- 1The Second School of Clinical Medicine, Southern Medical University, Guangzhou, China
- 2Department of Medical Imaging, Guangdong Second Provincial General Hospital, Guangzhou, China
- 3Guangdong Traditional Medical and Sports Injury Rehabilitation Research Institute, Guangdong Second Provincial General Hospital, Guangzhou, China
- 4Department of Medical Imaging, Zhuhai People’s Hospital, Zhuhai, China
Recent studies have shown that the human gut microbiota (GM) plays a critical role in brain function and behavior via the complex microbiome–gut–brain axis. However, knowledge about the underlying relationship between the GM and changes in brain function in patients with chronic insomnia (CI) is still very limited. In this prospective study, 31 CI patients and 30 healthy controls were recruited. Resting-state functional magnetic resonance imaging scans were performed and brain functional alterations in CI patients were evaluated using the regional homogeneity (ReHo) method. We collected fecal samples of CI patients and used 16S rDNA amplicon sequencing to assess the relative abundance (RA) and alpha diversity of the GM. We also performed extensive sleep, mood, and cognitive assessments. Then, we tested for potential associations between the GM profile, ReHo alterations, and neuropsychological changes in CI patients. Our results showed associations between the RA of Lactobacilli, ReHo values in the left fusiform gyrus, and depression scores in CI patients. We also found some bacterial genera related to ReHo values of the right triangular inferior frontal gyrus. In addition, the RA of genus Coprobacter was correlated with ReHo values of the left angular gyrus and with specific cognitive performance. These findings revealed complex relationships between GM, brain function, and behavior in patients with CI.
Introduction
Chronic insomnia (CI) is one of the most common sleep disorders worldwide, with an estimated prevalence of 10% in the adult population (Morin and Benca, 2012). The condition is defined as subjective complaints of difficulty in initiating or maintaining sleep, or early morning awakening with associated daytime impairments, persisting over a period of 3 months (American Psychiatric Association [APA], 2014; Darien, 2014). CI can lead to mental symptoms such as depression (Baglioni et al., 2011) and can cause daytime cognitive impairments (Fortier-Brochu et al., 2012) such as reduced attention, decreased memory, and executive dysfunction. In the US alone, the cost of treating insomnia is estimated to exceed $20 billion, with total insomnia-related costs exceeding $100 billion per year, which causes a pronounced socioeconomic burden (Wickwire et al., 2016). Previous studies have proposed several models to explain the etiology and pathophysiology of insomnia, including hyperarousal, genetic vulnerability, and specific molecular and cellular mechanisms, but there is still no universally accepted model (Levenson et al., 2015). It is therefore important to investigate potential neuropathological mechanisms in CI and find a novel adjuvant therapeutic method.
In recent years, interest has surged regarding the potential role of the gut microbiota (GM) in the complex microbiome–gut–brain axis (MGBA). Within this axis, GM can modulate emotional behavior and cognitive function through several mechanistic pathways including the immunoregulatory pathway, the neuroendocrine pathway, the vagus nerve pathway, and activation of the hypothalamic–pituitary–adrenal (HPA) axis (Cryan and Dinan, 2012; Foster et al., 2016; Rogers et al., 2016; Sharon et al., 2016). In fact, GM has recently been associated with a series of neurodegenerative brain diseases including Alzheimer’s disease, Parkinson’s disease and amyotrophic lateral sclerosis (Spielman et al., 2018). Furthermore, in the context of neurodegenerative processes, orexin (a peptide also involved in the regulation of the sleep-wake cycle and mood, in eating behavior, in energy homeostasis and in cognitive processes) seems to play an important role, with mechanisms which might involve GM (Mogavero et al., 2021). Recently, several preclinical and clinical studies have identified a close potential relationship between gastrointestinal microbial perturbations and insomnia (Liu et al., 2019; Li Y. et al., 2020; Wang et al., 2020). For example, a study combining high-throughput sequencing and bioinformatic analysis found that the structural composition, signaling pathways, and metabolic function of the GM in insomnia patients were significantly perturbed compared with those of healthy controls (HCs). Additionally, the relative abundance (RA) of the GM at the phylum level was significantly correlated with clinical sleep parameters (Liu et al., 2019). Moreover, one recent study (Li Y. et al., 2020) found that the RA of signature bacteria such as Faecalibacterium and Blautia in CI patients was significantly correlated with self-reported sleep quality and inflammatory cytokines. The evidence suggests that the GM may be an integral contributor to the pathogenesis of insomnia. Despite these findings, there is still a significant gap in establishing a correlation between the GM and the brain in the condition of CI. However, combining advanced neuroimaging techniques with analysis of the GM has tremendous potential to enhance understanding of this connection (De Santis et al., 2019).
Emerging evidence supports the connection between structural or functional aberrance in the brain and the GM profile. For example, using the fractional amplitude of low-frequency fluctuations (fALFF) method, Liu et al. (2021) found a potential relationship between the RA of certain bacteria, changes in fALFF values in specific brain regions, and cognitive function in patients with amnestic mild cognitive impairment. Wang et al. (2019) found that patients with end-stage renal disease displayed complex associations between GM alterations, systemic inflammation, disrupted topology architecture of the default mode network (DMN), and cognitive impairment. In addition, one recent study (Li et al., 2021) combining voxel-based morphometry analysis and regional homogeneity (ReHo) analysis found that the alpha diversity of the GM was correlated with the values of both gray matter volume and ReHo in patients with schizophrenia. Recent clinical studies have revealed the potential association between the GM and insomnia. However, few studies have incorporated neuroimaging techniques, and the potential relationship between the GM and changes in brain function with CI remains unclear.
Advanced resting-state functional magnetic resonance imaging (rs-fMRI) analysis has become a widely used technique for exploring abnormal functional changes of the brain. The combination of rs-fMRI analysis and GM analysis can explore correlations between GM and brain activity in CI and further construct a GM-brain function-behavior model. The ReHo method was found to be reliable and effective in evaluating the level of spontaneous regional activity across the whole brain (Zang et al., 2004). The method has been successfully applied in various neuropsychological disorders including insomnia due to its favorable test-retest reliability and ease of data processing (Kiviniemi, 2008). Several previous studies (Dai et al., 2014; Wang et al., 2016) have found that CI patients showed abnormal ReHo indexes in several brain areas such as the insula, cingulate gyrus, and fusiform gyrus. These altered ReHo values were correlated with sleep quality and psychological scores, which suggests that abnormal ReHo in specific areas may serve as an early biomarker for brain activity changes in CI patients. However, research on the relationship between regional brain function activity and GM composition in the context of CI is still in its infancy.
Our study is the first to explore the relationship between the ReHo alterations and GM composition in CI patients, which may provide objective neuroimaging evidence for a potential neuropathological mechanism of the MGBA involved in CI. In this study, we hypothesized that regional neural activity changes of the brain are associated with the GM profile of CI patients. We aimed to leverage ReHo analysis based on rs-fMRI to investigate brain functional alterations in CI patients and further reveal the underlying relationships between GM profile, ReHo alterations, and neuropsychological changes.
Materials and Methods
Participants
A total of 61 subjects were recruited, including 31 CI patients from the Department of Neurology of Guangdong Second Provincial General Hospital, and 30 age-, gender-, and education level-matched HCs recruited from the local community by means of advertisements. All participants were right-handed according to the Edinburgh handedness inventory (Oldfield, 1971) and aged 18–60 years. This prospective study was approved by the Ethics Committee of the Guangdong Second Provincial General Hospital. Informed written consent was obtained from all participants or their legally authorized caregivers.
The diagnosis of CI was determined by two neurologists with 15 years of experience each, based on criteria of the Diagnostic and Statistical Manual of Mental Disorders, version 5 (DSM-V) (American Psychiatric Association [APA], 2014). The neurologists used a semi-standardized psychiatric and sleep-related interview to screen patients. Subjects chosen met the following criteria: (a) self-reported difficulty falling asleep or maintaining sleep, or early morning awakening; (b) difficulty sleeping at least three times per week over a period of 3 months; (c) at least one related daytime impairment (e.g., fatigue, mood disturbance, or impaired cognitive performance); (d) no other sleep disorders (e.g., obstructive sleep apnea or sleep-related movement disorder), serious organic diseases, or mental disorders such as depression or generalized anxiety determined by a semi-standardized psychiatric interview conducted by an experienced clinical psychiatrist with 5 years of experience in clinical psychiatry. In addition, all patients chosen were free of any psychoactive medications for at least 2 weeks prior to and during the study to eliminate drug effects.
CI patients were excluded from the study if they (a) had an abnormal signal in any region of the brain as verified by conventional T1- or T2-weighted fluid-attenuated inversion recovery (FLAIR) MRI; (b) had a history of severe malnutrition, infection, digestive problems such as obvious diarrhea, constipation, irritable bowel syndrome, or inflammatory bowel disease; (c) had a history of drug abuse, alcohol addiction, or regular smoking; (d) had a diet including spicy stimulating food, or took antibiotics, probiotics, prebiotics, or cathartic drugs within 2 months prior to fecal sample collection; (e) were pregnant, nursing, or menstruating females. Inclusion criteria for the HC subjects were as follows: (a) good sleep quality, with an Insomnia Severity Index (ISI) score of <7; (b) no brain lesions or prior substantial head trauma as verified by conventional T1- or T2-weighted FLAIR MRI; (c) no history of psychiatric or neurological diseases; (d) not pregnant, nursing, or menstruating if female.
Assessment of Sleep Quality, Mental Status, and Cognitive Ability
Prior to MRI scanning, each participant was asked to undergo a battery of standardized neuropsychological assessments. These tests included the Pittsburgh Sleep Quality Index (PSQI) and the Insomnia Severity Index (ISI) to evaluate sleep quality, the Self-Rating Depression Scale (SDS) and Self-Rating Anxiety Scale (SAS) to evaluate mental status, and the Montreal Cognitive Assessment Scale (MoCA) (Nasreddine et al., 2005) to measure global cognitive function. Information processing speed was evaluated using the Digit Symbol Substitution Test (DSST), and working memory was determined by the Digit Span Test (DST). Executive function and attention were evaluated with Trail-Making Test (TMT) A and B. The demographic and neuropsychological details for the included subjects are shown in Table 1.
Fecal Sample Collection and Gut Microbiota Analysis
Fecal samples of CI patients were collected in sterile containers and immediately stored in a −80°C freezer prior to further processing. First, microbial genome DNA samples were extracted from stool samples using the QIAamp DNA Mini Kit (QIAGEN, Hilden, Germany) according to the manufacturer’s instructions. After evaluating DNA quality and quantity, a specific hypervariable region of 16s rDNA was selected for PCR amplification based on the MiSeq Illumina platform and following the Illumina protocol. Next, the raw reads were filtered to remove adaptors and low-quality and ambiguous bases. Based on the overlapping relationships between the reads, the paired reads were assembled into a sequence to obtain tags of the hypervariable region, using the Fast Length Adjustment of Short reads program (FLASH, v1.2.11) (Magoč and Salzberg, 2011). Reads without overlapping relationships were removed. Tags with primers for specific fragment amplification were processed using CUTADAPT (Martin, 2011), and chimeras in the tags were removed by the UCHIME method in VSEARCH (v2.3.4) (Rognes et al., 2016). Remaining optimized spliced sequences were stepwise clustered into operational taxonomic units (OTUs) at a 97% similarity threshold using the UPARSE algorithm. These OTU representative sequences were mapped to the database [Silva: v128 (Quast et al., 2013); Greengene: Release13.8 (DeSantis et al., 2006); UNITE: Release 5.0 (Abarenkov et al., 2010)] for species annotation and classification using the Ribosomal Database Project (RDP) Classifier (v2.2) (Wang et al., 2007) with the confidence threshold set to 0.8. The RA of OTU indicates the species richness of the samples. Alpha diversity measures including the Chao index, Ace index, Shannon index, and Simpson index were also calculated using MOTHUR (v1.31.2) (Schloss et al., 2009).
Magnetic Resonance Imaging Data Acquisition
All participants were scanned using a 3.0-T MRI scanner (Ingenia; Philips, Best, Netherlands) at the Department of Medical Imaging at Guangdong Second Provincial General Hospital. Each subject wore ear plugs to minimize scanner noise and was positioned supine with the head snugly secured by a belt and foam pads to reduce head movements. During rs-fMRI data acquisition, all participants were instructed to lie quietly with their eyes closed but to try not to fall asleep or think of anything specific while inside the scanner. The rs-fMRI datasets were obtained using a gradient-echo planar imaging (EPI) sequence with the following parameters: repetition time (TR) = 2,000 ms; echo time (TE) = 30 ms; matrix = 64 × 61; field-of-view (FOV) = 224 mm × 224 mm; flip angle (FA) = 90°; slice thickness = 3.5 mm with a 1.0 mm gap; interleaved scanning. For each participant, 33 transverse slices covering the whole brain at all 240 volumes were acquired over approximately 8 min. In addition, high-resolution anatomical images were acquired using a T1-weighted 3D gradient-echo sequence with the following parameters: 185 axial slices, TR = 7.9 ms, TE = 3.6 ms, FA = 8°, slice thickness = 1.0 mm, no gap, matrix = 256 × 256, and FOV = 256 mm × 256 mm. We also obtained the T1-weighted images and T2-FLAIR images to detect brain lesions. Subsequently, some questions were asked to evaluate the cooperation status of the subjects.
Data Processing and Regional Homogeneity Calculations
The rs-fMRI datasets were preprocessed using DPARSF 4.3 Advanced Edition1. The first 10 time points for each participant were discarded to allow the signal to reach equilibrium and the subjects to adapt to the scanning environment. The remaining rs-fMRI datasets were then corrected for intra-volume acquisition time delay and inter-volume head motion. To minimize the influence of head motion, data from all participants with head motion >1.0 mm in any direction or 1.0° of any angular dimension and volume-to-volume mean frame-wise displacement (FD) > 2.0 mm (Power et al., 2015) were excluded. Individual T1 structural images were segmented (white matter, gray matter, and cerebrospinal fluid) using the Segmentation toolbox, and the DARTEL toolbox was used to create a study-specific template for accurate normalization. Subsequently, the functional images were co-registered to the structural images and normalized into standard Montreal Neurological Institute (MNI) space with an isotropic voxel size 3 mm × 3 mm × 3 mm. Several covariates and their temporal derivatives were then regressed out from the time course of each voxel, including the brain’s global mean, white matter, and cerebrospinal fluid signals, as well as the Friston 24 parameters of head motion (Friston et al., 1996). To reduce the effects of low-frequency drift and high-frequency noise, the data were further detrended and temporally band-pass filtered (0.01–0.1 Hz).
The ReHo calculation procedure was the same as those reported in previous studies (Zang et al., 2004). Briefly, the ReHo index was estimated by calculating Kendall’s coefficient of concordance (KCC) of the time series of a given voxel with those of its 26 nearest neighbors in voxel-wise way. The ReHo index indicated the degree of regional temporal synchronization of the cluster with a given voxel in the center. For optimization, each ReHo map was divided by its averaged KCC value for the whole brain. Then, the standardized ReHo map was spatially smoothed with a 12-mm full-width at half-maximum Gaussian kernel to reduce noise and residual differences in gyral anatomy.
Statistical Analysis
Statistical analyses were performed using the SPSS software package version 25.0 (SPSS Inc., Chicago, IL, United States). A two-sample t-test was used to compare the continuous variables and an χ2 test was used to compare the qualitative variables of gender. Before the analyses, the Shapiro–Wilk test was used to test for normality of quantitative data. Normally distributed data were presented as mean ± standard deviation and then assessed by independent two-sample t-test. Mann–Whitney U tests were used to analyze the data with non-normal distribution, which were reported as median and inter-quartile range.
To explore the ReHo differences between CI patients and HC subjects, a two-sample t-test was performed on the individually smoothed ReHo maps, taking age, gender, education level, body mass index (BMI), and mean FD as covariates. Benjamini–Hochberg false discovery rate (FDR) correction (Benjamini and Hochberg, 1995) was performed to address the multiple comparisons at a significance level of 0.05 (p < 0.05). Furthermore, the mean ReHo values of significant areas were extracted independently using the Resting-State fMRI Data Analysis Toolkit2. To further investigate the relationship among the factors derived from the gut microbiota, brain function and clinical variables, we selected several GM features including alpha diversity and specific gut taxa such as Bacilli, Lactobacillus, Erysipelatoclostridium, Tyzzerella 4, Ruminococcus gnavus group, and Coprobacter which have been reported to be associated with CI and other psychiatric disorders (Poroyko et al., 2016; Li et al., 2018; Aizawa et al., 2019; Ma et al., 2019; Vindegaard et al., 2020). Correlations between GM parameters (RA and alpha diversity), neuropsychological assessment scores, and ReHo values in significantly different areas were performed using partial correlation analysis, controlling for age, gender, education level, BMI, and mean FD. Bonferroni correction was carried out in correlation analyses for multiple comparisons. Clustering analysis based on correlation coefficient and graphic representation were performed using the pheatmap and ggplot2 packages of RStudio software (version 1.4.11063). All statistical tests were two-sided, and differences in values of p < 0.05 were considered statistically significant.
Results
Demographic and Neuropsychological Results
There were no significant inter-group differences in terms of age, gender, education level or BMI (all p > 0.05). As expected, in neuropsychological assessments, there were significant differences (p < 0.05) between CI patients and HC subjects in all of the sleep measures (PSQI and ISI), mood measures (SAS and SDS), and cognitive measures (TMTA, TMTB, DSST, DST, and MoCA). Demographic and neuropsychological assessment results are shown in Table 1. Notably, CI patients were found to score distinctly lower in each mental and cognitive assessment than HC subjects (p < 0.001).
Altered Regional Homogeneity in CI Patients
Compared to the HC group, the data showed that CI patients had significantly greater ReHo values in the left fusiform gyrus (LFG). Furthermore, we detected decreased ReHo values in the left angular gyrus (LAG) and the right triangular part of inferior frontal gyrus (IFG) that extended to the right insula (Table 2 and Figure 1) in CI patients when compared with the HC group.
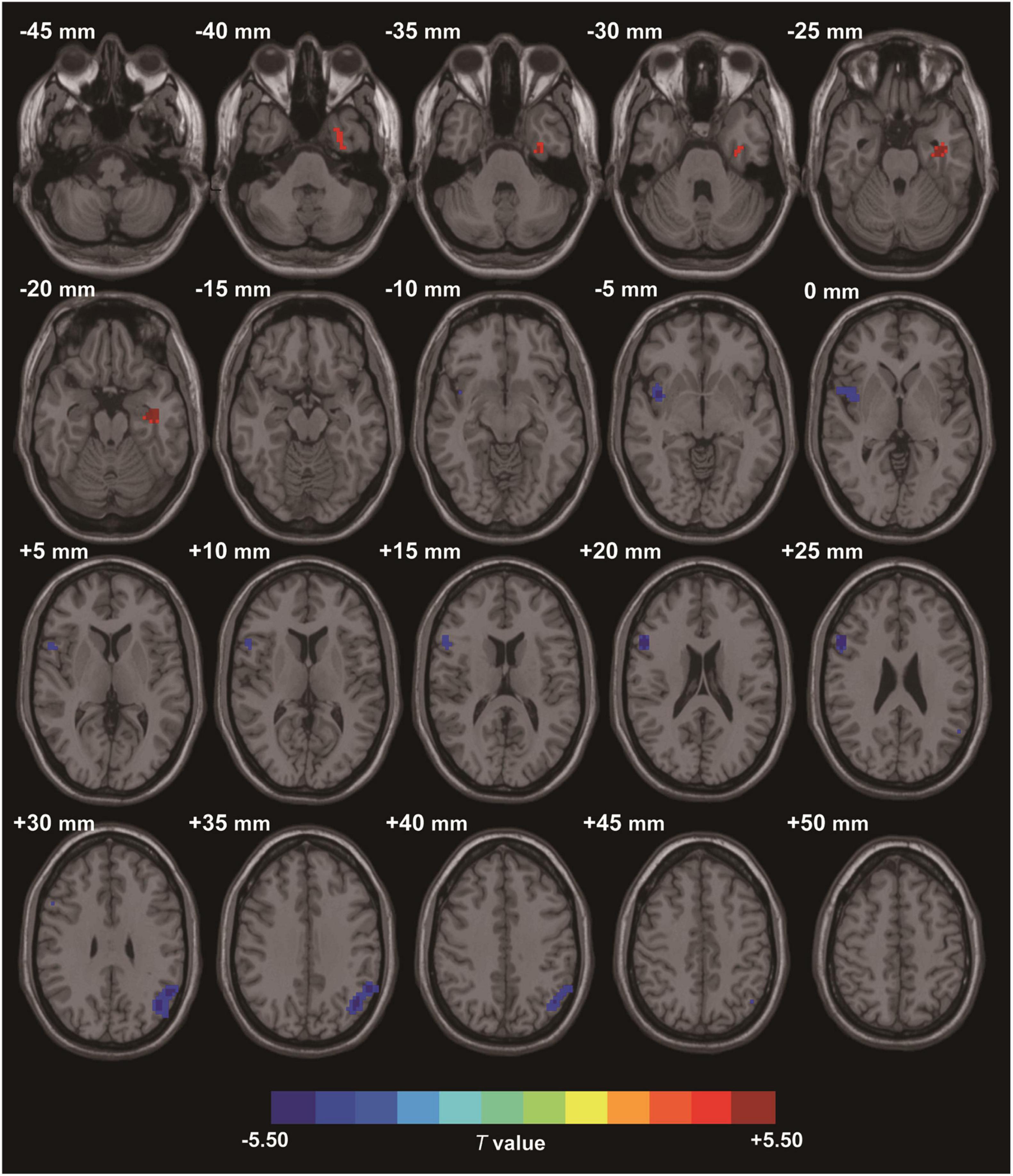
Figure 1. Clusters showing statistically significant different ReHo values in patients with CI compared with HCs (p < 0.05, FDR corrected). CI patients showed increased ReHo values in the left fusiform gyrus (warm colors), as well as decreased ReHo values in the right triangular inferior frontal gyrus and left angular gyrus (cold colors). AT- score bar is shown below. Red and green denote increases and decreases in ReHo, respectively.
Associations Between Gut Microbiome, Regional Homogeneity Values in Abnormal Regions, and Neuropsychological Assessments
We subsequently explored the potential relationships between GM parameters (RA and alpha diversity), ReHo values with group differences, and neuropsychological test scores in CI patients. The heat map of GM, ReHo measures, and neuropsychological assessments was constructed based on significant correlation coefficients (p < 0.05). Analysis revealed a variety of significant correlations between ReHo alterations and GM composition (Figure 2A). For instance, we observed that increased ReHo values of the LFG were negatively correlated with RA of the class Bacilli (r = −0.416, p = 0.020), order Lactobacillales (r = −0.408, p = 0.023), family Lactobacillaceae (r = −0.549, p = 0.001), genus Lactobacillus (r = −0.549, p = 0.001). After the strict Bonferroni correction, family Lactobacillaceae (r = −0.549, p = 0.004) and genus Lactobacillus (r = −0.549, p = 0.004) still showed significant negative correlations with ReHo values in the LFG (Supplementary Figure 1A). We also found that the decreased ReHo values of the right triangular part of IFG were negatively correlated with RA of the genus Erysipelatoclostridium (r = −0.449, p = 0.011), genus Tyzzerella 4 (r = −0.409, p = 0.022), and genus Ruminococcus gnavus group (r = −0.374, p = 0.038). Notably, the correlation between the genus Erysipelatoclostridium (r = −0.449, p = 0.034) and ReHo values of the right triangular IFG was still significant after the strict Bonferroni correction (Supplementary Figure 1B). In addition, the decreased ReHo values of the LAG were positively correlated with RA of the genus Coprobacter (r = 0.390, p = 0.030). There was no significant correlation between ReHo values in abnormal regions and alpha diversity index of the GM.
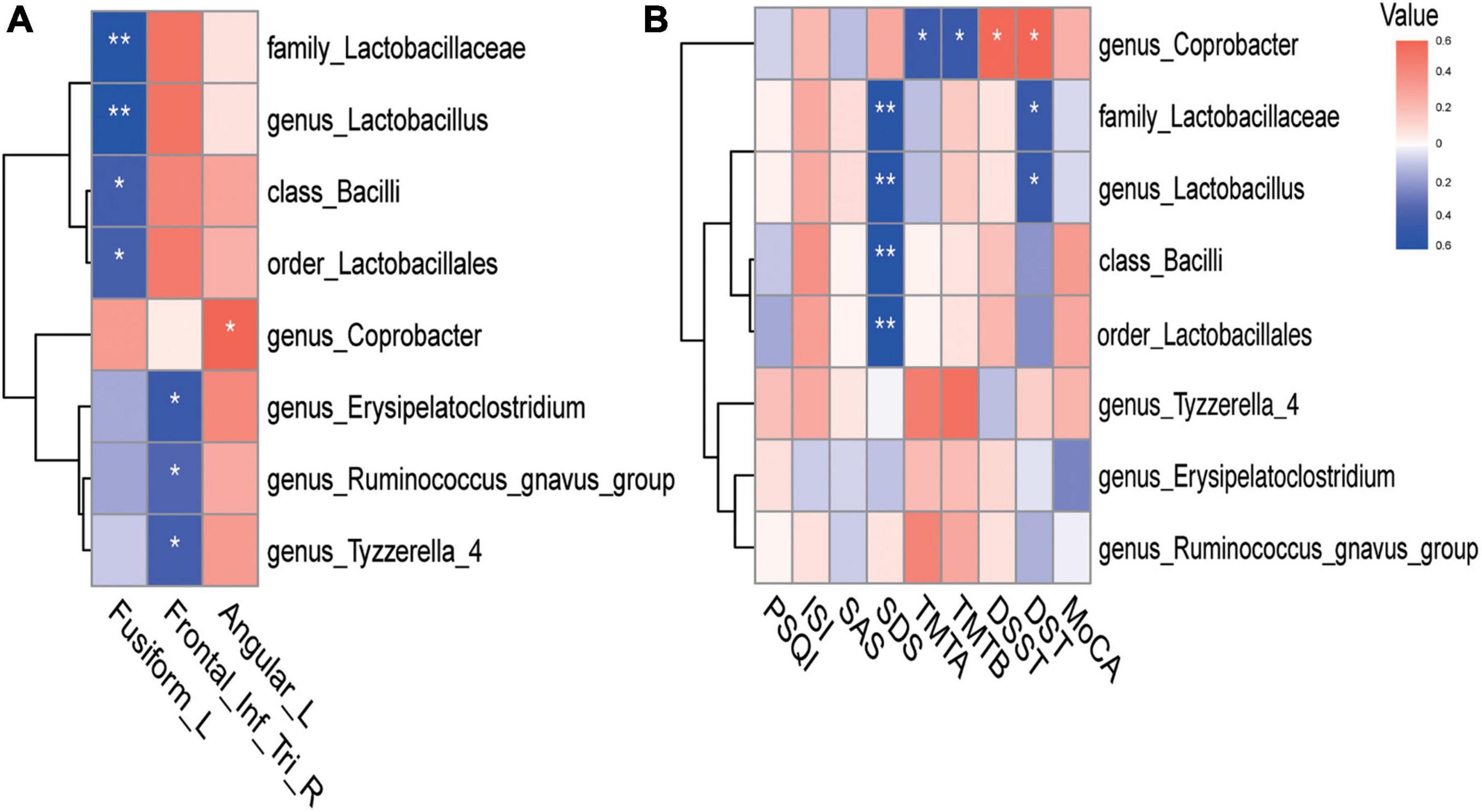
Figure 2. Heatmap of the associations between GM and ReHo values in abnormal regions and neuropsychological assessments in CI patients. (A) The associations between GM and ReHo values in abnormal regions. (B) The associations between GM and neuropsychological assessments. The Heat map shows the correlation coefficients between these variables, where the intensity of the color [positive (blue) or negative (red)] is proportional to the strength of the association. *p < 0.05, **p < 0.01. GM, gut microbiota; ReHo, regional homogeneity.
For microbiomes showing correlations with ReHo values, correlation analyses were further performed to evaluate their associations with clinical variables. Figure 2B illustrates that changes in emotion and cognition of CI patients showed several correlations with specific microbial traits. For example, depression scores (SDS) showed strong negative correlations with RA of the class Bacilli (r = −0.500, p = 0.017), order Lactobacillales (r = −0.495, p = 0.019), family Lactobacillaceae (r = −0.484, p = 0.023), and genus Lactobacillus (r = −0.484, p = 0.023), after Bonferroni correction (Supplementary Figures 2A–D). Poor cognitive scores in TMTA (r = −0.406, p = 0.023) and TMTB (r = −0.410, p = 0.022) tests showed strong negative correlations with RA of the genus Coprobacter. Better cognitive performance in DSST (r = 0.370, p = 0.040) and DST (r = 0.384, p = 0.033) tests were positively associated with RA of the genus Coprobacter. There were no significant associations between RA of the genus Coprobacter and these cognitive assessments after the Bonferroni correction.
In addition, for correlation analyses between ReHo values and neuropsychological assessments in CI patients, we found that ReHo values for the LFG showed a significant positive correlation with SDS scores (r = 0.639, p = 0.000) (Figure 3A). Decreased ReHo values of the right triangular part of the IFG were positively correlated with MoCA scores (r = 0.408, p = 0.023) (Figure 3B). There were positive correlations between decreased ReHo values of the LAG and DSST scores (r = 0.413, p = 0.032), and a negative correlation between TMTB scores (r = −0.388, p = 0.046) and ReHo values in the same area (Figure 3C). There were no significant correlations between ReHo values in these abnormal regions and sleep quality parameters (PSQI and ISI).
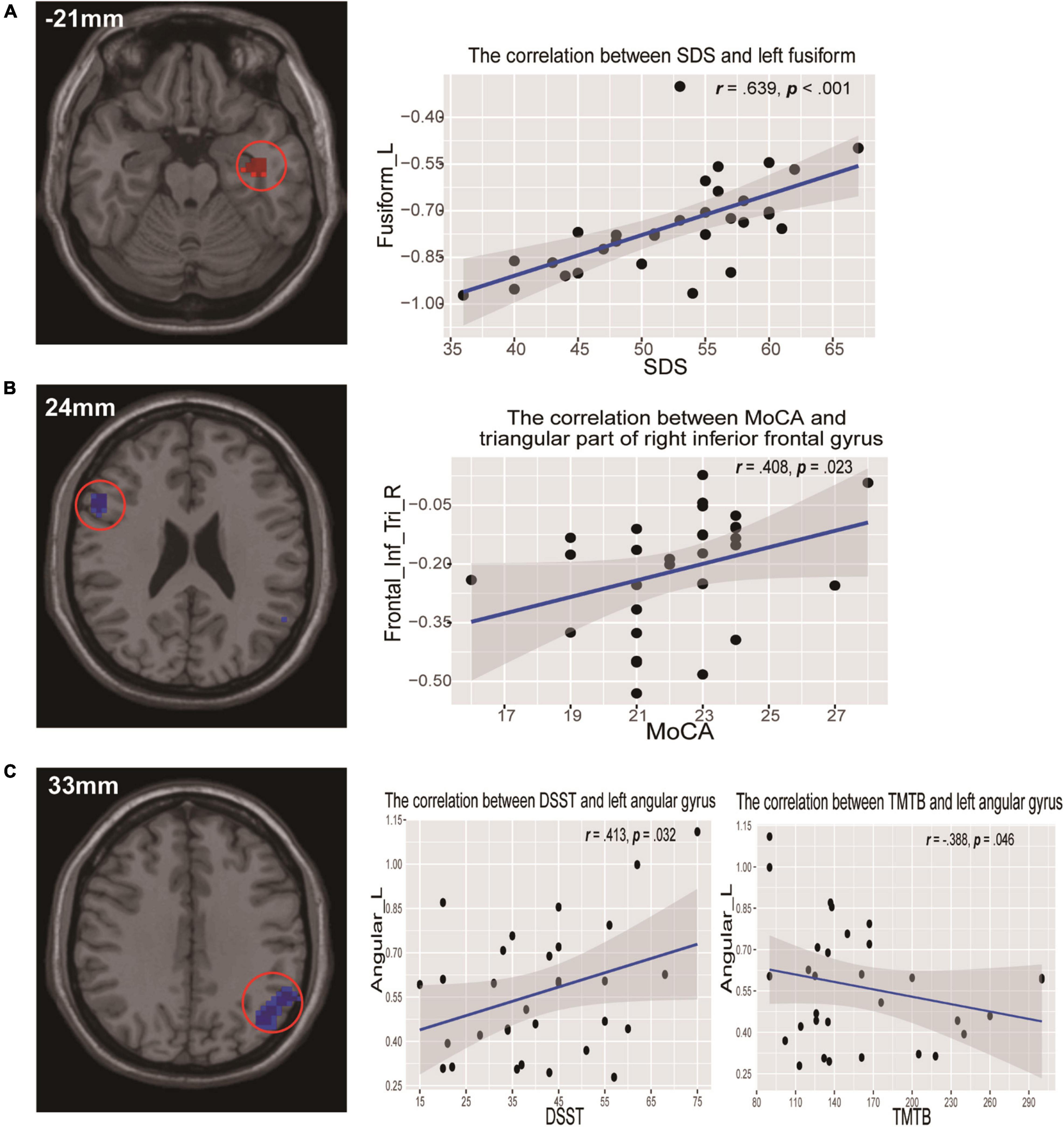
Figure 3. Scatterplots show the relationships between ReHo values and neuropsychological assessments in participants with CI. (A) ReHo values of the left fusiform gyrus were significantly positively correlated with SDS scores. (B) ReHo values of the right triangular inferior frontal gyrus were positively correlated with MoCA scores. (C) ReHo values of the left angular gyrus were positively correlated with DSST scores but negatively correlated with TMTB scores. DSST, Digit Symbol Substitution test; MoCA, Montreal Cognitive Assessment; ReHo, regional homogeneity; SDS, self-rating depression scale; TMTB, Trail Making Test B.
Discussion
To the best of our knowledge, this is the first study to explore whether resting-state regional functional homogeneity modulated by CI might be associated with microbial composition in patients with CI. The results revealed that CI patients revealed complex associations between the RA of specific gut taxa, abnormal regional neuronal activity (ReHo values), and corresponding neuropsychological changes. In particular, the data showed a correlation between the RA of Lactobacilli, ReHo values in the LFG, and SDS scores in CI patients. We also identified a relationship between some bacterial genera and the ReHo values of the right triangular IFG. In addition, the RA of genus Coprobacter was correlated with ReHo values of the LAG, as well as with specific cognitive performance. These findings may provide neuroimaging evidence to support the association between the GM and functional abnormalities of the brain in CI.
First, our exploratory analyses observed the relationships between Lactobacilli RA at levels from order to genus, ReHo values of the LFG, and SDS scores in CI patients. Our MRI analysis found increased ReHo values of the LFG in CI patients. The fusiform gyrus is known to be mainly involved in visual processing, face perception, and emotional perception processing, especially in emotional expression recognition (Groenewold et al., 2013; Bae et al., 2019; Li et al., 2019). Our findings were consistent with previous rs-fMRI studies in patients with insomnia (Dai et al., 2014; Li et al., 2016; Wang et al., 2016), which also found regional functional abnormalities in the fusiform region. In previous studies on depression, functional abnormality in the fusiform was also detected and positively correlated with the degree of depression (Wu et al., 2011; Wang et al., 2012; Gong et al., 2020). In the present study, the ReHo values in the LFG were positively associated with SDS scores, which further suggested that this area of the brain may play an important role in emotional regulation in patients with CI. Most interesting were the significant negative associations between Lactobacilli RA and ReHo values in the LFG in our study. Lactobacillus, as a probiotic microorganism, has been reported to confer a beneficial impact on physical and mental health. Many clinical trials have found that probiotic interventions could significantly ameliorate anxiety and depression symptoms in human subjects (Messaoudi et al., 2011; Akkasheh et al., 2016; Pinto-Sanchez et al., 2017). Our findings of a negative association between depression scores in CI and Lactobacilli RA further support the idea that Lactobacilli may play a role in regulating mood. Moreover, the RA of Lactobacilli was negatively associated with abnormal functional changes in the LFG, which may provide further insights on the relationship between Lactobacilli and brain function in patients with CI. Of interest, in previous studies, associations were also found between the Lactobacillus and functional connectivity changes within the resting-state brain network that included affective regions (Tillisch et al., 2013). However, the exact mechanism underlying the observed correlations is still unclear. It has been reported that Lactobacillus may synthesize and release gamma amino butyric acid (GABA) (Wu et al., 2017; Wang et al., 2018) and may also attenuate the response of the HPA axis to stress (Sudo et al., 2004; Bravo et al., 2011). It is speculated that dysregulation of GABA and the HPA axis could play a significant role in the pathogenesis of insomnia (Levenson et al., 2015) or depression (Verduijn et al., 2015). We speculated that these neuroendocrine pathways might mediate the influence of Lactobacilli on the neurological activity of emotion processing in the fusiform area of patients with CI, similar to how the complex MGBA occurs (Cryan and Dinan, 2012). Accordingly, our findings provide preliminary evidence of neural correlations for probiotic intervention as a possible new complementary treatment to improve sleep and mood in CI patients.
We also identified significant negative correlations between some bacterial genera in the gut and ReHo values of the right triangular IFG. The right triangular IFG is a part of the prefrontal cortex (Zhao et al., 2016), which acts as a modulator of higher-order cognitive processes including attention, working memory, and executive function (Lara and Wallis, 2015). This area has frequently been found to be abnormal in patients with CI (Altena et al., 2008; Dai et al., 2016; Pang et al., 2018). In our study, CI patients exhibited decreased ReHo in the right triangular IFG, and the ReHo values were positively correlated with cognitive function (MoCA score), which was lower in CI patients than in HC subjects. Thus, we supposed that chronic lack of sleep can impair the function of the right triangular IFG, which may contribute to cognitive deficits in CI patients. In addition, the association between the RA of some gut bacteria and abnormal ReHo values of the IFG observed in our study may suggest a potential communication mechanism between the GM and brain function in CI patients. Other studies have also detected abnormalities in the prefrontal cortex that were associated with the GM profile. For example, Cai et al. (2021) found significant positive correlations between intra-network connectivity in the lateral prefrontal cortex and GM diversity. Genus Erysipelatoclostridium, a taxon known to be associated with inflammation (Xiao et al., 2021), has positive associations with lipopolysaccharide (Li L. L. et al., 2020), an endotoxin that can elicit a strong immune response resulting in central nervous system inflammation (Galland, 2014). Genus Ruminococcus gnavus, populations of which bloom in various inflammatory diseases (Henke et al., 2019), has been reported to synthesize and secrete a polysaccharide that induces the production of inflammatory cytokines. Similarly, Tyzzerella_4 has been reported as the potential pathogenic bacteria genera (Fomenky et al., 2018), and its RA level was found to be profoundly increased in chronic inflammatory bowel disease (Qiu et al., 2017; Olaisen et al., 2021). In our results, these pro-inflammatory bacteria were negatively associated with abnormal brain function in CI patients. Interestingly, previous research suggested that systematic inflammation was associated with insomnia symptoms and could be a pathway linking gut microbiome with insomnia (Patel et al., 2009; Jackson et al., 2015; Fernandez-Mendoza et al., 2017). For example, one recent study Li Y. et al. (2020) found that the signature bacteria in CI patients were significantly correlated with insomnia symptoms and elevated levels of inflammatory cytokines. Therefore, we speculated that there was a possible association between pro-inflammatory bacteria and abnormal brain functional activity in CI patients, and that inflammatory pathways may play an important role in this association.
In addition, we discovered associations among decreased ReHo values of the LAG, the RA of genus Coprobacter in the gut, and specific cognitive performance in CI patients. The angular gyrus has been identified as a key parietal node of the DMN (Bluhm et al., 2008) and is involved in a variety of cognitive functions including language and semantic processing, recognition and attention, and episodic memory retrieval (Thakral et al., 2017). Previous resting-state brain function studies have suggested that DMN impairment may be a hallmark of insomnia (Nie et al., 2015; Marques et al., 2017). In addition, we observed that ReHo values of the LAG in CI patients were positively correlated with better scores on DSST and DST tests, which measure the cognitive domains of information processing, psychomotor speed, and working memory (Chung et al., 2020). Moreover, the ReHo values were negatively correlated with worse scores in TMT A and B, which measure the cognitive domains of executive function and attention (Bowie and Harvey, 2006). Accordingly, we speculated that decreased ReHo values for LAG might be involved in the extensive cognitive decline of CI patients. Notably, ReHo values in the LAG were positively associated with the RA of Coprobacter in our study. Genus Coprobacter has been found to be positively associated with insulin supplementation (Jena et al., 2020), an indigestible fiber that can generate short-chain fatty acids (Weitkunat et al., 2015) and improve digestion, memory, and mood in humans (Smith et al., 2015). Our results showed that the RA of this bacteria was positively correlated with better cognitive performance in CI patients, which may indicate potential benefits of this gut bacteria. We also noted a complex relationship between the genus Coprobacter, functional abnormality in a key region of the DMN (angular gyrus), and cognitive impairments in CI patients. There have been prior efforts to explore the associations between GM and DMN abnormalities and cognitive ability in healthy and clinical conditions. Wang et al. (2019) identified a relationship among the GM, DMN dysfunction, and cognitive impairments in patients with end-stage renal disease. Another recent study (Cai et al., 2021) in healthy young adults found that internetwork functional connectivity involving the DMN mediated the relations of GM with sleep quality and executive function. Our study extends these findings by discovering potential associations between the GM, regional brain functional abnormity, and cognitive impairments in CI patients. These findings further suggest that GM-brain function-behavior associations might play a role in the potential neurobiological mechanisms of CI.
Limitations
Our study had several limitations. First, we did not collect fecal samples from the HC group to detect GM differences between them and CI patients. However, many published studies have explored the relationship between the GM and insomnia, and have detected significant differences in GM composition between CI patients and HCs (Liu et al., 2019; Li Y. et al., 2020). Thus, although we did not measure GM differences between the two groups, the findings of this study should be reliable with regard to patients with CI. Second, this is a preliminary cross-sectional study. Future longitudinal studies with gut flora intervention may help to determine the causal relationship between the GM and the brain. Third, although we recruited all subjects from the same site and used strict exclusion criteria to eliminate the potential effect of factors such as dietary differences or other interventions, we could not eliminate all possible confounding factors affecting GM composition. Fourth, the metabolic and inflammatory changes of intestinal flora should be further studied in order to shed light on the possible underlying mechanisms. Finally, we analyzed only associations between regional brain functional activity and GM composition, and did not study the brain structural changes. Multi-modal neuroimaging combined with structural and functional data may explore the interaction between the GM and the brain more comprehensively.
Conclusion
Based on rs-fMRI data using a ReHo method, our study found that regional brain functional abnormalities and emotion/cognition related changes had significant correlations with the specific bacterial taxa of GM in CI patients. Our findings may provide insights into the potential role of the GM in the underlying neuropathology of CI patients and could have significant clinical value in targeting the GM as an intervention for treatment of CI patients.
Data Availability Statement
The raw data supporting the conclusions of this article will be made available by the authors, without undue reservation, to any qualified researcher.
Ethics Statement
The studies involving human participants were reviewed and approved by Ethics Committee of the Guangdong Second Provincial General Hospital. The patients/participants provided their written informed consent to participate in this study. Written informed consent was obtained from the individual(s) for the publication of any potentially identifiable images or data included in this article.
Author Contributions
GJ and CL contributed to the design of the study. YF, GL, ML, HL, JZ, and ZL contributed to the data acquisitions. YF, SF, FC, and ZL contributed to the data analysis. YF, SF, XM, YW, and GJ contributed to the results interpretation. YF, SF, CL, and GJ contributed to manuscript preparation. All authors contributed to the manuscript revision and approved the final version of the manuscript.
Funding
This study was funded by grants from the National Natural Science Foundation of China (Grant Numbers: U1903120, 81771807, and 81901729) and the Science and Technology Planning Project of Guangzhou (Grant Number: 202002030234).
Conflict of Interest
The authors declare that the research was conducted in the absence of any commercial or financial relationships that could be construed as a potential conflict of interest.
Publisher’s Note
All claims expressed in this article are solely those of the authors and do not necessarily represent those of their affiliated organizations, or those of the publisher, the editors and the reviewers. Any product that may be evaluated in this article, or claim that may be made by its manufacturer, is not guaranteed or endorsed by the publisher.
Supplementary Material
The Supplementary Material for this article can be found online at: https://www.frontiersin.org/articles/10.3389/fnins.2021.804843/full#supplementary-material
Footnotes
References
Abarenkov, K., Nilsson, R. H., Larsson, K. H., Alexander, I. J., Eberhardt, U., Erland, S., et al. (2010). The UNITE database for molecular identification of fungi - recent updates and future perspectives. New Phytol. 186, 281–285. doi: 10.1111/j.1469-8137.2009.03160.x
Aizawa, E., Tsuji, H., Asahara, T., Takahashi, T., Teraishi, T., Yoshida, S., et al. (2019). Bifidobacterium and Lactobacillus counts in the gut microbiota of patients with bipolar disorder and healthy controls. Front. Psychiatry 10:730. doi: 10.3389/fpsyt.2018.00730
Akkasheh, G., Kashani-Poor, Z., Tajabadi-Ebrahimi, M., Jafari, P., Akbari, H., Taghizadeh, M., et al. (2016). Clinical and metabolic response to probiotic administration in patients with major depressive disorder: a randomized, double-blind, placebo-controlled trial. Nutrition 32, 315–320. doi: 10.1016/j.nut.2015.09.003
Altena, E., Van Der Werf, Y. D., Sanz-Arigita, E. J., Voorn, T. A., Rombouts, S. A. R. B., Kuijer, J. P. A., et al. (2008). Prefrontal hypoactivation and recovery in insomnia. Sleep 31, 1271–1276. doi: 10.5665/sleep/31.9.1271
American Psychiatric Association [APA] (2014). Cautionary Statement for Forensic Use of DSM-5, Diagnostic and Statistical Manual of Mental Disorders, 5th Edn. Virginia: American Psychiatric Association.
Bae, S., Kang, K. D., Kim, S. W., Shin, Y. J., Nam, J. J., and Han, D. H. (2019). Investigation of an emotion perception test using functional magnetic resonance imaging. Comput. Methods Programs Biomed. 179:104994. doi: 10.1016/j.cmpb.2019.104994
Baglioni, C., Battagliese, G., Feige, B., Spiegelhalder, K., Nissen, C., Voderholzer, U., et al. (2011). Insomnia as a predictor of depression: a meta-analytic evaluation of longitudinal epidemiological studies. J. Affect. Disord. 135, 10–19. doi: 10.1016/j.jad.2011.01.011
Benjamini, Y., and Hochberg, Y. (1995). Controlling the false discovery rate: a practical and powerful approach to multiple testing. J. R. Stat. Soc. Ser. B 57, 289–300. doi: 10.1111/j.2517-6161.1995.tb02031.x
Bluhm, R. L., Osuch, E. A., Lanius, R. A., Boksman, K., Neufeld, R. W. J., Théberge, J., et al. (2008). Default mode network connectivity: effects of age, sex, and analytic approach. Neuroreport 19, 887–891. doi: 10.1097/WNR.0b013e328300ebbf
Bowie, C. R., and Harvey, P. D. (2006). Administration and interpretation of the trail making test. Nat. Protoc. 1, 2277–2281. doi: 10.1038/nprot.2006.390
Bravo, J. A., Forsythe, P., Chew, M. V., Escaravage, E., Savignac, H. M., Dinan, T. G., et al. (2011). Ingestion of Lactobacillus strain regulates emotional behavior and central GABA receptor expression in a mouse via the vagus nerve. Proc. Natl. Acad. Sci. U.S.A. 108, 16050–16055. doi: 10.1073/pnas.1102999108
Cai, H., Wang, C., Qian, Y., Zhang, S., Zhang, C., Zhao, W., et al. (2021). Large-scale functional network connectivity mediate the associations of gut microbiota with sleep quality and executive functions. Hum. Brain Mapp. 42, 3088–3101. doi: 10.1002/hbm.25419
Chung, T., Bae, S. W., Mun, E. Y., Suffoletto, B., Nishiyama, Y., Jang, S., et al. (2020). Mobile assessment of acute effects of marijuana on cognitive functioning in young adults: observational study. JMIR mHealth uHealth 8:e16240. doi: 10.2196/16240
Cryan, J. F., and Dinan, T. G. (2012). Mind-altering microorganisms: the impact of the gut microbiota on brain and behaviour. Nat. Rev. Neurosci. 13, 701–712. doi: 10.1038/nrn3346
Dai, X. J., Nie, X., Liu, X., Pei, L., Jiang, J., Peng, D. C., et al. (2016). Gender differences in regional brain activity in patients with chronic primary insomnia: evidence from a resting-state fMRI study. J. Clin. Sleep Med. 12, 363–374. doi: 10.5664/jcsm.5586
Dai, X. J., Peng, D. C., Gong, H. H., Wan, A. L., Nie, X., Li, H. J., et al. (2014). Altered intrinsic regional brain spontaneous activity and subjective sleep quality in patients with chronic primary insomnia: a resting-state fMRI study. Neuropsychiatr. Dis. Treat. 10, 2163–2175. doi: 10.2147/NDT.S69681
Darien, I. (2014). The International Classification of Sleep Disorders (ICSD-3). Darien, IL: American Academy of Sleep Medicine.
De Santis, S., Moratal, D., and Canals, S. (2019). Radiomicrobiomics: advancing along the gut-brain axis through big data analysis. Neuroscience 403, 145–149. doi: 10.1016/j.neuroscience.2017.11.055
DeSantis, T. Z., Hugenholtz, P., Larsen, N., Rojas, M., Brodie, E. L., Keller, K., et al. (2006). Greengenes, a chimera-checked 16S rRNA gene database and workbench compatible with ARB. Appl. Environ. Microbiol. 72, 5069–5072. doi: 10.1128/AEM.03006-05
Fernandez-Mendoza, J., Baker, J. H., Vgontzas, A. N., Gaines, J., Liao, D., and Bixler, E. O. (2017). Insomnia symptoms with objective short sleep duration are associated with systemic inflammation in adolescents. Brain. Behav. Immun. 61, 110–116. doi: 10.1016/j.bbi.2016.12.026
Fomenky, B. E., Do, D. N., Talbot, G., Chiquette, J., Bissonnette, N., Chouinard, Y. P., et al. (2018). Direct-fed microbial supplementation influences the bacteria community composition of the gastrointestinal tract of pre- and post-weaned calves. Sci. Rep. 8:14147. doi: 10.1038/s41598-018-32375-5
Fortier-Brochu, E., Beaulieu-Bonneau, S., Ivers, H., and Morin, C. M. (2012). Insomnia and daytime cognitive performance: a meta-analysis. Sleep Med. Rev. 16, 83–94. doi: 10.1016/j.smrv.2011.03.008
Foster, J. A., Lyte, M., Meyer, E., and Cryan, J. F. (2016). Gut microbiota and brain function: an evolving field in neuroscience. Int. J. Neuropsychopharmacol. 19:yv114. doi: 10.1093/ijnp/pyv114
Friston, K. J., Williams, S., Howard, R., Frackowiak, R. S. J., and Turner, R. (1996). Movement-related effects in fMRI time-series. Magn. Reson. Med. 35, 346–355. doi: 10.1002/mrm.1910350312
Galland, L. (2014). The gut microbiome and the brain. J. Med. Food. 17, 1261–1272. doi: 10.1089/jmf.2014.7000
Gong, L., Xu, R., Liu, D., Zhang, C., Huang, Q., Zhang, B., et al. (2020). Abnormal functional connectivity density in patients with major depressive disorder with comorbid insomnia. J. Affect. Disord. 266, 417–423. doi: 10.1016/j.jad.2020.01.088
Groenewold, N. A., Opmeer, E. M., de Jonge, P., Aleman, A., and Costafreda, S. G. (2013). Emotional valence modulates brain functional abnormalities in depression: evidence from a meta-analysis of fMRI studies. Neurosci. Biobehav. Rev. 37, 152–163. doi: 10.1016/j.neubiorev.2012.11.015
Henke, M. T., Kenny, D. J., Cassilly, C. D., Vlamakis, H., Xavier, R. J., and Clardy, J. (2019). Ruminococcus gnavus, a member of the human gut microbiome associated with Crohn’s disease, produces an inflammatory polysaccharide. Proc. Natl. Acad. Sci. U.S.A. 116, 12672–12677. doi: 10.1073/pnas.1904099116
Jackson, M. L., Butt, H., Ball, M., Lewis, D. P., and Bruck, D. (2015). Sleep quality and the treatment of intestinal micro biota imbalance in chronic fatigue syndrome: a pilot study. Sleep Sci. 8, 124–133. doi: 10.1016/j.slsci.2015.10.001
Jena, P. K., Sheng, L., Nguyen, M., Di Lucente, J., Hu, Y., Li, Y., et al. (2020). Dysregulated bile acid receptor-mediated signaling and IL-17A induction are implicated in diet-associated hepatic health and cognitive function. Biomark. Res. 8, 1–17. doi: 10.1186/s40364-020-00239-8
Kiviniemi, V. (2008). Endogenous brain fluctuations and diagnostic imaging. Hum. Brain Mapp. 29, 810–817. doi: 10.1002/hbm.20582
Lara, A. H., and Wallis, J. D. (2015). The role of prefrontal cortex in working memory: a mini review. Front. Syst. Neurosci. 9:173. doi: 10.3389/fnsys.2015.00173
Levenson, J. C., Kay, D. B., and Buysse, D. J. (2015). The pathophysiology of insomnia. Chest 147, 1179–1192. doi: 10.1378/chest.14-1617
Li, C., Ma, X., Dong, M., Yin, Y., Hua, K., and Li, M. (2016). Abnormal spontaneous regional brain activity in primary insomnia: a resting-state functional magnetic resonance imaging study. Neuropsychiatr. Dis. Treat. 12, 1371–1378. doi: 10.2147/NDT.S109633
Li, L. L., Wang, Y. T., Zhu, L. M., Liu, Z. Y., Ye, C. Q., and Qin, S. (2020). Inulin with different degrees of polymerization protects against diet-induced endotoxemia and inflammation in association with gut microbiota regulation in mice. Sci. Rep. 10:978. doi: 10.1038/s41598-020-58048-w
Li, S., Song, J., Ke, P., Kong, L., Lei, B., Zhou, J., et al. (2021). The gut microbiome is associated with brain structure and function in schizophrenia. Sci. Rep. 11:9743. doi: 10.1038/s41598-021-89166-8
Li, Y., Hao, Y., Fan, F., and Zhang, B. (2018). The role of microbiome in insomnia, circadian disturbance and depression. Front. Psychiatry 9:669. doi: 10.3389/fpsyt.2018.00669
Li, Y., Richardson, R. M., and Ghuman, A. S. (2019). Posterior fusiform and midfusiform contribute to distinct stages of facial expression processing. Cereb. Cortex 29, 3209–3219. doi: 10.1093/cercor/bhy186
Li, Y., Zhang, B., Zhou, Y., Wang, D., Liu, X., Li, L., et al. (2020). Gut microbiota changes and their relationship with inflammation in patients with acute and chronic insomnia. Nat. Sci. Sleep 12, 895–905. doi: 10.2147/NSS.S271927
Liu, B., Lin, W., Chen, S., Xiang, T., Yang, Y., Yin, Y., et al. (2019). Gut microbiota as an objective measurement for auxiliary diagnosis of insomnia disorder. Front. Microbiol. 10:1770. doi: 10.3389/fmicb.2019.01770
Liu, P., Jia, X. Z., Chen, Y., Yu, Y., Zhang, K., Lin, Y. J., et al. (2021). Gut microbiota interacts with intrinsic brain activity of patients with amnestic mild cognitive impairment. CNS Neurosci. Ther. 27, 163–173. doi: 10.1111/cns.13451
Ma, B., Liang, J., Dai, M., Wang, J., Luo, J., Zhang, Z., et al. (2019). Altered gut microbiota in Chinese children with autism spectrum disorders. Front. Cell. Infect. Microbiol. 9:40. doi: 10.3389/fcimb.2019.00040
Magoč, T., and Salzberg, S. L. (2011). FLASH: fast length adjustment of short reads to improve genome assemblies. Bioinformatics 27, 2957–2963. doi: 10.1093/bioinformatics/btr507
Marques, D. R., Gomes, A. A., Caetano, G., and Castelo-Branco, M. (2017). Insomnia disorder and brain’s default-mode network. Curr. Neurol. Neurosci. Rep. 18, 7–10. doi: 10.1007/s11910-018-0861-3
Martin, M. (2011). Cutadapt removes adapter sequences from high-throughput sequencing reads. EMBnet.journal 17, 10–12. doi: 10.14806/ej.17.1.200
Messaoudi, M., Lalonde, R., Violle, N., Javelot, H., Desor, D., Nejdi, A., et al. (2011). Assessment of psychotropic-like properties of a probiotic formulation (Lactobacillus helveticus R0052 and Bifidobacterium longum R0175) in rats and human subjects. Br. J. Nutr. 105, 755–764. doi: 10.1017/S0007114510004319
Mogavero, M. P., Silvani, A., Delrosso, L. M., Salemi, M., and Ferri, R. (2021). Focus on the complex interconnection between cancer, narcolepsy and other neurodegenerative diseases: a possible case of orexin-dependent inverse comorbidity. Cancers (Basel). 13:2612. doi: 10.3390/cancers13112612
Morin, C. M., and Benca, R. (2012). Chronic insomnia. Lancet 379, 1129–1141. doi: 10.1016/S0140-6736(11)60750-2
Nasreddine, Z. S., Phillips, N. A., Bédirian, V., Charbonneau, S., Whitehead, V., Collin, I., et al. (2005). The montreal cognitive assessment, MoCA: a brief screening tool for mild cognitive impairment. J. Am. Geriatr. Soc. 53, 695–699. doi: 10.1111/j.1532-5415.2005.53221.x
Nie, X., Shao, Y., Liu, S. Y., Li, H. J., Wan, A. L., Nie, S., et al. (2015). Functional connectivity of paired default mode network subregions in primary insomnia. Neuropsychiatr. Dis. Treat. 11, 3085–3093. doi: 10.2147/NDT.S95224
Olaisen, M., Flatberg, A., Granlund, A. V. B., Røyset, E. S., Martinsen, T. C., Sandvik, A. K., et al. (2021). Bacterial mucosa-associated microbiome in inflamed and proximal noninflamed ileum of patients with Crohn’s disease. Inflamm. Bowel Dis. 27, 12–24. doi: 10.1093/ibd/izaa107
Oldfield, R. C. (1971). The assessment and analysis of handedness: the Edinburgh inventory. Neuropsychologia 9, 97–113. doi: 10.1016/0028-3932(71)90067-4
Pang, R., Guo, R., Wu, X., Hu, F., Liu, M., Zhang, L., et al. (2018). Altered regional homogeneity in chronic insomnia disorder with or without cognitive impairment. Am. J. Neuroradiol. 39, 742–747. doi: 10.3174/ajnr.A5587
Patel, S. R., Zhu, X., Storfer-Isser, A., Mehra, R., Jenny, N. S., Tracy, R., et al. (2009). Sleep duration and biomarkers of inflammation. Sleep 32, 200–204. doi: 10.1093/sleep/32.2.200
Pinto-Sanchez, M. I., Hall, G. B., Ghajar, K., Nardelli, A., Bolino, C., Lau, J. T., et al. (2017). Probiotic bifidobacterium longum NCC3001 reduces depression scores and alters brain activity: a pilot study in patients with irritable bowel syndrome. Gastroenterology 153, 448.e8–459.e8. doi: 10.1053/j.gastro.2017.05.003
Poroyko, V. A., Carreras, A., Khalyfa, A., Khalyfa, A. A., Leone, V., Peris, E., et al. (2016). Chronic sleep disruption alters gut microbiota, induces systemic and adipose tissue inflammation and insulin resistance in mice. Sci. Rep. 6:35405. doi: 10.1038/srep35405
Power, J. D., Schlaggar, B. L., and Petersen, S. E. (2015). Recent progress and outstanding issues in motion correction in resting state fMRI. Neuroimage 105, 536–551. doi: 10.1016/j.neuroimage.2014.10.044
Qiu, Z., Yang, H., Rong, L., Ding, W., Chen, J., and Zhong, L. (2017). Targeted metagenome based analyses show gut microbial diversity of inflammatory bowel disease patients. Ind. J. Microbiol. 57, 307–315. doi: 10.1007/s12088-017-0652-6
Quast, C., Pruesse, E., Yilmaz, P., Gerken, J., Schweer, T., Yarza, P., et al. (2013). The SILVA ribosomal RNA gene database project: improved data processing and web-based tools. Nucleic Acids Res. 41, D590–D596. doi: 10.1093/nar/gks1219
Rogers, G. B., Keating, D. J., Young, R. L., Wong, M. L., Licinio, J., and Wesselingh, S. (2016). From gut dysbiosis to altered brain function and mental illness: mechanisms and pathways. Mol. Psychiatry 21, 738–748. doi: 10.1038/mp.2016.50
Rognes, T., Flouri, T., Nichols, B., Quince, C., and Mahé, F. (2016). VSEARCH: a versatile open source tool for metagenomics. PeerJ 4:e2584. doi: 10.7717/peerj.2584
Schloss, P. D., Westcott, S. L., Ryabin, T., Hall, J. R., Hartmann, M., Hollister, E. B., et al. (2009). Introducing mothur: open-source, platform-independent, community-supported software for describing and comparing microbial communities. Appl. Environ. Microbiol. 75, 7537–7541. doi: 10.1128/AEM.01541-09
Sharon, G., Sampson, T. R., Geschwind, D. H., and Mazmanian, S. K. (2016). The central nervous system and the gut microbiome. Cell 167, 915–932. doi: 10.1016/j.cell.2016.10.027
Smith, A. P., Sutherl, D., and Hewlett, P. (2015). An investigation of the acute effects of oligofructose-enriched inulin on subjective wellbeing, mood and cognitive performance. Nutrients 7, 8887–8896. doi: 10.3390/nu7115441
Spielman, L. J., Gibson, D. L., and Klegeris, A. (2018). Unhealthy gut, unhealthy brain: the role of the intestinal microbiota in neurodegenerative diseases. Neurochem. Int. 120, 149–163. doi: 10.1016/j.neuint.2018.08.005
Sudo, N., Chida, Y., Aiba, Y., Sonoda, J., Oyama, N., Yu, X. N., et al. (2004). Postnatal microbial colonization programs the hypothalamic-pituitary-adrenal system for stress response in mice. J. Physiol. 558, 263–275. doi: 10.1113/jphysiol.2004.063388
Thakral, P. P., Madore, K. P., and Schacter, D. L. (2017). A role for the left angular gyrus in episodic simulation and memory. J. Neurosci. 37, 8142–8149. doi: 10.1523/jneurosci.1319-17.2017
Tillisch, K., Labus, J., Kilpatrick, L., Jiang, Z., Stains, J., Ebrat, B., et al. (2013). Consumption of fermented milk product with probiotic modulates brain activity. Gastroenterology 144, 1394–1401. doi: 10.1053/j.gastro.2013.02.043
Verduijn, J., Milaneschi, Y., Schoevers, R. A., Van Hemert, A. M., Beekman, A. T. F., and Penninx, B. W. J. H. (2015). Pathophysiology of major depressive disorder: mechanisms involved in etiology are not associated with clinical progression. Transl. Psychiatry 5:e649. doi: 10.1038/tp.2015.137
Vindegaard, N., Speyer, H., Nordentoft, M., Rasmussen, S., and Benros, M. E. (2020). Gut microbial changes of patients with psychotic and affective disorders: a systematic review. Schizophr. Res. 234, 1–10. doi: 10.1016/j.schres.2019.12.014
Wang, H., Qin, X., Gui, Z., and Chu, W. (2020). The effect of Bailemian on neurotransmitters and gut microbiota in p-chlorophenylalanine induced insomnia mice. Microb. Pathog. 148:104474. doi: 10.1016/j.micpath.2020.104474
Wang, L., Dai, W., Su, Y., Wang, G., Tan, Y., Jin, Z., et al. (2012). Amplitude of low-frequency oscillations in first-episode, treatment-naive patients with major depressive disorder: a resting-state functional MRI study. PLoS One 7:e48658. doi: 10.1371/journal.pone.0048658
Wang, Q., Garrity, G. M., Tiedje, J. M., and Cole, J. R. (2007). Naïve Bayesian classifier for rapid assignment of rRNA sequences into the new bacterial taxonomy. Appl. Environ. Microbiol. 73, 5261–5267. doi: 10.1128/AEM.00062-07
Wang, Q., Liu, X., Fu, J., Wang, S., Chen, Y., Chang, K., et al. (2018). Substrate sustained release-based high efficacy biosynthesis of GABA by Lactobacillus brevis NCL912. Microb. Cell Fact. 17:80. doi: 10.1186/s12934-018-0919-6
Wang, T., Li, S., Jiang, G., Lin, C., Li, M., Ma, X., et al. (2016). Regional homogeneity changes in patients with primary insomnia. Eur. Radiol. 26, 1292–1300. doi: 10.1007/s00330-015-3960-4
Wang, Y. F., Zheng, L. J., Liu, Y., Ye, Y. B., Luo, S., Lu, G. M., et al. (2019). The gut microbiota-inflammation-brain axis in end-stage renal disease: perspectives from default mode network. Theranostics 9, 8171–8181. doi: 10.7150/thno.35387
Weitkunat, K., Schumann, S., Petzke, K. J., Blaut, M., Loh, G., and Klaus, S. (2015). Effects of dietary inulin on bacterial growth, short-chain fatty acid production and hepatic lipid metabolism in gnotobiotic mice. J. Nutr. Biochem. 26, 929–937. doi: 10.1016/j.jnutbio.2015.03.010
Wickwire, E. M., Shaya, F. T., and Scharf, S. M. (2016). Health economics of insomnia treatments: the return on investment for a good night’s sleep. Sleep Med. Rev. 30, 72–82. doi: 10.1016/j.smrv.2015.11.004
Wu, Q., Tun, H. M., Law, Y. S., Khafipour, E., and Shah, N. P. (2017). Common distribution of gad operon in Lactobacillus brevis and its GadA contributes to efficient GABA synthesis toward cytosolic near-neutral pH. Front. Microbiol. 8:206. doi: 10.3389/fmicb.2017.00206
Wu, Q. Z., Li, D. M., Kuang, W. H., Zhang, T. J., Lui, S., Huang, X. Q., et al. (2011). Abnormal regional spontaneous neural activity in treatment-refractory depression revealed by resting-state fMRI. Hum. Brain Mapp. 32, 1290–1299. doi: 10.1002/hbm.21108
Xiao, C., Fedirko, V., Beitler, J., Bai, J., Peng, G., Zhou, C., et al. (2021). The role of the gut microbiome in cancer-related fatigue: pilot study on epigenetic mechanisms. Support. Care Cancer 29, 3173–3182. doi: 10.1007/s00520-020-05820-3
Zang, Y., Jiang, T., Lu, Y., He, Y., and Tian, L. (2004). Regional homogeneity approach to fMRI data analysis. Neuroimage 22, 394–400. doi: 10.1016/j.neuroimage.2003.12.030
Keywords: gut microbiota, chronic insomnia, functional magnetic resonance imaging, resting-state, regional homogeneity
Citation: Feng Y, Fu S, Li C, Ma X, Wu Y, Chen F, Li G, Liu M, Liu H, Zhu J, Lan Z and Jiang G (2022) Interaction of Gut Microbiota and Brain Function in Patients With Chronic Insomnia: A Regional Homogeneity Study. Front. Neurosci. 15:804843. doi: 10.3389/fnins.2021.804843
Received: 29 October 2021; Accepted: 14 December 2021;
Published: 05 January 2022.
Edited by:
Dilshan Shanaka Harischandra, Covance, United StatesReviewed by:
Maria Paola Mogavero, Scientific Clinical Institute Maugeri (ICS Maugeri), ItalySuhua Chang, Peking University Sixth Hospital, China
Copyright © 2022 Feng, Fu, Li, Ma, Wu, Chen, Li, Liu, Liu, Zhu, Lan and Jiang. This is an open-access article distributed under the terms of the Creative Commons Attribution License (CC BY). The use, distribution or reproduction in other forums is permitted, provided the original author(s) and the copyright owner(s) are credited and that the original publication in this journal is cited, in accordance with accepted academic practice. No use, distribution or reproduction is permitted which does not comply with these terms.
*Correspondence: Guihua Jiang, amlhbmdnaEBnZDJoLm9yZy5jbg==
†These authors have contributed equally to this work