- 1Synapse Lab, Athinoula A. Martinos Center, Massachusetts General Hospital, Boston, MA, United States
- 2NHC Key Laboratory of Mental Health, National Clinical Research Center for Mental Disorders, Peking University Sixth Hospital, Beijing, China
- 3Department of Business Analytics, Bentley University, Waltham, MA, United States
- 4Department of Psychiatry, Beth Israel Deaconess Medical Center, Boston, MA, United States
Given the significance of validating reliable tests for the early detection of autism spectrum disorder (ASD), this systematic review aims to summarize available evidence of neuroimaging and neurophysiological changes in high-risk infants to improve ASD early diagnosis. We included peer-reviewed, primary research in English published before May 21, 2021, involving the use of magnetic resonance imaging (MRI), electroencephalogram (EEG), or functional near-infrared spectroscopy (fNIRS) in children with high risk for ASD under 24 months of age. The main exclusion criteria includes diagnosis of a genetic disorder and gestation age of less the 36 weeks. Online research was performed on PubMed, Web of Science, PsycINFO, and CINAHL. Article selection was conducted by two reviewers to minimize bias. This research was funded by Massachusetts General Hospital Sundry funding. IRB approval was not submitted as it was deemed unnecessary. We included 75 primary research articles. Studies showed that high-risk infants had divergent developmental trajectories for fractional anisotropy and regional brain volumes, increased CSF volume, and global connectivity abnormalities on MRI, decreased sensitivity for familiar faces, atypical lateralization during facial and auditory processing, and different spectral powers across multiple band frequencies on EEG, and distinct developmental trajectories in functional connectivity and regional oxyhemoglobin concentrations in fNIRS. These findings in infants were found to be correlated with the core ASD symptoms and diagnosis at toddler age. Despite the lack of quantitative analysis of the research database, neuroimaging and electrophysiological biomarkers have promising value for the screening of ASD as early as infancy with high accuracy, which warrants further investigation.
Introduction
Autism spectrum disorder (ASD) is a developmental disorder characterized by deficits in social interaction and repetitive and restrictive behaviors. The prevalence of ASD diagnosis has been steadily rising for decades; as of the most recent report in 2016, 1 in 54 children will be diagnosed with ASD by the age of 8 (Maenner, 2020), and, between 2002 and 2004, the average age of ASD diagnosis in Medicaid-enrolled children was not until 64.9 months (Mandell et al., 2010). Clinical diagnosis of autism is typically not reliable until after 24 months of age (Sanchack and Thomas, 2016). Current clinical diagnosis of ASD is based on the presentation of core symptoms (Sanchack and Thomas, 2016). The Autism Diagnostic Observation Schedule, Second Edition (ADOS-2), commonly referred to as the gold standard diagnostic tool for autism, assesses the clinical presentation of these symptoms, in order to guide ASD diagnosis (Carr, 2013).
Various psychometric screening instruments have been proposed and applied to improve early detection and diagnosis in ASD (Levy et al., 2020; Petrocchi et al., 2020). In recent years, information and communication technologies (ICT) related products such as smartphones, tablets, eye trackers, or robots (Desideri et al., 2021), home video (Tariq et al., 2018), multi-modular AI approaches (Abbas et al., 2020), and machine learning strategies (Achenie et al., 2019) have offered easier and comparable alternative psychometric screening for ASD. However, these psychometric screening methods are not readily implemented during infancy.
In recent years, early biological indicators of ASD have been studied even in infancy (Frye et al., 2019). In addition to genetic, immunological, and metabolic biomarkers, brain imaging and neurophysiology biomarkers may have diagnostic utility during infancy. Because ASD diagnosis is not yet possible in infants, most research into the early signs of ASD divides research populations into infants with high familial risk (HR infants) and infants without familial risk (LR infants). Recent research Hazlett et al. (2017) tested 106 HR infants and 42 LR infants for ASD in a longitudinal study using a deep learning algorithm to measure the surface area on Magnetic Resonance Imaging (MRI); this study was conducted in a cohort of ASD individuals at 6–12 months to predict the diagnosis at 24 months and found to have 88% sensitivity and 81% positive predictive value (Hazlett et al., 2017). Emerson et al. (2017) tested 59 infants with high risk for ASD in a longitudinal study and found functional connectivity at 6 months with a machine-learning algorithm predicted ASD diagnosis at 24 months with 81.8% sensitivity (Emerson et al., 2017). Other longitudinal studies have leveraged electroencephalogram (EEG) (Kolesnik et al., 2019) and functional Near-Infrared Spectroscopy (fNIRS) (Kolesnik et al., 2019; Zhang and Roeyers, 2019) during infancy to predict ASD diagnosis later in life. Neurological differences between HR infants who are later diagnosed with ASD (HR-ASD) and HR infants who test negative for ASD (HR-TD) must be studied, so that early detection becomes more accurate and can be clinically applied.
Detection of abnormal brain development and neurological activity in HR-ASD infants with neuroimaging and neurophysiological methods offers a promising avenue for early screening by directly reflecting the early pathological changes related to later development of ASD. The new findings in this field have not yet been reported or emphasized. We will focus on MRI, EEG, and fNIRS, the three most used and reported neuroimaging and neurophysiological tools in the field for early ASD screening. This systematic review aims to summarize available evidence from primary research of these three neuroimaging and neurophysiological methods to characterize neurological differences in HR infants and compare the pros and cons of each modality in ASD early screening.
Materials and Methods
Inclusion and Exclusion Criteria
The study was conducted in the absence of an Internal Review Board approved protocol. The inclusion criteria was established and applied as follows: all articles were published in English; all articles were peer-reviewed, primary research; all articles conducted empirical research that involved the use of EEG, MRI, or fNIRS in children with HR for ASD; all articles had a scanning age of 24 months of age or younger; all articles grouped infants based on risk for developing ASD. The exclusion criteria was established and applied as follows: No articles included participants with genetic disorders, including but not limited to epilepsy, Fragile X syndrome, Rett syndrome, tuberous sclerosis, and cerebral palsy; no articles included infants with a gestational age of less than 36 weeks; no articles were designed not to report findings, such as methodological articles.
Literature Research Strategy
We conducted a comprehensive search for studies that focused on the developmental stage of infants and toddlers under age of 2 years and at risk for ASD. We focused on studies that used EEG, MRI or fNIRS. All articles were published before May 21st, 2021. The online research was performed on PubMed, Web of Science, PsycINFO, and CINAHL. Using keyword searches across four scientific databases, a total of 943 articles were initially considered for this systematic review. All articles evaluated in this study were gathered through searching for three keyword domains. First, the article needed to contain either “autism” or “ASD.” Second, the article needed to contain a word or phrase that indicated that participants had a high risk of developing ASD, such as “at risk” or “familial risk.” Third, the article needed to contain a keyword related to either MRI, EEG, or fNIRS.
The following types of articles were excluded during initial screening: anecdotal case reports of a single or few patients, analysis of clinical database or registries, review articles, commentaries, mechanism of action research articles, meta-analysis or systematic reviews, conference papers, preclinical and animal studies, interventional clinical trials, and studies designed not to find meaningful results. Titles and abstracts of the papers identified by this initial search strategy were evaluated by two independent reviewers. We obtained potentially relevant papers and conducted subsequent full text screens. From the database search, 66 articles were selected to be reviewed. We also conducted a recursive search of the literature based on the bibliographies of the relevant full text articles. Nine additional articles were identified, screened, and included based on that search of references in relevant full text articles. Two independent reviewers conducted full text screens using pre-designed eligibility criteria. Any disagreement between investigators was resolved by consensus. One paper, Stahl et al. (2012), was categorized as a methodological paper because it used the same dataset as Elsabbagh et al. (2009) and reported similar findings, so its contribution to the field was determined to be methodological in nature and was excluded (Elsabbagh et al., 2009; Stahl et al., 2012). Two papers, Brito et al. (2019) and Riva et al. (2019), while very similar to included articles, did not group infants by ASD risk at the start of the experiment and were categorized as the wrong subject matter and excluded (Brito et al., 2019; Riva et al., 2019). See Figure 1 for the details.
Assessment of Risk of Bias
Steps were taken by reviewers to minimize bias in this systematic review. Search terms were created prior to the start of the systematic review and were executed across four scientific databases. Search terms were optimized through the consensus of all reviewers. Inclusion and exclusion criteria is clearly stated and was appropriately applied to all articles. The quality or interpretation of article findings were not evaluated during the exclusion process. At least two reviewers achieved consensus on all included articles. The literature research strategy was followed closely, and no protocol deviations occurred to the knowledge of the reviewers. Because of the diversity of research included in this systematic review, no quantitative meta-analysis was conducted.
Data Extraction
Material information has been extracted from each and every selected study. One reviewer extracted individual imaging or electrophysiology data from each study (sample size, mean, standard deviation, and percentages of samples of ASD and control groups) into a pre-piloted Microsoft Excel spreadsheet (XP professional edition; Microsoft Corp., Redmond, WA, United States). A second reviewer cross-checked each data variable extracted against the original publication. Disagreement was resolved via consensus. In addition, the following information was extracted wherever available: age of participants, gender of participants, imaging or electrophysiology methodology, and definition of positive result. When necessary, we contacted the authors to obtain original data via e-mail.
Results
A total of 473 unique studies were identified, after excluding duplicates. Following a primary evaluation of titles and abstracts and exclusion based on article type, 297 articles were further assessed for eligibility. In the secondary evaluation, an additional 222 articles were excluded because of the exclusion criteria enumerated in Figure 1. Finally, 75 articles met our inclusion criteria for the present systematic review, including 26 articles using MRI, 41 EEG, and 8 fNIRS. See Figure 2 for further detail about MRI, EEG, and fNIRS article screening.
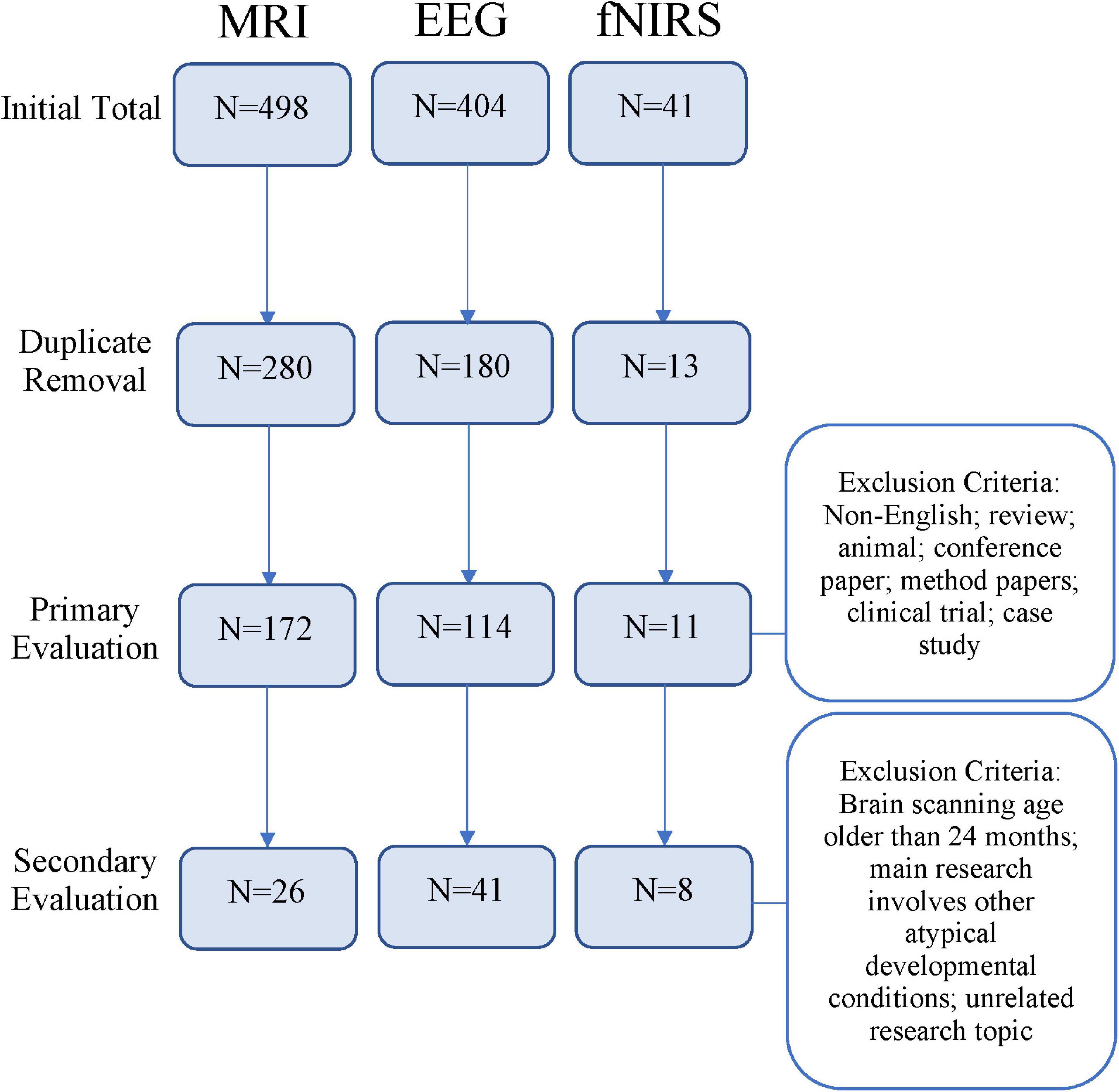
Figure 2. Flowchart depicting article screening process based on research methods [magnetic resonance imaging (MRI), electroencephalogram (EEG), or functional near-infrared spectroscopy (fNIRS)].
Magnetic Resonance Imaging Studies
Magnetic resonance imaging (MRI) is a non-invasive and sophisticated imaging technique that uses strong magnetic fields to generate two dimensional image slices of the human body. The MRI discussed in this review will be limited to imaging of the brain, although the application therein will be diverse. Namely, MRI can be carried out to assess structure, using methods such as diffusion-weighted imaging, or function, through methods such as BOLD imaging. Twenty-six MRI studies were selected and are reviewed below. Twenty structural studies and six functional studies have been reviewed.
Structural Studies
White Matter Tracts
Substantial investigation into white matter structures via analysis of fractional anisotropy (FA), a measurement of diffusion properties in tissues, has revealed divergent trajectories between HR infants who later develop ASD (HR-ASD) and HR infants who do not. HR-ASD infants were found to have greater FA values at 6 months, before exhibiting slower FA growth until 24 months for bilateral limbic (fornix) and association (inferior longitudinal fasciculus and uncinate fasciculus) fiber tracts (Wolff et al., 2012), and ASD toddlers continued to display slower age-related changes in FA after 24 months (Solso et al., 2016). Enriched FA in the right superior longitudinal fasciculus at 6 weeks of age in HR infants was associated with more severe ASD symptoms at 36 months (Liu et al., 2019). Starting at 6 months, HR-ASD infants displayed elevated corpus callosum area and thickness, particularly in the anterior corpus callosum (Wolff et al., 2015). Longitudinal analysis from 6 to 24 months revealed that cerebellar and callosal white matter tracts were associated with repetitive behaviors and sensory responsiveness but not social deficits (Wolff et al., 2017). While HR-ASD infants had delayed visual-orienting latencies, LR infants exhibited an association between microstructural organization in the corpus callosum and visual-orienting latencies (Elison et al., 2013).
Network efficiency, measured by the strength of association and length of white matter tracts between regions, has been found to be reduced in HR infants. Lewis et al. (2014) reported that global efficiencies in the temporal, parietal, and occipital lobes, and Broca’s area were reduced in HR-ASD infants (Lewis et al., 2014). Further, these global inefficiencies were negatively correlated to ASD symptom severity across all HR infants (Lewis et al., 2014). A follow-up study by the same research group found local network inefficiencies in the right auditory cortex at 6 months and global network inefficiencies across Broca’s area at 12 months, which were both associated with symptom severity at 24 months (Lewis et al., 2017). Notably, machine learning techniques that use FA measurements and network connectivity have been implemented to generate an ASD classifier with an accuracy of 76% (Jin et al., 2015).
Cerebrospinal Fluid (CSF) and Regional Volumes
Increased extra-axial CSF starting at 6 months (Shen et al., 2013) and persisting through 24 months (Shen et al., 2017) has been observed in HR-ASD. Predicting ASD diagnosis using 6 months extra-axial volume at 6 months had an accuracy of 69% (Shen et al., 2017). While generalized cerebral cortical overgrowth was observed in 2-year-old toddlers with ASD (Hazlett et al., 2011), a follow-up article by the same research group reported that HR infants at 6 months old presented with no significant differences in gross regional volumes (Hazlett et al., 2012). Hazlett et al. (2017) found that HR-ASD infants experienced cortical surface area hyper-expansion between 6 and 12 months, then brain volume overgrowth between 12 and 24 months, which was associated with social deficits at 24 months of age. These researchers leveraged surface area information from 6 and/or 12 months to predict the autism diagnosis at 24 months and achieved an accuracy of 81% (Hazlett et al., 2017). While Cárdenas-de-la-Parra et al. (2020) agrees that HR-ASD infants experience overall brain volume overgrowth after 12 months of age, they argue that, compared to LR and HR-TD infants, HR-ASD infants actually have increased growth in white matter areas, including the splenium of the corpus callosum, and decreased growth in gray matter areas, including the right fusiform gyrus and inferior temporal gyrus (Cárdenas-de-la-Parra et al., 2020).
More recent research builds upon the findings from Hazlett et al. (2017) and delineates significant relationships between abnormal brain volumes and ASD symptomology. Infants between 4 and 6 months old were found to have significantly larger cerebellar and subcortical volumes, which were associated with core repetitive behaviors at 36 months (Pote et al., 2019). Subcortical volumes at 12 months have been associated with language skills at 24 months (Swanson et al., 2017). The amygdala and hippocampus were found to be enlarged in HR infants and toddlers between the ages of 6 and 24 months (Li et al., 2019). HR-ASD infants were also found to not only have more difficulty sleeping, but these sleeping difficulties were found to be directly related to hippocampal volume (MacDuffie et al., 2020).
Functional Studies
Two studies established differences in functional connectivity development between HR and LR infants in 1.5-month-old infants. Liu et al. (2020a) reported decreased connectivity between the temporal and somatosensory regions at 1.5 months but hyperconnectivity between these regions and decreased interhemispheric connectivity at 9 months old (Liu et al., 2020a). Nair et al. (2021) found that HR infants at 1.5 months had under- and overconnectivity in different thalamic pathways and that these aberrant connectivities were correlated with atypical social development at prior to 36 months. Functional connectivity at 6 months of age correlated with psychometric scores related to social behavior, language, motor development, and repetitive, ritualistic, and stereotyped behavior at 24 months of age (Emerson et al., 2017; McKinnon et al., 2019). Functional connectivity data collected at 6 months successfully predicted ASD with a positive predictive value of 100% (Emerson et al., 2017).
Few studies have examined fMRI activity in response to stimuli. Blasi et al. (2015) found that, when presented with auditory stimuli during sleep, only LR infants demonstrated specialization for human voices in the temporal and medial regions and exhibited stronger sensitivity to sad vocalizations in the right fusiform gyrus and left hippocampus (Blasi et al., 2015). Notably, HR infants, unlike the LR infants, demonstrated a correlation between social engagement and degree of voice specialization, indicating the potential importance of specialization in HR infants. Similarly, Liu et al. (2020b) found greater activity in the left amygdala and learning-relating signaling in the left temporal region in the LR group, but this signaling in the left temporal region at 9 months was negatively correlated with ASD symptom severity at 36 months (Liu et al., 2020b).
In summary, while HR-ASD infants’ white matter tracts develop rapidly up until 6 months of age and then more slowly through toddlerhood compared to HR-TD and LR children, they simultaneously exhibit gray matter overgrowth after 6 months, both on the whole and in several regions in particular, such as the amygdala and hippocampus. HR-ASD infants have complex, abnormal connectivity, characterized by structural, global inefficiency and functional over- and underconnectivity along multiple major neural pathways. The different developmental trajectory of white matter, gray matter, and functional connectivity in HR-ASD infants have all been significantly associated with the core symptoms of ASD, including stereotyped behavior and social function. See Table 1 for details about each MRI article reviewed.
Electroencephalogram Studies
An electroencephalogram (EEG) is an inexpensive, commonly used, typically non-invasive neurophysiological technique that monitors electrical activity in the brain by measuring the electric potential between electrodes that are placed on the scalp (Bell and Cuevas, 2012). The main application of an EEG is to assess cerebral function, as opposed to detecting structural abnormalities as in MRI. A total of 41 EEG studies met the inclusion criteria of this review. These articles have been organized into several sections based on analysis methods and main findings.
Event-Related Potential
Facial Processing
It has long been thought that differential activation patterns related to face processing and social bonding may be potential early signs of ASD. One potential difference in face processing in HR-ASD infants is hemispherical asymmetry. HR infants, and even more so in HR-ASD infants, exhibited aberrant left lateralization of event-related gamma-band phase coherence compared to LR infants when presented with familiar and unfamiliar faces (Keehn et al., 2015).
Electroencephalogram P400 is hypothesized to be related to face familiarization, but it’s potential relation to divergence in HR infant processing remains highly debated. In response to a novel face as a compared to a familiar face, both shorter P400 latency (Key and Stone, 2012a; Jones et al., 2016) in HR infants and no difference in P400 latency (Luyster et al., 2011, 2014; Guy et al., 2018) have been reported. To better understand the potential role of P400 in HR development, numerous stimuli have been tested. Elsabbagh et al. (2012) found that 7-month old infants who later developed ASD did not exhibit a difference in P400 amplitude in response to a change in gaze toward the infant as opposed to a gaze change away from the infant; both LR infants and HR who did not later develop ASD (HR-TD) exhibited significantly larger P400 amplitude in response to gazes toward the infant. In a follow-up study, Elsabbagh et al. (2015) found that greater P400 latency positively correlated with positive affect in infants interacting with mothers only in the HR group, indicating a more complex role of P400 in social bonding. P400 latency was found to be delayed in HR infants in response to direct gaze, and not in response to averted gaze (Elsabbagh et al., 2009, 2015). A recent study was able to leverage ERP data from 6-month-old infants undergoing a direct/averted gaze paradigm to classify HR-ASD and HR-TD with an accuracy of 88.44% (Abou-Abbas et al., 2021). ERP activation to different facial expressions has also been investigated (Key et al., 2015). Differences between LR and HR groups were found to be most pronounced for subtle face expressions. The HR group exhibited shorter P400 latency in response to small smiles compared to the LR group, which indicates that LR infants may process these facial expressions more substantially (Key et al., 2015). Interestingly, another study found that HR infants had faster responses to objects than the LR infants but equal responses to faces according to P400 latency, indicating that HR infants may process objects differently (McCleery et al., 2009). P400 amplitude and latency differences between HR and LR infants may be an early sign of ASD diagnosis that indicates divergence in facial processing.
N290, the EEG component precursor to the face-processing N170 component in adults, has also been studied in HR infants. Similar to P400, HR infants displayed faster N290 responses to objects than LR infants did (McCleery et al., 2009). This study also reported that HR infants lacked the hemispheric asymmetry for both P400 and N290 amplitude that LR infants exhibited in response to faces and objects. One study found that changes in the facial features of the visual stimulus were associated with the N290 component only in 9-month-old LR infants and not HR infants (Key and Stone, 2012b). In response to non-face stimuli, HR infants at 7 months who exhibited decreased N290 amplitude also had more atypical activity patterns and more severe autism symptoms (Shephard et al., 2020). Based on these two studies, the N290 component may be more important for facial processing in LR infants and may be more related to other types processing in HR infants.
Differences in P400 and N290 latency and amplitude between LR and HR infants indicate that HR infants are less sensitive to novel faces and subtle facial expressions, although there is not complete agreement in the field. An alternative hypothesis that HR infants have atypical processing of objects may be related to or partially explain atypical facial processing. Multiple studies have observed differences in other EEG components, including Nc and P100, but the relation between these components and facial processing is not well understood.
Auditory Processing
Event-Related Potential (ERP) evoked by auditory stimuli has also been investigated for its utility in early screening. One article found that ERP responses to non-native speech were similar between groups, but, between 6 and 12 months, LR infants exhibited smaller and more lateralized negative-going later slow wave (LSW) to the speech sounds than HR infants (Seery et al., 2013). Interestingly, another study found that at 12 months, HR-ASD showed reversed lateralization of LSW to speech stimuli compared to the LR group (Finch et al., 2017). A recent study found that HR individuals at 14 months, but not 10 months, showed attenuated frontal positive going activity across both hemispheres in response to their own name compared to an unfamiliar name (Arslan et al., 2020). HR infants may have atypical lateralization for auditory processing prior to 1-year-old.
Atypical auditory processing in HR infants has been linked to later development of ASD symptoms. In response to non-speech stimuli, HR-ASD infants exhibited mismatch response (MMR) latency compared to the LR group, and HR-ASD infants had overall larger P3 amplitude at 12 months (Riva et al., 2018). Both MMR latency and larger P3 amplitude were correlated with later developed ASD symptomology (Riva et al., 2018). In response to concatenated syllables, alpha coherence at 3 months was found to be associated with word production at 18-month-olds (Tran et al., 2021). In response to repeated tones, cortical gamma amplitude and inter-trial coherence in HR children were associated with reduced growth in language skills between 8 months and 3 years and elevated levels of parent-rated social communication symptoms at 3 years (Kolesnik et al., 2019). Atypical neural response to both speech and non-speech stimuli are associated with ASD symptoms, particularly language and social development, in ASD infants.
Sensory Processing
Children with ASD often have increased sensory hypersensitivity compared to typically developing children. A recent study found that the HR group showed greater sensory hypersensitivity at 6 months and 2 years, which was predictive of improved neural responsiveness and social outcomes at 4 years of age (Jones et al., 2018). Another study found that HR toddlers at 18 months had increased sensory seeking, generally indicating under-sensitivity, which was associated with atypical frontal asymmetry and social communication deficits (Damiano-Goodwin et al., 2018). Piccardi et al. (2021) reported that reduced neural repetition in response to tactile stimuli, rather than just under-sensitivity, predicted later ASD diagnosis (Piccardi et al., 2021). HR infants and toddlers exhibit diversely atypical sensory sensitivity, but hypersensitivity, in particular, may be associated with better social symptoms.
Form and Motion Processing
Differential neural activity during shape recognition and motion observation in HR infants may also be useful in early screening. One study found that HR infants showed increased lateral frontal positivity (LFP), an index related to high level processing and learning, and N700, an occipital component, response to low probability conditions of shape pairs, while LR infants demonstrated increased LFP and N700 response to the high probability shape pairs (Marin et al., 2020). Notably, LFP at 3 months across both groups predicted visual reception skills but not ASD symptoms at 18 months. Five-month-old HR infants, observing coherent visual motion, were found to have divergent lateralization of ERP activation patterns in response to global visual changes but minimal divergence in response to local visual changes (Nyström et al., 2020). The authors hypothesized that the findings support that differences in global coherence processing, rather than local processing, are responsible for divergent cognition in ASD.
Resting State Electroencephalogram
Functional Connectivity
The relationship between restricted and repetitive behaviors and functional connectivity was supported by two studies, a primary article and a replication of that study (Orekhova et al., 2014; Haartsen et al., 2019). In the original study, functional connectivity at 14 months had a strong correlation with the severity of restricted and repetitive behaviors at 3 years in response to audio-visual stimuli. HR-ASD infants also exhibited significantly elevated phase-lagged alpha-ranged connectivity compared to both LR infants and HR-TD infants (Orekhova et al., 2014). While the replication study failed to find significantly elevated global connectivity in HR-ASD infants, strong correlation between functional connectivity and restricted and repetitive was further supported (Haartsen et al., 2019). Conversely, another study found that 12-month-old HR-ASD infants showed reduced functional connectivity relative to LR and HR infants in response to language-relevant stimuli (Righi et al., 2014). A recent study has shed light on perhaps a more complicated explanation for these conflicting results. Dickinson et al. (2020) found that HR-ASD infants with lower frontal connectivity and higher right temporoparietal connectivity at 3 months predicted more severe ASD symptoms at 18 months (Dickinson et al., 2020).
Frequency Bands and Autism Spectrum Disorder Symptomology
Several studies have demonstrated correlations between frequency bands and ASD-related symptoms. Researchers found that frontal theta change in HR infants correlated with greater non-verbal skills and verbal skills in toddlerhood (Jones et al., 2020). For HR-ASD infants, theta EEG explained over 80% of variance in non-verbal skills at 3 years of age. Two studies found that expressive language in HR infants correlated with frontal power in the gamma and alpha bands relative to LR infants (Levin et al., 2017; Wilkinson et al., 2019). Among HR infants, increased frontal gamma was only marginally associated with ASD diagnosis but significantly associated with reduced expressive language ability (Wilkinson et al., 2019); reduced frontal high-alpha power at 3 months was also robustly associated with poorer expressive language at 12 months (Levin et al., 2017). Another study found that peak alpha frequency at 12 months, while not a good predictor of ASD risk, did correlate with non-verbal cognitive ability at 36 month-olds (Carter Leno et al., 2021). Page et al. (2020) examined EEG during NREM sleep. Researchers, despite finding topographically distinct decreases in fast theta oscillations and fast sigma (15–16 Hz) and an increase in beta oscillations in HR-ASD infants compared to LR infants, found no significant correlations between ASD severity and these frequency bands (Page et al., 2020).
Several bands in the frontal area may be correlated with a critical developmental skills, even if they cannot be directly linked to ASD diagnosis. For example, the theta band may be related to non-verbal skills, and the gamma and alpha bands to expressive language. On the other hand, frontal alpha asymmetry may be positively associated with several ASD-related behaviors.
Developmental Trajectory
Recent research has evaluated how early differences in HR neural activity may relate to children’s neural development over time. During resting state, HR infants exhibited frontal alpha asymmetry differently from LR infants at 6 months and opposite growth in asymmetry by 12 months (Gabard-Durnam et al., 2015). Similarly, found that at 12 months the developmental trajectories of HR-ASD and LR infants diverged (Bosl et al., 2011). A longitudinal study in HR and LR children from 3 to 36 months found that power dynamics during the first year and delta and gamma bands in particular were most robust in differentiating ASD diagnoses (Gabard-Durnam et al., 2019). Tierney et al. (2012) investigated the spectral power across delta, theta, low alpha, high alpha, beta, and gamma frequencies in infants/toddlers at 6, 9, 12, 18, and 24 months of age. Reduced spectral power across all frequency bands were observed in HR infants at 6 months, but only the delta, theta, and beta frequency bands were rectified by 24 months, indicating divergent trajectories for individual frequency bands in HR individuals (Tierney et al., 2012). Huberty et al. (2021) also found that HR infants had divergent trajectories for power in each band but found that reduced power at 3 months and steeper change during later development was associated with familial risk and not later ASD diagnosis (Huberty et al., 2021).
Increasingly, developmental trajectory of certain EEG components are being used to predict ASD diagnosis and severity. Wilkinson et al. (2020) used spectral power across six frequency bands over a period from 3 to 12 months of age to accurately predict language scores at 24 months (Wilkinson et al., 2020). Another study found that multiscale entropy, a measure of functional brain complexity, also exhibited a divergent developmental trajectory in HR children (Bosl et al., 2011). When using multiscale entropy as a classifier for ASD, accuracy peaked at 9 months for boys (100% accuracy) and 6 months for girls (80% accuracy) and decreased at later developmental timepoints. Recently, statistical learning methods were implemented to classify individuals developing ASD based on EEG analyzes at 3, 6, 9, 12, 18, 24, and 36 months (Bosl et al., 2018). Using parameters including frequency bands and multiscale entropy, this algorithm accurately classified HR-ASD infants at greater than 95% for certain ages and predicted ADOS severity scores that strongly correlated to future ADOS scores.
In summary, HR infants have distinct P400 and N290 activity in response to both face and object stimuli, although the exact differences and the interpretation of those differences is debated. Across many different paradigms, HR infants exhibit atypical lateralization of neural activity. Neural response to auditory stimuli and sensory stimuli in HR infants was found to predict language and social communication development. Global functional connectivity was found to be strongly correlated with repetitive and restricted behaviors. Developmental trajectories across different frequency bands in HR infants were found to predict different ASD-related symptoms and could be leveraged to classify later ASD diagnosis with high accuracy. See Table 2 for details about each EEG article reviewed.
Functional Near-Infrared Spectroscopy Studies
Functional near-infrared spectroscopy (fNIRS) is a non-invasive, low-cost, easy set-up functional neuroimaging technique that is used to measure frontal-temporal brain activity. It allows functional imaging of brain activation through the monitoring of blood oxygenation and volume in specific parts of the brain. This is done by measuring the concentration of both oxyhemoglobin and deoxyhemoglobin—which scatter near infrared light of different wavelengths (Di Domenico et al., 2019). In short, researchers are able to monitor blood flow in the brain by measuring the changes in near-infrared light. Compared with MRI and EEG, fNIRS is more tolerant to head movement; however, it is a relatively new tool and less commonly used. A total of eight published articles were selected that utilized near-infrared spectroscopy. Of the eight articles, three used simple language as the stimuli, while five used more complex social and non-social stimuli.
Language
In Keehn et al. (2013), Edwards et al. (2017) and Pecukonis et al. (2020), a trisyllabic sequence in either ABB or ABC was used as a stimulus (Keehn et al., 2013; Edwards et al., 2017; Pecukonis et al., 2020). HR-ASD infants at 3 months had increased functional connectivity but at 12 months had decreased functional connectivity compared to the LR infants (Keehn et al., 2013). While LR infants at 6 months had greater response activation in the temporal and frontal lobes compared to occipital and parietal lobes, HR infants did not (Pecukonis et al., 2020). LR infants also had greater activation in the frontal and parietal lobes than HR infants (Pecukonis et al., 2020). Female HR infants at 3 months had constant neural activity over exposure to repetition-based stimuli, rather than decreased activity as observed in female LR infants (Edwards et al., 2017).
Social Function
One study showed that HR infants between the ages of 4–6 months showed less selective neural responses to both auditory and visual social stimuli than LR infants (Lloyd-Fox et al., 2013). Other studies have shown that HR-ASD infants exhibited reduced brain activation when presented with a social task-based stimuli (Fox et al., 2013; Braukmann et al., 2018; Lloyd-Fox et al., 2018; Bhat et al., 2019). More specifically, HR-ASD infants showed reduced oxyhemoglobin concentration in the lateral regions and right posterior temporal cortex and increased deoxyhemoglobin in the frontal region relative to LR infants (Fox et al., 2013; Braukmann et al., 2018). Furthermore, reduced activation to visual social stimuli across the inferior frontal region and posterior temporal regions were shown in HR-ASD infants (Lloyd-Fox et al., 2018). Parallel to this, two studies that compared response to social and non-social interactions in their analyses found that HR-ASD infants showed greater brain activity when presented with non-social tasks (Lloyd-Fox et al., 2018; Bhat et al., 2019). In Lloyd-Fox et al. (2018), HR-ASD infants exhibited increased activation for non-social stimuli within the left lateralized temporal regions compared to the LR and HR infants. These researchers also reported that these differences in brain activity correlated significantly with parent-rated ASD symptoms at 3 years of age. In Bhat et al. (2019), functional connectivity was found to be enhanced in HR infants in pre- and post- social periods, but reduced during social periods compared to LR infants (Bhat et al., 2019).
In summary, these fNIRS studies connected brain activity and language and social communication development in HR infants. In response to trisyllabic stimulus, HR infants at 3 months had hyperconnectivity, at 6 months had decreased activation in the frontal and parietal lobes, and at 12 months had underconnectivity. HR infants exhibited greater activation in response to non-social tasks and decreased activation for visual social stimuli. See Table 3 for details about each article reviewed.
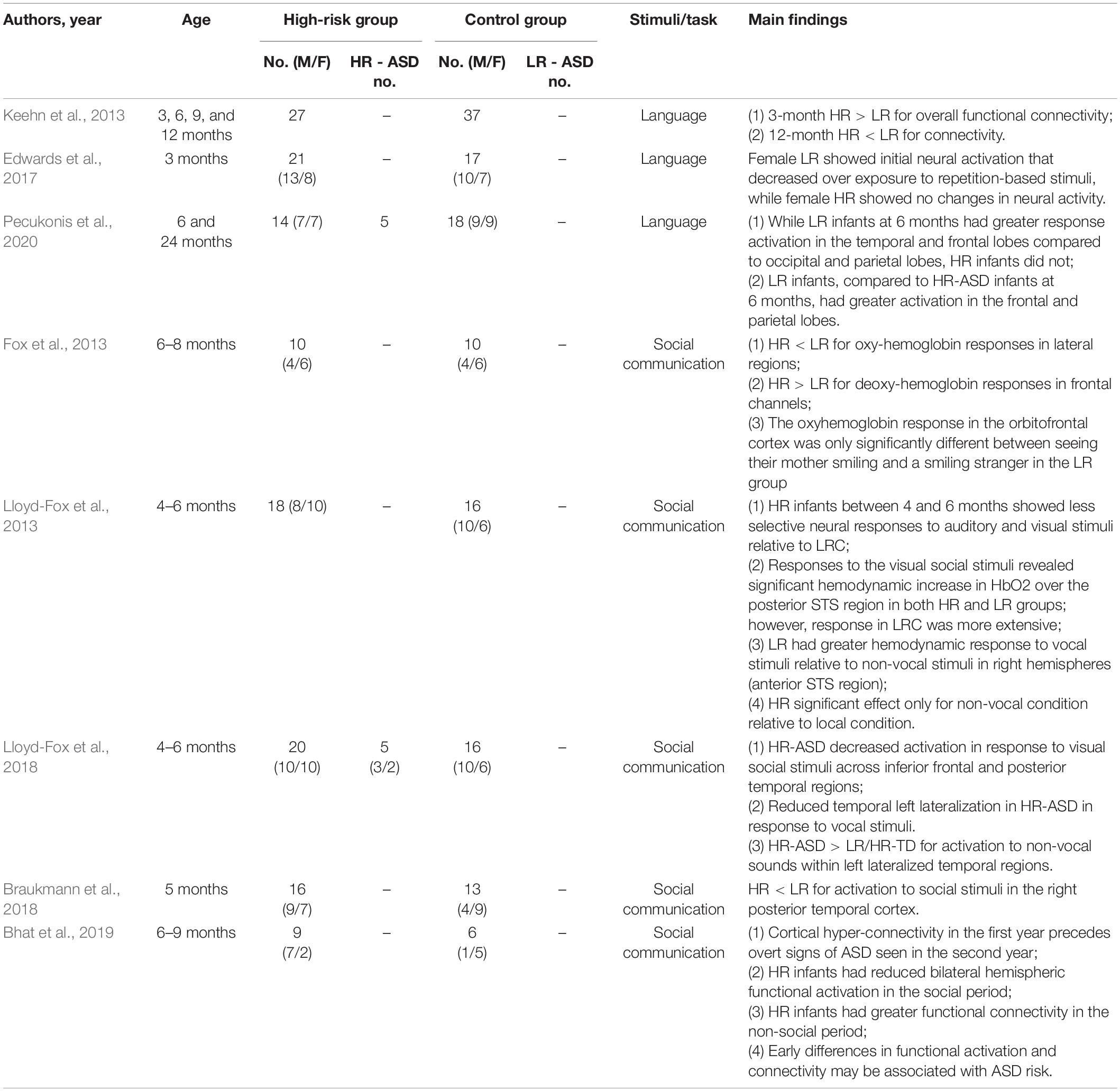
Table 3. Functional near-infrared spectroscopy (fNIRS) studies on early screening of autism spectrum disorders.
Discussion
To this day, psychometric testing remains the main tool for the screening and diagnosis of ASD. Multiple review articles have been written about psychometric early screening tools (Towle and Patrick, 2016; Thabtah and Peebles, 2019; Levy et al., 2020). However, psychometric testing has inherent limitations, which precludes application in infants. Recently, biological testing and its value in early screening has been explored and reported (Frye et al., 2019). Among these biomarkers, differences in brain structure and function in ASD are being identified at earlier stages of development. Neuroradiological and neurophysiological testing via MRI, EEG, and fNIRS have increasing promise as screening tools in infancy with high specificity and sensitivity. This systematic review is the first to provide comprehensive discussion of these neuroimaging and electrophysiological findings in infants at risk for ASD.
The findings in the present systematic review demonstrate how different research tools can synergize and contribute to a common research question. MRI, a sophisticated but commonly used technique, can produce high resolution images with dense information about structure and function. The acquisition of high quality MRI images in any infant population challenging but is becoming more feasible (Denisova, 2019). Notably, the research teams that were able to scan hundreds of patients, such as Shen et al. (2017) and Wolff et al. (2017), were also studies with definitive findings, which gives hope that future research can produce more statistically and clinically meaningful results (Shen et al., 2017; Wolff et al., 2017). EEG and fNIRS are comparatively more affordable and easier to administer to infants. The convenience and temporal resolution of EEG and fNIRS suit them well to more complex experimental paradigms that involve stimuli and social interaction. As our understanding of how ASD brain processing and development may differ from typically developing people grows, EEG and fNIRS experimental design will evolve as well. We propose that the use in combination of these imaging and physiological methods and the use of deep learning algorithms will increase sensitivity and specificity of ASD development prediction.
In order to better understand the underlying mechanisms of ASD, it is important to identify biological differences that are clinically meaningful. Certain structural and functional differences have been implicated in the development of certain ASD-related behaviors. For example, subcortical brain volumes between 4 and 6 months (Pote et al., 2019), FA in the callosal pathways at 6 months (Wolff et al., 2015), and EEG functional connectivity at 14 months (Orekhova et al., 2014; Haartsen et al., 2019) were all associated with repetitive behaviors. Regarding language processing, MRI studies implicated network inefficiencies in auditory-associated regions (Liu et al., 2020a), and ERP studies implicated atypical lateralization and activity in response to diverse auditory stimuli (Seery et al., 2013); each of these findings were independently associated with language development. Future research that clarifies the relationship between the brain of children with ASD and the symptoms they experience will be invaluable in better understanding and screening for ASD at a young age.
Brain structure in HR infants at 6 months may alone be a reliable predictor for later ASD diagnosis. Several studies found at 6 months old that HR infants have divergent white matter tract development (Wolff et al., 2015), increased extra-axial fluid (Shen et al., 2013, 2017), greater volumes in the corpus callosum, amygdala, and hippocampus (Li et al., 2019), network inefficiencies in auditory-associated regions (Lewis et al., 2017), and cortical surface area hyper-expansion (Hazlett et al., 2017). There is even evidence that these structural differences are directly related to the ASD pathology; however, only a few, recent articles have investigated structure-symptom severity relationship. Nevertheless, preliminary, machine-learning-based prediction of ASD diagnosis using structural MRI data conducted by Hazlett et al. (2017) screened HR infants with high accuracy (81%). Future research into the structural differences in HR infants can improve ASD screening accuracy.
Several motifs in atypical brain function were identified in HR infants across functional imaging and neurophysiological testing techniques. First of all, HR-ASD infants have reduced specialization for social stimuli compared to LR infants. MRI research found that HR infants had diminished specialization for human voices in the temporal and medial regions (Blasi et al., 2015; Liu et al., 2020b). EEG research analyzing the P400 and N290 components has shown that HR infants express relatively shortened reaction to novel faces (Key and Stone, 2012a; Jones et al., 2016) and changes in facial expression (Key et al., 2015). Multiple fNIRS studies showed HR infants had reduced activity for social stimuli (Fox et al., 2013; Braukmann et al., 2018; Lloyd-Fox et al., 2018; Bhat et al., 2019). On a related note, EEG (McCleery et al., 2009; Shephard et al., 2020) and fNIRS evidence (Lloyd-Fox et al., 2018) supports that HR-ASD infants may also have atypical reaction to non-social stimuli. Second, HR-ASD infants exhibit atypical lateralization of brain function, especially in response to auditory stimuli. fNIRS research revealed that HR-ASD infants exhibited reduced lateralization of brain activity in response to visual social stimuli (Lloyd-Fox et al., 2018), and EEG research revealed different laterization in response to facial (McCleery et al., 2009), auditory (Seery et al., 2013; Finch et al., 2017), and sensory-somatic stimuli (Damiano-Goodwin et al., 2018). Finally, functional connectivity was found to be aberrant, based on findings from MRI (Liu et al., 2020a; Nair et al., 2021), EEG (Dickinson et al., 2020), and fNIRS studies (Bhat et al., 2019). There is some agreement that HR infants between 6 and 12 months have hyperconnectivity in temporoparietal pathways (Dickinson et al., 2020; Liu et al., 2020a), but functional connectivity was found to vary greatly depending on developmental timepoint, even in the same brain region.
Nevertheless, functional measures have been successful in predicting ASD diagnosis. Emerson et al. (2017) used fMRI-based functional connectivity to predict ASD with an accuracy of 96.6%. Bosl et al. (2011) and Wilkinson et al. (2020) were able to use multiple EEG parameters, such as spectral power across multiple frequency bands and multiscale entropy, in infants to predict ASD development with high accuracy. From this systematic review, we foresee great value in future directions that combine multiple approaches to optimize screening. Biological diagnostic technologies in combination with deep learning and artificial intelligence prediction models have potential for impactful clinical application in ASD detection during infancy. There are clear advantages compared with most psychometric screening methods in terms of detection time and accuracy for ASD, which warrant urgent attention and further research.
Ultimately, leveraging modern technologies for the early screening of ASD allows for highly accurate methods for classification even in infants. Early diagnosis allows for early initiation of therapeutic intervention that may exert beneficial effects for the rest of an individual’s life. It has been widely hypothesized that a developmental critical period exists for ASD, after which time intervention has reduced effectiveness (LeBlanc and Fagiolini, 2011); therefore, early intervention may prove to be especially important for ASD. Psychometric testing relies on the perception and interpretation of symptoms and will always be limited as a result. As described in this systematic review, neuroimaging and neurophysiological methods mainly MRI, EEG, and fNIRS can detect potential characteristics of ASD as early as 1.5 months after birth. Genetic, immunological or metabolic biomarkers may indicate pathogenetic features in ASD individuals and should be checked in combination with these neuroimaging and electrophysiological tests (Frye et al., 2019). It is imperative that empirical methods for early screening of ASD are rigorously pursued in the future, in order to improve the timing and reliability of ASD diagnosis.
In conclusion, certain neuroimaging and electrophysiological features in infancy can be effectively assayed to predict later ASD diagnosis. By elucidating these distinctions and directly correlating them with ASD core symptoms, we improve our understanding of ASD pathology and our ability to diagnose ASD at younger ages. MRI, EEG, and fNIRS each have great potential to improve the accuracy and timeline of ASD diagnosis by use in combination with conventional psychometric methods and other biologically-grounded tests, such as genetic testing. This study is limited as we did not conduct any quantitative assessment of the research database. Also, we focused on neuroimaging and neurophysiological features; we did not search or compare with other quantitative tests, such as genetic or immunological markers, eye tracking, or robotic technology. Further studies are warranted to validate and implement neuroimaging and electrophysiological techniques to meet the urgent needs for early diagnosis and early intervention.
Data Availability Statement
The original contributions presented in the study are included in the article/supplementary material, further inquiries can be directed to the corresponding author.
Author Contributions
X-JK: conceptualization and funding acquisition. CC, JW, ST, HS, and MZ: investigation. CC, JW, and ST: writing—original draft preparation. CC, JW, HS, and X-JK: writing—review and editing. CC, JW, and ST: tables and figures. All authors have read and agreed to the published version of the manuscript.
Funding
This research was funded by Massachusetts General Hospital, grant numbers #230361 and #233263.
Conflict of Interest
The authors declare that the research was conducted in the absence of any commercial or financial relationships that could be construed as a potential conflict of interest.
Publisher’s Note
All claims expressed in this article are solely those of the authors and do not necessarily represent those of their affiliated organizations, or those of the publisher, the editors and the reviewers. Any product that may be evaluated in this article, or claim that may be made by its manufacturer, is not guaranteed or endorsed by the publisher.
Acknowledgments
We appreciate Yiqing Song for his valuable suggestions in methodology. We acknowledge Kevin Liu for his administrative assistance in submission and coordination.
References
Abbas, H., Garberson, F., Liu-Mayo, S., Glover, E., and Wall, D. P. (2020). Multi-modular AI approach to streamline autism diagnosis in young children. Sci. Rep. 10:5014. doi: 10.1038/s41598-020-61213-w
Abou-Abbas, L., van Noordt, S., Desjardins, J. A., Cichonski, M., and Elsabbagh, M. (2021). Use of empirical mode decomposition in ERP analysis to classify familial risk and diagnostic outcomes for autism spectrum disorder. Brain Sci. 11:409. doi: 10.3390/brainsci11040409
Achenie, L. E. K., Scarpa, A., Factor, R. S., Wang, T., Robins, D. L., and McCrickard, D. S. (2019). A machine learning strategy for autism screening in toddlers. J. Dev. Behav. Pediatr. 40, 369–376. doi: 10.1097/DBP.0000000000000668
Arslan, M., Warreyn, P., Dewaele, N., Wiersema, J. R., Demurie, E., and Roeyers, H. (2020). Development of neural responses to hearing their own name in infants at low and high risk for autism spectrum disorder. Dev. Cogn. Neurosci. 41:100739. doi: 10.1016/j.dcn.2019.100739
Bell, M. A., and Cuevas, K. (2012). Using EEG to study cognitive development: issues and practices. J. Cogn. Dev.? 13, 281–291. doi: 10.1080/15248372.2012.691143
Bhat, A. N., McDonald, N. M., Eilbott, J. E., and Pelphrey, K. A. (2019). Exploring cortical activation and connectivity in infants with and without familial risk for autism during naturalistic social interactions: a preliminary study. Infant. Behav. Dev. 57:101337. doi: 10.1016/j.infbeh.2019.101337
Blasi, A., Lloyd-Fox, S., Sethna, V., Brammer, M. J., Mercure, E., Murray, L., et al. (2015). Atypical processing of voice sounds in infants at risk for autism spectrum disorder. Cortex 71, 122–133. doi: 10.1016/j.cortex.2015.06.015
Bosl, W. J., Tager-Flusberg, H., and Nelson, C. A. (2018). EEG analytics for early detection of autism spectrum disorder: a data-driven approach. Sci. Rep. 8:6828. doi: 10.1038/s41598-018-24318-x
Bosl, W., Tierney, A., Tager-Flusberg, H., and Nelson, C. (2011). EEG complexity as a biomarker for autism spectrum disorder risk. BMC Med. 9:18. doi: 10.1186/1741-7015-9-18
Braukmann, R., Lloyd-Fox, S., Blasi, A., Johnson, M. H., Bekkering, H., Buitelaar, J. K., et al. (2018). Diminished socially selective neural processing in 5-month-old infants at high familial risk of autism. Eur. J. Neurosci. 47, 720–728. doi: 10.1111/ejn.13751
Brito, N. H., Elliott, A. J., Isler, J. R., Rodriguez, C., Friedrich, C., Shuffrey, L. C., et al. (2019). Neonatal EEG linked to individual differences in socioemotional outcomes and autism risk in toddlers. Dev. Psychobiol. 61, 1110–1119. doi: 10.1002/dev.21870
Cárdenas-de-la-Parra, A., Lewis, J. D., Fonov, V. S., Botteron, K. N., McKinstry, R. C., Gerig, G., et al. (2020). A voxel-wise assessment of growth differences in infants developing autism spectrum disorder. Neuroimage Clin. 29:102551. doi: 10.1016/j.nicl.2020.102551
Carr, T. (2013). “Autism diagnostic observation schedule,” in Encyclopedia of Autism Spectrum Disorders, ed. F. R. Volkmar (New York, NY: Springer New York).
Carter Leno, V., Pickles, A., van Noordt, S., Huberty, S., Desjardins, J., Webb, S. J., et al. (2021). 12-Month peak alpha frequency is a correlate but not a longitudinal predictor of non-verbal cognitive abilities in infants at low and high risk for autism spectrum disorder. Dev. Cogn. Neurosci. 48:100938. doi: 10.1016/j.dcn.2021.100938
Damiano-Goodwin, C. R., Woynaroski, T. G., Simon, D. M., Ibañez, L. V., Murias, M., Kirby, A., et al. (2018). Developmental sequelae and neurophysiologic substrates of sensory seeking in infant siblings of children with autism spectrum disorder. Dev. Cogn. Neurosci. 29, 41–53. doi: 10.1016/j.dcn.2017.08.005
Darki, F., Nyström, P., McAlonan, G., Bölte, S., and Falck-Ytter, T. (2021). T1-Weighted/T2-weighted ratio mapping at 5 months captures individual differences in behavioral development and differentiates infants at familial risk for autism from controls. Cereb. Cortex 31, 4068–4077. doi: 10.1093/cercor/bhab069
Denisova, K. (2019). Neurobiology, not artifacts: challenges and guidelines for imaging the high risk infant. Neuroimage 185, 624–640. doi: 10.1016/j.neuroimage.2018.07.023
Desideri, L., Pérez-Fuster, P., and Herrera, G. (2021). Information and communication technologies to support early screening of autism spectrum disorder: a systematic review. Children 8:93. doi: 10.3390/children8020093
Di Domenico, S. I., Rodrigo, A. H., Dong, M., Fournier, M. A., Ayaz, H., Ryan, R. M., et al. (2019). “Chapter 28 - functional near-infrared spectroscopy: proof of concept for its application in social neuroscience,” in Neuroergonomics eds H. Ayaz and F. Dehais, (Cambridge, MA: Academic Press), 169–173.
Dickinson, A., Daniel, M., Marin, A., Gaonkar, B., Dapretto, M., McDonald, N. M., et al. (2020). Multivariate neural connectivity patterns in early infancy predict later autism symptoms. Biol. Psychiatry Cogn. Neurosci. Neuroimaging 6, 59–69. doi: 10.1016/j.bpsc.2020.06.003
Edwards, L., Wagner, J., Tager-Flusberg, H., and Nelson, C. (2017). Differences in neural correlates of speech perception in 3 month olds at high and low risk for autism spectrum disorder. J. Autism Dev. Disord. 47, 3125–3138. doi: 10.1007/s10803-017-3222-1
Elison, J. T., Paterson, S. J., Wolff, J. J., Reznick, J. S., Sasson, N. J., Gu, H., et al. (2013). White matter microstructure and atypical visual orienting in 7-month-olds at risk for autism. Am. J. Psychiatry 170, 899–908. doi: 10.1176/appi.ajp.2012.12091150
Elsabbagh, M., Bruno, R., Wan, M. W., Charman, T., Johnson, M. H., Green, J., et al. (2015). Infant neural sensitivity to dynamic eye gaze relates to quality of parent–infant interaction at 7-Months in infants at risk for autism. J. Autism. Dev. Disord. 45, 283–291. doi: 10.1007/s10803-014-2192-9
Elsabbagh, M., Mercure, E., Hudry, K., Chandler, S., Pasco, G., Charman, T., et al. (2012). Infant neural sensitivity to dynamic eye gaze is associated with later emerging autism. Curr. Biol. 22, 338–342. doi: 10.1016/j.cub.2011.12.056
Elsabbagh, M., Volein, A., Csibra, G., Holmboe, K., Garwood, H., Tucker, L., et al. (2009). Neural correlates of eye gaze processing in the infant broader autism phenotype. Biol. Psychiatry 65, 31–38. doi: 10.1016/j.biopsych.2008.09.034
Emerson, R. W., Adams, C., Nishino, T., Hazlett, H. C., Wolff, J. J., Zwaigenbaum, L., et al. (2017). Functional neuroimaging of high-risk 6-month-old infants predicts a diagnosis of autism at 24 months of age. Sci. Transl. Med. 9:eaag2882. doi: 10.1126/scitranslmed.aag2882
Finch, K. H., Seery, A. M., Talbott, M. R., Nelson, C. A., and Tager-Flusberg, H. (2017). Lateralization of ERPs to speech and handedness in the early development of Autism Spectrum disorder. J. Neurodev. Disord. 9:4. doi: 10.1186/s11689-017-9185-x
Fox, S. E., Wagner, J., Shrock, C. L., Flusberg, H. T., and Nelson, C. A. (2013). Neural processing of facial identity and emotion in infants at high-risk for autism spectrum disorders. Front. Hum. Neurosci. 7:89. doi: 10.3389/fnhum.2013.00089
Frye, R. E., Vassall, S., Kaur, G., Lewis, C., Karim, M., and Rossignol, D. (2019). Emerging biomarkers in autism spectrum disorder: a systematic review. Ann. Transl. Med. 7:792. doi: 10.21037/atm.2019.11.53
Gabard-Durnam, L. J., Wilkinson, C., Kapur, K., Tager-Flusberg, H., Levin, A. R., and Nelson, C. A. (2019). Longitudinal EEG power in the first postnatal year differentiates autism outcomes. Nat. Commun. 10:4188. doi: 10.1038/s41467-019-12202-9
Gabard-Durnam, L., Tierney, A. L., Vogel-Farley, V., Tager-Flusberg, H., and Nelson, C. A. (2015). Alpha Asymmetry in Infants at Risk for autism spectrum disorders. J. Autism. Dev. Disord. 45, 473–480. doi: 10.1007/s10803-013-1926-4
Guy, M. W., Richards, J. E., Tonnsen, B. L., and Roberts, J. E. (2018). Neural correlates of face processing in etiologically-distinct 12-month-old infants at high-risk of autism spectrum disorder. Dev. Cogn. Neurosci. 29, 61–71. doi: 10.1016/j.dcn.2017.03.002
Haartsen, R., Jones, E. J. H., Orekhova, E. V., Charman, T., and Johnson, M. H. (2019). Functional EEG connectivity in infants associates with later restricted and repetitive behaviours in autism; a replication study. Transl. Psychiatry 9:66. doi: 10.1038/s41398-019-0380-2
Hazlett, H. C., Gu, H., McKinstry, R. C., Shaw, D. W. W., Botteron, K. N., Dager, S. R., et al. (2012). Brain volume findings in 6-month-old infants at high familial risk for autism. Am. J. Psychiatry 169, 601–608. doi: 10.1176/appi.ajp.2012.11091425
Hazlett, H. C., Gu, H., Munsell, B. C., Kim, S. H., Styner, M., Wolff, J. J., et al. (2017). Early brain development in infants at high risk for autism spectrum disorder. Nature 542, 348–351. doi: 10.1038/nature21369
Hazlett, H. C., Poe, M. D., Gerig, G., Styner, M., Chappell, C., Smith, R. G., et al. (2011). Early brain overgrowth in autism associated with an increase in cortical surface area before age 2 years. Arch. Gen. Psychiatry 68, 467–476. doi: 10.1001/archgenpsychiatry.2011.39
Huberty, S., Leno, V. C., Noordt, S. J. R., Bedford, R., Pickles, A., Desjardins, J. A., et al. (2021). Association between spectral electroencephalography power and autism risk and diagnosis in early development. Autism Res. 14, 1390–1403. doi: 10.1002/aur.2518
Jin, Y., Wee, C.-Y., Shi, F., Thung, K.-H., Ni, D., Yap, P.-T., et al. (2015). Identification of infants at high-risk for autism spectrum disorder using multiparameter multiscale white matter connectivity networks. Hum. Brain Mapp. 36, 4880–4896. doi: 10.1002/hbm.22957
Jones, E. J. H., Dawson, G., and Webb, S. J. (2018). Sensory hypersensitivity predicts enhanced attention capture by faces in the early development of ASD. Dev. Cogn. Neurosci. 29, 11–20. doi: 10.1016/j.dcn.2017.04.001
Jones, E. J. H., Goodwin, A., Orekhova, E., Charman, T., Dawson, G., Webb, S. J., et al. (2020). Infant EEG theta modulation predicts childhood intelligence. Sci. Rep. 10:11232. doi: 10.1038/s41598-020-67687-y
Jones, E. J. H., Venema, K., Earl, R., Lowy, R., Barnes, K., Estes, A., et al. (2016). Reduced engagement with social stimuli in 6-month-old infants with later autism spectrum disorder: a longitudinal prospective study of infants at high familial risk. J. Neurodevelop. Disord. 8, 1–20. doi: 10.1186/s11689-016-9139-8
Keehn, B., Vogel-Farley, V., Tager-Flusberg, H., and Nelson, C. A. (2015). Atypical hemispheric specialization for faces in infants at risk for autism spectrum disorder. Autism Res. 8, 187–198. doi: 10.1002/aur.1438
Keehn, B., Wagner, J., Tager-Flusberg, H., and Nelson, C. A. (2013). Functional connectivity in the first year of life in infants at-risk for autism: a preliminary near-infrared spectroscopy study. Front. Hum. Neurosci. 7:444. doi: 10.3389/fnhum.2013.00444
Key, A. P. F., and Stone, W. L. (2012a). Processing of novel and familiar faces in infants at average and high risk for autism. Dev. Cogn. Neurosci. 2, 244–255. doi: 10.1016/j.dcn.2011.12.003
Key, A. P. F., and Stone, W. L. (2012b). Same but different: nine-month-old infants at average and high risk for autism look at the same facial features but process them using different brain mechanisms. Autism Res. 5, 253–266. doi: 10.1002/aur.1231
Key, A. P., Ibanez, L. V., Henderson, H. A., Warren, Z., Messinger, D. S., and Stone, W. L. (2015). Positive affect processing and joint attention in infants at high risk for autism: an exploratory study. J. Autism. Dev. Disord. 45, 4051–4062.
Kolesnik, A., Begum Ali, J., Gliga, T., Guiraud, J., Charman, T., Johnson, M. H., et al. (2019). Increased cortical reactivity to repeated tones at 8 months in infants with later ASD. Transl. Psychiatry 9:46. doi: 10.1038/s41398-019-0393-x
LeBlanc, J. J., and Fagiolini, M. (2011). Autism: a “critical period” disorder? Neural Plast. 2011:921680.
Levin, A. R., Varcin, K. J., O’Leary, H. M., Tager-Flusberg, H., and Nelson, C. A. (2017). EEG power at 3 months in infants at high familial risk for autism. J. Neurodev. Disord. 9:34. doi: 10.1186/s11689-017-9214-9
Levy, S. E., Wolfe, A., Coury, D., Duby, J., Farmer, J., Schor, E., et al. (2020). Screening tools for autism spectrum disorder in primary care: a systematic evidence review. Pediatrics 145, S47–S59. doi: 10.1542/peds.2019-1895H
Lewis, J. D., Evans, A. C., Pruett, J. R., Botteron, K. N., McKinstry, R. C., Zwaigenbaum, L., et al. (2017). The emergence of network inefficiencies in infants with autism spectrum disorder. Biol. Psychiatry 82, 176–185. doi: 10.1016/j.biopsych.2017.03.006
Lewis, J. D., Evans, A. C., Pruett, J. R., Botteron, K., Zwaigenbaum, L., Estes, A., et al. (2014). Network inefficiencies in autism spectrum disorder at 24 months. Transl. Psychiatry 4:e388. doi: 10.1038/tp.2014.24
Li, G., Chen, M.-H., Li, G., Wu, D., Lian, C., Sun, Q., et al. (2019). A longitudinal MRI study of amygdala and hippocampal subfields for infants with risk of autism. Graph. Learn. Med. Imaging 11849, 164–171. doi: 10.1007/978-3-030-35817-4_20
Liu, J., Okada, N. J., Cummings, K. K., Jung, J., Patterson, G., Bookheimer, S. Y., et al. (2020a). Emerging atypicalities in functional connectivity of language-related networks in young infants at high familial risk for ASD. Dev. Cogn. Neurosci. 4:100814. doi: 10.1016/j.dcn.2020.100814
Liu, J., Tsang, T., Ponting, C., Jackson, L., Jeste, S. S., Bookheimer, S. Y., et al. (2020b). Lack of neural evidence for implicit language learning in 9-month-old infants at high risk for autism. Dev. Sci. 24:e13078. doi: 10.1111/desc.13078
Liu, J., Tsang, T., Jackson, L., Ponting, C., Jeste, S. S., Bookheimer, S. Y., et al. (2019). Altered lateralization of dorsal language tracts in 6-week-old infants at risk for autism. Dev. Sci. 22:e12768. doi: 10.1111/desc.12768
Lloyd-Fox, S., Blasi, A., Elwell, C. E., Charman, T., Murphy, D., and Johnson, M. H. (2013). Reduced neural sensitivity to social stimuli in infants at risk for autism. Proc. Biol. Sci. 280:20123026.
Lloyd-Fox, S., Blasi, A., Pasco, G., Gliga, T., Jones, E. J. H., Murphy, D. G. M., et al. (2018). Cortical responses before 6 months of life associate with later autism. Eur. J. Neurosci. 47, 736–749. doi: 10.1111/ejn.13757
Luyster, R. J., Powell, C., Tager-Flusberg, H., and Nelson, C. A. (2014). Neural measures of social attention across the first years of life: characterizing typical development and markers of autism risk. Dev. Cogn. Neurosci. 8, 131–143. doi: 10.1016/j.dcn.2013.09.006
Luyster, R. J., Wagner, J. B., Vogel-Farley, V., Tager-Flusberg, H., et al. (2011). Neural correlates of familiar and unfamiliar face processing in infants at risk for autism spectrum disorders. Brain Topogr. 24, 220–228. doi: 10.1007/s10548-011-0176-z
MacDuffie, K. E., Shen, M. D., Dager, S. R., Styner, M. A., Kim, S. H., Paterson, S., et al. (2020). Sleep onset problems and subcortical development in infants later diagnosed with autism spectrum disorder. Am. J. Psychiatry 177, 518–525. doi: 10.1176/appi.ajp.2019.19060666
Maenner, M. J. (2020). Prevalence of autism spectrum disorder among children aged 8 years — autism and developmental disabilities monitoring network, 11 sites. United States, 2016. MMWR Surveill. Summ. 69, 1–23. doi: 10.15585/mmwr.ss6904a1
Mandell, D. S., Morales, K. H., Xie, M., Lawer, L. J., Stahmer, A. C., and Marcus, S. C. (2010). Age of diagnosis among Medicaid-enrolled children with autism, 2001-2004. Psychiatr Serv. 61, 822–829. doi: 10.1176/ps.2010.61.8.822
Marin, A., Hutman, T., Ponting, C., McDonald, N. M., Carver, L., Baker, E., et al. (2020). Electrophysiological signatures of visual statistical learning in 3-month-old infants at familial and low risk for autism spectrum disorder. Dev. Psychobiol. 62, 858–870. doi: 10.1002/dev.21971
McCleery, J. P., Akshoomoff, N., Dobkins, K. R., and Carver, L. J. (2009). Atypical face versus object processing and hemispheric asymmetries in 10-month-old infants at risk for autism. Biol. Psychiatry 66, 950–957. doi: 10.1016/j.biopsych.2009.07.031
McKinnon, C. J., Eggebrecht, A. T., Todorov, A., Wolff, J. J., Elison, J. T., Adams, C. M., et al. (2019). Restricted and repetitive behavior and brain functional connectivity in infants at risk for developing autism spectrum disorder. Biol. Psychiatry Cogn. Neurosci. Neuroimaging 4, 50–61. doi: 10.1016/j.bpsc.2018.09.008
Nair, A., Jalal, R., Liu, J., Tsang, T., McDonald, N. M., Jackson, L., et al. (2021). Altered thalamocortical connectivity in 6-week-old infants at high familial risk for autism spectrum disorder. Cereb. Cortex 67, 1–23. doi: 10.1093/cercor/bhab078
Nyström, P., Jones, E., Darki, F., Bölte, S., and Falck-Ytter, T. (2020). Atypical topographical organization of global form and motion processing in 5-month-old infants at risk for autism. J. Autism Dev. Disord. 51, 364–370. doi: 10.1007/s10803-020-04523-2
Orekhova, E. V., Elsabbagh, M., Jones, E. J., Dawson, G., Charman, T., Johnson, M. H., et al. (2014). EEG hyper-connectivity in high-risk infants is associated with later autism. J. Neurodev. Disord. 6:40. doi: 10.1186/1866-1955-6-40
Page, J., Lustenberger, C., and Fröhlich, F. (2020). Nonrapid eye movement sleep and risk for autism spectrum disorder in early development: a topographical electroencephalogram pilot study. Brain Behav. 10:e01557. doi: 10.1002/brb3.1557
Pecukonis, M., Perdue, K. L., Wong, J., Tager-Flusberg, H., and Nelson, C. A. (2020). Exploring the relation between brain response to speech at 6-months and language outcomes at 24-months in infants at high and low risk for autism spectrum disorder: a preliminary functional near-infrared spectroscopy study. Dev. Cogn. Neurosci. 47:100897. doi: 10.1016/j.dcn.2020.100897
Petrocchi, S., Levante, A., and Lecciso, F. (2020). Systematic review of level 1 and level 2 screening tools for autism spectrum disorders in toddlers. Brain Sci. 10:180. doi: 10.3390/brainsci10030180
Piccardi, E. S., Begum Ali, J., Jones, E. J. H., Mason, L., Charman, T., Johnson, M. H., et al. (2021). Behavioural and neural markers of tactile sensory processing in infants at elevated likelihood of autism spectrum disorder and/or attention deficit hyperactivity disorder. J. Neurodev. Disord. 13:1. doi: 10.1186/s11689-020-09334-1
Pote, I., Wang, S., Sethna, V., Blasi, A., Daly, E., Kuklisova-Murgasova, M., et al. (2019). Familial risk of autism alters subcortical and cerebellar brain anatomy in infants and predicts the emergence of repetitive behaviors in early childhood. Autism Res.? 12, 614–627. doi: 10.1002/aur.2083
Righi, G., Tierney, A. L., Tager-Flusberg, H., and Nelson, C. A. (2014). Functional connectivity in the first year of life in infants at risk for autism spectrum disorder: an EEG study. PLoS One 9:e105176. doi: 10.1371/journal.pone.0105176
Riva, V., Cantiani, C., Mornati, G., Gallo, M., Villa, L., Mani, E., et al. (2018). Distinct ERP profiles for auditory processing in infants at-risk for autism and language impairment. Sci. Rep. 8:715. doi: 10.1038/s41598-017-19009-y
Riva, V., Marino, C., Piazza, C., Riboldi, E. M., Mornati, G., Molteni, M., et al. (2019). Paternal-but not maternal-autistic traits predict frontal EEG alpha asymmetry in infants with later symptoms of autism. Brain Sci. 9:342. doi: 10.3390/brainsci9120342
Sanchack, K. E., and Thomas, C. A. (2016). Autism spectrum disorder: primary care principles. Am. Fam. Physician 94, 972–979.
Seery, A. M., Vogel-Farley, V., Tager-Flusberg, H., and Nelson, C. A. (2013). Atypical lateralization of ERP response to native and non-native speech in infants at risk for autism spectrum disorder. Dev. Cogn. Neurosci. 5, 10–24. doi: 10.1016/j.dcn.2012.11.007
Shen, M. D., Kim, S. H., McKinstry, R. C., Gu, H., Hazlett, H. C., Nordahl, C. W., et al. (2017). Increased extra-axial cerebrospinal fluid in high-risk infants who later develop autism. Biol. Psychiatry 82, 186–193. doi: 10.1016/j.biopsych.2017.02.1095
Shen, M. D., Nordahl, C. W., Young, G. S., Wootton-Gorges, S. L., Lee, A., Liston, S. E., et al. (2013). Early brain enlargement and elevated extra-axial fluid in infants who develop autism spectrum disorder. Brain 136, 2825–2835. doi: 10.1093/brain/awt166
Shephard, E., Milosavljevic, B., Mason, L., Elsabbagh, M., Tye, C., Gliga, T., et al. (2020). Neural and behavioural indices of face processing in siblings of children with autism spectrum disorder (ASD): a longitudinal study from infancy to mid-childhood. Cortex 127, 162–179of possible axonal overconnectivity in frontal lobes in autism spectrum disorder toddlers. Biol. Psychiatry 79, 676–684. doi: 10.1016/j.cortex.2020.02.008
Solso, S., Xu, R., Proudfoot, J., Hagler, D. J. Jr., Campbell, K., Venkatraman, V., et al. (2016). Diffusion tensor imaging provides evidence of possible axonal overconnectivity in frontal lobes in autism spectrum disorder toddlers. Biol. Psychiatry 79, 676–684. doi: 10.1016/j.biopsych.2015.06.029
Stahl, D., Pickles, A., Elsabbagh, M., Johnson, M. H., and The, B. A. S. I. S., Team. (2012). Novel machine learning methods for ERP analysis: a validation from research on infants at risk for autism. Dev. Neuropsychol. 37, 274–298. doi: 10.1080/87565641.2011.650808
Swanson, M. R., Shen, M. D., Wolff, J. J., Elison, J. T., Emerson, R. W., Styner, M. A., et al. (2017). Subcortical brain and behavior phenotypes differentiate infants with autism versus language delay. Biol. Psychiatry Cogn. Neurosci. Neuroimaging 2, 664–672. doi: 10.1016/j.bpsc.2017.07.007
Tariq, Q., Daniels, J., Schwartz, J. N., Washington, P., Kalantarian, H., and Wall, D. P. (2018). Mobile detection of autism through machine learning on home video: a development and prospective validation study. PLoS Med. 15:e1002705. doi: 10.1371/journal.pmed.1002705
Thabtah, F., and Peebles, D. (2019). Early autism screening: a comprehensive review. Int. J. Environ. Res. Public Health 16:3502. doi: 10.3390/ijerph16183502
Tierney, A. L., Gabard-Durnam, L., Vogel-Farley, V., Tager-Flusberg, H., and Nelson, C. A. (2012). Developmental trajectories of resting EEG power: an endophenotype of autism spectrum disorder. PLoS One 7:e39127. doi: 10.1371/journal.pone.0039127
Towle, P. O., and Patrick, P. A. (2016). Autism spectrum disorder screening instruments for very young children: a systematic review. Autism Res. Treatment 2016:e4624829. doi: 10.1155/2016/4624829
Tran, X. A., McDonald, N., Dickinson, A., Scheffler, A., Frohlich, J., Marin, A., et al. (2021). Functional connectivity during language processing in 3-month-old infants at familial risk for autism spectrum disorder. Eur. J. Neurosci. 53, 1621–1637. doi: 10.1111/ejn.15005
Wilkinson, C. L., Gabard-Durnam, L. J., Kapur, K., Tager-Flusberg, H., Levin, A. R., and Nelson, C. A. (2020). Use of longitudinal EEG measures in estimating language development in infants with and without familial risk for autism spectrum disorder. Neurobiol. Lang. 1, 33–53. doi: 10.1162/nol_a_00002
Wilkinson, C. L., Levin, A. R., Gabard-Durnam, L. J., Tager-Flusberg, H., and Nelson, C. A. (2019). Reduced frontal gamma power at 24 months is associated with better expressive language in toddlers at risk for autism. Autism Res.? 12, 1211–1224. doi: 10.1002/aur.2131
Wolff, J. J., Gerig, G., Lewis, J. D., Soda, T., Styner, M. A., Vachet, C., et al. (2015). Altered corpus callosum morphology associated with autism over the first 2 years of life. Brain 138, 2046–2058. doi: 10.1093/brain/awv118
Wolff, J. J., Gu, H., Gerig, G., Elison, J. T., Styner, M., Gouttard, S., et al. (2012). Differences in White Matter Fiber Tract Development present From 6 to 24 months in infants with autism. Am. J. Pyschiatry 169, 589–600. doi: 10.1176/appi.ajp.2011.11091447
Wolff, J. J., Swanson, M. R., Elison, J. T., Gerig, G., Pruett, J. R., Styner, M. A., et al. (2017). Neural circuitry at age 6 months associated with later repetitive behavior and sensory responsiveness in autism. Mol. Autism 8:8. doi: 10.1186/s13229-017-0126-z
Keywords: autism spectrum disorder (ASD), early screening, magnetic resonance imaging (MRI), electroencephalogram (EEG), functional near-infrared spectroscopy (fNIRS)
Citation: Clairmont C, Wang J, Tariq S, Sherman HT, Zhao M and Kong X-J (2022) The Value of Brain Imaging and Electrophysiological Testing for Early Screening of Autism Spectrum Disorder: A Systematic Review. Front. Neurosci. 15:812946. doi: 10.3389/fnins.2021.812946
Received: 10 November 2021; Accepted: 09 December 2021;
Published: 03 February 2022.
Edited by:
Roberto Esposito, ASUR Marche, ItalyCopyright © 2022 Clairmont, Wang, Tariq, Sherman, Zhao and Kong. This is an open-access article distributed under the terms of the Creative Commons Attribution License (CC BY). The use, distribution or reproduction in other forums is permitted, provided the original author(s) and the copyright owner(s) are credited and that the original publication in this journal is cited, in accordance with accepted academic practice. No use, distribution or reproduction is permitted which does not comply with these terms.
*Correspondence: Xue-Jun Kong, xkong1@mgh.harvard.edu
†These authors have contributed equally to this work and share first authorship