- 1University Lyon 1, Lyon, France
- 2Lyon Neuroscience Research Center, CRNL, INSERM, U1028, CNRS, UMR 5292, Lyon, France
- 3Institut des Sciences Cognitives Marc Jeannerod, CNRS, UMR 5229, Bron, France
The development of reliable assistive devices for patients that suffer from motor impairments following central nervous system lesions remains a major challenge in the field of non-invasive Brain-Computer Interfaces (BCIs). These approaches are predominated by electroencephalography and rely on advanced signal processing and machine learning methods to extract neural correlates of motor activity. However, despite tremendous and still ongoing efforts, their value as effective clinical tools remains limited. We advocate that a rather overlooked research avenue lies in efforts to question neurophysiological markers traditionally targeted in non-invasive motor BCIs. We propose an alternative approach grounded by recent fundamental advances in non-invasive neurophysiology, specifically subject-specific feature extraction of sensorimotor bursts of activity recorded via (possibly magnetoencephalography-optimized) electroencephalography. This path holds promise in overcoming a significant proportion of existing limitations, and could foster the wider adoption of online BCIs in rehabilitation protocols.
Introduction
Central nervous system (CNS) lesions have a major socioeconomic impact on modern societies (World Health Organization, 2011). A grave manifestation involves upper limb motor deficits that affect the quality of life of patients to various degrees depending on both the extent and the exact nature of the lesions. Emerging medical technologies may alleviate these deficits and therefore improve the lives of millions of patients in several ways, diminishing the psychological burden and societal discrimination against people with disabilities.
A prominent direction lies in the development of Brain-Computer Interfaces (BCIs) whose output can be translated into motor commands for rehabilitation protocols (Raffin and Hummel, 2017; Coscia et al., 2019), or devices such as wheelchairs, spellers, exoskeletons, and prostheses (Rosenfeld and Wong, 2017), in order to assist patients in overcoming motor disabilities following stroke, spinal cord injuries, and other CNS pathologies or peripheral deficits, respectively. However, despite the attention that such devices have attracted over the last few years and the fact that numerous research teams are actively working on improving them, to date BCIs have not delivered on their promises. The reasons for this span multiple axes (Chavarriaga et al., 2016) including issues specific to the brain-signal acquisition techniques used, the underlying assumptions about the extracted signals and the targeted mental states, as well as the specific signal processing and feature extraction methods employed.
Broadly, BCIs can be categorized as invasive or non-invasive based on the techniques used to extract relevant brain signals (Daly and Wolpaw, 2008; Hatsopoulos and Donoghue, 2009). Typically, the former require surgery and enable the recording of single- or multi-unit activity, or local field potentials, with depth electrodes or electrocorticography (Hatsopoulos and Donoghue, 2009). The advantages of these techniques are that (a) they allow for close and precise intra- or supracortical placement of the electrodes and thus high spatial resolution and signal-to-noise ratio (SNR), and (b) they can avoid certain sources of noise while still recording high-frequency signals such as neural spiking or high-frequency gamma activity.
Nonetheless, these advantages are blunted by potential risks. As is the case for surgical implantation of electrodes in patients suffering from pharmacoresistant epilepsy or other diseases treated via deep-brain stimulation protocols, invasive techniques are inherently accompanied by risk of complications such as infections, subdural and intracerebral bleeding, and brain edema (Hariz, 2002; Wellmer et al., 2012). Moreover, invasive methods based on electrode implantation are characterized by instability over large periods of time due to extensive tissue scaring around the implantation site and electrode drift, which often reduce the numbers of recorded units (Hatsopoulos and Donoghue, 2009). In practice, these drawbacks often require the implanted electrodes to be removed after a fairly short amount of time [although see (Hochberg et al., 2012)].
Considering the risks inherent to surgically implanted electrodes, it is evident that at least for certain applications, non-invasive BCIs seem to be a more viable option for widespread adoption in the foreseeable future, a fact reflected in the predominance of EEG in most BCI applications. Despite the lower SNR and the lower frequency and spatial resolution of extracranially recorded brain signals, advanced signal processing and feature extraction methods (Blankertz et al., 2008; Iturrate et al., 2020), often paired with sophisticated machine and deep learning algorithms (Lotte et al., 2018; Mladenović et al., 2019; Roy et al., 2019), or hybrid BCI designs (Müller-Putz et al., 2015; Hong and Khan, 2017) have steadily improved the decoding capabilities of non-invasive BCIs.
However, most efforts have focused on the development of agent-agnostic algorithms, while only a few studies have focused on refining the neural correlates that are thought to be relevant for a given motor task. We propose that future efforts for online extraction of pertinent brain signals and features should instead concentrate on (a) redefining the neurophysiological markers and features to be extracted and, (b) adopting an individualized and neurophysiologically informed paradigm (Chavarriaga et al., 2016; Mladenović et al., 2019) specifically targeting these markers and features.
Taking advantage of recent work that emphasizes the pitfalls of indiscriminately performing time-frequency analyzes of neural signals (Jones, 2016; Cole et al., 2017; Cole and Voytek, 2017; Donoghue et al., 2021), the importance of transient bursts of frequency-specific activity for behavior (Jones, 2016; Lundqvist et al., 2016, 2018; Sherman et al., 2016; Shin et al., 2017; Lofredi et al., 2018; Torrecillos et al., 2018; Little et al., 2019; Anderson et al., 2020; Khawaldeh et al., 2020; Seedat et al., 2020; Yeh et al., 2020), and the ability to precisely localize these bursts (Bonaiuto et al., 2021), this line of research could vastly improve the decoding capabilities of BCIs and serve as a basis for a wide range of applications that leverage motor-related signals. As a final note, we discuss why we believe non-invasive BCIs should primarily target rehabilitation rather than restoration of control and list a few outstanding questions that remain to be answered.
Current Approaches to Non-Invasive, Motor Imagery Based Brain-Computer Interfaces
State-of-the-art non-invasive BCI paradigms vary greatly according to the techniques used to extract signals that are potentially informative for a task. Depending on the goal they aim to attain, several setups can exploit different brain signals sometimes even mixed with other, non-brain signals like eye movements and muscle activity (Choi et al., 2017). The recorded brain signals can be electrical, or hemodynamic, measured using electroencephalography (EEG) possibly magnetoencephalography (MEG)1, or functional near-infrared spectroscopy (fNIRS), respectively.
Contrary to hemodynamic signals (Nicolas-Alonso and Gomez-Gil, 2012), EEG and MEG signals provide direct measures of neuronal activity and are characterized by high temporal resolution. They are therefore privileged candidates for real-time applications. In this section we will briefly discuss these two techniques as well as the common physiological markers of motor-related cortical activity they record, highlighting the steps usually taken to analyze these signals and their respective shortcomings.
Electroencephalography and Magnetoencephalography Overview
Electroencephalography measures the electrical activity due to variations of post-synaptic potentials synchronized across large populations of cortical neurons (Buzsáki et al., 2012). The signal is measured as the difference in electric potentials recorded from electrodes relative to a reference (ground) electrode (Nicolas-Alonso and Gomez-Gil, 2012). These post-synaptic potentials also generate weak magnetic fields which are measured by MEG sensors (Hämäläinen and Hari, 2002; da Silva, 2010; Lecaignard and Mattout, 2015).
The major goal of any BCI application is to ultimately enhance and improve the everyday lives of its users. Hence, EEG is frequently the recording technique of choice for BCIs because EEG setups have the advantage of being portable and inexpensive compared to other signal acquisition technologies, thus making them suitable for a wide range of environments from the laboratory to the medical office, or even at home. On the contrary, current SQUID-based MEG systems are more expensive and inherently constrained to magnetic-shielded laboratory environments because of technical limitations imposed by the small amplitude of the measured fields in comparison to multiple sources of noise.
Electroencephalography electrodes are placed on the scalp surface and, as a result, must contend with two main challenges. Firstly, the recorded signals are significantly attenuated and contaminated by physiological and non-physiological noise as they have to traverse multiple layers such as the meninges, cerebrospinal fluid, skull, and muscle tissue before reaching the scalp (Nicolas-Alonso and Gomez-Gil, 2012). Secondly, each electrode samples the activity, distorted by the skull (Lopes da Silva, 2013), of a relatively large cortical area (Krusienski et al., 2011), therefore leading to poor spatial resolution (Lopes da Silva, 2013). Conversely, MEG is not affected to the same extent by either challenge. Although MEG sensors sample magnetic fields from an equivalent cortical area, these fields are only attenuated with distance and not distorted by the presence of layers of tissue and skull between the neural sources and the MEG sensors. As a consequence, MEG offers a higher spatial resolution than EEG. However, in practice it is limited by the head movements that subjects make while performing a task (Medvedovsky et al., 2007), as well as by co-registration errors with anatomical images (Hillebrand and Barnes, 2011). Finally, due to the dynamic nature of brain signals, both EEG and MEG recordings are characterized by great variability, both between individuals, and within recordings of the same individual over long periods of time or different recording sessions.
Motor Imagery in Electroencephalography and Magnetoencephalography
Brain-Computer Interfaces that aim to leverage motor-related brain activity usually depend on two types of signals. For patients, the signal of interest is occasionally recorded during an attempted movement (Muralidharan et al., 2011). The other common strategy for healthy subjects and patients involves asking subjects to imagine producing movements, known as (kinesthetic) motor imagery (MI) (Pfurtscheller and Lopes da Silva, 1999), on which we will focus hereafter. Whereas a signal recorded while a patient is attempting to perform a movement despite their disability is thought to reflect purely motor functions, MI may also implicate other cognitive processes like mental rotation or conscious access to the motor plan (Jeannerod, 1995; Hanakawa et al., 2003, 2008; Raffin et al., 2012; Meng et al., 2018).
Traditionally, both movement execution and MI have been linked to time-locked changes in induced power within various frequency bands recorded from sensorimotor cortex (Pfurtscheller and Lopes da Silva, 1999; Friston et al., 2006; Ramadan and Vasilakos, 2017). In the beta (∼13–30 Hz) and mu (∼8–12 Hz) frequency bands, these signals are marked by a gradual reduction in power prior to a real or imagined movement, named event-related desynchronization (ERD), followed by a post-movement increase called event-related synchronization (ERS) in the beta band (Pfurtscheller et al., 1996, 2006; Pfurtscheller and Neuper, 1997; Neuper et al., 2006; Tam et al., 2019). In the mu and beta bands, pre-movement ERD has long been considered to reflect neural processes related to movement preparation, initiation and realization, while post-movement ERS was related to the re-establishment of inhibition after the execution of a motor plan (Pfurtscheller et al., 1997; Pfurtscheller and Lopes da Silva, 1999). An ERS is also typically observed in the gamma band (30–120 Hz) around the time of movement onset (Ball et al., 2008; Darvas et al., 2010; Miller et al., 2010), and is thought to reflect movement execution or monitoring (Brovelli et al., 2005; Cheyne and Ferrari, 2013; Gaetz et al., 2013).
Common Techniques in Motor Imagery Based Brain-Computer Interfaces
Most approaches for MI signal analysis utilize a similar pipeline (Wolpaw, 2002) that involves preprocessing and time-frequency decomposition to extract movement-related ERD and ERS signals, and classification of these signals to predict some aspect of an imagined movement. A great repertoire of signal processing and feature extraction methods have been developed or adapted to particularly address the low SNR and source localization problems inherent in EEG and MEG (Lotte et al., 2018; Iturrate et al., 2020). Signal processing methods aim to improve the quality of the recorded signals by means of artifact rejection, frequency filtering, or spatial filtering. Such methods increase SNR by rejecting non-brain signals, filtering out irrelevant aspects of the signals, and reducing the contribution of non-pertinent areas in the feature extraction step. Dimensionality reduction techniques such as principal component analysis (Zarei et al., 2017) or independent component analysis (Kachenoura et al., 2008) are typically used to select artefactual sources to be removed, or relevant components to be further analyzed (Medeiros de Freitas et al., 2020). Feature extraction refers to the process of extracting the metrics of a signal that is most informative of the imagined movement. Standard procedures include channel selection, and time or spectral feature estimation. For analyzes of frequency-specific changes in power, time-frequency decomposition techniques such as the fast Fourier transform (Herman et al., 2008; Brodu et al., 2011), Hilbert–Huang transform (Wang et al., 2008), or Morlet wavelets (Herman et al., 2008; Brodu et al., 2011) are commonly used.
As the goal of MI based BCI applications is usually to decode aspects of the intended movement from recorded neural activity, the extracted features are used as input to some sort of classifier. Classifiers are trained offline on the sampled activity, yielding a model that maps the underlying features to a set of outputs (e.g., left vs. right imagined movement), and are then employed online to map the recorded activity to an output in real time (Iturrate et al., 2020). Common machine learning algorithms used in BCI applications for decoding a subject’s imagined movement include neural networks (Pfurtscheller and Neuper, 2001; Hazrati and Erfanian, 2010), linear discriminant analysis (Pfurtscheller and Neuper, 2001; Vidaurre et al., 2011; Llera et al., 2014), and support vector machines (Song et al., 2013), while during the last few years the field has experienced a steady shift of interest toward more elaborate deep learning architectures (Roy et al., 2019).
This general BCI pipeline has been widely adopted, but important limitations still exist. Most MI based applications are limited to binary classifications (e.g., left vs. right), or classifications among a few classes at best, and achieve modest results even when continuous paradigms are used, as classification accuracies steeply decrease with an increasing number of output classes (Hong and Khan, 2017). Notably, only a few studies have involved patients, and therefore the real value of BCIs for patients is still unproven. Additionally, despite the sophisticated methods employed, still about a third of subjects have great difficulty or are totally unable to control BCIs, a phenomenon known as BCI illiteracy (Vidaurre and Blankertz, 2010). We argue that this focus on advanced signal-processing and feature extraction techniques for MI based BCIs has effectively rendered ERD and ERS the only signals of interest, creating a gap between the BCI community and recent advances in neurophysiology.
Proposed Alternative
In light of these considerations we believe that significant progress toward the development of high-fidelity non-invasive BCIs, suitable for online motor decoding, will be achieved only if we attempt to bridge the gap between basic neuroscience and applied neuroengineering. To this end we propose a reassessment of the neurophysiological features of interest for the decoding of motor related activity, and a novel methodology that exploits the advantages of both MEG and EEG in order to take advantage of subtle, individualized physiological markers of motor processing.
The Quest for Refined Neurophysiological Markers
While standard non-invasive BCI features such as changes in frequency band power are somewhat informative of movement, these approaches discard potentially valuable information in the temporal domain. Time-frequency analyzes rarely take into account the temporal variability and waveform shape of the signals of interest (Donoghue et al., 2021), potentially overlooking the fact that neural activity can occur as bursts rather than oscillations (Jones, 2016; Lundqvist et al., 2016, 2018; Sherman et al., 2016; Shin et al., 2017; Lofredi et al., 2018; Torrecillos et al., 2018; Little et al., 2019; Anderson et al., 2020; Khawaldeh et al., 2020; Seedat et al., 2020; Yeh et al., 2020) and that oscillations can have non-sinusoidal waveform shapes (Cole et al., 2017; Cole and Voytek, 2017). Both of these potentially rich sources of information are lost in typical time frequency analyzes.
In the case of beta activity, it has recently been demonstrated that the majority of sensorimotor activity within this band occurs as discrete transient bursts (Jones, 2016; Lundqvist et al., 2016, 2018; Sherman et al., 2016; Shin et al., 2017; Little et al., 2019; Figure 1A), and that the patterns of pre-movement beta ERD and post-movement beta rebound are a result of averaging these bursts over multiple trials (Little et al., 2019; Wessel, 2020; Figure 1B). Indeed, it has been shown that the timing of beta bursts in motor cortex are, before movement, predictive of response times, and after movement, informative of behavioral errors, more so than changes in beta amplitude (Little et al., 2019). In the right inferior frontal cortex, beta burst timing is related to electromyography correlates of movement cancelation (Hannah et al., 2020), and may coordinate bursts in sensorimotor cortex following successful movement cancelation (Wessel, 2020). Beta burst activity can also be modulated through neurofeedback training (He et al., 2020), making beta burst timing and spatial distribution a potential rich source of information for MI decoding.
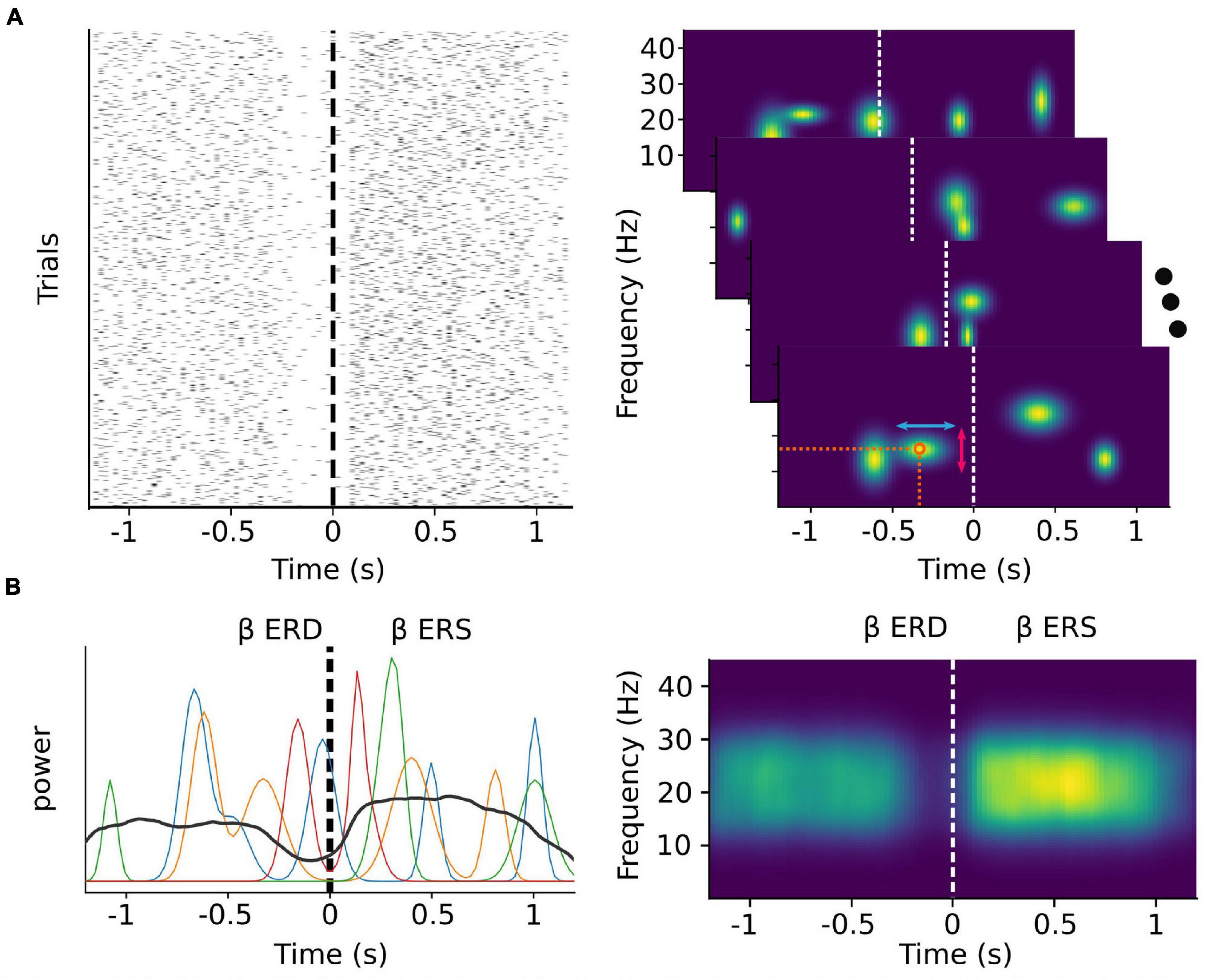
Figure 1. Trial-averaged discrete bursts can appear to be sustained oscillations. Simulated beta burst activity from multivariate Gaussian distributions with a time varying probability and random peak frequency, frequency span, and time duration. (A) Left: The timing of simulated bursts in each trial (N = 1,000). Right: Time-frequency decomposition analysis of each single trial level (shown for four random ones) allows for the extraction of features such as the exact timing and peak frequency (orange circle), the time duration (red vertical arrows) and the frequency span (light blue horizontal arrows) of each burst. (B) Beta band power of the same four random trials from the right panel of (A) (colored lines) depicted along with the average beta band power over all trials (black line). During each trial, beta power appears as transient peaks at varying time points. The classically described ERD and ERS phenomena emerge as a consequence of averaging over multiple trials.
In contrast to beta, activity in the mu frequency band is oscillatory even in single trials (Chen et al., 2021). This activity is typically analyzed using time-frequency decomposition techniques, which assume that the underlying signal is sinusoidal. However, there is now growing consensus that oscillatory neural activity is often non-sinusoidal (Cole and Voytek, 2017, 2019; Donoghue et al., 2021; Fabus et al., 2021), and that the raw waveform shape can be informative of movement (Quinn et al., 2021; Figure 2). Future efforts could take advantage of this possibility by using recently developed non-parametric cycle-by-cycle analyzes (Cole and Voytek, 2019).
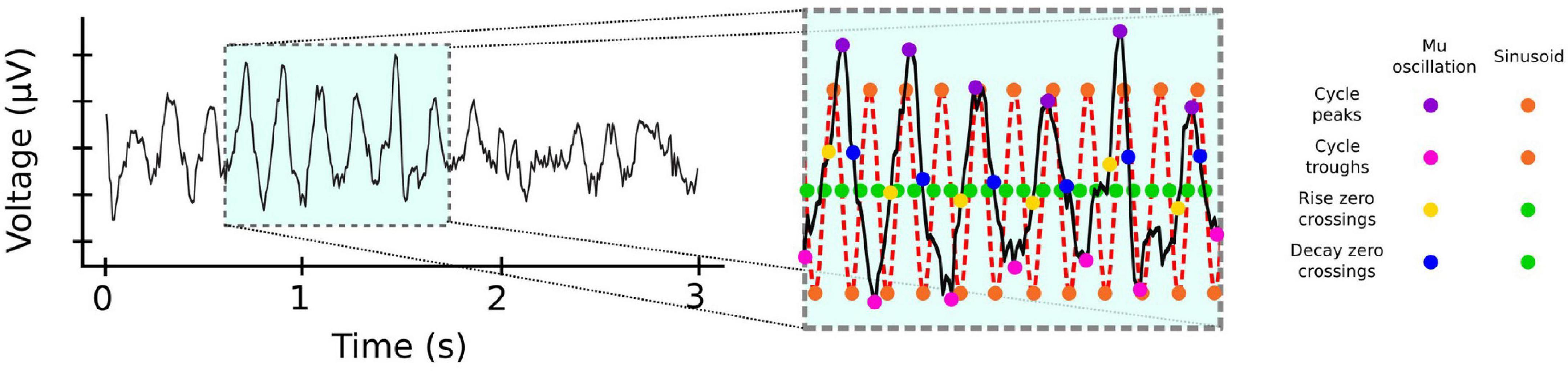
Figure 2. Frequency specific activity can be non-sinusoidal. Mu activity from EEG electrode C4. Inset: Cycle-by-cycle analysis (Cole and Voytek, 2019) of the activity reveals that mu occurs in bursts with significant variability for measures such as peaks (magenta dots) and troughs (pink dots), or rise (yellow dots) and decay (blue dots) duration between cycles, unlike the corresponding measures of a pure 10 Hz sinusoid (orange and green dots). EEG data from BCI Competition IV (Leeb et al., 2007) https://www.bbci.de/competition/iv.
Subject-Specific, Forward Models of Dynamic Activity
The major challenges for non-invasive BCIs are to deal with highly variable and noisy single trial activity, and to overcome the low SNR and limited spatial resolution in order to improve online feature extraction and decoding. We anticipate that these challenges will be even more acute when targeting short-lasting, potentially non-sinusoidal bursts of frequency specific activity. To overcome these challenges, we propose that MEG could be used to complement EEG. Hybrid designs (Allison et al., 2010; Pfurtscheller, 2010) that combine the two techniques yield superior results compared to either technique used alone in certain applications (Corsi et al., 2019; Lecaignard et al., 2021), however such designs are limited to laboratory settings. To deploy a system under more naturalistic settings, one alternative is to use MEG to develop personalized forward models that can subsequently be used to improve the decoding efficacy of online EEG-based BCI applications.
The spatial precision of MEG is traditionally limited by within-session subject movement and between-session co-registration error. However, recently developed high-precision MEG (hpMEG) techniques based on the use of 3D printed individualized head-casts (Meyer et al., 2017), have allowed for fine-grained analyzes of movement-related signals at the level of cortical laminae (Troebinger et al., 2014a,b; Bonaiuto et al., 2018a,b, 2021). Customized forward models based on offline hpMEG analysis could be used to identify the neural features most informative of imaged movements and isolate these features to subsequently optimize EEG-based real-time decoding. In the near future, optically-pumped magnetometers (OPMs) may be able to replace hybrid M/EEG (Boto et al., 2019, 2021; Iivanainen et al., 2019; Roberts et al., 2019; Borna et al., 2020; Hill et al., 2020; Paek et al., 2020; Wittevrongel et al., 2021), as they promise to be more portable and cheaper while also improving SNR.
A Paradigm Shift Toward Motor Rehabilitation
Lately the BCI community has been drifting away from the restoration of motor control to target motor rehabilitation instead (McFarland et al., 2017; Raffin and Hummel, 2017; Coscia et al., 2019; MacEira-Elvira et al., 2019; Lennon et al., 2020; Micera et al., 2020). Motor rehabilitation is a challenging yet more attainable goal compared to motor restoration, simply because of the amount of complexity that the control of multiple degrees of freedom devices introduces both for the BCI designers and users. Moreover, BCI-assisted rehabilitation protocols are relevant for a wider group of patients beyond those that need to overcome a peripheral handicap; from stroke patients with motor impairments to patients suffering from Parkinson’s disease. The features extracted through bursts analysis could revolutionize these protocols.
As an example, the coupling of this design with mirror therapy based on virtual or augmented reality could be important for a number of reasons. Visual feedback is known to reduce movement errors due to impaired proprioception (Ghez et al., 1995), while it has, also, long been shown in experiments with non-human primates that the role of vision is crucial for the formation of the internal representation of upper limb position, more so than proprioceptive feedback (Graziano, 1999). Additionally, it is established that erroneous visual feedback ultimately results in inaccurate movements being regarded as accurate representations of a subject’s movement intentions (Preston and Newport, 2014). Therefore, hpMEG-optimized BCI training accompanied by digital mirror or camera therapy may soon provide clinicians and, importantly, patients with novel therapeutic solutions.
Conclusion
Despite the significant effort toward the development of sophisticated algorithms and design paradigms, state-of-the-art EEG-based BCI applications exploiting MI face many limitations. To overcome these limitations, we propose that more subtle neurophysiological markers such as burst timing and waveform shape be explored, and techniques with high temporal and spatial resolution such as hpMEG and OPMs be adopted.
Still, open, outstanding questions related to the proposed paradigm exist. How is burst activity in the motor cortex modulated during imagined rather than performed movements, and how do bursts recorded from different areas of the broader motor network relate to each other? What is the relationship between the continuous nature of movements and burst activity, and how can we exploit it to serve as a reliable marker that will allow us to go beyond discrete classifications? Does the waveform of oscillations change with respect to the recorded area and/or imagined movement, and can it thus give us access to more, complementary features? Importantly, which features of the imagined movements are best explained by this analysis?
We believe that embracing the proposed approach will, in time, provide answers to these questions and significantly improve non-invasive BCIs by extracting richer features of interest from signals recorded with sensitive techniques designed to particularly tackle current limitations.
Data Availability Statement
The original contributions presented in the study are included in the article/supplementary material, further inquiries can be directed to the corresponding author/s.
Author Contributions
JB and JM contributed to the conception of the manuscript. SP drafted the manuscript. All authors contributed to manuscript revision, read, and approved the submitted version.
Funding
SP, JB, and JM are supported by the French National Research Agency (ANR) project HiFi (2020–2024). JB is supported by the European Research Council (ERC) under the European Union’s Horizon 2020 Research and Innovation Programe (ERC consolidator grant 864550 to JB).
Conflict of Interest
The authors declare that the research was conducted in the absence of any commercial or financial relationships that could be construed as a potential conflict of interest.
Publisher’s Note
All claims expressed in this article are solely those of the authors and do not necessarily represent those of their affiliated organizations, or those of the publisher, the editors and the reviewers. Any product that may be evaluated in this article, or claim that may be made by its manufacturer, is not guaranteed or endorsed by the publisher.
Acknowledgments
This work was performed within the framework of the LABEX CORTEX (ANR-11-LABX-0042) of Université de Lyon, within the program “Investissements d’Avenir” (decision n° 2019-ANR-LABX-02) operated by the French National Research Agency (ANR).
Footnotes
- ^ The emergence of optically-pumped magnetometers (OPMs) could, unlike currently available systems, render MEG portable and, thus, a very attractive platform for BCI applications in the future.
References
Allison, B. Z., Brunner, C., Kaiser, V., Müller-Putz, G. R., Neuper, C., and Pfurtscheller, G. (2010). Toward a hybrid brain-computer interface based on imagined movement and visual attention. J. Neural. Eng. 7:26007. doi: 10.1088/1741-2560/7/2/026007
Anderson, R. W., Kehnemouyi, Y. M., Neuville, R. S., Wilkins, K. B., Anidi, C. M., Petrucci, M. N., et al. (2020). A novel method for calculating beta band burst durations in Parkinson’s disease using a physiological baseline. J. Neurosci. Methods 343:108811. doi: 10.1016/j.jneumeth.2020.108811
Ball, T., Demandt, E., Mutschler, I., Neitzel, E., Mehring, C., Vogt, K., et al. (2008). Movement related activity in the high gamma range of the human EEG. Neuroimage 41, 302–310. doi: 10.1016/j.neuroimage.2008.02.032
Blankertz, B., Tomioka, R., Lemn, S., Kawanabe, M., and Muller, K.-R. (2008). Optimizing spatial filters for robust EEG single-trial analysis. IEEE Signal Process. Mag. 25, 41–56.
Bonaiuto, J. J., Little, S., Neymotin, S. A., Jones, S. R., Barnes, G. R., and Bestmann, S. (2021). Laminar dynamics of beta bursts in human motor cortex. Neuroimage 15:118479. doi: 10.1016/j.neuroimage.2021.118479
Bonaiuto, J. J., Meyer, S. S., Little, S., Rossiter, H., Callaghan, M. F., Dick, F., et al. (2018a). Laminar-specific cortical dynamics in human visual and sensorimotor cortices. Elife 7:e33977. doi: 10.7554/eLife.33977
Bonaiuto, J. J., Rossiter, H. E., Meyer, S. S., Adams, N., Little, S., Callaghan, M. F., et al. (2018b). Non-invasive laminar inference with MEG: comparison of methods and source inversion algorithms. Neuroimage 167, 372–383. doi: 10.1016/j.neuroimage.2017.11.068
Borna, A., Carter, T. R., Colombo, A. P., Jau, Y. Y., McKay, J., Weisend, M., et al. (2020). Non-invasive functional-brain-imaging with an OPM-based magnetoencephalography system. PLoS One 15:e0227684. doi: 10.1371/journal.pone.0227684
Boto, E., Hill, R. M., Rea, M., Holmes, N., Seedat, Z. A., Leggett, J., et al. (2021). Measuring functional connectivity with wearable MEG. Neuroimage 230:117815. doi: 10.1016/j.neuroimage.2021.117815
Boto, E., Seedat, Z. A., Holmes, N., Leggett, J., Hill, R. M., Roberts, G., et al. (2019). Wearable neuroimaging: combining and contrasting magnetoencephalography and electroencephalography. Neuroimage 201:116099. doi: 10.1016/j.neuroimage.2019.116099
Brodu, N., Lotte, F., and Lécuyer, A. (2011). “Comparative study of band-power extraction techniques for Motor Imagery classification,” in Proceedings of the CCMB 2011 2011 IEEE Symp. Comput. Intell. Cogn. Algorithms, Mind, Brain (Piscataway, NJ: IEEE), 95–100. doi: 10.1109/CCMB.2011.5952105
Brovelli, A., Lachaux, J. P., Kahane, P., and Boussaoud, D. (2005). High gamma frequency oscillatory activity dissociates attention from intention in the human premotor cortex. Neuroimage 28, 154–164. doi: 10.1016/j.neuroimage.2005.05.045
Buzsáki, G., Anastassiou, C. A., and Koch, C. (2012). The origin of extracellular fields and currents-EEG, ECoG, LFP and spikes. Nat. Rev. Neurosci. 13, 407–420. doi: 10.1038/nrn3241
Chavarriaga, R., Fried-Oken, M., Kleih, S., Lotte, F., and Scherer, R. (2016). Heading for new shores! Overcoming pitfalls in BCI design. Brain Comput. Interfaces 4, 60–73. doi: 10.1080/2326263X.2016.1263916
Chen, Y. Y., Lambert, K. J. M., Madan, C. R., and Singhal, A. (2021). Mu oscillations and motor imagery performance: a reflection of intra-individual success, not inter-individual ability. Hum. Mov. Sci. 78:102819. doi: 10.1016/j.humov.2021.102819
Cheyne, D., and Ferrari, P. (2013). MEG studies of motor cortex gamma oscillations: evidence for a gamma “fingerprint” in the brain? Front. Hum. Neurosci. 7:575. doi: 10.3389/fnhum.2013.00575
Choi, I., Rhiu, I., Lee, Y., Yun, M. H., and Nam, C. S. (2017). A systematic review of hybrid brain-computer interfaces: taxonomy and usability perspectives. PLoS One 12:e0176674. doi: 10.1371/journal.pone.0176674
Cole, S. R., and Voytek, B. (2017). Brain oscillations and the importance of waveform shape. Trends Cogn. Sci. 21, 137–149. doi: 10.1016/j.tics.2016.12.008
Cole, S., and Voytek, B. (2019). Cycle-by-cycle analysis of neural oscillations. J. Neurophysiol. 122, 849–861. doi: 10.1152/JN.00273.2019
Cole, X. S. R., Meij, R., Van Der Peterson, E. J., Hemptinne, C., De, Starr, X. A., et al. (2017). Nonsinusoidal beta oscillations reflect cortical pathophysiology in Parkinson’s disease. J. Neurosci. 37, 4830–4840. doi: 10.1523/JNEUROSCI.2208-16.2017
Corsi, M. C., Chavez, M., Schwartz, D., Hugueville, L., Khambhati, A. N., Bassett, D. S., et al. (2019). Integrating EEG and MEG signals to improve motor imagery classification in brain-computer interface. Int. J. Neural Syst. 29:1850014. doi: 10.1142/S0129065718500144
Coscia, M., Wessel, M. J., Chaudary, U., Millán, J., del, R., Micera, S., et al. (2019). Neurotechnology-aided interventions for upper limb motor rehabilitation in severe chronic stroke. Brain 142, 2182–2197. doi: 10.1093/brain/awz181
da Silva, F. H. L. (2010). “Electrophysiological basis of MEG signals,” in MEG: An Introduction To Methods, eds P. C. Hansen, M. L. Kringelbach, and R. Salmelin (Oxford: Oxford University Press), 1–23. doi: 10.1093/acprof:oso/9780195307238.003.0001
Daly, J. J., and Wolpaw, J. R. (2008). Brain-computer interfaces in neurological rehabilitation. Lancet Neurol. 7, 1032–1043. doi: 10.1016/S1474-4422(08)70223-0
Darvas, F., Scherer, R., Ojemann, J. G., Rao, R. P., Miller, K. J., and Sorensen, L. B. (2010). High gamma mapping using EEG. Neuroimage 49, 930–938. doi: 10.1016/j.neuroimage.2009.08.041
Donoghue, T., Schaworonkow, N., and Voytek, B. (2021). Methodological considerations for studying neural oscillations. Eur. J. Neurosci. 2021:16. doi: 10.1111/ejn.15361
Fabus, M. S., Quinn, A. J., Warnaby, C. E., and Woolrich, M. W. (2021). Automatic decomposition of electrophysiological data into distinct nonsinusoidal oscillatory modes. J. Neurophysiol. 126, 1670–1684. doi: 10.1152/jn.00315.2021
Friston, K., Henson, R., Phillips, C., and Mattout, J. (2006). Bayesian estimation of evoked and induced responses. Hum. Brain Mapp. 27, 722–735. doi: 10.1002/hbm.20214
Gaetz, W., Liu, C., Zhu, H., Bloy, L., and Roberts, T. P. L. (2013). Evidence for a motor gamma-band network governing response interference. Neuroimage 74, 245–253. doi: 10.1016/j.neuroimage.2013.02.013
Ghez, C., Gordon, J., and Ghilardi, M. F. (1995). Impairments of reaching movements in patients without proprioception. II. Effects of visual information on accuracy. J. Neurophysiol. 73, 361–372. doi: 10.1152/jn.1995.73.1.361
Graziano, M. S. A. (1999). Where is my arm? The relative role of vision and proprioception in the neuronal representation of limb position. Proc. Natl. Acad. Sci. U.S.A. 96, 10418–10421. doi: 10.1073/pnas.96.18.10418
Hämäläinen, M., and Hari, R. (eds). (2002). “Magnetoencephalographic (MEG) characterization of dynamic brain activation,” in Brain Mapping: The Methods (Amsterdam: Elsevier), 227–254.
Hanakawa, T., Dimyan, M. A., and Hallett, M. (2008). Motor planning, imagery, and execution in the distributed motor network: a time-course study with functional MRI. Cereb. Cortex 18, 2775–2788. doi: 10.1093/cercor/bhn036
Hanakawa, T., Immisch, I., Toma, K., Dimyan, M. A., Van Gelderen, P., and Hallett, M. (2003). Functional properties of brain areas associated with motor execution and imagery. J. Neurophysiol. 89, 989–1002. doi: 10.1152/jn.00132.2002
Hannah, R., Muralidharan, V., Sundby, K. K., and Aron, A. R. (2020). Temporally-precise disruption of prefrontal cortex informed by the timing of beta bursts impairs human action-stopping. Neuroimage 222:117222. doi: 10.1016/j.neuroimage.2020.117222
Hariz, M. I. (2002). Complications of deep brain stimulation surgery. Mov. Disord. 17, 162–166. doi: 10.1002/mds.10159
Hatsopoulos, N. G., and Donoghue, J. P. (2009). The Science of Neural Interface Systems. Annu. Rev. Neurosci. 32, 249–266. doi: 10.1146/annurev.neuro.051508.135241
Hazrati, M. K., and Erfanian, A. (2010). An online EEG-based brain-computer interface for controlling hand grasp using an adaptive probabilistic neural network. Med. Eng. Phys. 32, 730–739. doi: 10.1016/j.medengphy.2010.04.016
He, S., Everest-Phillips, C., Clouter, A., Brown, P., and Tan, H. (2020). Neurofeedback-linked suppression of cortical B bursts speeds up movement initiation in healthy motor control: a double-blind sham-controlled study. J. Neurosci. 40, 4021–4032. doi: 10.1523/JNEUROSCI.0208-20.2020
Herman, P., Prasad, G., McGinnity, T. M., and Coyle, D. (2008). Comparative analysis of spectral approaches to feature extraction for EEG-based motor imagery classification. IEEE Trans. Neural Syst. Rehabil. Eng. 16, 317–326. doi: 10.1109/TNSRE.2008.926694
Hill, R. M., Boto, E., Rea, M., Holmes, N., Leggett, J., Coles, L. A., et al. (2020). Multi-channel whole-head OPM-MEG: helmet design and a comparison with a conventional system. Neuroimage 219:116995. doi: 10.1016/j.neuroimage.2020.116995
Hillebrand, A., and Barnes, G. R. (2011). Practical constraints on estimation of source extent with MEG beamformers. Neuroimage 54, 2732–2740. doi: 10.1016/j.neuroimage.2010.10.036
Hochberg, L. R., Bacher, D., Jarosiewicz, B., Masse, N. Y., Simeral, J. D., Vogel, J., et al. (2012). Reach and grasp by people with tetraplegia using a neurally controlled robotic arm. Nature 485, 372–375. doi: 10.1038/nature11076
Hong, K. S., and Khan, M. J. (2017). Hybrid brain-computer interface techniques for improved classification accuracy and increased number of commands: a review. Front. Neurorobot. 11:35. doi: 10.3389/fnbot.2017.00035
Iivanainen, J., Zetter, R., Grön, M., Hakkarainen, K., and Parkkonen, L. (2019). On-scalp MEG system utilizing an actively shielded array of optically-pumped magnetometers. Neuroimage 194, 244–258. doi: 10.1016/j.neuroimage.2019.03.022
Iturrate, I., Chavarriaga, R., Millán, J., and del, R. (2020). General principles of machine learning for brain-computer interfacing. Handb. Clin. Neurol. 168, 311–328. doi: 10.1016/B978-0-444-63934-9.00023-8
Jeannerod, M. (1995). Mental imagery in the motor context. Neuropsychologia 33, 1419–1432. doi: 10.1016/0028-3932(95)00073-C
Jones, S. R. (2016). When brain rhythms aren’t ‘rhythmic’: implication for their mechanisms and meaning. Curr. Opin. Neurobiol. 40, 72–80. doi: 10.1016/j.conb.2016.06.010
Kachenoura, A., Albera, L., Senhadji, L., and Comon, P. (2008). ICA: a potential tool for BCI systems. IEEE Signal Process. Mag. 25, 57–68. doi: 10.1109/MSP.2008.4408442
Khawaldeh, S., Tinkhauser, G., Shah, S. A., Peterman, K., Debove, I., Khoa Nguyen, T. A., et al. (2020). Subthalamic nucleus activity dynamics and limb movement prediction in Parkinson’s disease. Brain 143, 582–586. doi: 10.1093/brain/awz417
Krusienski, D. J., Grosse-Wentrup, M., Galan, F., Coyle, D., Miller, K. J., Forney, E., et al. (2011). Criticalm issues in state-of-the-art brian-computer interface signal processing. J. Neural Eng. 8:025002. doi: 10.1088/1741-2560/8/2/025002
Lecaignard, F., and Mattout, J. (2015). Forward models for EEG/MEG. Brain Mapp. 1, 549–555. doi: 10.1016/B978-0-12-397025-1.00330-4
Lecaignard, F., Bertrand, O., Caclin, A., and Mattout, J. (2021). Empirical bayes evaluation of fused EEG-MEG source reconstruction: application to auditory mismatch evoked responses. Neuroimage 226:117468. doi: 10.1016/j.neuroimage.2020.117468
Leeb, R., Lee, F., Keinrath, C., Scherer, R., Bischof, H., and Pfurtscheller, G. (2007). Brain-computer communication: motivation, aim, and impact of exploring a virtual apartment. IEEE Trans. neural Syst. Rehabil. Eng. 15, 473–482. doi: 10.1109/TNSRE.2007.906956
Lennon, O., Tonellato, M., Felice, A., Del Di Marco, R., Fingleton, C., Korik, A., et al. (2020). A systematic review establishing the current state-of-the-art, the limitations, and the desired checklist in studies of direct neural interfacing with robotic gait devices in stroke rehabilitation. Front. Neurosci. 14:578. doi: 10.3389/fnins.2020.00578
Little, S., Bonaiuto, J., Barnes, G., and Bestmann, S. (2019). Human motor cortical beta bursts relate to movement planning and response errors. PLoS Biol. 17:e3000479. doi: 10.1371/journal.pbio.3000479
Llera, A., Gomez, V., and Kappen, H. J. (2014). Adaptive multiclass classification for brain computer interfaces. Neural. Comput. 26, 1108–1127. doi: 10.1162/NECO
Lofredi, R., Neumann, W. J., Bock, A., Horn, A., Huebl, J., Siegert, S., et al. (2018). Dopamine-dependent scaling of subthalamic gamma bursts with movement velocity in patients with Parkinson’s disease. Elife 7:e31895. doi: 10.7554/eLife.31895
Lopes da Silva, F. (2013). EEG and MEG: relevance to neuroscience. Neuron 80, 1112–1128. doi: 10.1016/j.neuron.2013.10.017
Lotte, F., Bougrain, L., Cichocki, A., Clerc, M., Congedo, M., Rakotomamonjy, A., et al. (2018). A review of classification algorithms for EEG-based brain-computer interfaces: a 10 year update. J. Neural. Eng. 15:031005. doi: 10.1088/1741-2552/aab2f2
Lundqvist, M., Herman, P., Warden, M. R., Brincat, S. L., and Miller, E. K. (2018). Gamma and beta bursts during working memory readout suggest roles in its volitional control. Nat. Commun. 9:394. doi: 10.1038/s41467-017-02791-8
Lundqvist, M., Rose, J., Herman, P., Brincat, S., Buschman, T., and Miller, E. (2016). Gamma and beta bursts underlie working memory. Neuron 90, 152–164. doi: 10.1016/j.neuron.2016.02.028
MacEira-Elvira, P., Popa, T., Schmid, A. C., and Hummel, F. C. (2019). Wearable technology in stroke rehabilitation: towards improved diagnosis and treatment of upper-limb motor impairment. J. Neuroeng. Rehabil. 16:142. doi: 10.1186/s12984-019-0612-y
McFarland, D. J., Daly, J., Boulay, C., and Parvaz, M. A. (2017). Therapeutic applications of BCI technologies. Brain Comput. Interfaces 4, 37–52. doi: 10.1080/2326263X.2017.1307625
Medeiros de Freitas, A., Sanchez, G., Lecaignard, F., Maby, E., Barbosa Soares, A., and Mattout, J. (2020). EEG artifact correction strategies for online trial-by-trial analysis. J. Neural Eng. 17:016035. doi: 10.1088/1741-2552/ab581d
Medvedovsky, M., Taulu, S., Bikmullina, R., and Paetau, R. (2007). Artifact and head movement compensation in MEG. Neurol. Neurophysiol. Neurosci. 2007, 1–10.
Meng, H. J., Pi, Y. L., Liu, K., Cao, N., Wang, Y. Q., Wu, Y., et al. (2018). Differences between motor execution and motor imagery of grasping movements in the motor cortical excitatory circuit. PeerJ 6:e5588. doi: 10.7717/peerj.5588
Meyer, S. S., Bonaiuto, J., Lim, M., Rossiter, H., Waters, S., Bradbury, D., et al. (2017). Flexible head-casts for high spatial precision MEG. J. Neurosci. Methods 276, 38–45. doi: 10.1016/j.jneumeth.2016.11.009
Micera, S., Caleo, M., Chisari, C., Hummel, F. C., and Pedrocchi, A. (2020). Advanced neurotechnologies for the restoration of motor function. Neuron 105, 604–620. doi: 10.1016/j.neuron.2020.01.039
Miller, K. J., Schalk, G., Fetz, E. E., Den Nijs, M., Ojemann, J. G., and Rao, R. P. N. (2010). Cortical activity during motor execution, motor imagery, and imagery-based online feedback. Proc. Natl. Acad. Sci. U.S.A. 107, 4430–4435. doi: 10.1073/pnas.0913697107
Mladenović, J., Mattout, J., and Lotte, F. (2019). “A generic framework for adaptive EEG-Based BCI training and operation,” in Brain Computer Interfaces Handbook, eds C. S. Nam, A. Nijholt, and F. Lotte (Boca Raton, FL: CRC Press), 595–612. doi: 10.1201/9781351231954-31
Müller-Putz, G., Leeb, R., Tangermann, M., Höhne, J., Kübler, A., Cincotti, F., et al. (2015). “Towards noninvasive hybrid brain-computer interfaces: framework, practice, clinical application, and beyond,” in Proceedings of the IEEE (Piscataway, NJ: IEEE), 926–943. doi: 10.1109/JPROC.2015.2411333
Muralidharan, A., Chae, J., and Taylor, D. M. (2011). Extracting attempted hand movements from eegs in people with complete hand paralysis following stroke. Front. Neurosci. 5:39. doi: 10.3389/fnins.2011.00039
Neuper, C., Wörtz, M., and Pfurtscheller, G. (2006). Chapter 14 ERD/ERS patterns reflecting sensorimotor activation and deactivation. Prog. Brain Res. 159, 211–222. doi: 10.1016/S0079-6123(06)59014-4
Nicolas-Alonso, L. F., and Gomez-Gil, J. (2012). Brain computer interfaces, a review. Sensors 12, 1211–1279. doi: 10.3390/s120201211
Paek, A. Y., Kilicarslan, A., Korenko, B., Gerginov, V., Knappe, S., and Contreras-Vidal, J. L. (2020). “Towards a portable magnetoencephalography based brain computer interface with optically-pumped magnetometers,” in Proceedings of the Annu. Int. Conf. IEEE Eng. Med. Biol. Soc. EMBS 2020-July (Piscataway, NJ: IEEE), 3420–3423. doi: 10.1109/EMBC44109.2020.9176159
Pfurtscheller, G., and Lopes da Silva, F. H. (1999). Event-relared EEG/MEG synchronization and desynchronization: basic principles. Clin. Neurophysiol. 110, 1842–1857. doi: 10.1016/S1388-2457(99)00141-8
Pfurtscheller, G., and Neuper, C. (1997). Motor imagery activates primary sensorimotor area in humans. Neurosci. Lett. 239, 65–68. doi: 10.1016/S0304-3940(97)00889-6
Pfurtscheller, G., and Neuper, C. (2001). Motor imagery direct communication. Proc. IEEE 89, 1123–1134. doi: 10.1109/5.939829
Pfurtscheller, G., Brunner, C., Schlögl, A., and Lopes da Silva, F. H. (2006). Mu rhythm (de)synchronization and EEG single-trial classification of different motor imagery tasks. Neuroimage 31, 153–159. doi: 10.1016/j.neuroimage.2005.12.003
Pfurtscheller, G., Neuper, C., Flotzinger, D., and Pregenzer, M. (1997). EEG-based discrimination between imagination of right and left hand movement. Electroencephalogr. Clin. Neurophysiol. 103, 642–651. doi: 10.1016/S0013-4694(97)00080-1
Pfurtscheller, G., Stancák, A., and Neuper, C. (1996). Post-movement beta synchronization. A correlate of an idling motor area? Electroencephalogr. Clin. Neurophysiol. 98, 281–293. doi: 10.1016/0013-4694(95)00258-8
Preston, C., and Newport, R. (2014). Noisy visual feedback training impairs detection of self-generated movement error: implications for anosognosia for hemiplegia. Front. Hum. Neurosci. 8:456. doi: 10.3389/fnhum.2014.00456
Quinn, A. J., Lopes-Dos-Santos, V., Huang, N., Liang, W. K., Juan, C. H., Yeh, J. R., et al. (2021). Within-cycle instantaneous frequency profiles report oscillatory waveform Dynamics. J. Neurophysiol. 126, 1190–1208. doi: 10.1152/jn.00201.2021
Raffin, E., and Hummel, F. C. (2017). Restoring motor functions after stroke: multiple approaches and opportunities. Neuroscientist 24, 400–416. doi: 10.1177/1073858417737486
Raffin, E., Mattout, J., Reilly, K. T., and Giraux, P. (2012). Disentangling motor execution from motor imagery with the phantom limb. Brain 135, 582–595. doi: 10.1093/brain/awr337
Ramadan, R. A., and Vasilakos, A. V. (2017). Brain computer interface: control signals review. Neurocomputing 223, 26–44. doi: 10.1016/j.neucom.2016.10.024
Roberts, G., Holmes, N., Alexander, N., Boto, E., Leggett, J., Hill, R. M., et al. (2019). Towards OPM-MEG in a virtual reality environment. Neuroimage 199, 408–417. doi: 10.1016/j.neuroimage.2019.06.010
Rosenfeld, J. V., and Wong, Y. T. (2017). Neurobionics and the brainecomputer interface: current applications and future horizons. Med. J. Aust. 206, 363–368. doi: 10.5694/mja16.01011
Roy, Y., Banville, H., Albuquerque, I., Gramfort, A., Falk, T. H., and Faubert, J. (2019). Deep learning-based electroencephalography analysis: a systematic review. J. Neural. Eng. 16:051001. doi: 10.1088/1741-2552/ab260c
Seedat, Z. A., Quinn, A. J., Vidaurre, D., Liuzzi, L., Gascoyne, L. E., Hunt, B. A. E., et al. (2020). The role of transient spectral ‘bursts’ in functional connectivity: a magnetoencephalography study. Neuroimage 209:116537. doi: 10.1016/j.neuroimage.2020.116537
Sherman, M. A., Lee, S., Law, R., Haegens, S., Thorn, C. A., and Hämäläinen, M. S. (2016). Neural mechanisms of transient neocortical beta rhythms: converging evidence from humans, computational modeling, monkeys, and mice. Proc. Natl. Acad. Sci. U.S.A. 113, E4885–E4894. doi: 10.1073/pnas.1604135113
Shin, H., Law, R., Tsutsui, S., Moore, C. I., and Jones, S. R. (2017). The rate of transient beta frequency events predicts impaired function across tasks and species. Elife 6:e29086. doi: 10.7554/eLife.29086
Song, X., Yoon, S. C., and Perera, V. (2013). “Adaptive common spatial pattern for single-trial EEG classification in multisubject BCI,” in Proceedings of the 6th International IEEE/EMBS Conference on Neural Engineering (NER) (Piscataway, NJ: IEEE), 411–414. doi: 10.1109/NER.2013.6695959
Tam, W., Wu, T., Zhao, Q., Keefer, E., and Yang, Z. (2019). Human motor decoding from neural signals: a review. BMC Biomed. Eng. 1:22. doi: 10.1186/s42490-019-0022-z
Torrecillos, F., Tinkhauser, G., Fischer, P., Green, A. L., Aziz, T. Z., Foltynie, T., et al. (2018). Modulation of beta bursts in the subthalamic nucleus predicts motor performance. J. Neurosci. 38, 8905–8917. doi: 10.1523/JNEUROSCI.1314-18.2018
Troebinger, L., López, J. D., Lutti, A., Bestmann, S., and Barnes, G. (2014a). Discrimination of cortical laminae using MEG. Neuroimage 102, 885–893. doi: 10.1016/j.neuroimage.2014.07.015
Troebinger, L., López, J. D., Lutti, A., Bradbury, D., Bestmann, S., and Barnes, G. (2014b). High precision anatomy for MEG. Neuroimage 86, 583–591. doi: 10.1016/j.neuroimage.2013.07.065
Vidaurre, C., and Blankertz, B. (2010). Towards a cure for BCI illiteracy. Brain Topogr. 23, 194–198. doi: 10.1007/s10548-009-0121-6
Vidaurre, C., Kawanabe, M., Von Bünau, P., Blankertz, B., and Müller, K. R. (2011). Toward unsupervised adaptation of LDA for brain-computer interfaces. IEEE Trans. Biomed. Eng. 58, 587–597. doi: 10.1109/TBME.2010.2093133
Wang, L., Xu, G., Wang, J., Yang, S., and Yan, W. (2008). Application of Hilbert-Huang transform for the study of motor imagery tasks. Annu. Int. Conf. IEEE Eng. Med. Biol. Soc. 2008, 3848–3851. doi: 10.1109/iembs.2008.4650049
Wellmer, J., Von Der Groeben, F., Klarmann, U., Weber, C., Elger, C. E., Urbach, H., et al. (2012). Risks and benefits of invasive epilepsy surgery workup with implanted subdural and depth electrodes. Epilepsia 53, 1322–1332. doi: 10.1111/j.1528-1167.2012.03545.x
Wessel, J. R. (2020). B -Bursts reveal the trial-to-trial dynamics of movement initiation and cancellation. J. Neurosci. 40, 411–423. doi: 10.1523/JNEUROSCI.1887-19.2019
Wittevrongel, B., Holmes, N., Boto, E., Hill, R., Rea, M., Libert, A., et al. (2021). Practical real-time MEG-based neural interfacing with optically pumped magnetometers. BMC Biol. 19:1–15. doi: 10.1186/s12915-021-01073-6
Wolpaw, J. R. (2002). Brain Computer Interfaces for communication and control. Front. Neurosci. 4, 767–791. doi: 10.3389/conf.fnins.2010.05.00007
World Health Organization (2011). World Report on Disability 2011. Geneva: World Health Organization.
Yeh, C. H., Al-Fatly, B., Kühn, A. A., Meidahl, A. C., Tinkhauser, G., Tan, H., et al. (2020). Waveform changes with the evolution of beta bursts in the human subthalamic nucleus. Clin. Neurophysiol. 131, 2086–2099. doi: 10.1016/j.clinph.2020.05.035
Keywords: beta bursts, Brain-Computer Interface (BCI), EEG, magnetoencephalography (MEG), motor imagery (MI), neurological rehabilitation, upper limb
Citation: Papadopoulos S, Bonaiuto J and Mattout J (2022) An Impending Paradigm Shift in Motor Imagery Based Brain-Computer Interfaces. Front. Neurosci. 15:824759. doi: 10.3389/fnins.2021.824759
Received: 29 November 2021; Accepted: 21 December 2021;
Published: 12 January 2022.
Edited by:
Marianna Semprini, Italian Institute of Technology (IIT), ItalyReviewed by:
Giacinto Barresi, Italian Institute of Technology (IIT), ItalyCopyright © 2022 Papadopoulos, Bonaiuto and Mattout. This is an open-access article distributed under the terms of the Creative Commons Attribution License (CC BY). The use, distribution or reproduction in other forums is permitted, provided the original author(s) and the copyright owner(s) are credited and that the original publication in this journal is cited, in accordance with accepted academic practice. No use, distribution or reproduction is permitted which does not comply with these terms.
*Correspondence: Sotirios Papadopoulos, sotirios.papadopoulos@univ-lyon1.fr
†These authors have contributed equally to this work