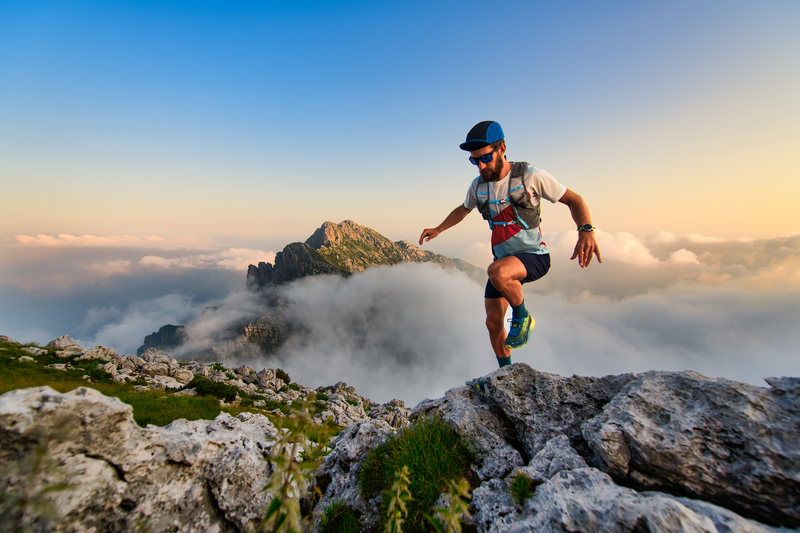
94% of researchers rate our articles as excellent or good
Learn more about the work of our research integrity team to safeguard the quality of each article we publish.
Find out more
REVIEW article
Front. Neurosci. , 07 October 2021
Sec. Sleep and Circadian Rhythms
Volume 15 - 2021 | https://doi.org/10.3389/fnins.2021.751730
This article is part of the Research Topic Unraveling sleep and its disorders using novel analytical approaches View all 12 articles
Parts of this article's content have been modified or rectified in:
Erratum: New and Emerging Approaches to Better Define Sleep Disruption and Its Consequences
Current approaches to quantify and diagnose sleep disorders and circadian rhythm disruption are imprecise, laborious, and often do not relate well to key clinical and health outcomes. Newer emerging approaches that aim to overcome the practical and technical constraints of current sleep metrics have considerable potential to better explain sleep disorder pathophysiology and thus to more precisely align diagnostic, treatment and management approaches to underlying pathology. These include more fine-grained and continuous EEG signal feature detection and novel oxygenation metrics to better encapsulate hypoxia duration, frequency, and magnitude readily possible via more advanced data acquisition and scoring algorithm approaches. Recent technological advances may also soon facilitate simple assessment of circadian rhythm physiology at home to enable sleep disorder diagnostics even for “non-circadian rhythm” sleep disorders, such as chronic insomnia and sleep apnea, which in many cases also include a circadian disruption component. Bringing these novel approaches into the clinic and the home settings should be a priority for the field. Modern sleep tracking technology can also further facilitate the transition of sleep diagnostics from the laboratory to the home, where environmental factors such as noise and light could usefully inform clinical decision-making. The “endpoint” of these new and emerging assessments will be better targeted therapies that directly address underlying sleep disorder pathophysiology via an individualized, precision medicine approach. This review outlines the current state-of-the-art in sleep and circadian monitoring and diagnostics and covers several new and emerging approaches to better define sleep disruption and its consequences.
Sleep, along with diet and exercise, is essential for optimal health and wellbeing. However, globally, nearly 2 billion people are estimated to have one or both of the two most common clinical sleep disorders–sleep apnea (Benjafield et al., 2019) and insomnia (Roth et al., 2011). Most people with sleep disorders remain undiagnosed and untreated, and thus vulnerable to the major adverse health and safety consequences associated with untreated sleep disorders.
Current sleep apnea diagnostic approaches rely on traditional labor-intensive overnight sleep tests and subjective manual scoring approaches developed around the constraints of paper-based methods from the 1960’s. This approach, in combination with the advent of continuous positive airway pressure (CPAP) to reverse airway collapse during sleep (Sullivan et al., 1981), led to rapid advances in the modern field of sleep medicine. Although efficacious irrespective of underlying mechanisms, sub-optimal patient acceptance and use of CPAP remain problematic and warrant personalized treatments that better target underlying causal mechanisms. However, traditional sleep assessment methods fail to identify the specific underlying causes and consequences of sleep disorders for individual patients. For example, relationships between perceived sleep quality and/or sleepiness and objective sleep measures derived from traditional gold-standard polysomnography are either absent, weak, or inconsistent (Buysse et al., 2008; Sforza et al., 2015; Adams et al., 2016). In the case of insomnia, diagnosis relies on clinical evaluation since traditional objective sleep measures do not relate to disorder incidence, severity, or recovery. While the gold standard treatment, cognitive behavioral therapy for insomnia (CBT-I) is efficacious for many, it is ineffective or only partially effective for some patients (Trauer et al., 2015). This is potentially because, like CPAP for sleep apnea, CBT-I is a one-size-fits-all treatment regardless of the underlying causal mechanisms (Harvey and Tang, 2003). As such, usual care for sleep disorders typically relies on a trial-and-error treatment approach which often fails to identify the underlying causes of sleep disruption or adequately address patient symptoms and health consequences for which individuals seek treatment. Accordingly, this review focuses on highlighting new and emerging approaches to better define sleep and circadian disruption that underpins sleep disorders based on their underlying pathophysiology and accompanying health impacts.
Current gold-standard methodology to quantify sleep relies on overnight polysomnographic (PSG) recordings. This includes collection of a wealth of neurophysiological data from electroencephalography (EEG), electrooculography (EOG), electromyography (EMG), electrocardiography (ECG), body position and movement, and respiratory-related signals including airflow, chest and abdominal motion, and oximetry. These signals are then manually reviewed and analyzed to classify wake, light through to deep non-rapid eye movement (NREM) (N1, N2, and N3), and rapid eye movement (REM) sleep in 30-s epochs. Transient cortical arousals (3–15 s) and longer awakening (>15 s) events are also manually scored on the basis of internationally standardized American Academy of Sleep Medicine (AASM) EEG criteria (Berry et al., 2017).
Traditional polysomnography scoring evolved from observations of behavioral responsiveness changes coincident with changes in EEG patterns of activity at a time when chart recorders necessitated manual scoring, quite literally page-by-30-s-page (Rechtschaffen, 1968). This pattern-matching “bottom-up” approach to sleep medicine was based on the practical constraints with the technology available at the time, rather than being driven by an understanding of underlying sleep neurobiology. Although computerized systems have replaced paper-based recordings, and despite exponential advances in modern computing, sleep medicine remains predominantly based on these manual scoring methods from the 1960s. Manual scoring is labor intensive, and therefore costly, and captures only gross visually discernible EEG features with much poorer time and frequency resolution than is available within the data (Figure 1). Thus, EEG scoring into discrete 30-s epochs ignores that wake and sleep are continuous and dynamic states, whereby physiological features within epochs classified as wake can be present during sleep, and vice versa (Prerau et al., 2014; Scott et al., 2020). Manual scoring also has large intra- and inter-scorer variability, which remains problematic in sleep medicine despite AASM scoring criteria updates that attempt to reduce scoring variability (Ruehland et al., 2009; Magalang et al., 2013).
Automated sleep scoring methods using advanced signal processing and machine learning approaches to analyze polysomnography signals have been widely developed and can achieve good agreement against consensus-based traditional human scoring (Fiorillo et al., 2019). However, most of the focus has been on reproducing existing manual approaches (Tsinalis et al., 2016; Supratak et al., 2017; Chambon et al., 2018; Olesen et al., 2021). Thus, while these approaches are more standardized and time efficient, the fundamental limitations of traditional sleep metrics remain. Robust evidence to support causal relationships and clinical utility of most existing sleep metrics also remains sparse. Thus, the finer-grained quantifiable features within polysomnography data that may ultimately be more informative regarding underlying sleep mechanisms and quality continue to be largely ignored.
For example, EEG delta waves are tightly coupled in time and precede pulsatile changes in cerebral blood volume and cerebrospinal fluid flow during deep sleep (Fultz et al., 2019). Furthermore, a single night without sleep in healthy volunteers leads to β-amyloid accumulation (Shokri-Kojori et al., 2018). These findings support that delta waves during deep NREM sleep are a major driver of glymphatic clearance of metabolites from the central nervous system (Benveniste et al., 2020; Braun and Iliff, 2020). Wake/sleep transitions, such as potentially fatal microsleeps while driving, and a range of other physiological changes during sleep also occur on shorter timescales than assessed through traditional manual sleep scoring methods. For example, traditional scoring most likely misses potentially clinically informative neurophysiological features of synaptic downscaling, re-organization, memory and learning processes thought to occur during NREM and REM sleep (Tononi and Cirelli, 2006). Thus, conventional sleep scoring can only provide relatively superficial insights into brain activity and other physiological changes during sleep that are unlikely to be as sensitive or specific to underlying mechanisms as shorter-time scale features of sleep. Accordingly, a more physiologically guided, top-down measurement approach is clearly needed to provide greater neurobiological insight into sleep health and disease, and how sleep disturbance features relate to clinically relevant outcomes (Léger et al., 2018).
Defining evidence-based electrophysiological sleep markers is important in the age of precision medicine, particularly following rapid growth in minimally intrusive recording and consumer wearable devices that allow for sleep-related monitoring over prolonged periods in the home environment (Liu et al., 2017; Kim et al., 2019). These and other emerging technologies are likely to change many aspects of polysomnography, such as via printed electrodes (Norton et al., 2015) or tripolar concentric ring EEG (Besio et al., 2006), by helping to uncover aspects of sleep health not routinely measured. For example, markers of circadian misalignment are technically difficult to monitor, so remain notably absent from conventional sleep studies. Emerging evidence highlights the potential to estimate circadian phase using non-intrusive physiological data such as skin temperature, heart rate variability and activity (Suárez et al., 2020; Cheng et al., 2021). Blood pressure surges along with vasoconstriction and heart rate responses occur frequently during sleep, especially with swallowing (Burke et al., 2020), but are not currently routinely captured or assessed. Continuous measurement of a range of biomarkers such as cortisol secretion during sleep through skin sensor devices (Parlak et al., 2018) may also have clinical utility.
Together, a range of new and emerging devices could routinely generate large volumes of sleep measurements over extended periods. This approach will require evidence to support clinical use and value, and software tools to assist clinicians to assess, analyze, and interpret sleep-omics (Redline and Purcell, 2021). To increase the uptake of new technologies in research and clinical settings, greater communication between sleep medicine experts and device manufacturers is needed. Rigorous standards for validation and evidence-based advances in medicine are required to ensure that new methods provide clinically useful insights that effectively and cost-effectively improve key patient outcomes (Depner et al., 2020). While not a complete list of all available approaches, the sections below highlight several examples of existing approaches and notable promising new and emerging methods based on underlying pathophysiology/neurobiology to move beyond key limitations of current sleep metrics. A schematic representation of some of these examples is provided in Figure 2.
Figure 2. Schematic overview of the current metrics derived from standard polysomnography and the potential to make better use of these extensive neurophysiological signals provide novel insight into sleep neurobiology, treatment prediction and to better link with key clinical and health outcomes. Refer to the text for further detail. CPAP = continuous positive airway pressure, CV = cardiovascular, EEG = electroencephalography, EMG = electromyography, EOG = electrooculography, ECG = electrocardiography, HGNS = hypoglossal nerve stimulation, MAS = mandibular advancement splint, PPG = Photoplethysmography, REM = rapid eye movement, SpO2 = estimated arterial blood oxygen saturation and UA = upper airway.
This review focuses on novel sleep metrics derived from EEG collected clinically using routine polysomnography. Other reviews regarding potential neurobiological insights of sleep physiology and circadian rhythms through high density EEG and intra-cranial/depth EEG are available elsewhere (Mosqueiro et al., 2014; Saper and Fuller, 2017; Scammell et al., 2017).
Slow waves (0.5–4.5 Hz) are the main feature of deep sleep and one of the fundamental electrophysiological features of synchronous neuronal “down states” of relative neuronal inactivity and “up states” as activity resumes (Nir et al., 2011). These waves are thought to play a major role in synaptic recovery and down-scaling to compensate for daily high neuronal activity and synaptic potentiation during wake (Tononi and Cirelli, 2006) and glymphatic system removal of metabolic waste products from the central nervous system (Benveniste et al., 2020; Braun and Iliff, 2020). Slow waves are ubiquitous during sleep, and decrease in quantity and magnitude with age (Chinoy et al., 2014). Several techniques have been developed to study specific aspects of slow waves, such as slow wave slope, absolute power, amplitude and phase in response to a range of experimental or naturalistic (e.g., aging) conditions (Massimini et al., 2004; Bersagliere and Achermann, 2010; Lazar et al., 2015; Lendner et al., 2020; Djonlagic et al., 2021). For example, the slope of half slow-waves (i.e., the slope between the up- and down-states) and slow wave amplitude/absolute power increase with sleep restriction and decrease with circadian phase, suggesting that sleep need and circadian rhythms have an effect on the shape and distribution of slow oscillations (Massimini et al., 2004; Bersagliere and Achermann, 2010; Lazar et al., 2015). Using the same features, reduced slow oscillations during sleep (low amplitude/absolute power) have recently been associated with poorer cognitive performance on a digit symbol coding test and the Trails B test in a large cross-sectional study of ∼3800 participants (Djonlagic et al., 2021). Many of these tools are available in open-source packages. With standardization, clinical validation and implementation, these novel metrics have substantial potential to provide unique insight into inter-individual vulnerability to specific health consequences in people with sleep disruption (Léger et al., 2018).
K-complexes are a form of isolated slow waves that provide unique insight into sleep stability and sleep disruption. They can occur spontaneously during sleep. However, K-complexes can also provide a sensitive marker of sensory disturbance to noise, respiratory and vibratory stimuli during sleep (Colrain, 2005; Scott et al., 2020; Lechat et al., 2021). Abnormal K-complex morphology (lower amplitude) and lower K-complex density (# per minutes) have been associated with the progression of amnestic mild cognitive impairment (pre-clinical phase of Alzheimer’s disease) in ∼70 patients (Liu et al., 2020). Abnormal K-complex morphology has also been associated with greater lapses in next-day alertness as measured using a psychomotor vigilance task (Parekh et al., 2019, 2021). At a population level, cross-sectional studies have suggested that a decrease in K-complex density may be a biomarker of sleep disorders, such as sleep apnea (Lechat et al., 2020). Further evidence regarding the functional significance of K-complexes is still emerging and warrants future investigation. This is likely to be facilitated via recent open-source tool developments (Parekh et al., 2015; Lechat et al., 2020).
Sleep spindles are bursts of 11–15 Hz EEG activity and are another characteristic feature of NREM sleep that may provide a useful biomarker of sleep regulation and cognitive functioning (Diekelmann and Born, 2010; Djonlagic et al., 2021). Sleep spindles are influenced by genetics and vary widely across the lifespan and different demographics (Purcell et al., 2017). Higher spindle occurrence (and density) have been associated with better memory performance and vigilance (Lafortune et al., 2014; Hennies et al., 2016) in cross-sectional studies with moderate sample sizes (n < 100). In a clinical population of 47 patients with obstructive sleep apnea (OSA), greater sleep spindle activity was associated with better implicit learning (Stevens et al., 2021). A recent analysis of two large US-cohorts (n∼3800) also supported an association between higher spindle occurrence and spindle power with greater performance on multiple cognitive tests (Djonlagic et al., 2021). In addition, the coupling (proximity and phase differences) between slow oscillations and spindles was also predictive of cognitive performance, further supporting a role of spindles in memory formation (Hahn et al., 2020) and consolidation (Helfrich et al., 2019; Muehlroth et al., 2019). Together, these results may explain, at least in part, the association between abnormal spindle activity during sleep and neurodegenerative diseases such as Alzheimer’s disease (Gorgoni et al., 2016) and Parkinson’s diseases (Christensen et al., 2015). However, spindle detection is still a challenge and algorithm refinements on public benchmark datasets remain warranted (Warby et al., 2014; Lacourse et al., 2020). Furthermore, recent evidence suggests that the current definition of sleep spindles may be too restrictive and traditionally defined spindles may only be a small subset of a more generalized class of sigma oscillations during sleep (Dimitrov et al., 2021).
Sleep EEG is ideally suited to frequency and time-frequency analysis, since different stages or micro-elements (such as spindles, K-complexes, slow waves) have specific frequency characteristics (Steriade, 2006; Scammell et al., 2017), as shown in Figure 3. Power spectral analysis of EEG (sometimes referred to as quantitative EEG [qEEG]) provides a more sensitive and objective marker of neurophysiological features of sleep, some of which may be unique to specific patient phenotypes. For example, several studies have used qEEG to calculate the mean absolute power of given frequency bands (delta, alpha, theta, sigma, and beta), usually averaged over NREM and REM sleep, some of which have been shown to be predictive of insomnia (Krystal et al., 2002; Krystal and Edinger, 2010; Lunsford-Avery et al., 2021; Zhao et al., 2021) and OSA (D’Rozario et al., 2017; Appleton et al., 2019). Emerging evidence also suggests that qEEG markers are associated with vigilance and cognitive performance (Vakulin et al., 2016; Djonlagic et al., 2021; Mullins et al., 2021).
Figure 3. Spectrogram of sleep EEG signals using methods developed in Prerau et al. (2017). A transition from slow wave sleep (1) to N2 sleep (3) with an arousal in the middle (2) is observed. Slow wave sleep is characterized by high absolute power at frequencies less than 4 Hz and very little power at high frequencies, thus making identification of high frequency (8–16 Hz) arousals straight-forward. The transition from arousal to N2 sleep is also very specific, with a reduction in high frequency power, a sparse low frequency burst (likely reflecting K-complexes), sometimes followed by a burst of 12–16 Hz activity.
The odds ratio product (ORP) is a novel EEG-derived metric that provides a continuous index of sleep depth and alertness (Younes et al., 2015; Younes and Hanly, 2016). ORP is calculated as a ratio of absolute power of different frequency bands over 3-s segments. The ratio ranges from 0 to 2.5, where 0 indicates very deep sleep and 2.5 is wide awake, and correlates well with the visual appearance of EEG across the night (Younes et al., 2015, 2020). ORP derived metrics may be useful for a wide range of clinical applications, such as phenotyping sleep disorders and associated health consequences (Younes and Giannouli, 2020; Azarbarzin et al., 2021; Younes et al., 2021). For example, sleep depth coherence between C3 and C4 channels measured using the ORP is associated with risk of motor vehicle crashes (Azarbarzin et al., 2021). A higher ORP during NREM sleep is also associated with the presence of OSA and insomnia, consistent with a more “alert” brain during NREM sleep in people with OSA and insomnia (Younes et al., 2021).
The scale-free component of neural activity (sometimes called “background brain activity” or “1/f” activity) is a further EEG component that may be an important biomarker of arousal level in human sleep (Lendner et al., 2020). Consistent with neuronal homeostatic and synaptic reorganization activity that takes place during REM sleep, 1/f activity is higher during REM sleep episodes. This observation may be especially important given the lack of targeted metrics designed to capture key physiological features of REM sleep. Eye movements, theta waves and atonia components require further investigation to test for relationships more comprehensively against other markers of REM sleep homeostasis and key clinical outcomes.
A limitation of all current biomarkers is the reliance on traditional manual scoring to express and evaluate summary values against conventional metrics with uncertain relationships with clinical endpoints. For example, absolute delta power, or ORP values, are usually averaged in NREM sleep. Spindles may be only detected in N2 sleep, and K-complex densities calculated in N2 and N3 sleep do not consider fluctuations in neurophysiological features across sleep cycles. EEG dynamics across sleep cycles are highly likely to be regulated by physiological processes such as circadian rhythms, brain metabolism, motor control learning, and memory consolidation processes (e.g., Figure 4). While averaging over traditionally scored sleep stages is convenient, it likely masks more subtle and potentially functionally important sleep-dependent changes over both short (<30 s) and longer cumulative time scales (minutes or hours). Secondly, current clinical utility of these biomarkers has mainly been studied cross-sectionally. Thus, well-designed randomized trials to investigate their potential additive benefit to sleep disorders management to improve health outcomes is warranted. Thirdly, methodologies used to calculate qEEG, ORP and other more fine-grained EEG elements are not standardized across research groups. Some methods are also not available under common license terms and therefore, independent cross-validation remains challenging.
Pulse oximeters can continuously and minimally intrusively estimate blood hemoglobin oxygen saturation (SpO2) and are an almost ubiquitous device in the hospital environment (Jubran, 2004). Overnight pulse oximetry also provides a key requisite measure for the evaluation of sleep apnea (Netzer et al., 2001; Terrill, 2020). Standard traditional time-series measures derived from the oxygen saturation signal include mean and nadir overnight SpO2, time spent below SpO2 of 90% and the oxygen desaturation index (ODI), typically calculated as the number of 3 or 4% desaturations below baseline levels per hour of sleep. However, these metrics have their limitations and agreed standards for their calculation remain lacking. For example, the ODI is partly dependent on the criteria used to define SpO2 dip onsets, offsets, and duration. The ODI also only reflects the frequency of hypoxemic events and fails to reflect the degree and duration of hypoxemia and further oxidative stress through rapid reoxygenation (Punjabi et al., 2008). The physiological consequence of a 3 or 4% drop is also likely dependent on the baseline saturation level and temporal pattern of desaturation which can vary widely between individuals and comorbidities (Ayache and Strohl, 2018). Nonetheless, worse overnight hypoxemia derived from these traditional metrics has been associated with adverse health outcomes, such as increased blood pressure (Pengo et al., 2016; Su et al., 2021) and more recently atrophy of cortical and subcortical brain areas (Marchi et al., 2020). However, relationships with traditional hypoxia measures and important health/physiological outcomes are often weak, with inconsistent reproducibility between studies and cohorts (Pretto et al., 2014; Baumert et al., 2020; Linz et al., 2020; Terrill, 2020).
Other non-traditional parameters from SpO2 such as the delta index measures the mean absolute difference between successive points at constant time intervals (Levy et al., 1996; Magalang et al., 2003; Lin et al., 2009), saturation impairment index computed as the time integral over which SpO2 is below certain threshold levels (i.e., baseline, 90, 80, 70, 60, and 50% saturation) (Kirby et al., 1992), and the hypoxic burden index computed as the area under the time versus desaturation curve (SpO2 < 90%) divided by total sleep time (Azarbarzin et al., 2019; Baumert et al., 2020) have been derived and used in research settings. Some of these parameters have been associated with important health outcomes. For example, hypoxic burden measures that incorporate frequency, duration and magnitude of hypoxemia have recently been shown to predict cardiovascular disease mortality in different cohorts, whereas traditional PSG metrics such as the AHI and ODI do not (Azarbarzin et al., 2019; Baumert et al., 2020). Quantification of an easily measured index of sleep apnea-related hypoxemia has recently been used to predict incident heart failure (Azarbarzin et al., 2020). Accordingly, there remains considerable scope to better understand the precise mechanisms and characteristics by which hypoxemic and reoxygenation events during sleep contribute to cardiovascular and other end-organ damage, and to derive sensitive metrics to quantify these and other important health consequences. These recent findings highlight the potential for improvement beyond current traditional metrics. Through pulsatile changes in light absorption, oximeters can also provide potentially clinically useful markers of vasoconstriction responses during sleep (Catcheside et al., 2001; Jordan et al., 2003) that may be clinically useful predictors of cardiovascular risk (Hirotsu et al., 2020).
Assessment of autonomic nervous system activity during sleep is facilitated using photoplethysmography and ECG. The use of these signals in sleep medicine including new analytical methods and the potential insights they can provide has been covered in recent in-depth reviews (Fischer and Penzel, 2019; Ucak et al., 2021).
High increases in heart rate following apneic events are associated with 30–60% increases in mortality risk and non-fatal/fatal cardiovascular disease compared to normal heart rate responses (Azarbarzin et al., 2020). New evidence also suggests that heart rate variability during wakefulness could be a useful marker of OSA severity and excessive daytime sleepiness, whereby OSA severity is associated with reduced and less complex dynamics of heart rate variability (Qin et al., 2021). Pulse wave amplitude (a marker of vasoconstriction in the finger) features (e.g., amplitude, frequency) have been associated with hypertension, cardiovascular events and diabetes (Hirotsu et al., 2020). Similarly, a decrease in pulse arrival time (time delay of pulse propagation between two points such as heart and finger) as a result of apneic events, is a predictor of subclinical cardiovascular disease and future cardiovascular events (Kwon et al., 2021). Pulse wave amplitude and heart rate responses are also sensitive markers to sensory disturbances during sleep such as noise (Catcheside et al., 2002; Griefahn et al., 2008) and may therefore provide unique insights into downstream health effect of environmental sleep disturbances.
While an exhaustive list of sleep metrics is not the objective of this review, and recent detailed reviews are available elsewhere (Mendonça et al., 2019; Lim et al., 2020), a few key metrics warrant brief coverage.
Motor system disorders such as periodic limb movement (PLM) and REM sleep behavior disorders (RBD) are associated with adverse outcomes. For example, PLMs are associated with stroke and cardiovascular risk factors in certain patient populations (Lindner et al., 2012). RBD may be an early biomarker of subsequent synucleinopathies such as Parkinson’s disease (Claassen et al., 2010) and may increase the risk of stroke (Ma et al., 2017). RBD in people with Parkinson’s disease is also associated with faster motor progression and cognitive decline (Pagano et al., 2018). However, diagnosis of motor system disorders can be challenging. For example, screening questionnaires for RBD have variable sensitivity and specificity (Stiasny-Kolster et al., 2007; Li et al., 2010; Boeve et al., 2011). Thus, there is a need for better diagnostic approaches for motor system disorders. These include leg actigraphy for PLMs (Plante, 2014), more standardized quantifiable approaches using EMG signals during polysomnography (Frauscher et al., 2012) and novel 3D video analysis approaches (Waser et al., 2020).
The cyclic alternating pattern (CAP) is an additional sleep scoring system beyond traditional AASM sleep scoring which aims to quantify NREM discontinuity by characterizing phases of activation (A phases) and periods of inactivity (B phases) (Terzano et al., 2001). Automatic methods of CAP scoring have been proposed (Hartmann and Baumert, 2019) and have been applied to study and define NREM instability in large population-based studies (Buysse et al., 2010; Hartmann et al., 2020) and may provide unique insight into sleep neurobiology. CAP and its potential utility is discussed in detail in recent comprehensive reviews (Mendonça et al., 2019; Lim et al., 2020).
Several research groups have investigated the coupling between multiple physiological signals, such as heart rate with respiratory signals (named cardio-pulmonary coupling) (Thomas et al., 2005, 2018; Bartscha et al., 2012; Penzel et al., 2016). Coupling-based analyses have also been applied between sleep EEG and heart rate (Brandenberger et al., 2001). The theoretical concept of coupling-functions between different physiological systems has been recently generalized under the framework of network physiology (Bashan et al., 2012; Ivanov et al., 2016). A more in-depth review of these techniques and their potential to provide insight into sleep neurobiology and consequences of impaired coupling is available in the literature (Ivanov et al., 2016; Penzel et al., 2016; de Zambotti et al., 2018b).
The underlying causes of the most common sleep-related breathing disorder, OSA, vary considerably between patients. Current evidence indicates that there are at least four key pathophysiological “phenotypes,” more recently termed “endotypes,” that contribute to OSA pathophysiology (Eckert et al., 2013; Eckert, 2018a; Malhotra et al., 2020). While impaired pharyngeal anatomy is the most influential endotype, the magnitude of impaired pharyngeal anatomy varies widely between patients. In addition, approximately 70% of patients also have one or more non-anatomical endotypes that contribute to their OSA (Eckert et al., 2013; Eckert, 2018a). These include impaired pharyngeal dilator muscle function during sleep, unstable control of breathing (high loop gain) and waking up too easily to minor airway narrowing events during sleep (low respiratory arousal threshold) (Figure 5). These advances in knowledge in OSA pathophysiology have major implications for targeted therapy through “precision medicine.” For example, detailed physiological studies in which the key OSA endotypes have been quantified and non-CPAP interventions delivered to improve one or more of the non-anatomical treatable traits can reduce OSA severity (Eckert et al., 2011; Edwards et al., 2012, 2016b; Sands et al., 2018a; Aishah and Eckert, 2019; Taranto-Montemurro et al., 2019; Op de Beeck et al., 2021). Identification of patients with a low respiratory arousal threshold endotype may be an important physiological predictor of CPAP treatment failure (Gray et al., 2017; Zinchuk et al., 2021) and the presence of a low arousal threshold endotype is associated with mortality (Butler et al., 2019). Similarly, identification of patients impairment in endotypes such as high loop gain and highly collapsible pharyngeal airways may be important predictors for non-CPAP treatment failure including upper airway surgery, mandibular advancement splint therapy, hypoglossal nerve stimulation, pharmacotherapy (Edwards et al., 2016a; Li et al., 2017; Aishah and Eckert, 2019; Op de Beeck et al., 2021) and potentially positional therapy (Eckert, 2018b).
Figure 5. Schematic of the four key endotypic traits that contribute to OSA pathophysiology. (A) Impaired pharyngeal anatomy/collapsible upper airway. Non-anatomical endotypes include: (B) Poor pharyngeal dilator muscle function including poor responsiveness/activation to negative pharyngeal pressure/airway narrowing, (C) a low respiratory arousal threshold (waking up too easily to minor pharyngeal narrowing events); and (D) Unstable respiratory control/increased sensitivity to minor changes in CO2 (high loop gain). Each of these endotypes is a target for therapy or a “treatable trait.” Adapted from Carberry et al. (2018) and Aishah and Eckert (2019).
However, current detailed physiological quantification of OSA endotypes is intrusive and far more complex and time-consuming to perform and analyze than standard polysomnography (Eckert, 2018a). Thus, this approach is impractical for clinical use. Accordingly, novel approaches to estimate the key OSA endotypes have been developed. These include more scalable advanced signal processing techniques (Sands et al., 2018a,b), machine learning approaches (Dutta et al., 2021) and algorithms (Edwards et al., 2014) which simply make better use of the existing rich neurophysiological and respiratory information acquired from diagnostic polysomnography recordings and standard clinical metrics such as age and BMI. Other strategies to estimate specific OSA endotypes include estimates based on a simple intervention during a CPAP titration study (Osman et al., 2020), the therapeutic CPAP level (Landry et al., 2017) and wakefulness upper airway physiology testing (Wang et al., 2018; Osman et al., 2019). These principles and recent proof-of-concept findings have opened multiple new lines of investigation for the development of more clinically feasible and scalable approaches to help better guide targeted therapy and precision medicine for OSA.
Aside from advances in PSG sleep and breathing metrics, new approaches are emerging in the assessment of circadian rhythms; another key determinant of sleep and its disorders (Borbély, 1982; Daan et al., 1984). These endogenous rhythms are ubiquitous, with nearly every cell in the human body influenced by a biological “clock.” The suprachiasmatic nucleus in the hypothalamus, colloquially termed the “master” clock, governs the timing of many circadian rhythms influential for sleep, including melatonin secretion, core body temperature (Cajochen et al., 2003), gene transcription and translation regulated clock behavior of nucleated cells throughout the body (Kondratova and Kondratov, 2012). Even non-nucleated red blood cells show circadian cycling of redox activity (O’Neill and Reddy, 2011). The effects of circadian rhythms on sleep disruption are most evident in circadian rhythm sleep disorders, such as delayed and advanced sleep-wake phase disorder, shift-work disorder, and non-24-h sleep disorder where the circadian phase (timing relative to clock time), amplitude of the rhythm, and/or period (duration of the circadian cycle) are poorly aligned with wake activities and environmental time cues, leading to disrupted sleep (Micic et al., 2016; James et al., 2017). Fortunately, disrupted circadian rhythms are treatable to improve sleep (Dodson and Zee, 2010).
Given the major role of circadian rhythms in mediating sleep patterns and behavior, methods to assess circadian rhythms across the different manifestations of sleep disruption are likely to be insightful. In chronic insomnia, circadian rhythm factors may importantly contribute to the underlying etiology and pathophysiology (Lack et al., 2008). Chronobiological interventions, such as bright light therapy, have been administered as a stand-alone treatment and combined with CBT-I to moderate effect (Jankù et al., 2020). Circadian rhythms could also play a role in OSA (von Allmen et al., 2018) and comorbid insomnia and OSA (COMISA) (Sweetman et al., 2021). Effects of circadian rhythms on respiratory control (Stephenson, 2003; Yamauchi et al., 2014) and hypoxia (von Allmen et al., 2018) have also been hypothesized and supported by recent evidence of circadian modulation of the key OSA endotypes (El-Chami et al., 2014, 2015; Puri et al., 2020). Circadian rhythms also have an influential effect on metabolism, diabetes, cardiovascular disorders, obesity, and the efficacy of a range of pharmacological interventions; factors often applicable to sleep disorder cohorts (Guo and Stein, 2003; Frazier and Chang, 2020; Ayyar and Sukumaran, 2021). Therefore, strategies to better define sleep disruption that incorporate circadian rhythm assessments have significant potential to improve diagnostic and targeted therapy outcomes.
The current “gold standard” measure of circadian rhythms is salivary or blood dim-light melatonin onset (Arendt et al., 1985; Benloucif et al., 2008). This method involves measuring the concentration of melatonin (in pmol/mL) via a blood draw or via half-hourly saliva samples for at least 3–4 h before bedtime, under dim-light conditions (light intensity < 10 lux) while the individual remains relatively stationary and avoids consuming food and drinks (Sletten et al., 2018). Samples are processed and analyzed to estimate the clock time of melatonin rise onset (>10 pmol/mL), which is a marker of circadian phase. Another common measure of circadian rhythms in sleep research is core body temperature via an ingestible capsule or rectal thermistor. Frequent sampling of temperature across an extended period (>24 h), where conditions and activities that affect body temperature are controlled (e.g., air temperature, body movement, food consumption, and hot drink consumption), enables assessment of several aspects of the underlying core body temperature rhythm, including circadian phase, amplitude, and period. However, these assessments require carefully controlled laboratory conditions and access to specialized equipment generally infeasible for routine administration outside of circadian rhythm-focused sleep research studies. Fortunately, technologies and analytical methodologies are emerging that promise to facilitate simpler and improved assessments of circadian rhythms.
Emerging methods include advanced monitoring devices and biomathematical modeling to infer circadian rhythm metrics (Reid, 2019). Newer technologies include skin temperature sensors incorporated into consumer sleep trackers that detect the peripheral temperature rhythm to estimate circadian phase (Hasselberg et al., 2013). Electronic chips that can be implanted in body patches are also being developed to assess the cortisol rhythm via sweat (Upasham and Prasad, 2020), as well as other important clinical indicators such as the cortisol awakening response (Law and Clow, 2020). Rather than direct assessment of circadian rhythms, another approach is to infer circadian timing via the measurement of factors associated with circadian rhythms. Sleep timing data collected from wearable and non-wearable sleep trackers over an extended period are being incorporated into biomathematical models to infer circadian timing, since rest-activity rhythms are highly correlated with circadian timing (Cheng et al., 2021). Light sensors incorporated into newer wearable devices are also being used to infer circadian timing (Stone et al., 2020), since light is the strongest exogenous influencer (zeitgeber) of circadian rhythms. This information, potentially coupled with pupillometry assessment of an individual’s retinal responsiveness to light, enables inference of circadian timing, which may be useful for the diagnosis of circadian disruption in sleep disorders. More recent discoveries of genes with circadian oscillations (clock-controlled genes) raises the possibility that certain aspects of circadian rhythms may be amenable to assessment from blood samples (Cogswell et al., 2020). As these newer technologies mature, their implementation in clinical and research practice may result in new discoveries regarding the role of circadian rhythms in sleep disorders and their health-related consequences.
The sleeping environment affects sleep ability, but is minimally assessed in routine clinical practice. Consequently, sleep disruption may be misattributed to endogenous factors alone, ignoring the potential impact of exogenous factors. These include noise, light, temperature, and other factors that impact comfort within the sleep context. In a laboratory environment, these factors are typically well-controlled and designed to be conducive for sleep. However, as the assessment of sleep disruption shifts from the laboratory to the less well-controlled home environment, the assessment and consideration of environmental factors becomes increasingly important to understand mechanisms of sleep disruption.
Potentially the strongest exogenous influencer of sleep is noise, which can adversely affect sleep attainment and maintenance and fragment sleep to reduce total sleep time and quality (Muzet, 2007; Basner et al., 2014). The most common self-reported outcomes in response to road, rail and aircraft noise exposure are awakenings from sleep, increased sleep latency, and disruption to sleep continuity (Basner and McGuire, 2018). For example, patients in hospital intensive care units consistently rate noise as the most sleep disturbing factor (Freedman et al., 2001; Gabor et al., 2003; Elliott et al., 2013) and polysomnography results indicate poor and fragmented sleep, with a median of only 5 h sleep/24 h, only 3 min of uninterrupted light sleep and almost total abolition of deep and REM sleep (Elliott et al., 2014). However, to date, noise is rarely assessed as a potential sleep disturbing factor in either clinical or home setting contexts. Studies that have investigated the effects of noise on sleep quality have employed generalized metrics that focus on overall noise levels only and/or do not consider specific noise characteristics such as spectral content, time varying noise components, tonality and noise intermittency. These factors are important contributors to noise annoyance (Ioannidou et al., 2016; Schäffer et al., 2016; Oliva et al., 2017), are thus likely to contribute to sleep disturbance, and warrant assessment to better inform clinical decision-making.
There are two seemingly opposing challenges regarding sleep monitoring and diagnostics. There is a need for greater in-depth insight into the underlying neurobiology of sleep, yet there is also a need for less intrusive and user-friendly technology. Detailed, in-depth assessments and monitoring approaches as well as smarter use of existing signals and information derived from traditional polysomnography approaches are required to better understand sleep pathology. Yet, given the burden of disease and the scale of sleep disruption in the community, there is also a pressing need for less intrusive sleep tracking technology that can be readily and easily adopted in a home-based setting.
A plethora of technologies have emerged to track sleep in the home setting (Figure 6). These include bedside Doppler (Zakrzewski et al., 2015; Tuominen et al., 2019) and instrumented mattresses for ballistographic assessment of heart rate, respiratory rate and body movements/position, which perform relatively well compared to polysomnography and are considerably easier to implement and use (Laurino et al., 2020). Similarly, wearable devices such as smart watches, rings, simplified EEG headbands, and actigraphy devices also provide similar performance in sleep/wake assessment (Griessenberger et al., 2013; de Zambotti et al., 2018a; Arnal et al., 2020; Chee et al., 2021; Scott et al., 2021). Infrared video has also been used to classify body motion to automatically score sleep and wake states (Wang et al., 2013), as well as monitor respiration, head posture, and body posture to detect abnormal breathing (Deng et al., 2018). Together, these devices open new pathways for non-invasive multi-night assessments in various sleep settings to support the clinical diagnosis and management of sleep disorders. This is especially important given that sleep disorder pathophysiology may show large variability between nights (Punjabi et al., 2020), and that variability and irregularity in some sleep components has been associated with downstream effects on health such as cardio-metabolic conditions (Linz et al., 2019a,b; Huang et al., 2020).
Figure 6. Schematic of novel and emerging approaches to monitor the sleeping environment and track key health measures via “the bedroom of the future.” Refer to the text for further detail.
New and emerging approaches to better define sleep and circadian disruption and its consequences offers considerable promise to move beyond the limitations of current sleep metrics and management. To improve outcomes, these approaches need to be underpinned by consideration for underlying neurobiology and will likely require a multisystem approach to capture the diverse impacts that sleep and circadian disruption can have on health and wellbeing. Development of practical, inexpensive methods to assess sleep and circadian disruption, its key contributors, and consequences at scale, including comprehensive, long-term remote monitoring has the potential to transform sleep medicine and management. This includes implementation of precision sleep medicine and targeted therapy approaches.
All authors contributed to drafting and/or revising one or more of the sections of this manuscript, provided feedback on the final version, and agreed to be accountable for the content of the work.
KH was supported by an Australian Research Council Discovery Early Career Researcher Award (DE180100022). AV was supported by a National Health and Medical Research Council (NHMRC) of Australia Career Development Fellowship (1159499). DE was supported by a NHMRC of Australia Senior Research Fellowship (1116942) and an Investigator Grant (1196261). BL was supported by the Nick Antic Sleep Research Scholarship from Flinders Foundation. This was an investigator-initiated, non-commercially funded study.
DE has a Collaborative Research Centre (CRC-P) Grant, a consortium grant between the Australian Government, Academia and Industry (Industry partner: Oventus Medical) and has research grants and serves as a consultant for Bayer and Apnimed. AV has received research grant funding and equipment from ResMed and Philips Respironics. PC has received research funding from Defence Science and Technology and received research grant funding and equipment from Philips Respironics. The funders Philips Respironics, ResMed, Oventus Medical, Bayer, Apnimed were not involved in the study design, collection, analysis, interpretation of data, the writing of this article or the decision to submit it for publication.
The remaining authors declare that the research was conducted in the absence of any commercial or financial relationships that could be construed as a potential conflict of interest.
All claims expressed in this article are solely those of the authors and do not necessarily represent those of their affiliated organizations, or those of the publisher, the editors and the reviewers. Any product that may be evaluated in this article, or claim that may be made by its manufacturer, is not guaranteed or endorsed by the publisher.
Adams, R. J., Appleton, S. L., Vakulin, A., Lang, C., Martin, S. A., Taylor, A. W., et al. (2016). Association of daytime sleepiness with obstructive sleep apnoea and comorbidities varies by sleepiness definition in a population cohort of men. Respirology 21, 1314–1321. doi: 10.1111/resp.12829
Aishah, A., and Eckert, D. J. (2019). Phenotypic approach to pharmacotherapy in the management of obstructive sleep apnoea. Curr. Opin. Pulmonary Med. 25, 594–601. doi: 10.1097/MCP.0000000000000628
Appleton, S. L., Vakulin, A., D’Rozario, A., Vincent, A. D., Teare, A., Martin, S. A., et al. (2019). Quantitative electroencephalography measures in rapid eye movement and nonrapid eye movement sleep are associated with apnea–hypopnea index and nocturnal hypoxemia in men. Sleep 42:zsz092. doi: 10.1093/sleep/zsz092
Arendt, J., Bojkowski, C., Franey, C., Wright, J., and Marks, V. (1985). Immunoassay of 6-hydroxymelatonin sulfate in human plasma and urine: abolition of the urinary 24-hour rhythm with atenolol. J. Clin. Endocrinol. Metab. 60, 1166–1173. doi: 10.1210/jcem-60-6-1166
Arnal, P. J., Thorey, V., Debellemaniere, E., Ballard, M. E., Bou Hernandez, A., Guillot, A., et al. (2020). The Dreem Headband compared to polysomnography for electroencephalographic signal acquisition and sleep staging. Sleep 43:zsaa097. doi: 10.1093/sleep/zsaa097
Ayache, M., and Strohl, K. P. (2018). High interrater reliability of overnight pulse oximetry interpretation among inexperienced physicians using a structured template. J. Clin. Sleep Med. 14, 541–548. doi: 10.5664/jcsm.7040
Ayyar, V. S., and Sukumaran, S. (2021). Circadian rhythms: influence on physiology, pharmacology, and therapeutic interventions. J. Pharmacokinet. Pharmacodyn. 48, 321–338. doi: 10.1007/s10928-021-09751-2
Azarbarzin, A., Sands, S. A., Stone, K. L., Taranto-Montemurro, L., Messineo, L., Terrill, P. I., et al. (2019). The hypoxic burden of sleep apnoea predicts cardiovascular disease-related mortality: the Osteoporotic Fractures in Men Study and the Sleep Heart Health Study. Eur. Heart J. 40, 1149–1157. doi: 10.1093/eurheartj/ehy624
Azarbarzin, A., Sands, S. A., Taranto-Montemurro, L., Vena, D., Sofer, T., Kim, S.-W., et al. (2020). The sleep apnea-specific hypoxic burden predicts incident heart failure. Chest 158, 739–750. doi: 10.1016/j.chest.2020.03.053
Azarbarzin, A., Younes, M., Sands, S. A., Wellman, A., Redline, S., Czeisler, C. A., et al. (2021). Interhemispheric sleep depth coherence predicts driving safety in sleep apnea. J. Sleep Res. 30:e13092. doi: 10.1111/jsr.13092
Bartscha, R., Schumann, A., Kantelhardt, J. W., Penzel, T., and Ivanov, P. C. (2012). Phase transitions in physiologic coupling. Proc. Natl. Acad. Sci. U.S.A. 9, 10181–10186. doi: 10.1073/pnas.1204568109
Bashan, A., Bartsch, R., Kantelhardt, J. W., Havlin, S., and Ivanov, P. C. (2012). Network physiology reveals relations between network topology and physiological function. Nat. Commun. 3:702. doi: 10.1038/ncomms1705
Basner, M., Babisch, W., Davis, A., Brink, M., Clark, C., Janssen, S., et al. (2014). Auditory and non-auditory effects of noise on health. Lancet 383, 1325–1332. doi: 10.1016/S0140-6736(13)61613-X
Basner, M., and McGuire, S. (2018). WHO environmental noise guidelines for the European region: a systematic review on environmental noise and effects on sleep. Int. J. Environ. Res. Public Health 15:519. doi: 10.3390/ijerph15030519
Baumert, M., Immanuel, S. A., Stone, K. L., Litwack Harrison, S., Redline, S., Mariani, S., et al. (2020). Composition of nocturnal hypoxaemic burden and its prognostic value for cardiovascular mortality in older community-dwelling men. Eur. Heart J. 41, 533–541. doi: 10.1093/eurheartj/ehy838
Benjafield, A. V., Ayas, N. T., Eastwood, P. R., Heinzer, R., Ip, M. S., Morrell, M. J., et al. (2019). Estimation of the global prevalence and burden of obstructive sleep apnoea: a literature-based analysis. Lancet Respiratory Med. 7, 687–698. doi: 10.1016/S2213-2600(19)30198-5
Benloucif, S., Burgess, H. J., Klerman, E. B., Lewy, A. J., Middleton, B., Murphy, P. J., et al. (2008). Measuring melatonin in humans. J. Clin. Sleep Med. 4, 66–69. doi: 10.5664/jcsm.27083
Benveniste, H., Elkin, R., Heerdt, P. M., Koundal, S., Xue, Y., Lee, H., et al. (2020). The glymphatic system and its role in cerebral homeostasis. J. Appl. Physiol. 129, 1330–1340. doi: 10.1152/japplphysiol.00852.2019
Berry, R. B., Brooks, R., Gamaldo, C., Harding, S. M., Lloyd, R. M., Quan, S. F., et al. (2017). AASM Scoring Manual Updates for 2017 (version 2.4). Darien, IL: American Academy of Sleep Medicine. doi: 10.5664/jcsm.6576
Bersagliere, A., and Achermann, P. (2010). Slow oscillations in human non-rapid eye movement sleep electroencephalogram: effects of increased sleep pressure. J. Sleep Res. 19, 228–237. doi: 10.1111/j.1365-2869.2009.00775.x
Besio, W., Aakula, R., Koka, K., and Dai, W. (2006). Development of a tri-polar concentric ring electrode for acquiring accurate Laplacian body surface potentials. Ann. Biomed. Eng. 34, 426–435. doi: 10.1007/s10439-005-9054-8
Boeve, B. F., Molano, J. R., Ferman, T. J., Smith, G. E., Lin, S.-C., Bieniek, K., et al. (2011). Validation of the Mayo Sleep Questionnaire to screen for REM sleep behavior disorder in an aging and dementia cohort. Sleep Med. 12, 445–453. doi: 10.1016/j.sleep.2010.12.009
Brandenberger, G., Ehrhart, J., Piquard, F., and Simon, C. (2001). Inverse coupling between ultradian oscillations in delta wave activity and heart rate variability during sleep. Clin. Neurophysiol. 112, 992–996. doi: 10.1016/S1388-2457(01)00507-7
Braun, M., and Iliff, J. J. (2020). The impact of neurovascular, blood-brain barrier, and glymphatic dysfunction in neurodegenerative and metabolic diseases. Int. Rev. Neurobiol. 154, 413–436. doi: 10.1016/bs.irn.2020.02.006
Burke, P., Carter, S., Knapman, F., Patti, J., Butlin, M., Gandevia, S., et al. (2020). Nocturnal swallowing augments arousal intensity and arousal tachycardia. Proc. Natl. Acad. Sci. 117, 8624–8632. doi: 10.1073/pnas.1907393117
Butler, M. P., Emch, J. T., Rueschman, M., Sands, S. A., Shea, S. A., Wellman, A., et al. (2019). Apnea-Hypopnea event duration predicts mortality in men and women in the sleep heart health study. Am. J. Respir. Crit. Care Med. 199, 903–912. doi: 10.1164/rccm.201804-0758OC
Buysse, D. J., Hall, M. L., Strollo, P. J., Kamarck, T. W., Owens, J., Lee, L., et al. (2008). Relationships between the Pittsburgh Sleep Quality Index (PSQI), Epworth Sleepiness Scale (ESS), and clinical/polysomnographic measures in a community sample. J. Clin. Sleep Med. 4, 563–571. doi: 10.5664/jcsm.27351
Buysse, D. J., Yu, L., Moul, D. E., Germain, A., Stover, A., Dodds, N. E., et al. (2010). Development and validation of patient-reported outcome measures for sleep disturbance and sleep-related impairments. Sleep 33, 781–792. doi: 10.1093/sleep/33.6.781
Cajochen, C., Kräuchi, K., and Wirz-Justice, A. (2003). Role of melatonin in the regulation of human circadian rhythms and sleep. J. Neuroendocrinol. 15, 432–437. doi: 10.1046/j.1365-2826.2003.00989.x
Carberry, J. C., Amatoury, J., and Eckert, D. J. (2018). Personalized management approach for OSA. Chest 153, 744–755. doi: 10.1016/j.chest.2017.06.011
Catcheside, P. G., Chiong, S. C., Orr, R. S., Mercer, J., Saunders, N. A., and McEvoy, R. D. (2001). Acute cardiovascular responses to arousal from non-REM sleep during normoxia and hypoxia. Sleep 24, 895–902. doi: 10.1093/sleep/24.8.895
Catcheside, P. G., Orr, R. S., Chiong, S. C., Mercer, J., Saunders, N. A., and McEvoy, R. D. (2002). Noninvasive cardiovascular markers of acoustically induced arousal from non–rapid-eye-movement sleep. Sleep 25, 797–804. doi: 10.1093/sleep/25.7.797
Chambon, S., Galtier, M. N., Arnal, P. J., Wainrib, G., and Gramfort, A. (2018). A deep learning architecture for temporal sleep stage classification using multivariate and multimodal time series. IEEE Trans. Neural Syst. Rehabil. Eng. 26, 758–769. doi: 10.1109/TNSRE.2018.2813138
Chee, N. I., Ghorbani, S., Golkashani, H. A., Leong, R. L., Ong, J. L., and Chee, M. W. (2021). Multi-night validation of a sleep tracking ring in adolescents compared with a research actigraph and polysomnography. Nat. Sci. Sleep 13:177. doi: 10.2147/NSS.S286070
Cheng, P., Walch, O., Huang, Y., Mayer, C., Sagong, C., Cuamatzi Castelan, A., et al. (2021). Predicting circadian misalignment with wearable technology: validation of wrist-worn actigraphy and photometry in night shift workers. Sleep 44:zsaa180. doi: 10.1093/sleep/zsaa180
Chinoy, E. D., Frey, D. J., Kaslovsky, D. N., Meyer, F. G., and Wright, K. P. Jr. (2014). Age-related changes in slow wave activity rise time and NREM sleep EEG with and without zolpidem in healthy young and older adults. Sleep Med. 15, 1037–1045. doi: 10.1016/j.sleep.2014.05.007
Christensen, J. A., Nikolic, M., Warby, S. C., Koch, H., Zoetmulder, M., Frandsen, R., et al. (2015). Sleep spindle alterations in patients with Parkinson’s disease. Front. Hum. Neurosci. 9:233. doi: 10.3389/fnhum.2015.00233
Claassen, D. O., Josephs, K. A., Ahlskog, J. E., Silber, M. H., Tippmann-Peikert, M., and Boeve, B. F. (2010). REM sleep behavior disorder preceding other aspects of synucleinopathies by up to half a century. Neurology 75, 494–499. doi: 10.1212/WNL.0b013e3181ec7fac
Cogswell, D., Bisesi, P., Markwald, R., Cruickshank-Quinn, C., Quinn, K., McHill, A., et al. (2020). 0050 identification of a plasma metabolome-based biomarker for dim-light melatonin offset and onset in humans. Sleep 43(Suppl. 1), A20–A21. doi: 10.1093/sleep/zsaa056.049
Colrain, I. M. (2005). The K-complex: a 7-decade history. Sleep 28, 255–273. doi: 10.1093/sleep/28.2.255
Daan, S., Beersma, D., and Borbély, A. A. (1984). Timing of human sleep: recovery process gated by a circadian pacemaker. Am. J. Physiol.-Regulat., Integrat. Comparat. Physiol. 246, R161–R183. doi: 10.1152/ajpregu.1984.246.2.R161
de Zambotti, M., Trinder, J., Silvani, A., Colrain, I. M., and Baker, F. C. (2018b). Dynamic coupling between the central and autonomic nervous systems during sleep: a review. Neurosci. Biobehav. Rev. 90, 84–103. doi: 10.1016/j.neubiorev.2018.03.027
de Zambotti, M., Goldstone, A., Claudatos, S., Colrain, I. M., and Baker, F. C. (2018a). A validation study of Fitbit Charge 2TM compared with polysomnography in adults. Chronobiol. Int. 35, 465–476. doi: 10.1080/07420528.2017.1413578
Deng, F., Dong, J., Wang, X., Fang, Y., Liu, Y., Yu, Z., et al. (2018). Design and implementation of a noncontact sleep monitoring system using infrared cameras and motion sensor. IEEE Trans. Instrument. Measurement 67, 1555–1563. doi: 10.1109/TIM.2017.2779358
Depner, C. M., Cheng, P. C., Devine, J. K., Khosla, S., De Zambotti, M., Robillard, R., et al. (2020). Wearable technologies for developing sleep and circadian biomarkers: a summary of workshop discussions. Sleep 43:zsz254. doi: 10.1093/sleep/zsz254
Diekelmann, S., and Born, J. (2010). The memory function of sleep. Nat. Rev. Neurosci. 11, 114–126. doi: 10.1038/nrn2762
Dimitrov, T., He, M., Stickgold, R., and Prerau, M. J. (2021). Sleep spindles comprise a subset of a broader class of electroencephalogram events. Sleep 44:zsab099. doi: 10.1093/sleep/zsab099
Djonlagic, I., Mariani, S., Fitzpatrick, A. L., Van Der Klei, V. M., Johnson, D. A., Wood, A. C., et al. (2021). Macro and micro sleep architecture and cognitive performance in older adults. Nat. Hum. Behav. 5, 123–145. doi: 10.1038/s41562-020-00964-y
Dodson, E. R., and Zee, P. C. (2010). Therapeutics for circadian rhythm sleep disorders. Sleep Med. Clinics 5, 701–715. doi: 10.1016/j.jsmc.2010.08.001
D’Rozario, A. L., Cross, N. E., Vakulin, A., Bartlett, D. J., Wong, K. K., Wang, D., et al. (2017). Quantitative electroencephalogram measures in adult obstructive sleep apnea–potential biomarkers of neurobehavioural functioning. Sleep Med. Rev. 36, 29–42. doi: 10.1016/j.smrv.2016.10.003
Dutta, R., Delaney, G., Toson, B., Jordan, A. S., White, D. P., Wellman, A., et al. (2021). A novel model to estimate key obstructive sleep apnea endotypes from standard polysomnography and clinical data and their contribution to obstructive sleep apnea severity. Ann. Am. Thoracic Soc. 18, 656–667. doi: 10.1513/AnnalsATS.202001-064OC
Eckert, D. J. (2018a). Phenotypic approaches to obstructive sleep apnoea–new pathways for targeted therapy. Sleep Med. Rev. 37, 45–59. doi: 10.1016/j.smrv.2016.12.003
Eckert, D. J. (2018b). Phenotypic approaches to positional therapy for obstructive sleep apnoea. Sleep Med. Rev. 37, 175–176. doi: 10.1016/j.smrv.2017.06.007
Eckert, D. J., Owens, R. L., Kehlmann, G. B., Wellman, A., Rahangdale, S., Yim-Yeh, S., et al. (2011). Eszopiclone increases the respiratory arousal threshold and lowers the apnoea/hypopnoea index in obstructive sleep apnoea patients with a low arousal threshold. Clin. Sci. 120, 505–514. doi: 10.1042/CS20100588
Eckert, D. J., White, D. P., Jordan, A. S., Malhotra, A., and Wellman, A. (2013). Defining phenotypic causes of obstructive sleep apnea. Identification of novel therapeutic targets. Am. J. Respiratory Critical Care Med. 188, 996–1004. doi: 10.1164/rccm.201303-0448OC
Edwards, B. A., Sands, S. A., Owens, R. L., Eckert, D. J., Landry, S., White, D. P., et al. (2016b). The combination of supplemental oxygen and a hypnotic markedly improves obstructive sleep apnea in patients with a mild to moderate upper airway collapsibility. Sleep 39, 1973–1983. doi: 10.5665/sleep.6226
Edwards, B. A., Andara, C., Landry, S., Sands, S. A., Joosten, S. A., Owens, R. L., et al. (2016a). Upper-Airway collapsibility and loop gain predict the response to oral appliance therapy in patients with obstructive sleep apnea. Am. J. Respir. Crit. Care Med. 194, 1413–1422. doi: 10.1164/rccm.201601-0099OC
Edwards, B. A., Eckert, D. J., McSharry, D. G., Sands, S. A., Desai, A., Kehlmann, G., et al. (2014). Clinical predictors of the respiratory arousal threshold in patients with obstructive sleep apnea. Am. J. Respiratory Crit. Care Med. 190, 1293–1300. doi: 10.1164/rccm.201404-0718OC
Edwards, B. A., Sands, S. A., Eckert, D. J., White, D. P., Butler, J. P., Owens, R. L., et al. (2012). Acetazolamide improves loop gain but not the other physiological traits causing obstructive sleep apnoea. J. Physiol. 590, 1199–1211. doi: 10.1113/jphysiol.2011.223925
El-Chami, M., Shaheen, D., Ivers, B., Syed, Z., Badr, M. S., Lin, H.-S., et al. (2014). Time of day affects chemoreflex sensitivity and the carbon dioxide reserve during NREM sleep in participants with sleep apnea. J. Appl. Physiol. 117, 1149–1156. doi: 10.1152/japplphysiol.00681.2014
El-Chami, M., Shaheen, D., Ivers, B., Syed, Z., Badr, M. S., Lin, H.-S., et al. (2015). Time of day affects the frequency and duration of breathing events and the critical closing pressure during NREM sleep in participants with sleep apnea. J. Appl. Physiol. 119, 617–626. doi: 10.1152/japplphysiol.00346.2015
Elliott, R., McKinley, S., Cistulli, P., and Fien, M. (2013). Characterisation of sleep in intensive care using 24-hour polysomnography: anobservational study. Crit. Care 17, 1–10. doi: 10.1186/cc12565
Elliott, R., Rai, T., and McKinley, S. (2014). Factors affecting sleep in the critically ill: an observational study. J. Crit. Care 29, 859–863. doi: 10.1016/j.jcrc.2014.05.015
Fiorillo, L., Puiatti, A., Papandrea, M., Ratti, P.-L., Favaro, P., Roth, C., et al. (2019). Automated sleep scoring: a review of the latest approaches. Sleep Med. Rev. 48:101204. doi: 10.1016/j.smrv.2019.07.007
Fischer, C., and Penzel, T. (2019). Continuous non-invasive determination of nocturnal blood pressure variation using photoplethysmographic pulse wave signals: comparison of pulse propagation time, pulse transit time and RR-interval. Physiol. Measurement 40:014001. doi: 10.1088/1361-6579/aaf298
Frauscher, B., Iranzo, A., Gaig, C., Gschliesser, V., Guaita, M., Raffelseder, V., et al. (2012). Normative EMG values during REM sleep for the diagnosis of REM sleep behavior disorder. Sleep 35, 835–847. doi: 10.5665/sleep.1886
Frazier, K., and Chang, E. B. (2020). Intersection of the gut microbiome and circadian rhythms in metabolism. Trends Endocrinol. Metab. 31, 25–36. doi: 10.1016/j.tem.2019.08.013
Freedman, N. S., Gazendam, J., Levan, L., Pack, A. I., and Schwab, R. J. (2001). Abnormal sleep/wake cycles and the effect of environmental noise on sleep disruption in the intensive care unit. Am. J. Respiratory Crit. Care Med. 163, 451–457. doi: 10.1164/ajrccm.163.2.9912128
Fultz, N. E., Bonmassar, G., Setsompop, K., Stickgold, R. A., Rosen, B. R., Polimeni, J. R., et al. (2019). Coupled electrophysiological, hemodynamic, and cerebrospinal fluid oscillations in human sleep. Science 366, 628–631. doi: 10.1126/science.aax5440
Gabor, J. Y., Cooper, A. B., Crombach, S. A., Lee, B., Kadikar, N., Bettger, H. E., et al. (2003). Contribution of the intensive care unit environment to sleep disruption in mechanically ventilated patients and healthy subjects. Am. J. Respiratory Crit. Care Med. 167, 708–715. doi: 10.1164/rccm.2201090
Gorgoni, M., Lauri, G., Truglia, I., Cordone, S., Sarasso, S., Scarpelli, S., et al. (2016). Parietal fast sleep spindle density decrease in Alzheimer’s disease and amnesic mild cognitive impairment. Neural Plasticity 2016:8376108. doi: 10.1155/2016/8376108
Gray, E. L., McKenzie, D. K., and Eckert, D. J. (2017). Obstructive sleep apnea without obesity is common and difficult to treat: evidence for a distinct pathophysiological phenotype. J. Clin. Sleep Med.: JCSM : Off. Public. Am. Acad. Sleep Med. 13, 81–88. doi: 10.5664/jcsm.6394
Griefahn, B., Bröde, P., Marks, A., and Basner, M. (2008). Autonomic arousals related to traffic noise during sleep. Sleep 31, 569–577. doi: 10.1093/sleep/31.4.569
Griessenberger, H., Heib, D. P. J., Kunz, A., Hödlmoser, K., and Schabus, M. (2013). Assessment of a wireless headband for automatic sleep scoring. Sleep Breathing 17, 747–752. doi: 10.1007/s11325-012-0757-4
Guo, Y.-F., and Stein, P. K. (2003). Circadian rhythm in the cardiovascular system: chronocardiology. Am. Heart J. 145, 779–786. doi: 10.1016/S0002-8703(02)94797-6
Hahn, M. A., Heib, D., Schabus, M., Hoedlmoser, K., and Helfrich, R. F. (2020). Slow oscillation-spindle coupling predicts enhanced memory formation from childhood to adolescence. Elife 9:e53730. doi: 10.7554/eLife.53730.sa2
Hartmann, S., and Baumert, M. (2019). Automatic a-phase detection of cyclic alternating patterns in sleep using dynamic temporal information. IEEE Trans. Neural Syst. Rehabil. Eng. 27, 1695–1703. doi: 10.1109/TNSRE.2019.2934828
Hartmann, S., Bruni, O., Ferri, R., Redline, S., and Baumert, M. (2020). Characterization of cyclic alternating pattern during sleep in older men and women using large population studies. Sleep 43:zsaa016. doi: 10.1093/sleep/zsaa016
Harvey, A. G., and Tang, N. K. (2003). Cognitive behaviour therapy for primary insomnia: can we rest yet? Sleep Med. Rev. 7, 237–262. doi: 10.1053/smrv.2002.0266
Hasselberg, M. J., McMahon, J., and Parker, K. (2013). The validity, reliability, and utility of the iButton® for measurement of body temperature circadian rhythms in sleep/wake research. Sleep Med. 14, 5–11. doi: 10.1016/j.sleep.2010.12.011
Helfrich, R. F., Lendner, J. D., Mander, B. A., Guillen, H., Paff, M., Mnatsakanyan, L., et al. (2019). Bidirectional prefrontal-hippocampal dynamics organize information transfer during sleep in humans. Nat. Commun. 10, 1–16. doi: 10.1038/s41467-019-11444-x
Hennies, N., Ralph, M. A. L., Kempkes, M., Cousins, J. N., and Lewis, P. A. (2016). Sleep spindle density predicts the effect of prior knowledge on memory consolidation. J. Neurosci. 36, 3799–3810. doi: 10.1523/JNEUROSCI.3162-15.2016
Hirotsu, C., Betta, M., Bernardi, G., Marques-Vidal, P., Vollenweider, P., Waeber, G., et al. (2020). Pulse wave amplitude drops during sleep: clinical significance and characteristics in a general population sample. Sleep 43:zsz322. doi: 10.1093/sleep/zsz322
Huang, T., Mariani, S., and Redline, S. (2020). Sleep irregularity and risk of cardiovascular events: the multi-ethnic study of atherosclerosis. J. Am. College Cardiol. 75, 991–999. doi: 10.1016/j.jacc.2019.12.054
Ioannidou, C., Santurette, S., and Jeong, C.-H. (2016). Effect of modulation depth, frequency, and intermittence on wind turbine noise annoyance. J. Acoustical Soc. Am. 139, 1241–1251. doi: 10.1121/1.4944570
Ivanov, P. C., Liu, K. K., and Bartsch, R. P. (2016). Focus on the emerging new fields of network physiology and network medicine. New J. Phys. 18:100201. doi: 10.1088/1367-2630/18/10/100201
James, S. M., Honn, K. A., Gaddameedhi, S., and Van Dongen, H. P. (2017). Shift work: disrupted circadian rhythms and sleep—implications for health and well-being. Curr. Sleep Med. Rep. 3, 104–112. doi: 10.1007/s40675-017-0071-6
Jankù, K., Šmotek, M., Fárková, E., and Kopøivová, J. (2020). Block the light and sleep well: Evening blue light filtration as a part of cognitive behavioral therapy for insomnia. Chronobiol. Int. 37, 248–259. doi: 10.1080/07420528.2019.1692859
Jordan, A. S., Eckert, D. J., Catcheside, P. G., and McEvoy, R. D. (2003). Ventilatory response to brief arousal from non–rapid eye movement sleep is greater in men than in women. Am. J. Respiratory Crit. Care Med. 168, 1512–1519. doi: 10.1164/rccm.200302-150OC
Kim, J., Campbell, A. S., de Ávila, B. E.-F., and Wang, J. (2019). Wearable biosensors for healthcare monitoring. Nat. Biotechnol. 37, 389–406. doi: 10.1038/s41587-019-0045-y
Kirby, S. C., Anderson, W. M., Chesson, A. L., and George, R. B. (1992). Computer quantitation of saturation impairment time as an index of oxygenation during sleep. Comput. Methods Programs Biomed. 38, 107–115. doi: 10.1016/0169-2607(92)90081-H
Kondratova, A. A., and Kondratov, R. V. (2012). The circadian clock and pathology of the ageing brain. Nat. Rev. Neurosci. 13, 325–335. doi: 10.1038/nrn3208
Krystal, A. D., and Edinger, J. D. (2010). Sleep EEG predictors and correlates of the response to cognitive behavioral therapy for insomnia. Sleep 33, 669–677. doi: 10.1093/sleep/33.5.669
Krystal, A. D., Edinger, J. D., Wohlgemuth, W. K., and Marsh, G. R. (2002). NREM sleep EEG frequency spectral correlates of sleep complaints in primary insomnia subtypes. Sleep 25, 626–636.
Kwon, Y., Wiles, C., Parker, B. E., Clark, B. R., Sohn, M.-W., Mariani, S., et al. (2021). Pulse arrival time, a novel sleep cardiovascular marker: the multi-ethnic study of atherosclerosis. Thorax [Online ahead of print] doi: 10.1136/thoraxjnl-2020-216399
Lack, L. C., Gradisar, M., Van Someren, E. J., Wright, H. R., and Lushington, K. (2008). The relationship between insomnia and body temperatures. Sleep Med. Rev. 12, 307–317. doi: 10.1016/j.smrv.2008.02.003
Lacourse, K., Yetton, B., Mednick, S., and Warby, S. C. (2020). Massive online data annotation, crowdsourcing to generate high quality sleep spindle annotations from EEG data. Sci. Data 7, 1–14. doi: 10.1038/s41597-020-0533-4
Lafortune, M., Gagnon, J. F., Martin, N., Latreille, V., Dubé, J., Bouchard, M., et al. (2014). Sleep spindles and rapid eye movement sleep as predictors of next morning cognitive performance in healthy middle-aged and older participants. J. Sleep Res. 23, 159–167. doi: 10.1111/jsr.12108
Landry, S. A., Joosten, S. A., Eckert, D. J., Jordan, A. S., Sands, S. A., White, D. P., et al. (2017). Therapeutic CPAP level predicts upper airway collapsibility in patients with obstructive sleep apnea. Sleep 40:zsx056. doi: 10.1093/sleep/zsx056
Laurino, M., Arcarisi, L., Carbonaro, N., Gemignani, A., Menicucci, D., and Tognetti, A. (2020). A smart bed for non-obtrusive sleep analysis in real world context. IEEE Access 8, 45664–45673. doi: 10.1109/ACCESS.2020.2976194
Law, R., and Clow, A. (2020). Stress, the cortisol awakening response and cognitive function. Int. Rev. Neurobiol. 150, 187–217. doi: 10.1016/bs.irn.2020.01.001
Lazar, A. S., Lazar, Z. I., and Dijk, D.-J. (2015). Circadian regulation of slow waves in human sleep: topographical aspects. Neuroimage 116, 123–134. doi: 10.1016/j.neuroimage.2015.05.012
Lechat, B., Hansen, K., Catcheside, P., and Zajamsek, B. (2020). Beyond K-complex binary scoring during sleep: probabilistic classification using deep learning. Sleep 43:zsaa077. doi: 10.1093/sleep/zsaa077
Lechat, B., Hansen, K., Micic, G., Decup, F., Dunbar, C., Liebich, T., et al. (2021). K-complexes are a sensitive marker of noise-related sensory processing during sleep: a pilot study. Sleep 44:zsab065. doi: 10.1093/sleep/zsab065
Léger, D., Debellemaniere, E., Rabat, A., Bayon, V., Benchenane, K., and Chennaoui, M. (2018). Slow-wave sleep: from the cell to the clinic. Sleep Med. Rev. 41, 113–132. doi: 10.1016/j.smrv.2018.01.008
Lendner, J. D., Helfrich, R. F., Mander, B. A., Romundstad, L., Lin, J. J., Walker, M. P., et al. (2020). An electrophysiological marker of arousal level in humans. Elife 9:e55092. doi: 10.7554/eLife.55092.sa2
Levy, P., Pépin, J. L., Deschaux-Blanc, C., Paramelle, B., and Brambilla, C. (1996). Accuracy of oximetry for detection of respiratory disturbances in sleep apnea syndrome. Chest 109, 395–399. doi: 10.1378/chest.109.2.395
Li, S., Wing, Y., Lam, S., Zhang, J., Yu, M., Ho, C., et al. (2010). Validation of a new REM sleep behavior disorder questionnaire (RBDQ-HK). Sleep Med. 11, 43–48. doi: 10.1016/j.sleep.2009.06.008
Li, Y., Ye, J., Han, D., Cao, X., Ding, X., Zhang, Y., et al. (2017). Physiology-Based modeling may predict surgical treatment outcome for obstructive sleep apnea. J. Clin. Sleep Med. 13, 1029–1037. doi: 10.5664/jcsm.6716
Lim, D. C., Mazzotti, D. R., Sutherland, K., Mindel, J. W., Kim, J., Cistulli, P. A., et al. (2020). Reinventing polysomnography in the age of precision medicine. Sleep Med. Rev. 52:101313. doi: 10.1016/j.smrv.2020.101313
Lin, C.-L., Yeh, C., Yen, C.-W., Hsu, W.-H., and Hang, L.-W. (2009). Comparison of the indices of oxyhemoglobin saturation by pulse oximetry in obstructive sleep apnea hypopnea syndrome. Chest 135, 86–93. doi: 10.1378/chest.08-0057
Lindner, A., Fornadi, K., Lazar, A. S., Czira, M. E., Dunai, A., Zoller, R., et al. (2012). Periodic limb movements in sleep are associated with stroke and cardiovascular risk factors in patients with renal failure. J. Sleep Res. 21, 297–307. doi: 10.1111/j.1365-2869.2011.00956.x
Linz, D., Baumert, M., Desteghe, L., Kadhim, K., Vernooy, K., Kalman, J. M., et al. (2019a). Nightly sleep apnea severity in patients with atrial fibrillation: potential applications of long-term sleep apnea monitoring. IJC Heart Vasculature 24:100424. doi: 10.1016/j.ijcha.2019.100424
Linz, D., Brooks, A. G., Elliott, A. D., Nalliah, C. J., Hendriks, J. M., Middeldorp, M. E., et al. (2019b). Variability of sleep apnea severity and risk of atrial fibrillation: the VARIOSA-AF study. JACC: Clin. Electrophys. 5, 692–701. doi: 10.1016/j.jacep.2019.03.005
Linz, D., Loffler, K. A., Sanders, P., Catcheside, P., Anderson, C. S., Zheng, D., et al. (2020). Low prognostic value of novel nocturnal metrics in patients with OSA and high cardiovascular event risk: post hoc analyses of the SAVE Study. Chest 158, 2621–2631. doi: 10.1016/j.chest.2020.06.072
Liu, S., Pan, J., Lei, Q., He, L., Zhong, B., Meng, Y., et al. (2020). Spontaneous K-Complexes may be biomarkers of the progression of amnestic mild cognitive impairment. Sleep Med. 67, 99–109. doi: 10.1016/j.sleep.2019.10.015
Liu, Y., Pharr, M., and Salvatore, G. A. (2017). Lab-on-skin: a review of flexible and stretchable electronics for wearable health monitoring. ACS Nano 11, 9614–9635. doi: 10.1021/acsnano.7b04898
Lunsford-Avery, J. R., Edinger, J. D., and Krystal, A. D. (2021). Optimizing computation of overnight decline in delta power: evidence for slower rate of decline in delta power in insomnia patients. Clin. Neurophysiol. 132, 545–553. doi: 10.1016/j.clinph.2020.12.004
Ma, C., Pavlova, M., Liu, Y., Liu, Y., Huangfu, C., Wu, S., et al. (2017). Probable REM sleep behavior disorder and risk of stroke: a prospective study. Neurology 88, 1849–1855. doi: 10.1212/WNL.0000000000003902
Magalang, U. J., Chen, N.-H., Cistulli, P. A., Fedson, A. C., Gíslason, T., Hillman, D., et al. (2013). Agreement in the scoring of respiratory events and sleep among international sleep centers. Sleep 36, 591–596. doi: 10.5665/sleep.2552
Magalang, U. J., Dmochowski, J., Veeramachaneni, S., Draw, A., Mador, M. J., El-Solh, A., et al. (2003). Prediction of the apnea-hypopnea index from overnight pulse oximetry. Chest 124, 1694–1701. doi: 10.1378/chest.124.5.1694
Malhotra, A., Mesarwi, O., Pepin, J.-L., and Owens, R. L. (2020). Endotypes and phenotypes in obstructive sleep apnea. Curr. Opin. Pulmonary Med. 26:609. doi: 10.1097/MCP.0000000000000724
Marchi, N. A., Ramponi, C., Hirotsu, C., Haba-Rubio, J., Lutti, A., Preisig, M., et al. (2020). Mean oxygen saturation during sleep is related to specific brain atrophy pattern. Ann. Neurol. 87, 921–930. doi: 10.1002/ana.25728
Massimini, M., Huber, R., Ferrarelli, F., Hill, S., and Tononi, G. (2004). The sleep slow oscillation as a traveling wave. J. Neurosci. 24, 6862–6870. doi: 10.1523/JNEUROSCI.1318-04.2004
Mendonça, F., Mostafa, S. S., Morgado-Dias, F., Ravelo-Garcia, A. G., and Penzel, T. (2019). A review of approaches for sleep quality analysis. IEEE Access 7, 24527–24546. doi: 10.1109/ACCESS.2019.2900345
Micic, G., Lovato, N., Gradisar, M., Ferguson, S. A., Burgess, H. J., and Lack, L. C. (2016). The etiology of delayed sleep phase disorder. Sleep Med. Rev. 27, 29–38. doi: 10.1016/j.smrv.2015.06.004
Mosqueiro, T., de Lecea, L., and Huerta, R. (2014). Control of sleep-to-wake transitions via fast amino acid and slow neuropeptide transmission. New J. Phys. 16:115010. doi: 10.1088/1367-2630/16/11/115010
Muehlroth, B. E., Sander, M. C., Fandakova, Y., Grandy, T. H., Rasch, B., Shing, Y. L., et al. (2019). Precise slow oscillation–spindle coupling promotes memory consolidation in younger and older adults. Sci. Rep. 9, 1–15. doi: 10.1038/s41598-018-36557-z
Mullins, A. E., Kim, J. W., Wong, K. K., Bartlett, D. J., Vakulin, A., Dijk, D.-J., et al. (2021). Sleep EEG microstructure is associated with neurobehavioural impairment after extended wakefulness in obstructive sleep apnea. Sleep Breathing 25, 347–354. doi: 10.1007/s11325-020-02066-5
Muzet, A. (2007). Environmental noise, sleep and health. Sleep Med. Rev. 11, 135–142. doi: 10.1016/j.smrv.2006.09.001
Netzer, N., Eliasson, A. H., Netzer, C., and Kristo, D. A. (2001). Overnight pulse oximetry for sleep-disordered breathing in adults: a review. Chest 120, 625–633. doi: 10.1378/chest.120.2.625
Nir, Y., Staba, R. J., Andrillon, T., Vyazovskiy, V. V., Cirelli, C., Fried, I., et al. (2011). Regional slow waves and spindles in human sleep. Neuron 70, 153–169. doi: 10.1016/j.neuron.2011.02.043
Norton, J. J., Lee, D. S., Lee, J. W., Lee, W., Kwon, O., Won, P., et al. (2015). Soft, curved electrode systems capable of integration on the auricle as a persistent brain–computer interface. Proc. Natl. Acad. Sci. U.S.A. 112, 3920–3925. doi: 10.1073/pnas.1424875112
Olesen, A. N., Jørgen Jennum, P., Mignot, E., and Sorensen, H. B. D. (2021). Automatic sleep stage classification with deep residual networks in a mixed-cohort setting. Sleep 44:zsaa161. doi: 10.1093/sleep/zsaa161
Oliva, D., Hongisto, V., and Haapakangas, A. (2017). Annoyance of low-level tonal sounds–Factors affecting the penalty. Build. Environ. 123, 404–414. doi: 10.1016/j.buildenv.2017.07.017
O’Neill, J. S., and Reddy, A. B. (2011). Circadian clocks in human red blood cells. Nature 469, 498–503. doi: 10.1038/nature09702
Op de Beeck, S., Wellman, A., Dieltjens, M., Strohl, K. P., Willemen, M., Van de Heyning, P. H., et al. (2021). Endotypic mechanisms of successful hypoglossal nerve stimulation for obstructive sleep apnea. Am. J. Respir. Crit. Care Med. 203, 746–755. doi: 10.1164/rccm.202006-2176OC
Osman, A. M., Carberry, J. C., Burke, P. G., Toson, B., Grunstein, R. R., and Eckert, D. J. (2019). Upper airway collapsibility measured using a simple wakefulness test closely relates to the pharyngeal critical closing pressure during sleep in obstructive sleep apnea. Sleep 42:zsz080. doi: 10.1093/sleep/zsz080
Osman, A. M., Tong, B. K., Landry, S. A., Edwards, B. A., Joosten, S. A., Hamilton, G. S., et al. (2020). An assessment of a simple clinical technique to estimate pharyngeal collapsibility in people with obstructive sleep apnea. Sleep 43:zsaa067. doi: 10.1093/sleep/zsaa067
Pagano, G., De Micco, R., Yousaf, T., Wilson, H., Chandra, A., and Politis, M. (2018). REM behavior disorder predicts motor progression and cognitive decline in Parkinson disease. Neurology 91, e894–e905. doi: 10.1212/WNL.0000000000006134
Parekh, A., Kam, K., Mullins, A. E., Castillo, B., Berkalieva, A., Mazumdar, M., et al. (2021). Altered K-complex morphology during sustained inspiratory airflow limitation is associated with next-day lapses in vigilance in obstructive sleep apnea. Sleep 44:zsab010. doi: 10.1093/sleep/zsab010
Parekh, A., Mullins, A. E., Kam, K., Varga, A. W., Rapoport, D. M., and Ayappa, I. (2019). Slow-wave activity surrounding stage N2 K-complexes and daytime function measured by psychomotor vigilance test in obstructive sleep apnea. Sleep 42:zsy256. doi: 10.1093/sleep/zsy256
Parekh, A., Selesnick, I. W., Rapoport, D. M., and Ayappa, I. (2015). Detection of K-complexes and sleep spindles (DETOKS) using sparse optimization. J. Neurosci. Methods 251, 37–46. doi: 10.1016/j.jneumeth.2015.04.006
Parlak, O., Keene, S. T., Marais, A., Curto, V. F., and Salleo, A. (2018). Molecularly selective nanoporous membrane-based wearable organic electrochemical device for noninvasive cortisol sensing. Sci. Adv. 4:eaar2904. doi: 10.1126/sciadv.aar2904
Pengo, M. F., Ratneswaran, C., Berry, M., Kent, B. D., Kohler, M., Rossi, G. P., et al. (2016). Effect of continuous positive airway pressure on blood pressure variability in patients with obstructive sleep apnea. J. Clin. Hypertens. 18, 1180–1184. doi: 10.1111/jch.12845
Penzel, T., Kantelhardt, J. W., Bartsch, R. P., Riedl, M., Kraemer, J. F., Wessel, N., et al. (2016). Modulations of heart rate, ECG, and cardio-respiratory coupling observed in polysomnography. Front. Physiol. 7:460. doi: 10.3389/fphys.2016.00460
Plante, D. T. (2014). Leg actigraphy to quantify periodic limb movements of sleep: a systematic review and meta-analysis. Sleep Med. Rev. 18, 425–434. doi: 10.1016/j.smrv.2014.02.004
Prerau, M. J., Brown, R. E., Bianchi, M. T., Ellenbogen, J. M., and Purdon, P. L. (2017). Sleep neurophysiological dynamics through the lens of multitaper spectral analysis. Physiology 32, 60–92. doi: 10.1152/physiol.00062.2015
Prerau, M. J., Hartnack, K. E., Obregon-Henao, G., Sampson, A., Merlino, M., Gannon, K., et al. (2014). Tracking the sleep onset process: an empirical model of behavioral and physiological dynamics. PLoS Computational Biol. 10:e1003866. doi: 10.1371/journal.pcbi.1003866
Pretto, J. J., Roebuck, T., Beckert, L., and Hamilton, G. (2014). Clinical use of pulse oximetry: official guidelines from the T horacic S ociety of A ustralia and N ew Z ealand. Respirology 19, 38–46. doi: 10.1111/resp.12204
Punjabi, N. M., Newman, A. B., Young, T. B., Resnick, H. E., and Sanders, M. H. (2008). Sleep-disordered breathing and cardiovascular disease: an outcome-based definition of hypopneas. Am. J. Respiratory Crit. Care Med. 177, 1150–1155. doi: 10.1164/rccm.200712-1884OC
Punjabi, N. M., Patil, S., Crainiceanu, C., and Aurora, R. N. (2020). Variability and misclassification of sleep apnea severity based on multi-night testing. Chest 158, 365–373. doi: 10.1016/j.chest.2020.01.039
Purcell, S., Manoach, D., Demanuele, C., Cade, B., Mariani, S., Cox, R., et al. (2017). Characterizing sleep spindles in 11,630 individuals from the National Sleep Research Resource. Nat. Commun. 8, 1–16. doi: 10.1038/ncomms15930
Puri, S., El-Chami, M., Shaheen, D., Ivers, B., Panza, G. S., Badr, M. S., et al. (2020). Variations in loop gain and arousal threshold during NREM sleep are affected by time of day over a 24-hour period in participants with obstructive sleep apnea. J. Appl. Physiol. 129, 800–809. doi: 10.1152/japplphysiol.00376.2020
Qin, H., Keenan, B. T., Mazzotti, D. R., Vaquerizo-Villar, F., Kraemer, J. F., Wessel, N., et al. (2021). Heart rate variability during wakefulness as a marker of obstructive sleep apnea severity. Sleep 44:zsab018. doi: 10.1093/sleep/zsab018
Rechtschaffen, A., and Kales, A. (1968). A Manual of Standardized Terminology, Techniques, and Scoring System for Sleep Stages of Human Subjects. Washington, DC: Washington Public Health Service, US Government Printing Office.
Redline, S., and Purcell, S. M. (2021). Sleep and Big Data: Harnessing Data, Technology, and Analytics for Monitoring Sleep and Improving Diagnostics, Prediction, and Interventions—an Era for Sleep-Omics?. Oxford: Oxford University Press US. doi: 10.1093/sleep/zsab107
Reid, K. J. (2019). Assessment of circadian rhythms. Neurol. Clin. 37, 505–526. doi: 10.1016/j.ncl.2019.05.001
Roth, T., Coulouvrat, C., Hajak, G., Lakoma, M. D., Sampson, N. A., Shahly, V., et al. (2011). Prevalence and perceived health associated with insomnia based on DSM-IV-TR; international statistical classification of diseases and related health problems, tenth revision; and research diagnostic criteria/international classification of sleep disorders, criteria: results from the America insomnia survey. Biol. Psychiatry 69, 592–600. doi: 10.1016/j.biopsych.2010.10.023
Ruehland, W. R., Rochford, P. D., O’Donoghue, F. J., Pierce, R. J., Singh, P., and Thornton, A. T. (2009). The new AASM criteria for scoring hypopneas: impact on the apnea hypopnea index. Sleep 32, 150–157. doi: 10.1093/sleep/32.2.150
Sands, S. A., Edwards, B. A., Terrill, P. I., Butler, J. P., Owens, R. L., Taranto-Montemurro, L., et al. (2018a). Identifying obstructive sleep apnoea patients responsive to supplemental oxygen therapy. Eur. Respiratory J. 52:1800674. doi: 10.1183/13993003.00674-2018
Sands, S. A., Terrill, P. I., Edwards, B. A., Taranto Montemurro, L., Azarbarzin, A., Marques, M., et al. (2018b). Quantifying the arousal threshold using polysomnography in obstructive sleep apnea. Sleep 41:zsx183. doi: 10.1093/sleep/zsx183
Saper, C. B., and Fuller, P. M. (2017). Wake–sleep circuitry: an overview. Curr. Opin. Neurobiol. 44, 186–192. doi: 10.1016/j.conb.2017.03.021
Scammell, T. E., Arrigoni, E., and Lipton, J. O. (2017). Neural circuitry of wakefulness and sleep. Neuron 93, 747–765. doi: 10.1016/j.neuron.2017.01.014
Schäffer, B., Schlittmeier, S. J., Pieren, R., Heutschi, K., Brink, M., Graf, R., et al. (2016). Short-term annoyance reactions to stationary and time-varying wind turbine and road traffic noise: a laboratory study. J. Acoustical Soc. Am. 139, 2949–2963. doi: 10.1121/1.4949566
Scott, H., Lechat, B., Lovato, N., and Lack, L. (2020). Correspondence between physiological and behavioural responses to vibratory stimuli during the sleep onset period: a quantitative electroencephalography analysis. J. Sleep Res. 30:e13232. doi: 10.1111/jsr.13232
Scott, H., Lovato, N., and Lack, L. (2021). The development and accuracy of the THIM wearable device for estimating sleep and wakefulness. Nat. Sci. Sleep 13:39. doi: 10.2147/NSS.S287048
Sforza, E., Pichot, V., Saint Martin, M., Barthélémy, J. C., and Roche, F. (2015). Prevalence and determinants of subjective sleepiness in healthy elderly with unrecognized obstructive sleep apnea. Sleep Med. 16, 981–986. doi: 10.1016/j.sleep.2015.03.010
Shokri-Kojori, E., Wang, G.-J., Wiers, C. E., Demiral, S. B., Guo, M., Kim, S. W., et al. (2018). β-Amyloid accumulation in the human brain after one night of sleep deprivation. Proc. Natl. Acad. Sci. U.S.A. 115, 4483–4488. doi: 10.1073/pnas.1721694115
Sletten, T. L., Magee, M., Murray, J. M., Gordon, C. J., Lovato, N., Kennaway, D. J., et al. (2018). Efficacy of melatonin with behavioural sleep-wake scheduling for delayed sleep-wake phase disorder: a double-blind, randomised clinical trial. PLoS Med. 15:e1002587. doi: 10.1371/journal.pmed.1002587
Stephenson, R. (2003). Do circadian rhythms in respiratory control contribute to sleep-related breathing disorders? Sleep Med. Rev. 7, 475–490. doi: 10.1016/S1087-0792(03)90002-5
Steriade, M. (2006). Grouping of brain rhythms in corticothalamic systems. Neuroscience 137, 1087–1106. doi: 10.1016/j.neuroscience.2005.10.029
Stevens, D., Leong, C. W. Y., Cheung, H., Arciuli, J., Vakulin, A., Kim, J. W., et al. (2021). Sleep spindle activity correlates with implicit statistical learning consolidation in untreated obstructive sleep apnea patients. Sleep Med. [Online ahead of print] doi: 10.1016/j.sleep.2021.01.035
Stiasny-Kolster, K., Mayer, G., Schäfer, S., Moller, J. C., Heinzel-Gutenbrunner, M., and Oertel, W. H. (2007). The REM sleep behavior disorder screening questionnaire—a new diagnostic instrument. Mov. Disord. 22, 2386–2393. doi: 10.1002/mds.21740
Stone, J. E., McGlashan, E. M., Quin, N., Skinner, K., Stephenson, J. J., Cain, S. W., et al. (2020). The role of light sensitivity and intrinsic circadian period in predicting individual circadian timing. J. Biol. Rhythms 35, 628–640. doi: 10.1177/0748730420962598
Su, W., Chen, G., Ma, D., Zeng, J., Yan, F., Lin, X., et al. (2021). Higher Apnea-Hypopnea Index (AHI) and Oxygen Desaturation Index (ODI) were independently associated with increased risks of hypertension in patients with T2DM: a cross-sectional study. Int. J. Hypertens. 2021:8887944. doi: 10.1155/2021/8887944
Suárez, A., Núñez, F., and Rodriguez-Fernandez, M. (2020). Circadian phase prediction from non-intrusive and ambulatory physiological data. IEEE J. Biomed. Health Inform. 25, 1561–1571. doi: 10.1109/JBHI.2020.3019789
Sullivan, C., Berthon-Jones, M., Issa, F., and Eves, L. (1981). Reversal of obstructive sleep apnoea by continuous positive airway pressure applied through the nares. Lancet 317, 862–865. doi: 10.1016/S0140-6736(81)92140-1
Supratak, A., Dong, H., Wu, C., and Guo, Y. (2017). DeepSleepNet: a model for automatic sleep stage scoring based on raw single-channel EEG. IEEE Trans. Neural Syst. Rehabil. Eng. 25, 1998–2008. doi: 10.1109/TNSRE.2017.2721116
Sweetman, A., Reynolds, A., and Lack, L. C. (2021). Circadian factors in comorbid insomnia and sleep apnea (COMISA). J. Clin. Sleep Med. [Online ahead of print] jcsm.9408. doi: 10.5664/jcsm.9408
Taranto-Montemurro, L., Messineo, L., Sands, S. A., Azarbarzin, A., Marques, M., Edwards, B. A., et al. (2019). The combination of atomoxetine and oxybutynin greatly reduces obstructive sleep apnea severity. A randomized, placebo-controlled, double-blind crossover trial. Am. J. Respiratory Crit. Care Med. 199, 1267–1276. doi: 10.1164/rccm.201808-1493OC
Terrill, P. I. (2020). A review of approaches for analysing obstructive sleep apnoea-related patterns in pulse oximetry data. Respirology 25, 475–485. doi: 10.1111/resp.13635
Terzano, M. G., Parrino, L., Sherieri, A., Chervin, R., Chokroverty, S., Guilleminault, C., et al. (2001). Atlas, rules, and recording techniques for the scoring of cyclic alternating pattern (CAP) in human sleep. Sleep Med. 2, 537–553. doi: 10.1016/S1389-9457(01)00149-6
Thomas, R. J., Mietus, J. E., Peng, C.-K., and Goldberger, A. L. (2005). An electrocardiogram-based technique to assess cardiopulmonary coupling during sleep. Sleep 28, 1151–1161. doi: 10.1093/sleep/28.9.1151
Thomas, R. J., Wood, C., and Bianchi, M. T. (2018). Cardiopulmonary coupling spectrogram as an ambulatory clinical biomarker of sleep stability and quality in health, sleep apnea, and insomnia. Sleep 41:zsx196. doi: 10.1093/sleep/zsx196
Tononi, G., and Cirelli, C. (2006). Sleep function and synaptic homeostasis. Sleep Med. Rev. 10, 49–62. doi: 10.1016/j.smrv.2005.05.002
Trauer, J. M., Qian, M. Y., Doyle, J. S., Rajaratnam, S. M., and Cunnington, D. (2015). Cognitive behavioral therapy for chronic insomnia: a systematic review and meta-analysis. Ann. Internal Med. 163, 191–204. doi: 10.7326/M14-2841
Tsinalis, O., Matthews, P. M., Guo, Y., and Zafeiriou, S. (2016). Automatic sleep stage scoring with single-channel EEG using convolutional neural networks. arXiv preprint arXiv 1610.01683. (accessed June, 2019).
Tuominen, J., Peltola, K., Saaresranta, T., and Valli, K. (2019). Sleep parameter assessment accuracy of a consumer home sleep monitoring ballistocardiograph beddit sleep tracker: a validation study. J. Clin. Sleep Med. 15, 483–487. doi: 10.5664/jcsm.7682
Ucak, S., Dissanayake, H. U., Sutherland, K., de Chazal, P., and Cistulli, P. A. (2021). Heart rate variability and obstructive sleep apnea: current perspectives and novel technologies. J. Sleep Res. 30:e13274. doi: 10.1111/jsr.13274
Upasham, S., and Prasad, S. (2020). SLOCK (sensor for circadian clock): passive sweat-based chronobiology tracker. Lab Chip 20, 1947–1960. doi: 10.1039/D0LC00134A
Vakulin, A., D’Rozario, A., Kim, J.-W., Watson, B., Cross, N., Wang, D., et al. (2016). Quantitative sleep EEG and polysomnographic predictors of driving simulator performance in obstructive sleep apnea. Clin. Neurophysiol. 127, 1428–1435. doi: 10.1016/j.clinph.2015.09.004
von Allmen, D. C., Francey, L. J., Rogers, G. M., Ruben, M. D., Cohen, A. P., Wu, G., et al. (2018). Circadian dysregulation: the next frontier in obstructive sleep apnea research. Otolaryngol.–Head Neck Surg. 159, 948–955. doi: 10.1177/0194599818797311
Wang, D., Wong, K. K., Rowsell, L., Don, G. W., Yee, B. J., and Grunstein, R. R. (2018). Predicting response to oxygen therapy in obstructive sleep apnoea patients using a 10-minute daytime test. Eur. Respiratory J. 51:1701587. doi: 10.1183/13993003.01587-2017
Wang, Y.-K., Chen, H.-Y., Chen, J.-R., Lin, C.-M., and Chiu, H.-C. (2013). “A novel sleep/wake identification method with video analysis,” in Proceeding of the 2013 International Conference on Machine Learning and Cybernetics: IEEE, (IEEE), 1130–1135. doi: 10.1109/ICMLC.2013.6890761
Warby, S. C., Wendt, S. L., Welinder, P., Munk, E. G., Carrillo, O., Sorensen, H. B., et al. (2014). Sleep-spindle detection: crowdsourcing and evaluating performance of experts, non-experts and automated methods. Nat. Methods 11, 385–392. doi: 10.1038/nmeth.2855
Waser, M., Stefani, A., Holzknecht, E., Kohn, B., Hackner, H., Brandauer, E., et al. (2020). Automated 3D video analysis of lower limb movements during REM sleep: a new diagnostic tool for isolated REM sleep behavior disorder. Sleep 43:zsaa100. doi: 10.1093/sleep/zsaa100
Yamauchi, M., Jacono, F. J., Fujita, Y., Kumamoto, M., Yoshikawa, M., Campanaro, C. K., et al. (2014). Effects of environment light during sleep on autonomic functions of heart rate and breathing. Sleep Breathing 18, 829–835. doi: 10.1007/s11325-014-0951-7
Younes, M., Azarbarzin, A., Reid, M., Mazzotti, D. R., and Redline, S. (2021). Characteristics and reproducibility of novel sleep EEG biomarkers and their variation with sleep apnea and insomnia in a large community-based cohort. Sleep [Online ahead of print] doi: 10.1093/sleep/zsab145
Younes, M., and Giannouli, E. (2020). Mechanism of excessive wake time when associated with obstructive sleep apnea or periodic limb movements. J. Clin. Sleep Med. 16, 389–399. doi: 10.5664/jcsm.8214
Younes, M., and Hanly, P. J. (2016). Immediate postarousal sleep dynamics: an important determinant of sleep stability in obstructive sleep apnea. J. Appl. Physiol. 120, 801–808. doi: 10.1152/japplphysiol.00880.2015
Younes, M., Ostrowski, M., Soiferman, M., Younes, H., Younes, M., Raneri, J., et al. (2015). Odds ratio product of sleep EEG as a continuous measure of sleep state. Sleep 38, 641–654. doi: 10.5665/sleep.4588
Younes, M., Schweitzer, P. K., Griffin, K. S., Balshaw, R., and Walsh, J. K. (2020). Comparing two measures of sleep depth/intensity. Sleep 43:zsaa127. doi: 10.1093/sleep/zsaa127
Zakrzewski, M., Vehkaoja, A., Joutsen, A. S., Palovuori, K. T., and Vanhala, J. J. (2015). Noncontact respiration monitoring during sleep with microwave Doppler radar. IEEE Sensors J. 15, 5683–5693. doi: 10.1109/JSEN.2015.2446616
Zhao, W., Van Someren, E. J., Li, C., Chen, X., Gui, W., Tian, Y., et al. (2021). EEG spectral analysis in insomnia disorder: a systematic review and meta-analysis. Sleep Med. Rev. 59:101457. doi: 10.1016/j.smrv.2021.101457
Keywords: sleep disordered breathing, sleep apnea, insomnia, circadian rhythm, polysomnography, signal processing, apnea/hypopnea index, precision medicine
Citation: Lechat B, Scott H, Naik G, Hansen K, Nguyen DP, Vakulin A, Catcheside P and Eckert DJ (2021) New and Emerging Approaches to Better Define Sleep Disruption and Its Consequences. Front. Neurosci. 15:751730. doi: 10.3389/fnins.2021.751730
Received: 01 August 2021; Accepted: 16 September 2021;
Published: 07 October 2021.
Edited by:
David Gozal, University of Missouri, United StatesReviewed by:
Robert J. Thomas, Beth Israel Deaconess Medical Center and Harvard Medical School, United StatesCopyright © 2021 Lechat, Scott, Naik, Hansen, Nguyen, Vakulin, Catcheside and Eckert. This is an open-access article distributed under the terms of the Creative Commons Attribution License (CC BY). The use, distribution or reproduction in other forums is permitted, provided the original author(s) and the copyright owner(s) are credited and that the original publication in this journal is cited, in accordance with accepted academic practice. No use, distribution or reproduction is permitted which does not comply with these terms.
*Correspondence: Danny J. Eckert, ZGFubnkuZWNrZXJ0QGZsaW5kZXJzLmVkdS5hdQ==
†These authors have contributed equally to this work
Disclaimer: All claims expressed in this article are solely those of the authors and do not necessarily represent those of their affiliated organizations, or those of the publisher, the editors and the reviewers. Any product that may be evaluated in this article or claim that may be made by its manufacturer is not guaranteed or endorsed by the publisher.
Research integrity at Frontiers
Learn more about the work of our research integrity team to safeguard the quality of each article we publish.