- 1Department of Psychiatry, The Jikei University School of Medicine, Tokyo, Japan
- 2Department of Clinical and Experimental Epilepsy, University College London Institute of Neurology, London, United Kingdom
- 3Department of Human Anatomy and Cell Science, Rady Faculty of Health Sciences, Max Rady College of Medicine, University of Manitoba, Winnipeg, MB, Canada
Epilepsy is a common neurological disorder characterized by recurrent and disabling seizures. An increasing number of clinical and experimental applications of machine learning (ML) methods for epilepsy and other neurological and psychiatric disorders are available. ML methods have the potential to provide a reliable and optimal performance for clinical diagnoses, prediction, and personalized medicine by using mathematical algorithms and computational approaches. There are now several applications of ML for epilepsy, including neuroimaging analyses. For precise and reliable clinical applications in epilepsy and neuroimaging, the diverse ML methodologies should be examined and validated. We review the clinical applications of ML models for brain imaging in epilepsy obtained from a PubMed database search in February 2021. We first present an overview of typical neuroimaging modalities and ML models used in the epilepsy studies and then focus on the existing applications of ML models for brain imaging in epilepsy based on the following clinical aspects: (i) distinguishing individuals with epilepsy from healthy controls, (ii) lateralization of the temporal lobe epilepsy focus, (iii) the identification of epileptogenic foci, (iv) the prediction of clinical outcomes, and (v) brain-age prediction. We address the practical problems and challenges described in the literature and suggest some future research directions.
Introduction
Machine learning (ML) is an emerging trend in medicine including the fields of neurology and epileptology. The advantages of ML over conventional methods include accurate, automated, and fast pattern learning, which can be used to develop and/or optimize clinically useful algorithms for clinical medicine and basic research.
Epilepsy is a common neurological disease characterized by recurrent seizures associated with abnormal neuronal activities in the brain. Approximately 50 million people suffer from epilepsy worldwide, with symptoms that range from recurrent seizures and their physical problems to various psychosocial and psychiatric comorbidities (Collaborators, 2019). To better treat patients with epilepsy, appropriate seizure management and therapies for other aspects of epilepsy are important. However, there is a certain level of heterogeneity in epilepsy, which may prevent the best treatment for each individual patient (Pitkanen et al., 2016). ML methods could potentially outperform conventional approaches in terms of optimizing clinical diagnoses, prediction, and personalized medicine.
Recent clinical and experimental applications of ML for epilepsy include automatic seizure detection from clinical data, pre-surgical planning, the prediction of medical and surgical outcomes, and automated neuroimaging analyses (Abbasi and Goldenholz, 2019). Neuroimaging is one of the clinically essential exams for epilepsy (Bernasconi et al., 2019). While the main role of neuroimaging in epilepsy is the detection of the focus lesion in drug-resistant epilepsy, there is promising evidence of further usefulness of neuroimaging, such as the prediction of cognitive functions and postsurgical seizure outcomes in epilepsy (Bernasconi and Wang, 2021). In addition, ML methods usually require “big data” from multiple databases to provide reliable results, and in fact the development of ML has been driven by improved data collection, storage, and processing (Abbasi and Goldenholz, 2019). In this regard, neuroimaging may have some advantages for data sharing, since it has standardized protocols across various institutes and covers essentially the whole brain. The neuroimaging modalities MRI and PET are widely used in clinical practice and have been thoroughly investigated. Given the rapid development in neuroimaging techniques and ML, both of these can be expected to continue to further progress interactively. Thus, to efficiently understand and promote such development, it is meaningful to thoroughly review the current literature on ML applications for neuroimaging in epilepsy.
In this review, we have focused mainly on the existing applications of ML for brain MRI (including structural, diffusion, and functional MRI) and PET in epilepsy, aiming to provide an at-a-glance overview of these modalities. We first present a brief overview of neuroimaging modalities and ML models that are commonly used in epilepsy, such as data reduction/feature selection, classification/regression, and validation methods. We then provide a comprehensive review of the state-of-the-art ML models for epilepsy in clinical settings. To this end, we considered the following clinical aspects related to applications of ML models for brain imaging in the field of epilepsy: (i) the differentiation of individuals with epilepsy from healthy controls, (ii) the lateralization of the temporal lobe epilepsy focus, (iii) identifying the epileptogenic foci, (iv) the prediction of clinical outcomes, and (v) brain-age estimation. Lastly, we address the challenges and limitations of the existing studies, and we present potential future lines of research in this field.
Literature Search and Study Selection
In February 2021, we systematically reviewed the relevant articles in the PubMed database by first performing a literature search concentrated on the application of ML models for brain imaging in epilepsy along with a Preferred Reporting Items for Systematic Reviews and Meta-Analyses (PRISMA) diagram (Moher et al., 2009; Figure 1). The search strategy used “Epilepsy” combined with the following terms: “machine learning,” “deep learning,” “MRI,” “PET,” and “neuroimaging.” The search yielded 118 studies, of which we excluded 14 reviews and case reports at the initial screening. Studies not focusing on clinical epileptology, neuroimaging, or machine learning were also excluded from the review (n = 20). A final total of 84 studies were reviewed, based on the study purposes, participants, imaging modalities, feature extractions, and ML models in epilepsy (Figure 1).
From Neuroimaging to Prediction Frameworks: An Overview
Neuroimaging Modalities in Epilepsy
The typical structural brain MRI modalities in epilepsy include T1-weighted images (T1WI), T2-weighted images (T2WI), and fluid-attenuated inversion recovery (FLAIR), which are recommended as standard clinical protocols for epilepsy (Bernasconi et al., 2019). T1WI is used for evaluations of brain morphology. The cortical thickness of each gyrus and the volumes of each brain structure, e.g., hippocampus, can be calculated using T1WI, which has been frequently used for ML analyses. T2WI is useful to evaluate hippocampal internal structures, the amygdala, and parahippocampal cortices, while the FLAIR image sequence is suitable for the detection of focal cortical dysplasia type II, which frequently shows hyperintense FLAIR signals (Bernasconi et al., 2019). Diffusion MRI is also widely investigated in epilepsy, particularly when the white matter tract integrity in the brain is examined (Otte et al., 2012). Diffusion tensor imaging (DTI) metrics, such as fractional anisotropy (FA) and mean diffusivity (MD), have been conventionally utilized for white matter evaluations as well as ML applications. Multi-shell protocols of diffusion MRI including diffusion kurtosis imaging (DKI) and neurite orientation dispersion and density imaging (NODDI) have provided further information on brain microstructures (Jensen et al., 2005; Zhang et al., 2012). In addition to microstructural evaluations, brain structural networks can be measured by diffusion MRI.
Functional MRI provides information on hemodynamic brain activities by measuring blood oxygen level-dependent (BOLD) signals. Resting-state BOLD signals have recently been used to evaluate brain functional networks; in addition, metrics derived from resting-state functional MRI (e.g., functional connectivity, regional homogeneity, and the amplitude of low-frequency fluctuation) are sometimes used for ML analyses. 18F-fluorodeoxyglucose (FDG)-PET is an established examination for epilepsy, as it shows reduced signals around epileptogenic foci reflecting abnormal glucose metabolisms (Kumar and Chugani, 2013). FDG-PET signals thus indicate brain regional metabolisms and are sometimes used for a ML analysis in epilepsy. The uses of the different neuroimaging modalities described in this review are depicted in Figure 2. As can be seen in Figure 2, T1WI measurements have been used the most widely in machine learning-based epilepsy studies, probably due to the availability of plentiful T1WI brain scan data.
For the uses of machine learning, we need to extract features from the imaging modalities, and as described above, morphological features from T1WI, signal intensity from T2WI or FLAIR, diffusion metrics (e.g., FA, MD) from DTI, connectivity metrics from functional MRI, or glycometabolism data from FDG-PET are commonly used for feature extraction in machine learning. The feature extraction technique and the imaging modality are crucial factors for successful ML classification as well as easier and wider clinical applications.
Feature Selection and Data Reduction
The raw feature space in neuroimaging data is generally much greater than the number of samples, particularly for voxel-based feature extraction strategies. The main objectives of feature-selection/data-reduction methods are avoiding both the “curse of dimensionality” and overfitting, and selecting the most informative feature sets. The aim of feature reduction algorithms is to represent a lower dimensional space of the high-dimensional original data. Among the different data reduction methods, the principal component analysis (PCA) has been widely used in epilepsy studies (Beheshti et al., 2020c; Sone et al., 2021). It should be noted that the PCA method is categorized as an unsupervised technique which only reduces the input space without improving the prediction accuracy.
The main objectives of feature-selection methods are (i) exploring the features that are relevant to the specific ML task, (ii) selecting the most informative features, and (iii) improving the prediction accuracy. Various feature-selection methods have been used in the field of epilepsy, including feature ranking (Vasta et al., 2018; Beheshti et al., 2020b), analysis of variance (ANOVA)-based feature selection (Cantor-Rivera et al., 2015), correlation-based feature selection (Cantor-Rivera et al., 2015), the F-score, least absolute shrinkage and a selection operator, and mutual information (Vasta et al., 2018).
ML Models
The aim of a ML model is to explore a pattern between a dependent variable and corresponding independent variables in the training dataset (after feature reduction/selection) to determine a predicted status (or value) on test datasets (i.e., unseen data). The following is a brief discussion of ML algorithms (i.e., classification and regression models) used in existing epilepsy studies.
Classification Models
The most frequently used classification techniques in epilepsy are a support vector machine (SVM), neural networks (NN), random forest, and deep learning. An SVM is the powerful classifier based on statistical learning principles, and the SVM technique has been widely used for epilepsy classification tasks. During the training phase, an SVM finds the best class separating a hyperplane, which contributes to the maximum margin between classes. An SVM with a linear kernel can be used for linearly separable data, whereas non-linear kernel transformations such as quadratic, polynomial, and radial basis function (RBF) kernels can be used for non-linearly separable data. For example, Beheshti et al. (2020a) used an SVM classifier with a linear kernel for the classification and lateralization of MRI-negative temporal lobe epilepsy (TLE) based on FLAIR data.
An artificial neural network (ANN) is a compactional model based on biological neural networks that compose the human brain. An ANN is formed based on a set of layers (i.e., layer, one or more hidden layers, and an output layer) that are independent of each other, plus connected nodes that are called “artificial neurons.” The number of nodes in each layer is arbitrary. In an AAN structure, each node is connected to every other node and each connection has a weight and threshold. Different ANN structures have been used for brain imaging data in epilepsy (Kerr et al., 2013a, b; Pedersen et al., 2015). For example, Kerr et al. (2013b) used a multilayer perceptron (MLP) model as a classifier for the diagnosis and localization of lateralized TLE. In that study, the authors compared the SVM algorithm with a feed-forward multi-layer persectron neural network (MLPNN) for the lateralization of epileptogenic hippocampus based on MRI data.
Deep learning is a set of machine-leaning algorithms (essentially a neural network with three or more layers) that is able to learn features from the data in order to reach a high degree of abstraction (Plis et al., 2014). Deep learning embeds the feature-extraction stage in the learning phase (Shen et al., 2017). Although deep-learning methods have attracted much attention in neuroimaging studies (Zhang et al., 2020), it should be noted that these methods require a large training sample size in the training phase—which can be viewed as a limitation for this type of brain study with a limited dataset. There is a large variety of deep-learning architectures that can be used in brain imaging data, including a convolutional neural network (CNN), a recurrent neural network (RNN), and an auto encoder (AE). Hosseini et al. (2020) used a CNN deep learning structure for the localization and prediction of epileptogenicity based on EEG and rs-fMRI data. In an investigation by Si et al. (2020), a CNN-wise transfer learning technique combined with high angular resolved diffusion imaging (HARDI) and NODDI data were used for the detection of juvenile myoclonic epilepsy. A CNN model based on rs-fMRI data was trained for the classification of pediatric refractory epilepsy (Nguyen et al., 2021).
A random forest classification model works based on an ensemble learning method and voting for multiple unpruned decision trees. The bootstrap sample of the original dataset generates a random distribution of the samples for each decision tree. By eliminating the overfitting problems in decision-making trees, a random forest model is able to improve the predicting accuracy. In the context of epilepsy, a random forest algorithm has been used in various studies (Paldino et al., 2017a, b; Vasta et al., 2018). For example, Park and Ohn (2019) used a random forest classifier for estimating the seizure frequency in TLE through structural MTI features. In addition to classification tasks, the random forest method has been used for the determination of feature importance and selection (Fallahi et al., 2020). Other classification algorithms have also been applied in epilepsy studies, including XGBoost (Torlay et al., 2017), a naïve Baysian classifier (Hwang et al., 2019b), Adaboost (Park et al., 2020), and a quadratic discriminant analysis (Chiang et al., 2015).
Regression Model
Support vector regression (SVR) is known as the most widely used regression model for the prediction of continuous variables. SVR is used to find an optimal hyperplane that deviates from the training data as little as possible, such as linear regression. Unlike linear regression (in which the algorithm is aimed at minimizing the observed training errors), an SVR model measures the error on the basis of data points rather than a “margin of tolerance.” SVR has shown a very good performance in regression analyses for neuroimaging (Hwang et al., 2020; Sone et al., 2021).
Gaussian process regression (GPR) is a non-parametric Bayesian method for regression tasks. GPR works based on a probability distribution of possible values. Both SVR and GPR models have been used for estimating the brain age in epilepsy (Pardoe et al., 2017; Hwang et al., 2020; Sone et al., 2021). Logistics regression is a statistical model that models the association between predictor variables and a categorical response variable. The output of a logistic regression model is a probability value that falls into a 0–1 range, but with the use of a classification cut-off (i.e., probability of 0.5), logistic regression can be used for classification tasks (Pustina et al., 2015; Peter et al., 2018). Logistic regression has been widely used a binary classifier in epilepsy studies (Ahmed et al., 2015; Mahmoudi et al., 2018; Guo et al., 2020).
Figure 3 displays the usage of the machine learning models described herein. SVM algorithms have been widely applied in epilepsy studies compared to other ML models. This is because SVM provides an optimal solution for solving a complex problem by using different kernels, which is appropriate for high-dimensional data and limited sample studies.
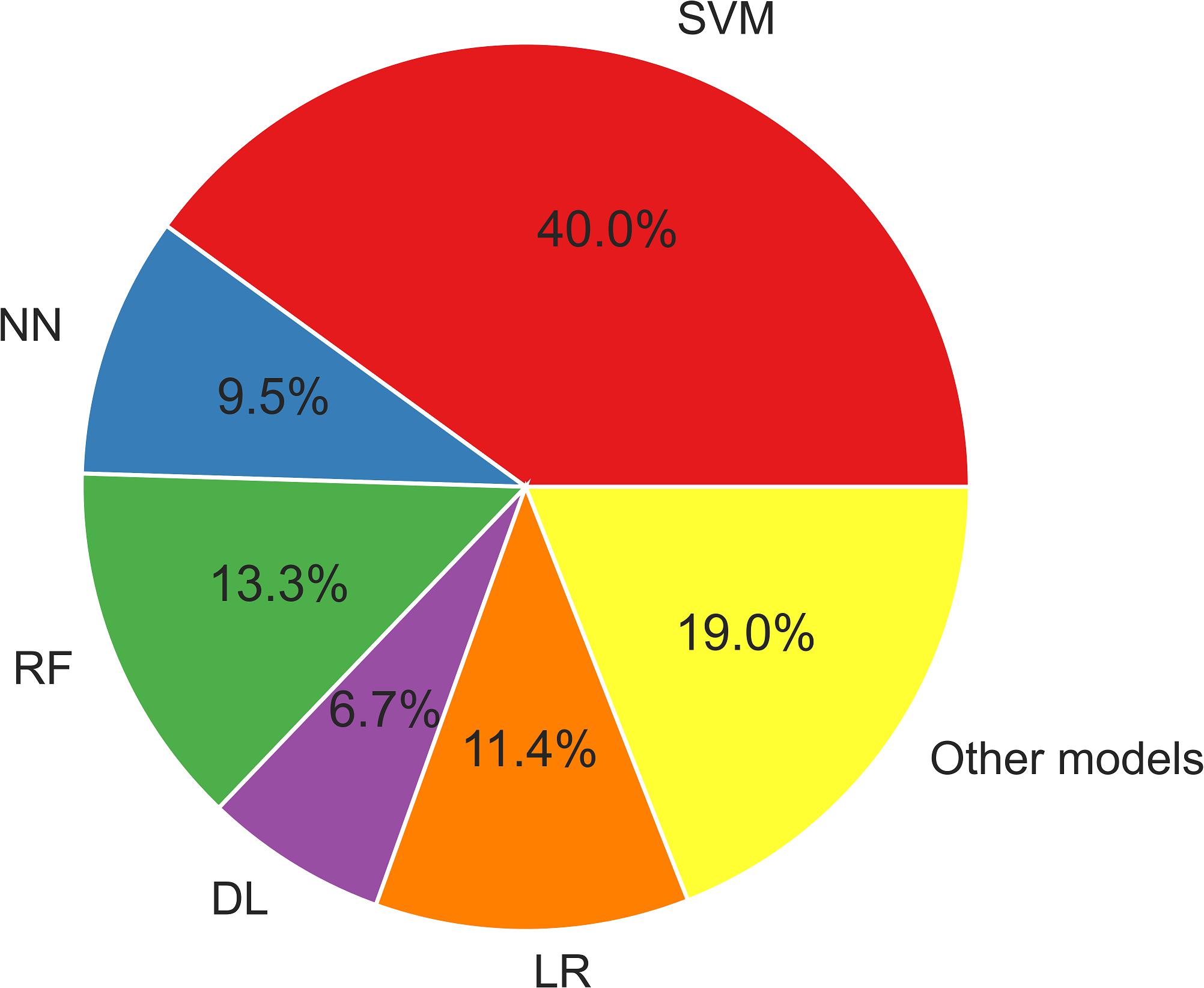
Figure 3. The ML models used in epilepsy studies. DL, deep learning; LR, logistic regression; NN, neural network; RF, random forest; SVM, support vector machine. Other models: XGBoost, LightGBM, CatBoost, decision tree, quadratic discriminant analysis.
Validation Strategies
Cross-validation is frequently used to evaluate the performance of ML models. The aim of cross-validation is to achieve an unbiased estimate of the classification/regressing performance and avoid overfitting by dividing the data into a training set and a test set. Data can be split once (i.e., split into a training set and a holdout test set) or several times (i.e., k-fold cross-validation). In the hold-out strategy, the data are randomly divided into a training set and an independent test set, and a training subset is used to train a prediction model (i.e., classification or regression) and optimize the respective parameters, whereas the independent test set is used to estimate the performance of the trained prediction model.
In the k-fold cross-validation strategy, data randomly split into k number of folds (i.e., k-subsets) and the learning process repeat k times such that for each iteration, k − 1 folds are used for training a prediction model, and the rest of the folds are used for a test. It should be noted that with the k-fold cross-validation strategy, there might be an overlap among the training subjects in different iterations (Noirhomme et al., 2014). Permutation tests can thus be used for assessing the statistical significance of k-fold cross-validation strategies (Noirhomme et al., 2014). In addition, fivefold and tenfold cross-validations have been suggested to provide a trade-off between bias and variance in ML models for brain imaging studies (Lemm et al., 2011). The leave-one-out strategy is a subtype of the k-fold cross-validation strategy in which the number of folds is equal to the number of samples; it is usually used for a small dataset. The k-fold cross-validation strategy has been widely used in epilepsy studies (Bharath et al., 2019; Beheshti et al., 2020a; Zhou et al., 2020; Sone et al., 2021).
The Differentiation of Individuals With Epilepsy From Healthy Controls
A common application of machine learning for brain imaging in epilepsy is the differentiation between brains with epilepsy and healthy brains. As summarized in Table 1, various ML classifiers have achieved over 70–80% accuracy to successfully discriminate between individuals with epilepsy and healthy controls, using T1 images (Vasta et al., 2018; Chen et al., 2020; Park et al., 2020), diffusion MRI (Cantor-Rivera et al., 2015; Del Gaizo et al., 2017; Park and Ohn, 2019; Huang et al., 2020; Si et al., 2020), and functional MRI (Pedersen et al., 2015; Torlay et al., 2017; Wang et al., 2018a; Bharath et al., 2019; Hwang et al., 2019a, b; Zhou et al., 2020; Nguyen et al., 2021). Studies targeting TLE achieved ∼90% accuracy (Cantor-Rivera et al., 2015; Bharath et al., 2019; Chen et al., 2020; Huang et al., 2020), but it has been more challenging to identify idiopathic generalized epilepsy (IGE), and only ∼75% accuracy has been obtained for this task (Wang et al., 2018a; Si et al., 2020). Though these impressive investigations yielded evidence of the potential of machine learning in epilepsy, the clinical usefulness of the findings might be limited, since a differentiation between individuals with epilepsy and healthy subjects is not a major role of neuroimaging.
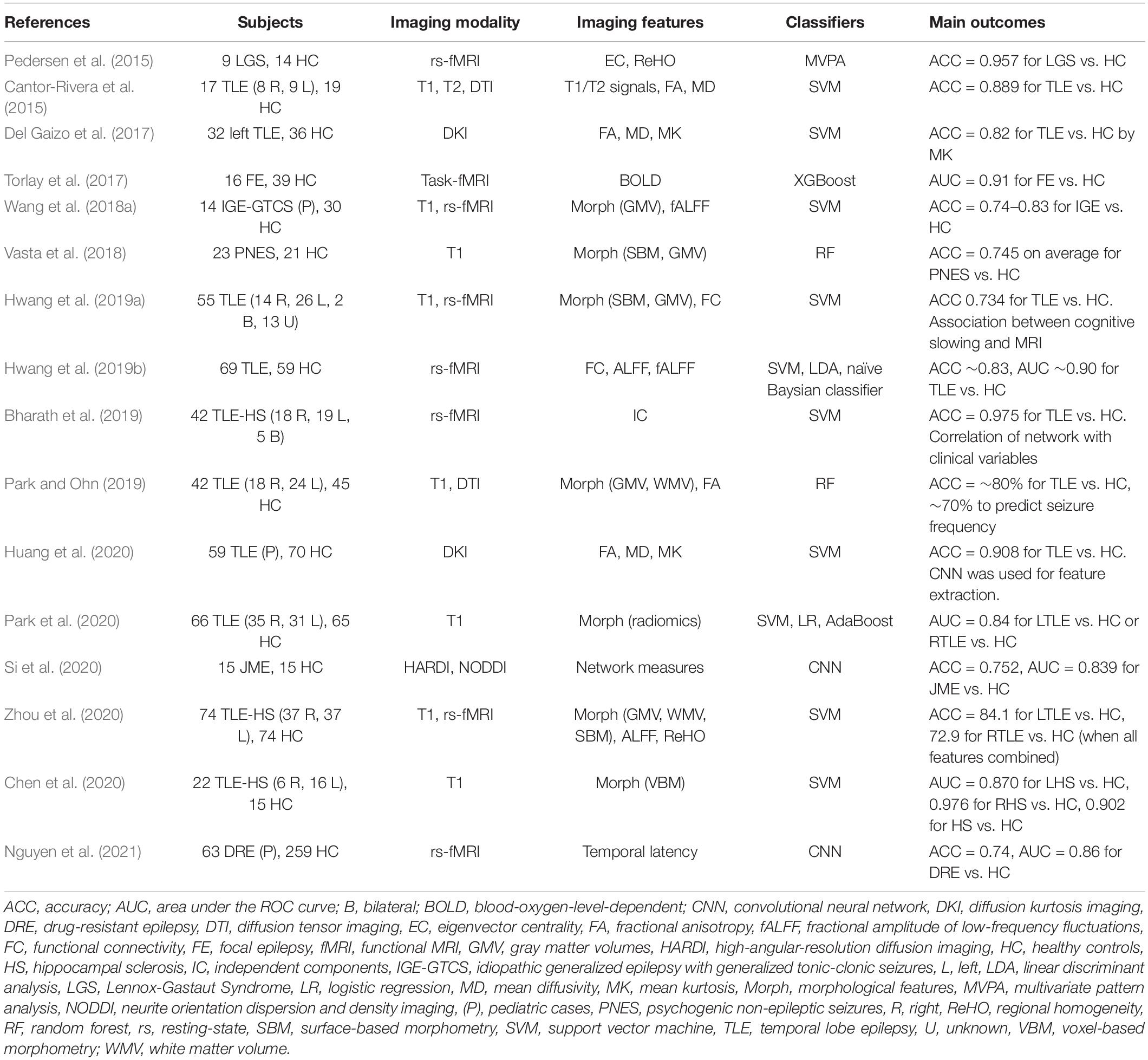
Table 1. ML applications used for the differentiation of individuals with epilepsy and healthy subjects.
Lateralization of TLE Foci
TLE is the most prevalent form of adult epilepsy and often causes drug-resistant seizures (Engel, 1996). There is clear evidence that surgical resection is more effective for refractory TLE than a continued use of anti-epilepsy drugs (Wiebe et al., 2001), and the accurate lateralization of the focus side in TLE is thus clinically important and one of the main targets of neuroimaging research in epilepsy.
As listed in Table 2, there have been various ML approaches to the lateralization of TLE foci, using T1-weighted images, diffusion MRI, FLAIR images, 18F-FDG-PET, or a combination of these (Focke et al., 2012; Keihaninejad et al., 2012; Kerr et al., 2013a, b; An et al., 2014; Hosseini et al., 2014; Chiang et al., 2015; Pustina et al., 2015; Yang et al., 2015; Kamiya et al., 2016; Fang et al., 2017; Mahmoudi et al., 2018; Peter et al., 2018; Bennett et al., 2019; Beheshti et al., 2020a, c; Fallahi et al., 2020; Hosseini et al., 2020). The applications of these approaches to cases without visually detectable lesions, i.e., so-called MRI-negative TLE, would be particularly beneficial in clinical settings by providing further clues to the focus beyond the conventional approaches. Although the current accuracy of ML lateralization for MRI-negative TLE seems not as high as that for MRI-positive cases (> 98%) (Bennett et al., 2019), this approach has achieved > 75% accuracy, which would be acceptable in clinical practice (Keihaninejad et al., 2012; Bennett et al., 2019; Beheshti et al., 2020a, c).
Identification of Epileptogenic Foci, Particularly in Focal Cortical Dysplasia (FCD)
The accurate localization of the epileptogenic focus is highly relevant for successful epilepsy surgery (Rathore and Radhakrishnan, 2015), which may remediate drug-resistant focal epilepsy. Structural MRI in particular plays a major role in the visual detection of focus lesions, and it has been widely used in clinical practice for epilepsy (Bernasconi et al., 2019). As seen in Table 3, there have been various applications of machine learning for lesion identification to improve the detection rate or to develop automated algorithms (Hong et al., 2014; Ahmed et al., 2015; Rudie et al., 2015; El Azami et al., 2016; Adler et al., 2017; Jin et al., 2018; Tan et al., 2018; Wang et al., 2018b; Mo et al., 2019; Alaverdyan et al., 2020; Lee et al., 2020a; Wagstyl et al., 2020; Snyder et al., 2021; Zhang et al., 2021), which would be concordant with the seizure onset zone detected by intracranial EEG (Kanber et al., 2021). Focal cortical dysplasia (FCD), which is a common cause of intractable epilepsy, is characterized by abnormal cortical thickness, blurring of the gray-white matter junction, and T2/FLAIR hyperintensity (Bernasconi et al., 2019). It is thus reasonable that an accurate ML diagnosis was usually achieved by structural MRI (such as T1 or FLAIR imaging, often using surface-based methods) rather than functional MRI (Table 3). More recent studies tend to use combined data from multimodal imaging, whereas earlier studies used only T1WI (Table 3). Differential diagnoses such as FCD type I vs. II and FCD vs. tumor were also reported (Hong et al., 2016; Guo et al., 2020).
Prediction of Clinical Outcomes
There are also various ML applications for more direct associations with clinical outcomes than lesion/focus detection (Table 4). A major trend in this section is the prediction of postsurgical seizure freedom (Bernhardt et al., 2015; Memarian et al., 2015; Munsell et al., 2015; He et al., 2017; Gleichgerrcht et al., 2018; Taylor et al., 2018; Gleichgerrcht et al., 2020; Larivière et al., 2020; Kini et al., 2021; Sinha et al., 2021), in light of the clinical importance. Most of the studies reported 70–90% accuracy for the prediction of seizure outcomes after resection surgery. Other studies presented approximately 85% accuracy for the identification of responders to vagus nerve stimulation (VNS) (Ibrahim et al., 2017; Mithani et al., 2019). In terms of surgery, ML methods were also applied and generated good predictive values for postsurgical functional deficit (Lee et al., 2020b), lateralization of the language hemisphere (Gazit et al., 2016), and optimal planning for laser surgery (Li et al., 2019).
Cognitive dysfunctions in epilepsy were also shown to be predicted by ML methods (Paldino et al., 2014, 2017a, 2017b, 2019; He et al., 2018; Zhang et al., 2018; Höller et al., 2020; Mazrooyisebdani et al., 2020). Functional neuroimaging and/or network measurement are often used for this prediction (Paldino et al., 2017a, b, 2019; He et al., 2018; Zhang et al., 2018; Mazrooyisebdani et al., 2020). Other ML applications included predicting drug responsiveness (Amarreh et al., 2014; Yao et al., 2019), acquiring epileptogenicity (Zhang et al., 2019; Akeret et al., 2020; La Rocca et al., 2020; Wang et al., 2020), and the differentiation of types of autoantibodies (Ernst et al., 2019).
Regression Models (Brain-Age Prediction)
Another trend in the field of neuroimaging and machine learning is regression models, which are often used for the prediction of brain aging (Cole and Franke, 2017). Human brains change with aging, and this may also be associated with various neuropsychiatric diseases. To investigate the relationships between brain aging and epilepsy, several research groups have the regression model technique (Pardoe et al., 2017; Chen et al., 2019; Hwang et al., 2020; Sone et al., 2021).
In general, an increase in the age of the brain by ∼4–10 years has been reported (Table 5), which is consistent with recent evidence of disease progression or tau deposition in epilepsy (Tai et al., 2016; Galovic et al., 2019). The increased brain age in individuals with epilepsy seems to be associated with longer disease duration, early onset age, and/or psychiatric comorbidity (Pardoe et al., 2017; Chen et al., 2019; Sone et al., 2021).
Methodological Aspects and Future Directions
As described, the current ML applications for epilepsy imaging are diverse in terms of the targeted epilepsy syndromes, imaging modalities, feature extractions, and ML strategies. Multimodal imaging is a recent trend in epilepsy research, and it may provide comprehensive information (Sidhu et al., 2018). Accordingly, there has been an increase in the number of ML studies using multiple imaging modalities, especially in recent years. However, as shown in Tables 1–5, each study group seems to have some tendencies regarding the choice of imaging modalities, which may have led to the diversity of research in this field.
Feature extraction is another significant factor in the diversity of this research. While most studies have used a region-of-interest (ROI) to extract imaging features, the choice of atlases for ROIs varies. For example, some investigations used traditional automated anatomical labeling (AAL) (Fallahi et al., 2020; Si et al., 2020; Kini et al., 2021), and a different atlas was used in other studies (Gleichgerrcht et al., 2018, 2020). Zhang et al. (2019, 2021) used radiomics as a novel method to extract imaging data, and this might provide greater usefulness than conventional methods (Gillies et al., 2016). For better clinical applications, we should develop and validate consistent methodologies, since these factors may directly affect the prediction of outcomes and the algorithm itself.
Regarding the ML algorithms, more recent studies have tended to use deep-learning methods such as a CNN (Hosseini et al., 2020; Lee et al., 2020b; Si et al., 2020; Nguyen et al., 2021; Zhang et al., 2021). Another important point about methodology is the shortage of studies using unsupervised classification; indeed, only two studies adopted unsupervised clustering (Bernhardt et al., 2015; Lee et al., 2020a). Given the potentials of unsupervised clustering for finding hidden patterns in unlabeled data, further studies using this method are needed to uncover data-driven information.
Conclusion
Machine learning is an emerging trend in the field of neuroimaging in epilepsy, and promising results have been obtained in many studies. The diversity in terms of targeted epilepsy syndromes, imaging modalities, feature extractions, and ML algorithms provides an extra challenge. Recent trends include the use of deep learning, multimodal imaging, and regression models, and additional investigations using unsupervised clustering are desired. For better clinical applications, consistent methodologies must be developed and validated.
Author Contributions
Both authors listed have made a substantial, direct and intellectual contribution to the work, and approved it for publication.
Funding
This work was supported by a grant to DS from the Japan Society for the Promotion of Science (KAKENHI), no. JP21K15720.
Conflict of Interest
The authors declare that the research was conducted in the absence of any commercial or financial relationships that could be construed as a potential conflict of interest.
References
Abbasi, B., and Goldenholz, D. M. (2019). Machine learning applications in epilepsy. Epilepsia 60, 2037–2047. doi: 10.1111/epi.16333
Adler, S., Wagstyl, K., Gunny, R., Ronan, L., Carmichael, D., Cross, J. H., et al. (2017). Novel surface features for automated detection of focal cortical dysplasias in paediatric epilepsy. Neuroimage Clin. 14, 18–27. doi: 10.1016/j.nicl.2016.12.030
Ahmed, B., Brodley, C. E., Blackmon, K. E., Kuzniecky, R., Barash, G., Carlson, C., et al. (2015). Cortical feature analysis and machine learning improves detection of “MRI-negative” focal cortical dysplasia. Epilepsy Behav. 48, 21–28. doi: 10.1016/j.yebeh.2015.04.055
Akeret, K., Stumpo, V., Staartjes, V. E., Vasella, F., Velz, J., Marinoni, F., et al. (2020). Topographic brain tumor anatomy drives seizure risk and enables machine learning based prediction. Neuroimage Clin. 28:102506. doi: 10.1016/j.nicl.2020.102506
Alaverdyan, Z., Jung, J., Bouet, R., and Lartizien, C. (2020). Regularized siamese neural network for unsupervised outlier detection on brain multiparametric magnetic resonance imaging: application to epilepsy lesion screening. Med. Image Anal 60:101618. doi: 10.1016/j.media.2019.101618
Amarreh, I., Meyerand, M. E., Stafstrom, C., Hermann, B. P., and Birn, R. M. (2014). Individual classification of children with epilepsy using support vector machine with multiple indices of diffusion tensor imaging. Neuroimage Clin. 4, 757–764. doi: 10.1016/j.nicl.2014.02.006
An, J., Fang, P., Wang, W., Liu, Z., Hu, D., and Qiu, S. (2014). Decreased white matter integrity in mesial temporal lobe epilepsy: a machine learning approach. Neuroreport 25, 788–794. doi: 10.1097/wnr.0000000000000178
Beheshti, I., Sone, D., Maikusa, N., Kimura, Y., Shigemoto, Y., Sato, N., et al. (2020a). FLAIR-wise machine-learning classification and lateralization of MRI-Negative (18)F-FDG PET-positive temporal lobe epilepsy. Front. Neurol. 11:580713. doi: 10.3389/fneur.2020.580713
Beheshti, I., Sone, D., Maikusa, N., Kimura, Y., Shigemoto, Y., Sato, N., et al. (2020b). Pattern analysis of glucose metabolic brain data for lateralization of MRI-negative temporal lobe epilepsy. Epilepsy Res. 167:106474. doi: 10.1016/j.eplepsyres.2020.106474
Beheshti, I., Sone, D., Maikusa, N., Kimura, Y., Shigemoto, Y., Sato, N., et al. (2020c). Pattern analysis of glucose metabolic brain data for lateralization of MRI-negative temporal lobe epilepsy. Epilepsy Res. 167:106474.
Bennett, O. F., Kanber, B., Hoskote, C., Cardoso, M. J., Ourselin, S., Duncan, J. S., et al. (2019). Learning to see the invisible: a data-driven approach to finding the underlying patterns of abnormality in visually normal brain magnetic resonance images in patients with temporal lobe epilepsy. Epilepsia 60, 2499–2507. doi: 10.1111/epi.16380
Bernasconi, A., Cendes, F., Theodore, W. H., Gill, R. S., Koepp, M. J., Hogan, R. E., et al. (2019). Recommendations for the use of structural magnetic resonance imaging in the care of patients with epilepsy: a consensus report from the International League Against Epilepsy Neuroimaging Task Force. Epilepsia 60, 1054–1068. doi: 10.1111/epi.15612
Bernasconi, N., and Wang, I. (2021). Emerging trends in neuroimaging of epilepsy. Epilepsy Curr. 21, 79–82. doi: 10.1177/1535759721991161
Bernhardt, B. C., Hong, S. J., Bernasconi, A., and Bernasconi, N. (2015). Magnetic resonance imaging pattern learning in temporal lobe epilepsy: classification and prognostics. Ann. Neurol. 77, 436–446. doi: 10.1002/ana.24341
Bharath, R. D., Panda, R., Raj, J., Bhardwaj, S., Sinha, S., Chaitanya, G., et al. (2019). Machine learning identifies “rsfMRI epilepsy networks” in temporal lobe epilepsy. Eur. Radiol. 29, 3496–3505. doi: 10.1007/s00330-019-5997-2
Cantor-Rivera, D., Khan, A. R., Goubran, M., Mirsattari, S. M., and Peters, T. M. (2015). Detection of temporal lobe epilepsy using support vector machines in multi-parametric quantitative MR imaging. Comput. Med. Imaging Graph. 41, 14–28. doi: 10.1016/j.compmedimag.2014.07.002
Chen, C. L., Shih, Y. C., Liou, H. H., Hsu, Y. C., Lin, F. H., and Tseng, W. I. (2019). Premature white matter aging in patients with right mesial temporal lobe epilepsy: a machine learning approach based on diffusion MRI data. Neuroimage Clin. 24:102033. doi: 10.1016/j.nicl.2019.102033
Chen, S., Zhang, J., Ruan, X., Deng, K., Zhang, J., Zou, D., et al. (2020). Voxel-based morphometry analysis and machine learning based classification in pediatric mesial temporal lobe epilepsy with hippocampal sclerosis. Brain Imaging Behav. 14, 1945–1954. doi: 10.1007/s11682-019-00138-z
Chiang, S., Levin, H. S., and Haneef, Z. (2015). Computer-automated focus lateralization of temporal lobe epilepsy using fMRI. J. Magn. Reson. Imaging 41, 1689–1694. doi: 10.1002/jmri.24696
Cole, J. H., and Franke, K. (2017). Predicting age using neuroimaging: innovative brain ageing biomarkers. Trends Neurosci. 40, 681–690. doi: 10.1016/j.tins.2017.10.001
Collaborators, G. B. D. E. (2019). Global, regional, and national burden of epilepsy, 1990-2016: a systematic analysis for the Global Burden of Disease Study 2016. Lancet Neurol. 18, 357–375. doi: 10.1016/S1474-4422(18)30454-X
Del Gaizo, J., Mofrad, N., Jensen, J. H., Clark, D., Glenn, R., Helpern, J., et al. (2017). Using machine learning to classify temporal lobe epilepsy based on diffusion MRI. Brain Behav. 7:e00801. doi: 10.1002/brb3.801
El Azami, M., Hammers, A., Jung, J., Costes, N., Bouet, R., and Lartizien, C. (2016). Detection of lesions underlying intractable epilepsy on T1-weighted MRI as an outlier detection problem. PLoS One 11:e0161498. doi: 10.1371/journal.pone.0161498
Ernst, L., David, B., Gaubatz, J., Domínguez-Narciso, I., Lüchters, G., Becker, A. J., et al. (2019). Volumetry of mesiotemporal structures reflects serostatus in patients with limbic encephalitis. AJNR Am. J. Neuroradiol. 40, 2081–2089. doi: 10.3174/ajnr.A6289
Fallahi, A., Pooyan, M., Lotfi, N., Baniasad, F., Tapak, L., Mohammadi-Mobarakeh, N., et al. (2020). Dynamic functional connectivity in temporal lobe epilepsy: a graph theoretical and machine learning approach. Neurol. Sci. 42, 2379–2390. doi: 10.1007/s10072-020-04759-x
Fang, P., An, J., Zeng, L. L., Shen, H., Qiu, S., and Hu, D. (2017). Mapping the convergent temporal epileptic network in left and right temporal lobe epilepsy. Neurosci. Lett. 639, 179–184. doi: 10.1016/j.neulet.2016.12.029
Focke, N. K., Yogarajah, M., Symms, M. R., Gruber, O., Paulus, W., and Duncan, J. S. (2012). Automated MR image classification in temporal lobe epilepsy. Neuroimage 59, 356–362. doi: 10.1016/j.neuroimage.2011.07.068
Galovic, M., van Dooren, V. Q. H., Postma, T., Vos, S. B., Caciagli, L., Borzi, G., et al. (2019). Progressive cortical thinning in patients with focal epilepsy. JAMA Neurol. 76, 1230–1239. doi: 10.1001/jamaneurol.2019.1708
Gazit, T., Andelman, F., Glikmann-Johnston, Y., Gonen, T., Solski, A., Shapira-Lichter, I., et al. (2016). Probabilistic machine learning for the evaluation of presurgical language dominance. J. Neurosurg. 125, 481–493. doi: 10.3171/2015.7.Jns142568
Gillies, R. J., Kinahan, P. E., and Hricak, H. (2016). Radiomics: images are more than pictures, they are data. Radiology 278, 563–577. doi: 10.1148/radiol.2015151169
Gleichgerrcht, E., Keller, S. S., Drane, D. L., Munsell, B. C., Davis, K. A., Kaestner, E., et al. (2020). Temporal lobe epilepsy surgical outcomes can be inferred based on structural connectome hubs: a machine learning study. Ann. Neurol. 88, 970–983. doi: 10.1002/ana.25888
Gleichgerrcht, E., Munsell, B., Bhatia, S., Vandergrift, W. A. III, Rorden, C., McDonald, C., et al. (2018). Deep learning applied to whole-brain connectome to determine seizure control after epilepsy surgery. Epilepsia 59, 1643–1654. doi: 10.1111/epi.14528
Guo, Y., Liu, Y., Ming, W., Wang, Z., Zhu, J., Chen, Y., et al. (2020). Distinguishing focal cortical dysplasia from glioneuronal tumors in patients with epilepsy by machine learning. Front. Neurol. 11:548305. doi: 10.3389/fneur.2020.548305
He, X., Bassett, D. S., Chaitanya, G., Sperling, M. R., Kozlowski, L., and Tracy, J. I. (2018). Disrupted dynamic network reconfiguration of the language system in temporal lobe epilepsy. Brain 141, 1375–1389. doi: 10.1093/brain/awy042
He, X., Doucet, G. E., Pustina, D., Sperling, M. R., Sharan, A. D., and Tracy, J. I. (2017). Presurgical thalamic “hubness” predicts surgical outcome in temporal lobe epilepsy. Neurology 88, 2285–2293. doi: 10.1212/wnl.0000000000004035
Höller, Y., Butz, K. H. G., Thomschewski, A. C., Schmid, E. V., Hofer, C. D., Uhl, A., et al. (2020). Prediction of cognitive decline in temporal lobe epilepsy and mild cognitive impairment by EEG, MRI, and neuropsychology. Comput. Intell. Neurosci. 2020:8915961. doi: 10.1155/2020/8915961
Hong, S. J., Bernhardt, B. C., Schrader, D. S., Bernasconi, N., and Bernasconi, A. (2016). Whole-brain MRI phenotyping in dysplasia-related frontal lobe epilepsy. Neurology 86, 643–650. doi: 10.1212/wnl.0000000000002374
Hong, S. J., Kim, H., Schrader, D., Bernasconi, N., Bernhardt, B. C., and Bernasconi, A. (2014). Automated detection of cortical dysplasia type II in MRI-negative epilepsy. Neurology 83, 48–55. doi: 10.1212/wnl.0000000000000543
Hosseini, M. P., Nazem-Zadeh, M. R., Mahmoudi, F., Ying, H., and Soltanian-Zadeh, H. (2014). Support Vector Machine with nonlinear-kernel optimization for lateralization of epileptogenic hippocampus in MR images. Annu. Int. Conf. IEEE Eng. Med. Biol. Soc. 2014, 1047–1050. doi: 10.1109/embc.2014.6943773
Hosseini, M. P., Tran, T. X., Pompili, D., Elisevich, K., and Soltanian-Zadeh, H. (2020). Multimodal data analysis of epileptic EEG and rs-fMRI via deep learning and edge computing. Artif. Intell. Med. 104:101813. doi: 10.1016/j.artmed.2020.101813
Huang, J., Xu, J., Kang, L., and Zhang, T. (2020). Identifying epilepsy based on deep learning using DKI images. Front. Hum. Neurosci. 14:590815. doi: 10.3389/fnhum.2020.590815
Hwang, G., Dabbs, K., Conant, L., Nair, V. A., Mathis, J., Almane, D. N., et al. (2019a). Cognitive slowing and its underlying neurobiology in temporal lobe epilepsy. Cortex 117, 41–52. doi: 10.1016/j.cortex.2019.02.022
Hwang, G., Hermann, B., Nair, V. A., Conant, L. L., Dabbs, K., Mathis, J., et al. (2020). Brain aging in temporal lobe epilepsy: chronological, structural, and functional. Neuroimage Clin. 25:102183. doi: 10.1016/j.nicl.2020.102183
Hwang, G., Nair, V. A., Mathis, J., Cook, C. J., Mohanty, R., Zhao, G., et al. (2019b). Using low-frequency oscillations to detect temporal lobe epilepsy with machine learning. Brain Connect. 9, 184–193. doi: 10.1089/brain.2018.0601
Ibrahim, G. M., Sharma, P., Hyslop, A., Guillen, M. R., Morgan, B. R., Wong, S., et al. (2017). Presurgical thalamocortical connectivity is associated with response to vagus nerve stimulation in children with intractable epilepsy. Neuroimage Clin. 16, 634–642. doi: 10.1016/j.nicl.2017.09.015
Jensen, J. H., Helpern, J. A., Ramani, A., Lu, H., and Kaczynski, K. (2005). Diffusional kurtosis imaging: the quantification of non-gaussian water diffusion by means of magnetic resonance imaging. Magn. Reson. Med. 53, 1432–1440. doi: 10.1002/mrm.20508
Jin, B., Krishnan, B., Adler, S., Wagstyl, K., Hu, W., Jones, S., et al. (2018). Automated detection of focal cortical dysplasia type II with surface-based magnetic resonance imaging postprocessing and machine learning. Epilepsia 59, 982–992. doi: 10.1111/epi.14064
Kamiya, K., Amemiya, S., Suzuki, Y., Kunii, N., Kawai, K., Mori, H., et al. (2016). Machine learning of DTI structural brain connectomes for lateralization of temporal lobe epilepsy. Magn. Reson. Med. Sci. 15, 121–129. doi: 10.2463/mrms.2015-0027
Kanber, B., Vos, S. B., de Tisi, J., Wood, T. C., Barker, G. J., Rodionov, R., et al. (2021). Detection of covert lesions in focal epilepsy using computational analysis of multimodal magnetic resonance imaging data. Epilepsia 62, 807–816. doi: 10.1111/epi.16836
Keihaninejad, S., Heckemann, R. A., Gousias, I. S., Hajnal, J. V., Duncan, J. S., Aljabar, P., et al. (2012). Classification and lateralization of temporal lobe epilepsies with and without hippocampal atrophy based on whole-brain automatic MRI segmentation. PLoS One 7:e33096. doi: 10.1371/journal.pone.0033096
Kerr, W. T., Cho, A. Y., Anderson, A., Douglas, P. K., Lau, E. P., Hwang, E. S., et al. (2013a). Balancing clinical and pathologic relevence in the machine learning diagnosis of epilepsy. Int. Workshop Pattern Recognit. Neuroimaging 2013, 86–89. doi: 10.1109/prni.2013.31
Kerr, W. T., Nguyen, S. T., Cho, A. Y., Lau, E. P., Silverman, D. H., Douglas, P. K., et al. (2013b). Computer-aided diagnosis and localization of lateralized temporal lobe epilepsy using interictal FDG-PET. Front. Neurol. 4:31. doi: 10.3389/fneur.2013.00031
Kini, L. G., Thaker, A. A., Hadar, P. N., Shinohara, R. T., Brown, M. G., Dubroff, J. G., et al. (2021). Quantitative ([)(18)(])FDG PET asymmetry features predict long-term seizure recurrence in refractory epilepsy. Epilepsy Behav. 116:107714. doi: 10.1016/j.yebeh.2020.107714
Kumar, A., and Chugani, H. T. (2013). The role of radionuclide imaging in epilepsy, Part 1: sporadic temporal and extratemporal lobe epilepsy. J. Nucl. Med. 54, 1775–1781. doi: 10.2967/jnumed.112.114397
La Rocca, M., Garner, R., Amoroso, N., Lutkenhoff, E. S., Monti, M. M., Vespa, P., et al. (2020). Multiplex networks to characterize seizure development in traumatic brain injury patients. Front. Neurosci. 14:591662. doi: 10.3389/fnins.2020.591662
Larivière, S., Weng, Y., Vos de Wael, R., Royer, J., Frauscher, B., Wang, Z., et al. (2020). Functional connectome contractions in temporal lobe epilepsy: microstructural underpinnings and predictors of surgical outcome. Epilepsia 61, 1221–1233. doi: 10.1111/epi.16540
Lee, H. M., Gill, R. S., Fadaie, F., Cho, K. H., Guiot, M. C., Hong, S. J., et al. (2020a). Unsupervised machine learning reveals lesional variability in focal cortical dysplasia at mesoscopic scale. Neuroimage Clin. 28:102438. doi: 10.1016/j.nicl.2020.102438
Lee, M. H., O’Hara, N., Sonoda, M., Kuroda, N., Juhasz, C., Asano, E., et al. (2020b). Novel deep learning network analysis of electrical stimulation mapping-driven diffusion MRI tractography to improve preoperative evaluation of pediatric epilepsy. IEEE Trans. Biomed. Eng. 67, 3151–3162. doi: 10.1109/tbme.2020.2977531
Lemm, S., Blankertz, B., Dickhaus, T., and Müller, K. R. (2011). Introduction to machine learning for brain imaging. Neuroimage 56, 387–399. doi: 10.1016/j.neuroimage.2010.11.004
Li, K., Vakharia, V. N., Sparks, R., França, L. G. S., Granados, A., McEvoy, A. W., et al. (2019). Optimizing trajectories for cranial laser interstitial thermal therapy using computer-assisted planning: a machine learning approach. Neurotherapeutics 16, 182–191. doi: 10.1007/s13311-018-00693-1
Mahmoudi, F., Elisevich, K., Bagher-Ebadian, H., Nazem-Zadeh, M. R., Davoodi-Bojd, E., Schwalb, J. M., et al. (2018). Data mining MR image features of select structures for lateralization of mesial temporal lobe epilepsy. PLoS One 13:e0199137. doi: 10.1371/journal.pone.0199137
Mazrooyisebdani, M., Nair, V. A., Garcia-Ramos, C., Mohanty, R., Meyerand, E., Hermann, B., et al. (2020). Graph theory analysis of functional connectivity combined with machine learning approaches demonstrates widespread network differences and predicts clinical variables in temporal lobe epilepsy. Brain Connect 10, 39–50. doi: 10.1089/brain.2019.0702
Memarian, N., Kim, S., Dewar, S., Engel, J. Jr., and Staba, R. J. (2015). Multimodal data and machine learning for surgery outcome prediction in complicated cases of mesial temporal lobe epilepsy. Comput. Biol. Med. 64, 67–78. doi: 10.1016/j.compbiomed.2015.06.008
Mithani, K., Mikhail, M., Morgan, B. R., Wong, S., Weil, A. G., Deschenes, S., et al. (2019). Connectomic profiling identifies responders to vagus nerve stimulation. Ann. Neurol. 86, 743–753. doi: 10.1002/ana.25574
Mo, J., Liu, Z., Sun, K., Ma, Y., Hu, W., Zhang, C., et al. (2019). Automated detection of hippocampal sclerosis using clinically empirical and radiomics features. Epilepsia 60, 2519–2529. doi: 10.1111/epi.16392
Moher, D., Liberati, A., Tetzlaff, J., Altman, D. G., and Group, P. (2009). Preferred reporting items for systematic reviews and meta-analyses: the PRISMA statement. PLoS Med. 6:e1000097. doi: 10.1371/journal.pmed.1000097
Munsell, B. C., Wee, C. Y., Keller, S. S., Weber, B., Elger, C., da Silva, L. A., et al. (2015). Evaluation of machine learning algorithms for treatment outcome prediction in patients with epilepsy based on structural connectome data. Neuroimage 118, 219–230. doi: 10.1016/j.neuroimage.2015.06.008
Nguyen, R. D., Kennady, E. H., Smyth, M. D., Zhu, L., Pao, L. P., Swisher, S. K., et al. (2021). Convolutional neural networks for pediatric refractory epilepsy classification using resting-state fMRI. World Neurosurg. 149, e1112–e1122. doi: 10.1016/j.wneu.2020.12.131
Noirhomme, Q., Lesenfants, D., Gomez, F., Soddu, A., Schrouff, J., Garraux, G., et al. (2014). Biased binomial assessment of cross-validated estimation of classification accuracies illustrated in diagnosis predictions. Neuroimage Clin. 4, 687–694. doi: 10.1016/j.nicl.2014.04.004
Otte, W. M., van Eijsden, P., Sander, J. W., Duncan, J. S., Dijkhuizen, R. M., and Braun, K. P. (2012). A meta-analysis of white matter changes in temporal lobe epilepsy as studied with diffusion tensor imaging. Epilepsia 53, 659–667. doi: 10.1111/j.1528-1167.2012.03426.x
Paldino, M. J., Golriz, F., Chapieski, M. L., Zhang, W., and Chu, Z. D. (2017a). Brain network architecture and global intelligence in children with focal epilepsy. AJNR Am. J. Neuroradiol. 38, 349–356. doi: 10.3174/ajnr.A4975
Paldino, M. J., Golriz, F., Zhang, W., and Chu, Z. D. (2019). Normalization enhances brain network features that predict individual intelligence in children with epilepsy. PLoS One 14:e0212901. doi: 10.1371/journal.pone.0212901
Paldino, M. J., Hedges, K., and Zhang, W. (2014). Independent contribution of individual white matter pathways to language function in pediatric epilepsy patients. Neuroimage Clin. 6, 327–332. doi: 10.1016/j.nicl.2014.09.017
Paldino, M. J., Zhang, W., Chu, Z. D., and Golriz, F. (2017b). Metrics of brain network architecture capture the impact of disease in children with epilepsy. Neuroimage Clin. 13, 201–208. doi: 10.1016/j.nicl.2016.12.005
Pardoe, H. R., Cole, J. H., Blackmon, K., Thesen, T., and Kuzniecky, R. (2017). Structural brain changes in medically refractory focal epilepsy resemble premature brain aging. Epilepsy Res. 133, 28–32. doi: 10.1016/j.eplepsyres.2017.03.007
Park, C. H., and Ohn, S. H. (2019). A challenge of predicting seizure frequency in temporal lobe epilepsy using neuroanatomical features. Neurosci. Lett. 692, 115–121. doi: 10.1016/j.neulet.2018.11.005
Park, Y. W., Choi, Y. S., Kim, S. E., Choi, D., Han, K., Kim, H., et al. (2020). Radiomics features of hippocampal regions in magnetic resonance imaging can differentiate medial temporal lobe epilepsy patients from healthy controls. Sci. Rep. 10:19567. doi: 10.1038/s41598-020-76283-z
Pedersen, M., Curwood, E. K., Archer, J. S., Abbott, D. F., and Jackson, G. D. (2015). Brain regions with abnormal network properties in severe epilepsy of Lennox-Gastaut phenotype: multivariate analysis of task-free fMRI. Epilepsia 56, 1767–1773. doi: 10.1111/epi.13135
Peter, J., Khosravi, M., Werner, T. J., and Alavi, A. (2018). Global temporal lobe asymmetry as a semi-quantitative imaging biomarker for temporal lobe epilepsy lateralization: a machine learning classification study. Hell. J. Nucl. Med. 21, 95–101. doi: 10.1967/s002449910800
Pitkanen, A., Loscher, W., Vezzani, A., Becker, A. J., Simonato, M., Lukasiuk, K., et al. (2016). Advances in the development of biomarkers for epilepsy. Lancet Neurol. 15, 843–856. doi: 10.1016/S1474-4422(16)00112-5
Plis, S. M., Hjelm, D. R., Salakhutdinov, R., Allen, E. A., Bockholt, H. J., Long, J. D., et al. (2014). Deep learning for neuroimaging: a validation study. Front. Neurosci. 8:229. doi: 10.3389/fnins.2014.00229
Pustina, D., Avants, B., Sperling, M., Gorniak, R., He, X., Doucet, G., et al. (2015). Predicting the laterality of temporal lobe epilepsy from PET, MRI, and DTI: a multimodal study. Neuroimage Clin. 9, 20–31. doi: 10.1016/j.nicl.2015.07.010
Rathore, C., and Radhakrishnan, K. (2015). Concept of epilepsy surgery and presurgical evaluation. Epileptic Disord. 17, 19–31,quiz31. doi: 10.1684/epd.2014.0720
Rudie, J. D., Colby, J. B., and Salamon, N. (2015). Machine learning classification of mesial temporal sclerosis in epilepsy patients. Epilepsy Res. 117, 63–69. doi: 10.1016/j.eplepsyres.2015.09.005
Shen, D., Wu, G., and Suk, H. I. (2017). Deep learning in medical image analysis. Annu. Rev. Biomed. Eng. 19, 221–248. doi: 10.1146/annurev-bioeng-071516-044442
Si, X., Zhang, X., Zhou, Y., Sun, Y., Jin, W., Yin, S., et al. (2020). Automated detection of juvenile myoclonic epilepsy using CNN based transfer learning in diffusion MRI. Annu. Int. Conf. IEEE Eng. Med. Biol. Soc. 2020, 1679–1682. doi: 10.1109/embc44109.2020.9175467
Sidhu, M. K., Duncan, J. S., and Sander, J. W. (2018). Neuroimaging in epilepsy. Curr. Opin. Neurol. 31, 371–378. doi: 10.1097/WCO.0000000000000568
Sinha, N., Wang, Y., Moreira da Silva, N., Miserocchi, A., McEvoy, A. W., de Tisi, J., et al. (2021). Structural brain network abnormalities and the probability of seizure recurrence after epilepsy surgery. Neurology 96, e758–e771. doi: 10.1212/wnl.0000000000011315
Snyder, K., Whitehead, E. P., Theodore, W. H., Zaghloul, K. A., Inati, S. J., and Inati, S. K. (2021). Distinguishing type II focal cortical dysplasias from normal cortex: a novel normative modeling approach. Neuroimage Clin. 30:102565. doi: 10.1016/j.nicl.2021.102565
Sone, D., Beheshti, I., Maikusa, N., Ota, M., Kimura, Y., Sato, N., et al. (2021). Neuroimaging-based brain-age prediction in diverse forms of epilepsy: a signature of psychosis and beyond. Mol. Psychiatry 26, 825–834. doi: 10.1038/s41380-019-0446-9
Tai, X. Y., Koepp, M., Duncan, J. S., Fox, N., Thompson, P., Baxendale, S., et al. (2016). Hyperphosphorylated tau in patients with refractory epilepsy correlates with cognitive decline: a study of temporal lobe resections. Brain 139(Pt 9), 2441–2455. doi: 10.1093/brain/aww187
Tan, Y. L., Kim, H., Lee, S., Tihan, T., Ver Hoef, L., Mueller, S. G., et al. (2018). Quantitative surface analysis of combined MRI and PET enhances detection of focal cortical dysplasias. Neuroimage 166, 10–18. doi: 10.1016/j.neuroimage.2017.10.065
Taylor, P. N., Sinha, N., Wang, Y., Vos, S. B., de Tisi, J., Miserocchi, A., et al. (2018). The impact of epilepsy surgery on the structural connectome and its relation to outcome. Neuroimage Clin. 18, 202–214. doi: 10.1016/j.nicl.2018.01.028
Torlay, L., Perrone-Bertolotti, M., Thomas, E., and Baciu, M. (2017). Machine learning-XGBoost analysis of language networks to classify patients with epilepsy. Brain Inform. 4, 159–169. doi: 10.1007/s40708-017-0065-7
Vasta, R., Cerasa, A., Sarica, A., Bartolini, E., Martino, I., Mari, F., et al. (2018). The application of artificial intelligence to understand the pathophysiological basis of psychogenic nonepileptic seizures. Epilepsy Behav. 87, 167–172. doi: 10.1016/j.yebeh.2018.09.008
Wagstyl, K., Adler, S., Pimpel, B., Chari, A., Seunarine, K., Lorio, S., et al. (2020). Planning stereoelectroencephalography using automated lesion detection: retrospective feasibility study. Epilepsia 61, 1406–1416. doi: 10.1111/epi.16574
Wang, J., Li, Y., Wang, Y., and Huang, W. (2018a). Multimodal data and machine learning for detecting specific biomarkers in pediatric epilepsy patients with generalized tonic-clonic seizures. Front. Neurol. 9:1038. doi: 10.3389/fneur.2018.01038
Wang, Y., Wei, W., Liu, Z., Liang, Y., Liu, X., Li, Y., et al. (2020). Predicting the type of tumor-related epilepsy in patients with low-grade gliomas: a radiomics study. Front. Oncol. 10:235. doi: 10.3389/fonc.2020.00235
Wang, Y., Zhou, Y., Wang, H., Cui, J., Nguchu, B. A., Zhang, X., et al. (2018b). Voxel-based automated detection of focal cortical dysplasia lesions using diffusion tensor imaging and T2-weighted MRI data. Epilepsy Behav. 84, 127–134. doi: 10.1016/j.yebeh.2018.04.005
Wiebe, S., Blume, W. T., Girvin, J. P., and Eliasziw, M. (2001). A randomized, controlled trial of surgery for temporal-lobe epilepsy. N. Engl. J. Med. 345, 311–318. doi: 10.1056/nejm200108023450501
Yang, Z., Choupan, J., Reutens, D., and Hocking, J. (2015). Lateralization of temporal lobe epilepsy based on resting-state functional magnetic resonance imaging and machine learning. Front. Neurol. 6:184. doi: 10.3389/fneur.2015.00184
Yao, L., Cai, M., Chen, Y., Shen, C., Shi, L., and Guo, Y. (2019). Prediction of antiepileptic drug treatment outcomes of patients with newly diagnosed epilepsy by machine learning. Epilepsy Behav. 96, 92–97. doi: 10.1016/j.yebeh.2019.04.006
Zhang, H., Schneider, T., Wheeler-Kingshott, C. A., and Alexander, D. C. (2012). NODDI: practical in vivo neurite orientation dispersion and density imaging of the human brain. Neuroimage 61, 1000–1016. doi: 10.1016/j.neuroimage.2012.03.072
Zhang, L., Wang, M., Liu, M., and Zhang, D. (2020). A survey on deep learning for neuroimaging-based brain disorder analysis. Front. Neurosci. 14:779. doi: 10.3389/fnins.2020.00779
Zhang, Q., Liao, Y., Wang, X., Zhang, T., Feng, J., Deng, J., et al. (2021). A deep learning framework for (18)F-FDG PET imaging diagnosis in pediatric patients with temporal lobe epilepsy. Eur. J. Nucl. Med. Mol. Imaging doi: 10.1007/s00259-020-05108-y [Epub ahead of print].
Zhang, W., Muravina, V., Azencott, R., Chu, Z. D., and Paldino, M. J. (2018). Mutual information better quantifies brain network architecture in children with epilepsy. Comput. Math. Methods Med. 2018:6142898. doi: 10.1155/2018/6142898
Zhang, Y., Yan, P., Liang, F., Ma, C., Liang, S., and Jiang, C. (2019). Predictors of epilepsy presentation in unruptured brain arteriovenous malformations: a quantitative evaluation of location and radiomics features on T2-weighted imaging. World Neurosurg. 125, e1008–e1015. doi: 10.1016/j.wneu.2019.01.229
Keywords: machine learning (ML), epilepsy, neuroimaging, magnetic resonance imaging, positron emission tomography (PET)
Citation: Sone D and Beheshti I (2021) Clinical Application of Machine Learning Models for Brain Imaging in Epilepsy: A Review. Front. Neurosci. 15:684825. doi: 10.3389/fnins.2021.684825
Received: 24 March 2021; Accepted: 31 May 2021;
Published: 22 June 2021.
Edited by:
Fahmi Khalifa, Mansoura University, EgyptReviewed by:
Zulfi Haneef, Baylor College of Medicine, United StatesHisham Abdeltawab, University of Louisville, United States
Kamal Hammouda, University of Louisville, United States
Aydin Akan, İzmir University of Economics, Turkey
Copyright © 2021 Sone and Beheshti. This is an open-access article distributed under the terms of the Creative Commons Attribution License (CC BY). The use, distribution or reproduction in other forums is permitted, provided the original author(s) and the copyright owner(s) are credited and that the original publication in this journal is cited, in accordance with accepted academic practice. No use, distribution or reproduction is permitted which does not comply with these terms.
*Correspondence: Daichi Sone, d.sone@ucl.ac.uk
†These authors have contributed equally to this work