- Department of Speech, Language, and Hearing Sciences, University of Texas at Austin, Austin, TX, United States
The efferent auditory nervous system may be a potent force in shaping how the brain responds to behaviorally significant sounds. Previous human experiments using the frequency following response (FFR) have shown efferent-induced modulation of subcortical auditory function online and over short- and long-term time scales; however, a contemporary understanding of FFR generation presents new questions about whether previous effects were constrained solely to the auditory subcortex. The present experiment used sine-wave speech (SWS), an acoustically-sparse stimulus in which dynamic pure tones represent speech formant contours, to evoke FFRSWS. Due to the higher stimulus frequencies used in SWS, this approach biased neural responses toward brainstem generators and allowed for three stimuli (/bɔ/, /bu/, and /bo/) to be used to evoke FFRSWS before and after listeners in a training group were made aware that they were hearing a degraded speech stimulus. All SWS stimuli were rapidly perceived as speech when presented with a SWS carrier phrase, and average token identification reached ceiling performance during a perceptual training phase. Compared to a control group which remained naïve throughout the experiment, training group FFRSWS amplitudes were enhanced post-training for each stimulus. Further, linear support vector machine classification of training group FFRSWS significantly improved post-training compared to the control group, indicating that training-induced neural enhancements were sufficient to bolster machine learning classification accuracy. These results suggest that the efferent auditory system may rapidly modulate auditory brainstem representation of sounds depending on their context and perception as non-speech or speech.
Introduction
The mammalian auditory system contains extensive efferent innervation descending from the cortex to subcortex and inner ear (Winer, 2005). Numerous animal modeling studies suggest that these projections facilitate neuroplastic functional changes on multiple time scales and at multiple levels of the subcortical auditory system. For example, online modulation of auditory function has been observed at the level of the cochlea (Xiao and Suga, 2002; May et al., 2004; Dragicevic et al., 2015; Terreros and Delano, 2015; Delano and Elgoyhen, 2016; Lauer et al., 2021), cochlear nucleus (Hernandez-Peon et al., 1956), and inferior colliculus (Slee and David, 2015; Shaheen et al., 2021). Short- and long-term training also alters physiologic function in the same structures (Gao and Suga, 1998, 2000; Yan and Suga, 1998, 1999; Suga et al., 2000, 2002; Ji et al., 2001; Ma and Suga, 2001; Yan et al., 2005; Malmierca et al., 2009). Inversely, obliterating or temporarily silencing corticofugal efferent connections disrupts online modulation and short- and long-term training effects measured subcortically (e.g., Bajo et al., 2010; León et al., 2012). Together, these studies suggest that efferent activity is a potent force in shaping how the nervous system responds to behaviorally significant sounds, even at the earliest stages of auditory processing.
Efferent-induced changes in human subcortical auditory function have, by necessity, almost exclusively been assessed through non-invasive objective measurements. Some reports have demonstrated that otoacoustic emissions (i.e., proxy measures of outer hair cell function) are modulated online by attention (Wittekindt et al., 2014; Smith and Cone, 2015; Hernandez-Perez et al., 2021) or through short- or long-term training (Perrot et al., 2006; de Boer and Thornton, 2008; Bidelman et al., 2014, 2016, 2017). Other reports using similar methodologies have failed to replicate these findings (Stuart and Butler, 2012; Francis et al., 2018; Jedrzejczak et al., 2020). A variety of electrophysiologic measures has been used to study online or training-based neuroplastic functional changes in the human auditory subcortex including the auditory brainstem response (ABR) and frequency following response (FFR). As a general principle, the “classic” ABR does not appear to be altered by attention (Picton and Hillyard, 1974; Woldorff et al., 1987; Connolly et al., 1989; Gregory et al., 1989; Hackley et al., 1990), whereas the FFR literature presents a less cohesive narrative. Seminal work by Galbraith and Arroyo (1993) and Galbraith et al. (1995, 1998, 2003) suggested that FFRs to simple (e.g., tonal) and complex (e.g., dichotic speech) stimuli were modulated during auditory or visual attention. While some researchers have replicated these findings (e.g., Hairston et al., 2013; Lehmann and Schönwiesner, 2014), others have failed to observe attention effects and have questioned whether previous results were influenced by task-based differences in FFR residual noise (Ruggles et al., 2012; Varghese et al., 2015). More recent studies demonstrate FFR enhancements during active listening to ecologically valid continuous speech (Forte et al., 2017; Etard et al., 2019; Saiz-Alía et al., 2019).
A larger body of FFR literature supports the supposition that short- and long-term training induce neuroplastic changes in the auditory subcortex over time. Studies in which listeners were trained to discriminate stimuli by focusing on a specific sound feature (e.g., global pitch or dynamic pitch contours) have reported enhancement of the neural representation of the trained feature (e.g., Russo et al., 2005; Song et al., 2008, 2012; Carcagno and Plack, 2011; Chandrasekaran et al., 2012; Skoe et al., 2014). These changes were noted after multiple hours or days of training; however, additional studies have reported rapid FFR modulation occurring within minutes of training onset (e.g., Skoe and Kraus, 2010; Skoe et al., 2013). Similar and more robust enhancements are observed in musicians (Wong et al., 2007; Bidelman and Krishnan, 2009) and tonal language speakers (Krishnan et al., 2005; Swaminathan et al., 2008; Krishnan and Gandour, 2009) who, by virtue of their lived experiences, have undergone a form of long-term auditory training (see Kraus and Chandrasekaran, 2010; Strait and Kraus, 2014; Kraus and White-Schwoch, 2015 for reviews).
The majority of FFR studies examining online or training-related changes in neural function have focused on neural representation of the speech envelope and its harmonics (FFRENV). Recent evidence suggests that although the FFRENV arises primarily from the auditory subcortex (Chandrasekaran and Kraus, 2010; Bidelman, 2015; Bidelman and Powers, 2018; Bidelman et al., 2018a), cortical contributions may also be present, particularly for stimuli with fundamental frequencies < ∼150 Hz (Coffey et al., 2016, 2019). This new understanding of FFRENV origins presents the possibility that neuroplastic changes observed in some previous studies may not be constrained to the subcortex. One way to ensure that measured neural responses are biased exclusively toward subcortical generators is to use stimuli comprised of behaviorally-significant higher frequency (>200 Hz) speech content, as more caudal generators begin to dominate the FFR with increasing stimulus frequencies (Gardi et al., 1979; Galbraith et al., 2001; Tichko and Skoe, 2017).
Sine-wave speech (SWS) is an acoustically manipulated form of speech in which formant trajectories are represented by time-variant sine waves, and the remainder of the acoustic signal is discarded (Remez et al., 1981). It can therefore be conceptualized as speech “fine structure” that has been spectrally reduced to two or three dynamic frequency components. The range of average first (F1) and second (F2) formant frequencies in adult American English speakers is ∼300–775 and ∼900–2,700 Hz, respectively (Lindblom, 1990). Because the upper frequency limit of the FFR is approximately ∼1,200–1,300 Hz (Bidelman and Powers, 2018), much of the F1 and F2 formant space may be captured by FFRs evoked by SWS (FFRSWS). A critical advantage of SWS is that naïve listeners do not hear it as speech (Remez et al., 1981; Barker and Cooke, 1999; Möttönen et al., 2006); however, with minimal instruction and/or training, listeners achieve a high level of SWS comprehension. Consequently, it is possible to use identical speech-like stimuli to evoke FFRSWS pre- and post-engagement of the auditory efferent system through online or brief short-term training activities. While the neural networks involved in this top-down process are not fully understood, recent reports examining cortical responses to SWS (or vocoded speech) indicate that activity from different brain networks is involved based on whether the signals are perceived as speech or non-speech (Davis and Johnsrude, 2003; Eisner et al., 2010; Hervais-Adelman et al., 2012; Khoshkhoo et al., 2018). Specifically, SWS is represented in the auditory cortex based on “bottom-up” acoustic features in naïve listeners. When listeners undergo a perceptual shift and begin to understand these degraded signals as speech, left inferior frontal cortex activity increases significantly while auditory cortex activity remains stable (Khoshkhoo et al., 2018). Given the observations that active listening sequentially modulates neural tuning in the same cortical networks in a top-down fashion (e.g., Atiani et al., 2014), it is possible that these modulatory effects continue into the auditory brainstem via the efferent system (Bidelman et al., 2019; Price and Bidelman, 2021).
In the present experiment, three SWS tokens, differing mainly in their F1 contours, were used to evoke FFRSWS before and after a brief auditory training paradigm in which listeners were informed that they were listening to degraded speech and were asked to classify each token. These results were compared to FFRSWS measured from a control group, which did not undergo training. FFRSWS were confirmed to be of neural origin with latencies suggesting brainstem generators and high stimulus-to-response cross-correlations. In the test group, all SWS stimuli were rapidly perceived as speech when presented with a SWS carrier phrase in a brief training phase, and average token identification reached ceiling performance within 25 training trials or less per stimulus. FFRSWS amplitudes in the test group were enhanced post-training for each stimulus compared to the control group. Further, linear support vector machine classification of FFRSWS significantly improved post-training in the test group compared to controls, indicating that training-induced neural enhancements were sufficient to bolster machine learning classification accuracy. These results suggest that the efferent auditory system may rapidly modulate auditory brainstem representation of sounds depending on their context and perception as non-speech or speech.
Materials and Methods
Participants
This study was approved by the University of Texas at Austin Institutional Review Board. Eighteen adults (mean age = 22.2 years) with no history of audiologic or neurologic injury were enrolled. Half of the participants were placed in a training group and the other half served as untrained controls. Participants had normal hearing (≤25 dB HL) from 250 to 8,000 Hz bilaterally. Each participant provided written consent and completed 3 h of testing for which they were compensated.
Sine Wave Speech Stimuli
Three naturally produced CV speech tokens, /bɔ/, /bu/, and /bo/, were recorded (44,100 Hz sampling rate) from an adult male speaker with a Standard American English accent. The speaker was told to maintain constant voice pitch across all recordings. Each CV token was 335 ms in duration, and cosine squared ramps were applied to the last 50 ms of each stimulus to equate and smooth offsets across stimuli. The natural CV tokens were then converted to SWS in Praat software (Boersma, 2009) using the approach developed by Darwin (2003). This approach uses linear predictive coding analysis to identify formant center frequencies and amplitudes within a sliding window over the stimulus. The formants are then replaced with time-varying sinusoids, and all other speech content is discarded (Figure 1). Only the first two formants from the original stimuli were kept, as FFRs were unlikely to be evoked by higher frequency formants. A carrier phrase (“The word is ____.”) that was only used in the brief training phase for the training group (described below) was also converted to SWS in the same manner described above. All SWS stimuli were RMS normalized to ensure equal presentation level.
The three vowels in the CV stimuli were selected for multiple reasons related to their relative positions in the F1/F2 formant space. First, phase-locking in the auditory nervous system becomes poorer as stimulus frequency increases. Consequently, stimuli comprised of lower frequencies generate more robust FFR responses (e.g., Bidelman and Powers, 2018). The vowels /ɔ/, /u/, and /o/ have the lowest possible F1 and F2 frequencies in American English and are therefore the most ideal SWS candidates for evoking robust FFRsWS. Second, the vowels primarily differ in their F1 contours, whereas the F2 contours are less disparate. The range in F1 frequencies for the three vowels was ∼300–675 Hz, whereas the range in F2 was ∼1,100–1,300 Hz. Because CV differences were most pronounced in their F1 frequencies, we anticipated that listeners in the training group would primarily focus on this feature to successfully complete the auditory training task and that neural enhancement related to the brief training period would be apparent at the F1 frequency (described below). Third, the total range of F1 and F2 stimulus frequencies (∼300–1,300 Hz) biases the FFR to reflect more caudal subcortical generators (e.g., Galbraith et al., 2001; Bidelman, 2018).
Procedure
Training Group
All experimental procedures occurred in a double-walled sound booth with participants seated in a reclining chair. Auditory stimuli were presented diotically through electromagnetically shielded ER-3 insert earphones (Etymotic Research, Elk Grove Village, IL), and visual prompts (used only in the training phase) were presented through a Dell PC monitor. Experiment stimuli were programmed and controlled via Neuroscan’s GenTask module (Compumedics Neuroscan, Charlotte, NC). The experiment began with a pre-training phase in which FFRSWS were evoked by /bɔ/, /bu/, and /bo/ SWS tokens presented in random order. Half of the stimulus presentations were in one polarity (“Polarity A”) and half were in the opposite polarity (“Polarity B”). Each stimulus was presented in each polarity 1,000 times for a total of 6,000 sweeps. The intertrial interval between stimuli was 600 ms. During the pre-training phase, each participant was asked to remain still while quietly watching a subtitled movie or show of his or her choosing.
The training phase of the experiment began upon conclusion of the pre-training phase and after a brief break. Participants were notified that the stimuli they were hearing in the previous block were modified speech signals and that the training phase would require them to learn and identify the speech signals using a response keypad (Compumedics Neuroscan, Charlotte, NC). No additional instruction was given. At the beginning of each training trial, participants heard the SWS carrier phrase “The word is _____.,” with one of the three SWS tokens randomly presented as the target word. Simultaneously to the auditory presentation of the carrier phrase and target word, the participants saw a visual prompt on a monitor located directly in front of them and outside of the sound booth. The prompt depicted a visual representation of the carrier phrase with a blank in the target word space, exactly as written in the italicized quote above. The total duration of the carrier phrase and target word was 1,500 ms; an additional 500 ms of silence was appended to the end of each carrier and target presentation to encourage participants to remain still prior to pressing the response keypad to submit their answer following the next prompt. Participants then saw a slide on the monitor with possible target words written non-phonetically as “bah,” “boo,” or “bow.” The participant indicated which SWS word was heard by pressing one of three buttons on the response keypad, which was then followed by a 600 ms intertrial interval. Visual feedback (“Correct” or “Incorrect”) was then given to participants, which was followed by another 600 ms interval before the onset of the next trial. This procedure was repeated 25 times per stimulus for a total of 75 training trials. Relatively few training trials were chosen based on previous reports that SWS becomes rapidly intelligible with very little training (Remez et al., 1981; Möttönen et al., 2006).
A testing phase followed the training phase. The main purpose of the testing phase was to ensure that participants retained SWS identification accuracy in the absence of the carrier phrase, which provided additional “samples” of the speaker’s formant structure. In the testing phase, each trial began with the random presentation of a SWS target token. After a 600 ms pause, participants were invited to indicate their responses on a keypad, using the same slide described above with written target words as a reference. Participants had 900 ms from the onset to indicate their responses. Feedback was not provided in the test phase. Following training and testing phases, a post-training phase, parametrically identical to the pre-training phase, was conducted.
Control Group
The control group underwent passive FFRSWS measurements that were identical to pre-training and post-training measurements in the training group. In place of the SWS training and testing phases, the control group was asked to watch an unrelated captioned television show and answer comprehension questions related to its content. While control group participants watched the captioned television show, they were exposed to the same carrier sentences as the test-group; however, they were never told that they were hearing modified speech at any point of the experiment. None of the control participants perceived the SWS stimuli to be speech according to a post-experiment survey. The purpose of including the control group in this study was to determine if pre- and post-training FFRSWS enhancements in the test group were simply related to exposure to the SWS stimuli during the recording session and not due to efferent modulation following a perceptual shift from non-speech to speech perception. Note that, for simplicity, we refer to the first and second passive FFRSWS measurements for test and control groups as “pre- and post-training” measurements throughout the manuscript, even though the control group did not undergo auditory training.
EEG Acquisition and Pre-processing
Electrophysiologic responses were obtained with a Neuroscan SynAmps2 system (Compumedics Neuroscan, Charlotte, NC). Responses were recorded at a 5,000 Hz sampling rate via a single-channel bipolar montage, Fpz (+), C7 vertebra (−), forehead (GND), and amplified by a factor of 100,000. Continuous data were exported from Curry 8 software, and further analyses were performed offline in MATLAB (The MathWorks, Natick, MA). All continuous data were first bandpass filtered from 100 to 2,400 Hz. For pre- and post-training FFRSWS, continuous responses were epoched from −50 to 550 ms (re: SWS token onset), and single-trial responses were grouped by stimulus type. Responses were corrected for insert earphone delays by subtracting 1 ms from the epoched data. Epochs were detrended, artifact rejected at ± 50 μV, and baseline corrected. Remaining sweeps were used to create grand average FFRSWS for each stimulus such that individual polarities (A and B) as well as “added” [(A+B)/2] and subtracted [(A−B)/2] waveforms could be independently evaluated. Individual polarities and subtracted waveforms were used in a cross-correlation analysis (described below) to verify that FFRSWS were neural in origin. Added polarity responses are generally used to accentuate neural representation of the envelope (Aiken and Picton, 2008). Because the stimuli in the present study did not have envelopes, added polarity waveforms were evaluated mainly as a quality control measure to ensure that FFRSWS were not obliterated (which indicates that the measured responses are stimulus artifact or cochlear microphonic). In some cases, low amplitude waveforms containing energy at F1*2 were observed in the added polarity. This likely occurs because phase locked neural responses evoked by one stimulus polarity are temporally shifted by a half-cycle relative to the opposite polarity due to half-wave rectification (see Aiken and Picton, 2008; Lichtenhan et al., 2013). Adding these responses together can produce a doubling of the stimulus frequency and provides additional evidence that the measured responses are from neural generators.
Analyses
Test Group Training- and Testing-Phase Response Accuracy and Reaction Time
Training group response accuracy and reaction time were evaluated using behavioral data from training and test phases, respectively, as both measures are indicative of auditory training effects (e.g., Ritter et al., 1972; Song et al., 2008). Response accuracy, defined binarily on each trial as “correct” or “incorrect,” was analyzed using mixed effects logistic regression with trial number and stimulus type as independent variables. Reaction time, defined as the post-stimulus onset time (re: to SWS target) at which respondents pressed the response keypad to indicate their choice, was evaluated using multiple linear regression with trial number and stimulus type as independent variables.
Stimulus-to-Response Cross-Correlation
Electromagnetic stimulus artifact, cochlear microphonic, and FFR waveforms can all mimic periodic characteristics of the input stimulus. A common method used to evaluate whether measured electrophysiologic responses are from neural generators or non-neural contaminants is to perform a cross-correlation between the stimulus and response. In this procedure, correlations between stimulus and FFR waveforms are calculated as the FFR waveform is temporally shifted relative to the stimulus waveform on a point-by-point basis (Skoe and Kraus, 2010). The time lag that produces the largest correlation coefficient is an estimated delay between stimulus and response. Responses generated by the auditory nerve and brainstem are expected to have a delay of ∼3–10 ms, depending on the electrode montage, stimulus frequency, and interaction between multiple neural generators as they reach scalp electrodes (e.g., Galbraith et al., 2001; Tichko and Skoe, 2017; Bidelman, 2018). In contrast, cochlear microphonic (arising from hair cell alternating currents primarily in the basal tail of the basilar membrane traveling wave; see Eggermont, 2017 for review) and stimulus artifact have short delays of ∼0–1 ms (Gardi et al., 1979). Stimulus-to-response cross-correlations were calculated for individual polarities (A and B) and subtracted waveforms evoked by each SWS stimulus in the pre- and post-training phases for test and control groups. SWS stimuli were first down-sampled from 44,100 to 5,000 Hz to match the FFRSWS sampling rate, resulting in 0.2 ms precision in delay estimates. The maximum possible time delay producing the largest correlation coefficient was constrained between ±20 ms. Responses for which the estimated delays were within 3–10 ms were considered to be of neural origin. These responses were kept for further analysis. Cross-correlation coefficients, which are constrained between −1 and 1 and are non-normally distributed, were transformed to Fisher z-values (Cohen et al., 2013). A three-way multiple analysis of variance (MANOVA) with repeated measures was conducted to assess the impacts of group (test vs. control). training status (pre- vs. post-training), and stimuli (/bɔ/, /bu/, and /bo/) on participants’ FFRSWS latencies and z-transformed cross-correlation coefficients.
Fourier Analyzer
In contrast to a Fourier transform, which is commonly used to analyze steady-state stimuli/responses, a Fourier analyzer (FA) provides a better estimate of response amplitudes at frequencies of interest for signals with time-varying spectra (Aiken and Picton, 2006). Because FFRs are expected to follow dynamic frequency changes of a stimulus over time, the FA uses the stimulus frequency trajectory as a “reference” to detect FFR spectral amplitudes at frequencies along this trajectory by integration (Aiken and Picton, 2006). The stimuli used in the present study have non-stationary F1 and F2. Therefore, an FA was implemented to calculate response amplitudes in frequency bins corresponding to F1 and F2 trajectories to determine the strength of neural phase locking to each simulated formant.
We used a similar approach to implement FA as described by Aiken and Picton (2006) and Choi et al. (2013). First, stimulus reference tracks following F1 and F2, respectively, were created by exporting only F1 or F2 SWS sine-waves from Praat. Complex representations of F1 and F2 stimuli were obtained by Hilbert transform, and the instantaneous phase was calculated by finding the angle of the output of the Hilbert transform. F1 and F2 instantaneous frequencies were then calculated as the derivatives of the unwrapped phases at each time point. Since calculating the derivatives in this manner is equivalent to applying a high-pass filter, it introduces sharp perturbations in the resulting frequency tracks. Consequently, we smoothed the obtained instantaneous frequencies across time by applying a 50-point boxcar moving average 3 times. Reference complex sinusoids were then created for F1 and F2 frequency tracks using the instantaneous phase angles for each stimulus.
As mentioned above, FFRs demonstrate a characteristic delay between stimulus and response of ∼3–10 ms due to neural conduction time. FFRSWS waveforms were shifted by – 6 ms based on pilot data testing to correct for neural delays and ensure that reference tracks were, on average, temporally aligned with the FFRSWS waveforms prior to integration (Purcell et al., 2004; Aiken and Picton, 2006). Reference tracks and FFRSWS waveforms were then integrated by multiplying the two waveforms over time (Choi et al., 2013) and computing the mean of the obtained complex numbers. The absolute value and the angle of the mean were then calculated as the FFR amplitude and the phase over the duration of the response (50–335 ms), respectively.
In order to determine whether FFRSWS amplitudes at F1 and F2 were above background noise levels, 10 adjacent frequency tracks were also created to measure response amplitudes at non-stimulus frequencies. Five noise tracks above and five below each F1 and F2 track were obtained by adding or subtracting a fixed number of cycles per second. Noise tracks began at F1 ± 5 and F2 ± 5 Hz, respectively, and increased or decreased in 1 Hz steps. The same FA procedures as above were then used to estimate noise levels in the 10 adjacent non-stimulus frequency bins. F1 and F2 responses were deemed “present” if their amplitudes exceeded the noise floor averaged across 10 adjacent frequency bins. This approach is more lenient than other statistically based methods for determining response presence/absence (e.g., F-tests or Hotelling’s T2-tests; see Picton et al., 2003 for review). However, because the primary focus of this study was to evaluate potential enhancement of FFRSWS following perceptual shifts, we did not want to remove participants who had low baseline FFRSWS.
Machine Learning Classification of FFRSWS
Previous experiments have used machine learning algorithms to assess whether the information contained in FFRs is sufficient to decode the stimulus classes that evoked them (Sadeghian et al., 2015; Holdgraf et al., 2017; Llanos et al., 2017; Yi et al., 2017; Xie et al., 2018, 2019). Under this approach, FFR classification performance (i.e., the accuracy with which FFRs are correctly classified by the machine learning algorithm) serves as an objective measure of stimulus discrimination. Importantly, FFR classification accuracy can be compared between levels of an independent variable (e.g., training or attention conditions) to determine how these factors impact classification performance (e.g., Xie et al., 2018). The rationale is that, if attention or training modulate neural function as captured by the FFR, the accuracies with which FFRs are classified should reflect this modulation via improving (enhancement) or declining (suppression) classification accuracy.
A MATLAB-constructed linear support vector machine (SVM; Cristianini and Shawe-Taylor, 2000) was used to classify pre- and post-training FFRSWS for test and control groups following the general procedures described by Xie et al. (2019). We first epoched all subtracted FFRSWS waveforms from 0 to 380 ms and used these 1,900 amplitude-by-time points as linear SVM input features. The model outputs were stimulus type (/bɔ/, /bu/, and /bo/). Because standard linear SVM can only classify data into binary classes, a one-against-one strategy was used. In this approach, the linear SVM constructs N(N-1)/2 classifiers, where N is the number of classes; N = 3 in this experiment, as three SWS stimuli were used. After FFR classification is performed on all possible pairwise combinations, the class with the highest accuracy is used as the classification label.
The model was cross-validated using a three-fold approach that was repeated 2,500 times (see Xie et al., 2019; Figure 1). For each iteration of the linear SVM classifier, participants were randomly and equally divided into 3 groups (or folds). A “leave-one-out” strategy then used two of the three-folds to train the classifier. After training the classifier, the held-out fold was used as test data. This was repeated within each iteration such that each fold was held-out as the test data and the other two-folds were used for training the classifier. The average classifier accuracy across cross-validations was calculated for each iteration. Outcomes of the 2,500 iterations were also used to create grand total cross-validation accuracies as well as a distribution of accuracies. A null distribution of model accuracies was also generated using the steps above, with the exception that model outputs (i.e., stimulus labels) were randomly assigned to FFRSWS inputs on each iteration of the loop. Statistical significance of “true” classifier performance was determined using p = (a + 1)/(n + 1), where a denotes the number of observations from the null classification distribution that surpasses the median of the “true” distribution and n is the total number of observations comprising the null distribution (Phipson and Smyth, 2010, as cited in Xie et al., 2019). The same equation was also used to test whether pre- and post-training FFRSWS classification accuracy distributions were significantly different for test and control groups.
Results
Test Group Training- and Testing-Phase Behavioral Performance
Modeled accuracy and reaction times for training and test phases are plotted in Figure 2. For the training phase, the mixed effects logistic regression model containing training time and stimulus type as predictors was statistically significant [X2(2) = 37.31, p < 0.001]. When holding stimulus type constant, the odds of a correct response increased by 3% [95% CI (0.13, 0.47)] for a one-unit increase in trials. When holding trial count constant, the accuracy decreased by 6% [95% CI (−0.76, −0.41)] when changing from /bɔ/ to /bu/ and /bu/ to /bo/. The multiple linear regression model evaluating reaction time suggested that training time and stimuli explained 9% of the variance, [R2 = 0.09, F(2, 672) = 33,28, p < 0.001]. When holding stimulus type constant, training time significantly predicted reaction time, β = −7.73, t = −8.09, p < 0.001, suggesting the reaction time decreased when the training time increased. When holding training time constant, stimulus type did not significantly predict reaction time (β = 15.62, t = 0.62, p = 0.54).
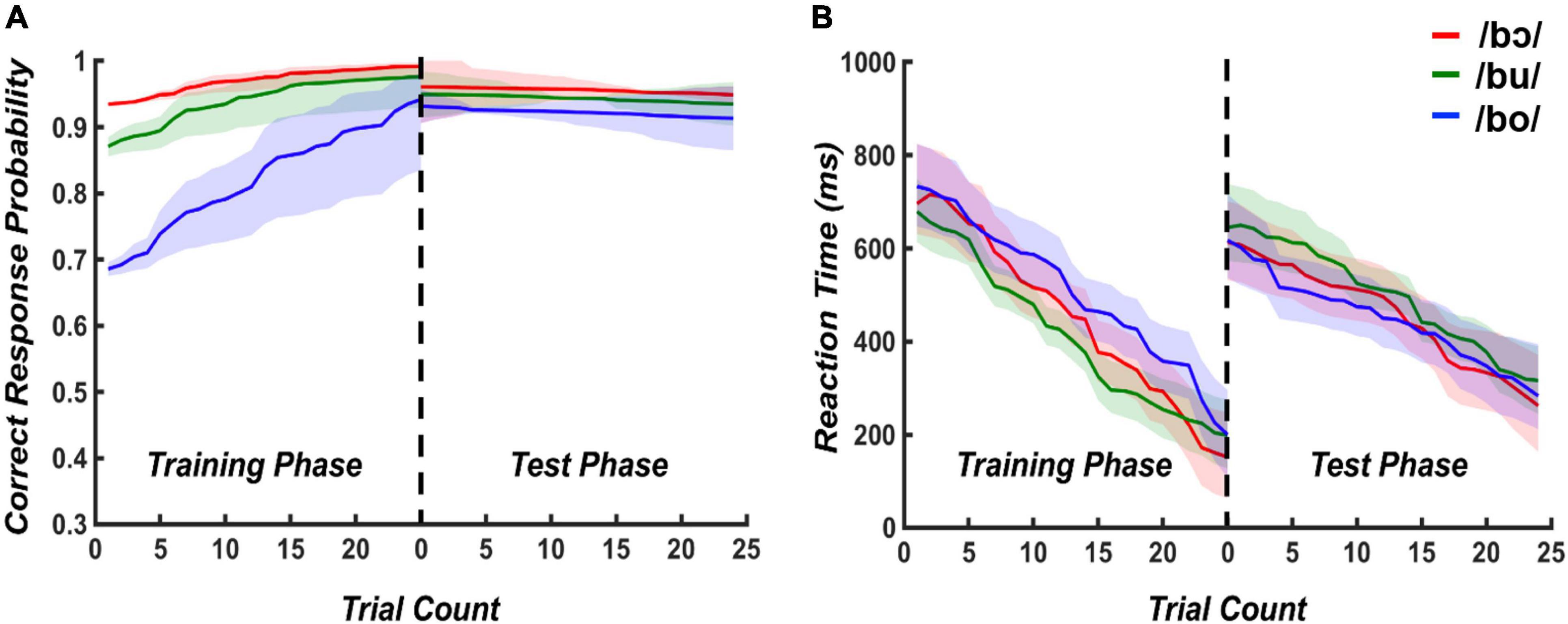
Figure 2. Modeled accuracy and reaction times for training and testing phases. Logistic and multiple linear regression model outputs using stimulus type and trial count as predictors were used to plot predicted accuracy (A) and reaction times (B), respectively. Shading indicates 95% confidence intervals of each modeled response.
For the test phase, the mixed effects logistic regression model containing test time and stimuli as predictors was not statistically significant [X2(2) = 5.38, p = 0.07]. The results of multiple linear regression revealed that testing time and stimuli explained 3% of the variance, [R2 = 0.03, F(2, 672) = 12,8, p < 0.001]. When stimulus type was held constant, testing time significantly predicted reaction time, β = −4.98, t = −5.06, p < 0.001. However, there is no significant prediction of stimuli on reaction time (β = 12.66, t = 0.95, p = 0.34). These results collectively suggest that accuracy improved with more exposure to the stimuli in the training phase, with /bɔ/ and /bu/ being more rapidly attained than /bo/. Further, all stimuli were discriminated with a high level of accuracy during the test phase. Irrespective of the target stimulus, reaction time similarly decreased during training and testing phases.
Stimulus-to-Response Cross-Correlations
Initial stimulus-to-response calculations for pre- and post-training FFRSWS showed sharply peaked cross-correlation functions between /bu/ and /bo/ and their respective SWS stimulus waveforms; because the stimulus waveforms are dominated by the F1 component, these results indicated strong neural phase locking to F1. In contrast, cross-correlations for the /bɔ/ were poor despite these FFRSWS waveforms being highly periodic. Spectrographic analysis of the average FFRSWS to /bɔ/ demonstrated that the neural response was in fact phase-locked to the quadratic distortion product (F2–F1) instead of F1. The F2–F1 distortion product is mechanically initiated by interactions between F2 and F1 traveling waves on the basilar membrane, and the nervous system can phase lock to this and other distortions as it would to acoustically-delivered stimuli of the same frequency (e.g., Siegel et al., 1982; Smith et al., 2017). Because F2–F1 is not present in the acoustic stimulus, the neural response does not bear a resemblance to the stimulus. To determine whether F2–F1 frequency tracking for /bɔ/ SWSFFR were of neural origin, we approximated an F2–F1 “stimulus” waveform by taking the analytic envelope of the original SWS stimulus and band-passing it between 100 and 2,400 Hz. Cross-correlations were then rerun between the F2–F1 stimulus waveform and /bɔ/ SWSFFR. With this adjustment, /bɔ/ SWSFFR cross-correlation functions demonstrated sharp peaks similar to the other responses.
Results of the cross-correlation analyses for test and control groups are shown in Figure 3. Multivariate analysis showed a significant effect of stimulus type on both latency and cross-correlation strength across groups and training status, [Wilks’ Lamda = 0.33, F(4, 62) = 11.42, p < 0.001, η2 = 0.42], suggesting that stimulus type affected cross-correlation strength and latencies of neural responses. The effect size, calculated using eta squared, indicated that this stimulus type effect accounted for 42% of the variance in cross-correlation strength and latency. Moreover, there was a significant effect of training status between test and control groups across stimuli, [Wilks’ Lamda = 0.66, F(2, 15) = 3.82, p < 0.05, η2 = 0.34], suggesting that the interaction of training status and group affected the cross-correlation strength and latency of neural responses. The effect size, calculated using eta squared, indicated the interaction of training status and groups effect accounted for 34% of the variance in cross-correlation strength and latency. However, there is no significant stimuli*group [F(4, 62) = 0.12, p = 0.98, η2 = 0.01], training status [F(2, 15) = 1.94, p = 0.18, η2 = 0.21], stimuli*training [F(4, 62) = 0.59, p = 0.67, η2 = 0.03], stimuli*training*group [F(4, 62) = 0.12, p = 0.98, η2 = 0.01] effect on cross-correlation strength and latency [F(2, 7), p = 0.49, η2 = 0.19].
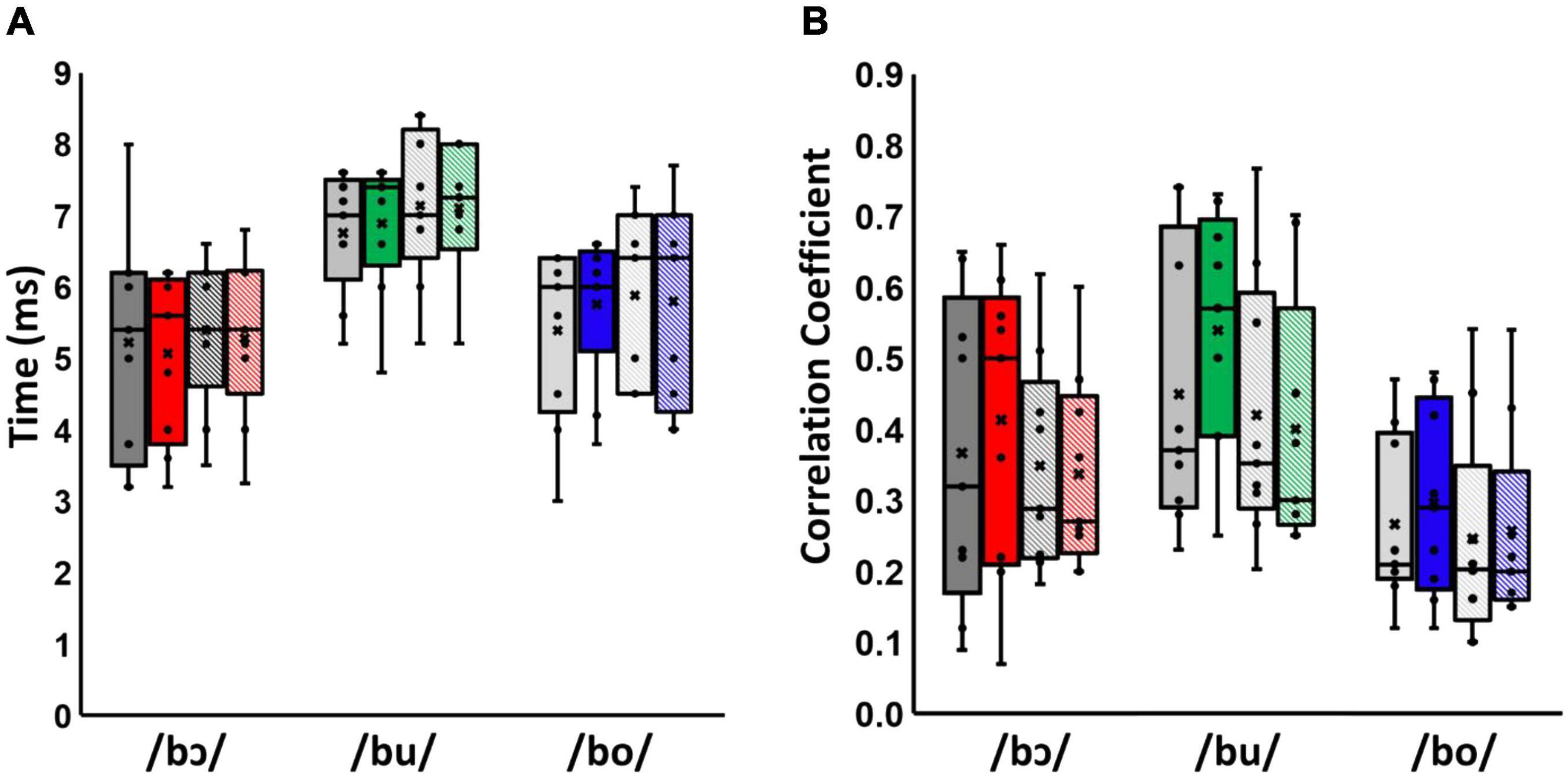
Figure 3. Latency (A) and cross-correlation strength (B) for /bɔ/, /bu/, and /bo/ FFRSWS. Pre-training responses are shown in gray and post-training responses are shown in color. Test group responses are solid-filled bars, whereas control group responses are cross-hatched. Means and medians are denoted by Xs and horizontal lines, respectively. Note that latencies and cross-correlations for /bɔ/ were calculated using the F2–F1 waveform as the “stimulus,” as described in the text.
Univariate tests were used to further examine the effects on latency and cross-correlation strength. These results show a significant stimulus effect on latency [Greenhouse-Geisser = 57.72, F(1.88, 30.08) = 15.93, p < 0.001, η2 = 0.50] and cross-correlation strength [Greenhouse-Geisser = 0.62, F(1.99, 31.87) = 11, p < 0.001, η2 = 0.41]. Moreover, there was a significant effect of the interaction between training status and groups on cross-correlation strength [Greenhouse-Geisser = 0.02, F(1, 1.68) = 4.62, p < 0.05, η2 = 0.22]. Within-subjects contrasts showed that latency in the /bu/ condition was significantly higher than /bɔ/ [F(1, 16) = 39.64, p < 0.001, η2 = 0.71] and /bo/ conditions [F(1, 16) = 14.59, p < 0.01, η2 = 0.48]. Additionally, cross-correlation strength in /bu/ was higher than /bo/ [F(1, 16) = 23.29, p < 0.001, η2 = 0.59]. Lastly, there was a significantly higher cross-correlation after training than before training in test group [F(1, 16) = 4.61, p < 0.05, η2 = 0.22]; this significant difference is larger than the pre- and post-training difference in control group.
Pre- and Post-training FFRSWS Fourier Analyzers
FFRSWS amplitudes at F1 and F2 were calculated over the duration of the response using FAs. These calculations produced a single amplitude estimate representing the strength of neural phase locking to the stimulus feature of interest over the entire duration of the stimulus. FFRSWS to /bu/ and /bo/ produced measurable F1 responses above the noise floor for all subjects in pre- and post-training measurements. The issue described above regarding neural phase locking to F2–F1 in /bɔ/ FFRSWS also impacted our initial FA calculations for the /bɔ/ stimulus such that F1 was not robustly represented. Consequently, we used the F2–F1 “stimulus” waveform to create an F2–F1 frequency track for /bɔ/ responses, using identical procedures described in the method section. Using this approach yielded measurable F2–F1 neural responses in every participant for /bɔ/ in pre- and post-training measurements. The following analyses focus on F1 amplitudes for /bu/ and /bo/ and F2–F1 amplitudes for /bɔ/. Because F2 was only measurable in <25% of responses, we did not further analyze these components.
Figure 4 depicts mean FFRSWS waveforms and spectrograms for pre- and post-training test group responses as well as test and control group FA results for each stimulus. All post-training FFRSWS responses are larger in amplitude than pre-training responses for the test group, which can be seen in waveform (Figure 4A) and spectrographic (Figure 4B) representations. Examination of the FA results for the test group (Figure 4C) demonstrates that these enhancements are at the frequency of interest only and are not observed in the adjacent noise bins. By comparison, FFRSWS enhancements are not apparent in the control group FA responses.
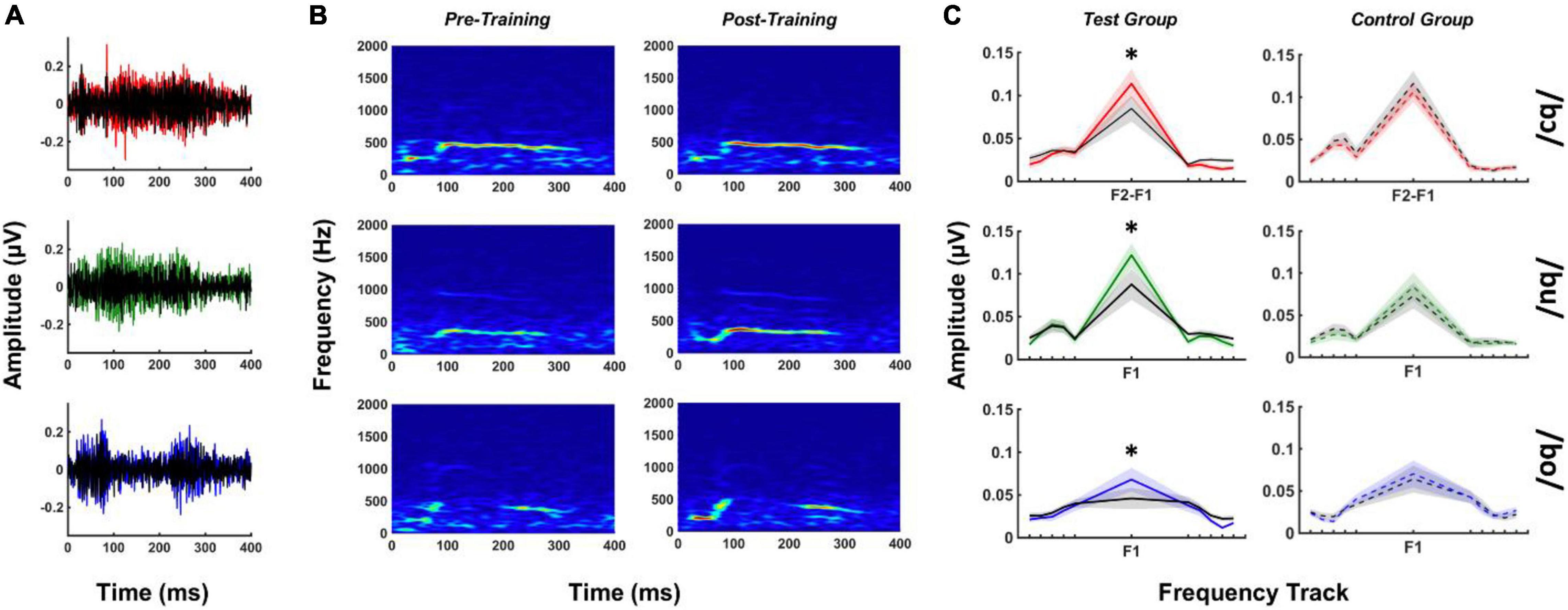
Figure 4. FFRSWS pre- and post-training waveforms (A), spectrograms (B), and FA results (C) for each stimulus. (A,B) Are from test group data only, whereas (C) includes test and control FA results for comparison. Colored (red, green, and blue) waveforms and FA spectra represent post-training responses, whereas black waveforms are their pre-training counterparts. FA center frequencies (F2–F1 or F1) are denoted for each FA plot; noise bins starting at ± 5 Hz relative to the frequency of interest are indicated by peripheral tick marks on the x-axis (shading = SEM). All results represented subtracted waveforms. *p < 0.05.
The effect of training status and group on FA amplitudes was analyzed using a two-way MANOVA with repeated measures. This analysis showed an interaction of training status and group [F(5, 12) = 3.09, p = 0.05, η2 = 0.56] on FA amplitude. Univariate tests revealed significant interactions between training status and group for /bɔ/ [Greenhouse-Geisser = 0.002, F(1, 16) = 6.72, p < 0.05, η2 = 0.30], /bu/ [Greenhouse-Geisser = 0.004, F(1, 16) = 8.44, p < 0.05, η2 = 0.34], and /bo/ [Greenhouse-Geisser = 0.002, F(1, 16) = 9.96, p < 0.01, η2 = 0.38] FA amplitudes. Pre- and post-training differences between test and control groups revealed that /bɔ/ [F(1, 16) = 6.73, p < 0.05, η2 = 0.30], /bu/ [F(1, 16) = 8.44, p < 0.05, η2 = 0.35], and /bo/ [F(1, 16) = 9.96, p < 0.01, η2 = 0.38] FA amplitudes were significantly higher after training in test group but not in the control group.
Machine Learning Classification of FFRSWS
Average linear SVM classification accuracies for test and control groups are depicted for pre-training and post-training FFRSWS in the confusion matrices of Figure 5. For the test group, pre-training classification accuracy was poorer for each stimulus classifier relative to post-training accuracy, whereas control group pre- and post-training classification results do not follow a clear pattern. Overall classification accuracy distributions representing all 2,500 iterations are depicted in the 3D histogram plots, as are empirical null distributions generated by randomly shuffling classifier outputs (i.e., response labels) for each iteration. Pre-training (p < 0.001) and post-training (p < 0.001) FFRSWS classification was significantly above the null distribution for test and control groups, as determined using p = (a + 1)/(n + 1). In the test group, post-training classification was significantly higher (p < 0.01) than pre-training classification using the same equation. In contrast, pre- and post-training classification were not different in the control group (p = 0.18). Collectively, these results indicate that classification of pre- and post-training FFRSWS was significantly above chance for test and control groups; however, post-training data were classified with significantly higher accuracy than pre-training data in the test group compared to the control group.
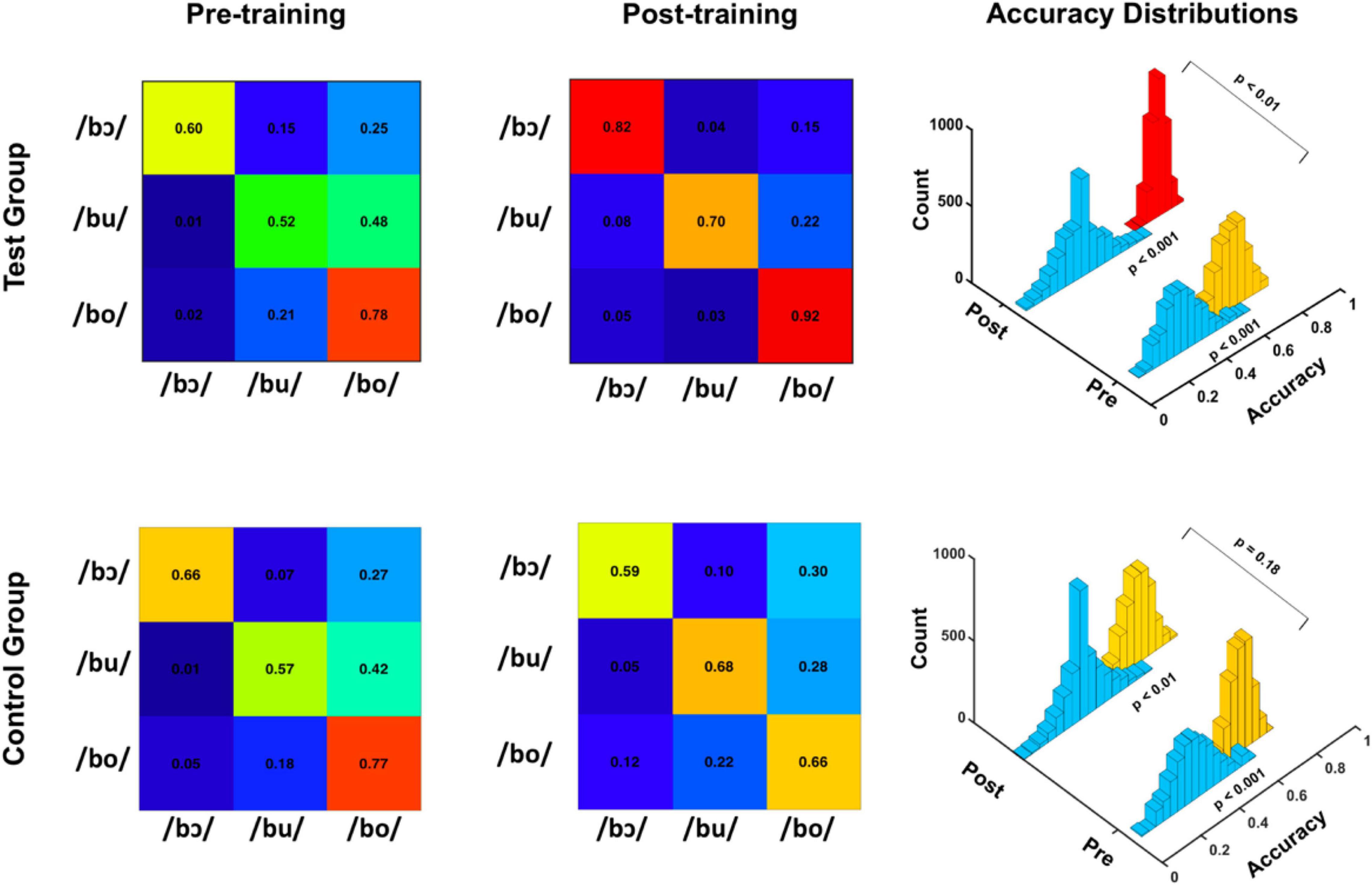
Figure 5. Pre-training and post-training classification accuracies for test (top) and control (bottom) groups. Confusion matrices on the left demonstrate linear SVM classification accuracies for pre-training FFRSWS, whereas the confusion matrices in the center demonstrate post-training accuracies. 3D histograms on the right show empirical null distributions (teal bins) and pre- and post-training average classification accuracy distributions for test and control groups. Pre- and post-training distributions were significantly above the null distribution for test and control groups. Additionally, the test group post-training distribution was significantly more accurate than the pre-training distribution.
Discussion
To our knowledge, this is the first study using FFRSWS to examine training or context effects in the auditory brainstem. Utilizing SWS in this context allowed for direct comparisons between pre- and post-training FFRSWS evoked by identical, acoustically-sparse speech stimuli that initiated neural responses from more caudal subcortical sources than stimuli used in previous reports. Because most listeners do not hear SWS as speech unless they are provided with additional instruction (Remez et al., 1981; Möttönen et al., 2006), pre-training neural representation of SWS theoretically offers a glimpse into bottom-up auditory processing of the “naïve” auditory nervous system. When additional instruction or context is provided to listeners regarding SWS, they often attain a high level of comprehension in a brief period of time or, in some cases, immediately (Remez et al., 1981; Möttönen et al., 2006). Thus, post-training FFRSWS may offer insight into how rapidly and potently the auditory brainstem can be functionally modulated via the efferent system when speech comprehension networks are engaged.
Our behavioral results suggest that SWS stimuli were rapidly attained in the training trials, albeit at slightly different rates. For example, /bɔ/ and /bu/ discrimination reached peak performance within relatively few trials, whereas /bo/ required more training before responses were consistently accurate. This pattern suggests that /bo/ was initially more difficult to discriminate than /bɔ/ and /bu/, which may simply be explained by acoustical differences (i.e., /bo/ and /bu/ F1 and F2 contours are more similar than /bɔ/ and were spaced such that they were unlikely to generate strong distortion products; see Figure 1). An additional revelation from our FFRSWS data was that participants may have benefitted from hearing the F2–F1 distortion product created by the /bɔ/ stimulus. The F2–F1 frequency is generated by mechanical interaction on the basilar membrane and “feeds forward” into the auditory nervous system, as do other distortion products (Siegel et al., 1982; Chertoff et al., 1992; Dhar et al., 2009; Smith et al., 2017), effectively converting a dynamic two-tone stimulus into a perceptually richer input (Goldstein et al., 1978). Because we did not assess psychophysical weighting of the F2–F1 cue, it is not clear whether its post-training enhancement in the FFR was a consequence of direct attention to this cue or a gross upscaling of any auditory stimuli relevant to the perceptual task.
Despite stimulus-related differences in behavioral training results, we observed that all FFRSWS in the test group were enhanced in the post-training phase relative to the pre-training phase and compared to a control group. This was indicated in larger FA amplitudes of F1 (for /bo/ and /bu/) and F2–F1 (for /bɔ/), as well as higher machine learning classification accuracy in the post-training phase. Importantly, these differences were due to FFRSWS amplitude enhancement and not differences in residual noise between pre- and post-training responses, as evidenced by the FA noise tracks (Figure 4). There are multiple potential explanations for the observed FFRSWS enhancements in the test group. First, the rapid perceptual shift from non-speech to speech may have engaged speech comprehension networks originating in frontal cortex and extending through auditory cortex and brain stem (Davis and Johnsrude, 2003; Eisner et al., 2010; Hervais-Adelman et al., 2012; Bidelman et al., 2018a,2019; Khoshkhoo et al., 2018). That FFRSWS enhancements reflect a more immediate online context shift and not short-term training per se is supported by a few congruent observations in our behavioral and neurophysiologic data. For example, perceptual shifts appear to have occurred quickly for /bɔ/ and /bu/ stimuli, whereas /bo/ required more exposure trials before it was attained. These results suggest that perceptual salience of the context shift may have differed slightly across stimuli. The size of FA enhancements and within-class changes in linear SVM classification accuracy between pre- and post-training trials mirror the behavioral results: more immediate behavioral SWS identification was associated with larger FA enhancements and greater changes in classification accuracy post-training. It is also notable that the enhancements observed in the present study appeared earlier than many reports on FFR training effects, which required multiple hours to days of training (e.g., Song et al., 2008; Carcagno and Plack, 2011). This may be related to the fact that SWS was initially processed as a completely different class of stimulus (e.g., uncorrelated “whistles”) before being recognized as speech, whereas participants in previous studies were aware from experiment onset that they were hearing speech or music stimuli. Further, SWS forces listeners to focus on a minimal number of cues (F1, F2, and/or F2–F1), whereas speech and music pitch may be determined in a variety of ways, such as listening to resolved and/or unresolved harmonics (e.g., Laudanski et al., 2014); therefore, attention may be allocated to different channels of information summating to produce the FFR. A limitation of our approach, which does not allow us to resolve single-trial FFRSWS, is that we cannot delineate whether the observed enhancements are related to online or short-term changes following the perceptual shift.
A control group was used in the present study to examine whether post-training vs. pre-training differences were simply a result of more exposure to the stimuli during the experimental protocol. Our results suggest that this is not the case, as the control group responses were not enhanced “post-training” relative to “pre-training.” These results comport with the multiple studies that have demonstrated high test-retest reliability of FFR amplitudes within and between passive test sessions (e.g., Song et al., 2011; Bidelman et al., 2018b; Easwar et al., 2020).
Future studies will examine afferent-efferent connectivity using similar SWS stimuli to examine the time course and neural substrates involved in perceptual shifts and/or training effects reported here. Because of the simple, sinusoidal nature of SWS, it may also be possible to measure simultaneous stimulus frequency otoacoustic emissions in addition to neural responses from the brainstem and cortex. Such an approach would allow for context or training effects to be studied from cochlea to cortex using the same stimuli.
Data Availability Statement
The raw data supporting the conclusions of this article will be made available by the authors, without undue reservation.
Ethics Statement
The studies involving human participants were reviewed and approved by the University of Texas at Austin IRB. The patients/participants provided their written informed consent to participate in this study.
Author Contributions
All authors listed have made a substantial, direct, and intellectual contribution to the work, and approved it for publication.
Funding
This research was funded by the National Institutes of Health, National Institute on Deafness and other Communication Disorders (K01DC017192).
Conflict of Interest
The authors declare that the research was conducted in the absence of any commercial or financial relationships that could be construed as a potential conflict of interest.
Publisher’s Note
All claims expressed in this article are solely those of the authors and do not necessarily represent those of their affiliated organizations, or those of the publisher, the editors and the reviewers. Any product that may be evaluated in this article, or claim that may be made by its manufacturer, is not guaranteed or endorsed by the publisher.
References
Aiken, S. J., and Picton, T. W. (2006). Envelope following responses to natural vowels. Audiol. Neurotol. 11, 213–232. doi: 10.1159/000092589
Aiken, S. J., and Picton, T. W. (2008). Envelope and spectral frequency-following responses to vowel sounds. Hear. Res. 245, 35–47. doi: 10.1016/j.heares.2008.08.004
Atiani, S., David, S. V., Elgueda, D., Locastro, M., Radtke-Schuller, S., Shamma, S. A., et al. (2014). Emergent selectivity for task-relevant stimuli in higher-order auditory cortex. Neuron 82, 486–499. doi: 10.1016/j.neuron.2014.02.029
Bajo, V. M., Nodal, F. R., Moore, D. R., and King, A. J. (2010). The descending corticocollicular pathway mediates learning-induced auditory plasticity. Nat. Neurosci. 13, 253–260. doi: 10.1038/nn.2466
Barker, J., and Cooke, M. (1999). Is the sine-wave speech cocktail party worth attending? Speech Commun. 27, 159–174. doi: 10.1016/s0167-6393(98)00081-8
Bidelman, G., and Powers, L. (2018). Response properties of the human frequency-following response (FFR) to speech and non-speech sounds: level dependence, adaptation and phase-locking limits. Int. J. Audiol. 57, 665–672. doi: 10.1080/14992027.2018.1470338
Bidelman, G. M. (2015). Multichannel recordings of the human brainstem frequency-following response: scalp topography, source generators, and distinctions from the transient ABR. Hear. Res. 323, 68–80. doi: 10.1016/j.heares.2015.01.011
Bidelman, G. M. (2018). Subcortical sources dominate the neuroelectric auditory frequency-following response to speech. Neuroimage 175, 56–69. doi: 10.1016/j.neuroimage.2018.03.060
Bidelman, G. M., and Krishnan, A. (2009). Neural correlates of consonance, dissonance, and the hierarchy of musical pitch in the human brainstem. J. Neurosci. 29, 13165–13171. doi: 10.1523/JNEUROSCI.3900-09.2009
Bidelman, G. M., Nelms, C., and Bhagat, S. P. (2016). Musical experience sharpens human cochlear tuning. Hear. Res. 335, 40–46. doi: 10.1016/j.heares.2016.02.012
Bidelman, G. M., Davis, M. K., and Pridgen, M. H. (2018a). Brainstem-cortical functional connectivity for speech is differentially challenged by noise and reverberation. Hear. Res. 367, 149–160. doi: 10.1016/j.heares.2018.05.018
Bidelman, G. M., Pousson, M., Dugas, C., and Fehrenbach, A. (2018b). Test–retest reliability of dual-recorded brainstem versus cortical auditory-evoked potentials to speech. J. Am. Acad. Audiol. 29, 164–174. doi: 10.3766/jaaa.16167
Bidelman, G. M., Price, C. N., Shen, D., Arnott, S. R., and Alain, C. (2019). Afferent-efferent connectivity between auditory brainstem and cortex accounts for poorer speech-in-noise comprehension in older adults. Hear. Res. 382:107795. doi: 10.1016/j.heares.2019.107795
Bidelman, G. M., Schneider, A. D., Heitzmann, V. R., and Bhagat, S. P. (2017). Musicianship enhances ipsilateral and contralateral efferent gain control to the cochlea. Hear. Res. 344, 275–283. doi: 10.1016/j.heares.2016.12.001
Bidelman, G. M., Schug, J. M., Jennings, S. G., and Bhagat, S. P. (2014). Psychophysical auditory filter estimates reveal sharper cochlear tuning in musicians. J. Acoust. Soc. Am. 136, EL33–EL39.
Boersma, P. (2009). Praat: Doing Phonetics by Computer (Version 5.1. 05). Available online at: http://www.praat.org/ (accessed August 20, 2018).
Carcagno, S., and Plack, C. J. (2011). Subcortical plasticity following perceptual learning in a pitch discrimination task. J. Assoc. Res. Otolaryngol. 12, 89–100. doi: 10.1007/s10162-010-0236-1
Chandrasekaran, B., and Kraus, N. (2010). The scalp-recorded brainstem response to speech: neural origins and plasticity. Psychophysiology 47, 236–246. doi: 10.1111/j.1469-8986.2009.00928.x
Chandrasekaran, B., Kraus, N., and Wong, P. C. (2012). Human inferior colliculus activity relates to individual differences in spoken language learning. J. Neurophysiol. 107, 1325–1336. doi: 10.1152/jn.00923.2011
Chertoff, M. E., Hecox, K. E., and Goldstein, R. (1992). Auditory distortion products measured with averaged auditory evoked potentials. J. Speech Lang. Hear. Res. 35, 157–166. doi: 10.1044/jshr.3501.157
Choi, J. M., Purcell, D. W., Coyne, J. A. M., and Aiken, S. J. (2013). Envelope following responses elicited by English sentences. Ear Hear. 34, 637–650. doi: 10.1097/AUD.0b013e31828e4dad
Coffey, E. B., Herholz, S. C., Chepesiuk, A. M., Baillet, S., and Zatorre, R. J. (2016). Cortical contributions to the auditory frequency-following response revealed by MEG. Nat. Commun. 7:11070. doi: 10.1038/ncomms11070
Coffey, E. B., Nicol, T., White-Schwoch, T., Chandrasekaran, B., Krizman, J., Skoe, E., et al. (2019). Evolving perspectives on the sources of the frequency-following response. Nat. Commun. 10:5036. doi: 10.1038/s41467-019-13003-w
Cohen, J., Cohen, P., West, S. G., and Aiken, L. S. (2013). Applied Multiple Regression/Correlation Analysis for the Behavioral Sciences. London: Routledge.
Connolly, J. F., Aubry, K., McGillivary, N., and Scott, D. W. (1989). Human brainstem auditory evoked potentials fail to provide evidence of efferent modulation of auditory input during attentional tasks. Psychophysiology 26, 292–303. doi: 10.1111/j.1469-8986.1989.tb01920.x
Cristianini, N., and Shawe-Taylor, J. (2000). An Introduction to Support Vector Machines and Other Kernel-Based Learning Methods. Cambridge: Cambridge university press.
Darwin, C. (2003). Sine-Wave Speech Produced Automatically Using a Script for the PRAAT Program. Available online at: http://www.lifesci.sussex.ac.uk/home/Chris_Darwin/SWS/ (accessed December 8, 2011).
Davis, M. H., and Johnsrude, I. S. (2003). Hierarchical processing in spoken language comprehension. J. Neurosci. 23, 3423–3431. doi: 10.1523/JNEUROSCI.23-08-03423.2003
de Boer, J., and Thornton, A. R. D. (2008). Neural correlates of perceptual learning in the auditory brainstem: efferent activity predicts and reflects improvement at a speech-in-noise discrimination task. J. Neurosci. 28, 4929–4937. doi: 10.1523/JNEUROSCI.0902-08.2008
Delano, P. H., and Elgoyhen, A. B. (2016). Editorial: auditory efferent system: new insights from cortex to cochlea. Front. Syst. Neurosci. 10:50. doi: 10.3389/fnsys.2016.00050
Dhar, S., Abel, R., Hornickel, J., Nicol, T., Skoe, E., Zhao, W., et al. (2009). Exploring the relationship between physiological measures of cochlear and brainstem function. Clin. Neurophysiol. 120, 959–966. doi: 10.1016/j.clinph.2009.02.172
Dragicevic, C. D., Aedo, C., León, A., Bowen, M., Jara, N., Terreros, G., et al. (2015). The olivocochlear reflex strength and cochlear sensitivity are independently modulated by auditory cortex microstimulation. J. Assoc. Res. Otolaryngol. 16, 223–240. doi: 10.1007/s10162-015-0509-9
Easwar, V., Scollie, S., Aiken, S., and Purcell, D. (2020). Test-Retest variability in the characteristics of envelope following responses evoked by speech stimuli. Ear Hear. 41, 150–164. doi: 10.1097/AUD.0000000000000739
Eggermont, J. J. (2017). Ups and downs in 75 years of electrocochleography. Front. Syst. Neurosci. 11:2. doi: 10.3389/fnsys.2017.00002
Eisner, F., McGettigan, C., Faulkner, A., Rosen, S., and Scott, S. K. (2010). Inferior frontal gyrus activation predicts individual differences in perceptual learning of cochlear-implant simulations. J. Neurosci. 30, 7179–7186. doi: 10.1523/JNEUROSCI.4040-09.2010
Etard, O., Kegler, M., Braiman, C., Forte, A. E., and Reichenbach, T. (2019). Decoding of selective attention to continuous speech from the human auditory brainstem response. Neuroimage 200, 1–11. doi: 10.1016/j.neuroimage.2019.06.029
Forte, A. E., Etard, O., and Reichenbach, T. (2017). The human auditory brainstem response to running speech reveals a subcortical mechanism for selective attention. elife 6:e27203. doi: 10.7554/eLife.27203
Francis, N. A., Zhao, W., and Guinan, J. J. Jr. (2018). Auditory attention reduced ear-canal noise in humans by reducing subject motion, not by medial olivocochlear efferent inhibition: implications for measuring otoacoustic emissions during a behavioral task. Front. Syst. Neurosci. 12:42. doi: 10.3389/fnsys.2018.00042I
Galbraith, G. C., Arbagey, P. W., Branski, R., Comerci, N., and Rector, P. M. (1995). Intelligible speech encoded in the human brain stem frequency-following response. Neuroreport 6, 2363–2367. doi: 10.1097/00001756-199511270-00021
Galbraith, G. C., and Arroyo, C. (1993). Selective attention and brainstem frequency-following responses. Biol. Psychol. 37, 3–22. doi: 10.1016/0301-0511(93)90024-3
Galbraith, G. C., Bagasan, B., and Sulahian, J. (2001). Brainstem frequency-following response recorded from one vertical and three horizontal electrode derivations. Percept. Motor Skills 92, 99–106. doi: 10.2466/pms.2001.92.1.99
Galbraith, G. C., Bhuta, S. M., Choate, A. K., Kitahara, J. M., and Mullen, T. A. Jr. (1998). Brain stem frequency-following response to dichotic vowels during attention. Neuroreport 9, 1889–1893. doi: 10.1097/00001756-199806010-00041
Galbraith, G. C., Olfman, D. M., and Huffman, T. M. (2003). Selective attention affects human brain stem frequency-following response. Neuroreport 14, 735–738. doi: 10.1097/00001756-200304150-00015
Gao, E., and Suga, N. (1998). Plasticity of midbrain auditory frequency map mediated by the corticofugal system in bat. Proc. Natl. Acad. Sci. U.S.A. 95, 12663–12670. doi: 10.1038/255
Gao, E., and Suga, N. (2000). Experience-dependent plasticity in the auditory cortex and the inferior colliculus of bats: role of the corticofugal system. Proc. Natl. Acad. Sci. U.S.A. 97, 8081–8086. doi: 10.1073/pnas.97.14.8081
Gardi, J., Merzenich, M., and McKean, C. (1979). Origins of the scalp-recorded frequency-following response in the cat. Audiology 18, 353–380. doi: 10.3109/00206097909070062
Goldstein, J. L., Buchsbaum, G., and Furst, M. (1978). Compatibility between psychophysical and physiological measurements of aural combination tones. J. Acoust. Soc. Am. 63, 474–485. doi: 10.1121/1.381739
Gregory, S. D., Heath, J. A., and Rosenberg, M. E. (1989). Does selective attention influence the brain-stem auditory evoked potential? Electroencephalogr. Clin. Neurophysiol. 73, 557–560. doi: 10.1016/0013-4694(89)90266-6
Hackley, S. A., Woldorff, M., and Hillyard, S. A. (1990). Cross-modal selective attention effects on retinal, myogenic, brainstem, and cerebral evoked potentials. Psychophysiology 27, 195–208. doi: 10.1111/j.1469-8986.1990.tb00370.x
Hairston, W. D., Letowski, T. R., and McDowell, K. (2013). Task-related suppression of the brainstem frequency following response. PLoS One 8:e55215. doi: 10.1371/journal.pone.0055215
Hernandez-Peon, R., Scherrer, H., and Jouvet, M. (1956). Modification of electric activity in cochlear nucleus during attention in unanesthetized cats. Science 123, 331–332. doi: 10.1126/science.123.3191.331
Hernandez-Perez, H., Mikiel-Hunter, J., McAlpine, D., Dhar, S., Boothalingam, S., Monaghan, J. J., et al. (2021). Perceptual gating of a brainstem reflex facilitates speech understanding in human listeners. bioRxiv [Preprint]. doi: 10.1101/2020.05.31.115444
Hervais-Adelman, A. G., Carlyon, R. P., Johnsrude, I. S., and Davis, M. H. (2012). Brain regions recruited for the effortful comprehension of noise-vocoded words. Lang. Cogn. Proces. 27, 1145–1166. doi: 10.1080/01690965.2012.662280
Holdgraf, C. R., Rieger, J. W., Micheli, C., Martin, S., Knight, R. T., and Theunissen, F. E. (2017). Encoding and decoding models in cognitive electrophysiology. Front. Syst. Neurosci. 11:61. doi: 10.3389/fnsys.2017.00061
Jedrzejczak, W. W., Milner, R., Ganc, M., Pilka, E., and Skarzynski, H. (2020). No change in medial olivocochlear efferent activity during an auditory or visual task: dual evidence from otoacoustic emissions and event-related potentials. Brain Sci. 10:894. doi: 10.3390/brainsci10110894
Ji, W., Gao, E., and Suga, N. (2001). Effects of acetylcholine and atropine on plasticity of central auditory neurons caused by conditioning in bats. J. Neurophysiol. 86, 211–225. doi: 10.1152/jn.2001.86.1.211
Khoshkhoo, S., Leonard, M. K., Mesgarani, N., and Chang, E. F. (2018). Neural correlates of sine-wave speech intelligibility in human frontal and temporal cortex. Brain Lang. 187, 83–91. doi: 10.1016/j.bandl.2018.01.007
Kraus, N., and Chandrasekaran, B. (2010). Music training for the development of auditory skills. Nat. Rev. Neurosci. 11, 599–605. doi: 10.1038/nrn2882
Kraus, N., and White-Schwoch, T. (2015). Unraveling the biology of auditory learning: a cognitive–sensorimotor–reward framework. Trends Cogn. Sci. 19, 642–654. doi: 10.1016/j.tics.2015.08.017
Krishnan, A., and Gandour, J. T. (2009). The role of the auditory brainstem in processing linguistically-relevant pitch patterns. Brain Lang. 110, 135–148. doi: 10.1016/j.bandl.2009.03.005
Krishnan, A., Xu, Y., Gandour, J., and Cariani, P. (2005). Encoding of pitch in the human brainstem is sensitive to language experience. Cogn. Brain Res. 25, 161–168. doi: 10.1016/j.cogbrainres.2005.05.004
Laudanski, J., Zheng, Y., and Brette, R. (2014). A structural theory of pitch. eNeuro 1:ENEURO.33-ENEURO.14. doi: 10.1523/ENEURO.0033-14.2014
Lauer, A. M., Jimenez, S. V., and Delano, P. H. (2021). Olivocochlear efferent effects on perception and behavior. Hear. Res. 108207. doi: 10.1016/j.heares.2021.108207
Lehmann, A., and Schönwiesner, M. (2014). Selective attention modulates human auditory brainstem responses: relative contributions of frequency and spatial cues. PLoS One 9:e85442. doi: 10.1371/journal.pone.0085442
León, A., Elgueda, D., Silva, M. A., Hamamé, C. M., and Delano, P. H. (2012). Auditory cortex basal activity modulates cochlear responses in chinchillas. PLoS One 7:e36203. doi: 10.1371/journal.pone.0036203
Lichtenhan, J. T., Cooper, N. P., and Guinan, J. J. (2013). A new auditory threshold estimation technique for low frequencies: proof of concept. Ear Hear. 34:42. doi: 10.1097/AUD.0b013e31825f9bd3
Lindblom, B. (1990). “Explaining phonetic variation: a sketch of the H&H theory,” in Speech Production and Speech Modelling, eds W. J. Hardcastle and A. Marchal (Dordrecht: Springer), 403–439. doi: 10.1121/1.405815
Llanos, F., Xie, Z., and Chandrasekaran, B. (2017). Hidden Markov modeling of frequency-following responses to Mandarin lexical tones. J. Neurosci. Methods 291, 101–112. doi: 10.1016/j.jneumeth.2017.08.010
Ma, X., and Suga, N. (2001). Plasticity of bat’s central auditory system evoked by focal electric stimulation of auditory and/or somatosensory cortices. J. Neurophysiol. 85, 1078–1087. doi: 10.1152/jn.2001.85.3.1078
Malmierca, M. S., Cristaudo, S., Pérez-González, D., and Covey, E. (2009). Stimulus-specific adaptation in the inferior colliculus of the anesthetized rat. J. Neurosci. 29, 5483–5493. doi: 10.1523/JNEUROSCI.4153-08.2009
May, B. J., Budelis, J., and Niparko, J. K. (2004). Behavioral studies of the olivocochlear efferent system: learning to listen in noise. Arch. Otolaryngol. Head Neck Surg. 130, 660–664. doi: 10.1001/archotol.130.5.660
Möttönen, R., Calvert, G. A., Jääskeläinen, I. P., Matthews, P. M., Thesen, T., Tuomainen, J., et al. (2006). Perceiving identical sounds as speech or non-speech modulates activity in the left posterior superior temporal sulcus. Neuroimage 30, 563–569. doi: 10.1016/j.neuroimage.2005.10.002
Perrot, X., Ryvlin, P., Isnard, J., GuÈnot, M., Catenoix, H., Fischer, C., et al. (2006). Evidence for corticofugal modulation of peripheral auditory activity in humans. Cereb. Cortex 16, 941–948. doi: 10.1093/cercor/bhj035
Phipson, B., and Smyth, G. K. (2010). Permutation P-values should never be zero: calculating exact P-values when permutations are randomly drawn. Stat. Appl. Genet. Mol. Biol. 9:39. doi: 10.2202/1544-6115.1585
Picton, T. W., and Hillyard, S. A. (1974). Human auditory evoked potentials II: effects of attention. Electroencephalogr. Clin. Neurophysiol. 36, 191–199. doi: 10.1016/0013-4694(74)90156-4
Picton, T. W., John, M. S., Dimitrijevic, A., and Purcell, D. (2003). Human auditory steady-state responses: respuestas auditivas de estado estable en humanos. Int. J. Audiol. 42, 177–219. doi: 10.3109/14992020309101316
Price, C. N., and Bidelman, G. M. (2021). Attention reinforces human corticofugal system to aid speech perception in noise. Neuroimage 235:118014. doi: 10.1016/j.neuroimage.2021.118014
Purcell, D. W., John, S. M., Schneider, B. A., and Picton, T. W. (2004). Human temporal auditory acuity as assessed by envelope following responses. J. Acoust. Soc. Am. 116, 3581–3593. doi: 10.1121/1.1798354
Remez, R., Rubin, P., Pisoni, D., and Carrell, T. (1981). Speech perception without traditional speech cues. Science 212, 947–949. doi: 10.1126/science.7233191
Ritter, W., Simson, R., and Vaughan, H. G. Jr. (1972). Association cortex potentials and reaction time in auditory discrimination. Electroencephalogr. Clin. Neurophysiol. 33, 547–555. doi: 10.1016/0013-4694(72)90245-3
Ruggles, D., Bharadwaj, H., and Shinn-Cunningham, B. G. (2012). Why middle-aged listeners have trouble hearing in everyday settings. Curr. Biol. 22, 1417–1422. doi: 10.1016/j.cub.2012.05.025
Russo, N. M., Nicol, T. G., Zecker, S. G., Hayes, E. A., and Kraus, N. (2005). Auditory training improves neural timing in the human brainstem. Behav. Brain Res. 156, 95–103. doi: 10.1016/j.bbr.2004.05.012
Sadeghian, A., Dajani, H. R., and Chan, A. D. (2015). Classification of speech-evoked brainstem responses to English vowels. Speech Commun. 68, 69–84. doi: 10.1016/j.specom.2015.01.003
Saiz-Alía, M., Forte, A. E., and Reichenbach, T. (2019). Individual differences in the attentional modulation of the human auditory brainstem response to speech inform on speech-in-noise deficits. Sci. Rep. 9:14131. doi: 10.1038/s41598-019-50773-1
Shaheen, L. A., Slee, S. J., and David, S. V. (2021). Task engagement improves neural discriminability in the auditory midbrain of the marmoset monkey. J. Neurosci. 41, 284–297. doi: 10.1523/JNEUROSCI.1112-20.2020
Siegel, J. H., Kim, D. O., and Molnar, C. E. (1982). Effects of altering organ of Corti on cochlear distortion products f2-f1 and 2f1-f2. J. Neurophysiol. 47, 303–328. doi: 10.1152/jn.1982.47.2.303
Skoe, E., Chandrasekaran, B., Spitzer, E. R., Wong, P. C., and Kraus, N. (2014). Human brainstem plasticity: the interaction of stimulus probability and auditory learning. Neurobiol. Learn. Mem. 109, 82–93. doi: 10.1016/j.nlm.2013.11.011
Skoe, E., and Kraus, N. (2010). Hearing it again and again: on-line subcortical plasticity in humans. PLoS One 5:e13645. doi: 10.1371/journal.pone.0013645
Skoe, E., Krizman, J., Spitzer, E., and Kraus, N. (2013). The auditory brainstem is a barometer of rapid auditory learning. Neuroscience 243, 104–114.
Slee, S. J., and David, S. V. (2015). Rapid task-related plasticity of spectrotemporal receptive fields in the auditory midbrain. J. Neurosci. 35, 13090–13102. doi: 10.1523/JNEUROSCI.1671-15.201
Smith, S. B., and Cone, B. (2015). The medial olivocochlear reflex in children during active listening. Int. J. Audiol. 54, 518–523. doi: 10.3109/14992027.2015.1008105
Smith, S. B., Ichiba, K., Velenovsky, D. S., and Cone, B. (2017). Efferent modulation of pre-neural and neural distortion products. Hear. Res. 356, 25–34. doi: 10.1016/j.heares.2017.10.009
Song, J. H., Nicol, T., and Kraus, N. (2011). Test–retest reliability of the speech-evoked auditory brainstem response. Clin. Neurophysiol. 122, 346–355.
Song, J. H., Skoe, E., Banai, K., and Kraus, N. (2012). Training to improve hearing speech in noise: biological mechanisms. Cereb. Cortex 22, 1180–1190. doi: 10.1093/cercor/bhr196
Song, J. H., Skoe, E., Wong, P. C., and Kraus, N. (2008). Plasticity in the adult human auditory brainstem following short-term linguistic training. J. Cogn. Neurosci. 20, 1892–1902. doi: 10.1162/jocn.2008.20131
Strait, D. L., and Kraus, N. (2014). Biological impact of auditory expertise across the life span: musicians as a model of auditory learning. Hear. Res. 308, 109–121. doi: 10.1016/j.heares.2013.08.004
Stuart, A., and Butler, A. K. (2012). Contralateral suppression of transient otoacoustic emissions and sentence recognition in noise in young adults. J. Am. Acad. Audiol. 23, 686–696. doi: 10.3766/jaaa.23.9.3
Suga, N., Gao, E., Zhang, Y., Ma, X., and Olsen, J. F. (2000). The corticofugal system for hearing: recent progress. Proc. Natl. Acad. Sci. U.S.A. 97, 11807–11814. doi: 10.1073/pnas.97.22.11807
Suga, N., Xiao, Z., Ma, X., and Ji, W. (2002). Plasticity and corticofugal modulation for hearing in adult animals. Neuron 36, 9–18. doi: 10.1016/s0896-6273(02)00933-9
Swaminathan, J., Krishnan, A., and Gandour, J. T. (2008). Pitch encoding in speech and nonspeech contexts in the human auditory brainstem. Neuroreport 19:1163. doi: 10.1097/WNR.0b013e3283088d31
Terreros, G., and Delano, P. H. (2015). Corticofugal modulation of peripheral auditory responses. Front. Syst. Neurosci. 9:134. doi: 10.3389/fnsys.2015.00134
Tichko, P., and Skoe, E. (2017). Frequency-dependent fine structure in the frequency-following response: the byproduct of multiple generators. Hear. Res. 348, 1–15. doi: 10.1016/j.heares.2017.01.014
Varghese, L., Bharadwaj, H. M., and Shinn-Cunningham, B. G. (2015). Evidence against attentional state modulating scalp-recorded auditory brainstem steady-state responses. Brain Res. 1626, 146–164. doi: 10.1016/j.brainres.2015.06.038
Wittekindt, A., Kaiser, J., and Abel, C. (2014). Attentional modulation of the inner ear: a combined otoacoustic emission and EEG study. J. Neurosci. 34, 9995–10002. doi: 10.1523/JNEUROSCI.4861-13.2014
Woldorff, M., Hansen, J. C., and Hillyard, S. A. (1987). Evidence for effects of selective attention in the mid-latency range of the human auditory event-related potential. Electroencephalogr. Clin. Neurophysiol. Suppl. 40, 146–154.
Wong, P. C., Skoe, E., Russo, N. M., Dees, T., and Kraus, N. (2007). Musical experience shapes human brainstem encoding of linguistic pitch patterns. Nat. Neurosci. 10, 420–422. doi: 10.1038/nn1872
Xiao, Z., and Suga, N. (2002). Modulation of cochlear hair cells by the auditory cortex in the mustached bat. Nat. Neurosci. 5, 57–63. doi: 10.1038/nn786
Xie, Z., Reetzke, R., and Chandrasekaran, B. (2018). Taking attention away from the auditory modality: context-dependent effects on early sensory encoding of speech. Neuroscience 384, 64–75. doi: 10.1016/j.neuroscience.2018.05.023
Xie, Z., Reetzke, R., and Chandrasekaran, B. (2019). Machine learning approaches to analyze speech-evoked neurophysiological responses. J. Speech Lang. Hear. Res. 62, 587–601. doi: 10.1044/2018_JSLHR-S-ASTM-18-0244
Yan, J., and Suga, N. (1999). Corticofugal amplification of facilitative auditory responses of subcortical combination-sensitive neurons in the mustached bat. J. Neurophysiol. 81, 817–824. doi: 10.1152/jn.1999.81.2.817
Yan, J., Zhang, Y., and Ehret, G. (2005). Corticofugal shaping of frequency tuning curves in the central nucleus of the inferior colliculus of mice. J. Neurophysiol. 93, 71–83. doi: 10.1152/jn.00348.2004
Yan, W., and Suga, N. (1998). Corticofugal modulation of the midbrain frequency map in the bat auditory system. Nat. Neurosci. 1, 54–58.
Keywords: frequency following response (FFR), efferent, top-down, sine-wave speech perception, auditory learning
Citation: Cheng F-Y, Xu C, Gold L and Smith S (2021) Rapid Enhancement of Subcortical Neural Responses to Sine-Wave Speech. Front. Neurosci. 15:747303. doi: 10.3389/fnins.2021.747303
Received: 26 July 2021; Accepted: 02 December 2021;
Published: 20 December 2021.
Edited by:
Erika Skoe, University of Connecticut, United StatesReviewed by:
Zilong Xie, University of Kansas Medical Center, United StatesHilmi Dajani, University of Ottawa, Canada
Copyright © 2021 Cheng, Xu, Gold and Smith. This is an open-access article distributed under the terms of the Creative Commons Attribution License (CC BY). The use, distribution or reproduction in other forums is permitted, provided the original author(s) and the copyright owner(s) are credited and that the original publication in this journal is cited, in accordance with accepted academic practice. No use, distribution or reproduction is permitted which does not comply with these terms.
*Correspondence: Spencer Smith, c3BlbmNlci5zbWl0aEBhdXN0aW4udXRleGFzLmVkdQ==
†These authors have contributed equally to this work