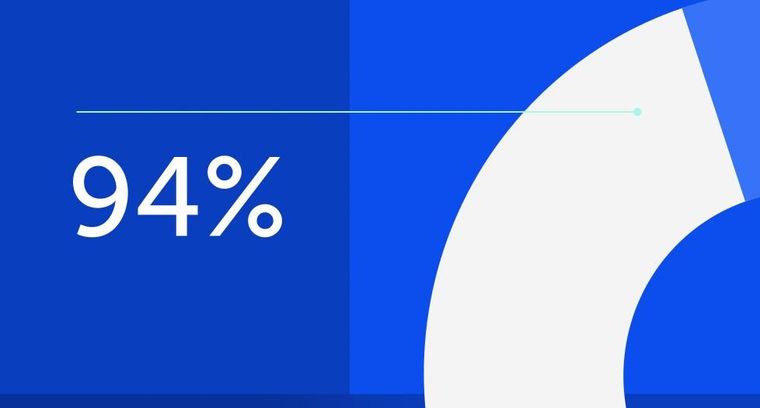
94% of researchers rate our articles as excellent or good
Learn more about the work of our research integrity team to safeguard the quality of each article we publish.
Find out more
ORIGINAL RESEARCH article
Front. Neurosci., 19 April 2022
Sec. Neurodegeneration
Volume 16 - 2022 | https://doi.org/10.3389/fnins.2022.792996
This article is part of the Research TopicPreventing Late-Onset Dementia: Mechanisms, Modifiable Risk Factors, and InterventionsView all 5 articles
Background: Changes in intestinal microbiome composition have been described in animal models of Alzheimer’s disease (AD) and AD patients. Here we investigated how well taxonomic and functional intestinal microbiome data and their combination with clinical data can be used to discriminate between amyloid-positive AD patients and cognitively healthy elderly controls.
Methods: In the present study we investigated intestinal microbiome in 75 amyloid-positive AD patients and 100 cognitively healthy controls participating in the AlzBiom study. We randomly split the data into a training and a validation set. Intestinal microbiome was measured using shotgun metagenomics. Receiver operating characteristic (ROC) curve analysis was performed to examine the discriminatory ability of intestinal microbiome among diagnostic groups.
Results: The best model for discrimination of amyloid-positive AD patients from healthy controls with taxonomic data was obtained analyzing 18 genera features, and yielded an area under the receiver operating characteristic curve (AUROC) of 0.76 in the training set and 0.61 in the validation set. The best models with functional data were obtained analyzing 17 GO (Gene Ontology) features with an AUROC of 0.81 in the training set and 0.75 in the validation set and 26 KO [Kyoto Encyclopedia of Genes and Genomes (KEGG) ortholog] features with an AUROC of 0.83 and 0.77, respectively. Using ensemble learning for these three models including a clinical model with the 4 parameters age, gender, BMI and ApoE yielded an AUROC of 0.92 in the training set and 0.80 in the validation set.
Discussion: In conclusion, we identified a specific Alzheimer signature in intestinal microbiome that can be used to discriminate amyloid-positive AD patients from healthy controls. The diagnostic accuracy increases from taxonomic to functional data and is even better when combining taxonomic, functional and clinical models. Intestinal microbiome represents an innovative diagnostic supplement and a promising area for developing novel interventions against AD.
Alzheimer’s disease (AD) is the leading cause of dementia in the elderly. Neuropathological hallmarks of AD are the deposition of extracellular β amyloid (Aβ) plaques, intracellular accumulation of tau-containing neurofibrillary tangles (NFTs), cortical atrophy and neuroinflammation in the brain (Querfurth and Laferla, 2010). The mechanisms leading to this AD pathology are still unknown. A multifactorial genesis seems likely, with inflammatory changes in the brain including altered microglia function playing an important role (McGeer and McGeer, 1995; Wyss-Coray, 2006). The mechanisms triggering these inflammatory changes are also unknown.
The intestine with its mucosal lymphoid tissue is one of the most important components of the immune system, containing about 70–80% of the body’s immune system (Sochocka et al., 2019). The colon contains about 39 × 1,012 bacteria and many more microorganisms (Sender et al., 2016). The totality of these microorganisms is called intestinal microbiome and contains about 100 × more genetic information than the human genome. The gut microbiome plays a pivotal role in supporting human health by regulation of metabolic, endocrine, immune and neurotrophic functions, e.g., by production of short-chain fatty acids (SCFAs) (Silva et al., 2020) and brain-derived neurotrophic factor (BDNF) (Lee et al., 2018; Ranuh et al., 2019). The influence of the gut microbiome is not restricted to the gastrointestinal (GI) tract, it also plays an important role in the bidirectional communication between the GI tract and the brain (microbiota-gut-brain axis; Morais et al., 2021).
Taxonomic and functional changes to the composition of the gut microbiome have been associated with multiple human diseases, e.g., with chronic inflammatory diseases such as Crohn’s disease, ulcerative colitis, and rheumatoid arthritis (Manichanh et al., 2006; Vigsnæs et al., 2012; Zhang et al., 2015). The composition of the gut microbiome can be impacted by host and environmental factors. It also holds different functional features across various life periods (Kundu et al., 2017). While gut microbiome remains mostly stable during the adulthood, it starts at the age of 65 years—interestingly the predilection age for AD—to shift into a less diverse and resilient state, which makes the microbiome more susceptible to environmental factors (Thursby and Juge, 2017).
Changes in the gut microbiome seem to be also linked with AD. Several recent studies have demonstrated changes in intestinal microbiome composition in animal models of AD (Harach et al., 2017; Chen et al., 2020), in human AD patients (Vogt et al., 2017; Li et al., 2019; Liu et al., 2019; Ling et al., 2020) and in MCI patients (Li et al., 2019; Liu et al., 2019). Most of these studies examined taxonomic data, only a few also functional data and none investigated the combination of taxonomic, functional and clinical data using an ensemble learning approach. Composition of intestinal microbiome is altered in animal models of AD even before the presence of amyloid plaques in the brain and thus seems to be an early phenomenon within AD pathogenesis (Chen et al., 2020). In addition, it has been shown that animal models of AD develop in the absence of intestinal microbiome less amyloid pathology in the brain than animal models of AD with existing or replaced intestinal microbiome (Harach et al., 2017; Dodiya et al., 2019, 2020).
The aim of the present study was to examine how well taxonomic and functional intestinal microbiome data and their combination with clinical data can be used to discriminate between amyloid-positive AD patients and cognitively healthy elderly controls.
Seventy-five amyloid-positive AD patients and 100 cognitively healthy controls were included in the study (Table 1). These participants were recruited from the AlzBiom study. AlzBiom is an observational longitudinal study performed in the Section for Dementia Research at the Department of Psychiatry and Psychotherapy in Tübingen. The aim of this ongoing study is to enroll mild cognitive impairment (MCI) patients, AD dementia patients and control subjects without subjective or objective cognitive decline. All participants underwent Mini-Mental State Examination (MMSE)-scoring (Folstein et al., 1975) and clinical assessment of cognitive status by means of the Clinical Dementia Rating (CDR) scale (Morris, 1993, 1997). For further statistical analysis, we randomly split the data into training set consisting of 132 individuals (AD: n = 59, HC: n = 73) and validation set comprising 43 individuals (AD: n = 16, HC: n = 27).
Table 1. Clinical and demographic characteristics of healthy control (HC) individuals and amyloid-positive Alzheimer’s disease (AD) patients.
AD patients fulfilled the NIA-AA core clinical criteria for probable AD dementia (McKhann et al., 2011), had a global CDR score of 0.5–1.0 and had evidence of cerebral Aβ accumulation with Aβ42 cerebrospinal fluid (CSF) levels of <600 pg/ml. HC individuals never reported subjective cognitive decline (SCD), had no history of neurological or psychiatric disease or any sign of cognitive decline and had a global CDR score of 0 and a MMSE score of ≥27.
The regional ethical committee approved the study and written informed consent was obtained from each individual.
The procedure for determining the apolipoprotein (ApoE) genotype was performed as previously described (Operto et al., 2019). Briefly, total DNA was obtained from the blood cellular fraction by proteinase K digestion followed by alcohol precipitation, using the QIAamp DNA Blood Maxi Kit. APOE genotyping was carried out using Applied Biosystems, Assay-on-demand TaqMan® SNP genotyping Assays, C_3084793_20 and C_904973_10 corresponding to APOE SNPs rs429358 and rs7412, respectively, and run on a StepOne Real-Time PCR Systems instrument. The ApoE ε4 positive genotype was assigned if at least one ε4 allele was present.
CSF was obtained by lumbar puncture with aseptic technique at the L3-L4 or L4-L5 intervertebral spinous process space, using a 22- or 21-gauge needle. The determination of the CSF concentrations of Amyloid-beta 1-42 (Aβ42) (cut-off: <600 pg/ml), total tau (t-tau) and phospho-tau181 (p-tau) was performed by commercial ELISAs (INNOTEST§ β-AMYLOID[1-42], Fujirebio Germany, detection limit 225 pg/ml; INNOTEST§ hTAU Ag, Fujirebio Germany, detection limit 40 pg/ml; INNOTEST§ PHOSPHO-TAU[181P], Fujirebio Germany, detection limit 15 pg/ml) according to the manufacturer’s instructions.
We collected stool samples in a sterile plastic device (Commode Specimen Collection System, Thermo Fisher Scientific, Pittsburgh, United States). The majority of stool samples were collected at the participants’ home using the DNA/RNA Shield Fecal Collection Tube R1101 (Zymo Research, Irvine, United States) and immediately sent to our laboratory by post. Samples were stored at −20°C and DNA was extracted on the same day using ZymoBiomics DNA Miniprep Kit D4300 (Zymo Research, Irvine, United States). DNA extraction was carried out at the end of sample collection in batches of 12–18 samples. Shotgun metagenomic sequencing was carried out in one batch at the GATC Biotech AG (Konstanz, Germany) using the NEBNext Ultra DNA Library kit (New England Biolabs, Ipswich, United States) for DNA library preparation and an Illumina HiSeq platform for sequencing. A paired-end sequencing approach with a targeted read length of 150 bp and an insert size of 550 bp was conducted. We aimed for a median sequencing depth of 40–50 million reads per sample.
Trimmomatic (version 0.35) was used to acquire high-quality reads through adapter removing and through a sliding window trimming (Bolger et al., 2014). Reads were trimmed to a minimum length of 100 bp. Quality control of trimmed reads was performed with FastQC version 0.11.5.1 We used SPAdes (version 3.9.0) to assemble metagenomic scaffolds with a minimum length of 1,000 bp (Bankevich et al., 2012).
Human contamination was removed by mapping reads against the human genome (GRCh38) using KneadData.2 Taxonomic profiling was carried out with Kaiju (version 1.5.0) using the greedy mode with a minimum alignment length of 11 amino acids, a maximum of 1 mismatch, and a match score of 65 (Menzel et al., 2016). The NCBI RefSeq was used as reference database. Counts for taxonomic units were normalized to a relative abundance through dividing the hits by the sample read count and multiplying the quotient by 106. The resulting unit is hits per million reads (HPM).
Community functional profiles were analyzed using HUMAnN 2.0 (version 0.11.2) using the standard parameters (Franzosa et al., 2018). According to OUT and Phylogenetic Investigation of Communities by Reconstruction of Unobserved States (PICRUSt) (Langille et al., 2013), we identified the functional categories based on a comparison of the Kyoto Encyclopedia of Genes and Genomes (KEGG) ortholog (KO)3 and of Gene Ontology (GO) Resource.4
For demographic characteristics and clinical information, the statistical software package SPSS (version 23) was used for data analyses. For all tests, the level of statistical significance was set to p < 0.05. Levene’s test served to assess homogeneity of variances. In case of continuous variables (i.e., age and BMI) t-tests for independent samples were used to detect differences between both groups. The non-parametric Mann-Whitney-U-test was conducted to detect group differences in MMSE and geriatric depression scale (GDS). The Pearson chi-square test was used to detect group differences in gender distribution, ApoE status and medication. To investigate the potential influence of antidepressant use and AChE inhibitor use on the relative abundance of genera, we performed a Hotelling-T2 test on the first 101 principal components (to capture at least 95% of the variance) of 1,014 normalized and isometric-logratio transformed genera data.
As features we investigated taxonomic data (phyla, genera and species), functional data (EGGNOG, GO, KO) and clinical meta data (age, gender, BMI, ApoE). We randomly split the data into a training and a validation set (ratio was 3:1) using caret library (createDataPartition). Feature pre-selection was performed in the training set using Wilcoxon test, and balances were calculated as part of compositional data analysis (Rivera-Pinto et al., 2018). Resulting models were then trained applying a logistic regression approach. In this training phase further feature selection was achieved. For this we performed fivefold cross-validation with 30 repeats of models shrinking the number of features step by step. Best models (Genera, GO, KO and clinical meta data) were then assessed by application to the validation data sets.
In the last step we joined the best performing models in an ensemble learning model, as described in http://www.scholarpedia.org/article/Ensemble_learning. Like with individual models, we trained the ensemble model with training data and assessed the quality using the validation data.
Receiver operating characteristic (ROC) curve analysis was performed to examine the discriminatory ability of intestinal microbiome among diagnostic groups. From the best models (Genera, GO, KO), bar plots were generated and heat maps showed their correlation with clinical and biomarker data. The pre-processing and statistical analysis of data was done using customized R scripts and HeidiSQL (1.3) as a database management tool in connection with RMariaDB (1.1.1). The model training and its feature selection was coded in R scripts relying on mlr (2.18.0) package. ROC curves were calculated employing OptimalCutpoints (1.1-4) and plotted with ggplot2 (3.3.5). Codes and data as well as some instructions are available at https://github.com/UliSchopp/AlzBiom. We give a full account of the method and all steps involved in Supplementary Material. The datasets presented in this study can be found at https://www.ebi.ac.uk/ena, PRJEB47976.
Characteristics of the study sample are presented in Table 1. HCs revealed significantly higher age (p = 0.032) and MMSE scores (p < 0.0001) compared to amyloid-positive AD patients. Gender was equally distributed between the investigated groups (p = 0.257). As expected, amyloid-positive AD patients took more frequently AChE inhibitors (p < 0.0001) and antidepressants (p < 0.0001). However, we found no statistically significant influence of antidepressant use (p = 0.25) and AChE inhibitor use (p = 0.21) on the relative abundance of genera.
The best model for discrimination of amyloid-positive AD patients from healthy controls with taxonomic data was obtained analyzing 18 genera features, and yielded an area under the receiver operating characteristic curve (AUROC) of 0.76 (95% CI: 0.67–0.84) in the training set and 0.61 (95% CI: 0.42–0.80) in the validation set (Figure 1A). The best models with functional data were obtained analyzing 17 GO features with an AUROC of 0.81 (95% CI: 0.73–0.88) in the training set and 0.75 (95% CI: 0.56–0.94) in the validation set (Figure 1B) and 26 KO features with an AUROC of 0.83 (95% CI: 0.74–0.91) and 0.77 (95% CI: 0.60–0.93), respectively (Figure 1C). Using ensemble learning for these three models including a clinical model with the 4 parameters age, gender, BMI and ApoE yielded an AUROC of 0.92 (95% CI: 0.87–0.97) in the training set and 0.80 (95% CI: 0.65–0.94) in the validation set (Figure 2).
Figure 1. ROC curves for discrimination between amyloid-positive Alzheimer’s disease (AD) patients and healthy controls in a training set (AD: n = 59, HC: n = 73) and an independent validation set (AD: n = 16, HC: n = 27) based on (A) 18 genera features, (B) 17 GO (Gene Ontology) features and (C) 26 KO [Kyoto Encyclopedia of Genes and Genomes (KEGG) ortholog] features.
Figure 2. ROC curves for ensemble learning for discrimination between amyloid-positive Alzheimer’s disease (AD) patients and healthy controls in a training set (AD: n = 59, HC: n = 73) and an independent validation set (AD: n = 16, HC: n = 27) including 4 models with 18 genera features, 17 GO (Gene Ontology) features, 26 KO [Kyoto Encyclopedia of Genes and Genomes (KEGG) ortholog] features and 4 clinical features (age, gender, BMI, ApoE).
Box plots of the absolute abundances of the 18 genera used in the genera model compared to all genera in our study cohort are shown in Figure 3. It turns out that all model genera are quite evenly distributed around the median of all genera. The differences of logarithmised medians of the features included in the genera, GO and KO model are shown in Tables 2–4. The non-significant associations between genera, GO and KO features and AD clinical and biomarker characteristics are presented in Tables 5–7.
Figure 3. Box plots of the absolute abundances of the 18 genera used in the genera model compared to all genera in our study cohort. The 0.1, 1.0, 5.0, and 10.0% quantiles are given as horizontal lines starting from below.
Table 3. The differences of logarithmised medians of the features included in the GO (Gene Ontology) model.
Table 4. The differences of logarithmised medians of the features included in the KO [Kyoto Encyclopedia of Genes and Genomes (KEGG) ortholog] model.
Table 5. The associations between genera and Alzheimer’s disease (AD) clinical and biomarker characteristics.
Table 6. The associations between GO (Gene Ontology) features and Alzheimer’s disease (AD) clinical and biomarker characteristics.
Table 7. The associations between KO [Kyoto Encyclopedia of Genes and Genomes (KEGG) ortholog] features and Alzheimer’s disease (AD) clinical and biomarker characteristics.
This study investigated the capability of taxonomic and functional intestinal microbiome data and their combination with clinical data in discriminating amyloid-positive AD patients from cognitively healthy elderly controls. To our knowledge, this is the first study addressing this question using taxonomic and functional data of shotgun metagenomics in a training cohort and an independent validation cohort. Within taxonomic data, we identified a genera model and within functional data, we identified a GO and KO model showing best results for discrimination of amyloid-positive AD patients from healthy controls. Functional data showed a higher diagnostic accuracy than taxonomic data. In a next step, we combined taxonomic, functional and clinical models with an ensemble learning approach. This ensemble model performed better than any of its parts in the training data and with respect to genera and clinical model also in the validation data. Our finding of an added value of including clinical variables to gut microbiome data is in line with previous studies, demonstrating an improved accuracy for AD diagnosis adding clinical variables to blood-based biomarkers (O’Bryant et al., 2011) or MRI data (Vemuri et al., 2008).
Several recent studies have demonstrated an altered intestinal microbiome composition in animal models of AD (Harach et al., 2017) and in human AD patients (Vogt et al., 2017; Li et al., 2019; Liu et al., 2019; Ling et al., 2020) and MCI patients (Li et al., 2019; Liu et al., 2019). Three studies investigated the diagnostic accuracy for discrimination of clinically diagnosed AD patients from healthy controls using genera data with AUROC values ranging from 0.78 to 0.94 (Li et al., 2019; Liu et al., 2019; Ling et al., 2020). However, all three studies had some limitations: They used limited bacterial 16S rRNA sequencing technology, they did not validate their results in an independent cohort with the same diagnosis and they did not examine the diagnostic accuracy of functional data. Instead, Li and colleagues (Li et al., 2019) showed that their genera model from AD patients was able to predict 28 of 30 patients with MCI, suggesting a similar pattern of gut microbiome changes in AD and MCI patients. In contrast, Liu and colleagues (Liu et al., 2019) could discriminate AD patients and MCI patients using a genera model with an AUROC of 0.93, suggesting a different pattern of gut microbiome changes in AD and MCI patients. Both results are conflicting and also surprising, because MCI is a heterogeneous syndrome with varying clinical outcomes: up to 60% of MCI patients develop dementia within a 10-year period, however, many people remain cognitively stable or regain normal cognitive function (Manly et al., 2008; Mitchell and Shiri-Feshki, 2009; Korolev et al., 2016). We hope in the future to be able to resolve these conflicting results by providing more information for differentiating microbiome patterns of MCI patients and testing how much overlap there is with more advanced pathology.
Interestingly, 10 of the 18 taxa in our genera model belong to the phylum proteobacteria. Of these, 5 show higher levels in healthy individuals and 5 in AD patients. This means, that 50% of taxa with higher levels in healthy controls and 62.5% of taxa with higher levels in AD patients belong to the phylum proteobacteria. However, on the phylum level we found no significant difference of the relative abundance of proteobacteria between AD patients and healthy controls (p = 0.41). This result indicates that differences of taxa may play rather a role on the genera level than on phylum level. The potential meaning of proteobacteria in AD has been shown in a previous study, describing increased proteobacteria levels in AD patients, which correlated with the severity of cognitive impairment (Liu et al., 2019). From the 17 features in the GO model, aldehyde dehydrogenase showed the highest activity in AD patients. This is an interesting finding, as this enzyme has been associated with neuroprotective effects due to its aldehyde detoxification and with neurodegenerative diseases such as AD (Michel et al., 2010; Grünblatt and Riederer, 2016). From the 26 features in the KO model, putative multiple sugar transport system permease protein showed the highest activity in AD patients. This protein belongs to the ATP-binding cassette (ABC) transporters which have been associated with AD pathogenesis due to their ability to transport Aβ peptides out of the brain (Aβ brain clearance; Behl et al., 2021).
The intestinal microbiome may have a direct impact on amyloid pathology and neuroinflammation in the brain. This hypothesis is supported by recent preclinical studies showing that the absence or presence of intestinal microbiome influenced the degree of cerebral amyloid pathology in animal models of AD (Harach et al., 2017; Dodiya et al., 2019, 2020). Germ-free Alzheimer’s mice had less amyloid plaques in the brain than Alzheimer’s mice with normal intestinal microbiome. The colonization of germ-free Alzheimer’s mice with microbiota from conventionally reared Alzheimer’s mice resulted in a significant increase in cerebral amyloid load in the brain, but not the microbiota of control mice (Harach et al., 2017). In addition, treatment of male Alzheimer’s mice with an antibiotic cocktail resulted in changes of gut microbiome and was associated with reduced amyloid pathology in the brain and transplants of fecal microbiota from male Alzheimer’s mice restored the gut microbiome and partially restored amyloid pathology in the brain (Dodiya et al., 2019). A recent study showed that when the gut microbiota from AD patients were transplanted into Alzheimer’s mice, the recipient mice showed more severe cognitive impairment, activated intestinal NLRP3 inflammasome, increased levels of inflammatory factors in peripheral blood and activated microglia in the hippocampus, and these effects could be reversed by transplantation of healthy human gut microbiota (Shen et al., 2020).
Which mechanisms could mediate the effects of intestinal microbiome on amyloid pathology in AD patients? A recent study has identified the bacterial products lipopolysaccharide (LPS) and the short chain fatty acids (SCFAs) acetic acid and valeric acid to be positively correlated with amyloid load in the brain of AD patients, whereas butyric acid showed an inverse correlation (Marizzoni et al., 2020). In addition, an in vitro study revealed an anti-Aβ aggregation efficacy for the SCFAs valeric acid, butyric acid, and propionic acid (Ho et al., 2018). This anti-amyloidogenic effect of some SCFAs could be explained in part by their influence on maturation and function of microglia in the brain (Erny et al., 2015), which can clean up Aβ fibrils and typically surrounds amyloid plaques in the brains of AD patients (Calsolaro and Edison, 2016). In contrast, LPS is part of the outer membrane of Gram-positive bacteria and has been shown to trigger systemic inflammation and the release of proinflammatory cytokines (Zhao and Lukiw, 2015) and to potentiate amyloid fibrillogenesis (Asti and Gioglio, 2014).
The findings that gut microbiome is changed in AD and directly influences the degree of amyloid pathology and neuroinflammation in the brain indicate that it could represent a promising target for the prevention or treatment of AD. In this context, the use of a probiotic cocktail for 12 weeks has shown beneficial effects on cognitive performance in AD patients (Akbari et al., 2016). The beneficial effect of gut microbiota on cognition could be explained in part by the upregulation of hippocampal BDNF and cAMP-response element-binding protein (CREB), as shown for different lactobacillus species (Lee et al., 2018; Ranuh et al., 2019). However, a meta-analysis examining the effects of probiotic supplementation on cognition in dementia could not find a significant beneficial effect (Krüger et al., 2021).
Despite its significant implications, the present study has some limitations to be discussed. First, this study used a cross-sectional design. To determine whether the characteristic intestinal microbiome in AD is the cause or result of the disease, well designed longitudinal studies with adequate sample size are needed. Second, CSF was not available in our healthy control group. The reason for that is that we got no permission from our ethics committee to perform lumbar puncture in healthy controls without any signs of cognitive impairment. As the prevalence of preclinical AD among cognitively healthy elderly people is estimated to be about 20%, we cannot exclude the same prevalence for our cohort (Parnetti et al., 2019). Therefore, our findings should be replicated in an independent cohort including healthy controls without signs of AD pathology. Third, even though we found no statistically significant influence of antidepressant use (p = 0.25) and AChE inhibitor use (p = 0.21) on the relative abundance of genera, taxonomic and functional differences between the AD patients and healthy controls may have been influenced by drug exposure. Furthermore, we measured no metabolites in the collected gut samples.
In conclusion, we showed that intestinal microbiome can be used to discriminate amyloid-positive AD patients from healthy controls. The diagnostic accuracy increases from taxonomic to functional data and is even better when combining taxonomic, functional and clinical models. Intestinal microbiome represents a promising area for developing novel interventions against AD.
The datasets presented in this study can be found in online repositories. The names of the repository/repositories and accession number(s) can be found below: https://www.ebi.ac.uk/ena, PRJEB47976.
The studies involving human participants were reviewed and approved by Institut für Ethik und Geschichte der Medizin, Gartenstr. 47, 72074 Tübingen. The patients/participants provided their written informed consent to participate in this study.
CL and MW participated in study concept and design. CL, SM, OP, VR, MM, SP, US, and MW participated in the acquisition, analysis, and interpretation of data, and in the critical revision of the manuscript. CL drafted the manuscript. US did the statistical analysis. All authors read and approved the submitted version of the manuscript.
This study was supported in part by an AKF-Grant (374-1-0) of the University of Tübingen and a grant by the Forum Gesundheitsstandort BW (AZ: 32-5400/58/3).
The authors declare that the research was conducted in the absence of any commercial or financial relationships that could be construed as a potential conflict of interest.
All claims expressed in this article are solely those of the authors and do not necessarily represent those of their affiliated organizations, or those of the publisher, the editors and the reviewers. Any product that may be evaluated in this article, or claim that may be made by its manufacturer, is not guaranteed or endorsed by the publisher.
The Supplementary Material for this article can be found online at: https://www.frontiersin.org/articles/10.3389/fnins.2022.792996/full#supplementary-material
Akbari, E., Asemi, Z., Daneshvar Kakhaki, R., Bahmani, F., Kouchaki, E., Tamtaji, O. R., et al. (2016). Effect of probiotic supplementation on cognitive function and metabolic status in alzheimer’s disease: a randomized, double-blind and controlled trial. Front. Aging Neurosci. 8:256.
Asti, A., and Gioglio, L. (2014). Can a bacterial endotoxin be a key factor in the kinetics of amyloid fibril formation? J. Alzheimers Dis. 39, 169–179. doi: 10.3233/JAD-131394
Bankevich, A., Nurk, S., Antipov, D., Gurevich, A. A., Dvorkin, M., Kulikov, A. S., et al. (2012). SPAdes: a new genome assembly algorithm and its applications to single-cell sequencing. J. Comput. Biol. 19, 455–477. doi: 10.1089/cmb.2012.0021
Behl, T., Kaur, I., Sehgal, A., Kumar, A., Uddin, M. S., and Bungau, S. (2021). The Interplay of ABC Transporters in Aβ translocation and cholesterol metabolism: implicating their roles in Alzheimer’s Disease. Mol. Neurobiol. 58, 1564–1582. doi: 10.1007/s12035-020-02211-x
Bolger, A. M., Lohse, M., and Usadel, B. (2014). Trimmomatic: a flexible trimmer for Illumina sequence data. Bioinformatics 30, 2114–2120. doi: 10.1093/bioinformatics/btu170
Calsolaro, V., and Edison, P. (2016). Neuroinflammation in Alzheimer’s disease: current evidence and future directions. Alzheimers Dement. 12, 719–732. doi: 10.1016/j.jalz.2016.02.010
Chen, Y., Fang, L., Chen, S., Zhou, H., Fan, Y., Lin, L., et al. (2020). Gut microbiome alterations precede cerebral amyloidosis and microglial pathology in a mouse model of Alzheimer’s Disease. Biomed. Res. Int. 2020:8456596. doi: 10.1155/2020/8456596
Dodiya, H. B., Frith, M., Sidebottom, A., Cao, Y., Koval, J., Chang, E., et al. (2020). Synergistic depletion of gut microbial consortia, but not individual antibiotics, reduces amyloidosis in APPPS1-21 Alzheimer’s transgenic mice. Sci. Rep. 10:8183. doi: 10.1038/s41598-020-64797-5
Dodiya, H. B., Kuntz, T., Shaik, S. M., Baufeld, C., Leibowitz, J., Zhang, X., et al. (2019). Sex-specific effects of microbiome perturbations on cerebral Aβ amyloidosis and microglia phenotypes. J. Exp. Med. 216, 1542–1560. doi: 10.1084/jem.20182386
Erny, D., Hrabe De Angelis, A. L., Jaitin, D., Wieghofer, P., Staszewski, O., David, E., et al. (2015). Host microbiota constantly control maturation and function of microglia in the CNS. Nat. Neurosci. 18, 965–977. doi: 10.1038/nn.4030
Folstein, M. F., Folstein, S. E., and Mchugh, P. R. (1975). Mini-mental state”. A practical method for grading the cognitive state of patients for the clinician. J. Psychiatr. Res. 12, 189–198.
Franzosa, E. A., Mciver, L. J., Rahnavard, G., Thompson, L. R., Schirmer, M., Weingart, G., et al. (2018). Species-level functional profiling of metagenomes and metatranscriptomes. Nat. Methods 15, 962–968. doi: 10.1038/s41592-018-0176-y
Grünblatt, E., and Riederer, P. (2016). Aldehyde dehydrogenase (ALDH) in Alzheimer’s and Parkinson’s disease. J. Neural. Transm. 123, 83–90. doi: 10.1007/s00702-014-1320-1
Harach, T., Marungruang, N., Duthilleul, N., Cheatham, V., Mc Coy, K. D., Frisoni, G., et al. (2017). Reduction of Abeta amyloid pathology in APPPS1 transgenic mice in the absence of gut microbiota. Sci. Rep. 7:41802.
Ho, L., Ono, K., Tsuji, M., Mazzola, P., Singh, R., and Pasinetti, G. M. (2018). Protective roles of intestinal microbiota derived short chain fatty acids in Alzheimer’s disease-type beta-amyloid neuropathological mechanisms. Expert. Rev. Neurother. 18, 83–90. doi: 10.1080/14737175.2018.1400909
Korolev, I. O., Symonds, L. L., and Bozoki, A. C. (2016). Predicting progression from mild cognitive impairment to Alzheimer’s Dementia using clinical, MRI, and Plasma Biomarkers via probabilistic pattern classification. PLoS One 11:e0138866. doi: 10.1371/journal.pone.0138866
Krüger, J. F., Hillesheim, E., Pereira, A., Camargo, C. Q., and Rabito, E. I. (2021). Probiotics for dementia: a systematic review and meta-analysis of randomized controlled trials. Nutr. Rev. 79, 160–170. doi: 10.1093/nutrit/nuaa037
Kundu, P., Blacher, E., Elinav, E., and Pettersson, S. (2017). Our gut microbiome: the evolving inner self. Cell 171, 1481–1493. doi: 10.1016/j.cell.2017.11.024
Langille, M. G., Zaneveld, J., Caporaso, J. G., Mcdonald, D., Knights, D., Reyes, J. A., et al. (2013). Predictive functional profiling of microbial communities using 16S rRNA marker gene sequences. Nat. Biotechnol. 31, 814–821. doi: 10.1038/nbt.2676
Lee, H. J., Lim, S. M., and Kim, D. H. (2018). Lactobacillus johnsonii CJLJ103 Attenuates scopolamine-induced memory impairment in mice by increasing BDNF expression and Inhibiting NF-κB Activation. J. Microbiol. Biotechnol. 28, 1443–1446. doi: 10.4014/jmb.1805.05025
Li, B., He, Y., Ma, J., Huang, P., Du, J., Cao, L., et al. (2019). Mild cognitive impairment has similar alterations as Alzheimer’s disease in gut microbiota. Alzheimers Dement. 15, 1357–1366. doi: 10.1016/j.jalz.2019.07.002
Ling, Z., Zhu, M., Yan, X., Cheng, Y., Shao, L., Liu, X., et al. (2020). Structural and functional dysbiosis of fecal microbiota in chinese patients with Alzheimer’s Disease. Front. Cell Dev. Biol. 8:634069.
Liu, P., Wu, L., Peng, G., Han, Y., Tang, R., Ge, J., et al. (2019). Altered microbiomes distinguish Alzheimer’s disease from amnestic mild cognitive impairment and health in a Chinese cohort. Brain Behav. Immun. 80, 633–643. doi: 10.1016/j.bbi.2019.05.008
Manichanh, C., Rigottier-Gois, L., Bonnaud, E., Gloux, K., Pelletier, E., Frangeul, L., et al. (2006). Reduced diversity of faecal microbiota in Crohn’s disease revealed by a metagenomic approach. Gut 55, 205–211. doi: 10.1136/gut.2005.073817
Manly, J. J., Tang, M. X., Schupf, N., Stern, Y., Vonsattel, J. P., and Mayeux, R. (2008). Frequency and course of mild cognitive impairment in a multiethnic community. Ann. Neurol. 63, 494–506. doi: 10.1002/ana.21326
Marizzoni, M., Cattaneo, A., Mirabelli, P., Festari, C., Lopizzo, N., Nicolosi, V., et al. (2020). Short-chain fatty acids and lipopolysaccharide as mediators between gut dysbiosis and amyloid pathology in Alzheimer’s Disease. J. Alzheimers Dis. 78, 683–697. doi: 10.3233/JAD-200306
McGeer, P. L., and McGeer, E. G. (1995). The inflammatory response system of brain: implications for therapy of Alzheimer and other neurodegenerative diseases. Brain Res. Brain Res. Rev. 21, 195–218. doi: 10.1016/0165-0173(95)00011-9
McKhann, G. M., Knopman, D. S., Chertkow, H., Hyman, B. T., Jack, C. R. Jr., Kawas, C. H., et al. (2011). The diagnosis of dementia due to Alzheimer’s disease: recommendations from the National Institute on Aging-Alzheimer’s Association workgroups on diagnostic guidelines for Alzheimer’s disease. Alzheimers Dement. 7, 263–269. doi: 10.1016/j.jalz.2011.03.005
Menzel, P., Ng, K. L., and Krogh, A. (2016). Fast and sensitive taxonomic classification for metagenomics with Kaiju. Nat. Commun. 7:11257. doi: 10.1038/ncomms11257
Michel, T. M., Gsell, W., Käsbauer, L., Tatschner, T., Sheldrick, A. J., Neuner, I., et al. (2010). Increased activity of mitochondrial aldehyde dehydrogenase (ALDH) in the putamen of individuals with Alzheimer’s disease: a human postmortem study. J. Alzheimers Dis. 19, 1295–1301. doi: 10.3233/JAD-2010-1326
Mitchell, A. J., and Shiri-Feshki, M. (2009). Rate of progression of mild cognitive impairment to dementia–meta-analysis of 41 robust inception cohort studies. Acta Psychiatr. Scand. 119, 252–265. doi: 10.1111/j.1600-0447.2008.01326.x
Morais, L. H., Schreiber, H. L. T., and Mazmanian, S. K. (2021). The gut microbiota-brain axis in behaviour and brain disorders. Nat. Rev. Microbiol. 19, 241–255. doi: 10.1038/s41579-020-00460-0
Morris, J. C. (1993). The Clinical Dementia Rating (CDR): current version and scoring rules. Neurology 43, 2412–2414. doi: 10.1212/wnl.43.11.2412-a
Morris, J. C. (1997). Clinical dementia rating: a reliable and valid diagnostic and staging measure for dementia of the Alzheimer type. Int. Psychogeriatr. 9, 173–176. doi: 10.1017/s1041610297004870
O’Bryant, S. E., Xiao, G., Barber, R., Huebinger, R., Wilhelmsen, K., Edwards, M., et al. (2011). A blood-based screening tool for Alzheimer’s disease that spans serum and plasma: findings from TARC and ADNI. PLoS One 6:e28092. doi: 10.1371/journal.pone.0028092
Operto, G., Molinuevo, J. L., Cacciaglia, R., Falcon, C., Brugulat-Serrat, A., Suárez-Calvet, M., et al. (2019). Interactive effect of age and APOE-ε4 allele load on white matter myelin content in cognitively normal middle-aged subjects. Neuroimage Clin. 24:101983. doi: 10.1016/j.nicl.2019.101983
Parnetti, L., Chipi, E., Salvadori, N., D’andrea, K., and Eusebi, P. (2019). Prevalence and risk of progression of preclinical Alzheimer’s disease stages: a systematic review and meta-analysis. Alzheimers Res. Ther. 11:7. doi: 10.1186/s13195-018-0459-7
Ranuh, R., Athiyyah, A. F., Darma, A., Risky, V. P., Riawan, W., Surono, I. S., et al. (2019). Effect of the probiotic Lactobacillus plantarum IS-10506 on BDNF and 5HT stimulation: role of intestinal microbiota on the gut-brain axis. Iran J. Microbiol. 11, 145–150.
Rivera-Pinto, J., Egozcue, J. J., Pawlowsky-Glahn, V., Paredes, R., Noguera-Julian, M., and Calle, M. L. (2018). Balances: a new perspective for microbiome analysis. mSystems 3:53. doi: 10.1128/mSystems.00053-18
Sender, R., Fuchs, S., and Milo, R. (2016). Are we really vastly outnumbered? revisiting the ratio of bacterial to host cells in humans. Cell 164, 337–340. doi: 10.1016/j.cell.2016.01.013
Shen, H., Guan, Q., Zhang, X., Yuan, C., Tan, Z., Zhai, L., et al. (2020). New mechanism of neuroinflammation in Alzheimer’s disease: the activation of NLRP3 inflammasome mediated by gut microbiota. Prog. Neuropsychopharmacol. Biol. Psychiatry 100:109884. doi: 10.1016/j.pnpbp.2020.109884
Silva, Y. P., Bernardi, A., and Frozza, R. L. (2020). The role of short-chain fatty acids from gut microbiota in gut-brain communication. Front. Endocrinol. 11:25.
Sochocka, M., Donskow-Łysoniewska, K., Diniz, B. S., Kurpas, D., Brzozowska, E., and Leszek, J. (2019). The gut microbiome alterations and inflammation-driven pathogenesis of alzheimer’s disease-a critical review. Mol. Neurobiol. 56, 1841–1851. doi: 10.1007/s12035-018-1188-4
Thursby, E., and Juge, N. (2017). Introduction to the human gut microbiota. Biochem. J. 474, 1823–1836. doi: 10.1042/bcj20160510
Vemuri, P., Gunter, J. L., Senjem, M. L., Whitwell, J. L., Kantarci, K., Knopman, D. S., et al. (2008). Alzheimer’s disease diagnosis in individual subjects using structural MR images: validation studies. Neuroimage 39, 1186–1197. doi: 10.1016/j.neuroimage.2007.09.073
Vigsnæs, L. K., Brynskov, J., Steenholdt, C., Wilcks, A., and Licht, T. R. (2012). Gram-negative bacteria account for main differences between faecal microbiota from patients with ulcerative colitis and healthy controls. Benef. Microb. 3, 287–297. doi: 10.3920/BM2012.0018
Vogt, N. M., Kerby, R. L., Dill-Mcfarland, K. A., Harding, S. J., Merluzzi, A. P., Johnson, S. C., et al. (2017). Gut microbiome alterations in Alzheimer’s disease. Sci. Rep. 7:13537.
Wyss-Coray, T. (2006). Inflammation in Alzheimer disease: driving force, bystander or beneficial response? Nat. Med. 12, 1005–1015. doi: 10.1038/nm1484
Zhang, X., Zhang, D., Jia, H., Feng, Q., Wang, D., Liang, D., et al. (2015). The oral and gut microbiomes are perturbed in rheumatoid arthritis and partly normalized after treatment. Nat. Med. 21, 895–905. doi: 10.1038/nm.3914
Keywords: Alzheimer’s disease, intestinal microbiome, taxonomic data, functional data, ensemble learning
Citation: Laske C, Müller S, Preische O, Ruschil V, Munk MHJ, Honold I, Peter S, Schoppmeier U and Willmann M (2022) Signature of Alzheimer’s Disease in Intestinal Microbiome: Results From the AlzBiom Study. Front. Neurosci. 16:792996. doi: 10.3389/fnins.2022.792996
Received: 11 October 2021; Accepted: 24 March 2022;
Published: 19 April 2022.
Edited by:
Genevieve Zara Steiner, Western Sydney University, AustraliaReviewed by:
Amy Mackos, The Ohio State University, United StatesCopyright © 2022 Laske, Müller, Preische, Ruschil, Munk, Honold, Peter, Schoppmeier and Willmann. This is an open-access article distributed under the terms of the Creative Commons Attribution License (CC BY). The use, distribution or reproduction in other forums is permitted, provided the original author(s) and the copyright owner(s) are credited and that the original publication in this journal is cited, in accordance with accepted academic practice. No use, distribution or reproduction is permitted which does not comply with these terms.
*Correspondence: Christoph Laske, Y2hyaXN0b3BoLmxhc2tlQG1lZC51bmktdHVlYmluZ2VuLmRl; Ulrich Schoppmeier, dWxyaWNoLnNjaG9wcG1laWVyQG1lZC51bmktdHVlYmluZ2VuLmRl
†These authors have contributed equally to this work
Disclaimer: All claims expressed in this article are solely those of the authors and do not necessarily represent those of their affiliated organizations, or those of the publisher, the editors and the reviewers. Any product that may be evaluated in this article or claim that may be made by its manufacturer is not guaranteed or endorsed by the publisher.
Research integrity at Frontiers
Learn more about the work of our research integrity team to safeguard the quality of each article we publish.