- 1Rehabilitation Innovation Centre, Health and Rehabilitation Research Institute, Auckland University of Technology, Auckland, New Zealand
- 2Department of Health Science and Technology, Aalborg University, Aalborg, Denmark
- 3Centre for Chiropractic Research, New Zealand College of Chiropractic, Auckland, New Zealand
The movement-related cortical potential (MRCP) is a brain signal that can be recorded using surface electroencephalography (EEG) and represents the cortical processes involved in movement preparation. The MRCP has been widely researched in simple, single-joint movements, however, these movements often lack ecological validity. Ecological validity refers to the generalizability of the findings to real-world situations, such as neurological rehabilitation. This scoping review aimed to synthesize the research evidence investigating the MRCP in ecologically valid movement tasks. A search of six electronic databases identified 102 studies that investigated the MRCP during multi-joint movements; 59 of these studies investigated ecologically valid movement tasks and were included in the review. The included studies investigated 15 different movement tasks that were applicable to everyday situations, but these were largely carried out in healthy populations. The synthesized findings suggest that the recording and analysis of MRCP signals is possible in ecologically valid movements, however the characteristics of the signal appear to vary across different movement tasks (i.e., those with greater complexity, increased cognitive load, or a secondary motor task) and different populations (i.e., expert performers, people with Parkinson’s Disease, and older adults). The scarcity of research in clinical populations highlights the need for further research in people with neurological and age-related conditions to progress our understanding of the MRCPs characteristics and to determine its potential as a measure of neurological recovery and intervention efficacy. MRCP-based neuromodulatory interventions applied during ecologically valid movements were only represented in one study in this review as these have been largely delivered during simple joint movements. No studies were identified that used ecologically valid movements to control BCI-driven external devices; this may reflect the technical challenges associated with accurately classifying functional movements from MRCPs. Future research investigating MRCP-based interventions should use movement tasks that are functionally relevant to everyday situations. This will facilitate the application of this knowledge into the rehabilitation setting.
Introduction
The movement-related cortical potential (MRCP) is an event-related potential that can be recorded over various centroparietal brain regions prior to, and at the onset of, voluntary movement (Shibasaki and Hallett, 2006). It reflects motor planning and is detectable in self-paced, cued, and imagined movement (Deeke, 1996). In self-paced movement the MRCP is commonly referred to as the bereitschaftspotential or readiness potential, while in cued movement it is termed the contingent negative variation (Shibasaki and Hallett, 2006; Shakeel et al., 2015). For the purposes of this review, the umbrella term MRCP will be utilized.
The MRCP is commonly recorded using surface electroencephalography (EEG), where electrodes placed on the scalp measure voltage changes correlating with underlying activity in the superficial layers of the cortex (Holmes and Khazipov, 2007; Kirschstein and Kohling, 2009). A typical MRCP begins with a slow negative shift around 1.5–2 s prior to movement onset, with peak negativity observed around the time of movement onset, followed by a positive shift after movement execution (Do Nascimento et al., 2006; Shibasaki and Hallett, 2006; Shakeel et al., 2015) (refer to Figure 1). The primary generators of the MRCP are thought to be the bilateral supplementary motor areas, bilateral pre-supplementary motor areas, bilateral cingulate motor areas, and the contralateral M1, with some evidence also suggesting involvement of the ipsilateral M1 (Mackinnon, 2003). The MRCP is easily detected over the central electrodes near the midline. For finger movements the MRCP amplitude is largest at the C1 or C2 electrodes (International 10–20 system) (Shibasaki et al., 1980), whereas for ankle movements the amplitude peaks at the Cz or CPz electrode (Do Nascimento et al., 2006). The timing and amplitude of the MRCP varies with the type of movement, preparatory state (cued or self-paced), speed of the task, force required, the level of uncertainty about the type of movement, and the presence of neurological conditions (Brunia, 2003; Ikeda and Shibasaki, 2003; Rektor, 2003; Do Nascimento et al., 2006).
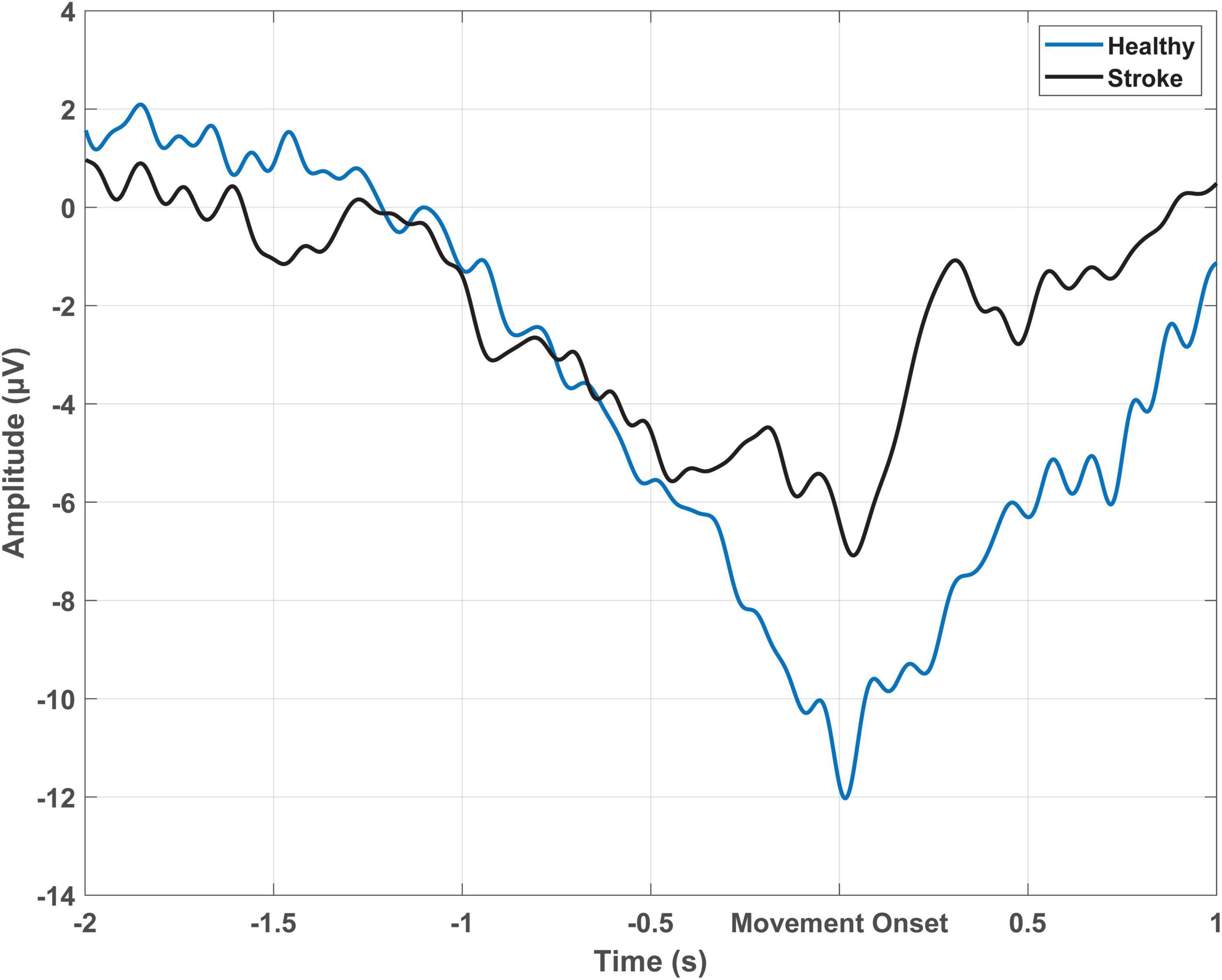
Figure 1. Epoch-averaged MRCP from Cz which has been filtered with a large Laplacian filter for one participant with stroke (51 trials) and one healthy participant (54 trials) performing cued voluntary ballistic ankle dorsiflexion.
MRCP signals have been investigated across different fields such as biomedical engineering, neurophysiology, and clinical research. Observational research, where MRCP data is recorded in a sample of participants under various task-related or environmental conditions, has informed our understanding of the phases and characteristics of the MRCP, dating back to its discovery in 1964 (Kornhuber and Deecke, 1964; Kornhuber and Deecke, 1965). While these observational studies have largely focused on the MRCP during simple, single-joint movements (Jahanshahi and Hallett, 2003), there is a developing body of research examining the MRCP signal during more complex tasks (Bibian et al., 2017; Eilbeigi and Setarehdan, 2018b). MRCP research has also focused on the development of online and offline brain–computer interfaces (BCIs) (Mrachacz-Kersting et al., 2012; Niazi et al., 2012; Xu et al., 2014; Jochumsen et al., 2015a). Accurate recording and processing of MRCPs by BCIs may allow users to control assistive devices, such as BCI-triggered lower limb exoskeletons (Lopez-Larraz et al., 2016) or upper limb neuroprostheses (Müller-Putz et al., 2019; Ofner et al., 2019). In addition, BCIs can deliver MRCP-based neuromodulatory interventions. These interventions have been investigated in experimental studies, where the researcher manipulates the delivery of the intervention and measures the effects on outcomes of interest. Studies have shown that MRCP-based neuromodulatory interventions induce neuroplasticity and improve lower limb impairment following stroke (Mrachacz-Kersting et al., 2016, 2019b; Olsen et al., 2020). A further area of MRCP research concerns the effects of motor learning on changes in MRCP onset and amplitude (Hatta et al., 2009; Wright et al., 2012a; Berchicci et al., 2017); in this case, the MRCP is used as the outcome measure within an experimental study. For example, a decrease in MRCP amplitude has been observed following repeated motor task training (Jochumsen et al., 2017) which may reflect lower cortical effort required to complete the task (Wright et al., 2011).
Research exploring the MRCP has particular relevance to the field of neurological rehabilitation through the enhanced understanding of: motor impairment, recovery processes following neurological injury, and the effect of rehabilitation interventions. However, a key limitation of its application to rehabilitation is the aforementioned focus on understanding the MRCP during simple, single-joint movements, such as isolated finger flexion or ankle movements (Wright et al., 2011; Ahmadian et al., 2013). These tasks bear little resemblance to movements people perform in real life or in rehabilitation, and therefore lack ecological validity. Ecological validity refers to the extent to which a movement being analyzed for research purposes resembles actual human behavior carried out in real-world environments (Davids, 1988). There are many examples of limited ecological validity in the field of MRCP research. MRCPs recorded during simple single-joint movements have been used to determine differences between experts and novices, yet the real-world task under investigation was much more complex (e.g., rifle shooting, martial arts) (Kita et al., 2001; Di Russo et al., 2005; Hatta et al., 2009). The effect of task training on healthy participants has been assessed using the MRCP recorded during simple grasping movements, rather than the fine motor task that was trained (Jochumsen et al., 2017), and the effect of rehabilitation interventions following stroke has been assessed using a simple finger flexion task rather than the goal-directed, complex upper limb tasks being rehabilitated (Kopp et al., 1999; Tarkka et al., 2008). In addition, an MRCP-based neuromodulatory intervention has been applied during a simple ankle dorsiflexion task in people with stroke (Mrachacz-Kersting et al., 2016, 2019b; Olsen et al., 2020), yet this single-joint movement lacks specificity to the real-world tasks required for lower limb function (for example, sit to stand, walking, or climbing stairs). This lack of ecological validity in MRCP research may be attributed to challenges that arise while recording EEG during more complex movements as the low-frequency MRCP signal can be easily masked by artifacts due to background noise, eye blinks, or other body movements (Wright et al., 2011; Ahmadian et al., 2013). However, with advances in technology and knowledge, recording the MRCP under more real-world conditions has become more feasible (Jochumsen et al., 2020a; Schwarz et al., 2020a), and this progression is essential to understanding the MRCP during real-world movement tasks. Without this understanding, knowledge about the MRCP will remain limited to controlled laboratory-based paradigms, rather than the complex movement tasks that are the focus of neurological rehabilitation (Langhorne et al., 2009, 2011; Pollock et al., 2014).
Synthesis of the MRCP literature is a challenge due to its diverse research objectives and the different terminologies used across various fields of research (Jahanshahi and Hallett, 2003). Previous literature reviews have focused on the characteristics of the MRCP and their physiological implications (Shibasaki and Hallett, 2006), the application of the MRCP to motor learning (Wright et al., 2011), its use as a predictor of an upcoming movement (Ahmadian et al., 2013; Shakeel et al., 2015), and its potential to assess outcomes following stroke (Monge-Pereira et al., 2017). However, no reviews have specifically focused on understanding the MRCP during ecologically valid movements. Therefore, this paper uses a scoping review method to explore this body of literature (Munn et al., 2018). Scoping reviews provide a systematic approach to determine the volume of evidence in an area and to provide an overview of its focus (Munn et al., 2018). The aim of this scoping review is to identify, describe, and synthesize the research evidence investigating the MRCP in ecologically valid movement tasks.
Materials and Methods
Search Strategy
A database search was conducted to identify literature across the biomedical engineering, neurophysiology, and clinical fields of research (refer to Table 1 for search terms, latest search 9th March 2021). The databases searched were: MEDline, CINAHL, SportDISCUS, Scopus, AMED, and Web of Science. Results from all databases were exported to EndNote X9, where duplicates were subsequently removed.
Screening
Title and abstract screening was independently completed by two pairs of reviewers (SO and MW; GA and SC) using the inclusion/exclusion criteria described in Table 2. Results were compared and any disagreements were settled by discussion. Full-text versions of the screened articles were then independently assessed by pairs of reviewers (MW and SC; SO and GA) for inclusion in the final review. Results were compared and any disagreement or uncertainty was settled by consultation with a third reviewer (SO or IN). Additional references were sourced by hand-searching reference lists of relevant articles. The initial inclusion criteria required studies to investigate the MRCP during voluntary upper or lower limb multi-joint movement; these were defined as limb movements involving two or more segments moving either simultaneously or sequentially. Due to the large volume of articles meeting the initial inclusion criteria, the criteria were refined to focus on the ecological validity of the movement task under investigation and its generalizability to rehabilitation. The final criteria required the MRCP movement task to be categorized in the activity or participation domains of the International Classification of Functioning, Disability and Health (ICF) (World Health Organisation, 2001). Components of walking were included (e.g., stepping), but upper limb tasks that involved only part of the task (due to simplification), or that had no clear functional application, were excluded. For example, one excluded study modified a drawing task so that the forearm and wrist were fully supported by an apparatus only allowing horizontal shoulder and elbow movement, with the fingers fixed around a cone (Fang et al., 2007).
Data Extraction and Analysis
The following information was extracted: participant characteristics, study design, study aim, purpose of the MRCP recording, task(s) used to record the MRCP, whether movements were self-paced or cued, and the key findings related to the MRCP. In addition, information about recording methods including EEG recording sites, amplifiers, filtering, pre-processing, and epochs (time/response locking and duration) were also extracted. Data extraction was carried out by three authors (MW, SC, or SO), and checked for accuracy by additional authors (SO, MJ, and UR). Extracted data was synthesized and analyzed descriptively with a focus on common objectives, and similarities and differences between populations, types of motor tasks, and recording methods. Gaps in the literature were identified.
Results
Identification and Selection of Studies
The literature search includes studies published prior to 9th March 2021. A total of 102 articles met the initial eligibility criteria, and after further refinement of the inclusion criteria, 59 articles were finally included (refer to Figure 2).
Description of Included Studies
Descriptive data for all included studies can be found in Table 3 for observational studies and Table 4 for experimental studies. A description of the EEG recording and processing methods for all studies can be found in Supplementary Table 1.
Study Designs
Within the 59 studies included, 53 studies used an observational design, while six used an experimental paradigm. Of the observational research, 25 studies utilized a single group, cross-sectional, multiple condition protocol, where two or more movements were compared in the same participants (Jung, 1982; Yazawa et al., 1997; Bayliss and Ballard, 2000; Do Nascimento et al., 2005; Jacobs et al., 2011; Welke et al., 2011; Fromer et al., 2012; Zaepffel and Brochier, 2012; Kourtis et al., 2013; Bulea et al., 2014; Jiang et al., 2015; Euler et al., 2016; Koester and Schack, 2016; Varghese et al., 2016; Martinez-Exposito et al., 2017; Peters et al., 2018; Schwarz et al., 2018; Nann et al., 2019; Berchicci et al., 2020; Braquet et al., 2020; Chaisaen et al., 2020; Reiser et al., 2020; Tomyta and Seki, 2020; Jochumsen and Niazi, 2020b; Schwarz et al., 2020b). Six studies utilized a single group, cross-sectional, single condition protocol, where only one movement task was investigated (Boulenger et al., 2008; De Oliveira et al., 2012; Knaepen et al., 2015; Sburlea et al., 2015a,b; Bodda et al., 2020). Ten studies utilized a between-group, cross-sectional design, where two groups (e.g., experts versus novices) performed one movement task (Mann et al., 2011; Wright et al., 2012b; Khanmohammadi et al., 2015; Vogt et al., 2017; Skrzeba and Vogt, 2018), or where two groups performed multiple movement tasks (O’connor, 1986; Vidailhet et al., 1993, 1995; Berchicci et al., 2017; Fearon et al., 2021). Twelve studies investigated multiple EEG measurement or signal processing techniques (Khaliliardali et al., 2012, 2015; Singh et al., 2016; Jeong et al., 2017; Sburlea et al., 2017; Rashid et al., 2018; Eilbeigi and Setarehdan, 2018a,b; Karimi and Jiang, 2019; Moinnereau et al., 2019; Russo et al., 2019; Schwarz et al., 2020a). The six experimental studies consisted of two randomized controlled trials (Mizusaki et al., 2019; Peters et al., 2020), one non-randomized controlled trial (Fromer et al., 2016), one randomized cross-over trial (Barthel et al., 2001), one non-randomized cross-over trial (Mrachacz-Kersting et al., 2019a), and one non-controlled trial (Wright et al., 2012a).
Participants
Within the 59 included studies, 50 investigated healthy populations, one investigated healthy young and older adults (Khanmohammadi et al., 2015), and four investigated both healthy and clinical populations [stroke (Sburlea et al., 2017), Parkinson’s Disease (PD) (Vidailhet et al., 1993; Fearon et al., 2021), and gait-ignition failure syndrome (Vidailhet et al., 1995)]. A further three studies investigated stroke only populations (Sburlea et al., 2015a; Peters et al., 2018; Peters et al., 2020) and one study investigated smokers (O’connor, 1986). Sample sizes ranged from 2 to 120 participants and ages ranged from 18 to 84 years.
Movement Tasks Generating the Movement-Related Cortical Potential
There were a wide range of movement tasks investigated. These movements could be categorized into five groups: (1) specialized goal-directed activities, which included movement tasks such as juggling, guitar playing, golf putting, and bungee jumping, (2) walking-related tasks, (3) reach and grasp, (4) virtual driving, and (5) sit-to-stand.
Many of the articles categorized under ‘walking-related’ or ‘sit-to-stand’ activities had an overarching aim focused on developing BCI-assistive robotic devices to aid their respective task (Do Nascimento et al., 2005; Bulea et al., 2014; Jiang et al., 2015; Sburlea et al., 2015a,b, 2017; Singh et al., 2016; Jeong et al., 2017; Karimi and Jiang, 2019; Chaisaen et al., 2020; Jochumsen and Niazi, 2020b). While the virtual driving literature often focused on the development of intelligent cars (Welke et al., 2011; Khaliliardali et al., 2012, 2015; Moinnereau et al., 2019). The reach-to-grasp literature primarily aimed to investigate MRCP differences between various reach/grasp types or task goals (De Oliveira et al., 2012; Koester and Schack, 2016; Schwarz et al., 2018, 2020a,b). This was mainly to investigate if the MRCP could be used to differentiate between similar movement tasks. Additionally, articles using reach-to-grasp movements investigated the MRCP under different environmental conditions (Boulenger et al., 2008; Zaepffel and Brochier, 2012; Kourtis et al., 2013; Koester and Schack, 2016), such as when reaching for emotionally unpleasant objects (De Oliveira et al., 2012).
Mode (Self-Paced Versus Cued)
A similar number of studies used self-paced movement (n = 28) compared to cued movement (n = 28), with three studies investigating both (Jung, 1982; Bulea et al., 2014; Tomyta and Seki, 2020). Two studies compared self-paced with cued movements and found no significant differences in MRCP characteristics (Bulea et al., 2014; Tomyta and Seki, 2020).
Observational Research: Movement-Related Cortical Potential Characteristics
Movement Complexity and Expertise
Of the observational research, eight studies compared differences between simple and complex movements (e.g., ankle dorsiflexion compared with forward stepping, 1-ball versus 2-ball juggling, large-target versus small-target throwing) (Vidailhet et al., 1993, 1995; Yazawa et al., 1997; Fromer et al., 2012; Berchicci et al., 2017; Martinez-Exposito et al., 2017; Rashid et al., 2018; Tomyta and Seki, 2020). In six of these eight studies, MRCP amplitudes were significantly larger in more complex movement tasks (Vidailhet et al., 1993, 1995; Yazawa et al., 1997; Fromer et al., 2012; Berchicci et al., 2017; Tomyta and Seki, 2020). One study found the prefrontal MRCP onset was earlier in more complex juggling movements in both expert jugglers and novices (Berchicci et al., 2017). In contrast, a study that compared step-ups with simple ankle dorsiflexion in healthy participants found a larger peak negativity for the simple movement, and no difference in the peak negativity timing (Rashid et al., 2018).
Movement complexity was also manipulated with the use of targets. For example, Fromer et al. found that simulated dart throwing with a Wii remote resulted in larger MRCP amplitudes when aiming for a small target (increased difficulty) compared with a large target (Fromer et al., 2012). Jung compared slow finger pointing and rapid punching to the same target and observed a longer MRCP duration for the slow pointing condition (Jung, 1982).
The interaction between movement task complexity and an individual’s stage of learning was investigated in five studies which compared novices and experts performing tasks such as juggling (Berchicci et al., 2017), golf putting (Mann et al., 2011), badminton serving (Skrzeba and Vogt, 2018), archery (Vogt et al., 2017), and guitar playing (Wright et al., 2012b). Four of five studies found larger MRCP amplitudes in the expert groups (Mann et al., 2011; Berchicci et al., 2017; Vogt et al., 2017; Skrzeba and Vogt, 2018), while one study found smaller MRCP amplitudes in experts (Wright et al., 2012b). Two of these studies also investigated MRCP latency and showed a later MRCP onset in skilled versus non-skilled archers (Vogt et al., 2017) and a later MRCP negative slope in expert guitar players compared to novices (Wright et al., 2012b).
Reach and Grasp
Several studies investigated MRCPs during different grasp types. Unimanual and bimanual reach-to-grasp movements showed the characteristic peak negativity near movement onset, but also had a second smaller negative peak approximately 250–400 ms after movement onset (Schwarz et al., 2018, 2020a,b). Schwarz et al. found no differences in MRCP amplitudes between unimanual versus bimanual tasks or between lateral grasps (of a spoon) and palmar grasps (of a jar) (Schwarz et al., 2020a,b), whereas Koester and Schack found larger MRCP negativity 100–300 ms after movement onset with a two-finger precision grasp (of a small cube) versus a palmar grasp (of a large cube) (Koester and Schack, 2016). Schwarz et al. found differences in MRCP timing with different grasp types; the positive rebound after the second negative peak (which coincided with the completion of the grasp movement) occurred earlier for key grasps compared with pincer or palmer grasps (Schwarz et al., 2018).
Walking and Mobility
Several studies compared the MRCP under different stepping conditions. Gait initiation or stepping in the backward direction produced larger amplitude MRCPs than the forward direction (Do Nascimento et al., 2005; Russo et al., 2019; Berchicci et al., 2020). Forward gait initiation produced smaller amplitude MRCPs than step-up, side-step, backward step, and stand-to-sit movements (Jochumsen and Niazi, 2020b). In addition, lateral stepping produced smaller MRCPs if the weight was pre-shifted to the supporting leg than if the weight was equally distributed prior to stepping (Varghese et al., 2016).
Driving
Studies that investigated MRCPs during virtual driving could identify an MRCP prior to the presentation of Go and Stop signals, a peak negativity aligning with the Go/Stop signals, and a larger peak negativity for Stop versus Go movements (Bayliss and Ballard, 2000; Khaliliardali et al., 2012). MRCP onsets occurred approximately 190ms prior to left and right turns, with no differences between the two (Welke et al., 2011).
Attention and Emotion
Several studies investigated the effects of different attentional loads by manipulating the visual cue. Disorientating visual cues during a stepping task did not alter MRCP amplitude or latency (Braquet et al., 2020), but unfamiliar complex visual cues during an upper limb movement sequence produced a smaller MRCP amplitude and later peak negativity (Euler et al., 2016). Subliminal exposure to action verbs versus concrete nouns during movement preparation of a reach-to-grasp movement produced a smaller MRCP amplitude (Boulenger et al., 2008). Whereas, providing a visual cue denoting the force level and grasp type required (3 s prior to the Go signal), produced a larger MRCP amplitude than providing no cuing information (Zaepffel and Brochier, 2012).
Two studies manipulated attention under a dual-tasking paradigm, where the MRCP was recorded during a simple hand movement while participants also performed a walking task (Reiser et al., 2020; Fearon et al., 2021). In one study, participants performed a cognitive task that resulted in pressing a response handle while also performing a secondary walking task; the MRCP amplitudes for the hand movement were smaller while walking compared to standing still (Reiser et al., 2020). In the second study, healthy participants or people with PD performed a button pressing task while either sitting or walking in place; the MRCP duration was longer during the dual-task condition in people with PD and freezing of gait, compared to people with PD without freezing of gait and healthy controls (Fearon et al., 2021).
Attention was also manipulated by performing a shared task with a partner; during a shared task where one person picked up and passed an object to another person, the peak negativity of the MRCP of the person receiving the object aligned more closely with the movement onset of the person who had picked up the object than their own movement onset (Kourtis et al., 2013). In terms of emotional loads, reaching for emotionally unpleasant stimuli produced larger amplitude MRCPs (De Oliveira et al., 2012), but a 192-m bungee jump produced the same MRCP onset and amplitude as jumping off a 1-m platform in two professional cliff divers (Nann et al., 2019).
Aging and Neurological Conditions
When comparing younger and older adults performing a forward step, older adults had an earlier MRCP peak negativity and a smaller amplitude of the late MRCP (Khanmohammadi et al., 2015). When comparing healthy individuals with people with PD, MRCP amplitudes were smaller in the PD group during both gait initiation and seated dorsiflexion (Vidailhet et al., 1993). However, the MRCP for a simple hand movement when recorded under a walking dual-task condition, had a larger amplitude in people with PD and freezing of gait, compared to people with PD without freezing of gait and healthy controls (Fearon et al., 2021). When comparing healthy individuals with those with isolated gait ignition failure, no consistent differences in MRCP amplitudes were observed; although this was a small descriptive study (Vidailhet et al., 1995). Of the four studies that investigated stroke populations, none specifically compared the MRCP characteristics between healthy and stroke participants. However, one study compared step-ups with the more-affected and less-affected legs and found no differences in MRCP amplitude or duration (Peters et al., 2018).
Movement-Related Cortical Potential Extraction, Detection, and Classification
Ten articles investigated different EEG measurement or processing systems during mobility-related tasks (Bulea et al., 2014; Sburlea et al., 2015a,b, 2017; Jeong et al., 2017; Rashid et al., 2018; Karimi and Jiang, 2019; Russo et al., 2019; Chaisaen et al., 2020; Jochumsen and Niazi, 2020b). Findings from all but one of these studies (Russo et al., 2019) demonstrated that the MRCP signal could be successfully classified or enhanced using multiple techniques (this was not the aim of Russo et al., 2019). Accuracy rates varied when using the different signal processing techniques, but similar accuracies were reported when comparing healthy and stroke participants (Sburlea et al., 2015b, 2017). Five articles in the reach-to-grasp dataset examined different EEG measurement or processing techniques (Eilbeigi and Setarehdan, 2018a,b; Schwarz et al., 2018, 2020a,b). Schwarz et al. found a dry electrode system produced a lower amplitude MRCP with attenuated peaks compared to gel- or water-based electrodes (Schwarz et al., 2020a). Eilbeigi and Setarehdan found global optimized constraint independent component analysis (GocICA) more effective at denoising the EEG for enhancing multichannel EEG signal detection of the MRCP compared to constrained independent component analysis (cICA) (Eilbeigi and Setarehdan, 2018a,b). Schwarz et al. found binary single-trial classification to be superior to multiclass single-trial classification, with accuracy rates of 93.5 and 65.9%, respectively (Schwarz et al., 2018). Similarly, the virtual driving research focused on analysis of EEG processing algorithms used to remove excess signal noise (Khaliliardali et al., 2012, 2015; Moinnereau et al., 2019). For example, Moinnereau et al. (2019) compared accelerometer-based ICA, cICA and empirical model decomposition (EMP) analysis, and found that denoising with cICA led to the highest classification accuracy. They also found a processing window of greater than 3 s sufficient to provide reliable classification. Two studies (Singh et al., 2016; Russo et al., 2019) compared the effect of different means of synchronization. It was reported that the peak negativity of the MRCP occurred earlier when synchronizing the EEG to EMG compared with gyroscope data (Singh et al., 2016), while synchronization of the EEG using a force plate was associated with MRCPs of greater amplitude compared to synchronization with EMG or stereophotogrammetry (Russo et al., 2019).
Experimental Research
Six studies had an experimental design (Table 4), with one study using the MRCP as part of their intervention (Mrachacz-Kersting et al., 2019a). This associative BCI-intervention was applied to seven healthy participants and involved timing radial nerve electrical stimulation to the MRCP generated during either a simple wrist extension task or a complex reach-to-grasp task. Increased corticomotor excitability was observed with the simple wrist extension condition, although no statistical analysis was performed (Mrachacz-Kersting et al., 2019a).
The remaining five experimental studies used the MRCP as an outcome measure to determine the effect of an intervention. The movement tasks used to measure the MRCP were all used as part of the interventions, for example; pedaling (Barthel et al., 2001), dart throwing (Fromer et al., 2016; Mizusaki et al., 2019), guitar playing (Wright et al., 2012a), and step-ups (Peters et al., 2020). Two studies showed a decrease in MRCP amplitude with improved performance in dart throwing (Fromer et al., 2016) and guitar playing (Wright et al., 2012a). Another study showed a decrease in MRCP amplitude with physical exertion over a single session (Barthel et al., 2001). The only experimental study that was undertaken with participants with stroke (n = 7) observed a decrease in the duration of MRCPs recorded during step-ups following 12 training sessions (physical therapy or fast stepping training), although there was no statistical analysis (Peters et al., 2020). A final study investigated MRCP changes following nine dart throwing sessions using eye gaze training or control training; they reported no significant between-group differences in MRCP amplitude, but did not report within-group changes (Mizusaki et al., 2019).
Discussion
This review is the first of its kind in this field and offers researchers and clinicians important insight into the breadth of research investigating the MRCP during ecologically valid movements. The following discussion focuses on: the characteristics of the MRCP in various populations and under various task conditions, the potential use of the MRCP as an outcome measure, and the application of the MRCP within rehabilitation interventions.
Observational Research: Movement-Related Cortical Potential Characteristics
This review identified a number of studies that compared the MRCP during complex and simple movements. In general, larger MRCP amplitudes were seen with more complex movements, for example in 2-ball versus 1-ball juggling (Berchicci et al., 2017), dart throwing movement versus button release (Fromer et al., 2012), 4-finger tapping versus 1-finger tapping or drum-stick tapping (Tomyta and Seki, 2020), forward stepping versus ankle dorsiflexion (Vidailhet et al., 1993, 1995), and gait initiation versus ankle dorsiflexion (Yazawa et al., 1997). This aligns with the understanding that MRCPs reflect motor planning processes, and that more difficult tasks elicit cortical responses of greater magnitude, thereby generating larger MRCP amplitudes (Shibasaki and Hallett, 2006). This is further supported by studies of walking tasks that found that more complex gait tasks, such as backward walking, backward stepping, and forward step-ups, produced larger MRCP amplitudes than forward stepping or walking (Do Nascimento et al., 2005; Russo et al., 2019; Berchicci et al., 2020; Jochumsen and Niazi, 2020b). In contrast, one study showed smaller MRCP amplitudes during step-ups compared with simple ankle dorsiflexion (Rashid et al., 2018). The reason for these contrasting findings is not clear and does not appear to be related to differences in study design, but readers should consider that this body of evidence is small and that factors such as motivation, effort, and the kinematics of the movement may influence the findings (Do Nascimento et al., 2006; Shibasaki and Hallett, 2006; Jochumsen et al., 2013).
Previous research on simple movements has established the idea that as an individual develops expertise in a movement task, the relative difficulty of that task decreases and the associated MRCP amplitude is smaller (Wright et al., 2011). This review of more complex movements included one study that reflected this; during a guitar-playing task, experienced guitarists had smaller-amplitude MRCPs than non-musicians (Wright et al., 2012b). However, we also identified four studies in which experts demonstrated larger-amplitude MRCPs than novices during tasks such as juggling (Berchicci et al., 2017), golf putting (Mann et al., 2011), badminton serving (Skrzeba and Vogt, 2018), and archery (Vogt et al., 2017). These contrasting findings may be explained by the different task requirements. The smaller-amplitude MRCP was observed when expert guitarists played the G-major scale which may reflect the relative automaticity of this task in these experts, where they likely required less cognitive workload than non-musicians to manipulate the guitar strings in a seated position (Wright et al., 2012b). Whereas, the motor tasks that produced larger MRCPs in experts (juggling, golf, badminton, archery) have a high degree of uncertainty and require a high level of motor control and precision; thus, the increased performance of experts in these more complex tasks appeared to be associated with greater activation of motor preparation areas, due to the multisensory integration required. The increased motor preparation required for target-based tasks such as badminton and juggling aligns with findings of Fromer et al. where simulated dart throwing with a small target (i.e., a more difficult task) produced larger amplitude MRCPs than a large target (Fromer et al., 2012).
A number of studies manipulated various factors related to attention in order to understand the MRCP under different task conditions. The presentation of an unfamiliar complex visual cue prior to an upper limb sequence task produced a smaller MRCP amplitude and later peak negativity (Euler et al., 2016). Similar findings have been observed during simple movements. For example, smaller MRCP amplitudes have been recorded when a cognitively demanding task preceded a button-pushing movement (Baker et al., 2011) and when an attention-diverting auditory task was performed concurrently with an ankle dorsiflexion task (Aliakbaryhosseinabadi et al., 2017). This might suggest that increasing the cognitive load associated with a movement task reduces resources available for motor preparation (Baker et al., 2011). However, other research findings in this review contrasted with this. Disorientating visual cues during a stepping task did not affect the MRCP (Braquet et al., 2020), perhaps because of the automatic nature of the stepping task (Clark, 2015), and the provision of additional cues about the type of upcoming grasp movement produced a larger MRCP amplitude (Zaepffel and Brochier, 2012). These combined results might suggest that when the cognitive conditions of the task increase attention onto the upcoming complex motor task there is an increase in motor preparation, whereas when the cognitive load diverts attention away from the movement task there is a reduction in motor preparation. The resources available for motor preparation can also be reduced by adding a secondary physical task as seen when the MRCP recorded during a simple hand movement had a smaller amplitude when participants performed a concurrent walking task compared to standing still (Reiser et al., 2020). When a similar dual-tasking paradigm was performed by people with PD and healthy controls, there was no difference in MRCP amplitude between single and dual-task conditions, but people with PD and freezing of gait had a longer MRCP duration under the dual-task condition (Fearon et al., 2021), suggesting that the addition of the secondary walking task altered the cortical processes required for the preparation of a simple hand movement. These findings related to the presence of concurrent cognitive or physical task demands may have implications for neurological rehabilitation where patients frequently experience impairments in attention (Rabinowitz and Levin, 2014; Loetscher et al., 2019) and where the rehabilitation environment can be noisy and distracting. Therapists should consider whether the presence of attention-diverting stimuli is hindering the patients ability to activate motor cortical areas, or in contrast, whether it presents a useful challenge to their motor planning when incorporated into a dual-task training program (Fritz et al., 2015).
In terms of emotional stimuli, MRCP amplitudes were larger when reaching for unpleasant compared with pleasant objects (De Oliveira et al., 2012), reinforcing that the MRCP is susceptible to task-related factors. Given this effect, it was surprising that two cliff divers produced comparable MRCPs when jumping off a 1-m platform and bungee jumping from 192 m (Nann et al., 2019), however, their familiarity with this task may have reduced their perception of risk and limited the influence of their emotions. Previous literature has established that MRCP characteristics during simple movement tasks are modulated by emotional and stressful stimuli, and also by the individuals level of anxiety (Knott and Irwin, 1973; Glanzmann and Froehlich, 1984; Perri et al., 2014). This area requires further research in ecologically valid movements to enable clinicians to understand whether emotionally stimulating environments facilitate or inhibit motor preparation and execution.
An important finding of this review is the scarcity of studies investigating MRCPs in people with clinical conditions. The seven observational studies in clinical or older adult populations investigated forward stepping, gait initiation, step-ups, or used walking as a secondary task (Vidailhet et al., 1993, 1995; Khanmohammadi et al., 2015; Sburlea et al., 2015a, 2017; Peters et al., 2018; Fearon et al., 2021), which is encouraging given the strong focus on mobility tasks in rehabilitation (Langhorne et al., 2011). However, most of the sample sizes were small, particularly for the study of people with gait ignition failure (n = 4) (Vidailhet et al., 1995). There were a few notable findings from these studies. The MRCP peak negativity occurred earlier in older adults compared to younger adults during a cued forward-stepping task, which might reflect an impaired ability to anticipate the timing of the upcoming stimulus (Khanmohammadi et al., 2015) or compensation for delayed force production (Klass et al., 2008). Older adults also had a smaller amplitude of the late MRCP (Fz) during stepping (Khanmohammadi et al., 2015), aligning with findings in simple finger movement tasks (Michalewski et al., 1980; Golob et al., 2005) and suggesting age-related changes in motor planning. People with PD had smaller MRCP amplitudes compared to healthy adults during self-paced ankle dorsiflexion or forward stepping (Vidailhet et al., 1993), but people with PD and freezing of gait had larger MRCP amplitudes compared to healthy adults and people with PD without freezing of gait during a cued button-pressing task (Fearon et al., 2021). These contrasting results may reflect differences between the samples or variability between the tasks. At this stage there is insufficient evidence to draw conclusions from this literature.
Only one study in this review provided information about the characteristics of the MRCP following stroke. This study found no difference in MRCP amplitude or duration during step-ups between the more-affected and less-affected legs (Peters et al., 2018). Thus, knowledge about MRCP characteristics during ecologically valid movement in people with stroke is very limited. More stroke research has been carried out during simple movements, but with variable findings. Fattapposta et al. found that people with acute stroke had a smaller MRCP amplitude during index finger movements when compared to healthy participants (Fattapposta et al., 2008), which might reflect the suppression of cortical excitability in the affected hemisphere that occurs early after stroke (Stinear et al., 2015). Interestingly, in this study the MRCP amplitude increased over the subsequent 12 months (Fattapposta et al., 2008), possibly reflecting motor recovery. Similarly, other chronic stroke studies have shown MRCPs with earlier onsets and larger amplitudes; this has been observed in the more-affected limb compared with a healthy control limb during a horizontal shoulder flexion and elbow extension task (Daly et al., 2006) and during attempted finger flexion/extension in people with stroke who have severe paresis (Yilmaz et al., 2014). This suggests that people with chronic stroke may need greater levels of cortical activation to produce or attempt simple movements of the affected arm. In contrast, other studies of simple finger movements have shown that smaller MRCP amplitudes are maintained in the affected hemisphere in the chronic stage of stroke (Wiese et al., 2005; Dean et al., 2012), which may reflect poor recovery of movement. Studies of simple movements following stroke have also shown variability in the location of MRCP signals (Daly et al., 2006; Yilmaz et al., 2014), which likely reflects cortical reorganization in response to the lesion (Hosp and Luft, 2011). Further research is needed in stroke and other clinical populations. Longitudinal studies across the recovery process would increase our understanding of how the MRCP could be used as a biomarker of recovery. Ideally, ecologically valid movements should be investigated, however, it is acknowledged that simple movement tasks may be more achievable for individuals with more severe impairment.
Movement-Related Cortical Potentials as Outcome Measures
Five of the six experimental studies utilized the MRCP as an outcome measure; three of these studies detected changes in the MRCP in response to the interventions (Barthel et al., 2001; Wright et al., 2012a; Fromer et al., 2016) and one small study observed changes but did not perform a statistical analysis (Peters et al., 2020). The movement tasks used to record the signal were all relevant to the interventions being assessed (Barthel et al., 2001; Wright et al., 2012a; Fromer et al., 2016; Mizusaki et al., 2019; Peters et al., 2020). For example, Peters et al. used step-ups to record the MRCP, which were also part of the fast muscle training and stepping activation intervention under investigation (Peters et al., 2020). Two other studies trained dart throwing and used a simulated or real dart throw to record the MRCP (Fromer et al., 2016; Mizusaki et al., 2019). While this body of research is small, it is promising that these studies have successfully measured intervention efficacy using an MRCP recorded during ecologically valid movements. However, the ecological validity of these movements could be further improved; for example, in Fromer et al. the dart throw was simulated with a Wii remote rather than using a real dart and dartboard (Fromer et al., 2016). One of the limitations of analyzing MRCPs is that the signal is detected prior to movement, and therefore more suited to measuring discrete tasks (e.g., stepping) rather than continuous tasks (e.g., walking, climbing stairs). This limitation was illustrated in a study where the movement of interest was continuous cycling but the MRCP was recorded during right-leg kick-type movements on a cycle ergometer (Barthel et al., 2001). Given the specificity of neural plasticity, one would not necessarily expect changes in one task to transfer to improvements in another (Kleim and Jones, 2008). One paper in this review did detect an MRCP-like signal during continuous gait (Knaepen et al., 2015). Further research in continuous movements, such as walking, is needed to determine whether the MRCP can be measured in these tasks and to determine the potential of MRCPs to measure changes following walking interventions. Importantly, if the MRCP is to be useful as an outcome measure following rehabilitation interventions, its reliability must be established. Surprisingly, despite its discovery over 50 years ago, the reliability of the MRCP remains untested in both simple and complex movements. Lack of stability in the MRCP signal could explain many of the contrasting findings in the literature and therefore must be a priority for future research.
Movement-Related Cortical Potential-Driven Interventions
One experimental study in this review utilized the MRCP within a BCI neuromodulatory intervention; this study reported that the MRCP was recorded during a complex reach-to-grasp task, and thus was determined to be ecologically valid, however, there was limited detail about the features of the movement task (Mrachacz-Kersting et al., 2019a). In addition, results from the seven participants in this study were preliminary and no statistical analysis was performed. Multiple other studies have tested the same MRCP-based intervention, but these have been largely limited to simple movements and laboratory environments, with most studies involving healthy participants (Mrachacz-Kersting et al., 2012; Niazi et al., 2012; Kristensen et al., 2013; Jochumsen et al., 2015b, 2016; Mrachacz-Kersting and Aliakbaryhosseinabadi, 2018; Olsen et al., 2018), a few laboratory-based studies involving people with stroke (Mrachacz-Kersting et al., 2016; Olsen et al., 2020), and only one stroke study carried out in a subacute rehabilitation setting (Mrachacz-Kersting et al., 2019b). Expanding the use of this MRCP-based neuromodulatory intervention into ecologically valid movement tasks would increase the likelihood of feasibly translating this intervention into rehabilitation practice, where simple, single-joint movements are unlikely to provide the demands needed to promote recovery (Kleim and Jones, 2008).
Within rehabilitation there is also potential for other MRCP-driven devices. For example, for people with severe motor impairments such as spinal cord injury or amyotrophic lateral sclerosis, the MRCP may be a means for controlling assistive devices such as wheelchairs, robotic arms, or communication tools (Millan et al., 2010). This review did not identify any studies which used the MRCP from an ecologically valid movement as a control signal for an external assistive device. Several studies in this review showed that the MRCP from various ecologically valid movements can be detected and classified in healthy people and people with stroke. However, the MRCPs slow potential makes it difficult to obtain high classification accuracies. The highest classification accuracies are, not surprisingly, obtained when the number of classes is low, with accuracy reducing as more classes are added into the classification. The best discrimination is generally obtained when classifying between a movement and idle/rest activity (Jochumsen and Niazi, 2020b; Schwarz et al., 2020b). One of the challenges of using the MRCP during ecologically valid movement is that it shares the signal bandwidth with motion artifacts and therefore it can be challenging to implement the pre-processing required to remove noise during online classification (Kline et al., 2015; Oliveira et al., 2016; Richer et al., 2020). Indeed, a number of studies in this review excluded participant data for this reason (see Table 3). From the studies reviewed, it is difficult to determine which ecologically valid movement types are easiest to classify due to: (1) similarities in the morphology of the premovement signal, (2) different signal processing techniques used for denoising, feature extraction, and classification, (3) the different number of movement classes, and (4) the high inter-participant variability which is inherent in these types of analyses and exacerbated by the different participants used in each study. In addition, in some studies epochs are rejected (with varying criteria) to make the data set cleaner for classification but gives it less resemblance to a real-world scenario. Given the difficulties obtaining high classification accuracies, using traditional machine learning algorithms to process the MRCP signal from ecologically valid movements may not be ideal for controlling external assistive devices. However, given the aim of many BCI assistive devices is to support individuals with more-severe paralysis, MRCPs recorded during simple attempted movements may be sufficient in the early phase of rehabilitation and may still provide some level of device control. Studies have shown that MRCPs during attempted hand movements can be recorded from individuals with spinal cord injury and have the potential to control an upper limb neuroprosthesis (Müller-Putz et al., 2019; Ofner et al., 2019). If a BCI-controlled device requires EEG signals generated during more complex movements, different techniques for enhancing MRCP control should be explored by improving decoding algorithms or introducing cyclic command menus (Xu et al., 2019). Another limitation of MRCPs is the slower transfer rate; more accurate movement classification may be obtained from BCI control signals such as steady-state evoked potentials or P300, which allow higher information transfer rates (Wolpaw et al., 2002). The disadvantage of these approaches is that the user needs to focus on a screen with flickering icons or characters to elicit the signals for controlling the external devices; this can be exhausting and provides additional challenges when transferring the BCI to an outdoor environment. An additional area for development is the use of BCIs to control intelligent vehicles for individuals left unable to drive following injury or illness; however, again, control signals other than MRCPs could be more useful depending on the amount of automation/intelligence that is built into the vehicle. Thus, while there is potential for MRCP-driven device control, MRCPs may be more suited to intervention paradigms where prediction of a movement task (before onset) is required to exploit Hebbian plasticity (Mrachacz-Kersting et al., 2019b).
Limitations
As a scoping review, this paper has described the breadth of MRCP literature related to ecologically valid movements but has not attempted to critique the quality of research methods or the validity of the findings. It was beyond the scope of this review to provide an in-depth critique of various surface EEG recording or signal processing methods, however, such methods have been described in Supplementary Table 1. A previous narrative review has summarized different EEG methods for decoding movement intention from MRCPs (Shakeel et al., 2015). A future systematic review could investigate such methods in more detail in a more defined population or movement type. In addition, this review was limited to surface EEG recordings and does not address invasive EEG methods; such methods offer an improved signal to noise ratio but require surgical intervention (Volkova et al., 2019) and thus have limited clinical feasibility. The scope of this review did not include imagined, single-joint, or partial upper limb movements; however, it is acknowledged that these simpler or more constrained movements have often been utilized in studies of clinical populations such as stroke (Daly et al., 2006; Fang et al., 2007) and spinal cord injury (Lopez-Larraz et al., 2016; Trincado-Alonso et al., 2018; Ofner et al., 2019), and that the body of clinical literature is broader than what is presented here. Given the lack of clinical studies utilizing MRCPs during ecologically valid movements, future systematic reviews of clinical populations should incorporate a broader range of movement types but ask a more specific question. For example, a systematic review could explore the use of the MRCP to control assistive devices in neurological populations.
In terms of the limitations of the primary research in this review, the description of the MRCP movement parameters was often poor; this may have resulted in the exclusion of studies that used ecologically valid movements but did not adequately describe them. Movement tasks were often modified due to constraints of the research environment and current EEG technology, thus preventing them from meeting the inclusion criteria for an ecologically valid movement. While the 59 included articles investigated tasks deemed to be ecologically valid, there are still limitations in generalizing these findings to the real world. For example, the findings from Mizusaki et al. in seated dart throwing may not necessarily transfer to standing dart throwing, but may resemble dart throwing in wheelchair users (Mizusaki et al., 2019). In addition, due to the heterogeneity of study protocols and the variable findings, caution should be taken when attempting to generalize the findings to different tasks and populations.
Conclusion
This scoping review synthesized the research evidence investigating the MRCP in ecologically valid movement tasks. The 59 included studies demonstrated that the MRCP has been investigated across a broad range of functional and complex motor tasks, but largely in healthy participants. MRCP characteristics appear to vary across different movement tasks and participant groups. MRCP amplitudes are larger with movements of greater complexity. In addition, the MRCP is altered when a cognitive or secondary physical task is performed prior to, or during the movement, reflecting changes in the resources available for motor preparation. The small body of literature examining clinical populations focused on walking-related tasks. While some of these studies demonstrated differences in MRCP characteristics in older adults and people with PD, more research is needed in populations with neurological or age-related impairments to clearly establish how the MRCP changes with disease progression and recovery, and to determine how altering the physical or cognitive requirements of the task influences motor preparation processes. The MRCP has potential to be used as a measure of intervention efficacy, as shown in five studies in this review; however, further research is needed to establish the reliability of the MRCP during movement tasks that are functionally relevant to rehabilitation and recovery. There is minimal research exploring MRCP-based neuromodulatory interventions during ecologically valid movement tasks and this is an area for further development. Although the MRCP can be used to classify real-world movements, it is difficult to obtain high classification accuracies, and this likely explains the absence of studies using MRCPs from ecologically valid movements to control BCI-driven robotics or intelligent cars. Further research is needed to address the technical challenges associated with recording MRCPs to ensure future studies can focus on ecological validity. This will facilitate the implementation of this research into rehabilitation practice.
Author Contributions
DT, NS, IN, MJ, SO, GA, and UR devised the conceptual framework for the wider body of research that encompasses this study. SO and GA designed the study and provided supervision of MW and SC. MW, SC, and SO conducted the database search. SO, GA, MW, and SC conducted manuscript screening, with input from IN. MW, SC, SO, GA, MJ, and UR, extracted and cross-checked data. All the authors contributed to the interpretation of data, provided critical feedback, and helped shape the writing. SO, GA, MW, and SC drafted the manuscript.
Funding
This study was funded by the Auckland University of Technology.
Conflict of Interest
The authors declare that the research was conducted in the absence of any commercial or financial relationships that could be construed as a potential conflict of interest.
Publisher’s Note
All claims expressed in this article are solely those of the authors and do not necessarily represent those of their affiliated organizations, or those of the publisher, the editors and the reviewers. Any product that may be evaluated in this article, or claim that may be made by its manufacturer, is not guaranteed or endorsed by the publisher.
Supplementary Material
The Supplementary Material for this article can be found online at: https://www.frontiersin.org/articles/10.3389/fnins.2021.721387/full#supplementary-material
References
Ahmadian, P., Cagnoni, S., and Ascari, L. (2013). How capable is non-invasive EEG data of predicting the next movement? A mini review. Front. Hum. Neurosci. 7:124. doi: 10.3389/fnhum.2013.00124
Aliakbaryhosseinabadi, S., Kamavuako, E. N., Jiang, N., Farina, D., and Mrachacz-Kersting, N. (2017). Influence of dual-tasking with different levels of attention diversion on characteristics of the movement-related cortical potential. Brain Res. 1674, 10–19. doi: 10.1016/j.brainres.2017.08.016
Baker, K. S., Mattingley, J. B., Chambers, C. D., and Cunnington, R. (2011). Attention and the readiness for action. Neuropsychologia 49, 3303–3313. doi: 10.1016/j.neuropsychologia.2011.08.003
Barthel, T., Mechau, D., Wehr, T., Schnittker, R., Liesen, H., and Weiss, M. (2001). Readiness potential in different states of physical activation and after ingestion of taurine and/or caffeine containing drinks. Amino Acids 20, 63–73. doi: 10.1007/s007260170066
Bayliss, J. D., and Ballard, D. H. (2000). Recognizing evoked potentials in a virtual environment. Adv. Neural. Inf. Process Syst. 12, 3–9.
Berchicci, M., Quinzi, F., Dainese, A., and Di Russo, F. (2017). Time-source of neural plasticity in complex bimanual coordinative tasks: juggling. Behav. Brain Res. 328, 87–94. doi: 10.1016/j.bbr.2017.04.011
Berchicci, M., Russo, Y., Bianco, V., Quinzi, F., Rum, L., Macaluso, A., et al. (2020). Stepping forward, stepping backward: a movement-related cortical potential study unveils distinctive brain activities. Behav. Brain Res. 388:112663. doi: 10.1016/j.bbr.2020.112663
Bibian, C., Lopez-Larraz, E., Irastorza-Landa, N., Birbaumer, N., Ramos-Murguialday, A., and Ieee. (2017). “Evaluation of filtering techniques to extract movement intention information from low-frequency EEG activity,” in 2017 39th Annual International Conference of the Ieee Engineering in Medicine and Biology Society (Jeju Island) 2960–2963.
Bodda, S., Maya, S., Em Potti, M. N., Sohan, U., Bhuvaneshwari, Y., Mathiyoth, R., et al. (2020). Computational analysis of EEG activity during stance and swing gait phases. Procedia Comp. Sci. 171, 1591–1597.
Boulenger, V., Silber, B. Y., Roy, A. C., Paulignan, Y., Jeannerod, M., and Nazir, T. A. (2008). Subliminal display of action words interferes with motor planning: a combined EEG and kinematic study. J. Physiol. Paris. 102, 130–136. doi: 10.1016/j.jphysparis.2008.03.015
Braquet, A., Bayot, M., Tard, C., Defebvre, L., Derambure, P., Dujardin, K., et al. (2020). A new paradigm to study the influence of attentional load on cortical activity for motor preparation of step initiation. Exp. Brain Res. 2020:5739. doi: 10.1007/s00221-020-05739-5
Brunia, C. (2003). “CNV and SPN. Indices of anticipatory behaviour,” in The Bereitschaftspotential. Movement-Related Cortical Potentials, eds M. Jahanshahi and M. Hallett (New York, NY: Springer).
Bulea, T. C., Prasad, S., Kilicarslan, A., and Contreras-Vidal, J. L. (2014). Sitting and standing intention can be decoded from scalp EEG recorded prior to movement execution. Front. Neurosci. 8:376. doi: 10.3389/fnins.2014.00376
Chaisaen, R., Autthasan, P., Mingchinda, N., Leelaarporn, P., Kunaseth, N., Tammajarung, S., et al. (2020). Decoding EEG rhythms during action observation. motor imagery, and execution for standing and sitting. IEEE Sensors J. 20, 13776–13786. doi: 10.1109/jsen.2020.3005968
Clark, D. J. (2015). Automaticity of walking: functional significance, mechanisms, measurement and rehabilitation strategies. Front. Hum. Neurosci. 9:246. doi: 10.3389/fnhum.2015.00246
Daly, J. J., Fang, Y., Perepezko, E. M., Siemionow, V., and Yue, G. H. (2006). Prolonged cognitive planning time, elevated cognitive effort, and relationship to coordination and motor control following stroke. Ieee Transac. Neural Syst. Rehabilit. Eng. 14, 168–171. doi: 10.1109/tnsre.2006.875554
Davids, K. (1988). Ecological validity in understanding sport performance: some problems of definition. QUEST 40, 126–136.
De Oliveira, L. A. S., Imbiriba, L. A., Russo, M. M., Nogueira-Campos, A. A., Rodrigues, E. C., Pereira, M. G., et al. (2012). Preparing to grasp emotionally laden stimuli. PLoS One 7:45235. doi: 10.1371/journal.pone.0045235
Dean, P. J., Seiss, E., and Sterr, A. (2012). Motor planning in chronic upper-limb hemiparesis: evidence from movement-related potentials. PLoS One 7:e44558. doi: 10.1371/journal.pone.0044558
Deeke, L. (1996). Planning, preparation, execution, and imagery of volitional action. Cogn. Brain Res. 3, 59–64.
Di Russo, F., Pitzalis, S., Aprile, T., and Spinelli, D. (2005). Effect of practice on brain activity: an investigation in top-level rifle shooters. Med. Sci. Sports Exerc. 37, 1586–1593. doi: 10.1249/01.mss.0000177458.71676.0d
Do Nascimento, O. F., Nielsen, K. D., and Voigt, M. (2005). Influence of directional orientations during gait initiation and stepping on movement-related cortical potentials. Behav. Brain Res. 161, 141–154. doi: 10.1016/j.bbr.2005.02.031
Do Nascimento, O. F., Nielsen, K. D., and Voigt, M. (2006). Movement-related parameters modulate cortical activity during imaginary isometric plantar-flexions. Exp. Brain Res. 171, 78–90. doi: 10.1007/s00221-005-0247-z
Eilbeigi, E., and Setarehdan, S. K. (2018a). Detecting intention to execute the next movement while performing current movement from EEG using global optimal constrained ICA. Comput. Biol. Med. 99, 63–75. doi: 10.1016/j.compbiomed.2018.05.024
Eilbeigi, E., and Setarehdan, S. K. (2018b). Global optimal constrained ICA and its application in extraction of movement related cortical potentials from single-trial EEG signals. Comput. Methods Prog. Biomed. 166, 155–169. doi: 10.1016/j.cmpb.2018.07.013
Euler, M. J., Niermeyer, M. A., and Suchy, Y. (2016). Neurocognitive and neurophysiological correlates of motor planning during familiar and novel contexts. Neuropsychology 30, 109–119. doi: 10.1037/neu0000219
Fang, Y., Yue, G. H., Hrovat, K., Sahgal, V., and Daly, J. J. (2007). Abnormal cognitive planning and movement smoothness control for a complex shoulder/elbow motor task in stroke survivors. J. Neurolog. Sci. 256, 21–29. doi: 10.1016/j.jns.2007.01.078
Fattapposta, F., D’agostino, V. C., My, F., Locuratolo, N., Vanacore, N., Inghilleri, M., et al. (2008). Psychophysiological aspects of voluntary skilled movement after stroke: a follow-up study. Arch. Ital. Biol. 146, 147–163.
Fearon, C., Butler, J. S., Waechter, S. M., Killane, I., Kelly, S. P., Reilly, R. B., et al. (2021). Neurophysiological correlates of dual tasking in people with Parkinson’s disease and freezing of gait. Exp. Brain Res. 239, 175–187. doi: 10.1007/s00221-020-05968-8
Fritz, N. E., Cheek, F. M., and Nichols-Larsen, D. S. (2015). motor-cognitive dual-task training in persons with neurologic disorders: a systematic review. J. Neurol. Phys. Ther. 39, 142–153. doi: 10.1097/NPT.0000000000000090
Fromer, R., Hafner, V., and Sommer, W. (2012). Aiming for the bull’s eye: Preparing for throwing investigated with event-related brain potentials. Psychophysiology 49, 335–344. doi: 10.1111/j.1469-8986.2011.01317.x
Fromer, R., Sturmer, B., and Sommer, W. (2016). (Don’t) Mind the effort: Effects of contextual interference on ERP indicators of motor preparation. Psychophysiology 53, 1577–1586. doi: 10.1111/psyp.12703
Glanzmann, P., and Froehlich, W. D. (1984). Anxiety. Stress, and Contingent Negative Variation Reconsidered. Ann. N. Y. Acad. Sci. 425, 578–584.
Golob, E. J., Ovasapyan, V., and Starr, A. (2005). Event-related potentials accompanying motor preparation and stimulus expectancy in the young, young-old and oldest-old. Neurobiol. Aging. 26, 531–542. doi: 10.1016/j.neurobiolaging.2004.04.002
Hatta, A., Nishihira, Y., Higashiura, T., Kim, S. R., and Kaneda, T. (2009). Long-term motor practice induces practice-dependent modulation of movement-related cortical potentials (MRCP) preceding a self-paced non-dominant handgrip movement in kendo players. Neurosci. Lett. 459, 105–108. doi: 10.1016/j.neulet.2009.05.005
Holmes, G. L., and Khazipov, R. (2007). “Basic neurophysiology and the cortical basis of EEG,” in The Clinical Neurophysiology Primer, eds A. S. Blum and S. B. Rutkove (New Jersey: Humana Press).
Hosp, J. A., and Luft, A. R. (2011). Cortical plasticity during motor learning and recovery after ischemic stroke. Neural. Plast. 2011:871296. doi: 10.1155/2011/871296
Ikeda, A., and Shibasaki, H. (2003). “Generator mechanisms of bereitschaftpotentials as studied by epicortical recordings in patients with intractable partial epilepsy,” in The Bereitschaftspotential. Movement-Related Cortical Potentials, eds M. Jahanshahi and M. Hallett (New York, NY: Springer).
Jacobs, J. V., Yaguchi, C., Kaida, C., Irei, M., Naka, M., Henry, S. M., et al. (2011). Effects of experimentally induced low back pain on the sit-to-stand movement and electroencephalographic contingent negative variation. Exp. Brain Res. 215, 123–134. doi: 10.1007/s00221-011-2880-z
Jahanshahi, M., and Hallett, M. (2003). The Bereitschaftspotential. Movement-Related Cortical Potentials. New York, NY: Springer Science and Business Media.
Jeong, J. H., Lee, M. H., Kwak, N. S., Lee, S. W., and IEEE (2017). “Single-Trial Analysis of Readiness Potentials for Lower Limb Exoskeleton Control,” in Proceedings of the 2017 5th International Winter Conference on Brain-Computer Interface, (New York, NY) 50–52.
Jiang, N., Gizzi, L., Mrachacz-Kersting, N., Dremstrup, K., and Farina, D. (2015). A brain-computer interface for single-trial detection of gait initiation from movement related cortical potentials. Clin. Neurophys. 126, 154–159. doi: 10.1016/j.clinph.2014.05.003
Jochumsen, M., Khan Niazi, I., Samran Navid, M., Nabeel Anwar, M., Farina, D., and Dremstrup, K. (2015a). Online multi-class brain-computer interface for detection and classification of lower limb movement intentions and kinetics for stroke rehabilitation. Brain-Comput. Interf. 2, 202–210. doi: 10.1080/2326263x.2015.1114978
Jochumsen, M., Knoche, H., Kjaer, T. W., Dinesen, B., and Kidmose, P. (2020a). EEG headset evaluation for detection of single-trial movement intention for brain-computer interfaces. Sensors 20:2804. doi: 10.3390/s20102804
Jochumsen, M., and Niazi, I. K. (2020b). Detection and classification of single-trial movement-related cortical potentials associated with functional lower limb movements. J. Neural. Eng. 17:035009. doi: 10.1088/1741-2552/ab9a99
Jochumsen, M., Niazi, I. K., Mrachacz-Kersting, N., Farina, D., and Dremstrup, K. (2013). Detection and classification of movement-related cortical potentials associated with task force and speed. J. Neural. Eng. 10:056015. doi: 10.1088/1741-2560/10/5/056015
Jochumsen, M., Niazi, I. K., Signal, N., Nedergaard, R. W., Holt, K., Haavik, H., et al. (2016). Pairing voluntary movement and muscle-located electrical stimulation increases cortical excitability. Front. Hum. Neurosci. 10:482. doi: 10.3389/fnhum.2016.00482
Jochumsen, M., Rovsing, C., Rovsing, H., Cremoux, S., Signal, N., Allen, K., et al. (2017). Quantification of movement-related EEG correlates associated with motor training: A study on movement-related cortical potentials and sensorimotor rhythms. Front. Hum. Neurosci. 11:604. doi: 10.3389/fnhum.2017.00604
Jochumsen, M., Signal, N., Nedergaard, R. W., Taylor, D., Haavik, H., and Niazi, I. K. (2015b). Induction of long-term depression-like plasticity by pairings of motor imagination and peripheral electrical stimulation. Front. Hum. Neurosci. 9:644. doi: 10.3389/fnhum.2015.00644
Jung, R. (1982). Postural support of goal-directed movements: the preparation and guidance of voluntary action in man. Acta Biolog. Acad. Sci. Hung. 33, 201–213.
Karimi, F., and Jiang, N. (2019). A reference-based source extraction algorithm to extract movement related cortical potentials for brain-computer interface applications. New York, NY: Institute of Electrical and Electronics Engineers Inc. 3603–3607.
Khaliliardali, Z., Chavarriaga, R., Andrei Gheorghe, L., and Millán, J. D. R. (2012). Detection of anticipatory brain potentials during car driving. Conference proceedings Annual International Conference of the IEEE Engineering in Medicine and Biology Society. IEEE Eng. Med. Biol. Soc. Ann. Conf. 2012, 3829–3832. doi: 10.1109/EMBC.2012.6346802
Khaliliardali, Z., Chavarriaga, R., Gheorghe, L. A., and Millán, J. D. R. (2015). Action prediction based on anticipatory brain potentials during simulated driving. J. Neural Eng. 12:066006. doi: 10.1088/1741-2560/12/6/066006
Khanmohammadi, R., Talebian, S., Hadian, M. R., Olyaei, G., and Bagheri, H. (2015). Preparatory postural adjustments during gait initiation in healthy younger and older adults: Neurophysiological and biomechanical aspects. Brain Res. 1629, 240–249. doi: 10.1016/j.brainres.2015.09.039
Kirschstein, T., and Kohling, R. (2009). What is the source of the EEG? Clin. EEG Neurosci. 40, 146–149.
Kita, Y., Mori, A., and Nara, M. (2001). Two types of movement-related cortical potentials preceding wrist extension in humans. Neuroreport 12, 2221–2225.
Klass, M., Baudry, S., and Duchateau, J. (2008). Age-related decline in rate of torque development is accompanied by lower maximal motor unit discharge frequency during fast contractions. J. Appl. Physiol. 104, 739–746. doi: 10.1152/japplphysiol.00550.2007
Kleim, J. A., and Jones, T. A. (2008). Principles of experience-dependent neural plasticity: Implications for rehabilitation after brain damage. J. Speech Lang. Hear. Res. 51, S225–S239. doi: 10.1044/1092-4388(2008/018)
Kline, J. E., Huang, H. J., Snyder, K. L., and Ferris, D. P. (2015). Isolating gait-related movement artifacts in electroencephalography during human walking. J. Neural. Eng. 12:046022. doi: 10.1088/1741-2560/12/4/046022
Knaepen, K., Mierau, A., Fernandez Tellez, H., Lefeber, D., and Meeusen, R. (2015). Temporal and spatial organization of gait-related electrocortical potentials. Neurosci. Lett. 599, 75–80. doi: 10.1016/j.neulet.2015.05.036
Knott, J. R., and Irwin, D. A. (1973). Anxiety. Stress, and the contingent negative variation. Arch. Gen. Psychiatry 29, 538–541. doi: 10.1001/archpsyc.1973.04200040080013
Koester, D., and Schack, T. (2016). Action priority: early neurophysiological interaction of conceptual and motor representations. PLoS One 11:165882. doi: 10.1371/journal.pone.0165882
Kopp, B., Kunkel, A., Mühlnickel, W., Villringer, K., Taub, E., and Flor, H. (1999). Plasticity in the motor system related to therapy-induced improvement of movement after stroke. NeuroReport 10, 807–810. doi: 10.1097/00001756-199903170-00026
Kornhuber, H. H., and Deecke, L. (1964). Changes in human brain potentials before and after voluntary movement, displayed by magnetic tape storage and backward analysis. Pflungers Archiv. Fur Die Gesamte Physiologie des Menschen und der Tiere 281:52.
Kornhuber, H. H., and Deecke, L. (1965). Changes in the brain potential in voluntary movements and passive movements in man: Readiness potential and reafferent potentials. Pflugers Arch. Gesamte Physiol. Menschen. Tiere 284, 1–17.
Kourtis, D., Sebanz, N., and Knoblich, G. (2013). Predictive representation of other people’s actions in joint action planning: An EEG study. Soc. Neurosci. 8, 31–42. doi: 10.1080/17470919.2012.694823
Kristensen, S. R., Niazi, I. K., Jochumsen, M., Jiang, N., Farina, D., and Mrachacz-Kersting, N. (2013). “Changes in corticospinal excitability following the use of a BCI based protocol combined with sham visual feedback,” in Converging Clinical and Engineering Research on Neurorehabilitation, Biosystems & Biorobotics, eds J. L. Pons, D. Torricelli, and M. Pajaro (Berlin: Springer).
Langhorne, P., Bernhardt, J., and Kwakkel, G. (2011). Stroke rehabilitation. Lancet 377, 1693–1702. doi: 10.1016/s0140-6736(11)60325-5
Langhorne, P., Coupar, F., and Pollock, A. (2009). Motor recovery after stroke: a systematic review. Lancet Neurol. 8, 741–754. doi: 10.1016/S1474-4422(09)70150-4
Loetscher, T., Potter, K. J., Wong, D., and Das Nair, R. (2019). Cognitive rehabilitation for attention deficits following stroke. Cochrane Database Syst. Rev. 11, 1465–1858. doi: 10.1002/14651858.CD002842.pub3
Lopez-Larraz, E., Trincado-Alonso, F., Rajasekaran, V., Perez-Nombela, S., Del-Ama, A. J., Aranda, J., et al. (2016). Control of an Ambulatory Exoskeleton with a Brain-Machine Interface for Spinal Cord Injury Gait Rehabilitation. Front. Neurosci. 10:359. doi: 10.3389/fnins.2016.00359
Mackinnon, C. D. (2003). “Recordings of movement-related potentials combined with PET, MRI or MEG,” in The Bereitschaftspotential. Movement-Related Cortical Potentials, eds M. Jahanshahi and M. Hallett (New York, NY: Springer Science and Business Media).
Mann, D. T. Y., Coombes, S. A., Mousseau, M. B., and Janelle, C. M. (2011). Quiet eye and the Bereitschaftspotential: visuomotor mechanisms of expert motor performance. Cogn. Proc. 12, 223–234. doi: 10.1007/s10339-011-0398-8
Martinez-Exposito, A., Ibanez, J., Resquin, F., and Pons, J. L. (2017). “Task Influence on Motor-Related Cortical Signals: Comparison Between Upper and Lower Limb Coordinated and Analytic Movements,” in Converging Clinical and Engineering Research on Neurorehabilitation Ii, Vols 1 and 2, eds J. Ibanez, J. Gonzalezvargas, J. M. Azorin, M. Akay, and J. L. Pons (Berlin: Springer) 1139–1143.
Michalewski, H. J., Thompson, L. W., Smith, D. B., Patterson, J. V., Bowman, T. E., Litzelman, D., et al. (1980). Age Differences in the Contingent Negative Variation (CNV): Reduced Frontal Activity in the Elderly. J. Gerontol. 35, 542–549.
Millan, J. D., Rupp, R., Muller-Putz, G. R., Murray-Smith, R., Giugliemma, C., Tangermann, M., et al. (2010). Combining Brain-Computer Interfaces and Assistive Technologies: State-of-the-Art and Challenges. Front. Neurosci. 4:161. doi: 10.3389/fnins.2010.00161
Mizusaki, Y., Ikudome, S., Ishii, Y., Unenaka, S., Funo, T., Takeuchi, T., et al. (2019). Why does the Quiet Eye improve aiming accuracy? Testing a motor preparation hypothesis with brain potential. Cogn. Proc. 20, 55–64. doi: 10.1007/s10339-018-0890-5
Moinnereau, M. A., Karimian-Azari, S., Sakuma, T., Boutani, H., Gheorghe, L., and Falk, T. H. (2019). EEG Artifact Removal for Improved Automated Lane Change Detection while Driving. New York, NY: Institute of Electrical and Electronics Engineers Inc, 1076–1080.
Monge-Pereira, E., Molina-Rueda, F., Rivas-Montero, F. M., Ibanez, J., Serrano, J. I., Alguacil-Diego, I. M., et al. (2017). Electroencephalography as a post-stroke assessment method: An updated review. Neurologia 32, 40–49. doi: 10.1016/j.nrl.2014.07.002
Mrachacz-Kersting, N., and Aliakbaryhosseinabadi, S. (2018). Comparison of the efficacy of a real-time and offline associative brain-computer-interface. Front. Neurosci. 12:455. doi: 10.3389/fnins.2018.00455
Mrachacz-Kersting, N., Stevenson, A. J. T., Jorgensen, H. R. M., Severinsen, K. E., Aliakbaryhosseinabadi, S., Jiang, N., et al. (2019b). Brain state-dependent stimulation boosts functional recovery following stroke. Ann. Neurol. 85, 84–95. doi: 10.1002/ana.25375
Mrachacz-Kersting, N., Dosen, S., Aliakbaryhosseinabadi, S., Pereira, E. M., Stevenson, A. J. T., Jiang, N., et al. (2019a). Brain-State Dependent Peripheral Nerve Stimulation for Plasticity Induction Targeting Upper-Limb in Biosystems and Biorobotics. Berlin: Springer International Publishing.
Mrachacz-Kersting, N., Jiang, N., Stevenson, A. J. T., Niazi, I. K., Kostic, V., Pavlovic, A., et al. (2016). Efficient neuroplasticity induction in chronic stroke patients by an associative brain-computer interface. J. Neurophysiol. 115, 1410–1421. doi: 10.1152/jn.00918.2015
Mrachacz-Kersting, N., Kristensen, S. R., Niazi, I. K., and Farina, D. (2012). Precise temporal association between cortical potentials evoked by motor imagination and afference induces cortical plasticity. J. Physiol. 590, 1669–1682. doi: 10.1113/jphysiol.2011.222851
Müller-Putz, G. R., Ofner, P., Pereira, J., Pinegger, A., Schwarz, A., Zube, M., et al. (2019). Applying intuitive EEG-controlled grasp neuroprostheses in individuals with spinal cord injury: Preliminary results from the MoreGrasp clinical feasibility study. in Proceedings of the 41st Annual International Conference of the IEEE Engineering in Medicine and Biology Society (EMBC) (New York, NY:IEEE) 5949–5955. doi: 10.1109/EMBC.2019.8856491
Munn, Z., Peters, M. D. J., Stern, C., Tufanaru, C., Mcarthur, A., and Aromataris, E. (2018). Systematic review or scoping review? Guidance for authors when choosing between a systematic or scoping review approach. BMC Med. Res. Methodol. 18:143. doi: 10.1186/s12874-018-0611-x
Nann, M., Cohen, L. G., Deecke, L., and Soekadar, S. R. (2019). To jump or not to jump - The Bereitschaftspotential required to jump into 192-meter abyss. Sci. Rep. 9:38447. doi: 10.1038/s41598-018-38447-w
Niazi, I. K., Mrachacz-Kersting, N., Jiang, N., Demstrup, K., and Farina, D. (2012). Peripheral electrical stimulation triggered by self-paced detection of motor intention enhances motor evoked potentials. IEEE Trans. Neural. Syst. Rehabil. Eng. 20, 595–604. doi: 10.1109/TNSRE.2012.2194309
O’connor, K. (1986). Motor potentials and motor performance associated with introverted and extraverted smokers. Neuropsychobiology 16, 109–116.
Ofner, P., Schwarz, A., Pereira, J., Wyss, D., Wildburger, R., and Muller-Putz, G. R. (2019). Attempted Arm and Hand Movements can be Decoded from Low-Frequency EEG from Persons with Spinal Cord Injury. Sci. Rep. 9:43594. doi: 10.1038/s41598-019-43594-9
Oliveira, A. S., Schlink, B. R., Hairston, W. D., Konig, P., and Ferris, D. P. (2016). Induction and separation of motion artifacts in EEG data using a mobile phantom head device. J. Neural. Eng. 13:036014. doi: 10.1088/1741-2560/13/3/036014
Olsen, S., Signal, N., Niazi, I. K., Christensen, T., Jochumsen, M., and Taylor, D. (2018). Paired Associative Stimulation Delivered by Pairing Movement-Related Cortical Potentials With Peripheral Electrical Stimulation: An Investigation of the Duration of Neuromodulatory Effects. Neuromodulation 21, 362–367. doi: 10.1111/ner.12616
Olsen, S., Signal, N., Niazi, I. K., Rashid, U., Alder, G., Mawston, G., et al. (2020). Peripheral Electrical Stimulation Paired with Movement-Related Cortical Potentials Improves Isometric Muscle Strength and Voluntary Activation following Stroke. Front. Hum. Neurosci. 14:156. doi: 10.3389/fnhum.2020.00156
Perri, R. L., Berchicci, M., Lucci, G., Cimmino, R. L., Bello, A., and Di Russo, F. (2014). Getting ready for an emotion: specific premotor brain activities for self-administered emotional pictures. Front. Behav. Neurosci. 8:197. doi: 10.3389/fnbeh.2014.00197
Peters, S., Ivanova, T. D., Lakhani, B., Boyd, L. A., and Garland, S. J. (2020). Neuroplasticity of cortical planning for initiating stepping poststroke: a case series. J. Neurol. Phys. Ther. 44, 164–172. doi: 10.1097/NPT.0000000000000311
Peters, S., Ivanova, T. D., Lakhani, B., Boyd, L. A., Staines, W. R., Handy, T. C., et al. (2018). Symmetry of cortical planning for initiating stepping in sub-acute stroke. Clin. Neurophys. 129, 787–796. doi: 10.1016/j.clinph.2018.01.018
Pollock, A., Farmer, S. E., Brady, M. C., Langhorne, P., Mead, G. E., Mehrholz, J., et al. (2014). Interventions for improving upper limb function after stroke. Cochrane Database Syst. Rev. 2014:CD010820. doi: 10.1002/14651858.CD010820.pub2
Rabinowitz, A. R., and Levin, H. S. (2014). Cognitive sequelae of traumatic brain injury. Psychiatr. Clin. North Am. 37, 1–11. doi: 10.1016/j.psc.2013.11.004
Rashid, U., Niazi, I. K., Signal, N., and Taylor, D. (2018). An EEG experimental study evaluating the performance of texas instruments ADS1299. Sensors 18:18113721. doi: 10.3390/s18113721
Reiser, J. E., Wascher, E., Rinkenauer, G., and Arnau, S. (2020). Cognitive-motor interference in the wild: assessing the effects of movement complexity on task switching using mobile EEG. Eur. J. Neurosci. 2020:14959. doi: 10.1111/ejn.14959
Rektor, I. (2003). “Intracerebal recordings of the bereitschaftspotential and related potentials in cortical and subcortical structures in human subjects,” in The Bereitschaftspotential. Movement-Related Cortical Potentials, eds M. Jahanshahi and M. Hallett (New York, NY: Springer Science and Business Media).
Richer, N., Downey, R. J., Hairston, W. D., Ferris, D. P., and Nordin, A. D. (2020). Motion and muscle artifact removal validation using an electrical head phantom, robotic motion platform, and dual layer mobile EEG. IEEE Trans. Neural. Syst. Rehabil. Eng. 28, 1825–1835. doi: 10.1109/TNSRE.2020.3000971
Russo, Y., Berchicci, M., Di Russo, F., and Vannozzi, G. (2019). How do different movement references influence ERP related to gait initiation? A comparative methods’ assessment. J. Neurosci. Methods 311, 95–101. doi: 10.1016/j.jneumeth.2018.10.006
Sburlea, A. I., Montesano, L., Cano-De La Cuerda, R., Alguacil Diego, I. M., Miangolarra-Page, J. C., and Minguez, J. (2015a). Detecting intention to walk in stroke patients from pre-movement EEG correlates. J. NeuroEng Rehabili. 12:87. doi: 10.1186/s12984-015-0087-4
Sburlea, A. I., Montesano, L., and Minguez, J. (2015b). Continuous detection of the self-initiated walking pre-movement state from EEG correlates without session-to-session recalibration. J. Neur. Eng. 12:36007. doi: 10.1088/1741-2560/12/3/036007
Sburlea, A. I., Montesano, L., and Minguez, J. (2017). Advantages of EEG phase patterns for the detection of gait intention in healthy and stroke subjects. J. Neur. Eng. 14:aa5f2f. doi: 10.1088/1741-2552/aa5f2f
Schwarz, A., Escolano, C., Montesano, L., and Muller-Putz, G. R. (2020a). Analyzing and decoding natural reach-and-grasp actions using gel, water and dry EEG Systems. Front. Neurosci. 14:849. doi: 10.3389/fnins.2020.00849
Schwarz, A., Ofner, P., Pereira, J., Sburlea, A. I., and Muller-Putz, G. R. (2018). Decoding natural reach-and-grasp actions from human EEG. J. Neural. Eng. 15:aa8911. doi: 10.1088/1741-2552/aa8911
Schwarz, A., Pereira, J., Kobler, R., and Muller-Putz, G. R. (2020b). Unimanual and Bimanual Reach-and-Grasp Actions Can Be Decoded From Human EEG. IEEE Transac. Bio-Med. Eng. 67, 1684–1695. doi: 10.1109/TBME.2019.2942974
Shakeel, A., Navid, M. S., Anwar, M. N., Mazhar, S., Jochumsen, M., and Niazi, I. K. (2015). A review of techniques for detection of movement intention using movement-related cortical potentials. Comput. Mathem. Methods Med. 2015:346217. doi: 10.1155/2015/346217
Shibasaki, H., Barrett, G., Halliday, E., and Halliday, A. M. (1980). Components of the movement-related cortical potential and their scalp topography. Electro. Clin. Neurophys. 49, 213–226. doi: 10.1016/0013-4694(80)90216-3
Shibasaki, H., and Hallett, M. (2006). What is the Bereitschaftspotential? Clin. Neurophysiol. 117, 2341–2356. doi: 10.1016/j.clinph.2006.04.025
Singh, B., Wagatsuma, H., and Natsume, K. (2016). The bereitschaftspotential for rise of stand-up towards robot-assist device preparation. New York, NY: Institute of Electrical and Electronics Engineers Inc, 998–1003.
Skrzeba, C., and Vogt, T. (2018). A cross-educational approach on skill-related movement technique performance: Central neuronal motor behaviour preceding the short badminton backhand serve. Neurosci. Lett. 686, 155–160. doi: 10.1016/j.neulet.2018.09.005
Stinear, C. M., Petoe, M. A., and Byblow, W. D. (2015). Primary motor cortex excitability during recovery after stroke: implications for neuromodulation. Brain Stimul. 2015, 15. doi: 10.1016/j.brs.2015.06.015
Tarkka, I. M., Kononen, M., Pitkanen, K., Sivenius, J., and Mervaala, E. (2008). Alterations in cortical excitability in chronic stroke after constraint-induced movement therapy. Neurolog. Res. 30, 504–510. doi: 10.1179/016164107x252519
Tomyta, K., and Seki, Y. (2020). Effects of motor style on timing control and EEG waveforms in self-paced and synchronization tapping tasks. Neurosci. Lett. 739:135410. doi: 10.1016/j.neulet.2020.135410
Trincado-Alonso, F., Lopez-Larraz, E., Resquin, F., Ardanza, A., Perez-Nombela, S., Pons, J. L., et al. (2018). A Pilot Study of Brain-Triggered Electrical Stimulation with Visual Feedback in Patients with Incomplete Spinal Cord Injury. J. Med. Biolog. Eng. 38, 790–803. doi: 10.1007/s40846-017-0343-0
Varghese, J. P., Merino, D. M., Beyer, K. B., and Mcilroy, W. E. (2016). Cortical control of anticipatory postural adjustments prior to stepping. Neuroscience 313, 99–109. doi: 10.1016/j.neuroscience.2015.11.032
Vidailhet, M., Atchison, P. R., Stocchi, F., Thompson, P. D., Rothwell, J. C., and Marsden, C. D. (1995). The Bereitschaftspotential preceding stepping in patients with isolated gait ignition failure. Movement Dis. 10, 18–21. doi: 10.1002/mds.870100105
Vidailhet, M., Stocchi, F., Rothwell, J. C., Thompson, P. D., Day, B. L., Brooks, D. J., et al. (1993). The bereitschaftspotential preceding simple foot movement and initiation of gait in Parkinson’s disease. Neurology 43, 1784–1788.
Vogt, T., Kato, K., Schneider, S., Türk, S., and Kanosue, K. (2017). Central neuronal motor behaviour in skilled and less skilled novices – Approaching sports-specific movement techniques. Hum. Movement Sci. 52, 151–159. doi: 10.1016/j.humov.2017.02.003
Volkova, K., Lebedev, M. A., Kaplan, A., and Ossadtchi, A. (2019). Decoding Movement From Electrocorticographic activity: a review. Front. Neuroinform. 13:74. doi: 10.3389/fninf.2019.00074
Welke, S., Protzak, J., Rotting, M., and Jurgensohn, T. (2011). “What Is Human? How the Analysis of Brain Dynamics Can Help to Improve and Validate Driver Models,” in Digital Human Modeling, ed. V. G. Duffy (New York, NY: Springer) 513–522.
Wiese, H., Stude, P., Sarge, R., Nebel, K., Diener, H. C., and Keidel, M. (2005). Reorganization of motor execution rather than preparation in poststroke hemiparesis. Stroke 36, 1474–1479. doi: 10.1161/01.STR.0000170639.26891.30
Wolpaw, J. R., Birbaumer, N., Mcfarland, D. J., Pfurtscheller, G., and Vaughan, T. M. (2002). Brain–computer interfaces for communication and control. Clin. Neurophysiol. 113, 767–791.
World Health Organisation. (2001). International Classification of Functioning, Disability and Health Browser. Geneva: WHO.
Wright, D. J., Holmes, P., Di Russo, F., Loporto, M., and Smith, D. (2012a). Reduced motor cortex activity during movement preparation following a period of motor skill practice. PLoS One 7:51886. doi: 10.1371/journal.pone.0051886
Wright, D. J., Holmes, P. S., Di Russo, F., Loporto, M., and Smith, D. (2012b). Differences in cortical activity related to motor planning between experienced guitarists and non-musicians during guitar playing. Hum. Movement Sci. 31, 567–577. doi: 10.1016/j.humov.2011.07.001
Wright, D. J., Holmes, P. S., and Smith, D. (2011). Using the movement-related cortical potential to study motor skill learning. J. Motor Behav. 43, 193–201. doi: 10.1080/00222895.2011.557751
Xu, R., Dosen, S., Jiang, N., Yao, L., Farooq, A., Jochumsen, M., et al. (2019). Continuous 2D control via state-machine triggered by endogenous sensory discrimination and a fast brain switch. J. Neural. Eng. 16:056001. doi: 10.1088/1741-2552/ab20e5
Xu, R., Jiang, N., Mrachacz-Kersting, N., Lin, C., Asin Prieto, G., Moreno, J. C., et al. (2014). A closed-loop brain-computer interface triggering an active ankle-foot orthosis for inducing cortical neural plasticity. IEEE Trans. Biomed. Eng. 61, 2092–2101. doi: 10.1109/TBME.2014.2313867
Yazawa, S., Shibasaki, H., Ikeda, A., Terada, K., Nagamine, T., and Honda, M. (1997). Cortical mechanism underlying externally cued gait initiation studied by contingent negative variation. Electro. Motor Contr. Electroencep. Clin. Neurophysiol. 105, 390–399. doi: 10.1016/s0924-980x(97)00034-9
Yilmaz, O., Birbaumer, N., and Ramos-Murguialday, A. (2014). Movement related slow cortical potentials in severely paralyzed chronic stroke patients. Front. Hum. Neurosci. 8:1033. doi: 10.3389/fnhum.2014.01033
Keywords: movement related cortical potential (MRCP), electroencephalograph (EEG), ecological validity, review (article), rehabilitation, movement, bereitschaftspotential (BP), contingent negative variation (CNV)
Citation: Olsen S, Alder G, Williams M, Chambers S, Jochumsen M, Signal N, Rashid U, Niazi IK and Taylor D (2021) Electroencephalographic Recording of the Movement-Related Cortical Potential in Ecologically Valid Movements: A Scoping Review. Front. Neurosci. 15:721387. doi: 10.3389/fnins.2021.721387
Received: 07 June 2021; Accepted: 27 August 2021;
Published: 28 September 2021.
Edited by:
Lourdes De Martínez, Panamerican University, MexicoReviewed by:
Veeky Baths, Birla Institute of Technology and Science, IndiaGernot R. Müller-Putz, Graz University of Technology, Austria
Copyright © 2021 Olsen, Alder, Williams, Chambers, Jochumsen, Signal, Rashid, Niazi and Taylor. This is an open-access article distributed under the terms of the Creative Commons Attribution License (CC BY). The use, distribution or reproduction in other forums is permitted, provided the original author(s) and the copyright owner(s) are credited and that the original publication in this journal is cited, in accordance with accepted academic practice. No use, distribution or reproduction is permitted which does not comply with these terms.
*Correspondence: Sharon Olsen, sharon.olsen@aut.ac.nz