- 1Department of Epidemiology and Biostatistics, School of Public Health, Xuzhou Medical University, Xuzhou, China
- 2Center for Medical Statistics and Data Analysis, School of Public Health, Xuzhou Medical University, Xuzhou, China
- 3Key Laboratory of Human Genetics and Environmental Medicine, Xuzhou Medical University, Xuzhou, China
Background: Neurodegenerative diseases (NDDs) are the leading cause of disability worldwide while their metabolic pathogenesis is unclear. Genome-wide association studies (GWASs) offer an unprecedented opportunity to untangle the relationship between metabolites and NDDs.
Methods: By leveraging two-sample Mendelian randomization (MR) approaches and relying on GWASs summary statistics, we here explore the causal association between 486 metabolites and five NDDs including Alzheimer’s Disease (AD), amyotrophic lateral sclerosis (ALS), frontotemporal dementia (FTD), Parkinson’s disease (PD), and multiple sclerosis (MS). We validated our MR results with extensive sensitive analyses including MR-PRESSO and MR-Egger regression. We also performed linkage disequilibrium score regression (LDSC) and colocalization analyses to distinguish causal metabolite-NDD associations from genetic correlation and LD confounding of shared causal genetic variants. Finally, a metabolic pathway analysis was further conducted to identify potential metabolite pathways.
Results: We detected 164 metabolites which were suggestively associated with the risk of NDDs. Particularly, 2-methoxyacetaminophen sulfate substantially affected ALS (OR = 0.971, 95%CIs: 0.961 ∼ 0.982, FDR = 1.04E-4) and FTD (OR = 0.924, 95%CIs: 0.885 ∼ 0.964, FDR = 0.048), and X-11529 (OR = 1.604, 95%CIs: 1.250 ∼ 2.059, FDR = 0.048) and X-13429 (OR = 2.284, 95%CIs: 1.457 ∼ 3.581, FDR = 0.048) significantly impacted FTD. These associations were further confirmed by the weighted median and maximum likelihood methods, with MR-PRESSO and the MR-Egger regression removing the possibility of pleiotropy. We also observed that ALS or FTD can alter the metabolite levels, including ALS and FTD on 2-methoxyacetaminophen sulfate. The LDSC and colocalization analyses showed that none of the identified associations could be driven by genetic correlation or confounding by LD with common causal loci. Multiple metabolic pathways were found to be involved in NDDs, such as “urea cycle” (P = 0.036), “arginine biosynthesis” (P = 0.004) on AD and “phenylalanine, tyrosine and tryptophan biosynthesis” (P = 0.046) on ALS.
Conclusion: our study reveals robust bidirectional causal associations between servaral metabolites and neurodegenerative diseases, and provides a novel insight into metabolic mechanism for pathogenesis and therapeutic strategies of these diseases.
Background
Neurodegenerative diseases (NDDs; Blennow et al., 2010; Parnetti, 2011), such as Alzheimer’s disease (AD), amyotrophic lateral sclerosis (ALS), frontotemporal dementia (FTD), Parkinson’s disease (PD), and multiple sclerosis (MS), are a prominent group of progressive and fatal neurological diseases currently without an effective cure, representing one of the fastest and largest increasing categories of the global disease burden especially because of aging populations (Roth et al., 2018; Bakhta et al., 2019). Therefore, identifying potential biomarkers for early diagnosis and unraveling risk factors for prevention and treatment become critical in the clinic. Although great advances have been made in discovering biomarkers and risk factors for various NDDs over the past few years (Trojanowski, 2000; Barnham et al., 2004; Emerit et al., 2004; Rachakonda et al., 2004; Amor et al., 2010; Blennow et al., 2010; Parnetti, 2011; Doty, 2017; Leng et al., 2019), the knowledge regarding the physiological and pathological mechanism underlying these diseases remains largely unclear.
As part of efforts to understand such mechanism, the relationship between metabolites and NDDs has been attracted active research attention (Wang et al., 2012; Jové et al., 2014; To et al., 2019; Chatterjee et al., 2020; Shang et al., 2020). Metabolites are the intermediate or end products that drive essential biological functions of human bodies and reflect the physiological and pathological disease phenotypes (Johnson et al., 2016; Shang et al., 2020). There is also a growing literature indicating that profiling metabolites in biofluids offers deep insights into biomarkers of NDDs (Wang et al., 2012; Mendelsohn and Larrick, 2013; Jové et al., 2014; González-Domínguez et al., 2015; Kori et al., 2016; To et al., 2019; Chatterjee et al., 2020; Shang et al., 2020). For example, it was demonstrated lipids and amino acids were associated with cognitive decline and the progression of dementia (Jiang et al., 2019); primary fatty amides in plasma were associated with brain amyloid burden and memory (Kim et al., 2019); blood metabolite changes in the periphery reflected the asymptomatic, prodromal and symptomatic stages of memory and AD (Kiddle et al., 2014; Mapstone et al., 2014). However, due to unknown confounders and reverse causality, these findings obtained from observational studies remain problematical as to whether metabolites are subsequent or consequent to NDDs, and it is also unknown whether there is a definite association between metabolites and NDDs, and whether such relationship is causal.
Recent advances in statistical genetic approaches of causal inference, along with publicly available summary statistics from large-scale genome-wide association studies (GWASs) of metabolites and NDDs provide an unprecedented opportunity to systematically evaluate their relationship through Mendelian randomization (MR; Greenland, 2000; Thomas and Conti, 2004; Tobin et al., 2004; Wheatley and Gray, 2004; Evans and Davey Smith, 2015; Zeng et al., 2019; Zeng and Zhou, 2019b; Yu et al., 2020a,b). In brief, MR is an instrumental-variable based method, which performs the causal inference with single nucleotide polymorphisms (SNPs) as instrumental variables to assess the causal effect of an exposure of focus (i.e., metabolite) on an outcome (i.e., ALS). The attractive strength of MR is that it is often less susceptible to reverse causation and confounders compared to other study designs since the two alleles of an SNP are randomly segregated under the Mendel’s law and such segregation can be considered to be independent of many unmeasured or unknown confounders. More importantly, MR can be implemented with only publicly available summary statistics of the exposure and outcome rather than individual-level genotypes and phenotypes, circumventing privacy concerns stemming from data sharing (Pasaniuc and Price, 2016). Therefore, over the past few years MR has been widely applied to disentangle the causal relationship between an exposure and an outcome in various application fields (Greenland, 2000; Thomas and Conti, 2004; Tobin et al., 2004; Wheatley and Gray, 2004; Evans and Davey Smith, 2015; Davies et al., 2018; Zeng et al., 2019; Zeng and Zhou, 2019b; Yu et al., 2020a,b).
Hereby making full use of the latest GWAS summary statistics of 486 metabolites and five major NDDs, we conducted a two-sample MR analysis to assess the causal effects of metabolites on these diseases or vice versa. Extensive sensitivity analyses, including linkage disequilibrium (LD) score regression (LDSC) and colocalization analysis (Giambartolomei et al., 2014), were also implemented to investigate whether the MR findings could be driven by genetic similarity or confounding due to LD with causal genetic loci. Overall, we found that there existed a bidirectional causal association between three metabolites and two types of NDDs (i.e., ALS and FTD). We further demonstrated that the identified associations were robust against used MR approaches and instrumental pleiotropy, and were not likely driven by shared genetic components or confounded by LD with common causal SNPs. We also identified multiple significant metabolic pathways that might be involved in the development of NDDs.
Materials and Methods
Genome-Wide Association Study Data Sources
We obtained summary statistics of metabolites from the metabolomics GWAS (Shin et al., 2014), which was the most comprehensive study performed to date on human blood metabolites and was a meta-analysis of two cohorts including TwinsUK and KORA F4. After quality control, a total of 486 metabolites and approximately 2.1 million SNPs up to 7,824 individuals of European ancestry were reserved for analysis (Shin et al., 2014). These metabolites can be classified as being known (309) or unknown (177). These unknown metabolites indicated that their chemical identity had not yet been conclusively established. All known metabolites can be further classified into eight broad metabolic groups (i.e., amino acid, carbohydrate, cofactors and vitamin, energy, lipid, nucleotide, peptide, and xenobiotic metabolism) (Kanehisa et al., 2012). The association of every genetic variant with individual metabolites was analyzed using a linear additive regression with age and sex as covariates.
In addition, we yielded European-only summary statistics of five NDDs (Table 1), including AD (Jansen et al., 2019), ALS (Nicolas et al., 2018), FTD (Ferrari et al., 2014), PD (Nalls et al., 2019), and MS (International Multiple Sclerosis Genetics Consortium, 2019). The Manhattan and QQ plots of P-values are shown in Supplementary Figures 1, 2. Although a marked departure is observed in the QQ plots of some diseases (i.e., λ = 1.13 for MS), the estimated λ1000 and the intercept obtained by LDSC (Bulik-Sullivan et al., 2015) indicate that the observed inflation is mainly due to polygenic signals rather than confounding factors such as population stratification or cryptic relatedness (Supplementary Table 1). Therefore, the genomic control for test statistics is not necessary for of these NDDs.
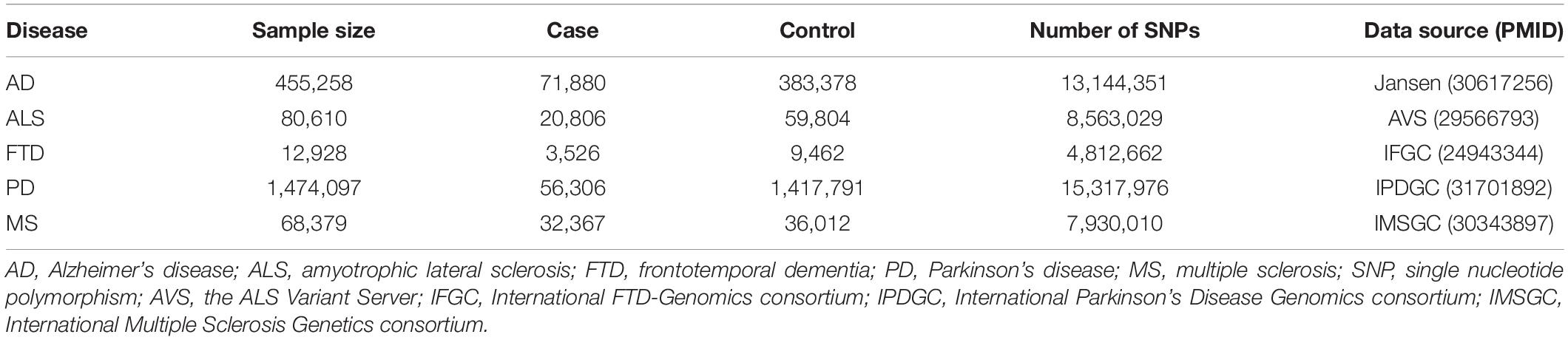
Table 1. Genome-wide association study data sets of neurodegenerative diseases employed in our analysis.
Selection of Instrumental Variables
We generated a set of uncorrelated index SNPs serving as instrumental variables for each of metabolites using the clumping procedure of PLINK (version v1.90b3.38) (Purcell et al., 2007). Specifically, we set both the primary significance level and the secondary significance level for index SNPs to be 1.00E-5, the LD, and the physical distance to be 0.10 and 500kb, respectively. Genotypes of 503 European individuals from the 1000 Genomes Project were applied as the reference panel during clumping (The 1000 Genomes Project Consortium, 2015). The relaxed statistical threshold of 1.00E-5 was employed here because of the relatively small sample size of the metabolite GWAS. In practical MR studies, a smaller significance threshold (e.g., 1.00E-5) was generally used to explain a larger variation for power enhancement when few SNPs were available for the exposure at the genome-wide significance level of 5.00E-8 (Sanna et al., 2019). In addition, to avoid horizontal pleiotropy in instrumental variables, we further removed index SNPs that were located within 1 Mb of disease-associated loci and that may be potentially related to a given neurodegenerative disease if selected genetic variants had a Bonferroni-adjusted P-value less than 0.05 for that disease. This was a conservative manner protecting against the pleiotropic impact of instruments to ensure valid causal inference in MR analysis (Ahmad et al., 2015; Larsson et al., 2017; Zeng et al., 2019; Zeng and Zhou, 2019a; Yu et al., 2020a).
Causal Effect Estimation With Inverse-Variance-Weighted Mendelian Randomization Methods
For each index SNP that was utilized as instrumental variable in turn, we first examined whether it was strongly associated with the metabolite. To do so, we calculated the proportion of phenotypic variance of metabolite explained (PVE) by instruments using summary statistics using the approach proposed in Shim et al. (2015) and computed the F statistic to quantitatively measure the strength of instruments (Cragg and Donald, 1993; Burgess et al., 2017). The Cochran’s Q test was applied to examine the heterogeneity in effect sizes of instruments to determine whether fixed or random-effects IVW method would be utilized (Thompson and Sharp, 1999). We then undertook the inverse-variance-weighted (IVW) MR analysis for one metabolite at a time to estimate its causal effect on each of the five diseases (Burgess et al., 2017; Hartwig et al., 2017; Yavorska and Burgess, 2017). We declared an association to be statistically significant if the false discovery rate (FDR) <0.05 (Benjamini and Hochberg, 1995).
Sensitivity Analyses for Identified Causal Associations
Based on the IVW MR analysis, we discovered several causal associations between metabolites and NDDs. However, these significant associations may reflect four explanations including: (1) causality from metabolites to NDDs, which indicates that the metabolites are risk factors related to the diseases; (2) reverse causality from NDDs to metabolites, which implies that the metabolites are biomarkers of the diseases; (3) undetected horizontal pleiotropy, which suggests the diseases and the metabolites may share common genetic foundation; and (4) confounding by LD among leading causal SNPs shared by metabolites and NDDs, which means that the observed associations are spurious (Supplementary Figure 3). Therefore, it is of importance to untangle the causal association from other explanations. To this aim, for each identified association we further implemented a series of sensitivity analyses: (i) the weighted median-based method (Bowden et al., 2016b) and the maximum likelihood method (Burgess et al., 2013) to evaluate the robustness of the discovered associations; (ii) the MR-Egger regression (Bowden et al., 2016a; Burgess and Thompson, 2017) to detect directional pleiotropic effects of instruments; (iii) the MR-PRESSO test to assess horizontal pleiotropy and examine potential instrumental outliers (Verbanck et al., 2018); (iv) the reverse causality analysis with one of NDDs as the exposure and the identified metabolite as the outcome; the instrumental variables of these NDDs were selected via the similar PLINK clumping procedure described above but with a genome-wide significance level of 5E-8 (Supplementary Material); (v) the multivariable MR analysis to examine the independent relationship between one associated metabolite and NDDs while adjusting for the effects of other associated metabolites (Burgess et al., 2015); (vi) LDSC to assess the overall genetic correlation (rg) using all available SNPs; (vii) the colocalization analysis to investigate whether the identified association between metabolites and NDDs was attributable to shared causal genetic variants (Giambartolomei et al., 2014; Supplementary Material).
Metabolic Pathway Analysis
We finally conducted a metabolic pathway analysis for identified metabolites via MetaboAnalyst (Chong et al., 2018) and exploited the functional enrichment analysis module to search potential metabolite pathways for metabolites that might be involved in biological processes of the five NDDs analyzed here. Our metabolic pathway analysis included two datasets: 99 metabolite sets from The Small Molecule Pathway Database (SMPDB; Frolkis et al., 2010) and 84 metabolite sets from the KEGG database (Kanehisa et al., 2012). SMPDB is designed to support pathway discovery in clinical metabolomics, transcriptomics, proteomics, and systems biology, and further provides diagrams of human metabolic and metabolite signaling pathways.
Results
Causal Effects of the 486 Blood Metabolites on Neurodegenerative Diseases
The number of instrumental variables of metabolite varied from 4 to 585, with a median number of 29. These instrumental variables on average explained approximately 38.4% of phenotypic variance across all metabolites, and the minimum F statistic among all instruments was 20.8, indicating that weak instrumental bias is unlikely to occur and the used instruments for these metabolites were sufficiently informative (F statistic >10) for our MR analysis (Supplementary Table 2). Based on these instrumental variables we implemented the IVW MR analysis for each pair of metabolites and NDDs (Supplementary Table 2). We identified a total of 164 suggestive associations (136 unique metabolites) (P < 0.05), including 99 associations for 85 known metabolites and 65 associations for 51 unknown metabolites (Supplementary Table 3). Among these, 18, 23, 20, 20, and 18 associations of known metabolites (Figure 1) and 15, 12, 14, 13, and 11 associations of unknown metabolites (Supplementary Figure 4) are detected to be associated with the risk of AD, ALS, FTD, PD, and MS, respectively. However, after correcting multiple comparisons, we only obtain four significant associations (FDR < 0.05; involving three metabolites), including 2-methoxyacetaminophen sulfate with ALS [odd ratio (OR) = 0.971, 95% confidence intervals [CIs]: 0.961 ∼ 0.982, FDR = 1.04E-4] and FTD (OR = 0.924, 95%CIs: 0.885 ∼ 0.964, FDR = 0.048); X-11529 (OR = 1.604, 95%CIs: 1.250 ∼ 2.059, FDR = 0.048) and X-13429 (OR = 2.284, 95%CIs: 1.457 ∼ 3.581, FDR = 0.048) with FTD. Furthermore, we used a stricter r2 threshold of <0.001 in the clumping procedure to avoid the inflation of test statistics. As a result, it is shown that the four associations are still significant, with consistent effects in direction magnitude (Supplementary Table 4). 2-methoxyacetaminophen sulfate, also known as 4-(acetylamino)-3-methoxyphenyl hydrogen sulfate, is a member of the acetamide class and has a role as a drug metabolite (Mrochek et al., 1974; Bozzoni et al., 2016). Acetamides have long been recognized to be related to levels of glutathione and N-acetylcysteine, which are known biomarkers for ALS (Przedborski et al., 1996) and other NDDs such as AD (Saharan and Mandal, 2014).
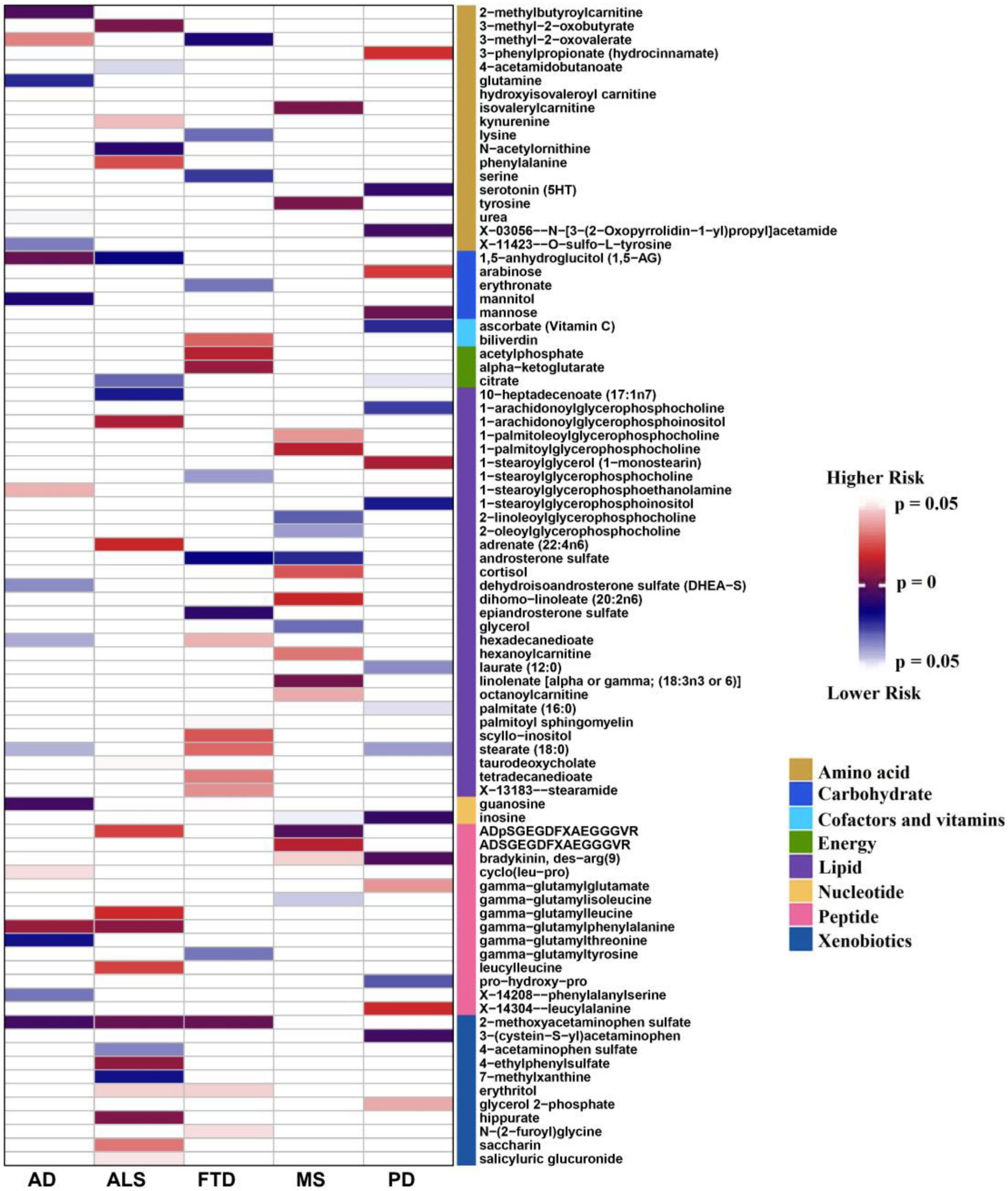
Figure 1. Identified causal associations between known metabolites and the risk of five neurodegenerative diseases using the IVW MR analysis. IVW, inverse-variance weighted; AD: Alzheimer’s disease; ALS: amyotrophic lateral sclerosis; FTD: frontotemporal dementia; PD: Parkinson’s disease; MS: multiple sclerosis.
It is worth noting that 2-methoxyacetaminophen sulfate also exhibits a suggestive association with AD (OR = 0.998, 95%CIs: 0.996 ∼ 0.999, P = 0.006), implying shared metabolic mechanisms might exist among these NDDs. Moreover, 12 metabolites are suggestively related to at least two NDDs (P < 0.05) (Table 2). For example, besides 2-methoxyacetaminophen sulfate, 3-methyl-2-oxovalerate is also identified to be associated with both AD and FTD. In addition, we find the direction of causal effect sizes of some metabolites is inconsistent across NDDs, including 3-methyl-2-oxovalerate on AD (OR = 1.075, 95%CIs: 1.007 ∼ 1.148, P = 0.031) and FTD (OR = 0.148, 95%CIs: 0.033 ∼ 0.662, P = 0.012), hexadecanedioate on AD (OR = 0.971, 95%CIs: 0.945 ∼ 0.999, P = 0.039) and FTD (OR = 1.865, 95%CIs: 1.034 ∼ 3.362, P = 0.038), stearate (18:0) on AD (OR = 0.932, 95%CIs: 0.872 ∼ 0.997, P = 0.040) and FTD (OR = 4.780, 95%CIs: 1.193 ∼ 19.150, P = 0.027), ADpSGEGDFXAEGGGVR on ALS (OR = 1.363, 95%CIs: 1.048 ∼ 1.773, P = 0.021) and MS (OR = 0.580, 95%CIs: 0.407 ∼ 0.828, P = 0.003), suggesting potentially different functional roles of these metabolites implicated in NDDs. In brief, these findings provide important knowledge for understanding the metabolic mechanism underlying the relationship between metabolites and NDDs.
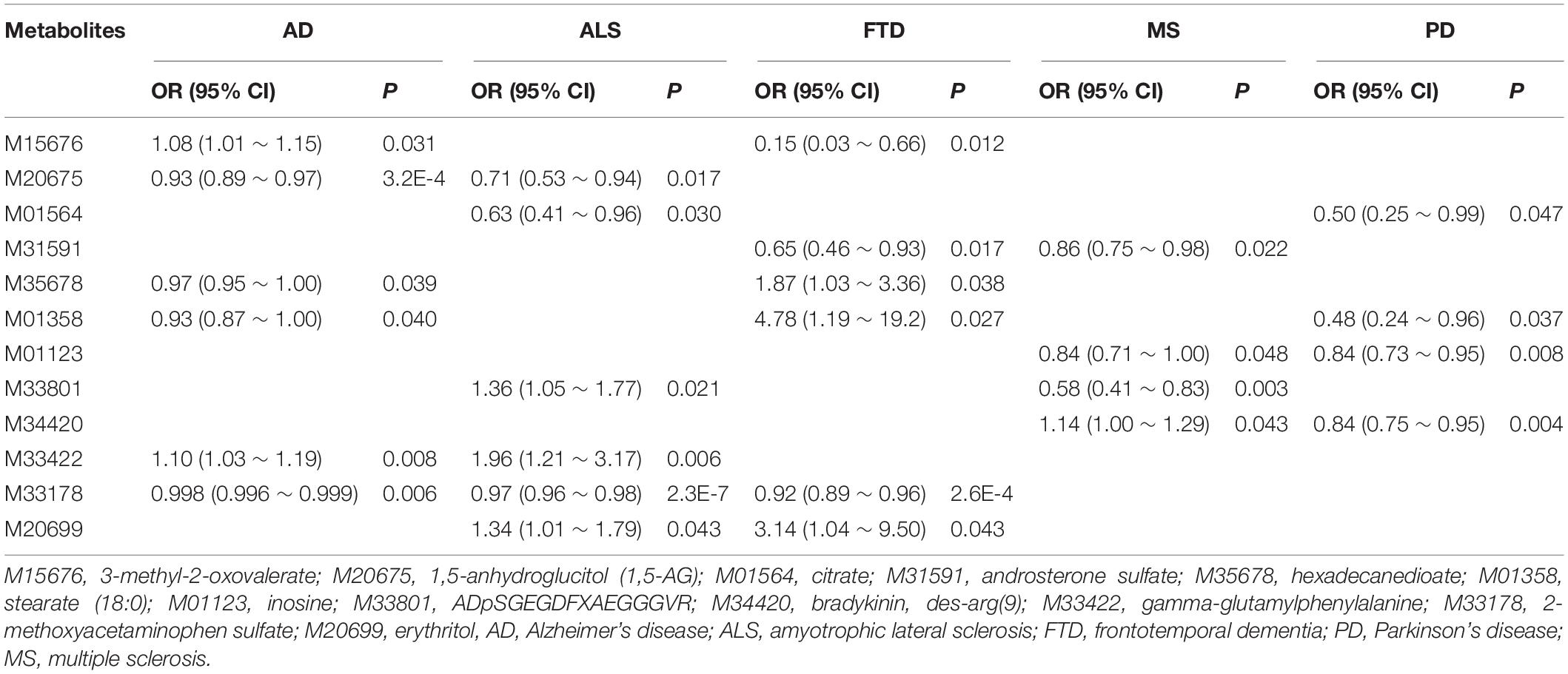
Table 2. Overlapped known metabolites existing causal association with at least two neurodegenerative diseases.
Results of Sensitivity Analyses
To evaluate the influence of horizontal pleiotropy on our MR estimates, we conducted sensitivity and pleiotropy analyses to examine the robustness of the four associations discovered above. Here we only show some important findings, with more complete and detailed results demonstrated in the Supplementary Material. First, it is displayed that these causal associations are robust against other MR methods except the MR-Egger regression (Figure 2 and Supplementary Table 5). This might be that the MR-Egger method is substantially less efficient than other methods since it was proposed based on weaker modeling assumptions in causal inference. Second, the MR-PRESSO analysis offers little evidence for the presence of horizontal pleiotropy (PMR–PRESSO global > 0.05) and instrumental outliers (P > 0.05) (Supplementary Figures 5, 6). Third, the intercept of MR-Egger is not significantly deviated from zero, also indicating the absence of apparent horizontal pleiotropy (Supplementary Table 5). For the three associated metabolites identified above, to study whether the causal effect of one metabolite on FTD can be affected by the other two, we performed the multivariable MR analysis and find that, except X-11529, the direction and magnitude of the causal effects of other two metabolites (i.e., 2-methoxyacetaminophen sulfate and X-13429) are almost consistent with the unadjusted ones obtained via the IVW method (Supplementary Table 6), partly suggesting the independent role of these two metabolites in the risk of FTD.
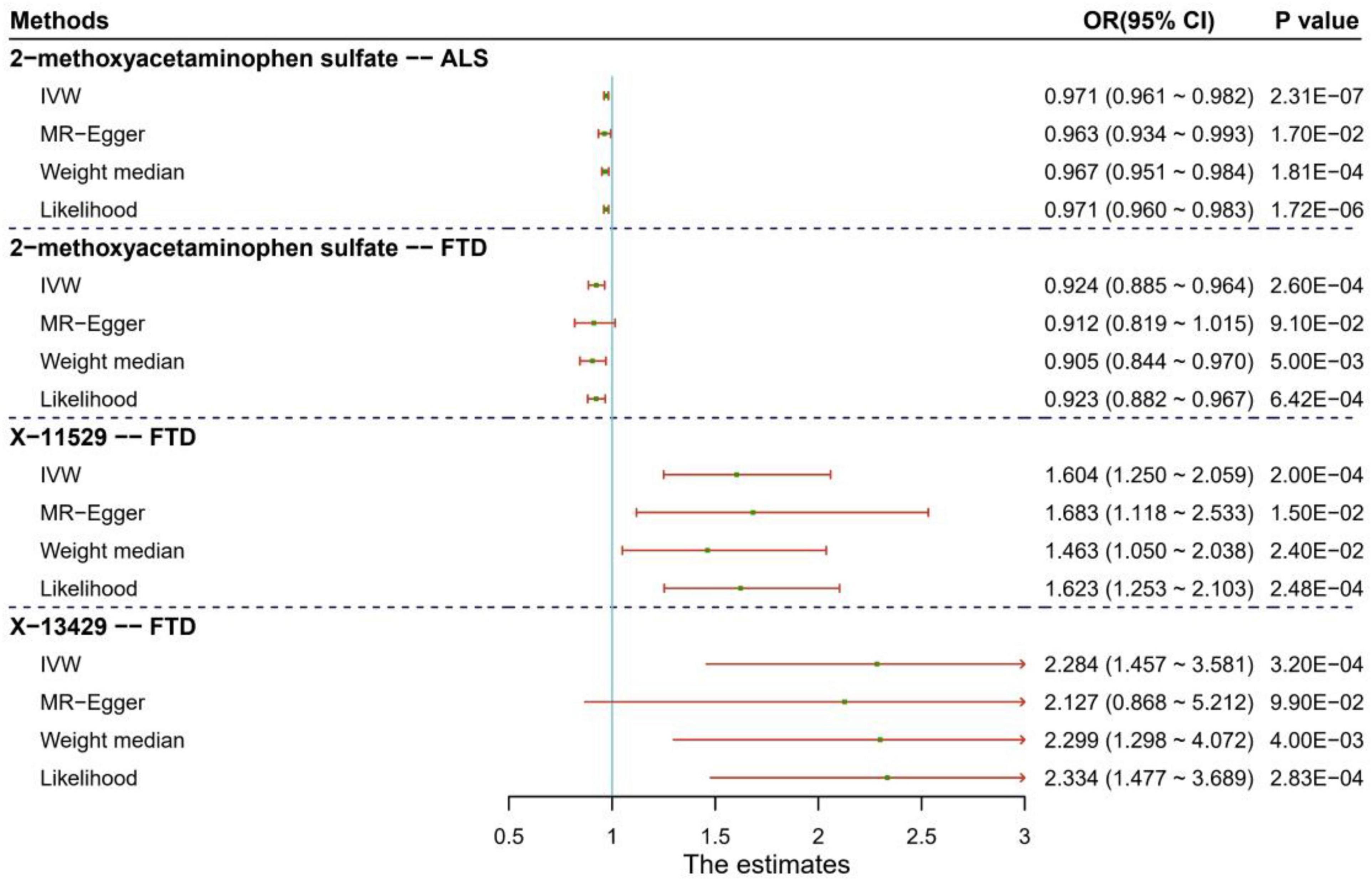
Figure 2. Results of sensitivity analysis for the four significant causal associations identified between human blood metabolites and two types of neurodegenerative diseases (e.g., ALS and FTD). ALS: amyotrophic lateral sclerosis; FTD: frontotemporal dementia.
We report another nine metabolites which have suggestive associations with the five NDDs (P < 0.05) in Table 3. For these associations, we also do not discover any evidence supporting horizontal pleiotropy, such as glutamine on AD (PMR–Egger Intercept = 0.065, and PMR–PRESSO global = 0.444), guanosine on AD (PMR–Egger intercept = 0.244, and PMR–PRESSO global = 0.739), mannose on PD (PMR–Egger intercept = 0.260, and PMR–PRESSO global = 0.162), citrate on PD (PMR–Egger intercept = 0.115, and PMR–PRESSO global = 0.408) and isovalerylcarnitine on MS (PMR–Egger intercept = 0.222, and PMR–PRESSO global = 0.410).
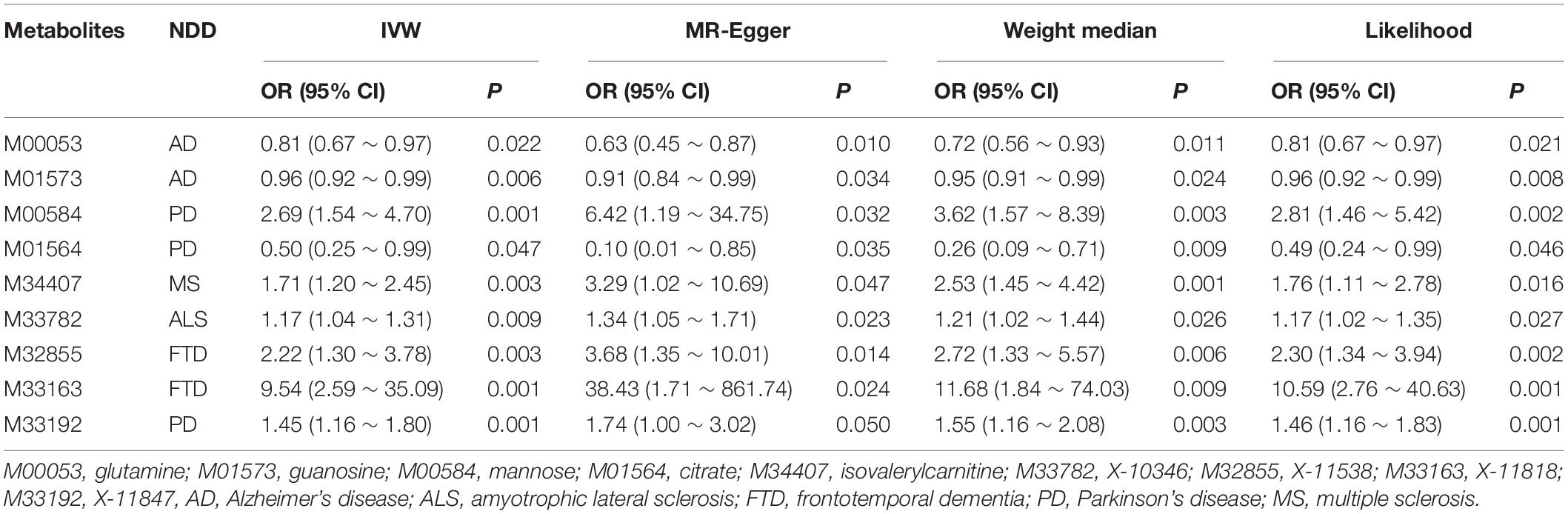
Table 3. Suggestive association metabolites passing all MR analyses at the nomial significance level of 0.05.
Bidirectional Mendelian Randomization Examining Reverse Association From Amyotrophic Lateral Sclerosis/Frontotemporal Dementia to Metabolites
For the four significant associations between metabolites and ALS/FTD identified above, we further carried out a MR analysis using instrumental variables of ALS/FTD to estimate their reverse causal effects on metabolites (Supplementary Material). We observe that ALS/FTD also can alter the level of metabolites at the nominal significance level of 0.05 (Supplementary Table 7), such as ALS on 2-methoxyacetaminophen sulfate (OR = 0.838, 95%CIs: 0.722 ∼ 0.972, P = 0.020), FTD on 2-methoxyacetaminophen sulfate (OR = 1.050, 95%CIs: 1.007 ∼ 1.095, P = 0.021), and FTD on X-11529 (OR = 0.976, 95%CIs: 0.961 ∼ 0.991, P = 0.002), implying that the emergence of possible bidirectional causal relationships between these metabolites and ALS/FTD.
In this reverse MR analysis, similar results are also generated by other MR tests (PLikelihood = 0.022 and PMR–PRESSO = 0.038 for ALS on 2-methoxyacetaminophen sulfate; PWeight–median = 0.030, PLikelihood = 0.021 and PMR–PRESSO = 3.44E-05 for FTD on 2-methoxyacetaminophen sulfate; PWeight–median = 0.009, PLikelihood = 0.002 and PMR–PRESSO = 0.001 for FTD on X-11529). Moreover, there is no evidence of horizontal pleiotropy for any association (PMR–Egger intercept = 0.975 and PMR–PRESSO global = 0.558 for ALS on 2-methoxyacetaminophen sulfate; PMR–Egger intercept = 0.416 and PMR–PRESSO global = 0.999 for FTD on 2-methoxyacetaminophen sulfate; PMR–Egger intercept = 0.347 and PMR–PRESSO global = 0.918 for FTD on X-11529) (Supplementary Table 7 and Supplementary Figures 7, 8).
Causal Association Among Identified Metabolites
In order to acquire a much deeper insight into the association between the three metabolites and ALS/FTD, we performed an additional MR analysis to investigate the presence of causal relationship among these metabolites. Of interest, we ultimately observe several interaction associations between them (Supplementary Table 8), such as 2-methoxyacetaminophen sulfate on X-11529 (β = 0.012, 95%CIs: 0.002 ∼ 0.021, P = 0.012), X-11529 on X-13429 (β = 0.500, 95%CIs: 0.463 ∼ 0.537, P = 1.44E-153), suggesting that metabolites may interact to affect NDDs (Figure 3).
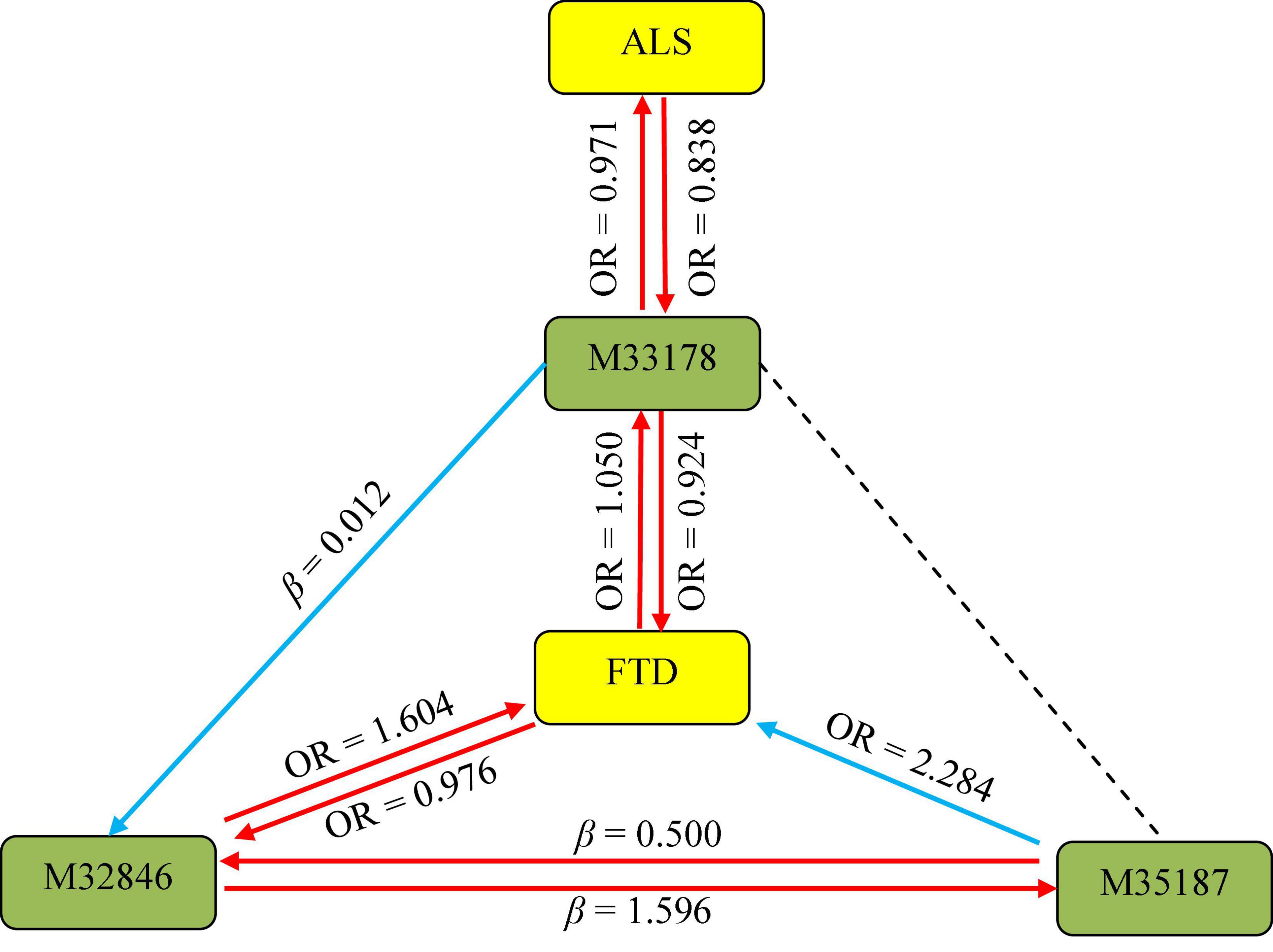
Figure 3. Association pathways between three metabolites and ALS/FTD with MR analysis. M33178: 2-methoxyacetaminophen sulfate; M32846: X-11529; M35187: X-13429; ALS: amyotrophic lateral sclerosis; FTD: frontotemporal dementia. The solid arrow stands for the presence of the association, while the dot line represents the absence of the association.
Genetic Correlation and Colocalization Analyses
To examine the alternative explanation of common genetic component, we undertook the LDSC and colocalization analyses to investigate whether the genetic associations underlying metabolites and ALS/FTD were likely due to shared causal genetic variants (Supplementary Figure 3). In terms of LDSC (Supplementary Table 9), we do not observe the existence of substantial genetic correlations (rg = −0.107 and P = 0.370 between 2-methoxyacetaminophen sulfate and ALS; rg = 0.116 and P = 0.589 between 2-methoxyacetaminophen sulfate and FTD; rg = −0.151 and P = 0.771 between X-11529 and FTD; and rg = −0.224 and P = 0.675 between X-13429 and FTD). However, we cannot fully rule out the possibility of low statistical power due to small sample sizes of metabolites. Furthermore, upon performing colocalization analysis (Supplementary Table 10), we do not find any evidence of colocalization for the four associations (the posterior probability that both metabolite and NDDs are associated with common causal genetic variants < 80%), suggesting that none of these MR findings could be driven by genetic confounding by LD with causal SNPs.
Metabolic Pathway Analysis
We were also interested in elucidating plausible metabolic pathways for the five NDDs. Therefore, we further carried out the metabolic pathway analysis using all metabolites discovered through the IVW approach (P < 0.05), and identified seven significant metabolic pathways for these diseases except PD (Table 4). Among them, three pathways are separately related to ALS, FTD and MS, and four are associated with AD, including “urea cycle” (P = 0.036), “arginine biosynthesis” (P = 0.004), “purine metabolism” (P = 0.009), and “D-glutamine and D-glutamate metabolism” (P = 0.042). There exists a common metabolic pathway (i.e., phenylalanine, tyrosine and tryptophan biosynthesis) shared by ALS (P = 0.046) and MS (P = 0.023).
Discussion
Leveraging genetic variants as proxies, in the present work we assessed the causal relationship between many metabolites and five NDDs using various statistical methods (Greenland, 2000; Thomas and Conti, 2004; Tobin et al., 2004; Wheatley and Gray, 2004; Evans and Davey Smith, 2015; Davies et al., 2018; Zeng et al., 2019; Zeng and Zhou, 2019b; Yu et al., 2020a,b). Totally, we discovered 164 suggestive associations, among which four were statistically significant for three metabolites, including 2-methoxyacetaminophen sulfate (known metabolite) affecting ALS and FTD, as well as X-11529 and X-13429 (unknown metabolites) affecting FTD. Genetic studies have revealed that ALS and FTD share a high extent of common genetic origin (Wood, 2011). If 2-methoxyacetaminophen sulfate is considered a promising metabolite that has an effect on both of the two diseases, then, 2-methoxyacetaminophen sulfate can be treated as a therapeutic biomarker of diseases. Due to limited understanding of the role of this metabolite in the specific pathophysiological mechanism of ALS and FTD, this would be a suggestive finding. We further demonstrated that there also existed a reverse association between these metabolites and ALS/FTD, implying a bidirectional influence on each other. Extensive sensitivity analyses showed that these associations were robust against used MR approaches and instrumental pleiotropy, and were not likely driven by shared genetic components or confounded by LD with common causal SNPs. In addition, we found these identified metabolites interacted to influence each other, implying that there might exist a complicated network among metabolites which impact NDDs in both direct and indirect manners. It needs to highlight that a complete investigation of such role of metabolites in NDDs is beyond the scope of this work as it requires additional methodological development, which we leave for future study. Furthermore, seven significant metabolic pathways involved in the five NDDs were also detected. Our metabolic pathway analysis showed that “phenylalanine, tyrosine and tryptophan biosynthesis” was associated with both ALS and MS. It should be noted that the onset age of MS is mainly 20–40 years old (Klineova and Lublin, 2018), which is relatively younger than the average onset age of ALS. Whether phenylalanine has a mediation effect between ALS and MS needs to be further studied. In prior literature, tryptophan and competing neutral amino acid levels were found to be diminished in the plasma of patients with neurodegenerative diseases, the greatest decrease being of tryptophan (Monaco et al., 1979; Zhang et al., 2020). Evidence was also shown that higher serum phenylalanine concentrations related to immune activation are detectable in a subgroup of AD patients (Wissmann et al., 2013). The paired conversion of phenylalanine may affect not only the production of tyrosine but also the biosynthesis of the neurotransmitters dopamine, norepinephrine and epinephrine (Fernstrom and Fernstrom, 2007).
In conclusion, our findings provide an insightful perspective into the understanding of the relationship between metabolites and NDDs, and have important implications for pathology, drug development and clinical treatment. For example, given few effective drugs are available for these diseases (Al-Chalabi and Hardiman, 2013), these identified metabolites may be prioritized as candidate therapeutic targets for NDDs (Cummings et al., 2014; Lu et al., 2016), especially 2-methoxyacetaminophen sulfate for ALS or FTD. On the other hand, in terms of the findings in the reverse causality analysis, metabolites such as 2-methoxyacetaminophen sulfate can also serve as predictive biomarkers for the development of NDDs. Our study also found that some NDDs might share common metabolic mechanism, suggesting that multiple similar etiological pathways may give rise to different clinical manifestations (e.g., hexadecanedioate on AD and FTD) (Couratier et al., 2017; Ferrari et al., 2017; Broce et al., 2018; Karch et al., 2018; Abramzon et al., 2020). Overall, our study presents robust associations between multiple metabolites and NDDs, indicating the avenue for follow-up studies to improve the diagnostics, prevention and treatment of NDDs.
There are several strengths to our study. First, because it depends only on publicly available summary statistics rather than individual-level datasets, in the current work we can undertake a comprehensive MR causal inference evaluating the relationship between a large number of metabolites and NDDs in an unprecedented manner. Second, methodologically, in contrast to previous observational studies, our MR analysis implemented instrumental variable-based causal inference to assess the association between metabolites and NDDs, while minimizing the possibility of bias due to unknown confounding. Third, to provide robust MR assessment for identified associations, a wide range of sensitivity analyses were performed to distinguish causal effects from horizontal pleiotropy, reverse causation, and genetic confounding. However, this study also has some limitations. First, MR studies are generally recommended to be implemented using GWASs with large sample size; however, in our work the metabolite GWAS had a relatively small sample size, which may undermine the validity of our MR findings. Second, our study identified bidirectional causal relationships between metabolites (such as 2-methoxyacetaminophen sulfate) and NDDs (such as ALS); however, the small effect size would limit its potential utility as important biomarkers or therapeutic targets in practice. Third, we used a multivariate MR analysis to investigate whether metabolites interacted with each other to influence the causal effect of disease, but this method may be not unsuitable for unknown pleiotropy (Burgess et al., 2015). Fourth, metabolites levels are known to differ among cell and tissue types (Holmes et al., 2008; Wang et al., 2012; Shin et al., 2014); however, in this study we can only evaluate the influence of metabolites measured in blood on NDDs but unable to assess the relevance of metabolites levels in more biologically relevant tissues such as brain.
Conclusion
In summary, our study reveals robust bidirectional causal associations between servaral metabolites and neurodegenerative diseases, and provides a novel insight into metabolic mechanism for pathogenesis and therapeutic strategies of these diseases.
Data Availability Statement
The original contributions presented in the study are included in the article/Supplementary Material, further inquiries can be directed to the corresponding author.
Author Contributions
PZ conceived the idea for the study. PZ, TW, SH, and HC obtained the genetic data. PZ and SH developed the study methods. PZ, TW, ZS, and HC performed the data analyses. PZ, ZS, JQ, and HC interpreted the results of the data analyses. PZ, JQ, and HC wrote the manuscript with suggestions from other authors. All authors contributed to the article and approved the submitted version.
Funding
The research of PZ was supported in part by the National Natural Science Foundation of China (82173630 and 81402765), the Youth Foundation of Humanity and Social Science funded by Ministry of Education of China (18YJC910002), the Natural Science Foundation of Jiangsu Province of China (BK20181472), the China Postdoctoral Science Foundation (2018M630607 and 2019T120465), the QingLan Research Project of Jiangsu Province for Outstanding Young Teachers, the Six-Talent Peaks Project in Jiangsu Province of China (WSN-087), the Training Project for Youth Teams of Science and Technology Innovation at Xuzhou Medical University (TD202008), and the Statistical Science Research Project from National Bureau of Statistics of China (2014LY112). The research of SH was supported in part by the Social Development Project of Xuzhou City (KC19017). The research of TW was supported in part by the Social Development Project of Xuzhou City (KC20062).
Conflict of Interest
The authors declare that the research was conducted in the absence of any commercial or financial relationships that could be construed as a potential conflict of interest.
Publisher’s Note
All claims expressed in this article are solely those of the authors and do not necessarily represent those of their affiliated organizations, or those of the publisher, the editors and the reviewers. Any product that may be evaluated in this article, or claim that may be made by its manufacturer, is not guaranteed or endorsed by the publisher.
Acknowledgments
We thank the complex traits genetic lab, ALS Variant Server, International FTD-Genomics consortium, International Parkinson’s Disease Genomics consortium, International Multiple Sclerosis Genetics consortium for making the Alzheimer’s disease, amyotrophic lateral sclerosis, frontotemporal dementia, Parkinson’s disease, and multiple sclerosis summary data publicly available and are grateful of all the investigators and participants contributed to this study. The human blood metabolites data is publicly available from Metabolomics GWAS Server at http://metabolomics.helmholtz-muenchen.de/gwas/. The data analyses in the present study were carried out with the high-performance computing cluster that was supported by the special central finance project of local universities for Xuzhou Medical University. We thank two reviewers for their constructive comments, which substantially improved our manuscript.
Supplementary Material
The Supplementary Material for this article can be found online at: https://www.frontiersin.org/articles/10.3389/fnins.2021.680104/full#supplementary-material
Abbreviations
NDD, neurodegenerative disease; GWAS, genome-wide association study; MR, Mendelian randomization; AD, Alzheimer’s Disease; ALS, amyotrophic lateral sclerosis; FTD, frontotemporal dementia; PD, Parkinson’s disease; MS, multiple sclerosis; MR-PRESSO, Mendelian Randomization Pleiotropy RESidual Sum and Outlier; LDSC, linkage disequilibrium score regression; LD, linkage disequilibrium; FDR, false discover rate; SNP, single nucleotide polymorphisms; PVE, phenotypic variance explained; IVW, inverse-variance-weighted.
References
Abramzon, Y., Fratta, P., Traynor, B., and Chia, R. (2020). The overlapping genetics of amyotrophic lateral sclerosis and frontotemporal dementia. Front. Neurosci. 14:42. doi: 10.3389/fnins.2020.00042
Ahmad, O. S., Morris, J. A., Mujammami, M., Forgetta, V., Leong, A., Li, R., et al. (2015). A Mendelian randomization study of the effect of type-2 diabetes on coronary heart disease. Nat. Commun. 6:7060. doi: 10.1038/ncomms8060
Al-Chalabi, A., and Hardiman, O. (2013). The epidemiology of ALS: a conspiracy of genes, environment and time. Nat. Rev. Neurol. 9, 617–628. doi: 10.1038/nrneurol.2013.203
Amor, S., Puentes, F., Baker, D., and Van Der Valk, P. (2010). Inflammation in neurodegenerative diseases. Immunology 129, 154–169. doi: 10.1111/j.1365-2567.2009.03225.x
Bakhta, K., Cecillon, E., Lacombe, E., Lamy, M., Leboucher, A., and Philippe, J. (2019). Alzheimer’s disease and neurodegenerative diseases in France. Lancet 394, 466–467. doi: 10.1016/s0140-6736(19)31633-2
Barnham, K. J., Masters, C. L., and Bush, A. I. (2004). Neurodegenerative diseases and oxidative stress. Nat. Rev. Drug Discov. 3, 205–214. doi: 10.1038/nrd1330
Benjamini, Y., and Hochberg, Y. (1995). Controlling the false discovery rate: a practical and powerful approach to multiple testing. J. R. Statist. Soc. B 57, 289–300. doi: 10.1111/j.2517-6161.1995.tb02031.x
Blennow, K., Hampel, H., Weiner, M., and Zetterberg, H. (2010). Cerebrospinal fluid and plasma biomarkers in Alzheimer disease. Nat. Rev. Neurol. 6, 131–144. doi: 10.1038/nrneurol.2010.4
Bowden, J., Smith, G. D., Haycock, P. C., and Burgess, S. (2016b). Consistent estimation in mendelian randomization with some invalid instruments using a weighted median estimator. Genet. Epidemiol. 40, 304–314. doi: 10.1002/gepi.21965
Bowden, J., Del Greco, M. F., Minelli, C., Smith, G. D., Sheehan, N. A., and Thompson, J. R. (2016a). Assessing the suitability of summary data for two-sample Mendelian randomization analyses using MR-Egger regression: the role of the I-2 statistic. Int. J. Epidemiol. 45, 1961–1974. doi: 10.1093/ije/dyw220
Bozzoni, V., Pansarasa, O., Diamanti, L., Nosari, G., Cereda, C., and Ceroni, M. (2016). Amyotrophic lateral sclerosis and environmental factors. Funct. Neurol. 31, 7–19. doi: 10.11138/fneur/2016.31.1.007
Broce, I., Karch, C. M., Wen, N., Fan, C. C., Wang, Y., Hong Tan, C., et al. (2018). Immune-related genetic enrichment in frontotemporal dementia: an analysis of genome-wide association studies. PLoS Med. 15:e1002487. doi: 10.1371/journal.pmed.1002487
Bulik-Sullivan, B., Finucane, H. K., Anttila, V., Gusev, A., Day, F. R., Loh, P.-R., et al. (2015). An atlas of genetic correlations across human diseases and traits. Nat. Genet. 47, 1236–1241. doi: 10.1038/ng.3406
Burgess, S., Butterworth, A., and Thompson, S. G. (2013). Mendelian randomization analysis with multiple genetic variants using summarized data. Genet. Epidemiol. 37, 658–665. doi: 10.1002/gepi.21758
Burgess, S., Dudbridge, F., and Thompson, S. G. (2015). Re: “Multivariable Mendelian randomization: the use of pleiotropic genetic variants to estimate causal effects”. Am. J. Epidemiol. 181, 290–291. doi: 10.1093/aje/kwv017
Burgess, S., Small, D. S., and Thompson, S. G. (2017). A review of instrumental variable estimators for Mendelian randomization. Stat. Methods Med. Res. 26, 2333–2355. doi: 10.1177/0962280215597579
Burgess, S., and Thompson, S. G. (2017). Interpreting findings from Mendelian randomization using the MR-Egger method. Eur. J. Epidemiol. 32, 377–389. doi: 10.1007/s10654-017-0255-x
Chatterjee, P., Cheong, Y.-J., Bhatnagar, A., Goozee, K., Wu, Y., McKay, M., et al. (2020). Plasma metabolites associated with biomarker evidence of neurodegeneration in cognitively normal older adults. J. Neurochem. 159, 389–402. doi: 10.1111/jnc.15128 n/a(n/a),
Chong, J., Soufan, O., Li, C., Caraus, I., Li, S., Bourque, G., et al. (2018). MetaboAnalyst 4.0: towards more transparent and integrative metabolomics analysis. Nucleic Acids Res. 46, W486–W494. doi: 10.1093/nar/gky310
Couratier, P., Corcia, P., Lautrette, G., Nicol, M., and Marin, B. (2017). ALS and frontotemporal dementia belong to a common disease spectrum. Rev. Neurol. 173, 273–279. doi: 10.1016/j.neurol.2017.04.001
Cragg, J. G., and Donald, S. G. (1993). Testing identifiability and specification in instrumental variable models. Econometric Theory 9, 222–240.
Cummings, J. L., Morstorf, T., and Zhong, K. (2014). Alzheimer’s disease drug-development pipeline: few candidates, frequent failures. Alzheimers Res. Ther. 6:37. doi: 10.1186/alzrt269
Davies, N. M., Holmes, M. V., and Davey Smith, G. (2018). Reading Mendelian randomisation studies: a guide, glossary, and checklist for clinicians. Br. Med. J. 362:k601. doi: 10.1136/bmj.k601
Doty, R. L. (2017). Olfactory dysfunction in neurodegenerative diseases: is there a common pathological substrate? Lancet Neurol. 16, 478–488. doi: 10.1016/s1474-4422(17)30123-0
Emerit, J., Edeas, M., and Bricaire, F. (2004). Neurodegenerative diseases and oxidative stress. Biomed. Pharmacother. 58, 39–46. doi: 10.1016/j.biopha.2003.11.004
Evans, D. M., and Davey Smith, G. (2015). Mendelian randomization: new applications in the coming age of hypothesis-free causality. Annu. Rev. Genomics Hum. Genet. 16, 327–350. doi: 10.1146/annurev-genom-090314-050016
Fernstrom, J. D., and Fernstrom, M. H. (2007). Tyrosine, phenylalanine, and catecholamine synthesis and function in the brain. J. Nutr. 137(6 Suppl. 1) 1539S–1547S. doi: 10.1093/jn/137.6.1539S discussion 1548S,
Ferrari, R., Hernandez, D. G., Nalls, M. A., Rohrer, J. D., Ramasamy, A., Kwok, J. B., et al. (2014). Frontotemporal dementia and its subtypes: a genome-wide association study. Lancet Neurol. 13, 686–699. doi: 10.1016/S1474-4422(14)70065-1
Ferrari, R., Wang, Y., Vandrovcova, J., Guelfi, S., Witeolar, A., Karch, C. M., et al. (2017). Genetic architecture of sporadic frontotemporal dementia and overlap with Alzheimer’s and Parkinson’s diseases. J. Neurol. Neurosurg. Psychiatry 88, 152–164. doi: 10.1136/jnnp-2016-314411
Frolkis, A., Knox, C., Lim, E., Jewison, T., Law, V., Hau, D. D., et al. (2010). SMPDB: the small molecule pathway database. Nucleic Acids Res. 38(Database issue) D480–D487. doi: 10.1093/nar/gkp1002
Giambartolomei, C., Vukcevic, D., Schadt, E. E., Franke, L., Hingorani, A. D., Wallace, C., et al. (2014). Bayesian test for colocalisation between pairs of genetic association studies using summary statistics. PLoS Genet. 10:e1004383. doi: 10.1371/journal.pgen.1004383
González-Domínguez, R., García-Barrera, T., and Gómez-Ariza, J. L. (2015). Metabolite profiling for the identification of altered metabolic pathways in Alzheimer’s disease. J. Pharm. Biomed. Anal. 107, 75–81. doi: 10.1016/j.jpba.2014.10.010
Greenland, S. (2000). An introduction to instrumental variables for epidemiologists. Int. J. Epidemiol. 29, 722–729. doi: 10.1093/ije/29.4.722
Hartwig, F. P., Davey Smith, G., and Bowden, J. (2017). Robust inference in summary data Mendelian randomization via the zero modal pleiotropy assumption. Int. J. Epidemiol. 46, 1985–1998. doi: 10.1093/ije/dyx102
Holmes, E., Loo, R. L., Stamler, J., Bictash, M., Yap, I. K., Chan, Q., et al. (2008). Human metabolic phenotype diversity and its association with diet and blood pressure. Nature 453, 396–400. doi: 10.1038/nature06882
International Multiple Sclerosis Genetics Consortium (2019). Multiple sclerosis genomic map implicates peripheral immune cells and microglia in susceptibility. Science 365:eaav7188. doi: 10.1126/science.aav7188
Jansen, I. E., Savage, J. E., Watanabe, K., Bryois, J., Williams, D. M., Steinberg, S., et al. (2019). Genome-wide meta-analysis identifies new loci and functional pathways influencing Alzheimer’s disease risk. Nat. Genet. 51, 404–413. doi: 10.1038/s41588-018-0311-9
Jiang, Y., Zhu, Z., Shi, J., An, Y., Zhang, K., Wang, Y., et al. (2019). Metabolomics in the development and progression of dementia: a systematic review. Front. Neurosci. 13:343. doi: 10.3389/fnins.2019.00343
Johnson, C. H., Ivanisevic, J., and Siuzdak, G. (2016). Metabolomics: beyond biomarkers and towards mechanisms. Nat. Rev. Mol. Cell Biol. 17, 451–459. doi: 10.1038/nrm.2016.25
Jové, M., Portero-Otín, M., Naudí, A., Ferrer, I., and Pamplona, R. (2014). Metabolomics of human brain aging and age-related neurodegenerative diseases. J. Neuropathol. Exp. Neurol. 73, 640–657. doi: 10.1097/nen.0000000000000091
Kanehisa, M., Goto, S., Sato, Y., Furumichi, M., and Tanabe, M. (2012). KEGG for integration and interpretation of large-scale molecular data sets. Nucleic Acids Res. 40(Database issue) D109–D114. doi: 10.1093/nar/gkr988
Karch, C. M., Wen, N., Fan, C. C., Yokoyama, J. S., Kouri, N., Ross, O. A., et al. (2018). Selective genetic overlap between amyotrophic lateral sclerosis and diseases of the frontotemporal dementia spectrum. JAMA Neurol. 75, 860–875. doi: 10.1001/jamaneurol.2018.0372
Kiddle, S. J., Sattlecker, M., Proitsi, P., Simmons, A., Westman, E., Bazenet, C., et al. (2014). Candidate blood proteome markers of Alzheimer’s disease onset and progression: a systematic review and replication study. J. Alzheimers Dis. 38, 515–531. doi: 10.3233/jad-130380
Kim, M., Snowden, S., Suvitaival, T., Ali, A., Merkler, D. J., Ahmad, T., et al. (2019). Primary fatty amides in plasma associated with brain amyloid burden, hippocampal volume, and memory in the European Medical Information Framework for Alzheimer’s Disease biomarker discovery cohort. Alzheimers Dement. 15, 817–827. doi: 10.1016/j.jalz.2019.03.004
Klineova, S., and Lublin, F. D. (2018). Clinical course of multiple sclerosis. Cold Spring Harb. Perspect. Med. 8:a028928. doi: 10.1101/cshperspect.a028928
Kori, M., Aydın, B., Unal, S., Arga, K. Y., and Kazan, D. (2016). Metabolic biomarkers and neurodegeneration: a pathway enrichment analysis of Alzheimer’s disease, Parkinson’s disease, and amyotrophic lateral sclerosis. OMICS 20, 645–661. doi: 10.1089/omi.2016.0106
Larsson, S. C., Burgess, S., and Michaëlsson, K. (2017). Association of genetic variants related to serum calcium levels with coronary artery disease and myocardial infarction. JAMA 318, 371–380. doi: 10.1001/jama.2017.8981
Leng, Y., Musiek, E. S., Hu, K., Cappuccio, F. P., and Yaffe, K. (2019). Association between circadian rhythms and neurodegenerative diseases. Lancet Neurol. 18, 307–318. doi: 10.1016/s1474-4422(18)30461-7
Lu, H., Le, W. D., Xie, Y.-Y., and Wang, X.-P. (2016). Current therapy of drugs in amyotrophic lateral sclerosis. Curr. Neuropharmacol. 14, 314–321. doi: 10.2174/1570159x14666160120152423
Mapstone, M., Cheema, A. K., Fiandaca, M. S., Zhong, X., Mhyre, T. R., MacArthur, L. H., et al. (2014). Plasma phospholipids identify antecedent memory impairment in older adults. Nat. Med. 20, 415–418. doi: 10.1038/nm.3466
Mendelsohn, A. R., and Larrick, J. W. (2013). Sleep facilitates clearance of metabolites from the brain: glymphatic function in aging and neurodegenerative diseases. Rejuvenation Res. 16, 518–523. doi: 10.1089/rej.2013.1530
Monaco, F., Fumero, S., Mondino, A., and Mutani, R. (1979). Plasma and cerebrospinal fluid tryptophan in multiple sclerosis and degenerative diseases. J. Neurol. Neurosurg. Psychiatry 42, 640–641. doi: 10.1136/jnnp.42.7.640
Mrochek, J. E., Katz, S., Christie, W. H., and Dinsmore, S. R. (1974). Acetaminophen metabolism in man, as determined by high-resolution liquid chromatography. Clin. Chem. 20, 1086–1096.
Nalls, M. A., Blauwendraat, C., Vallerga, C. L., Heilbron, K., Bandres-Ciga, S., Chang, D., et al. (2019). Identification of novel risk loci, causal insights, and heritable risk for Parkinson’s disease: a meta-analysis of genome-wide association studies. Lancet Neurol. 18, 1091–1102. doi: 10.1016/s1474-4422(19)30320-5
Nicolas, A., Kenna, K. P., Renton, A. E., Ticozzi, N., Faghri, F., Chia, R., et al. (2018). Genome-wide analyses identify KIF5A as a novel ALS gene. Neuron 97, 1268–1283.e6. doi: 10.1016/j.neuron.2018.02.027
Parnetti, L. (2011). Biochemical diagnosis of neurodegenerative diseases gets closer. Lancet Neurol. 10, 203–205. doi: 10.1016/s1474-4422(11)70019-9
Pasaniuc, B., and Price, A. L. (2016). Dissecting the genetics of complex traits using summary association statistics. Nat. Rev. Genet. 18, 117–127. doi: 10.1038/nrg.2016.142
Przedborski, S., Donaldson, D., Jakowec, M., Kish, S. J., Guttman, M., Rosoklija, G., et al. (1996). Brain superoxide dismutase, catalase, and glutathione peroxidase activities in amyotrophic lateral sclerosis. Ann. Neurol. 39, 158–165. doi: 10.1002/ana.410390204
Purcell, S., Neale, B., Todd-Brown, K., Thomas, L., Ferreira, M. A., Bender, D., et al. (2007). PLINK: a tool set for whole-genome association and population-based linkage analyses. Am. J. Hum. Genet. 81, 559–575. doi: 10.1086/519795
Rachakonda, V., Pan, T. H., and Le, W. D. (2004). Biomarkers of neurodegenerative disorders: how good are they? Cell Res. 14, 347–358.
Roth, G. A., Abate, D., Abate, K. H., Abay, S. M., Abbafati, C., Abbasi, N., et al. (2018). Global, regional, and national age-sex-specific mortality for 282 causes of death in 195 countries and territories, 1980–2017: a systematic analysis for the Global Burden of Disease Study 2017. Lancet 392, 1736–1788. doi: 10.1016/S0140-6736(18)32203-7
Saharan, S., and Mandal, P. K. (2014). The emerging role of glutathione in Alzheimer’s disease. J. Alzheimers Dis. 40, 519–529. doi: 10.3233/jad-132483
Sanna, S., van Zuydam, N. R., Mahajan, A., Kurilshikov, A., Vila, A. V., Vosa, U., et al. (2019). Causal relationships among the gut microbiome, short-chain fatty acids and metabolic diseases. Nat. Genet. 51, 600–605. doi: 10.1038/s41588-019-0350-x
Shang, L., Smith, J. A., and Zhou, X. (2020). Leveraging gene co-expression patterns to infer trait-relevant tissues in genome-wide association studies. PLoS Genet. 16:e1008734. doi: 10.1371/journal.pgen.1008734
Shim, H., Chasman, D. I., Smith, J. D., Mora, S., Ridker, P. M., Nickerson, D. A., et al. (2015). A multivariate genome-wide association analysis of 10 LDL subfractions, and their response to statin treatment, in 1868 Caucasians. PLoS One 10:e0120758. doi: 10.1371/journal.pone.0120758
Shin, S. Y., Fauman, E. B., Petersen, A. K., Krumsiek, J., Santos, R., Huang, J., et al. (2014). An atlas of genetic influences on human blood metabolites. Nat. Genet. 46, 543–550. doi: 10.1038/ng.2982
The 1000 Genomes Project Consortium (2015). A global reference for human genetic variation. Nature 526, 68–74. doi: 10.1038/nature15393
Thomas, D. C., and Conti, D. V. (2004). Commentary: the concept of ‘Mendelian randomization’. Int. J. Epidemiol. 33, 21–25. doi: 10.1093/ije/dyh048
Thompson, S. G., and Sharp, S. J. (1999). Explaining heterogeneity in meta-analysis: a comparison of methods. Stat. Med. 18, 2693–2708. doi: 10.1002/(sici)1097-0258(19991030)18:20<2693::aid-sim235<3.0.co;2-v
To, T., Zhu, J., Stieb, D., Gray, N., Fong, I., Pinault, L., et al. (2019). Early life exposure to air pollution and incidence of childhood asthma, allergic rhinitis and eczema. Eur. Respir. J. 55:1900913. doi: 10.1183/13993003.00913-2019
Tobin, M. D., Minelli, C., Burton, P. R., and Thompson, J. R. (2004). Commentary: development of Mendelian randomization: from hypothesis test to ‘Mendelian deconfounding’. Int. J. Epidemiol. 33, 26–29.
Trojanowski, J. (2000). Biochemical markers of neurodegenerative diseases: τ and synucleins. Arch. Neurol. 57, 1236–1236.
Verbanck, M., Chen, C. Y., Neale, B., and Do, R. (2018). Detection of widespread horizontal pleiotropy in causal relationships inferred from Mendelian randomization between complex traits and diseases. Nat. Genet. 50, 693–698. doi: 10.1038/s41588-018-0099-7
Wang, J., Wu, Z., Li, D., Li, N., Dindot, S. V., Satterfield, M. C., et al. (2012). Nutrition, epigenetics, and metabolic syndrome. Antioxid. Redox Signal. 17, 282–301. doi: 10.1089/ars.2011.4381
Wheatley, K., and Gray, R. (2004). Commentary: Mendelian randomization—an update on its use to evaluate allogeneic stem cell transplantation in leukaemia. Int. J. Epidemiol. 33, 15–17. doi: 10.1093/ije/dyg313
Wissmann, P., Geisler, S., Leblhuber, F., and Fuchs, D. (2013). Immune activation in patients with Alzheimer’s disease is associated with high serum phenylalanine concentrations. J. Neurol. Sci. 329, 29–33. doi: 10.1016/j.jns.2013.03.007
Wood, H. (2011). A hexanucleotide repeat expansion in C9ORF72 links amyotrophic lateral sclerosis and frontotemporal dementia. Nat. Rev. Neurol. 7:595. doi: 10.1038/nrneurol.2011.162
Yavorska, O. O., and Burgess, S. (2017). MendelianRandomization: an R package for performing Mendelian randomization analyses using summarized data. Int. J. Epidemiol. 46, 1734–1739. doi: 10.1093/ije/dyx034
Yu, X., Wang, T., Chen, Y., Shen, Z., Gao, Y., Xiao, L., et al. (2020a). Alcohol drinking and amyotrophic lateral sclerosis: an instrumental variable causal inference. Ann. Neurol. 88, 195–198. doi: 10.1002/ana.25721
Yu, X., Yuan, Z., Lu, H., Gao, Y., Chen, H., Shao, Z., et al. (2020b). Relationship between birth weight and chronic kidney disease: evidence from systematics review and two-sample Mendelian randomization analysis. Hum. Mol. Genet. 29, 2261–2274. doi: 10.1093/hmg/ddaa074
Zeng, P., Wang, T., Zheng, J., and Zhou, X. (2019). Causal association of type 2 diabetes with amyotrophic lateral sclerosis: new evidence from Mendelian randomization using GWAS summary statistics. BMC Med. 17:225. doi: 10.1186/s12916-019-1448-9
Zeng, P., and Zhou, X. (2019b). Causal effects of blood lipids on amyotrophic lateral sclerosis: a Mendelian randomization study. Hum. Mol. Genet. 28, 688–697. doi: 10.1093/hmg/ddy384
Zeng, P., and Zhou, X. (2019a). Causal association between birth weight and adult diseases: evidence from a Mendelian randomization analysis. Front. Genet. 10:618. doi: 10.3389/fgene.2019.00618
Keywords: metabolites, neurodegenerative diseases, Mendelian randomization, metabolic pathway, amyotrophic lateral sclerosis, frontotemporal dementia, causal association
Citation: Chen H, Qiao J, Wang T, Shao Z, Huang S and Zeng P (2021) Assessing Causal Relationship Between Human Blood Metabolites and Five Neurodegenerative Diseases With GWAS Summary Statistics. Front. Neurosci. 15:680104. doi: 10.3389/fnins.2021.680104
Received: 13 March 2021; Accepted: 22 November 2021;
Published: 09 December 2021.
Edited by:
Henrik Zetterberg, University of Gothenburg, SwedenReviewed by:
Junling Wang, Central South University, ChinaDavid Otaegui, Biodonostia Health Research Institute (IIS Biodonostia), Spain
Copyright © 2021 Chen, Qiao, Wang, Shao, Huang and Zeng. This is an open-access article distributed under the terms of the Creative Commons Attribution License (CC BY). The use, distribution or reproduction in other forums is permitted, provided the original author(s) and the copyright owner(s) are credited and that the original publication in this journal is cited, in accordance with accepted academic practice. No use, distribution or reproduction is permitted which does not comply with these terms.
*Correspondence: Ping Zeng, zpstat@xzhmu.edu.cn
†These authors share first authorship