- 1Department of Physiology and Biophysics, Howard University, Washington, DC, United States
- 2Department of Medicine, Georgetown University, Washington, DC, United States
Background: Myalgic encephalomyelitis/chronic fatigue syndrome (ME/CFS) is characterized by disabling fatigue and postexertional malaise. We developed a provocation paradigm with two submaximal bicycle exercise stress tests on consecutive days bracketed by magnetic resonance imaging, orthostatic intolerance, and symptom assessments before and after exercise in order to induce objective changes of exercise induced symptom exacerbation and cognitive dysfunction.
Method: Blood oxygenation level dependent (BOLD) scans were performed while at rest on the preexercise and postexercise days in 34 ME/CFS and 24 control subjects. Seed regions from the FSL data library with significant BOLD signals were nodes that clustered into networks using independent component analysis. Differences in signal amplitudes between groups on pre- and post-exercise days were determined by general linear model and ANOVA.
Results: The most striking exercise-induced effect in ME/CFS was the increased spontaneous activity in the medial prefrontal cortex that is the anterior node of the Default Mode Network (DMN). In contrast, this region had decreased activation for controls. Overall, controls had higher BOLD signals suggesting reduced global cerebral blood flow in ME/CFS.
Conclusion: The dynamic increase in activation of the anterior DMN node after exercise may be a biomarker of postexertional malaise and symptom exacerbation in CFS. The specificity of this postexertional finding in ME/CFS can now be assessed by comparison to post-COVID fatigue, Gulf War Illness, fibromyalgia, chronic idiopathic fatigue, and fatigue in systemic medical and psychiatric diseases.
Introduction
Myalgic encephalomyelitis/chronic fatigue syndrome (ME/CFS, abbreviated here as CFS) has been defined by subjective symptom criteria. The 1994 Center for Disease Control (“Fukuda”) criteria required unrelenting fatigue for over 6 months with no medical or psychiatric explanation plus four of eight ancillary criteria: short term memory or concentration, sleep disturbances, post-exertional malaise (PEM), headache, myalgia, arthralgia, sore throat or sore lymph nodes (Fukuda et al., 1994). The criteria were improved by including autonomic and flu-like symptoms in the Canadian Consensus Criteria (Carruthers et al., 2003, 2011; Carruthers, 2007). The emphasis shifted to fatigue, PEM, and unrefreshing sleep with either cognitive dysfunction or orthostatic intolerance in the 2015 Institute of Medicine criteria for Systemic Exercise Intolerance Disease (SEID)(Institute of Medicine, 2015). The 2020 National Institute for Health and Care Excellence (NICE) guidance reinforced the core symptoms of fatigue, PEM, cognition, and sleep (National Institute for Health and Care Excellence [NICE], 2021).
Post-exertional malaise has been described as exertional exhaustion or postexertional symptom exacerbation. Performing modestly greater than usual physical, cognitive, emotional or other effort leads to a “collapse” and relapse of dysfunctional impairment with fatigue, flu-like malaise, widespread pain, tenderness, orthostatic intolerance, and cognitive dysfunction of attention and working memory. Onset may be immediate or delayed overnight. The consequences are out of proportion to the effort as subjects with PEM can become bed bound and unable to complete usual daily activities or productive work for several days or longer.
The characteristics of PEM were evaluated using a submaximal bicycle exercise provocation paradigm to provoke and quantify fatigue, cognition, autonomic control of postural tachycardia, and other processes that may contribute to this dynamic alteration. Subjects completed two submaximal bicycle exercise stress tests on consecutive days. We discovered the novel finding that submaximal exercise induced transient postural tachycardia in about 25% of study participants (Rayhan et al., 2013; Garner et al., 2018; Garner and Baraniuk, 2019) and as a result investigated exercise-induced orthostatic tachycardia as a potential contributor to cognitive dysfunction and PEM.
Functional magnetic resonance imaging was performed before the stress tests to identify baseline differences in blood oxygenation level dependent (BOLD) signals between groups that may be diagnostic, and again after provocation to identify exertion-induced alterations that may be markers of PEM (Rayhan et al., 2013, 2019; Garner et al., 2018; Garner and Baraniuk, 2019; Washington et al., 2020a, b). The current study evaluates the resting state (no task) and can be compared to scans during a simple 0-back task of attention, and a difficult 2-back verbal working memory task to assess changes related to increasing cognitive task load. Brain regions that were activated or deactivated were identified as nodes; their correlated, synchronous activation with other nodes defined large scale cognitive networks. Networks provide the integrated services needed to cognitively observe and evaluate the environment, plan tasks and marshal resources for task completion, then rest and contemplate the actions during periods with no externally directed activity.
Being at rest leads to activation of nodes in the Default Mode Network (DMN) that have increased spontaneous activity at rest or, alternatively, are activated “by default” when there is no task to perform (Esposito et al., 2006). This network is activated for inward reflective thought, planning and self - referential contemplation. The DMN is anatomically housed in the medial anterior prefrontal cortex (mPFC, DMN_mPFC), posterior cingulate cortex (PCC, DMN_PCC), precuneus (DMN_Prec), and lateral parietal and inferior parahippocampal nodes (Uddin et al., 2009). A remarkable feature of the BOLD analysis during rest is that traces of task, sensorimotor, auditory, visual, and other networks are also apparent (Fox et al., 2005). Task networks have roles for salience, attention, and executive control. The salience network (SAL) provides oversight of task activity by monitoring ongoing sensory and other stimuli through the bilateral anterior insulae (aINS) for higher level management by the dorsal anterior cingulate cortex (dACC). Focus on the task is maintained by surveillance of the Dorsal Attention Network (DAN) in the frontal eye fields of the rostral middle frontal gyri and bilateral intraparietal sulci. Executive control (EC) is maintained by bilateral dorsolateral prefrontal cortex (DLPFC) and parietal regions of the Frontal Parietal Network (FPN) that encodes task actions that are carried out by the sensorimotor network (SMN). Occipital nodes form the Visual network (VIS).
Local structural injury or molecular dysfunction of neurons in the cortex, oligodendrocytes in myelin sheaths of long fiber tracts, or supporting microglia and astrocytes can disrupt the coordination between nodes and compromise network activities during tasks and at rest. Mechanisms leading to atrophy or hypertrophy in one region can spread along axons in long white matter tracts to transmit these pathological alterations to other nodes in the interconnected network. The network denegation hypothesis (Vanasse et al., 2021) contends that pathological injury in one localized region can be transmitted to connected brain regions via networks, and suggests that dysfunction in one node will predict that other regions in the associated network will become preferentially affected. The concept of network dysfunction may also predict that diseases with different pathologies may have superficial similarities that can be resolved by objective fMRI or other investigations of effects on networks. This hypothesis motivated the search for dysfunctional nodes and networks in CFS.
Materials and Methods
Ethics
The protocol was approved by the Georgetown University Institutional Review Board (IRB 2013-0943 and 2015-0579) and listed in clinicaltrials.gov (NCT01291758 and NCT00810225). All clinical investigations were conducted according to the principles expressed in the Declaration of Helsinki.
Subjects
Myalgic encephalomyelitis/chronic fatigue syndrome and healthy sedentary control subjects were recruited to these 4 day long in-patient studies in the Clinical Research Unit of the Georgetown–Howard Universities Center for Clinical and Translational Science. Subjects had history and physical examinations to ensure their inclusion by meeting Fukuda (Fukuda et al., 1994) and Carruthers Canadian Consensus Criteria for CFS (Carruthers et al., 2003; Carruthers, 2007), confirmation of sedentary lifestyle for control subjects (less than 40 min of aerobic activity per week), and exclusion because of serious medical or psychiatric conditions such as psychosis. History of posttraumatic stress disorder (PTSD) or depression were not exclusions unless the subject had been hospitalized in the past 5 years. Subjects completed a battery of questionnaires to assess self-reported complaints and symptoms (Rayhan et al., 2019).
Orthostatic Status
The provocation component of the paradigm was two submaximal bicycle exercise stress tests that were performed 24 h apart. Subjects cycled for 25 min at 70% predicted maximum heart rate (HR) (220-patient’s age), followed by a climb to 85% maximum HR to reach anaerobic threshold (Garner et al., 2018). Many subjects could not reach this endpoint; exercise performance will be reported separately. Subjective complaints of post-exertional malaise, BOLD at rest and during the cognitive tests, and orthostatic status were compared between pre-exercise and post-exercise time periods.
Postural tachycardia was tested before and after exercise as a common element of the protocol (Rayhan et al., 2013; Garner et al., 2018; Garner and Baraniuk, 2019). Subjects rested supine for 5 min and had HR measured by continuous EKG monitor. After standing up, HR and blood pressure were measured every minute for 5 min. The incremental changes in HR between supine and standing (ΔHR) were calculated to identify episodes of postural tachycardia with ΔHR ≥ 30 bpm. The normal ΔHR was 12 ± 5 bpm (mean ± SD).
Stress Test Originated Phantom Perception (STOPP) was defined by ΔHR < 30 bpm at all times before and after exercise. STOPP subjects had normal cardiac responses when standing up and no change after exercise.
Stress Test Activated Reversible Tachycardia (START) was defined by having a normal ΔHR before exercise, but at least two measurements of postural tachycardia with ΔHR ≥ 30 bpm after exercise. This phenomenon was transient as ΔHR returned to normal 24–48 h following exercise.
Postural Orthostatic Tachycardia Syndrome (POTS) was defined by ΔHR ≥ 30 bpm at two or more measurements prior to exercise during the 5-min standing periods (Freeman et al., 2011). POTS criteria were met before and after exercise, and exercise did not exacerbate postural tachycardia in this subgroup indicating that POTS subjects were distinct from the START and STOPP groups.
MRI Acquisition
Brain images were collected on a Siemens 3-Tesla Tim Trio scanner with a standard 12-channel head coil array. The fMRI data were attained using a T2∗-weighted gradient-echo planar imaging (EPI) during the resting state scan with the following imaging parameters: repetition time (TR) = 2500 ms, echo time (TE) = 30 ms, 90 degrees flip angle, FOV = 205mm2, matrix size = 64 × 64, number of slices = 47, voxel size = 3.2 mm2 isotropic. Runtime was 7 min with 168 total volumes acquired.
Structural scans were acquired with a 3D T1-weighted magnetization-prepared rapid acquisition with gradient echo (MPRAGE) pulse sequence with the following imaging parameters: TE = 2.52 ms, TR = 1900 ms, TI = 900 ms, FOV = 250 mm, 176 slices, slice resolution = 1.0 mm, voxel size 1 × 1 × 1 mm.
Preprocessing of fMRI Data
Imaging data was preprocessed using FSL 5.0.11 (FMRIB, 2021; Smith et al., 2004), and Python 2.7. Preprocessing steps included removal of the first five images to allow for signal equilibration, brain extraction (FSL-BET), motion correction (FSL-MCFLIRT), interleaved slice-timing correction and linear co-registration (FSL-FLIRT) to subject specific T1-weighted MPRAGE plus standard MNI structural image. During motion correction (FSL-MCFLIRT), subject mean displacement was calculated. Subsequently, any subjects with relative mean displacement greater than 0.2 mm on either pre-exercise or post-exercise functional scans were excluded. This cutoff led to the exclusion of three ME/CFS participants from further analysis. Spatial smoothing of data was set at 5mm FWHM.
After standard preprocessing steps, FSL’s ICA-AROMA without temporal filtering was employed (Pruim et al., 2015a, b). We employed the non-aggressive option, which performed a partial component regression. ICA-AROMA carries out probabilistic ICA and does not require study-specific training prior to its use (i.e., manual classification of independent components). Outputs for each subject’s pre and post exercise rsfMRI data are interrogated utilizing four robust spatial and temporal features of motion-related components. The program then removed these components using an ordinary least squares regression. Temporal filtering can impose a high level of autocorrelation and ICA-AROMA is an robust alternative that preserves signal of interest and reproducibility or networks (Pruim et al., 2015a, b).
Final steps following ICA-AROMA included nuisance regression of structured noise (using subject-specific white matter, cerebrospinal fluid, and linear trends), and high-pass filtering (Pruim et al., 2015a, b). To aid in data preprocessing, multiple custom scripts were employed via command line.
Network Analysis
Nodes and networks were identified by independent component analysis and MELODIC in FSL (MELODIC, 2021). A temporal concatenation approach was applied separately to pre-exercise and post-exercise scans by combining both ME/CFS and control patients (Beckmann et al., 2005). The method was successful in producing identifiable networks. However, the ability to compare across days was limited because group-ICA networks such as the DMN from pre-exercise and post-exercise had spatial differences. To provide comparable resting state networks across pre and post-exercise scans, we used a whole brain map template from a prior data set that generated 70 functional nodes (Smith et al., 2009). The nodal time-series for each subject’s pre and post exercise scans were extracted using the first step of the dual regression method in FSL using the template atlas (Smith et al., 2009). Following extraction of the 70 time-series for each scan, visual inspection identified 41 nodes that were artifacts and so were discarded.
The remaining 29 nodes were then entered into FSLnets and analyses was performed in MATLAB. Data from each node was entered to form matrices where individual and group networks were computed using the full-correlation option. Identified nodes/parcels were hierarchically clustered to reveal larger resting state networks. Visual inspection and comparison with well-defined resting state networks confirmed the clustered nodes and networks (Damoiseaux et al., 2006; Smith et al., 2009).
Because the spatial distribution of the network maps from the functional atlas was the same for both days, we analyzed the amplitude changes of the BOLD signal time-series between groups and across days. Changes within the amplitude may provide insight into dynamic exercise induced changes. Alterations of the BOLD amplitude have been associated with task-load during brain activation, pharmacological simulation, and illness (McKiernan et al., 2003; Kiviniemi et al., 2005; Vargas et al., 2013).
Amplitude strength for each node was calculated using the standard deviation of its associated time series (Li et al., 2017). Group differences were analyzed with two-tailed unpaired and paired t-tests across all analyzed nodes followed by Bonferroni correction for fifty-six comparisons (α = 0.05/56 = 0.000892) ANOVA and Tukey Honest Significant Difference for differences between groups and by paired Student’s t-test for exercise effects between days within groups in SPSS V.23. Functional connectivity was reported as Pearson’s correlation coefficient and α = 0.001 uncorrected (Clarke et al., 2019). To evaluate independent variables, general linear models used CFS status, orthostatic status, gender as fixed factors with age and BMI as continuous covariates. Multivariate general linear modeling was performed to assess the effects of Disease status (CFS vs. control) and Orthostatic status (STOPP vs. START vs. POTS).
Results
Demographics and Self-Reported Questionnaires
There were no significant differences in demographic variables between controls (n = 24) and ME/CFS subjects (n = 34) (Table 1). CFS severity questionnaire data revealed CFS subjects had significantly higher scores for fatigue, exertional exhaustion, unrefreshing sleep, and muscle pain (P < 0.001) (Baraniuk et al., 2013). Chalder fatigue total score (sum of 11 items, Σ11) and derived subscores were significantly higher in CFS than controls (P < 0.001) (Chalder et al., 1993; Cella and Chalder, 2010; Fong et al., 2015). No significant differences were found for the Center for Epidemiological Studies-Depression (CESD) domains (Vilagut et al., 2016).
Group Average Hierarchical Clustering and Network Identification
A total of 29 independent functional nodes extracted from the BOLD time series data were assessed using FSL by hierarchical clustering for network identification. The nodes were clustered into seven resting state networks: DMN, FPN, DAN, SMN, SAL, SUB, and VIS (Figure 1 and Table 2).
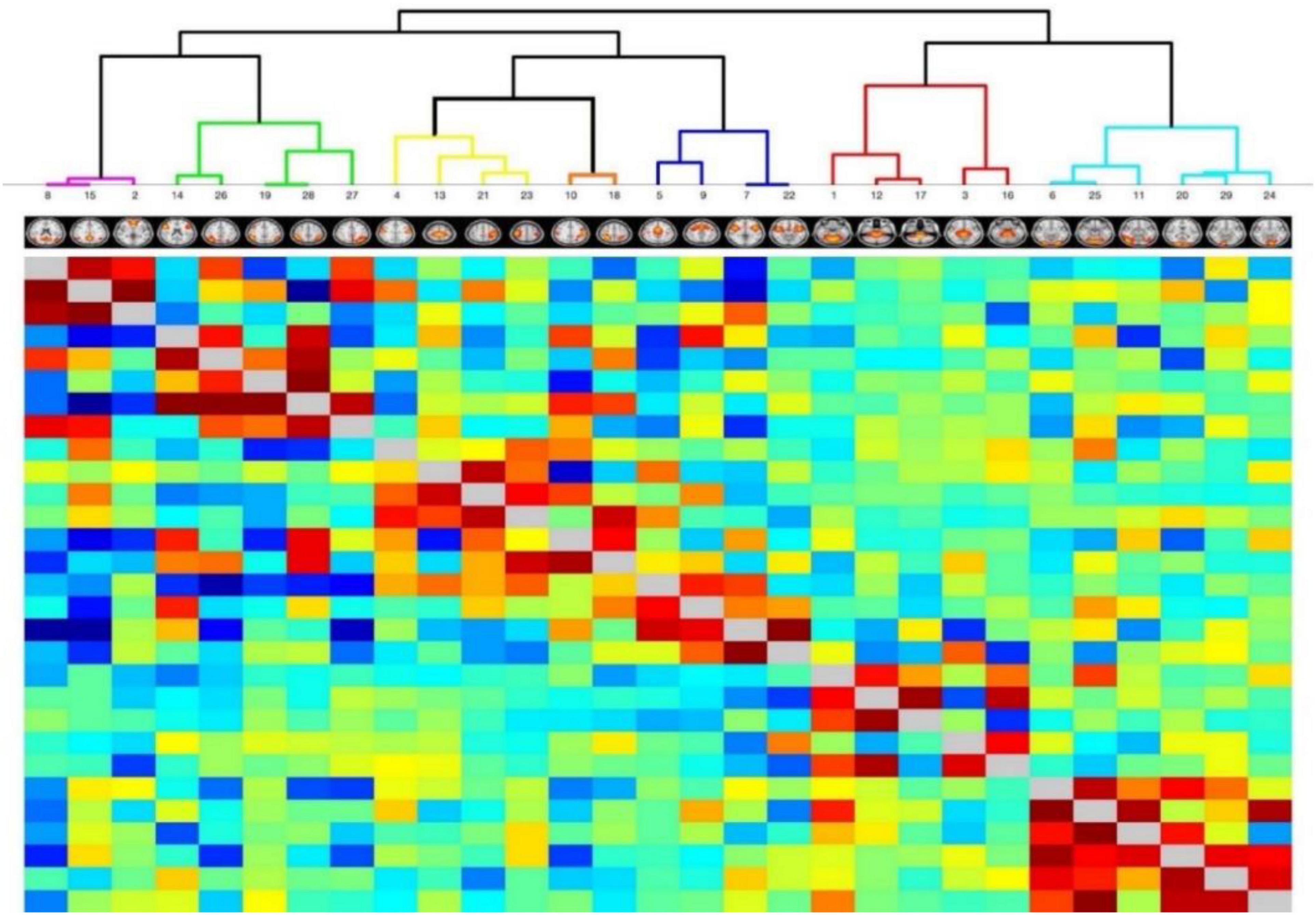
Figure 1. Hierarchical clustering heatmaps. Time series data identified 29 nodes in seven resting state networks as independent components (IC) using templates in FSLnets. Heatmap overlay is red-blue, where red signifies positive and blue signifies negative correlations, respectively. Clade color corresponds to parent resting state network. Purple clade = Default Mode Network (DMN). Green clade = Frontal Parietal Network (FPN). Yellow clade = Sensorimotor Network (SMN). Orange clade = Dorsal Attention Network (DAN). Blue clade = Salience Network (SAL). Red clade = Subcortical Network (SUB). Aqua clade = Visual Network (VIS). The insert figures show representative FSL probability maps to localize each node.
General Linear Models
Signal amplitude data were assessed by multivariate general linear model with CFS status, Orthostatic status and gender as fixed factors and age and body mass index (BMI) as independent co-variates. Disease status (CFS vs. control) was significant in models before and aft er exercise.
Preexercise control had significantly higher estimated marginal means than CFS for SAL_SMA, SM_RS2, SM_LS2, SUB_vDien, and VIS0063 after adjusting for the other variables (Table 3). Postexercise control had greater activation than CFS for salience (SAL_dACC, SAL_SMA, and SAL_mIns), sensorimotor SM_LS2, subcortical (SUB_Thal, SUB_Midbrain, SUB16vDien, and SUB_Pons), and visual (VIS0017, VIS0041, VIS0053, and VIS0063) network nodes. DMN_mPFC was significantly higher for CFS than control postexercise after adjusting for the other variables (Table 4).
Age was significant in univariate analysis for many nodes before and after exercise. The interactions of age, Disease status and gender were evaluated as fixed factors. Using the preexercise data, age was significant for FP_LPar, FP_RPar, SAL_aIns, SAL_dACC, both DAN nodes, DMN_Prec, and VIS0053. However, only FP_RPar was significant for CFS status (estimated marginal means for Control 2.181 ± 0.136 and CFS 1.701 ± 0.117, mean ± 95%CI, p = 0.016) in this model. Postexercise confirmed significant age effects for FP_LPar, FP_RPar, SAL_aIns, and VIS0053 plus FP_biPar, but only DMN_mPFC was significantly associated with CFS after accounting for age and gender.
Orthostatic status and BMI were not significant before or after exercise.
The incremental differences between postexercise and preexercise (Δ) were significantly different in two nodes. DMN_mPFC was larger for CFS than control postexercise, while the difference for VIS0017 was larger in control than CFS (Table 5).
Analysis of Variance
The data were compared by ANOVA after taking the significant variables into account. The GLM results showed that BOLD was significantly different by Disease status, exercise day, and age in univariate analyses. The demographics found different proportions by gender in control and CFS. Orthostatic status and BMI were not significant variables. Therefore, signal amplitude data were regressed for age and gender and the differences between CFS and control were assessed on the preexercise and postexercise days (Table 6). Several general trends were identified that were compared to those found by GLM.
The average BOLD amplitude from all measurements before exercise was significantly higher for control (1.749 ± 0.854, mean ± SD) than CFS (1.410 ± 0.616, p = 10–20 by two-tailed unpaired t-test comparing all regions between all control and CFS subjects). After exercise, control (1.691 ± 0.724) was again significantly higher than CFS (1.450 ± 0.664, p = 10–12). This suggested an overarching disease effect with lower cerebral blood flow in CFS than controls, and an exercise effect with significantly higher activation preexercise than postexercise in both control (p = 0.0017, two-tailed paired t-test) and CFS (p = 0.0070, two-tailed paired t-test).
DMN_mPFC was the most interesting node because of the reciprocal effects of exercise in CFS compared to control. The two groups were equivalent prior to exercise. BOLD in sedentary control was greater in preexercise than postexercise indicating a relative reduction in the activity in DMN_mPFC on the second day. In contrast, CFS had increased activation of DMN_mPFC in the resting state following the exercise stressor. As a result, CFS had significantly greater BOLD than control after exercise. This was consistent with the GLM of incremental changes (Table 5).
Four regions had persistently lower BOLD in CFS compared to control before and after exercise (Table 6) indicating a persistent deficit that did not change with provocation. The regions were SAL_mIns, SUB_Midbrain, SUB_Pons, and VIS0063.
CFS had lower BOLD than control at baseline for DAN_LIPS, DMN_PCC, FP_biTPar, FP_RPar, SAL_SMA, SM_rS2, SM_LS2, SUB_Thal and SUB_vDien. Estimated marginal means were not significantly different after exercise.
Exercise caused reduced BOLD in CFS compared to postexercise controls in VIS0017, VIS0057, VIS0041, and VIS0053.
Other nodes were not different between control and CFS or with exercise indicating that they were not affected by disease status or provocation.
There was general agreement between the results of the GLMs using raw amplitude data and ANOVA performed after the data were regressed versus age and gender. The most important was the reciprocal changes in DMN_mPFC after exercise (Figure 2).
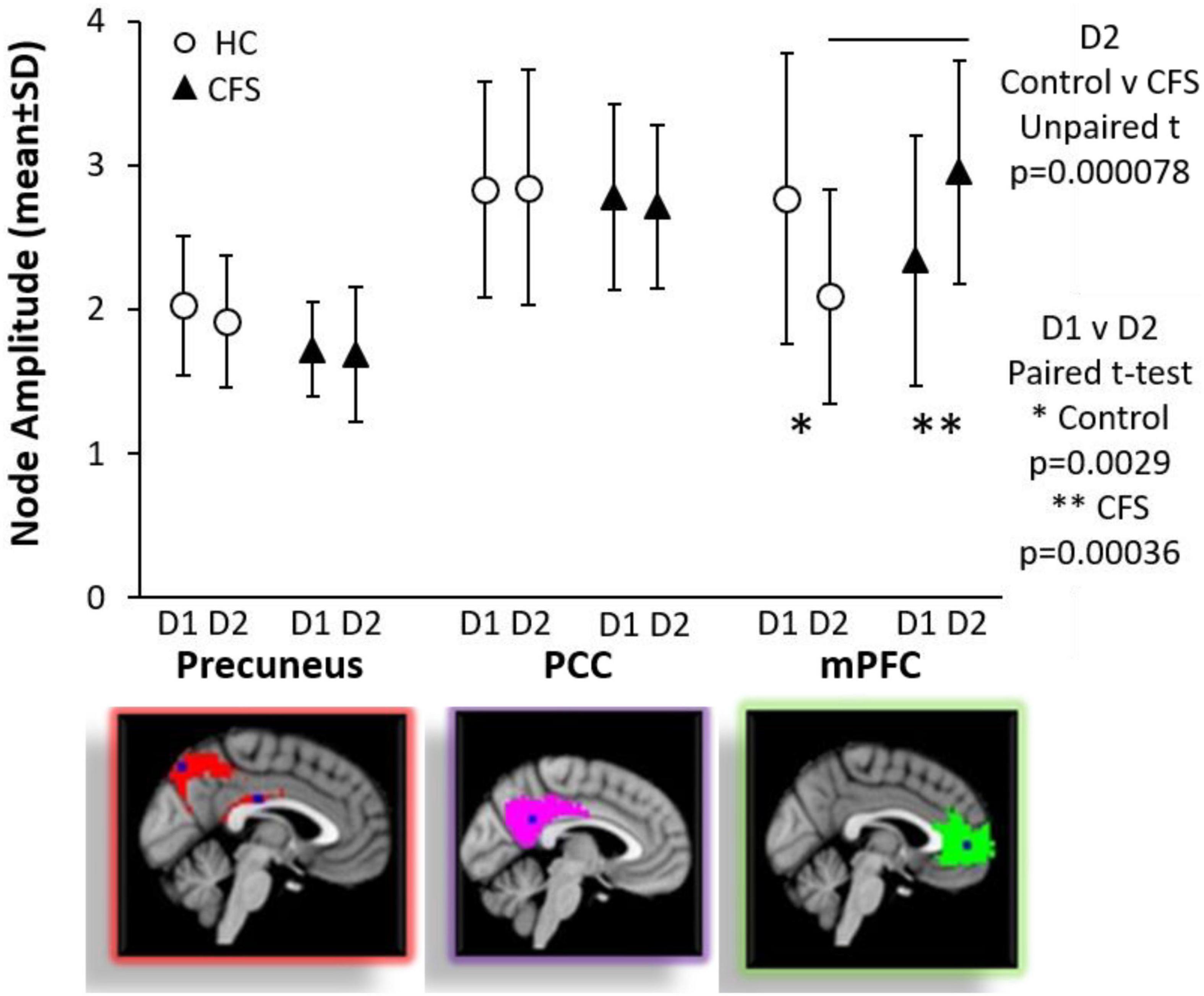
Figure 2. Default mode network nodes. Node amplitude strengths were compared by ANOVA and Tukey Honest Significant Difference for differences between groups and by paired Student’s t-test for exercise effects between days within groups. DMN_PCC (red in top figure on right) and DMN_Prec (magenta in middle figure on right) were equivalent between control and CFS and between preexercise and postexercise scans. In contrast, exercise had significant effects on the anterior node in the DMN_mPFC (green in the bottom figure on the right). Node amplitude strengths were equivalent for CFS and control preexercise. Exercise caused a significant decrease in control (∗p = 0.0029 by paired test), but a significant increase for CFS (∗∗p = 0.00036 by paired test). As a result of the dynamic changes, CFS had significantly higher signal than control postexercise (line above error bars, p = 0.000078). Mean ± SD.
Connectivity
Connectivity between nodes was assessed by Pearson correlations using the age and gender regressed data from the CFS and control groups on each study day. Each set of regressed amplitudes was demeaned by the respective group averages (subject minus average) for each node. Net BOLD signals from each node were correlated using two-tailed Pearson tests. There were no significant negative correlations.
Prexercise control showed correlations within each network but particularly Sensorimotor (yellow edge), Visual (light blue edge), and Salience (red edge) (Figure 3). DMN_mPFC was significantly correlated (R > 0.7, p < 0.001 uncorrected) with DMN_Prec, FP_DLPFC, DAN_RIPS, DAN_LIPS, SAL_dACC, SAL_SMA, and SAL_aIns.
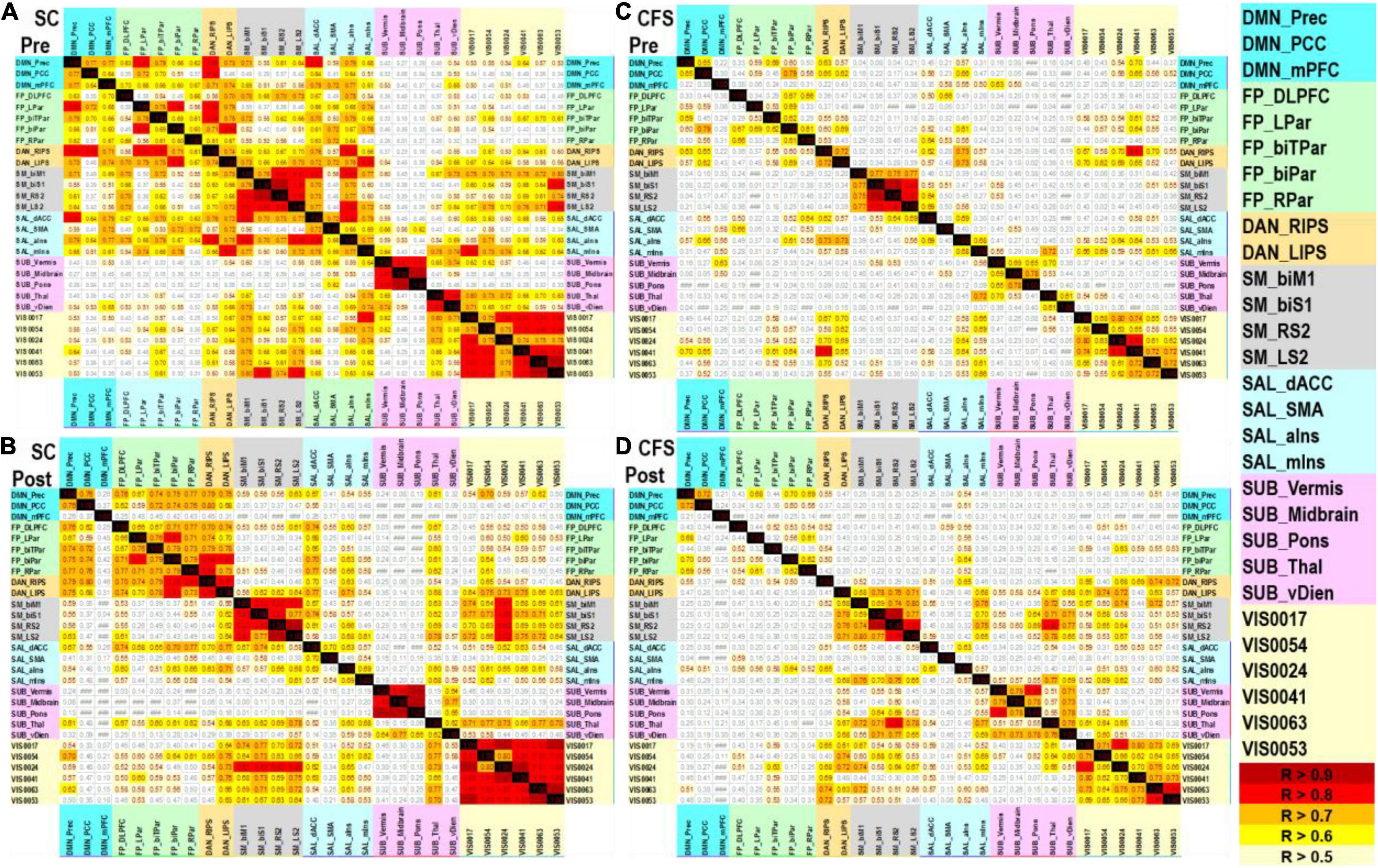
Figure 3. Pearson correlation matrices. Age and gender regressed BOLD amplitude data were correlated for (A) sedentary control preexercise, (B) sedentary control postexercise, (C) CFS preexercise, and (D) CFS postexercise. Correlations are shown with R > 0.5 (light yellow), R > 0.6 (yellow), R > 0.7 (orange), R > 0.8 (red), and R > 0.9 (dark red) that were all p < 0.001 uncorrected. There were no significant negative correlations. Networks and nodes were highlighted in color on the right: DMN cyan, FPN green, DAN orange, SMN gray, SAL blue, SUB pink, and VIS yellow.
Postexercise control was more highly correlated within each network than preexercise suggesting improved efficiency when resting in the scanner on the second day. DMN_mPFC had zero significant correlations postexercise (R < 0.5).
Preexercise CFS was markedly different as there were significant correlations with R > 0.8 only within the sensorimotor and visual networks. DMN_mPFC was correlated with SUB_Vermis (R = 0.633).
Exercise modestly increased the number of significant correlations in CFS, but this number was smaller than control following exercise. DMN_mPFC in CFS had no correlations larger than 0.3 despite the increased activation of this node after exercise.
The age and gender regressed BOLD values had no significant correlations with measures of fatigue, pain, tenderness, depression, and other subjective symptoms (all R < 0.3, data not shown).
Discussion
The remarkable discordant finding in CFS was the exercise – induced signal increase within the medial prefrontal cortex of the DMN. This change may be a functional indicator of post-exertional malaise that is a requirement for the diagnosis of CFS. The DMN is a collection of brain regions with correlated activation during rest when there is no externally oriented task to be performed (Raichle, 2015). DMN regions become deactivated when switching from rest to task performance as other regions simultaneously become coordinated in specific task networks. As a result, the relative activation levels of DMN and task network nodes are anti-correlated. The medial prefrontal cortex (mPFC) is the anterior hub of the DMN (Müller et al., 2020). Generally, it is recognized that the DMN is involved in self-referential though, mind-wandering, and autobiographic memory retrieval (Gusnard et al., 2001; Mason et al., 2007; Spreng and Grady, 2010). The mPFC also contributes to other aspects of cognition such as social awarenes, affective processing, and goal-directed behavior (Schilbach et al., 2008; Spreng and Grady, 2010; Roy et al., 2012). The relative activation of the mPFC caused by exercise in CFS may represent a decoupling from the posterior nodes of the DMN and loss of regulatory input. Thus, exercise – induced uncoupling may be a pathological consequence and a cognitive biomarker of post-exertional malaise. If so, this finding has significant potential to become a criterion for disease diagnosis.
All regions except mPFC of the DMN tended to have lower resting state BOLD signal in CFS compared to control before and after exercise (Table 6). There were three general patterns. First, CFS had a pre-exercise deficit in parietal, SMA, thalamus, and ventral diencephalon regions, but after exercise the differential narrowed and became insignificant because of a general decline in control with unchanged values in CFS. Second, visual regions showed the opposite trend as they were equivalent prior to exercise. However, after exercise the combination of a slight increase in signal for controls and no change in CFS led to significant exercise-induced deficits. The last pattern was persistent deficits in CFS for Midbrain, Pons, and insular nodes pre and post exercise. The insula, midbrain and pons are hubs for interoception, autonomic regulation, pain, sleep, and motor regulation (Bracha, 2004; Critchley et al., 2004; Blanchard et al., 2011; Buhle et al., 2013; Nakatomi et al., 2014; Blanchard, 2017; Franklin, 2019). Deficits in these regions may correlate to the persistent visceral symptoms experienced in CFS. The general trend for lower BOLD signal at rest in CFS compared to controls is consistent with the general reduction in cerebral blood flow and reports of orthostatic intolerance that are recurrent findings in CFS (Razumovsky et al., 2003; Yoshiuchi, 2006; Pardini et al., 2010; Tajima et al., 2010; Biswal et al., 2011; Stewart et al., 2011; Ocon et al., 2012; Medow et al., 2014; Gay et al., 2016; Finkelmeyer et al., 2018; van Campen et al., 2020a, b, c).
Chronic fatigue syndrome had blunted activity throughout the cerebrum compared to controls before and after exercise except for within the mPFC. Increased signal in the mPFC has been associated with the fatigue in TBI and during processing of fatigue as a sensation (Pardini et al., 2010; Tajima et al., 2010). A similar finding of elevated anterior DMN activation during a low cognitive load task following exercise was also found in Gulf War Illness (GWI) (Rayhan et al., 2019). GWI is a chronic disease affecting approximately 25% of the veterans who served in the 1991 Persian Gulf War (Fukuda et al., 1998; Steele, 2000; White et al., 2016). CFS and GWI have similar symptoms of fatigue, cognitive dysfunction and post-exertional malaise (Rayhan et al., 2019; Washington et al., 2020a, b).
Age is an important confounder for DMN function and uncoupling between DMN nodes can be a result of aging (Damoiseaux et al., 2008; Dennis and Thompson, 2014; Puttaert et al., 2020; Kavroulakis et al., 2021). However, aging does not explain the increase in BOLD in the mPFC seen in CFS as age was regressed within the GLM and ANOVA analyses (Figure 2).
Limitations of the study are findings may only be limited to our resting state and submaximal exercise provocation protocol. Replicative studies will help provide further validate the results. BOLD signals were processed extensively to account for age, gender and motion, and were reported as the standard deviations of the nodal amplitude changes. Data analysis by ANOVA of age and gender regressed data and GLM gave comparable outcomes. It is possible that other variables related to fatigue, regulation of pain or interoceptive signaling, affect, anxiety, autonomic dysfunction, and/or molecular mechanisms of neurovascular coupling may have had significant effects on our findings. Functions related to the diencephalon, brainstem, vermis, and insula that were significantly activated here may also provide additional covariates for future evaluation. Comparison of anterior DMN activation between CFS and GWI was only qualitative but future studies including both groups may provide additional results into exercise-induced dysfunction and the role of the mPFC in fatigue processing. Comparisons to other cognitive tasks such as Stroop and Flanker are likely to provide additional information about baseline and post-exertional cognitive processing. A standard template was used to generate the initial seed regions for the analyses. It is possible those actual regions of activation and deactivation did not conform to these seeds, and that the apparent levels were contaminated by BOLD activity bleeding over from adjacent brain regions. Despite this possibility, the anterior DMN node had reciprocal changes after exercise in CFS versus controls.
Future analysis using ICA nodes generated from native subject data may provide a clearer picture to the exercise-induced dysfunction and network interaction occurring in CFS patients. Future studies will explore the effectiveness of potential therapies by assessing whether they can prevent the post-exertional increase in activation within the anterior DMN (mPFC) in CFS. Samples sizes were relatively large; the mean and standard deviations provide effect size estimates to power future confirmatory studies of anterior DMN dysfunction in post-exertional symptom exacerbation in CFS.
Conclusion
The important exercise effect was the differential change in anterior DMN node activation following exercise. CFS had significantly increased activation while control had a decrease in activation compared to the preexercise day. CFS is defined clinically by postexertional malaise and cognitive difficulties (Fukuda et al., 1994; Carruthers et al., 2003; Carruthers, 2007; Institute of Medicine, 2015). The postexertional increase in BOLD activation in the medial prefrontal cortex may represent a biomarker for CFS that indicates loss of focus or excessive mind wandering during the resting state compared to controls. Alternative explanations for reduced cognition would be a decrease in salience, dorsal attention, and frontoparietal executive functioning but these were not detected in the resting state.
Data Availability Statement
The original contributions presented in the study are included in the article/supplementary material, further inquiries can be directed to the corresponding author.
Ethics Statement
The studies involving human participants were reviewed and approved by Georgetown University. The patients/participants provided their written informed consent to participate in this study.
Author Contributions
JB obtained the funding. JB and RR performed the research program, data analysis, and wrote the manuscript. Both authors contributed to the article and approved the submitted version.
Funding
This study was supported by funding from the Sergeant Sullivan Circle, Barbara Cottone, Department of Defense Congressionally Directed Medical Research Program (CDMRP) W81XWH-15-1-0679 and W81-XWH-09-1-0526, and National Institute of Neurological Disorders and Stroke (NINDS) R21NS088138 and RO1NS085131. Additional funding sources include the NINDS NRSA F30 MD/Ph.D. Pre-doctoral award F30NS103563. This project has been funded in whole or in part with Federal funds (Grant #UL1TR000101 previously UL1RR031975) from the National Center for Advancing Translational Sciences (NCATS), National Institutes of Health (NIH), through the Clinical and Translational Science Awards Program (CTSA), a trademark of DHHS, part of the Roadmap Initiative, “Re-Engineering the Clinical Research Enterprise.”
Conflict of Interest
The authors declare that the research was conducted in the absence of any commercial or financial relationships that could be construed as a potential conflict of interest.
Publisher’s Note
All claims expressed in this article are solely those of the authors and do not necessarily represent those of their affiliated organizations, or those of the publisher, the editors and the reviewers. Any product that may be evaluated in this article, or claim that may be made by its manufacturer, is not guaranteed or endorsed by the publisher.
References
Baraniuk, J. N., Adewuyi, O., Merck, S. J., Ali, M., Ravindran, M. K., Timbol, C. R., et al. (2013). A chronic fatigue syndrome (CFS) severity score based on case designation criteria. Am. J. Transl. Res. 5, 53–68.
Beckmann, C. F., DeLuca, M., Devlin, J. T., and Smith, S. M. (2005). Investigations into resting-state connectivity using independent component analysis. Philos. Trans. R. Soc. Lond. B Biol. Sci. 360, 1001–1013. doi: 10.1098/rstb.2005.1634
Biswal, B., Kunwar, P., and Natelson, B. H. (2011). Cerebral blood flow is reduced in chronic fatigue syndrome as assessed by arterial spin labeling. J. Neurol. Sci. 301, 9–11.
Blanchard, D. C. (2017). Translating dynamic defense patterns from rodents to people. Neurosci. Biobehav. Rev. 76, 22–28. doi: 10.1016/j.neubiorev.2016.11.001
Blanchard, D. C., Griebel, G., Pobbe, R., and Blanchard, R. J. (2011). Risk assessment as an evolved threat detection and analysis process. Neurosci. Biobehav. Rev. 35, 991–998. doi: 10.1016/j.neubiorev.2010.10.016
Bracha, H. S. (2004). Freeze, flight, fight, fright, faint: adaptationist perspectives on the acute stress response spectrum. CNS Spectr. 9, 679–685. doi: 10.1017/s1092852900001954
Buhle, J. T., Kober, H., Ochsner, K. N., Mende-Siedlecki, P., Weber, J., Hughes, B. L., et al. (2013). Common representation of pain and negative emotion in the midbrain periaqueductal gray. Soc. Cogn. Affect. Neurosci. 8, 609–616. doi: 10.1093/scan/nss038
Carruthers, B. M. (2007). Definitions and aetiology of myalgic encephalomyelitis: how the Canadian consensus clinical definition of myalgic encephalomyelitis works. J. Clin. Pathol. 60, 117–119. doi: 10.1136/jcp.2006.042754
Carruthers, B. M., Jain, A. K., De Meirleir, K. L., Peterson, D. L., Klimas, N. G., Lerner, A. M., et al. (2003). Myalgic encephalomyelitis/chronic fatigue syndrome. J. Chronic Fatigue Syndr. 11, 7–115. doi: 10.1300/J092v11n01_02
Carruthers, B. M., van de Sande, M. I., De Meirleir, K. L., Klimas, N. G., Broderick, G., Mitchell, T., et al. (2011). Myalgic encephalomyelitis: international consensus criteria. J. Intern. Med. 270, 327–338. doi: 10.1111/j.1365-2796.2011.02428.x
Cella, M., and Chalder, T. (2010). Measuring fatigue in clinical and community settings. J. Psychosom. Res. 69, 17–22. doi: 10.1016/j.jpsychores.2009.10.007
Chalder, T., Berelowitz, G., Pawlikowska, T., Watts, L., Wessely, S., Wright, D., et al. (1993). Development of a fatigue scale. J. Psychosom. Res. 37, 147–153. doi: 10.1016/0022-3999(93)90081-P
Clarke, T., Jamieson, J. D., Malone, P., Rayhan, R. U., Washington, S., VanMeter, J. W., et al. (2019). Connectivity differences between Gulf War Illness (GWI) phenotypes during a test of attention. PLoS One 14:e0226481. doi: 10.1371/journal.pone.0226481
Critchley, H. D., Wiens, S., Rotshtein, P., Ohman, A., and Dolan, R. J. (2004). Neural systems supporting interoceptive awareness. Nat. Neurosci. 7, 189–195. doi: 10.1038/nn1176
Damoiseaux, J. S., Beckmann, C. F., Arigita, E. J. S., Barkhof, F., Scheltens, P., Stam, C. J., et al. (2008). Reduced resting-state brain activity in the “default network” in normal aging. Cereb. Cortex 18, 1856–1864. doi: 10.1093/cercor/bhm207
Damoiseaux, J. S., Rombouts, S. A. R. B., Barkhof, F., Scheltens, P., Stam, C. J., Smith, S. M., et al. (2006). Consistent resting-state networks across healthy subjects. Proc. Natl. Acad. Sci. U.S.A. 103, 13848–13853. doi: 10.1073/pnas.0601417103
Dennis, E. L., and Thompson, P. M. (2014). Functional brain connectivity using fMRI in aging and Alzheimer’s disease. Neuropsychol. Rev. 24, 49–62. doi: 10.1007/s11065-014-9249-6
Esposito, F., Bertolino, A., Scarabino, T., Latorre, V., Blasi, G., Popolizio, T., et al. (2006). Independent component model of the default-mode brain function: assessing the impact of active thinking. Brain Res. Bull. 70, 263–269. doi: 10.1016/j.brainresbull.2006.06.012
Finkelmeyer, A., He, J., Maclachlan, L., Blamire, A. M., and Newton, J. L. (2018). Intracranial compliance is associated with symptoms of orthostatic intolerance in chronic fatigue syndrome. PLoS One 13:e0200068. doi: 10.1371/journal.pone.0200068
Fong, T. C. T., Chan, J. S. M., Chan, C. L. W., Ho, R. T. H., Ziea, E. T. C., Wong, V. C. W., et al. (2015). Psychometric properties of the Chalder Fatigue scale revisited: an exploratory structural equation modeling approach. Qual. Life Res. 24, 2273–2278. doi: 10.1007/s11136-015-0944-4
Fox, M. D., Snyder, A. Z., Vincent, J. L., Corbetta, M., Van Essen, D. C., and Raichle, M. E. (2005). From the cover: the human brain is intrinsically organized into dynamic, anticorrelated functional networks. Proc. Natl. Acad. Sci. U.S.A. 102, 9673–9678. doi: 10.1073/pnas.0504136102
Franklin, T. B. (2019). Recent Advancements Surrounding the Role of the Periaqueductal Gray in Predators and Prey. Front. Behav. Neurosci. 13:60. doi: 10.3389/fnbeh.2019.00060
Freeman, R., Wieling, W., Axelrod, F. B., Benditt, D. G., Benarroch, E., Biaggioni, I., et al. (2011). Consensus statement on the definition of orthostatic hypotension, neurally mediated syncope and the postural tachycardia syndrome. Clin. Auton. Res. 21, 69–72. doi: 10.1007/s10286-011-0119-5
Fukuda, K., Nisenbaum, R., Stewart, G., Thompson, W. W., Robin, L., Washko, R. M., et al. (1998). Chronic multisymptom illness affecting Air Force veterans of the Gulf War. J. Am. Med. Assoc. 280, 981–988. doi: 10.1001/jama.280.11.981
Fukuda, K., Straus, S. E., Hickie, I., Sharpe, M. C., Dobbins, J. G., and Komaroff, A. (1994). The chronic fatigue syndrome: a comprehensive approach to its definition and study. Ann. Intern. Med. 121, 953–959. doi: 10.7326/0003-4819-121-12-199412150-00009
Garner, R. S., Rayhan, R. U., and Baraniuk, J. N. (2018). Verification of exercise-induced transient postural tachycardia phenotype in Gulf War Illness. Am. J. Transl. Res. 10, 3254–3264.
Garner, R., and Baraniuk, J. N. (2019). Orthostatic intolerance in chronic fatigue syndrome. J. Transl. Med. 17:185. doi: 10.1186/s12967-019-1935-y
Gay, C. W., Robinson, M. E., Lai, S., O’Shea, A., Craggs, J. G., Price, D. D., et al. (2016). Abnormal resting-state functional connectivity in patients with chronic fatigue syndrome: results of seed and data-driven analyses. Brain Connect. 6, 48–56. doi: 10.1089/brain.2015.0366
Gusnard, D. A., Akbudak, E., Shulman, G. L., and Raichle, M. E. (2001). Medial prefrontal cortex and self-referential mental activity: relation to a default mode of brain function. Proc. Natl. Acad. Sci. U.S.A. 98, 4259–4264. doi: 10.1073/pnas.071043098
Institute of Medicine (2015). Beyond Myalgic Encephalomyelitis/Chronic Fatigue Syndrome. Washington, DC: National Academies Press, doi: 10.17226/19012
Kavroulakis, E., Simos, N. J., Maris, T. G., Zaganas, I., Panagiotakis, S., and Papadaki, E. (2021). Evidence of age-related hemodynamic and functional connectivity impairment: a resting state fMRI study. Front. Neurol. 12:633500. doi: 10.3389/fneur.2021.633500
Kiviniemi, V., Ruohonen, J., and Tervonen, O. (2005). Separation of physiological very low frequency fluctuation from aliasing by switched sampling interval fMRI scans. Magn. Reson. Imaging 23, 41–46. doi: 10.1016/j.mri.2004.09.005
Li, H., Nickerson, L. D., Nichols, T. E., and Gao, J.-H. (2017). Comparison of a non-stationary voxelation-corrected cluster-size test with TFCE for group-Level MRI inference. Hum. Brain Mapp. 38, 1269–1280. doi: 10.1002/hbm.23453
Mason, M. F., Norton, M. I., Van, Horn JD, Wegner, D. M., Grafton, S. T., and Macrae, C. N. (2007). Wandering minds: the default network and stimulus-independent thought. Science 315, 393–395. doi: 10.1126/science.1131295
McKiernan, K. A., Kaufman, J. N., Kucera-Thompson, J., and Binder, J. R. (2003). A parametric manipulation of factors affecting task-induced deactivation in functional neuroimaging. J. Cogn. Neurosci. 15, 394–408. doi: 10.1162/089892903321593117
Medow, M. S., Sood, S., Messer, Z., Dzogbeta, S., Terilli, C., and Stewart, J. M. (2014). Phenylephrine alteration of cerebral blood flow during orthostasis: effect on n-back performance in chronic fatigue syndrome. J. Appl. Physiol. 117, 1157–1164. doi: 10.1152/japplphysiol.00527.2014
Müller, N. C. J., Dresler, M., Janzen, G., Beckmann, C. F., Fernández, G., and Kohn, N. (2020). Medial prefrontal decoupling from the default mode network benefits memory. Neuroimage 210:116543. doi: 10.1016/j.neuroimage.2020.116543
Nakatomi, Y., Mizuno, K., Ishii, A., Wada, Y., Tanaka, M., Tazawa, S., et al. (2014). Neuroinflammation in patients with chronic fatigue syndrome/myalgic encephalomyelitis: an11C-(R)-PK11195 PET study. J. Nucl. Med. 55, 945–950. doi: 10.2967/jnumed.113.131045
National Institute for Health and Care Excellence [NICE] (2021). Myalgic Encephalomyelitis (or Encephalopathy)/Chronic Fatigue Syndrome: Diagnosis and Management. NICE Guideline [NG206]. Available online at: https://www.nice.org.uk/guidance/ng206 (accessed October 29, 2021).
Ocon, A. J., Messer, Z. R., Medow, M. S., and Stewart, J. M. (2012). Increasing orthostatic stress impairs neurocognitive functioning in chronic fatigue syndrome with postural tachycardia syndrome. Clin. Sci. (Lon.) 122, 227–238. doi: 10.1042/CS20110241
Pardini, M., Krueger, F., Raymont, V., and Grafman, J. (2010). Ventromedial prefrontal cortex modulates fatigue after penetrating traumatic brain injury. Neurology 9, 749–754.
Pruim, R. H. R., Mennes, M., Buitelaar, J. K., and Beckmann, C. F. (2015a). Evaluation of ICA-AROMA and alternative strategies for motion artifact removal in resting state fMRI. Neuroimage 112, 278–287. doi: 10.1016/j.neuroimage.2015.02.063
Pruim, R. H. R., Mennes, M., van Rooij, D., Llera, A., Buitelaar, J. K., and Beckmann, C. F. (2015b). ICA-AROMA: a robust ICA-based strategy for removing motion artifacts from fMRI data. Neuroimage 112, 267–277. doi: 10.1016/j.neuroimage.2015.02.064
Puttaert, D., Coquelet, N., Wens, V., Peigneux, P., Fery, P., Rovai, A., et al. (2020). Alterations in resting-state network dynamics along the Alzheimer’s disease continuum. Sci. Rep. 10:21990. doi: 10.1038/s41598-020-76201-3
Raichle, M. E. (2015). The Brain’s default mode network. Annu. Rev. Neurosci. 8, 433–447. doi: 10.1146/annurev-neuro-071013-014030
Rayhan, R. U., Stevens, B. W., Raksit, M. P., Ripple, J. A., Timbol, C. R., Adewuyi, O., et al. (2013). Exercise challenge in gulf war illness reveals two subgroups with altered brain structure and function. PLoS One 8:e63903. doi: 10.1371/journal.pone.0063903
Rayhan, R. U., Washington, S. D., Garner, R., Zajur, K., Martinez Addiego, F., Vanmeter, J. W., et al. (2019). Exercise challenge alters default mode network dynamics in Gulf War Illness. BMC Neurosci. 20:7. doi: 10.1186/s12868-019-0488-6
Razumovsky, A. Y., DeBusk, K., Calkins, H., Snader, S., Lucas, K. E., Vyas, P., et al. (2003). Cerebral and systemic hemodynamics changes during upright tilt in chronic fatigue syndrome. Neuroimaging 13, 57–67. doi: 10.1152/ajpheart.00994.2011
Roy, M., Shohamy, D., and Wager, T. D. (2012). Ventromedial prefrontal-subcortical systems and the generation of affective meaning ventromedial prefrontal cortical involvement across psychological. Trends Cogn. Sci. 16, 147–156. doi: 10.1016/j.tics.2012.01.005.Ventromedial
Schilbach, L., Eickhoff, S. B., Rotarska-Jagiela, A., Fink, G. R., and Vogeley, K. (2008). Minds at rest? Social cognition as the default mode of cognizing and its putative relationship to the “default system” of the brain. Conscious. Cogn. 17, 457–467. doi: 10.1016/j.concog.2008.03.013
Smith, S. M., Fox, P. T., Miller, K. L., Glahn, D. C., Fox, P. M., Mackay, C. E., et al. (2009). Correspondence of the brain’s functional architecture during activation and rest. Proc. Natl. Acad. Sci. U.S.A. 106, 13040–13045. doi: 10.1073/pnas.0905267106
Smith, S. M., Jenkinson, M., Woolrich, M. W., Beckmann, C. F., Behrens, T. E. J., Johansen-Berg, H., et al. (2004). Advances in functional and structural MR image analysis and implementation as FSL. Neuroimage 23, S208–S219. doi: 10.1016/j.neuroimage.2004.07.051
Spreng, R. N., and Grady, C. L. (2010). Patterns of brain activity supporting autobiographical memory, prospection, and theory of mind, and their relationship to the default mode network. J. Cogn. Neurosci. 22, 1112–1123. doi: 10.1162/jocn.2009.21282
Steele, L. (2000). Prevalence and patterns of Gulf War illness in Kansas veterans: association of symptoms with characteristics of person, place, and time of military service. Am. J. Epidemiol. 152, 992–1002. doi: 10.1093/aje/152.10.992
Stewart, J. M., Medow, M. S., Messer, Z. R., Baugham, I. L., Terilli, C., and Ocon, A. J. (2011). Postural neurocognitive and neuronal activated cerebral blood flow deficits in young chronic fatigue syndrome patients with postural tachycardia syndrome. Am. J. Physiol. Heart Circ. Physiol. 302, 1185–1194.
Tajima, S., Yamaoto, S., Tanaka, M., Kataoka, Y., Masoa, I., Yoshikawa, E., et al. (2010). Medial orbitofrontal cortex is associated with fatigue sensation. Neurol. Res. Int. 2010:671421. doi: 10.1155/2010/671421
Uddin, L. Q., Clare Kelly, A. M., Biswal, B. B., Xavier Castellanos, F., and Milham, M. P. (2009). Functional connectivity of default mode network components: correlation, anticorrelation, and causality. Hum. Brain Mapp. 30, 625–637. doi: 10.1002/hbm.20531
van Campen, C. L. M. C., Rowe, P. C., Verheugt, F. W. A., and Visser, F. C. (2020a). Cognitive function declines following orthostatic stress in adults with Myalgic Encephalomyelitis/Chronic Fatigue syndrome (ME/CFS). Front. Neurosci. 14:688. doi: 10.3389/fnins.2020.00688
van Campen, C. L. M. C., Rowe, P. C., Verheugt, F. W. A., and Visser, F. C. (2020b). Orthostatic stress testing in myalgic encephalomyelitis/chronic fatigue syndrome patients with or without concomitant fibromyalgia: effects on pressure pain thresholds and temporal summation. Clin. Exp. Rheumatol. 39(Suppl 130), 39–47.
van Campen, C. L. M. C., Verheugt, F. W. A., Rowe, P. C., and Visser, F. C. (2020c). Cerebral blood flow is reduced in ME/CFS during head-up tilt testing even in the absence of hypotension or tachycardia: a quantitative, controlled study using Doppler echography. Clin. Neurophysiol. Pract. 5, 50–58. doi: 10.1016/j.cnp.2020.01.003
Vanasse, T. J., Fox, P. T., Fox, P. M., Cauda, F., Costa, T., Smith, S. M., et al. (2021). Brain pathology recapitulates physiology: a network meta-analysis. Commun. Biol. 4, 301. doi: 10.1038/s42003-021-01832-9
Vargas, C., ópez-Jaramillo, C. L., and Vieta, E. (2013). A systematic literature review of resting state network-functional MRI in bipolar disorder. J. Affect. Disord. 150, 727–735. doi: 10.1016/j.jad.2013.05.083
Vilagut, G., Forero, C. G., Barbaglia, G., and Alonso, J. (2016). Screening for depression in the general population with the center for epidemiologic studies depression (CES-D): a systematic review with meta-analysis. PLoS One 11:e0155431. doi: 10.1371/journal.pone.0155431
Washington, S. D., Rayhan, R. U., Garner, R., Provenzano, D., Zajur, K., Addiego, F. M., et al. (2020a). Exercise alters brain activation in gulf war illness and Myalgic Encephalomyelitis/Chronic Fatigue Syndrome. Brain Commun. 2:fcaa070. doi: 10.1093/braincomms/fcaa070
Washington, S. D., Rayhan, R. U., Garner, R., Provenzano, D., Zajur, K., Addiego, F. M., et al. (2020b). Exercise alters cerebellar and cortical activity related to working memory in phenotypes of Gulf War Illness. Brain Commun. 2:fcaa070. doi: 10.1093/braincomms/fcz039
White, R. F., Steele, L., O’Callaghan, J. P., Sullivan, K., Binns, J. H., Golomb, B. A., et al. (2016). Recent research on Gulf War illness and other health problems in veterans of the 1991 Gulf War: effects of toxicant exposures during deployment. Cortex 74, 449–475. doi: 10.1016/j.cortex.2015.08.022
Keywords: postexertional malaise, default mode network, DMN, blood oxygenation level dependent, BOLD, fibromyalgia, chronic idiopathic fatigue, submaximal exercise
Citation: Rayhan RU and Baraniuk JN (2021) Submaximal Exercise Provokes Increased Activation of the Anterior Default Mode Network During the Resting State as a Biomarker of Postexertional Malaise in Myalgic Encephalomyelitis/Chronic Fatigue Syndrome. Front. Neurosci. 15:748426. doi: 10.3389/fnins.2021.748426
Received: 27 July 2021; Accepted: 15 November 2021;
Published: 15 December 2021.
Edited by:
George Alexandrakis, University of Texas at Arlington, United StatesReviewed by:
Keerthana Deepti Karunakaran, Harvard University, United StatesHaiqing Huang, University of Pittsburgh, United States
Copyright © 2021 Rayhan and Baraniuk. This is an open-access article distributed under the terms of the Creative Commons Attribution License (CC BY). The use, distribution or reproduction in other forums is permitted, provided the original author(s) and the copyright owner(s) are credited and that the original publication in this journal is cited, in accordance with accepted academic practice. No use, distribution or reproduction is permitted which does not comply with these terms.
*Correspondence: James N. Baraniuk, YmFyYW5pdWpAZ2VvcmdldG93bi5lZHU=