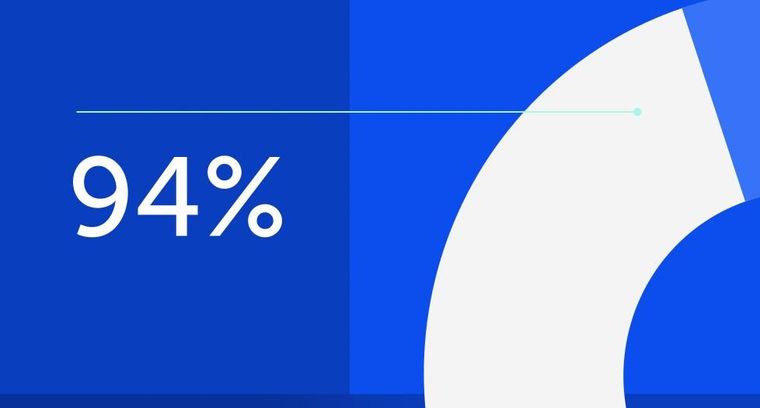
94% of researchers rate our articles as excellent or good
Learn more about the work of our research integrity team to safeguard the quality of each article we publish.
Find out more
EDITORIAL article
Front. Neurosci., 06 January 2023
Sec. Brain Imaging Methods
Volume 16 - 2022 | https://doi.org/10.3389/fnins.2022.1128938
This article is part of the Research TopicMultimodal Brain Image Fusion: Methods, Evaluations, and ApplicationsView all 13 articles
Editorial on the Research Topic
Multimodal brain image fusion: Methods, evaluations, and applications
Multimodal medical imaging is playing an increasingly critical role in the diagnosis and treatment of various brain diseases like glioma, Alzheimer, ischemic stroke, epilepsy, etc. Medical images with different modalities such as computed tomography (CT), magnetic resonance imaging (MRI), and positron emission tomography (PET) focus on different categories of pathological information. Medical image fusion aims to combine the complementary information captured by different imaging modalities for better disease diagnosis and treatment. In recent years, medical image fusion has emerged as a very active topic with various fusion methods being proposed. In addition, the performance evaluation and downstream applications of medical image fusion are also attracting more and more attention. This Research Topic focuses on reporting advanced studies related to multimodal brain image fusion, including image fusion methods, objective evaluation approaches and specific applications in clinical problems. Twelve of the 16 articles submitted to this Research Topic were accepted for publication after a thorough peer-review process. A summary of the key research findings of these works is provided from three aspects as below.
Image registration is the prerequisite of many medical image processing tasks such as fusion and segmentation. Wang J. et al. proposed a medical image registration method based on the bounded generalized Gaussian mixture model (BGGMM), which can thoroughly describe the joint intensity vector distribution of pixels and highlight image details. The mixture model is formulated based on a maximum likelihood framework, and is solved by an expectation-maximization algorithm. With regard to image fusion methods, Wang A. et al. presented a disentangled representation-based multimodal brain image fusion method via group lasso penalty using an auto-encoder-based deep learning framework, aiming to fully exploit the redundancy and complement prior relationships among multimodal source images. A complementary group lasso penalty was designed to promote the disentanglement ability and ensure the complementary feature maps of significant modality information. This study demonstrated that the disentangled representation can improve the interpretability of feature representation, leading to better fusion quality. Zhang et al. proposed a local extreme map guided multimodal brain image fusion method to improve the feature extraction ability of the guided image filter. By iteratively applying this local extreme map guided image filter, the proposed method can extract multiple scales of bright and dark features from the multimodal brain images, and integrate these salient features into one informative fused image. In addition, the proposed scheme can be incorporated with various guided filters or other similar filters in pursuit of improving their feature extraction ability. In comparison to the great attention paid to the study of image fusion methods, few works have explored dedicated quality assessment approaches for medical image fusion. To address this issue, Tang et al. proposed a novel quality assessment method for medial image fusion based on the conditional generative adversarial networks by adopting the mean opinion scores (MOS) of the radiologists as the guiding condition. They demonstrated that their proposed method outperforms several commonly-used quality assessment metrics of image fusion, with excellent agreement with subjective evaluations.
Multimodal medical image fusion has been verified to be of great significance in various related high-level vision tasks such as classification and segmentation. Yi et al. proposed a multimodal classification architecture for the severity diagnosis of glaucoma. The proposed method integrates fundus images and gray scale images of the visual field as the input of the classification model. In addition, they introduced a plug-and-play classifier that adopts the Vision Transformer to extract the global dependencies of images, leading to improved accuracy of the diagnostic task. Li et al. conducted a study to investigate the stage of bi-modal fusion based on EEG and fNIRS for the classification task in hybrid brain-computer interfaces (BCIs). A Y-shaped neural network that fuses the bi-modal information in different stages was proposed. This study demonstrated that the early-stage fusion of EEG and fNIRS have significantly higher performance compared to middle-stage fusion and late-stage fusion. Liu et al. introduced both pixel-level and feature-level medical image fusion techniques for brain tumor segmentation, aiming to achieve more sufficient utilization of multimodal information. They presented a convolutional neural network (CNN)-based 3D pixel-level image fusion network to enrich the input modalities of the segmentation model and designed an attention-based feature fusion module for multimodal feature refinement. Xu et al. proposed a hybrid feature extraction network for medical image segmentation based on CNNs and Transformer. The proposed network can integrate the advantages of Transformer in capturing global contextual information and CNNs in extracting local features. Additionally, a multi-dimensional statistical feature extraction module was designed to strengthen low-dimensional texture features and enhance the segmentation performance. Tian et al. presented a method to combine light sheet microscopy (LSM) data with magnetic resonance histology (MRH) of the same specimen, with the aim of restoring the morphology of the LSM images to the in-skull geometry. They developed an image processing pipeline to restore the correct brain morphology of 3-dimensional cleared or stained mouse brain by registering the cleared brain data to MRH of the same specimen. Peng et al. introduced the minimally invasive puncture and drainage (MIPD) surgery using mixed reality holographic navigation technology (MRHNT) via integrating the holographic image and the real head. By wearing mixed reality holographic equipment, the precise location of intracranial hematomas, tumors, ventricles, and other structures with the perspective function can be understood, laying a theoretical foundation for implementation in neurosurgery.
Mononen et al. conducted a study to evaluate the variability among tasks of magnetoencephalography (MEG)-functional magnetic resonance imaging (fMRI) relationship using data recorded during three distinct naming tasks from the same set of participants. The results demonstrated that the MEG-fMRI correlation pattern varies according to the performed task. In addition, the electromagnetic-hemodynamic correlation could serve as a more sensitive proxy for task-dependent neural engagement in cognitive tasks than isolated within-modality measures. Gallego-Rudolf et al. characterized the impact of the ballistocardiographic (BCG) artifact on resting-state EEG spectral properties and compared the effectiveness of seven common BCG correction methods to preserve EEG spectral features. They also assessed if these methods retained posterior alpha power reactivity to an eyes closure-opening task and compared the results from EEG-informed fMRI analysis using different BCG correction approaches.
All authors listed have made a substantial, direct, and intellectual contribution to the work and approved it for publication.
We appreciate the contributions of all the authors, the reviewers, and the editorial board members for contributing to this Research Topic. We hope that this Research Topic can make a broad impact on the study of multimodal brain image fusion including methods, evaluations and applications.
The authors declare that the research was conducted in the absence of any commercial or financial relationships that could be construed as a potential conflict of interest.
All claims expressed in this article are solely those of the authors and do not necessarily represent those of their affiliated organizations, or those of the publisher, the editors and the reviewers. Any product that may be evaluated in this article, or claim that may be made by its manufacturer, is not guaranteed or endorsed by the publisher.
Keywords: multimodal medical images, image registration, image fusion, quality assessment, pattern recognition, deep learning
Citation: Liu Y, Ma J, Zhang Q, Wei W, Chen X and Liu Z (2023) Editorial: Multimodal brain image fusion: Methods, evaluations, and applications. Front. Neurosci. 16:1128938. doi: 10.3389/fnins.2022.1128938
Received: 21 December 2022; Accepted: 28 December 2022;
Published: 06 January 2023.
Edited and reviewed by: Vince D. Calhoun, Georgia State University, United States
Copyright © 2023 Liu, Ma, Zhang, Wei, Chen and Liu. This is an open-access article distributed under the terms of the Creative Commons Attribution License (CC BY). The use, distribution or reproduction in other forums is permitted, provided the original author(s) and the copyright owner(s) are credited and that the original publication in this journal is cited, in accordance with accepted academic practice. No use, distribution or reproduction is permitted which does not comply with these terms.
*Correspondence: Yu Liu, eXVsaXVAaGZ1dC5lZHUuY24=; Jiayi Ma,
anltYTIwMTBAZ21haWwuY29t; Qiang Zhang,
cXpoYW5nQHhpZGlhbi5lZHUuY24=; Wei Wei,
d2Vpd2VpbGxAMTI2LmNvbQ==; Xun Chen,
eHVuY2hlbkB1c3RjLmVkdS5jbg==; Zheng Liu,
emhlbmcubGl1QHViYy5jYQ==
Disclaimer: All claims expressed in this article are solely those of the authors and do not necessarily represent those of their affiliated organizations, or those of the publisher, the editors and the reviewers. Any product that may be evaluated in this article or claim that may be made by its manufacturer is not guaranteed or endorsed by the publisher.
Research integrity at Frontiers
Learn more about the work of our research integrity team to safeguard the quality of each article we publish.