memory is linked to spine density and mushroom spines. PLoS One 10:e0139739. doi: 10.1371/journal.pone.0139739
Maienschein, V., Marxen, M., Volknandt, W., and Zimmermann, H. (1999). A plethora of presynaptic proteins associated with ATP-storing organelles in cultured astrocytes. Glia 26, 233–244. doi: 10.1002/(SICI)1098-1136(199905)26:3<233::AID-GLIA5<3.0.CO;2-2
Mancuso, J. J., Chen, Y., Li, X., Xue, Z., and Wong, S. T. C. (2013). Methods of dendritic spine detection: from golgi to high-resolution optical imaging. Neuroscience 251, 129–140. doi: 10.1016/j.neuroscience.2012.04.010
Manuel, I., Barreda-Gómez, G., González de San Román, E., Veloso, A., Fernández, J. A., Giralt, M. T., et al. (2015). Neurotransmitter receptor localization: from autoradiography to imaging mass spectrometry. ACS Chem. Neurosci. 6, 362–373. doi: 10.1021/cn500281t
Markram, H., Toledo-Rodriguez, M., Wang, Y., Gupta, A., Silberberg, G., and Wu, C. (2004). Interneurons of the neocortical inhibitory system. Nat. Rev. Neurosci. 5, 793–807. doi: 10.1038/nrn1519
Marrone, D. F., LeBoutillier, J. C., and Petit, T. L. (2004). Comparative analyses of synaptic densities during reactive synaptogenesis in the rat dentate gyrus. Brain Res. 996, 19–30. doi: 10.1016/j.brainres.2003.09.073
Martineau, M., Guzman, R. E., Fahlke, C., and Klingauf, J. (2017). VGLUT1 functions as a glutamate/proton exchanger with chloride channel activity in hippocampal glutamatergic synapses. Nat. Commun. 8:2279. doi: 10.1038/s41467-017-02367-6
Marttinen, M., Takalo, M., Natunen, T., Wittrahm, R., Gabbouj, S., Kemppainen, S., et al. (2018). Molecular mechanisms of synaptotoxicity and neuroinflammation in Alzheimer’s disease. Front. Neurosci. 12:963. doi: 10.3389/fnins.2018.00963
Mattheisen, M., Mühleisen, T. W., Strohmaier, J., Treutlein, J., Nenadic, I., Alblas, M., et al. (2012). Genetic variation at the synaptic vesicle gene SV2A is associated with schizophrenia. Schizophrenia Res. 141, 262–265. doi: 10.1016/j.schres.2012.08.027
Maurer, R. (1984). Comparative in vitro / in vivo autoradiography using the opiate ligand 3 H-(-)-bremazocine. J. Receptor Res. 4, 155–163. doi: 10.3109/10799898409042546
Mayford, M., Siegelbaum, S. A., and Kandel, E. R. (2012). Synapses and memory storage. Cold Spring Harbor Perspect. Biol. 4:a005751. doi: 10.1101/cshperspect.a005751
McLeod, F., Marzo, A., Podpolny, M., Galli, S., and Salinas, P. (2017). Evaluation of synapse density in hippocampal rodent brain slices. J. Vis. Exp. 128:56153. doi: 10.3791/56153
Mendoza-Torreblanca, J. G., Vanoye-Carlo, A., Phillips-Farfán, B. V., Carmona-Aparicio, L., and Gómez-Lira, G. (2013). Synaptic vesicle protein 2A: basic facts and role in synaptic function. Eur. J. Neurosci. 38, 3529–3539. doi: 10.1111/ejn.12360
Meng, G., Liang, Y., Sarsfield, S., Jiang, W., Lu, R., Dudman, J. T., et al. (2019). High-throughput synapse-resolving two-photon fluorescence microendoscopy for deep-brain volumetric imaging in vivo. eLife 8:40805. doi: 10.7554/eLife.40805
Menten-Dedoyart, C., Serrano Navacerrada, M. E., Bartholome, O., Sánchez Gil, J., Neirinckx, V., Wislet, S., et al. (2016). Development and validation of a new mouse model to investigate the role of SV2A in epilepsy. PLoS One 11:e0166525. doi: 10.1371/journal.pone.0166525
Merchan-Pérez, A. (2009). Counting synapses using FIB/SEM microscopy: a true revolution for ultrastructural volume reconstruction. Front. Neuroanat. 3:2009. doi: 10.3389/neuro.05.018.2009
Mercier, J., Provins, L., and Valade, A. (2017). Discovery and development of SV2A PET tracers: potential for imaging synaptic density and clinical applications. Drug Dis. Today Technol. 25, 45–52. doi: 10.1016/j.ddtec.2017.11.003
Metaxas, A., Thygesen, C., Briting, S. R. R., Landau, A. M., Darvesh, S., and Finsen, B. (2019). Increased inflammation and unchanged density of synaptic vesicle glycoprotein 2A (SV2A) in the postmortem frontal cortex of Alzheimer’s disease patients. Front. Cell. Neurosci. 13:538. doi: 10.3389/fncel.2019.00538
Micheva, K. D., and Smith, S. J. (2007). Array tomography: a new tool for imaging the molecular architecture and ultrastructure of neural circuits. Neuron 55, 25–36. doi: 10.1016/j.neuron.2007.06.014
Mielnicka, A., and Michaluk, P. (2021). Exocytosis in astrocytes. Biomolecules 11:1367. doi: 10.3390/biom11091367
Mikula, S., Binding, J., and Denk, W. (2012). Staining and embedding the whole mouse brain for electron microscopy. Nat. Methods 9, 1198–1201. doi: 10.1038/nmeth.2213
Miranda, A., de Weerdt, C., Bertoglio, D., Stroobants, S., Staelens, S., and Verhaeghe, J. (2021). The Effect of Isoflurane Anesthesia On [18F]SynVesT-1 Imaging: A Brain PET Study in Freely Moving Mice. Göttingen.
Monteiro, P., and Feng, G. (2017). SHANK proteins: roles at the synapse and in autism spectrum disorder. Nat. Rev. Neurosci. 18, 147–157. doi: 10.1038/nrn.2016.183
Morris, A. W. J. (2016). Brain imaging: synaptic density directly visualized in human brains. Nat. Rev. Neurol. 12, 494–494. doi: 10.1038/nrneurol.2016.116
Moyer, C. E., Shelton, M. A., and Sweet, R. A. (2015). Dendritic spine alterations in schizophrenia. Neurosci. Lett. 601, 46–53. doi: 10.1016/j.neulet.2014.11.042
Murmu, M. S., Salomon, S., Biala, Y., Weinstock, M., Braun, K., and Bock, J. (2006). Changes of spine density and dendritic complexity in the prefrontal cortex in offspring of mothers exposed to stress during pregnancy. Eur. J. Neurosci. 24, 1477–1487. doi: 10.1111/j.1460-9568.2006.05024.x
Murphy, T. H., and Corbett, D. (2009). Plasticity during stroke recovery: from synapse to behaviour. Nat. Rev. Neurosci. 10, 861–872. doi: 10.1038/nrn2735
Mutch, S. A., Kensel-Hammes, P., Gadd, J. C., Fujimoto, B. S., Allen, R. W., Schiro, P. G., et al. (2011). Protein quantification at the single vesicle level reveals that a subset of synaptic vesicle proteins are trafficked with high precision. J. Neurosci. 31, 1461–1470. doi: 10.1523/JNEUROSCI.3805-10.2011
Nabulsi, N. B., Mercier, J., Holden, D., Carre, S., Najafzadeh, S., Vandergeten, M.-C., et al. (2016). Synthesis and preclinical evaluation of 11C-UCB-J as a PET tracer for imaging the synaptic vesicle glycoprotein 2A in the brain. J. Nuclear Med. Off. Publi. Soc. Nuclear Med. 57, 777–784. doi: 10.2967/jnumed.115.168179
Neniskyte, U., and Gross, C. T. (2017). Errant gardeners: glial-cell-dependent synaptic pruning and neurodevelopmental disorders. Nature Reviews Neuroscience 18, 658–670. doi: 10.1038/nrn.2017.110
Niell, C. M., Meyer, M. P., and Smith, S. J. (2004). In vivo imaging of synapse formation on a growing dendritic arbor. Nat. Neurosci. 7, 254–260. doi: 10.1038/nn1191
Nimchinsky, E. A., Sabatini, B. L., and Svoboda, K. (2002). Structure and function of dendritic spines. Ann. Rev. Physiol. 64, 313–353. doi: 10.1146/annurev.physiol.64.081501.160008
O’Dell, R. S., Mecca, A. P., Chen, M.-K., Naganawa, M., Toyonaga, T., Lu, Y., et al. (2021). Association of Aβ deposition and regional synaptic density in early Alzheimer’s disease: a PET imaging study with [11C]UCB-J. Alzheimer’s Res. Ther. 13:11. doi: 10.1186/s13195-020-00742-y
O’Neal, M. A., Stallings, N. R., and Malter, J. S. (2018). Alzheimer’s disease, dendritic spines, and calcineurin inhibitors: a new approach? ACS Chem. Neurosci. 9, 1233–1234. doi: 10.1021/acschemneuro.8b00213
Obashi, K., Taraska, J. W., and Okabe, S. (2021). The role of molecular diffusion within dendritic spines in synaptic function. J. General Physiol. 153:814. doi: 10.1085/jgp.202012814
Oberman, L., and Pascual-Leone, A. (2013). Changes in plasticity across the lifespan: cause of disease and target for intervention. Prog. Brain Res. 207, 91–120. doi: 10.1016/B978-0-444-63327-9.00016-3
Obi-Nagata, K., Temma, Y., and Hayashi-Takagi, A. (2019). Synaptic functions and their disruption in schizophrenia: from clinical evidence to synaptic optogenetics in an animal model. Proc. Japan Acad. Ser. B 95, 179–197. doi: 10.2183/pjab.95.014
Ohno, Y., Okumura, T., Terada, R., Ishihara, S., Serikawa, T., and Sasa, M. (2012). Kindling-associated SV2A expression in hilar GABAergic interneurons of the mouse dentate gyrus. Neurosci. Lett. 510, 93–98. doi: 10.1016/j.neulet.2012.01.009
Onwordi, E. C., Halff, E. F., Whitehurst, T., Mansur, A., Cotel, M.-C., Wells, L., et al. (2020). Synaptic density marker SV2A is reduced in schizophrenia patients and unaffected by antipsychotics in rats. Nat. Commun. 11:246. doi: 10.1038/s41467-019-14122-0
Onwordi, E. C., Whitehurst, T., Mansur, A., Statton, B., Berry, A., Quinlan, M., et al. (2021). The relationship between synaptic density marker SV2A, glutamate and N-acetyl aspartate levels in healthy volunteers and schizophrenia: a multimodal PET and magnetic resonance spectroscopy brain imaging study. Trans. Psychiatry 11:393. doi: 10.1038/s41398-021-01515-3
Osimo, E. F., Beck, K., Reis Marques, T., and Howes, O. D. (2019). Synaptic loss in schizophrenia: a meta-analysis and systematic review of synaptic protein and mRNA measures. Mol. Psychiatry 24, 549–561. doi: 10.1038/s41380-018-0041-5
Paasonen, J., Stenroos, P., Salo, R. A., Kiviniemi, V., and Gröhn, O. (2018). Functional connectivity under six anesthesia protocols and the awake condition in rat brain. NeuroImage 172, 9–20. doi: 10.1016/j.neuroimage.2018.01.014
Paolicelli, R. C., Bolasco, G., Pagani, F., Maggi, L., Scianni, M., Panzanelli, P., et al. (2011). Synaptic pruning by microglia is necessary for normal brain development. Science 333, 1456–1458. doi: 10.1126/science.1202529
Parajuli, L. K., Wako, K., Maruo, S., Kakuta, S., Taguchi, T., Ikuno, M., et al. (2020). Developmental changes in dendritic spine morphology in the striatum and their alteration in an A53T α-synuclein transgenic mouse model of Parkinson’s disease. eneuro 7:ENEURO.0072-20.2020. doi: 10.1523/ENEURO.0072-20.2020
Parra-Damas, A., and Saura, C. A. (2020). Tissue clearing and expansion methods for imaging brain pathology in neurodegeneration: from circuits to synapses and beyond. Front. Neurosci. 14:914. doi: 10.3389/fnins.2020.00914
Passamonti, L., Vázquez Rodríguez, P., Hong, Y. T., Allinson, K. S. J., Williamson, D., Borchert, R. J., et al. (2017). 18 F-AV-1451 positron emission tomography in Alzheimer’s disease and progressive supranuclear palsy. Brain 140, 781–791. doi: 10.1093/brain/aww340
Patel, S., Knight, A., Krause, S., Teceno, T., Tresse, C., Li, S., et al. (2020). Preclinical in vitro and in vivo characterization of synaptic vesicle 2A–targeting compounds amenable to F-18 labeling as potential PET radioligands for imaging of synapse integrity. Mol. Imag. Biol. 22, 832–841. doi: 10.1007/s11307-019-01428-0
Patrizio, A., and Specht, C. G. (2016). Counting numbers of synaptic proteins: absolute quantification and single molecule imaging techniques. Neurophotonics 3:041805. doi: 10.1117/1.NPh.3.4.041805
Pereda, A. E. (2014). Electrical synapses and their functional interactions with chemical synapses. Nat. Rev. Neurosci. 15, 250–263. doi: 10.1038/nrn3708
Pérez-González, R., Alvira-Botero, M. X., Robayo, O., Antequera, D., Garzón, M., Martín-Moreno, A. M., et al. (2014). Leptin gene therapy attenuates neuronal damages evoked by amyloid-β and rescues memory deficits in APP/PS1 mice. Gene Ther. 21, 298–308. doi: 10.1038/gt.2013.85
Peter, R. H. (1979). Synaptic density in human frontal cortex — developmental changes and effects of aging. Brain Res. 163, 195–205. doi: 10.1016/0006-8993(79)90349-4
Pilati, N., Barker, M., Panteleimonitis, S., Donga, R., and Hamann, M. (2008). A rapid method combining golgi and nissl staining to study neuronal morphology and cytoarchitecture. J. Histochem. Cytochem. 56, 539–550. doi: 10.1369/jhc.2008.950246
Power, J. D., and Schlaggar, B. L. (2017). Neural plasticity across the lifespan. Wiley Int. Rev. Dev. Biol. 6:e216. doi: 10.1002/wdev.216
Prange, O., Wong, T. P., Gerrow, K., Wang, Y. T., and El-Husseini, A. (2004). A balance between excitatory and inhibitory synapses is controlled by PSD-95 and neuroligin. Proc. Natl. Acad. Sci. U.S.A. 101, 13915–13920. doi: 10.1073/pnas.0405939101
Prieto, G. A., and Cotman, C. (2017). On the road towards the global analysis of human synapses. Neural Regen. Res. 12:1586. doi: 10.4103/1673-5374.217321
Qiao, H., Li, M.-X., Xu, C., Chen, H.-B., An, S.-C., and Ma, X.-M. (2016). Dendritic spines in depression: what we learned from animal models. Neural Plasticity 2016, 1–26. doi: 10.1155/2016/8056370
Rabiner, E. A. (2018). Imaging synaptic density: a different look at neurologic diseases. J. Nuclear Med. 59, 380–381. doi: 10.2967/jnumed.117.198317
Radhakrishnan, R., Skosnik, P. D., Ranganathan, M., Naganawa, M., Toyonaga, T., Finnema, S., et al. (2021). In vivo evidence of lower synaptic vesicle density in schizophrenia. Mol. Psychiatry [Epub ahead of print], doi: 10.1038/s41380-021-01184-0
Ranjan, A., and Mallick, B. N. (2010). A modified method for consistent and reliable golgi–cox staining in significantly reduced time. Front. Neurol. 1:157. doi: 10.3389/fneur.2010.00157
Raval, N. R., Johansen, A., Donovan, L. L., Ros, N. F., Ozenne, B., Hansen, H. D., et al. (2021b). A single dose of psilocybin increases synaptic density and decreases 5-HT2A receptor density in the pig brain. Int. J. Mol. Sci. 22:835. doi: 10.3390/ijms22020835
Raval, N. R., Gudmundsen, F., Juhl, M., Andersen, I. V., Speth, N., Videbæk, A., et al. (2021a). Synaptic density and neuronal metabolic function measured by positron emission tomography in the unilateral 6-OHDA rat model of Parkinson’s disease. Front. Syn. Neurosci. 13:715811. doi: 10.3389/fnsyn.2021.715811
Rensing, N., Ouyang, Y., Yang, X.-F., Yamada, K. A., Rothman, S. M., and Wong, M. (2005). In vivo imaging of dendritic spines during electrographic seizures. Ann. Neurol. 58, 888–898. doi: 10.1002/ana.20658
Reza-Zaldivar, E. E., Hernández-Sápiens, M. A., Minjarez, B., Gómez-Pinedo, U., Sánchez-González, V. J., Márquez-Aguirre, A. L., et al. (2020). Dendritic spine and synaptic plasticity in Alzheimer’s disease: a focus on microRNA. Front. Cell Dev. Biol. 8:255. doi: 10.3389/fcell.2020.00255
Rizzoli, S. O., and Betz, W. J. (2005). Synaptic vesicle pools. Nat. Rev. Neurosci. 6, 57–69. doi: 10.1038/nrn1583
Robinson, T. E., and Kolb, B. (1999). Alterations in the morphology of dendrites and dendritic spines in the nucleus accumbens and prefrontal cortex following repeated treatment with amphetamine or cocaine. Eur. J. Neurosci. 11, 1598–1604. doi: 10.1046/j.1460-9568.1999.00576.x
Ruszczycki, B., Szepesi, Z., Wilczynski, G. M., Bijata, M., Kalita, K., Kaczmarek, L., et al. (2012). Sampling issues in quantitative analysis of dendritic spines morphology. BMC Bioinform. 13:213. doi: 10.1186/1471-2105-13-213
Sadasivam, P., Fang, X. T., Toyonaga, T., Lee, S., Xu, Y., Zheng, M.-Q., et al. (2021). Quantification of SV2A binding in rodent brain using [18F]SynVesT-1 and PET imaging. Mol. Imag. Biol. 23, 372–381. doi: 10.1007/s11307-020-01567-9
Sahl, S. J., and Hell, S. W. (2019). “High-resolution 3D light microscopy with STED and RESOLFT,” in High Resolution Imaging in Microscopy and Ophthalmology: New Frontiers in Biomedical Optics, ed. J. F. Bille (Cham: Springer), 3–32.
Saito, K., Kakizaki, T., Hayashi, R., Nishimaru, H., Furukawa, T., Nakazato, Y., et al. (2010). The physiological roles of vesicular GABA transporter during embryonic development: a study using knockout mice. Mol. Brain 3:40. doi: 10.1186/1756-6606-3-40
Sakai, J. (2020). Core concept: how synaptic pruning shapes neural wiring during development and, possibly, in disease. Proc. Natl. Acad. Sci. U.S.A. 117, 16096–16099. doi: 10.1073/pnas.2010281117
Sala, C. (2005). Key role of the postsynaptic density scaffold proteins shank and homer in the functional architecture of Ca2+ homeostasis at dendritic spines in hippocampal neurons. J. Neurosci. 25, 4587–4592. doi: 10.1523/JNEUROSCI.4822-04.2005
Salaka, R. J., Nair, K. P., Annamalai, K., Srikumar, B. N., Kutty, B. M., and Shankaranarayana Rao, B. S. (2021). Enriched environment ameliorates chronic temporal lobe epilepsy-induced behavioral hyperexcitability and restores synaptic plasticity in CA3–CA1 synapses in male wistar rats. J. Neurosci. Res. 99, 1646–1665. doi: 10.1002/jnr.24823
Sankaranarayanan, S., de Angelis, D., Rothman, J. E., and Ryan, T. A. (2000). The use of pHluorins for optical measurements of presynaptic activity. Biophys. J. 79, 2199–2208. doi: 10.1016/S0006-3495(00)76468-X
Santuy, A., Rodriguez, J. R., DeFelipe, J., and Merchan-Perez, A. (2018). Volume electron microscopy of the distribution of synapses in the neuropil of the juvenile rat somatosensory cortex. Brain Struct. Funct. 223, 77–90. doi: 10.1007/s00429-017-1470-7
Santuy, A., Tomás-Roca, L., Rodríguez, J.-R., González-Soriano, J., Zhu, F., Qiu, Z., et al. (2020). Estimation of the number of synapses in the hippocampus and brain-wide by volume electron microscopy and genetic labeling. Sci. Rep. 10:14014. doi: 10.1038/s41598-020-70859-5
Savage, J. C., St-Pierre, M.-K., Carrier, M., el Hajj, H., Novak, S. W., Sanchez, M. G., et al. (2020). Microglial physiological properties and interactions with synapses are altered at presymptomatic stages in a mouse model of Huntington’s disease pathology. J. Neuroinflam. 17:98. doi: 10.1186/s12974-020-01782-9
Scheff, S. W., Neltner, J. H., and Nelson, P. T. (2014). Is synaptic loss a unique hallmark of Alzheimer’s disease? Biochem. Pharmacol. 88, 517–528. doi: 10.1016/j.bcp.2013.12.028
Scheff, S. W., Scott, S. A., and DeKosky, S. T. (1991). Quantitation of synaptic density in the septal nuclei of young and aged fischer 344 rats. Neurobiol. Aging 12, 3–12. doi: 10.1016/0197-4580(91)90032-F
Scheuss, V., and Bonhoeffer, T. (2014). Function of dendritic spines on hippocampal inhibitory neurons. Cerebral Cortex 24, 3142–3153. doi: 10.1093/cercor/bht171
Schmidt, K. C., and Smith, C. B. (2005). Resolution, sensitivity and precision with autoradiography and small animal positron emission tomography: implications for functional brain imaging in animal research. Nuclear Med. Biol. 32, 719–725. doi: 10.1016/j.nucmedbio.2005.04.020
Sekar, A., Bialas, A. R., de Rivera, H., Davis, A., Hammond, T. R., Kamitaki, N., et al. (2016). Schizophrenia risk from complex variation of complement component 4. Nature 530, 177–183. doi: 10.1038/nature16549
Selkoe, D. J. (2002). Alzheimer’s disease is a synaptic failure. Science 298, 789–791. doi: 10.1126/science.1074069
Serrano, M. E., Bahri, M. A., Becker, G., Seret, A., Germonpré, C., Lemaire, C., et al. (2020). Exploring with [18F]UCB-H the in vivo variations in SV2A expression through the kainic acid rat model of temporal lobe epilepsy. Mol. Imag. Biol. 22, 1197–1207. doi: 10.1007/s11307-020-01488-7
Serrano, M. E., Bahri, M. A., Becker, G., Seret, A., Mievis, F., Giacomelli, F., et al. (2018). Quantification of [18F]UCB-H binding in the rat brain: from kinetic modelling to standardised uptake value. Mol. Imag. Biol. ?21, 888–897. doi: 10.1007/s11307-018-1301-0
Sheffield, M. E. J., and Dombeck, D. A. (2015). Calcium transient prevalence across the dendritic arbour predicts place field properties. Nature 517, 200–204. doi: 10.1038/nature13871
Sheng, M., and Kim, E. (2011). The postsynaptic organization of synapses. Cold Spring Harbor Perspect. Biol. 3:a005678. doi: 10.1101/cshperspect.a005678
Sherwood, C. C., Miller, S. B., Karl, M., Stimpson, C. D., Phillips, K. A., Jacobs, B., et al. (2020). Invariant synapse density and neuronal connectivity scaling in primate neocortical evolution. Cerebral Cortex 30, 5604–5615. doi: 10.1093/cercor/bhaa149
Shi, J., Anderson, D., Lynch, B. A., Castaigne, J.-G., Foerch, P., and Lebon, F. (2011). Combining modelling and mutagenesis studies of synaptic vesicle protein 2A to identify a series of residues involved in racetam binding. Biochem. Soc. Trans. 39, 1341–1347. doi: 10.1042/BST0391341
Shihan, M. H., Novo, S. G., le Marchand, S. J., Wang, Y., and Duncan, M. K. (2021). A simple method for quantitating confocal fluorescent images. Biochem. Biophys. Rep. 25:100916. doi: 10.1016/j.bbrep.2021.100916
Siksou, L., Triller, A., and Marty, S. (2009). An emerging view of presynaptic structure from electron microscopic studies. J. Neurochem. 108, 1336–1342. doi: 10.1111/j.1471-4159.2009.05888.x
Sills, G. J. (2010). SV2A in epilepsy: the plot thickens. Epilepsy Curr. 10, 47–49. doi: 10.1111/j.1535-7511.2009.01351.x
Silva, A. J. (2017). Miniaturized two-photon microscope: seeing clearer and deeper into the brain. Light Sci. Appl. 6:e17104. doi: 10.1038/lsa.2017.104
Singh, P., Jorgaèevski, J., Kreft, M., Grubišiæ, V., Stout, R. F., Potokar, M., et al. (2014). Single-vesicle architecture of synaptobrevin2 in astrocytes. Nat. Commun. 5:3780. doi: 10.1038/ncomms4780
Smart, K., Liu, H., Matuskey, D., Chen, M.-K., Torres, K., Nabulsi, N., et al. (2021). Binding of the synaptic vesicle radiotracer [11 C]UCB-J is unchanged during functional brain activation using a visual stimulation task. J. Cerebral Blood Flow Metab. 41, 1067–1079. doi: 10.1177/0271678X20946198
Solon, E. G. (2012). Use of radioactive compounds and autoradiography to determine drug tissue distribution. Chem. Res. Toxicol. 25, 543–555. doi: 10.1021/tx200509f
Spangler-Bickell, M. G., de Laat, B., Fulton, R., Bormans, G., and Nuyts, J. (2016). The effect of isoflurane on 18F-FDG uptake in the rat brain: a fully conscious dynamic PET study using motion compensation. EJNMMI Res. 6:86. doi: 10.1186/s13550-016-0242-3
Stallings, N. R., O’Neal, M. A., Hu, J., Kavalali, E. T., Bezprozvanny, I., and Malter, J. S. (2018). Pin1 mediates Aβ 42 -induced dendritic spine loss. Sci. Sign. 11:eaa8734. doi: 10.1126/scisignal.aap8734
Stampanoni Bassi, M., Iezzi, E., Gilio, L., Centonze, D., and Buttari, F. (2019). Synaptic plasticity shapes brain connectivity: implications for network topology. Internat. J. Mol. Sci. 20:6193. doi: 10.3390/ijms20246193
Steiner, A. R., Rousseau-Blass, F., Schroeter, A., Hartnack, S., and Bettschart-Wolfensberger, R. (2020). Systematic review: anaesthetic protocols and management as confounders in rodent blood oxygen level dependent functional magnetic resonance imaging (BOLD fMRI)–part a: effects of changes in physiological parameters. Front. Neurosci. 14:577119. doi: 10.3389/fnins.2020.577119
Stockburger, C., Miano, D., Baeumlisberger, M., Pallas, T., Arrey, T. N., Karas, M., et al. (2015). A Mitochondrial role of SV2a protein in aging and Alzheimer’s disease: studies with levetiracetam. J. Alzheimer’s Dis. 50, 201–215. doi: 10.3233/JAD-150687
Südhof, T. C. (2008). Neuroligins and neurexins link synaptic function to cognitive disease. Nature 455, 903–911. doi: 10.1038/nature07456
Südhof, T. C. (2017). Synaptic neurexin complexes: a molecular code for the logic of neural circuits. Cell 171, 745–769. doi: 10.1016/j.cell.2017.10.024
Szabo, T. M. (2004). Transient electrical coupling delays the onset of chemical neurotransmission at developing synapses. J. Neurosci. 24, 112–120. doi: 10.1523/JNEUROSCI.4336-03.2004
Sze, C.-I., Bi, H., Kleinschmidt-DeMasters, B. K., Filley, C. M., and Martin, L. J. (2000). Selective regional loss of exocytotic presynaptic vesicle proteins in Alzheimer’s disease brains. J. Neurol. Sci. 175, 81–90. doi: 10.1016/S0022-510X(00)00285-9
Taoufik, E., Kouroupi, G., Zygogianni, O., and Matsas, R. (2018). Synaptic dysfunction in neurodegenerative and neurodevelopmental diseases: an overview of induced pluripotent stem-cell-based disease models. Open Biol. 8:180138. doi: 10.1098/rsob.180138
Taylor, W. D., McQuoid, D. R., Payne, M. E., Zannas, A. S., MacFall, J. R., and Steffens, D. C. (2014). Hippocampus atrophy and the longitudinal course of late-life depression. Am. J. Geriatric Psychiatry 22, 1504–1512. doi: 10.1016/j.jagp.2013.11.004
Tendilla-Beltrán, H., Antonio Vázquez-Roque, R., Judith Vázquez-Hernández, A., Garcés-Ramírez, L., and Flores, G. (2019). Exploring the dendritic spine pathology in a schizophrenia-related neurodevelopmental animal model. Neuroscience 396, 36–45. doi: 10.1016/j.neuroscience.2018.11.006
Thomsen, M. B., Jacobsen, J., Lillethorup, T. P., Schacht, A. C., Simonsen, M., Romero-Ramos, M., et al. (2021). In vivo imaging of synaptic SV2A protein density in healthy and striatal-lesioned rats with [11C]UCB-J PET. J. Cerebral Blood Flow Metab. 41, 819–830. doi: 10.1177/0271678X20931140
Tiwari, D., Schaefer, T. L., Schroeder-Carter, L. M., Krzeski, J. C., Bunk, A. T., Parkins, E. V., et al. (2020). The potassium channel Kv4.2 regulates dendritic spine morphology, electroencephalographic characteristics and seizure susceptibility in mice. Exp. Neurol. 334:113437. doi: 10.1016/j.expneurol.2020.113437
Todd, K. L., Kristan, W. B., and French, K. A. (2010). Gap junction expression is required for normal chemical synapse formation. J. Neurosci. 30, 15277–15285. doi: 10.1523/JNEUROSCI.2331-10.2010
Tomasi, G., Turkheimer, F., and Aboagye, E. (2012). Importance of quantification for the analysis of PET data in oncology: review of current methods and trends for the future. Mol. Imag. Biol.? 14, 131–146. doi: 10.1007/s11307-011-0514-2
Tønnesen, J., Inavalli, V. V. G. K., and Nägerl, U. V. (2018). Super-resolution imaging of the extracellular space in living brain tissue. Cell 172, 1108–1121.e15. doi: 10.1016/j.cell.2018.02.007
Torres, J. J., and Varona, P. (2012). “Modeling biological neural networks,” in Handbook of Natural Computing, eds G. Rozenberg, T. Bäck, and J. N. Kok (Berlin: Springer). doi: 10.1007/978-3-540-92910-9_17
Torres, M. D., Garcia, O., Tang, C., and Busciglio, J. (2018). Dendritic spine pathology and thrombospondin-1 deficits in down syndrome. Free Rad. Biol. Med. 114, 10–14. doi: 10.1016/j.freeradbiomed.2017.09.025
Toyonaga, T., Smith, L. M., Finnema, S. J., Gallezot, J.-D., Naganawa, M., Bini, J., et al. (2019). In vivo synaptic density imaging with 11 C-UCB-J detects treatment effects of saracatinib in a mouse model of alzheimer disease. J. Nuclear Med. 60, 1780–1786. doi: 10.2967/jnumed.118.223867
Tuncel, H., Boellaard, R., Coomans, E. M., de Vries, E. F., Glaudemans, A. W., Feltes, P. K., et al. (2021). Kinetics and 28-day test–retest repeatability and reproducibility of [11 C]UCB-J PET brain imaging. J. Cerebral Blood Flow Metab. 41, 1338–1350. doi: 10.1177/0271678X20964248
Ueda, H. R., Ertürk, A., Chung, K., Gradinaru, V., Chédotal, A., Tomancak, P., et al. (2020). Tissue clearing and its applications in neuroscience. Nat. Rev. Neurosci. 21, 61–79. doi: 10.1038/s41583-019-0250-1
Urbina, F. L., and Gupton, S. L. (2020). SNARE-mediated exocytosis in neuronal development. Front. Mol. Neurosci. 13:133. doi: 10.3389/fnmol.2020.00133
Valtorta, F., Fesce, R., Grohovaz, F., Haimann, C., Hurlbut, W. P., Iezzi, N., et al. (1990). Neurotransmitter release and synaptic vesicle recycling. Neuroscience 35, 477–489. doi: 10.1016/0306-4522(90)90323-V
van Aalst, J., Ceccarini, J., Sunaert, S., Dupont, P., Koole, M., and van Laere, K. (2021). In vivo synaptic density relates to glucose metabolism at rest in healthy subjects, but is strongly modulated by regional differences. J. Cerebral Blood Flow Metab. 41, 1978–1987. doi: 10.1177/0271678X20981502
van der Linden, A., and Hoehn, M. (2022). Monitoring neuronal network disturbances of brain diseases: a preclinical MRI approach in the rodent brain. Front. Cell. Neurosci. 15:815552. doi: 10.3389/fncel.2021.815552
van Spronsen, M., and Hoogenraad, C. C. (2010). Synapse pathology in psychiatric and neurologic disease. Curr. Neurol. Neurosci. Rep. 10, 207–214. doi: 10.1007/s11910-010-0104-8
van Vliet, E. A., Aronica, E., Redeker, S., Boer, K., and Gorter, J. A. (2009). Decreased expression of synaptic vesicle protein 2A, the binding site for levetiracetam, during epileptogenesis and chronic epilepsy. Epilepsia 50, 422–433. doi: 10.1111/j.1528-1167.2008.01727.x
Vardar, G., Chang, S., Arancillo, M., Wu, Y.-J., Trimbuch, T., and Rosenmund, C. (2016). Distinct functions of syntaxin-1 in neuronal maintenance, synaptic vesicle docking, and fusion in mouse neurons. J. Neurosci. 36, 7911–7924. doi: 10.1523/JNEUROSCI.1314-16.2016
Varghese, M., Keshav, N., Jacot-Descombes, S., Warda, T., Wicinski, B., Dickstein, D. L., et al. (2017). Autism spectrum disorder: neuropathology and animal models. Acta Neuropathol. 134, 537–566. doi: 10.1007/s00401-017-1736-4
Varnäs, K., Stepanov, V., and Halldin, C. (2020). Autoradiographic mapping of synaptic vesicle glycoprotein 2A in non-human primate and human brain. Synapse 74:22157. doi: 10.1002/syn.22157
Ventura, R., and Harris, K. M. (1999). Three-dimensional relationships between hippocampal synapses and astrocytes. J. Neurosci. 19, 6897–6906. doi: 10.1523/JNEUROSCI.19-16-06897.1999
Verpelli, C., Schmeisser, M. J., Sala, C., and Boeckers, T. M. (2012). Scaffold proteins at the postsynaptic density. Adv. Exp. Med. Biol. 970, 29–61. doi: 10.1007/978-3-7091-0932-8_2
Verstraelen, P., Garcia-Diaz Barriga, G., Verschuuren, M., Asselbergh, B., Nuydens, R., Larsen, P. H., et al. (2020). Systematic quantification of synapses in primary neuronal culture. iScience 23:101542. doi: 10.1016/j.isci.2020.101542
Vervaeke, K., Lorincz, A., Nusser, Z., and Silver, R. A. (2012). Gap junctions compensate for sublinear dendritic integration in an inhibitory network. Science 335, 1624–1628. doi: 10.1126/science.1215101
Vicidomini, G., Bianchini, P., and Diaspro, A. (2018). STED super-resolved microscopy. Nat. Methods 15, 173–182. doi: 10.1038/nmeth.4593
Vints, K., Vandael, D., Baatsen, P., Pavie, B., Vernaillen, F., Corthout, N., et al. (2019). Modernization of golgi staining techniques for high-resolution, 3-dimensional imaging of individual neurons. Sci. Rep. 9:130. doi: 10.1038/s41598-018-37377-x
von Bohlen und Halbach, O. (2010). Dendritic spine abnormalities in mental retardation. Cell Tissue Res. 342, 317–323. doi: 10.1007/s00441-010-1070-9
Waites, C. L., Leal-Ortiz, S. A., Okerlund, N., Dalke, H., Fejtova, A., Altrock, W. D., et al. (2013). Bassoon and piccolo maintain synapse integrity by regulating protein ubiquitination and degradation. EMBO J. 32, 954–969. doi: 10.1038/emboj.2013.27
Wang, L., Zhou, X., Shi, J., Wu, G., and Hong, Z. (2018). Overexpression of synaptic vesicle protein 2A inhibits seizures and amygdaloid electroencephalogram activity in pilocarpine-induced pharmacoresistant epileptic rats. JNeuropsychiatry 8, 595–605.
Warnock, G. I., Aerts, J., Bahri, M. A., Bretin, F., Lemaire, C., Giacomelli, F., et al. (2014). Evaluation of 18F-UCB-H as a novel PET tracer for synaptic vesicle protein 2A in the brain. J. Nuclear Med. Off. Publi. Soc. Nuclear Med. 55, 1336–1341. doi: 10.2967/jnumed.113.136143
Weiss, J. J., Calvi, R., Naganawa, M., Toyonaga, T., Farhadian, S. F., Chintanaphol, M., et al. (2021). Preliminary in vivo evidence of reduced synaptic density in human immunodeficiency virus (HIV) despite antiretroviral therapy. Clin. Infect. Dis. 73, 1404–1411. doi: 10.1093/cid/ciab484
Wells, W. A. (2005). The discovery of synaptic vesicles. J. Cell Biol. 168, 12–13. doi: 10.1083/jcb1681fta2
Wilhelm, A., Volknandt, W., Langer, D., Nolte, C., Kettenmann, H., and Zimmermann, H. (2004). Localization of SNARE proteins and secretory organelle proteins in astrocytes in vitro and in situ. Neurosci. Res. 48, 249–257. doi: 10.1016/j.neures.2003.11.002
Wong, M. (2005). Modulation of dendritic spines in epilepsy: cellular mechanisms and functional implications. Epilepsy Behavior 7, 569–577. doi: 10.1016/j.yebeh.2005.08.007
Wong, M., and Guo, D. (2013). Dendritic spine pathology in epilepsy: cause or consequence? Neuroscience 251, 141–150. doi: 10.1016/j.neuroscience.2012.03.048
Wu, B., Warnock, G., Zaiss, M., Lin, C., Chen, M., Zhou, Z., et al. (2016). An overview of CEST MRI for non-MR physicists. EJNMMI Physics 3:19. doi: 10.1186/s40658-016-0155-2
Xiao, S., Song, L.-L., Li, J.-T., Wang, H., Yu, N., Wang, Z.-Q., et al. (2020). Intraperitoneal administration of monoclonal antibody against pathologic Aβ42 aggregates alleviated cognitive deficits and synaptic lesions in APP/PS1 mice. J. Alzheimer’s Dis. 73, 657–670. doi: 10.3233/JAD-190874
Xie, L., Li, T., Song, X., Sun, H., Liu, J., Yang, J., et al. (2020). Dynamic alteration of dendrites and dendritic spines in the hippocampus and microglia in mouse brain tissues after kainate-induced status epilepticus. Int. J. Neurosci. 131, 1045–1057. doi: 10.1080/00207454.2020.1770246
Xiong, M., Roshanbin, S., Rokka, J., Schlein, E., Ingelsson, M., Sehlin, D., et al. (2021). In vivo imaging of synaptic density with [11C]UCB-J PET in two mouse models of neurodegenerative disease. NeuroImage 239:118302. doi: 10.1016/j.neuroimage.2021.118302
Yang, G., Lai, C. S. W., Cichon, J., Ma, L., Li, W., and Gan, W.-B. (2014). Sleep promotes branch-specific formation of dendritic spines after learning. Science 344, 1173–1178. doi: 10.1126/science.1249098
Yang, H. H., St-Pierre, F., Sun, X., Ding, X., Lin, M. Z., and Clandinin, T. R. (2016). Subcellular imaging of voltage and calcium signals reveals neural processing in vivo. Cell 166, 245–257. doi: 10.1016/j.cell.2016.05.031
Yao, J., Nowack, A., Kensel-Hammes, P., Gardner, R. G., and Bajjalieh, S. M. (2010). Cotrafficking of SV2 and synaptotagmin at the synapse. J. Neurosci. 30, 5569–5578. doi: 10.1523/JNEUROSCI.4781-09.2010
Zander, J.-F., Munster-Wandowski, A., Brunk, I., Pahner, I., Gomez-Lira, G., Heinemann, U., et al. (2010). Synaptic and vesicular coexistence of VGLUT and VGAT in selected excitatory and inhibitory synapses. J. Neurosci. 30, 7634–7645. doi: 10.1523/JNEUROSCI.0141-10.2010
Zatkova, M., Bakos, J., Hodosy, J., and Ostatnikova, D. (2016). Synapse alterations in autism: review of animal model findings. Biomed. Papers 160, 201–210. doi: 10.5507/bp.2015.066
Zhang, Q., Pangršiè, T., Kreft, M., Kržan, M., Li, N., Sul, J.-Y., et al. (2004). Fusion-related release of glutamate from astrocytes. J. Biol. Chem. 279, 12724–12733. doi: 10.1074/jbc.M312845200
Ziv, Y., Burns, L. D., Cocker, E. D., Hamel, E. O., Ghosh, K. K., Kitch, L. J., et al. (2013). Long-term dynamics of CA1 hippocampal place codes. Nat. Neurosci. 16, 264–266. doi: 10.1038/nn.3329
Zong, W., Wu, R., Chen, S., Wu, J., Wang, H., Zhao, Z., et al. (2021). Miniature two-photon microscopy for enlarged field-of-view, multi-plane and long-term brain imaging. Nat. Methods 18, 46–49. doi: 10.1038/s41592-020-01024-z
Keywords: electron microscopy, immunohistochemistry, SV2A, PET, GluCEST, synaptic density
Citation: Serrano ME, Kim E, Petrinovic MM, Turkheimer F and Cash D (2022) Imaging Synaptic Density: The Next Holy Grail of Neuroscience? Front. Neurosci. 16:796129. doi: 10.3389/fnins.2022.796129
Received: 15 October 2021; Accepted: 15 February 2022;
Published: 25 March 2022.
Edited by:
Nadja Van Camp, Commissariat à l’Energie Atomique et aux Energies Alternatives, FranceReviewed by:
Irina Dudanova, Max Planck Institute of Neurobiology (MPIN), GermanyCopyright © 2022 Serrano, Kim, Petrinovic, Turkheimer and Cash. This is an open-access article distributed under the terms of the Creative Commons Attribution License (CC BY). The use, distribution or reproduction in other forums is permitted, provided the original author(s) and the copyright owner(s) are credited and that the original publication in this journal is cited, in accordance with accepted academic practice. No use, distribution or reproduction is permitted which does not comply with these terms.
*Correspondence: Maria Elisa Serrano, TWFyaWEuc2VycmFub19uYXZhY2VycmFkYUBrY2wuYWMudWs=
Disclaimer: All claims expressed in this article are solely those of the authors and do not necessarily represent those of their affiliated organizations, or those of the publisher, the editors and the reviewers. Any product that may be evaluated in this article or claim that may be made by its manufacturer is not guaranteed or endorsed by the publisher.
Research integrity at Frontiers
Learn more about the work of our research integrity team to safeguard the quality of each article we publish.