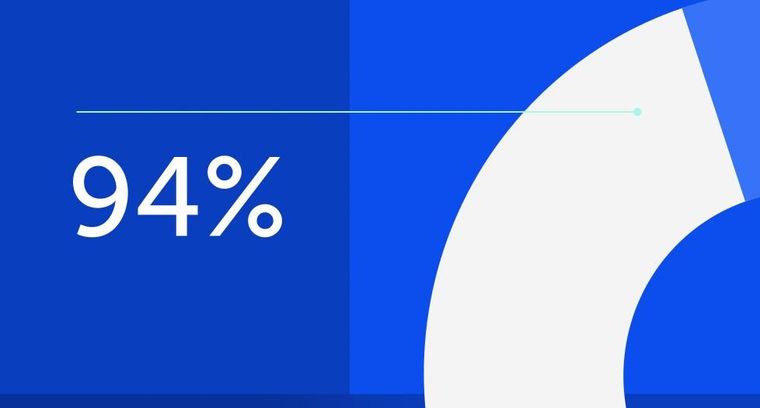
94% of researchers rate our articles as excellent or good
Learn more about the work of our research integrity team to safeguard the quality of each article we publish.
Find out more
BRIEF RESEARCH REPORT article
Front. Neurosci., 26 August 2021
Sec. Neurogenomics
Volume 15 - 2021 | https://doi.org/10.3389/fnins.2021.715048
This article is part of the Research TopicDecoding brain function through geneticsView all 11 articles
Borderline intellectual functioning (BIF) is a multifactorial condition in which both genetic and environmental factors are likely to contribute to the clinical outcome. Abnormal cortical development and lower IQ scores were shown to be correlated in BIF children, but the genetic components of this condition and their possible connection with intelligence and brain morphology have never been investigated in BIF. The synaptosomal-associated protein of 25 kD (SNAP-25) is involved in synaptic plasticity, neural maturation, and neurotransmission, affecting intellectual functioning. We investigated SNAP-25 polymorphisms in BIF and correlated such polymorphisms with intelligence and cortical thickness, using socioeconomic status and environmental stress as covariates as a good proxy of the variables that determine intellectual abilities. Thirty-three children with a diagnosis of BIF were enrolled in the study. SNAP-25 polymorphisms rs363050, rs363039, rs363043, rs3746544, and rs1051312 were analyzed by genotyping; cortical thickness was studied by MRI; intelligence was measured using the WISC-III/IV subscales; environmental stressors playing a role in neuropsychiatric development were considered as covariate factors. Results showed that BIF children carrying the rs363043(T) minor allele represented by (CT + TT) genotypes were characterized by lower performance Perceptual Reasoning Index and lower full-scale IQ scores (p = 0.04) compared to those carrying the (CC) genotype. This association was correlated with a reduced thickness of the left inferior parietal cortex (direct effect = 0.44) and of the left supramarginal gyrus (direct effect = 0.56). These results suggest a link between SNAP-25 polymorphism and intelligence with the mediation role of brain morphological features in children with BIF.
Borderline intellectual functioning (BIF) is a condition characterized by a mental ability at the border between normal intellectual functioning and intellectual disability, with an Intellectual Quotient (IQ) within 1 and 2 standard deviations below the mean of the normal curve of the distribution of intelligence that impacts on adaptive abilities (Salvador-Carulla et al., 2013; Peltopuro et al., 2014). In primary school age, children with BIF are burdened with difficulties in school achievements due to learning difficulties in more than one executive functions domain, such as attention, concentration, planning, and inhibition of impulsive responses, as well as memory and motor skill limitations (Alloway, 2010; Vuijk et al., 2010; Salvador-Carulla et al., 2013; Peltopuro et al., 2014; Contena and Taddei, 2017). Furthermore, limitations in social skills, emotional competencies, and behavioral problems affect the social participation of these children (Baglio et al., 2016; Predescu et al., 2020). Children with BIF are thus at high risk of school failure and dropout (Fernell and Ek, 2010), and are more likely to develop psychiatric problems in adulthood (Douma et al., 2007; Emerson et al., 2010; Gigi et al., 2014; Hassiotis, 2015; Hassiotis et al., 2019). Potential risk factors for BIF include low weight at birth, low socioeconomic status, maltreatment, and high levels of maternal stress. However, the negative social condition does not explain all the BIF cases and their development across the life span.
Intelligence is one of the most heritable behavioral traits (Deary et al., 2009). Intelligence is nevertheless also a malleable entity under the influence of environmental conditions (Sauce and Matzel, 2018). As a logical consequence of this, intellectual disability, as well as the development of psychiatric disorders were suggested to be the result of an interaction between social environment and genetic background (Rizzi et al., 2012). Finally, a multifactorial and multigenic set may be responsible for BIF development (Ropers, 2008).
An important role in intelligence is likely played by the synaptosomal-associated protein of 25 kD (SNAP-25) gene, which is located on chromosome 20p12-p11.2, an area of previous suggestive linkage to intelligence (Posthuma et al., 2005). SNAP-25 protein takes part in the regulation of calcium-dependent synaptic vesicles exocytosis, ensuring the efficient release of neurotransmitters and the propagation of action potentials. The key role of SNAP-25 is to initiate exocytosis through the formation of a SNARE complex (Südhof, 2015). The SNARE complex is therefore involved in the processes of learning, locomotion, memory formation, and ultimately the normal functioning of the brain as a whole. SNAP-25 single nucleotide polymorphisms (SNPs) were associated with variation of performance IQ in non-clinical, population based samples (Gosso et al., 2006, 2008). Interestingly, polymorphisms in the SNAP-25 gene, as well as an altered expression of the SNAP-25 protein, are also associated with abnormal behavioral phenotype both in humans (Thompson et al., 2003; Guerini et al., 2011; Braida et al., 2015; Liu et al., 2017) and in animal models (Bruno et al., 2006; Gunn et al., 2011). Finally, evidence derived from multiple organisms suggested that SNAP-25 is involved in the process of axonal growth and synaptic plasticity (Martinez-Arca et al., 2001). Therefore, any variation of SNAP-25 protein expression may interfere with neural maturation and neurotransmission, affecting intellectual functioning.
Neural plasticity during development was investigated with neuroimaging techniques that evaluated longitudinal changes in cortical thickness, a parameter influenced by genetics, which modulates intelligence (Brans et al., 2010). Notably, a number of results show how differences in a distributed network that include frontal and parietal cortices predict individual profiles in intelligence (Jung and Haier, 2007). Earlier data from our group, in particular, showed the presence of significant differences in cortical volume in areas belonging to this network in children with BIF and the relationship of this difference with intelligence (Baglio et al., 2014).
The aim of our work was to examine the complex relation between SNAP-25 and cortical thickness in determining intelligence. To accomplish this aim, we selected four SNPs located in the intron region which were proved to be involved in both typical (Gosso et al., 2006, 2008) and atypical development (Barr et al., 2000; Guerini et al., 2011; Braida et al., 2015). We first identified the presence of possible correlations between the SNAP-25 rs363050, rs363039, rs363043, rs3746544, and rs1051312 genetic polymorphisms with brain area morphology, and IQ scale in 33 children with BIF. Next, we conducted a mediation analysis in which genetic polymorphisms, brain area morphology and IQ scale were modeled in a comprehensive fashion. An association of the SNAP-25 rs363043 polymorphism with PRI as well as with IQ scores was reported to be mediated by brain cortical thickness in the inferior parietal lobule.
Children were recruited from the Child and Adolescent Neuropsychiatry Unit of IRCCS Don Carlo Gnocchi Foundation and the ASST S. Paolo and S. Carlo Hospital; both in Milan, Italy. The sample included thirty-three children (6–11 years old) with BIF, i.e., a Full-Scale Intelligence Quotient (FSIQ) score in the borderline range, and clinical criteria, that attend primary mainstream school. The clinical evaluation consisted of a detailed medical history and social skills of the child and of his/her family and clinical observations reports.
Excluded criteria were: (1) ADHD, autism spectrum disorder, or other major neuropsychiatric disorders; (2) epilepsy, traumatic brain injury, brain malformation, infectious disease and other neurological conditions involving the central nervous system, and perinatal complications such as prematurity or other adverse events; (3) genetic syndromes such as Down syndrome or Fragile X syndrome and (4) systemic diseases such as diabetes or dysimmune disorders; and (5) current or past substance abuse (psychoactive drugs, psycho stimulants, neuroleptics, antidepressants, benzodiazepines, and antiepileptic drugs).
Informed consent was obtained from all parents/legal guardians prior to inclusion in the study. The study was conducted according to the guidelines of the Declaration of Helsinki and was approved by the institutional review board of the Don Carlo Gnocchi ONLUS Foundation, Milan (Protocol nr: 06_18-05-2016).
Socioeconomic status was assessed by the SES questionnaire, an integrated measure of parent’s education grade and occupation, widely used in research to identify the child/family social standing. The socio-cultural levels are low (range 8–19), middle-low (range 20–29), middle (range 30–39), middle-high (range 40–54) and high (range 55–66) (Hollingshead, 2011). Environmental stress was defined according to the ESCL, a list of V-codes from DSM-5, and Z-codes from ICD-10, to detect relational, neglect, physical, sexual and/or psychological abuse, educational and occupational, housing and economic, social exclusion or rejection problems, plus the presence of the following three conditions: social services intervention, major psychiatric diagnosis and/or substance abuse within the family members. The scoring is binary with a 0 (absence) or 1 (presence) attribution to each item, with a total score ranging from 0 to 24 (Blasi et al., 2020).
The neuropsychological evaluation included: (1) the WISC –III (Orsini and Picone, 2006), with the exception of nine children evaluated with the WISC-IV (Orsini et al., 2012) that assess the global intellectual functioning and the cognitive profile; (2) the Socioeconomic Status (SES) (Hollingshead, 2011), to evaluate the family education level and financial well-being; (3) the Environmental Stress Check List (ESCL), a tool to detect all possible sources of environmental stress (Blasi et al., 2020) to which the children were exposed to.
The WISC-III provides three principal scores: the FSIQ, the Verbal IQ (VIQ), and the Performance (PIQ); in addition, to better describe the cognitive profile, it is possible to calculate four indices: the Verbal Comprehension Index (VCI), the Perceptual Organization Index (POI), the Freedom from Distractibility Index (FDI) and the Processing Speed Index (PSI). The WISC–IV provides an FSIQ and a four-index framework similar to that of the WISC III: the VCI, the Perceptual Reasoning Index (PRI), the Working Memory Index (WMI), and the PSI. The increased emphasis on fluid reasoning abilities and on working memory, with the introduction of new subtests, has resulted in the renaming of the POI as the Perceptual Reasoning Index (PRI) and the FDI as the Working Memory Index (WMI) respectively. Moreover, a high correlation between FSIQ, VCI, and PRI of both versions is demonstrated (FSIQ-FSIQ = 0.89; VCI-VCI = 0.88; POI-PRI = 0.72) (Flanagan and Kaufman, 2012). Finally, all indices are expressed in the standard score (Mean = 100; SD = 15). For the present study, we will refer to both POI and PRI indices with the acronym PRI and to both FDI and WMI as WMI. All the data were age-corrected when measured.
All subjects underwent a magnetic resonance imaging (MRI) evaluation. MRI was performed on a 1.5 T Siemens Magnetom Avanto (Erlangen, Germany) scanner equipped with a 12-channels head coil. The acquisition included: (1) a 3D T1-weighted Magnetization Prepared Rapid Gradient-Echo (MPRAGE) image, (repetition time (TR)/echo time (TE) = 1900/3.37 ms, Filed of View (FoV) = 192 × 256 mm2, voxel size = 1 mm isotropic, 176 axial slices); (2) two conventional anatomical sequences (axial PD/T2 and coronal FLAIR) to exclude gross brain abnormalities.
The 3D-T1 images were segmented and parcellated using FreeSurfer version 5.31 into 68 cortical areas (34 for each hemisphere) according to the Desikan atlas (Desikan et al., 2006). Furthermore, the FreeSurfer automatic labeling process was used to extract seven subcortical regions per hemisphere (thalamus, caudate, putamen, pallidum, and nucleus accumbens, amygdala, and hippocampus) and the brain stem for a total of 82 parcels. The quality of recon-all parcelation was assessed in each subject according to ENIGMA guidelines2 for cortical and subcortical areas.
Mean thickness was then computed for each cortical area, and mean volume for each subcortical region.
Single nucleotide polymorphisms typing: Three SNAP-25 SNPs: rs363050, rs363039, and rs363043 located within intron 1, in a region of about 13.8 kb, known to affect transcription factor binding sites (Gosso et al., 2008) as well as two SNAP-25 SNPs: rs3746544 and rs1051312 located in the 3′ untranslated region predicted as a binding site of miRNAs (endogenous non-coding RNA regulators of gene activity at the post-transcriptional level) (Ambros, 2004; Bartel, 2004) were investigated; these SNPs have previously been associated with ADHD (Barr et al., 2000). Genomic DNA was isolated from peripheral blood mononuclear cells by phenol-chloroform extraction. SNPs were typed using the Taqman SNP Genotyping Assays (Applied Biosystems by Life Technologies, Foster City, CA, United States) on an ABI PRISM 7000 Sequence Detection System. For rs363039, rs363043, rs363050, and rs3746544, respectively, the C_327976_10, C_2488346_10, C_329097_10 and C_27494002_10 Human Pre-Designed Assays (Applied Biosystems by Life Technologies) were used. The restriction enzyme polymorphism rs1051312 was genotyped by DdeI digestion as previously described (Barr et al., 2000).
Age, socioeconomic status, environmental stress index, the total score, and the subscales of the Wechsler Intelligence Scale for Children were described by median and 5th to 95th centile range. SNAP-25 polymorphisms were described by frequencies (Table 1).
Table 1. Median, 5th–95th centiles of age, socioeconomic status, environmental stress and psychometric scores.
Exact Hardy Weinberg analysis was applied to verify if SNAP-25 SNPs genotype distribution among children with BIF were in Equilibrium (HWE).
SNAP-25 Linkage disequilibrium was calculated by SHEsis (Shi and He, 2005) by adding data from 615 healthy control from a previous study (Guerini et al., 2014). Haplotype correlation with IQ profile was calculated by regression analysis, adjusting by gender, SES, and ESCL, using PLINK software (Purcell et al., 2007).
A causal network approach was applied to investigate the association between SNAP-25 SNPs, MRI morphological data, and WISC-III-IV subscales (FSIQ, VCI, PRI, WMI, PSI) (Agler and De Boeck, 2017). Specifically, we considered a network in which SNAP-25 SNPs may act on psychometric scores through a direct relation and by an indirect pathway in which morphological data, measured by MRI, may act as a mediator. Firstly, to reduce skewness, all outcome data were standardized and transformed in normal ranks using Blom’s transformation (Gumbel, 1959). Afterward, models bearing the direct association (SNAP-25 to WISC III-IV subscale) and two indirect associations (SNAP-25 to brain morphology and brain morphology to WISC III-IV subscale), were applied to investigate the above causal pathway. Those models were based on a Kernel not parametric regression adjusted for sex, socioeconomic status, and Environmental Stress Check List score (ESCL). Regression coefficients were estimated and tested for significance by a procedure based on 5,000 bootstrap replications. To take into account numerous comparisons and to manage the related false discovery rate, the threshold P-value to detect significant associations was set according to the Benjamini–Hochberg procedure (Chen et al., 2017). Briefly, this method was chosen, instead of more conservative approaches, because of the small sample size and the need to reduce the false-negative rate (reduce the type-II error). Heat maps of statistically significant results were the graphical tool chosen to represent multiple associations between SNAP-25 SNPs, MRI morphological data, and psychometric scores.
Afterward, according to the above explorative analyses, a mediation analysis was used to investigate the role of brain morphology in the association between SNAP-25 SNPs and psychometric scores. In the mediation analyses, the direct and indirect effects were reported as standardized and rescaled regression coefficients. The total effect of SNAP-25 SNPs on psychometric scores was computed as a + b × c and as b × c as the total and indirect effects respectively. Here the term “a” was the slope of the direct effect, while the terms “b” and “c” were the slopes of the two sides of the indirect effects, the association between single nucleotide polymorphism with MRI data, and the association between MRI data and the psychometric scores, respectively. Standardized coefficients were reported for all the associations. All statistical tests were two-tailed and the type-I error rate was set according to the Benjamini–Hochberg procedure for the single Kernel non-parametric models while an ordinary type-I error rate of 5% (α = 0.05) was considered for the mediation analyses. The NP and the LAVAAN packages of the R software vers. 3.6. were used to conduct the kernel non-parametric regressions and the mediation analysis, respectively.
Given the small sample size, a priori and a posteriori power calculations were performed. In particular, the statistical power of Kernel non-parametric regression was investigated using the MultNonParam package of the R software vers. 3.6 while the statistical power of the mediation analysis was investigated using the pwr package of the R software vers. 3.6. According to this evaluation and considering the current sample size and type-I error rate used, medium to large standardized effect size in the range of 0.3–0.5, could be detected with a type-II error rate below 20% (Statistical power above 80%) for those two methods. According to statistical power, the current study could be defined as explorative.
The sample was composed of 19 boys and 14 girls with a median age of 9 years (5th to 95th centile 6–10 years old). Age, socioeconomic status, IQ evaluation scores, and SNAP-25 polymorphisms were similar in boys and girls; the ESCL score, on the other hand, was lower in girls compared to boys, although not significantly (p = 0.3964). All WISC-III-IV scores had a median lower than 85, below 1 standard deviation with respect to the normal population (Table 1).
Initial results showed that SNAP-25 rs363039, rs363043, rs363050, rs3746544, and rs1051312 SNPs genotype distribution was in HWE (p = 0.96; p = 0.05; p = 0.07; p = 0.71; p = 0.57 respectively) (Figure 1). The SNAP-25 genetic patterns of distribution in BIF children, clustered into carriers of the minor allele (i.e., heterozygous + homozygous for minor) and carriers of the homozygote major allele, were analyzed next in relationship with FSIQ, VCI, PRI, WMI, and PSI scores (Figure 1).
Figure 1. Violin plots of Kernel’s smoothed distributions of WISC-III/IV scores by SNAP-25 genotypes. The box plots present the distribution of each IQ score (median and interquartile range) relatively to the genotype distribution. The “violin” area indicate the percentage of children with BIF for each allele (in gray the minor allele and white the major allele).
Analysis of the relation between SNAP-25 polymorphisms and IQ scores showed the presence of a significant association between the rs363043 (T) minor allele represented by (CT + TT) genotypes and lower FSIQ and PRI scores (p = 0.04). Notably, additional results showed that the rs3746544 (TT) genotype was also significantly associated with reduced WMI scores (p = 0.04) (Figure 1).
Linkage haplotype analyses were used to evaluate the linkage disequilibrium between SNAP-25 variants, as well as to verify the presence of an association between the different haplotypes and FSIQ, VCI, PRI, WMI, and PSI scores. Haplotype analysis evidenced, the presence of a linkage disequilibrium (LD) between: (1) rs363050 and rs363039 (r2 > 0.3); (2) rs363043 and rs363050 (r2 = 0.24); and (3) rs363043 and rs363039 (r2 = 0.17) and a linkage between rs3746544 and rs105312 polymorphisms (r2 = 0.17). Haplotype distributions were not significantly associated with IQ scores (data not shown).
The relations between SNAP-25 polymorphisms and MRI morphological data, along with the relations between MRI morphological data from IQ evaluation were analyzed next. Results showed the presence of a number of associations between these features (Supplementary Figures 1, 2). In particular, this exploratory association identified the cortical thickness in the left inferior parietal cortex as a possible mediator between rs363043 and PRI scores. Moreover, the thickness of the left supramarginal gyrus was suggested to act as a possible mediator between rs363043 and FSIQ. Finally, when considering the relation between the PRI score with the left inferior parietal cortex a significant variance of 21% was observed, whereas the relation between FSIQ with the left supramarginal gyrus resulted in an explained variance of 24%.
According to our mediation analyses, the association of the rs363043 polymorphism influenced the PRI score with a direct effect of 0.44. This association was mediated by a cortical thickness of the left inferior parietal by 3.1%. This means that a lower score of PRI associated with rs363043 (CT + TT) genotypes is directly related to a reduced cortical thickness. Similarly, the association of the rs363043 polymorphism with the FSIQ score had a direct effect of 0.56 and a mediated indirect effect of the cortical thickness of the left supramarginal gyrus of 3.5%. This means that the lower FSIQ score associated with the rs363043 (CT + TT) genotypes is directly related to a reduced cortical thickness of the left supramarginal gyrus. Results of the mediation analyses are presented in Figure 2.
Figure 2. Causal mediation analyses of SNAP-25 rs363043 on PRI and FSIQ scores of the WISC-III/IV. The Left inferoparietal and supramarginal cortex thickness as the mediators for PRI and FSIQ, respectively. The Left inferior parietal and supramarginal cortex thickness were observed to be the mediators for PRI and FSIQ, respectively. The upper part of the figure shows the association of the rs363043 polymorphism with PRI score (direct effect = 0.44; contribution of the left inferior parietal = 3.1%). The lower part of the figure shows the association of the rs363043 polymorphism with FSIQ score (direct effect = 0.56; contribution of the left Supramarginal gyrus = 3.5%).
In the present study, we show that SNAP-25 rs363043(T) minor allele represented by (CT + TT) genotypes are associated with lower PRI scores in children with BIF; such association was found to be mediated by the left inferior parietal cortex thickness: lower thickness mediates lower PRI scores. Notably, the same children carrying the rs363043 (CT) or (TT) genotypes showed a lower full-scale IQ score, and this association was mediated by the cortical thickness in the left supramarginal gyrus.
The SNAP-25 SNP rs363043, along with other polymorphisms on the SNAP-25 gene, has previously been associated with intelligence in a normal population of Dutch children, adolescents, and adults (Gosso et al., 2006, 2008). In that case, though, the rs363043 (T) allele was associated with higher Verbal and performance IQ. Differences in the populations that have been analyzed, as well as in environmental components, might explain these discrepant results. Specifically, in the previous work (Gosso et al., 2008) the authors studied a population with an average IQ while we analyzed a group of BIF children belonging to a low socioeconomic status; importantly, the effect of several environmental stressors was considered as well in our analyses.
The interplay between genetic and environmental factors is complex and most likely both these factors have an important impact on individual differences in IQ (Sauce and Matzel, 2018). In the attempt to consider both aspects, we designed a model that includes the socioeconomic status and environmental stress as covariates; we believe this approach to be a good proxy of the diverse variables that determine intellectual abilities.
The SNAP-25 rs363043 polymorphism herein reported is a (non) coding variant within the intron 1 of the SNAP-25 gene that was shown to be involved in the regulation of SNAP-25 protein expression (Gosso et al., 2006). SNAP-25 protein is differentially expressed in the brain and is primarily present in the cerebral cortex, cerebellum, hippocampus, and caudate3. Notably, chronic reduction of SNAP-25 expression was shown to affect behavior in animal models. Thus, the coloboma mouse model, characterized by halved SNAP-25 levels (Hess et al., 1992), displays a hyperactive phenotype (Hess et al., 1992), associated with abnormal thalamic spike-wave discharges (Hess et al., 1995; Zhang et al., 2004; Faraone et al., 2005; Russell, 2007). Similarly, juvenile SNAP-25 heterozygous mice display moderate hyperactivity, which disappears in the adult animals, as well as impaired associative learning and memory, which persist in adulthood (Corradini et al., 2014).
Multiple studies have shown that different SNAP-25 SNPs are associated with related traits of autism (Guerini et al., 2011) and ADHD (Forero et al., 2009; Braida et al., 2015), as well as with working memory ability (Söderqvist et al., 2010; Gao et al., 2015), short-/long-term memory and visual attention (Golimbet et al., 2010), and intellectual disability (Rizzi et al., 2012), These observations can be explained by the fact that the markers studied here are located close to a locus linked with behavioral and cognitive functions. A genetic linkage disequilibrium effect, which would explain the involvement of different SNPs in the same SNAP-25 genetic locus cannot be excluded either in our cohort of BIF children.
In the attempt to find an explanation for our results, we investigated if SNAP-25 SNPs could influence differences in brain morphology, thus explaining the connection between genetics and IQ scores. Results showed the involvement of different areas in the left hemisphere. In particular, the left inferior parietal cortex and the left supramarginal gyrus were found to mediate between genetics and the PRI and the FSIQ respectively. The PRI is a measure of the non-verbal components of intelligence such as visuospatial and visuomotor abilities involved in the reasoning and solving of new problems, while FSIQ is a composite measure derived by all IQ scores relative to verbal and non-verbal abilities. Interestingly, we observed that the relationship between PRI and FSIQ and genetics was mediated by the inferior parietal cortex and the supramarginal gyrus, which are both parts of the inferior parietal lobule (IPL), a multimodal region, considered a hub for its great interconnectivity with several areas in the brain (Igelström and Graziano, 2017). Our results are in line with previous evidence showing how the IPL has a relevant role in multimodal information integration for higher-order cognitive functions such as abstraction and symbolization (Igelström and Graziano, 2017). Moreover, the IPL is part of the mirror neuron system (Rizzolatti and Craighero, 2004), which is involved in the visuomotor integration processing of gestures, relevant not only for action understanding but also for learning. In support of these data, previous results showed how multimodal rehabilitation interventions to improve the intellectual abilities of children with BIF were more effective than single domain treatment (Blasi et al., 2020). Notably, our results can be seen in the light of previous studies showing how the variation in the cortical thickness of a distributed network comprising the dorsolateral prefrontal cortex, the inferior and superior parietal lobule, the anterior cingulate, and regions within the temporal and occipital lobes, predicts individual differences in the g-factor of intelligence (Jung and Haier, 2007). Specifically, a positive correlation between intelligence and cortical thickness in the IPL has been demonstrated in both children and adults (Menary et al., 2013).
Results herein are in line with previous studies showing that individual differences in frontal and parietal cortical thickness are strongly influenced by genetic components (Posthuma et al., 2002). The interplay between genetic and environment is complex and most likely both these factors have an important impact on individual differences in IQ (Sauce and Matzel, 2018). In our study, all children belonged to high-risk environments, had a medium to low SES, and were undergoing the effect of environmental stressors, all factors indirectly associated with low thickness in the frontoparietal network (Rosen et al., 2018). Our data suggest that the genetic background interacts with environmental factors in shaping brain configuration, thus determining the outcome of BIF.
The current work has remarkable strengths. Firstly, we investigated determinants of intelligence in a sample of children with borderline intellectual functioning, a population of great clinical interest. Secondly, we adopted a rigorous research methodology resulting in reliable genetic polymorphisms, measures of intelligence, and brain morphology. Moreover, we used a comprehensive approach that included genetic, brain morphology, and intelligence outcomes as a whole, in an integrated analytical framework in which the issue of false discovery rate given by the limited sample size and large numbers of comparisons were taken into account using the appropriate statistical approaches. Finally, we would like to underline that this is the first study conducted in children with BIF that describes a multimodal association between SNAP-25 polymorphisms, intelligence, and brain morphological features.
As usually is the case, though, this work also has limitations. Firstly, results were drawn from analyses performed in a limited number of children which could result in false-negative results. Moreover, the lack of a deeper analysis of the SNAP-25 gene by next generation sequencing does not allow us to exclude that other polymorphisms could also be involved in shaping the intellectual functioning of children with BIF. Future studies evaluating the rs363043 polymorphism regulatory ability in larger cohorts of patients as well as an expression analysis of SNAP-25 gene would be necessary.
Further, the observational nature of the study, the reduced sample size, and the skewness of the outcomes considered led to the use of non-parametric multivariate-adjusted models; this might have reduced the statistical power of statistical analyses. The use of Benjamini–Hochberg procedure to adjust for multiple comparisons and the use of bootstrap, nevertheless likely took care of this issue. Finally, without a control group, our study could be defined just as an explorative attempt. More rigorous observational studies, with larger sample size and possibly based on a matched case-control design, should be performed to validate our results.
The data presented in this study are available on request from the corresponding author. The data are not publicly available due to privacy concerns.
The studies involving human participants were reviewed and approved by the Ethics Committees of the Don Gnocchi Foundation. Written informed consent to participate in this study was provided by the participants’ legal guardian/next of kin.
FG, VB, MaC, and EB: conceptualization. FG, VB, CR, and EB: methodology. EB, MilZ, GB, and MoC: formal analysis. FG, VB, FB, EB, CR, MoC, and GB: investigation. FG and VB: data curation, writing—original draft preparation, and project administration. MPC, MW, and MicZ: resources. FG, VB, CR, and MaC: writing—review and editing. FG, VB, MaC, and FB: funding acquisition. All authors have read and agreed to the published version of the manuscript.
This study was funded by the Ministry of Health (Ricerca Corrente). The funder did not have any influence in the design, implementation, analysis or interpretation of the data in this study.
The authors declare that the research was conducted in the absence of any commercial or financial relationships that could be construed as a potential conflict of interest.
All claims expressed in this article are solely those of the authors and do not necessarily represent those of their affiliated organizations, or those of the publisher, the editors and the reviewers. Any product that may be evaluated in this article, or claim that may be made by its manufacturer, is not guaranteed or endorsed by the publisher.
The Supplementary Material for this article can be found online at: https://www.frontiersin.org/articles/10.3389/fnins.2021.715048/full#supplementary-material
Supplementary Figure 1 | Association Heatmaps between SNAP-25 genotypes and morphological brain features. Dark areas represents significant associations according to the Benjamini–Hochberg procedure. “All statistical significances are in the range between 0.05 and 0.01.”
Supplementary Figure 2 | Association Heatmaps between WISC-III scores and morphological brain features. Dark areas represents significant associations according to the Benjamini–Hochberg procedure. “All statistical significances are in the range between 0.05 and 0.01.”
Agler, R., and De Boeck, P. (2017). On the interpretation and use of mediation: multiple perspectives on mediation analysis. Front. Psychol. 15:1984. doi: 10.3389/fpsyg.2017.01984
Alloway, T. P. (2010). Working memory and executive function profiles of individuals with borderline intellectual functioning. J. Intellect. Disabil. Res. 54, 448–456. doi: 10.1111/j.1365-2788.2010.01281.x
Baglio, F., Cabinio, M., Ricci, C., Baglio, G., Lipari, S., Griffanti, L., et al. (2014). Abnormal development of sensory-motor, visual temporal and parahippocampal cortex in children with learning disabilities and borderline intellectual functioning. Front. Hum. Neurosci. 8:806. doi: 10.3389/fnhum.2014.00806
Baglio, G., Blasi, V., Sangiuliano Intra, F., Castelli, I., Massaro, D., Baglio, F., et al. (2016). Social competence in children with borderline intellectual functioning: delayed development of theory of mind across all complexity levels. Front. Psychol. 7:1604. doi: 10.3389/fpsyg.2016.01604
Barr, C. L., Feng, Y., Wigg, K., Bloom, S., Roberts, W., Malone, M., et al. (2000). Identification of DNA variants in the SNAP-25 gene and linkage study of these polymorphisms and attention-deficit hyperactivity disorder. Mol. Psychiatry. 5, 405–409. doi: 10.1038/sj.mp.4000733
Bartel, D. P. (2004). MicroRNAs: genomics, biogenesis, mechanism, and function. Cell 116, 281–297. doi: 10.1016/s0092-8674(04)00045-5
Blasi, V., Pirastru, A., Cabinio, M., Di Tella, S., Laganà, M. M., Giangiacomo, A., et al. (2020). Early life adversities and borderline intellectual functioning negatively impact limbic system connectivity in childhood: a connectomics-based study. Front. Psychiatry. 11:497116. doi: 10.3389/fpsyt.2020.497116
Braida, D., Guerini, F. R., Ponzoni, L., Corradini, I., De Astis, S., Pattini, L., et al. (2015). Association between SNAP-25 gene polymorphisms and cognition in autism: functional consequences and potential therapeutic strategies. Transl. Psychiatry 5:e500. doi: 10.1038/tp.2014.136
Brans, R. G., Kahn, R. S., Schnack, H. G., van Baal, G. C., Posthuma, D., van Haren, N. E., et al. (2010). Brain plasticity and intellectual ability are influenced by shared genes. J. Neurosci. 30, 5519–5524. doi: 10.1523/JNEUROSCI.5841-09.2010
Bruno, K. J., Freet, C. S., Twining, R. C., Egami, K., Grigson, P. S., and Hess, E. J. (2006). Abnormal latent inhibition and impulsivity in coloboma mice, a model of ADHD. Neurobiol Dis. 25, 206–216. doi: 10.1016/j.nbd.2006.09.009
Chen, S. Y., Feng, Z., and Yi, X. (2017). A general introduction to adjustment for multiple comparisons. J. Thorac. Dis. 9, 1725–1729. doi: 10.21037/jtd.2017.05.34
Contena, B., and Taddei, S. (2017). Psychological and cognitive aspects of borderline intellectual functioning. Eur. Psychol. 22, 159–166. doi: 10.1027/1016-9040/a000293
Corradini, I., Donzelli, A., Antonucci, F., Welzl, H., Loos, M., Martucci, R., et al. (2014). Epileptiform activity and cognitive deficits in SNAP-25(±) mice are normalized by antiepileptic drugs. Cereb. Cortex. 24, 364–376. doi: 10.1093/cercor/bhs316
Deary, I. J., Johnson, W., and Houlihan, L. M. (2009). Genetic foundations of human intelligence. Hum. Genet. 126, 215–232. doi: 10.1007/s00439-009-0655-4
Desikan, R. S., Ségonne, F., Fischl, B., Quinn, B. T., Dickerson, B. C., Blacker, D., et al. (2006). An automated labeling system for subdividing the human cerebral cortex on MRI scans into gyral based regions of interest. Neuroimage 31, 968–980. doi: 10.1016/j.neuroimage.2006.01.021
Douma, J. C., Dekker, M. C., de Ruiter, K. P., Tick, N. T., and Koot, H. M. (2007). Antisocial and delinquent behaviors in youths with mild or borderline disabilities. Am. J. Ment. Retard. 112, 207–220. doi: 10.1352/0895-8017(2007)112[207:aadbiy]2.0.co;2
Emerson, E., Einfeld, S., and Stancliffe, R. J. (2010). The mental health of young children with intellectual disabilities or borderline intellectual functioning. Soc. Psychiatr. Psychiatr. Epidemiol. 45, 579–587. doi: 10.1007/s00127-009-0100-y
Faraone, S. V., Perlis, R. H., Doyle, A. E., Smoller, J. W., Goralnick, J. J., Holmgren, M. A., et al. (2005). Molecular genetics of attention-deficit/hyperactivity disorder. Biol. Psychiatry 57, 1313–1323. doi: 10.1016/j.biopsych.2004.11.024
Fernell, E., and Ek, U. (2010). Borderline intellectual functioning in children and adolescents – insufficiently recognized difficulties. Acta Paediatr. 99, 748–753. doi: 10.1111/j.1651-2227.2010.01707.x
Flanagan, D. P., and Kaufman, A. S. (2012). Fondamenti per l’assessment con la WISC-IV. Firenze: Giunti OS Organizzazioni speciali.
Forero, D. A., Arboleda, G. H., Vasquez, R., and Arboleda, H. (2009). Candidate genes involved in neural plasticity and the risk for attention-deficit hyperactivity disorder: a meta-analysis of 8 common variants. J. Psychiatry Neurosci. 34, 361–366.
Gao, Q., Liu, L., Chen, Y., Li, H., Yang, L., Wang, Y., et al. (2015). Synaptosome-related (SNARE) genes and their interactions contribute to the susceptibility and working memory of attention-deficit/hyperactivity disorder in males. Prog. Neuropsychopharmacol. Biol. Psychiatry 57, 132–139. doi: 10.1016/j.pnpbp.2014.11.001
Gigi, K., Werbeloff, N., Goldberg, S., Portuguese, S., Reichenberg, A., Fruchter, E., et al. (2014). Borderline intellectual functioning is associated with poor social functioning, increased rates of psychiatric diagnosis and drug use–a cross sectional population based study. Eur. Neuropsychopharmacol. 24, 1793–1797. doi: 10.1016/j.euroneuro.2014.07.016
Golimbet, V. E., Alfimova, M. V., Gritsenko, I. K., Lezheiko, T. V., Lavrushina, O. M., Abramova, L. I., et al. (2010). Association between a synaptosomal protein (SNAP-25) gene polymorphism and verbal memory and attention in patients with endogenous psychoses and mentally healthy subjects. Neurosci. Behav. Physiol. 40, 461–465. doi: 10.1007/s11055-010-9280-x
Gosso, M. F., de Geus, E. J., Polderman, T. J., Boomsma, D. I., Heutink, P., and Posthuma, D. (2008). Common variants underlying cognitive ability: further evidence for association between the SNAP-25 gene and cognition using a family-based study in two independent Dutch cohorts. Genes Brain Behav. 7, 355–364. doi: 10.1111/j.1601-183X.2007.00359.x
Gosso, M. F., de Geus, E. J., van Belzen, M. J., Polderman, T. J., Heutink, P., Boomsma, D. I., et al. (2006). The SNAP-25 gene is associated with cognitive ability: evidence from a family-based study in two independent Dutch cohorts. Mol. Psychiatry 11, 878–886. doi: 10.1038/sj.mp.4001868
Guerini, F. R., Agliardi, C., Sironi, M., Arosio, B., Calabrese, E., Zanzottera, M., et al. (2014). Possible association between SNAP-25 single nucleotide polymorphisms and alterations of categorical fluency and functional MRI parameters in Alzheimer’s disease. J. Alzheimers Dis. 42, 1015–1028. doi: 10.3233/JAD-140057
Guerini, F. R., Bolognesi, E., Chiappedi, M., Manca, S., Ghezzo, A., Agliardi, C., et al. (2011). SNAP-25 single nucleotide polymorphisms are associated with hyperactivity in autism spectrum disorders. Pharmacol. Res. 64, 283–288. doi: 10.1016/j.phrs.2011.03.015
Gumbel, E. (1959). Statistical estimates and transformed beta-variables, by Gunnar Blom. Can. Math. Bull. 3, 201–202. doi: 10.1017/s0008439500025571
Gunn, R. K., Keenan, M. E., and Brown, R. E. (2011). Analysis of sensory, motor and cognitive functions of the coloboma (C3Sn.Cg-Cm/J) mutant mouse. Genes Brain Behav. 10, 579–588. doi: 10.1111/j.1601-183X.2011.00697.x
Hassiotis, A. (2015). Borderline intellectual functioning and neurodevelopmental disorders: prevalence, comorbidities and treatment approaches. Adv. Mental Health Intellect. Disabil. 9:28. doi: 10.1108/AMHID-06-2015-0028
Hassiotis, A., Brown, E., Harris, J., Helm, D., Munir, K., Salvador-Carulla, L., et al. (2019). Association of borderline intellectual functioning and adverse childhood experience with adult psychiatric morbidity. Findings from a British birth cohort. BMC Psychiatry 19:387. doi: 10.1186/s12888-019-2376-0
Hess, E. J., Jinnah, H. A., Kozak, C. A., and Wilson, M. C. (1992). Spontaneous locomotor hyperactivity in a mouse mutant with a deletion including the Snap gene on chromosome 2. J. Neurosci. 12, 2865–2874. doi: 10.1523/JNEUROSCI.12-07-02865.1992
Hess, E. J., Rogan, P. K., Domoto, M., Tinker, D. E., Ladda, R. L., and Ramer, J. C. (1995). Absence of linkage of apparently single gene mediated ADHD with the human syntenic region of the mouse mutant Coloboma. Am. J. Med. Genet. 60, 573–579. doi: 10.1002/ajmg.1320600619
Igelström, K. M., and Graziano, M. S. A. (2017). The inferior parietal lobule and temporoparietal junction: a network perspective. Neuropsychologia 105, 70–83. doi: 10.1016/j.neuropsychologia.2017.01.001
Jung, R. E., and Haier, R. J. (2007). The Parieto-Frontal Integration Theory (P-FIT) of intelligence: converging neuroimaging evidence. Behav Brain Sci. 30, 135–154. doi: 10.1017/s0140525x07001185
Liu, Y. S., Dai, X., Wu, W., Yuan, F. F., Gu, X., Chen, J. G., et al. (2017). The association of SNAP25 gene polymorphisms in attention deficit/hyperactivity disorder: a systematic review and meta-analysis. Mol. Neurobiol. 54, 2189–2200. doi: 10.1007/s12035-016-9810-9
Martinez-Arca, S., Coco, S., Mainguy, G., Schenk, U., Alberts, P., Bouillé, P., et al. (2001). A common exocytotic mechanism mediates axonal and dendritic outgrowth. J. Neurosci. 1, 3830–3838. doi: 10.1523/JNEUROSCI.21-11-03830.2001
Menary, K., Collins, P. F., Porter, J. N., Muetzel, R., Olson, E. A., Kumar, V., et al. (2013). Associations between cortical thickness and general intelligence in children, adolescents and young adults. Intelligence 41, 597–606. doi: 10.1016/j.intell.2013.07.010
Orsini, A., and Picone, L. (2006). Wechsler Intelligence scale for Children. Adattamento italiano. Florence: Giunti OS.
Orsini, A., Pezzuti, L., and Picone, L. (2012). Contributo alla Taratura Italiana [WISC-IV Italian Edition]. Florence: Giunti OS.
Peltopuro, M., Ahonen, T., Kaartinen, J., Seppälä, H., and Närhi, V. (2014). Borderline intellectual functioning: a systematic literature review. Intellect. Dev. Disabil. 52, 419–443. doi: 10.1352/1934-9556-52.6.419
Posthuma, D., De Geus, E. J., Baaré, W. F., Hulshoff Pol, H. E., Kahn, R. S., and Boomsma, D. I. (2002). The association between brain volume and intelligence is of genetic origin. Nat. Neurosci. 5, 83–84. doi: 10.1038/nn0202-83
Posthuma, D., Luciano, M., Geus, E. J., Wright, M. J., Slagboom, P. E., Montgomery, G. W., et al. (2005). A genomewide scan for intelligence identifies quantitative trait loci on 2q and 6p. Am. J. Hum. Genet. 77, 318–326. doi: 10.1086/432647
Predescu, E., Sipos, R., Costescu, C. A., Ciocan, A., and Rus, D. I. (2020). Executive functions and emotion regulation in attention-deficit/hyperactivity disorder and borderline intellectual disability. J. Clin. Med. 9:986. doi: 10.3390/jcm9040986
Purcell, S., Neale, B., Todd-Brown, K., Thomas, L., Ferreira, M. A., Bender, D., et al. (2007). PLINK: a tool set for whole-genome association and population-based linkage analyses. Am. J. Hum. Genet. 81, 559–575. doi: 10.1086/519795
Rizzi, T. S., Beunders, G., Rizzu, P., Sistermans, E., Twisk, J. W., van Mechelen, W., et al. (2012). Supporting the generalist genes hypothesis for intellectual ability/disability: the case of SNAP25. Genes Brain Behav. 11, 767–771. doi: 10.1111/j.1601-183X.2012.00819.x
Rizzolatti, G., and Craighero, L. (2004). The mirror-neuron system. Annu. Rev. Neurosci. 27, 169–192. doi: 10.1146/annurev.neuro.27.070203.144230
Ropers, H. H. (2008). Genetics of intellectual disability. Curr. Opin. Genet. Dev. 18, 241–250. doi: 10.1016/j.gde.2008.07.008
Rosen, M. L., Sheridan, M. A., Sambrook, K. A., Meltzoff, A. N., and McLaughlin, K. A. (2018). Socioeconomic disparities in academic achievement: a multi-modal investigation of neural mechanisms in children and adolescents. Neuroimage 173, 298–310. doi: 10.1016/j.neuroimage.2018.02.043
Russell, V. A. (2007). Neurobiology of animal models of attention-deficit hyperactivity disorder. J. Neurosci. Methods 161, 185–198. doi: 10.1016/j.jneumeth.2006.12.005
Salvador-Carulla, L., García-Gutiérrez, J. C., Ruiz Gutiérrez-Colosía, M., Artigas-Pallarès, J., García Ibáñez, J., and González Pérez, J. (2013). Borderline intellectual functioning: consensus and good practice guidelines. Rev. Psiquiatr. Salud Ment. 6, 109–120. doi: 10.1016/j.rpsm.2012.12.001
Sauce, B., and Matzel, L. D. (2018). The paradox of intelligence: heritability and malleability coexist in hidden gene-environment interplay. Psychol. Bull. 144, 26–47. doi: 10.1037/bul0000131
Shi, Y. Y., and He, L. (2005). SHEsis, a powerful software platform for analyses of linkage disequilibrium, haplotype construction, and genetic association at polymorphism loci. Cell Res. 15, 97–98. doi: 10.1038/sj.cr.7290272
Söderqvist, S., McNab, F., Peyrard-Janvid, M., Matsson, H., Humphreys, K., Kere, J., et al. (2010). The SNAP25 gene is linked to working memory capacity and maturation of the posterior cingulate cortex during childhood. Biol. Psychiatry 68, 1120–1125. doi: 10.1016/j.biopsych.2010.07.036
Südhof, T. C. (2015). La machinerie moléculaire de sécrétion des neurotransmetteurs [The molecular machinery of neurotransmitter secretion]. Biol. Aujourdhui 209, 3–33. doi: 10.1051/jbio/2015012
Thompson, P. M., Egbufoama, S., and Vawter, M. P. (2003). SNAP-25 reduction in the hippocampus of patients with schizophrenia. Prog. Neuropsychopharmacol. Biol. Psychiatry 27, 411–417. doi: 10.1016/S0278-5846(03)00027-7
Vuijk, P. J., Hartman, E., Scherder, E., and Visscher, C. (2010). Motor performance of children with mild intellectual disability and borderline intellectual functioning. J. Intellect. Disabil. Res. 54, 955–965. doi: 10.1111/j.1365-2788.2010.01318.x
Keywords: genetics, brain imaging, learning disabilities, neural plasticity, rehabilitation, child psychiatry
Citation: Blasi V, Bolognesi E, Ricci C, Baglio G, Zanzottera M, Canevini MP, Walder M, Cabinio M, Zanette M, Baglio F, Clerici M and Guerini FR (2021) SNAP-25 Single Nucleotide Polymorphisms, Brain Morphology and Intelligence in Children With Borderline Intellectual Functioning: A Mediation Analysis. Front. Neurosci. 15:715048. doi: 10.3389/fnins.2021.715048
Received: 26 May 2021; Accepted: 04 August 2021;
Published: 26 August 2021.
Edited by:
Noriyoshi Usui, Osaka University, JapanReviewed by:
Henrik Rasmussen, Sankt Hans Hospital, DenmarkCopyright © 2021 Blasi, Bolognesi, Ricci, Baglio, Zanzottera, Canevini, Walder, Cabinio, Zanette, Baglio, Clerici and Guerini. This is an open-access article distributed under the terms of the Creative Commons Attribution License (CC BY). The use, distribution or reproduction in other forums is permitted, provided the original author(s) and the copyright owner(s) are credited and that the original publication in this journal is cited, in accordance with accepted academic practice. No use, distribution or reproduction is permitted which does not comply with these terms.
*Correspondence: Mario Clerici, bWNsZXJpY2lAZG9uZ25vY2NoaS5pdA==
†These authors have contributed equally to this work and share last authorship
Disclaimer: All claims expressed in this article are solely those of the authors and do not necessarily represent those of their affiliated organizations, or those of the publisher, the editors and the reviewers. Any product that may be evaluated in this article or claim that may be made by its manufacturer is not guaranteed or endorsed by the publisher.
Research integrity at Frontiers
Learn more about the work of our research integrity team to safeguard the quality of each article we publish.