- 1Department of Psychiatry and Biobehavioral Sciences, University of California, Los Angeles, Los Angeles, CA, United States
- 2Brain Research Institute, David Geffen School of Medicine, University of California, Los Angeles, Los Angeles, CA, United States
- 3Department of Molecular and Medical Pharmacology, University of California, Los Angeles, Los Angeles, CA, United States
Decision-making strategies shift during normal aging and can profoundly affect wellbeing. Although overweighing losses compared to gains, termed “loss aversion,” plays an important role in choice selection, the age trajectory of this effect and how it may be influenced by associated changes in brain structure remain unclear. We therefore investigated the relationship between age and loss aversion, and tested for its mediation by cortical thinning in brain regions that are susceptible to age-related declines and are implicated in loss aversion — the insular, orbitofrontal, and anterior and posterior cingulate cortices. Healthy participants (n = 106, 17–54 years) performed the Loss Aversion Task. A subgroup (n = 78) provided structural magnetic resonance imaging scans. Loss aversion followed a curvilinear trajectory, declining in young adulthood and increasing in middle-age, and thinning of the posterior cingulate cortex mediated this trajectory. The findings suggest that beyond a threshold in middle adulthood, atrophy of the posterior cingulate cortex influences loss aversion.
Introduction
The proportion of the global population that is 65 years or older is increasing faster than those of other age groups; it is estimated that by 2050, one in four people in North America and Europe, and one in six people worldwide, will be over 65 (United Nations, 2019). As older adults face a myriad of choices that involve uncertainty and loss across multiple domains, changes in decision-making can substantially impact their quality of life (Samanez-Larkin, 2013; MacLeod et al., 2017). Accordingly, the impact of aging on decision-making is of substantial interest (Löckenhoff, 2018; Lighthall, 2020). Findings have been mixed, showing worsening in some respects, particularly in more deliberative domains, such as applying decision rules (Brown and Ridderinkhof, 2009). Yet, older adults can show more optimal decision-making than their younger counterparts, especially for choices that rely on life experience and acquired knowledge (Li et al., 2013).
Many everyday decisions present a potential for loss, which increases in salience with age (Ebner et al., 2006; Depping and Freund, 2011; Mata and Hertwig, 2011; Löckenhoff, 2018). When making a choice that balances the chance of gain against the risk of loss, people of all ages tend to be risk averse and to accept a gamble only if the magnitude of the win vastly outweighs that of the loss. This phenomenon has been explained by loss aversion, which reflects the overweighing of losses compared to equivalent gains (Kahneman and Tversky, 1979; Tversky and Kahneman, 1992). Despite reports of greater loss aversion in adults over compared to under 40 (Arora and Kumari, 2015; Kurnianingsih et al., 2015; O’Brien and Hess, 2020), other studies find no differences (Li et al., 2013; Rutledge et al., 2016; Pachur et al., 2017; Seaman et al., 2018). This discrepancy could be due to nonlinear effects of age on loss aversion, the exclusion of middle-aged participants in comparisons of older and younger groups (Li et al., 2013), or different methods of measuring loss aversion (Rutledge et al., 2016; Seaman et al., 2018).
Although aversions to risk and loss are presumably evolutionarily adaptive mechanisms (Robson, 1996; Chen et al., 2005; Zhang et al., 2014; Hintze et al., 2015), extreme sensitivity to potential loss can impair decision-making during laboratory tasks (Benjamin and Robbins, 2007; Cassotti et al., 2014) and real-world choices (Mishina et al., 2010; Herweg and Mierendorff, 2013; Schleich et al., 2019), and by people with psychiatric pathologies, such as affective disorders (Stamatis et al., 2020; Xu et al., 2020). Notably, a curvilinear relationship exists between age and both real-world financial choices (Agarwal et al., 2009) and laboratory risky decision-making (Read and Read, 2004; Tymula et al., 2013; Di Rosa et al., 2017), with better performance by middle-aged adults than their younger or older counterparts.
The goal of this study was to determine whether loss aversion followed a curvilinear relationship with age, and whether such a relationship is mediated by thickness of the insula, ventromedial prefrontal/orbitofrontal cortex (OFC), and/or anterior and posterior cingulate cortices, all of which are particularly vulnerable to age-related atrophy and are implicated in loss aversion (Tom et al., 2007; Canessa et al., 2013; Markett et al., 2016). Because risky decision-making (Tymula et al., 2013; Di Rosa et al., 2017) and associated cognitive functions (Verhaeghen and Salthouse, 1997; Brockmole and Logie, 2013; Hartshorne and Germine, 2015) follow curvilinear trajectories with age, we hypothesized that age and loss aversion would be related by a quadratic function, and that cortical thickness would influence this relationship. Considering reports that the cortical regions selected for study exhibit linear age-related thinning (Tamnes et al., 2009; Lemaitre et al., 2012; Storsve et al., 2014), we hypothesized that cortical thickness would influence loss aversion after a threshold of atrophy had been reached. Loss aversion was measured using the Loss Aversion Task, and structural MRI was performed on participants from young adulthood through middle age (17 to 54 years).
Materials and Methods
Participants
Data presented here are from healthy, right-handed volunteers between the ages of 17 and 54 who participated in studies that were approved by the University of California, Los Angeles Institutional Review Board. 130 participants (40 women) completed the Loss Aversion Task and 24 were excluded during analysis of the behavioral data (see procedures for exclusion under Loss Aversion Task below), leaving 106 for final analysis. MRI and behavioral data from these participants, other than performance on the Loss Aversion Task, have been published in other reports (Dean et al., 2011, 2015, 2018, 2020; Ghahremani et al., 2011, 2012; Morales et al., 2012, 2015a, 2015b; Payer et al., 2012; Zorick et al., 2012; Kohno et al., 2014; Ballard et al., 2015a, b; Jones et al., 2016; Okita et al., 2016a,b,c, 2018; Moeller et al., 2018; London et al., 2020). Recruitment utilized online and print advertisements. After initial screening, participants received detailed information about each study and gave written informed consent before screening for eligibility by physical examination, medical history, and psychiatric evaluation. Drug use history and demographic information were collected using questionnaires. Participants were excluded for medical or neurological disorders or any current Axis I psychiatric disorder except Nicotine Dependence, determined by the Structured Clinical Interview for DSM-IV (First et al., 1998). After intake, participants returned on a different day to perform the Loss Aversion Task, which was administered using identical procedures for all studies. A subset of participants (n = 83) also completed structural magnetic resonance imaging (sMRI) on a different day. Data from 5 of those participants were excluded during preprocessing, leaving 78 for analysis. The average time between behavioral testing and the sMRI scan was 7 days. At intake and on each test day, participants were required to provide a urine sample that was negative for amphetamine, cocaine, methamphetamine, benzodiazepines, opioids, and cannabis. They were compensated in the form of cash, gift cards, or vouchers.
Loss Aversion Task
The task consisted of 128 sequential monetary choices to accept or reject a mixed gamble offering a 50/50 chance of winning a certain amount of money and losing a different amount of money (e.g., gaining $30 or losing $7) (Tom et al., 2007). On each trial, an image representing a 50/50 choice was presented on the screen, and the participants indicated whether they strongly accept, weakly accept, weakly reject, or strongly reject the choice (Figure 1A). Four options were provided instead of two (i.e., accept or reject) to discourage reliance on rule-based choice (e.g., always accepting when the loss exceeded $5). The probability of winning or losing was kept constant at 50%, and the alternative to accepting the gamble was always to remain at the status quo (i.e., win and lose nothing). The gains ranged from $10--40 in increments of $2, and the losses ranged from $5--20 in increments of $1. Once the participant decided, the next choice was presented without showing the outcome of the previous choice; if no selection was made within 3 s, the next gamble appeared on the screen. The task was presented using MATLAB (Mathworks, Natick, MA, United States) and the Psychtoolbox1 on an Apple PowerMac laptop computer running Mac OSX (Apple Computers, Cupertino, CA, United States), with most of the code being the same as used previously (Tom et al., 2007). Participants responded using the 1, 2, 3, and 4 keys on the keyboard.
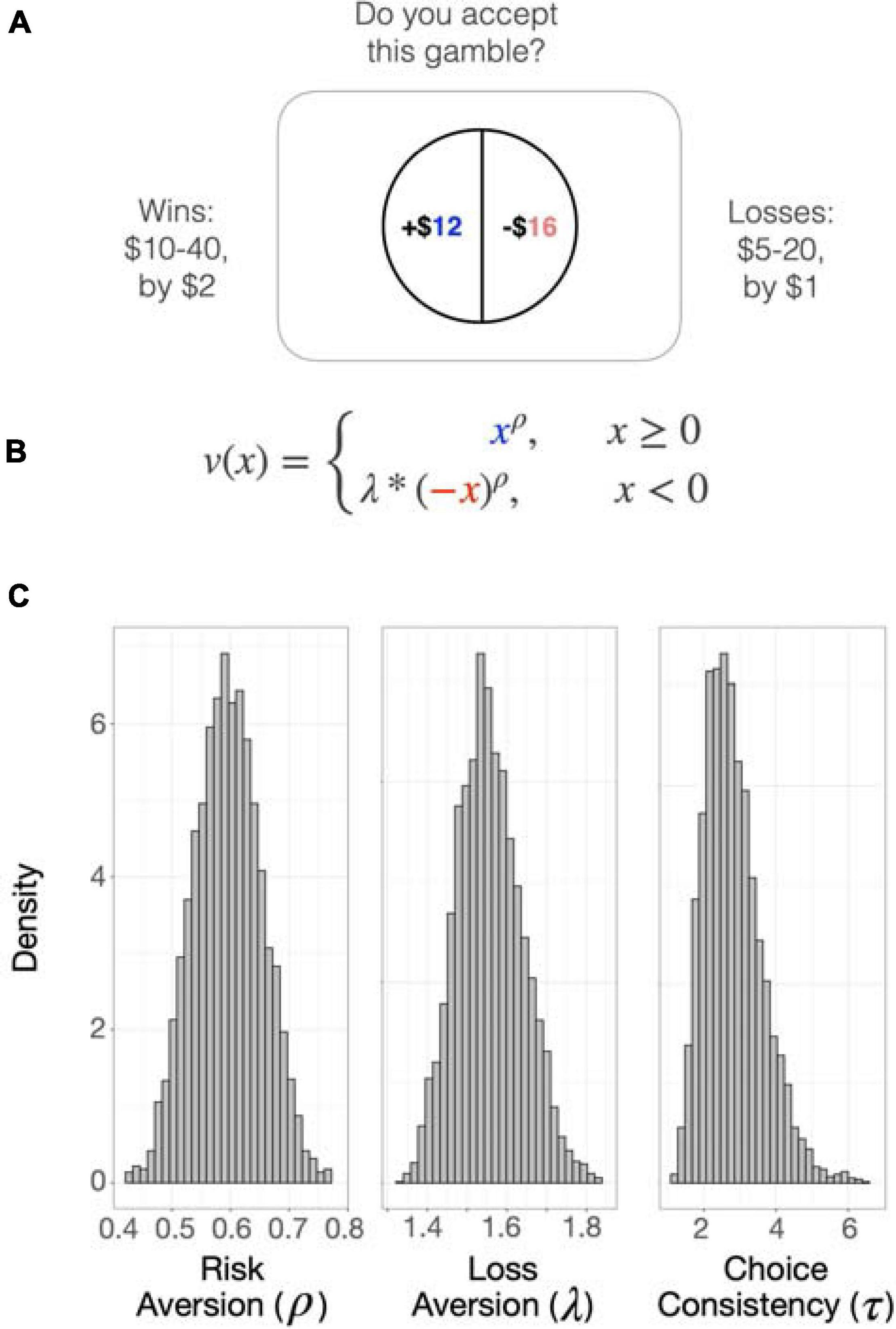
Figure 1. The Loss Aversion Task. (A) The task consisted of 128 sequential monetary choices to accept or reject a mixed gamble offering a 50/50 chance of winning a certain amount of money (blue) and losing a different amount of money (red). On each trial, an image representing a 50/50 choice was presented on the screen, and the participants indicated whether they strongly accept, weakly accept, weakly reject, or strongly reject the choice. Before testing, participants received thorough instruction and practice on how to perform the task. One choice was randomly selected to be paid out at the end of the task. (B). Gain (x) and loss (–x) magnitudes of each choice were inserted into the subjective value equation v(x). The loss aversion parameter (λ) represents the sensitivity to potential loss relative to potential gain. Rho (ρ) describes the curvature of the utility function and represents attitude toward risk. (C) Posterior distributions of parameters estimated using hierarchical Bayesian analysis, which enables the joint estimation of individual and group parameters. The distribution densities of each parameter are plotted. Higher values of λ indicate higher loss aversion and that the participant assigns more weight to losses than to gains of equal magnitude. When ρ < 1, the participant is risk-seeking for losses (more likely to take a gamble over a sure loss) and risk-averse for gains (more likely to choose a sure gain over a riskier prospect). The opposite is true when ρ > 1. Tau (τ) is the logit sensitivity and represents choice consistency, or the sensitivity of the participant to the difference between the certain amount and the gamble.
Before testing, participants received thorough instruction on how to perform the task. Instructions were read aloud, and the participant was encouraged to ask questions while viewing training slides and performing 5–10 practice trials. To ensure that participants were motivated on the task, they were told that one of their choices would be randomly selected to be paid out at the end of testing. They also were told that losses would be deducted from their earnings from participation in the study, but losses were not actually deducted.
The data were assessed for quality and cleaned in two ways: (1) trials with implausible reaction times (i.e., <200 ms) were excluded (0.0048% of trials); (2) data were excluded for any participant whose preferences were random, erratic, or inconsistent with trends predicted by our structural model (i.e., they were not more likely to accept the gamble for increasing magnitude of gain, decreasing magnitude of loss, or increasing expected value). Data from 24 participants were excluded.
Behavioral Choice Modeling
Choice parameters were estimated using a multi-parameter utility function (Sokol-Hessner et al., 2009) that represents subjective value (SV) (Eq. 1) based on original prospect theory (Kahneman and Tversky, 1979; Tversky and Kahneman, 1992):
The SV of the gamble is estimated using the objective magnitudes of gain (x) and loss (−x)given in each choice and the parameters of loss aversion (lambda; λ) and risk attitude (rho; ρ) (Figure 1B). The sensitivity to potential loss relative to potential gain is represented by λ. If λ = 1, the participant values gains and losses equally. When λ > 1, the participant is considered loss averse and assigns more weight to losses than to gains of equal magnitude. When λ < 1, the participant is considered gain-seeking, and overvalues gains compared to losses. Rho (ρ) describes the curvature of the utility function and represents attitude toward risk. If ρ = 1, the participant’s preferences can be modeled by a linear utility function, which signifies that each incremental increase in reward has equal utility. Values for ρ other than one indicate that the preferences of the participant can be described by a utility function that shows diminishing marginal utility. When ρ < 1, the participant is risk-seeking for losses (more likely to take a gamble over a sure loss) and risk-averse for gains (more likely to choose a sure gain over a riskier prospect). The opposite is true when ρ > 1. We did not explicitly measure risk attitudes in either the loss or gain domains.
The subjective values were then inserted into a logit (softmax) function (Eq. 2) that estimates the probability of accepting the gamble based on the difference in SVs between the lottery (50/50 choice or SVgamble) and the fixed amount ($0 or SVcertain). The responses “strongly accept” and “weakly accept” were both treated as accepting the gamble, and both “strongly reject” and “weakly reject” were treated as rejecting the gamble. Tau (τ) is the logit sensitivity and represents choice consistency, or the sensitivity of the participant to the difference between the certain amount and the gamble.
Parameter values were estimated using hierarchical Bayesian analysis with the “hBayesDM” package in R (Ahn et al., 2017), which enables the joint estimation of individual and group parameters and robustly identifies individual differences in decision-making (Ahn et al., 2011). Posterior inference was performed with Markov Chain Monte Carlo (MCMC) sampling using Stan (Carpenter et al., 2017) and RStan2. Models were validated by using the posterior distribution to generate data and visually inspecting whether the generated data corresponded to the underlying distribution.
Structural MRI
Structural T1-weighted magnetic resonance images of the brain were acquired from 83 participants using a Magnetization Prepared Rapid Gradient Echo (MPRAGE) sequence (see Table 1). Images were collected from 31 participants on Scanner 1: a 1.5-Tesla Siemens Sonata MRI scanner (Erlangen, Germany) with a standard quadrature head coil (TR = 1900 ms, TE = 4.38 ms, flip angle = 15°, FOV = 160 mm × 256 mm × 256 mm, 176 slices, resolution: 1 mm × 1 mm × 1 mm). Images from 33 participants were collected on Scanner 2: a 3-Tesla Trio TIM Siemens MRI scanner (Erlangen, Germany) using parameters of TR = 2530 ms, TE = 3.31 ms, flip angle = 7°, FOV = 176 mm × 256 mm × 256 mm, 176 slices, resolution: 1 mm × 1 mm × 1 mm. Data from the remaining 14 participants were acquired on Scanner 3: a different 3-Tesla Trio TIM Siemens scanner using the same parameters.
MRI Processing
Anatomical MRI images were processed using FreeSurfer 6.0.03, which generates a three-dimensional model of the cortical surface and provides measurements of local cortical thickness (Dale et al., 1999). Mean thickness within 72 automatically defined cortical parcels for each hemisphere were extracted from this model (Fischl et al., 2004; Desikan et al., 2006). Data quality was evaluated using the Qoala-T supervised learning quality control tool (Klapwijk et al., 2019), which identified data from 5 participants for exclusion, leaving data from the remaining 78 for the final analyses. As scans were acquired on different scanners, the ComBat procedure was used to harmonize the data and remove variability due to scanner type. ComBat has been validated on cortical thickness data and has been shown to robustly correct for scanner differences (Fortin et al., 2018). To preserve the variability due to age, we specified age as a biological variable for the ComBat model.
Statistical Analysis
Statistical analyses were performed using RStudio version 1.1.456. Analysis of variance (ANOVA) or correlation, as appropriate, was used to determine whether λ was significantly associated with biological sex, race/ethnicity, estimated IQ [using the Wechsler Test of Adult Reading (WTAR) (Wechsler, 2001)], years of education of the participant’s mother (as a proxy for socioeconomic status), or cigarette smoking status. As shown below, only race/ethnicity was associated with λ and was therefore included as a covariate in subsequent analyses.
A generalized linear model (GLM) was used to assess the effect of age on loss aversion. The parameter estimate (λ) from the behavioral choice model was used as the dependent variable in a GLM with the independent variable of age. Based on previous research demonstrating a curvilinear relationship between age and economic decision-making under risk (Tymula et al., 2013), a hierarchical regression analysis was used to test for a quadratic relationship between λ and age, with age2 added as an independent variable for the second step of the model. On an exploratory basis, the same associations were tested with the risk attitude parameter, ρ.
The average of the mean cortical thickness of both hemispheres, weighted by cortical volume, was calculated to determine whether λ was related to whole-brain cortical thickness. Based on prior research indicating brain regions important for loss aversion (Tom et al., 2007; Canessa et al., 2013; Markett et al., 2016) and cortical thinning of the cortex with age (Tamnes et al., 2009; Lemaitre et al., 2012; Storsve et al., 2014), a region of interest (ROI) analysis was performed, including the insula, OFC, anterior cingulate cortex (ACC), and posterior cingulate cortex (PCC). ROIs were created by calculating a weighted average of both hemispheres for each region. A weighted average was also used to combine the rostral and caudal ACC to create one ACC ROI, and the medial and lateral OFC to create one OFC ROI.
To assess the main effect of cortical thickness on λ, a GLM was used for each region with λ as the dependent variable and the linear and quadratic components of cortical thickness (cortical thickness and the square of cortical thickness) as independent variables. Estimated intracranial volume was included as a covariate. Results were corrected for multiple comparisons using the Holm-Bonferroni method.
For brain regions showing significant relationships of structure with λ, a mediation analysis was performed to test whether cortical thickness mediated the relationship between age and λ. Age-related cortical thinning was confirmed using a GLM with cortical thickness as the dependent variable, age as the independent variable, and biological sex, race/ethnicity, and estimated intracranial volume tested as covariates. Age2 was then added as an independent variable for the second step of the model to check for any nonlinear effects of age.
The mediation model tested whether cortical thickness mediated the effect of age on λ. Because of the quadratic relationship between age and λ, age2 was specified as the independent variable, with age and estimated total intracranial volume as covariates. To account for any nonlinearities, the square of cortical thickness was also included as a covariate. The mediation analysis used the “mediations” specification of the “mediation” package in R, which enables nonparametric causal mediation analysis (Imai et al., 2010, 2013). Indirect effects, given by the Average Causal Mediation Effects (ACME), were computed using Monte Carlo simulations, and the 95% confidence intervals were computed by determining the effects at the 2.5 and 97.5th percentiles.
Data Availability
All loss aversion task and cortical thickness data discussed in this manuscript, as well as the code used for statistical analyses, are publicly available at Open Science Framework under project title ‘‘Age Influences Loss Aversion Through Effects on Posterior Cingulate Cortical Thickness’’4.
Results
Relationship Between Loss Aversion and Demographic Variables
Biological sex, estimated IQ, cigarette smoking status, and years of mother’s education had no significant effects on λ (ps > 0.05), and, therefore, were not included in subsequent analyses (results were consistent when measures of socioeconomic status, such as father’s education, were used instead of mother’s education). An ANOVA revealed differences in λ based on race/ethnicity [F(4,101) = 5.78, p < 0.01], with post-hoc t-tests illustrating that Caucasians had higher λ than all other groups (ps < 0.05), and Hispanic/Latinx had higher λ than African Americans (p < 0.05); all other pairwise comparisons were nonsignificant (ps > 0.05). Based on these findings, subsequent analyses used race/ethnicity as a covariate which was coded as 1 = Caucasian, 2 = Hispanic/Latinx, 3 = African American, and 4 = Other.
Quadratic Relationship Between Loss Aversion and Age
In data from the full sample, parameter estimates of the behavioral choice model, estimated using hierarchical Bayesian analysis, were consistent with published values (Tom et al., 2007; Sokol-Hessner et al., 2009, 2012). Posterior distributions of the parameters are shown in Figure 1C. Means with standard errors and ranges were: λ = 1.58 (0.04; 0.76 – 2.61; loss aversion), ρ = 0.60 (0.0036; 0.44 – 0.70; risk attitudes), τ = 3.07 (0.09; 0.96 – 6.74; choice consistency) and reaction time = 1.45 (0.0059; 0.206 – 4.49). When the quadratic variable of age was added to the model, both age [β = −0.067, t(97) = −2.24, p = 0.028] and age2 [β = 0.0010, t(97) = 2.309, p = 0.023] had significant effects, and the model fit the data better than the linear model [ANOVA; F(97,98) = 5.33, p = 0.02, change in R2 = 0.0433; Figure 2A].
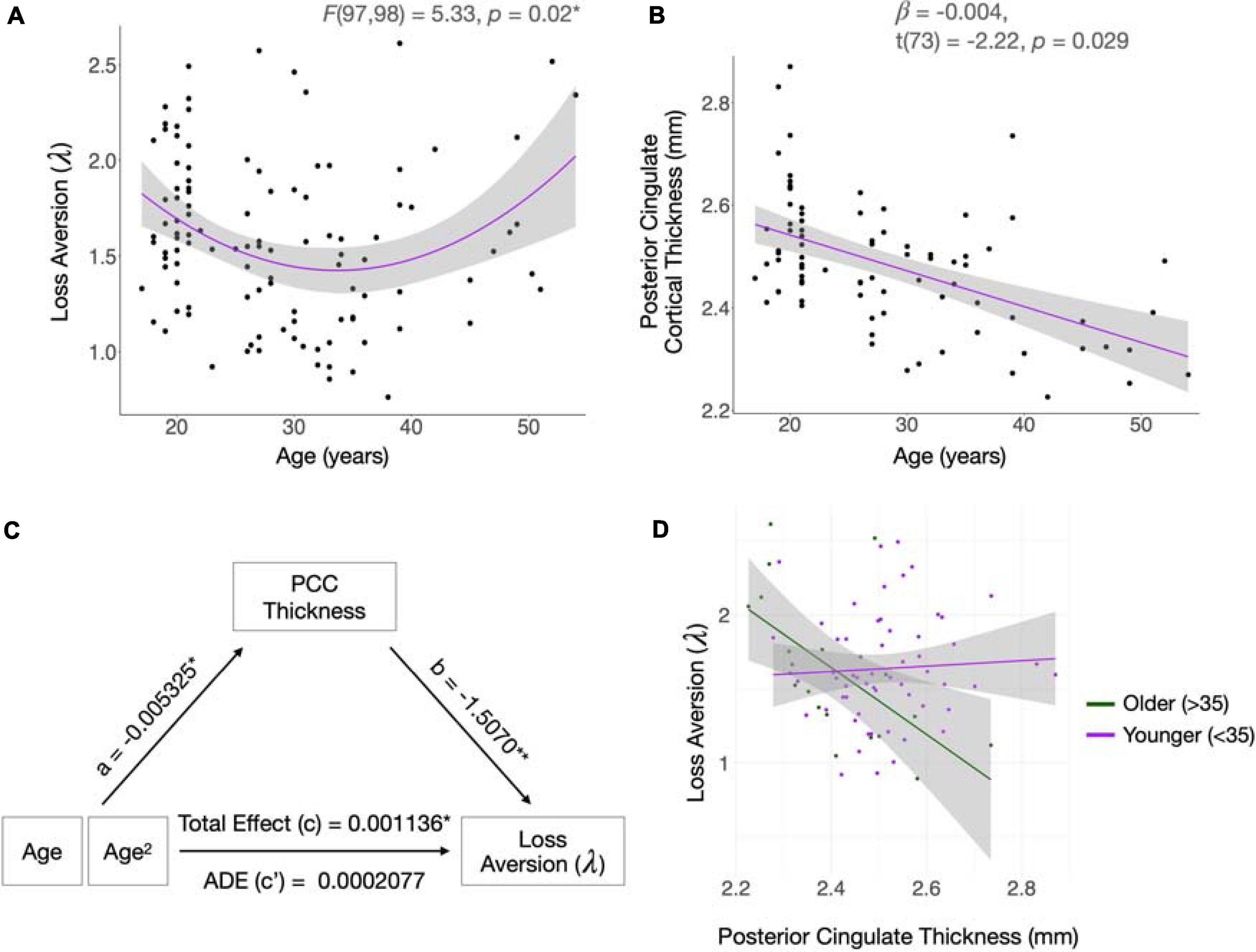
Figure 2. Relationships between age, loss aversion, and cortical thickness. (A,B) Loss aversion (λ) follows a quadratic trajectory with age, whereas cortical thickness of the posterior cingulate cortex (PCC) declines linearly with time. Shading indicates standard error confidence intervals. (C) Cortical thickness of the PCC mediates age-related changes in λ. The effect of age on PCC thickness is given by “a.” The effect of PCC thickness on λ is given by “b.” The Average Direct Effect (ADE; “c”) is the effect of age on λ when controlling for the mediator of PCC thickness. To calculate the Total Effect (c) of age on λ, without accounting for the mediator, both age and age2 were included in the model and the regression coefficient for age2 was taken as the strength of the effect. The causal mediation analysis was performed using nonparametric bootstrap confidence intervals and Monte Carlo simulations. The model included age, age2, race/ethnicity, scanner, and estimated intracranial volume, as well as PCC thickness as the mediator. Age2 was specified as the variable of interest. The measure of significance was given by the Average Causal Mediation Effect (ACME; p = 0.018*). Asterisks denote statistically significant results. *p < 0.05, **p < 0.01. (D) A negative relationship between PCC thickness and λ exists in older participants, but no relationship is present in participants under 35 years. The age of 35 was used to split the data into younger and older groups as it approximates the inflection point of the age-λ quadratic.
The curvilinear association between λ and age persisted in the subsample from which sMRI data were acquired (n = 78); when the quadratic variable of age was added to the model, both age [β = −0.0722, t(75) = −2.36, p = 0.021] and age2 [β = 0.0011, t(75) = 2.46, p = 0.016] were significantly related to λ. The quadratic model provided a significantly better fit for the data than the linear model [ANOVA; F(75,76) = 6.074, p = 0.016; change in R2 = 0.070].
Mediation by Posterior Cingulate Cortical Thickness of the Age Effect on Loss Aversion
Main Effects
Mean overall cortical thickness was not significantly related to loss aversion (β = 0.072, t(77) = 0.152, p = 0.88) and was therefore excluded from subsequent analyses. There were neither linear nor quadratic main effects of cortical thickness on λ in the insula [linear: β = −2.529, t(73) = −0.248, p = 0.805; quadratic: β = −0.467, t(73) = −0.278, p = 0.782], OFC [linear: β = 6.77, t(73) = 0.498, p = 0.620; quadratic: β = −1.32, t(73) = -0.515, p = 0.608], or ACC [linear: β = −1.209, t(73) = −1.071, p = 0.288; quadratic: β = 2.097, t(73) = 1.030, p = 0.306]. Although there were effects of both the linear and quadratic components of PCC thickness on λ [linear: β = −1.672, t(73) = −2.148, p = 0.035; quadratic: β = 3.30, t(73) = 2.13, p = 0.037], neither survived Holm-Bonferroni correction for multiple comparisons.
Mediation Analysis
Age-related cortical thinning of the PCC followed a linear course [β = −0.00728, t(73) = −5.19, p = 0.00000182; Figure 2B], with a small quadratic component [β = −0.000243, t(72) = 1.712, p = 0.091]. PCC thickness significantly mediated the age-loss aversion relationship, as quantified by the ACME (p = 0.028; Figure 2C). Since linear age-related change in the PCC was confirmed, but age and λ were quadratically related, we examined which component of the λ-age relationship was mediated by PCC thickness. To visualize the relationship between λ and PCC cortical thickness for different ages, we plotted the relationship between PCC thickness and λ by age for younger (<35) and older (>35) participants (Figure 2D). We split the data at the age of 35 as this was the inflection point of the age-loss aversion quadratic. The plot suggests that the mediation analysis captures an effect of PCC thickness on loss aversion that shifts throughout the lifespan, potentially mediating the increase in loss aversion in later life as opposed to the decrease in young adulthood.
Exploratory Analyses: Risk Attitudes (ρ) and Brain Structure
The risk attitude parameter (ρ) was not significantly correlated with age [β = −0.000520, t(98) = 0.83, p = 0.408] or the quadratic variable of age [β = 0.0000266, t(97) = 0.494, p = 0.622]. There were no main effects for cortical thickness or the cortical thickness2 on risk attitudes in any of the four ROIs: insula [linear: β = 0.499, t(73) = 0.489, p = 0.626; quadratic: β = −0.0764, t(73) = −0.454, p = 0.651]; OFC [linear: β = −0.083, t(73) = −0.061, p = 0.951; quadratic: β = 0.0151, t(73) = 0.058, p = 0.954]; ACC [linear: β = −0.724, t(73) = −0.633, p = 0.529; quadratic: β = 0.130, t(73) = 0.631, p = 0.530]; PCC [linear: β = 1.144, t(73) = 1.439, p = 0.154; quadratic: β = −0.222, t(73) = −1.401, p = 0.165].
Discussion
With the global population of those 65 years and older growing faster than all other age groups (United Nations, 2019), an understanding of the trajectory of decision-making over the lifespan may help people make better choices as they age (Agarwal et al., 2009; MacLeod et al., 2017). Providing unique insight into the relationship between aging and decision-making, this study found an association between age and loss aversion that followed a quadratic function, declining across young adulthood and reaching a minimum around age 35 before increasing in middle-age. We also showed that PCC thickness mediates the relationship between age and loss aversion, suggesting that cortical thinning of the PCC is likely one of several factors that contribute to changes in decision-making throughout the lifespan. Because we also confirmed that PCC thickness declines linearly with age (Tamnes et al., 2009; Lemaitre et al., 2012; Storsve et al., 2014), PCC thinning may emerge as an important factor in loss aversion when a certain threshold of atrophy begins in middle age.
A nonlinear relationship between age and loss aversion could unify seemingly conflicting results in the literature. Previous studies may have captured components of the quadratic relationship: participants aged 25–40 were less loss averse than those aged 41–55 (Arora and Kumari, 2015), and participants ∼18–28 were less loss averse than those aged ∼60–86 years (Kurnianingsih et al., 2015; O’Brien and Hess, 2020). Others may have missed differences due to the nonlinearities observed here (Li et al., 2013; Pachur et al., 2017). Our findings conflict with certain studies that did not find a quadratic relationship between age and loss aversion (Gächter et al., 2010; Rutledge et al., 2016; Seaman et al., 2018), which may be accounted for by the use of different tasks and methods to measure loss aversion (Gächter et al., 2010; Rutledge et al., 2016; Seaman et al., 2018). Nevertheless, the loss aversion and risk preference parameters were very similar to those recently reported in a study that fit a prospect theory utility function to choice data from 146 participants (Ackert et al., 2020).
The quadratic relationship between loss aversion and age mirrors the developmental trajectory of the cortex, during which the neurobiological mechanisms of cortical thinning differ in development and aging (Vidal-Pineiro et al., 2020). Cortical maturation includes thinning in sensory and eventually fronto-cortical areas, and may extend beyond the mid-twenties (Tamnes et al., 2009), whereas cortical thinning approaching middle-age could be considered the onset of senescence (Salat et al., 2004). Thus, PCC thickness may be unrelated to loss aversion during cortical maturation, but may arise as a contributing factor once cortical thinning is underway.
With normal aging, functional changes include the reduction of the integration of coordinated activity between brain regions and increases in the localization of function within regions (Bishop et al., 2010). Such reorganization can contribute to shifts in the mechanisms underlying decision-making, perhaps increasing reliance on certain regions and not others. The PCC has been linked to the representation of subjective value during probabilistic choice tasks (Kable and Glimcher, 2007; Levy et al., 2010), reward signaling (McCoy et al., 2003), attentional focus (Leech and Sharp, 2013), and the dynamic adaptation of behavior (Pearson et al., 2011). Beyond a threshold of cortical thinning of the PCC, such functions may be impeded, rendering the most adaptive strategy that which is the least cognitively demanding (Mata et al., 2007). Such adaptations could manifest in the use of an automatic or default heuristic, such as loss aversion, as shown by older adults using less cognitively taxing strategies in paradigms that involve risk (Weller et al., 2011). The plasticity of the brain coupled with an adaptive response to shifting cognitive resources (Gutchess, 2014) may result in older adults opting for choices that are “good enough” instead of searching to maximize outcomes [i.e., using “satisficing” instead of maximizing strategies (Kurnianingsih et al., 2015)]. During probabilistic choices involving loss, older adults are more likely to use such strategies when making decisions related to finances (Chen and Sun, 2003) and health (Besedeš et al., 2012). Satisficing strategies are related selectively to loss aversion and not to risk preferences; those who have greater loss aversion tend to stop searching for an optimal solution sooner (Schunk and Winter, 2009).
Notably, the Loss Aversion Task does not measure adaptive decision-making, and a loss-aversion strategy is not necessarily disadvantageous. Older individuals do not indiscriminately make worse decisions (Wood et al., 2005; Li et al., 2013, 2015; Bruine de Bruin et al., 2014), and heightened loss aversion may reflect naturally occurring shifts in values and motivations (Depping and Freund, 2011; Hess, 2014). Changes in cognitive faculties with age are not linear across time nor uniform across domains; the age-related decline of certain cognitive faculties, such as processing speed, episodic memory, and executive functions (Baltes and Lindenberger, 1997; Salthouse, 2019), may lead older adults to revert to a previously learned response, such as loss aversion, that requires less cognitive effort. Meanwhile, prioritizing the use of abilities that remain intact or even improve with age, such as those that depend on experience, emotional intelligence, and crystallized intelligence, may improve efficiency (Peters et al., 2007; Hess, 2014; Hartshorne and Germine, 2015; Zaval et al., 2015). Similarly, while young adults can take more risk than older adults, risk-seeking as measured in the laboratory is separable from loss aversion (Köbberling and Wakker, 2005). Thus, it is possible for a participant to display a certain level of loss aversion in the face of uncertain gambles but still be risk-seeking when presented different options.
The PCC also is implicated in emotional processing, as it is activated by emotional words (Maddock et al., 2003) and attending to emotional states (Terasawa et al., 2013). Emotional processing is necessary for adaptive decision-making (Loewenstein, 1996; Mellers et al., 1999; Phelps, 2009), and loss aversion is linked to the ability to regulate (Sokol-Hessner et al., 2009, 2012), and process (Bibby and Ferguson, 2011) emotions. Such faculties peak around age 45–60 (Hartshorne and Germine, 2015), and emotional content is particularly salient for older adults (Carstensen and Turk-Charles, 1994; Fung and Carstensen, 2003). Since reliance on emotional information can compensate for age-related declines in cognitively challenging situations (Hanoch et al., 2007; Peters et al., 2007), increases in loss aversion with age may reflect greater focus on emotional or experiential dimensions of decision-making. Related to emotional processing is interoception, which is also associated with the PCC (Kleckner et al., 2017; Stern et al., 2017) and tied to loss aversion (Sokol-Hessner et al., 2015). Thus, age-related cortical thinning in the PCC may hinder the ability to efficiently integrate affective responses into complex choices, especially those that include loss.
The present moment also gains salience with age, and prioritizing immediate or emotional wellbeing may intensify as time horizons constrict (Carstensen, 2006; Löckenhoff, 2011). Converging evidence, including self-reported goal orientations and performance on a probabilistic gambling task (Ebner et al., 2006; Depping and Freund, 2011; Mata and Hertwig, 2011), indicates a shift later in life toward avoiding losses instead of seeking gains. In fact, loss orientation in later adulthood is correlated with subjective well-being (Ebner et al., 2006). When motivations shift toward optimizing immediate, emotional wellbeing and processing power becomes limited with age, perhaps partly because cortical thinning of the PCC impedes probabilistic assessments, loss aversion may naturally emerge as a low-effort response when facing choices with uncertainty.
Higher loss aversion in younger participants and its subsequent decline across young adulthood may similarly reflect the underdevelopment of complex probabilistic decision-making (Weller et al., 2011; Beitz et al., 2014). The Loss Aversion Task requires the time-limited integration of the magnitude and probability of both reward and loss to decide whether the chance of reward is worth the risk of loss; this estimation of subjective value is critical to adaptive choice behavior. Sensitivity to the difference in expected value between options follows an inverted U-shaped function, suggesting that the ability to distinguish appropriately between reward-based options may not fully develop until the mid-20s (Weller et al., 2011).
While the age range of 17 to 54 covered in the current study does not represent the entire lifespan, prior studies point to the trajectory of the quadratic relationship observed here. Loss aversion was a main driver of behavior in children as young as 5–8 years old (Steelandt et al., 2013), and adults older than those examined here (aged 61–86) exhibited greater loss aversion than young adults (Kurnianingsih et al., 2015; O’Brien and Hess, 2020), consistent with the upward trend we observed from ages 35–54. Another limitation of this study is imbalance and relatively small samples of men and women; therefore, conclusive statements about effects of biological sex on loss aversion were not possible. That race/ethnicity was a significant factor in loss aversion also merits further investigation. The lack of an effect of age on risk-taking may reflect the type of task used, as the Loss Aversion Task is not necessarily designed to comprehensively elicit risk preferences. Finally, although there was no significant association between loss aversion and PCC thickness when correcting for multiple comparisons, lack of significance apparently reflected nonlinearities in the relationship – a negative correlation of loss aversion with PCC thickness in older participants, who had smaller PCC thickness, but not in participants whose PCC thickness crossed the inflection point on the U-shaped curve.
We conclude that cortical thickness of the PCC may supplement other cognitive and neurobiological age-related changes and arise as an important factor for loss aversion around the onset of age-related atrophy. Tracking age-related changes in the influence of decision-making biases, such as loss aversion, can inform policies that are tailored to the aging population (Samanez-Larkin, 2013). Moreover, determining the age at which changes begin can introduce opportunities for early intervention, such as services, education, or incentives that could better inform important life decisions, such as those related to health and finances (Johnson and Goldstein, 2003; Agarwal et al., 2009; MacLeod et al., 2017). Identification of brain regions that affect such choices when altered with age provides the opportunity to forecast – and perhaps forestall – future decision-making impairments. To this end, future longitudinal studies may go beyond cross-sectional investigations to use measurements from key brain regions (e.g., PCC) at mid-life to predict changes in decision making biases later in life.
Data Availability Statement
The datasets presented in this study can be found in online repositories. The names of the repository/repositories and accession number(s) can be found below: Open Science Framework under project title “Age Influences Loss Aversion Through Effects on Posterior Cingulate Cortical Thickness” (https://osf.io/ejr56/).
Ethics Statement
The studies involving human participants were reviewed and approved by the University of California, Los Angeles Institutional Review Board. The patients/participants provided their written informed consent to participate in this study.
Author Contributions
DG, AD, EL, and ZG contributed to conception and design of the study. ZG performed the behavioral analysis and performed the statistical analyses and wrote the first draft of the manuscript. J-BP performed the cortical thickness analysis. All authors contributed to manuscript revision, and read and approved the submitted version.
Funding
This research was supported by grants from the National Institutes of Health [K23 DA927734, R21 DA034928 (AD); R01 DA015179, R01 DA020726, and P20 DA022539 (EL)]. Additional funding was provided by UL1TR000124 (UCLA CTSI) and endowments from the Thomas P. and Katherine K. Pike Chair in Addiction Studies (EL) and the Marjorie M. Greene Trust. ZG was supported by F31 DA047110, T32 DA024635, and funding from the Iris Cantor-UCLA Women’s Health Center Executive Advisory Board (NCATS UCLA CTSI Grant Number UL1 TR001881). The funding sources had no role in study design and data collection.
Conflict of Interest
The authors declare that the research was conducted in the absence of any commercial or financial relationships that could be construed as a potential conflict of interest.
Acknowledgments
We thank Russell Poldrack for providing Matlab code for the Loss Aversion Task.
Footnotes
- ^ www.psychtoolbox.org
- ^ http://mc-stan.org/interfaces/rstan
- ^ http://surfer.nmr.mgh.harvard.edu
- ^ https://osf.io/ejr56/
References
Ackert, L. F., Deaves, R., Miele, J., and Nguyen, Q. (2020). Are time preference and risk preference associated with cognitive intelligence and emotional intelligence? J. Behav. Financ. 21, 136–156. doi: 10.1080/15427560.2019.1663850
Agarwal, S., Driscoll, J. C., Gabaix, X., and Laibson, D. (2009). The age of reason: financial decisions over the life cycle and implications for regulation. Brookings Pap. Econ. Act. 2009, 51–117. doi: 10.1353/eca.0.0067
Ahn, W. Y., Haines, N., and Zhang, L. (2017). Revealing neurocomputational mechanisms of reinforcement learning and decision-making with the hBayesDM package. Comput. Psychiatr. 1, 24–57. doi: 10.1162/cpsy_a_00002
Ahn, W.-Y., Krawitz, A., Kim, W., Busmeyer, J. R., and Brown, J. W. (2011). A model-based fMRI analysis with hierarchical bayesian parameter estimation. J. neurosci. Psychol. Econ. 4, 95–110. doi: 10.1037/a0020684
Arora, M., and Kumari, S. (2015). Risk taking in financial decisions as a function of age, gender: mediating role of loss aversion and regret. Int. J. Appl. Psychol. 5, 83–89.
Ballard, M. E., Dean, A. C., Mandelkern, M. A., and London, E. D. (2015a). Striatal dopamine D2/D3 receptor availability is associated with executive function in healthy controls but not methamphetamine users. PLoS One 10:e0143510. doi: 10.1371/journal.pone.0143510
Ballard, M. E., Mandelkern, M. A., Monterosso, J. R., Hsu, E., Robertson, C. L., Ishibashi, K., et al. (2015b). Low dopamine D2/D3 receptor availability is associated with steep discounting of delayed rewards in methamphetamine dependence. Int. J. Neuropsychopharmacol. 18:yu119.
Baltes, P. B., and Lindenberger, U. (1997). Emergence of a powerful connection between sensory and cognitive functions across the adult life span: a new window to the study of cognitive aging? Psychol. Aging 12, 12–21. doi: 10.1037/0882-7974.12.1.12
Beitz, K. M., Salthouse, T. A., and Davis, H. P. (2014). Performance on the Iowa gambling task: from 5 to 89 years of age. J. Exp. Psychol. Gen. 143, 1677–1689. doi: 10.1037/a0035823
Benjamin, A. M., and Robbins, S. J. (2007). The role of framing effects in performance on the balloon analogue risk task (BART). Pers. Individ. Diff. 43, 221–230. doi: 10.1016/j.paid.2006.11.026
Besedeš, T., Deck, C., Sarangi, S., and Shor, M. (2012). Age effects and heuristics in decision making. Rev. Econ. Stat. 94, 580–595. doi: 10.1162/rest_a_00174
Bibby, P. A., and Ferguson, E. (2011). The ability to process emotional information predicts loss aversion. Pers. Individ. Diff. 51, 263–266. doi: 10.1016/j.paid.2010.05.001
Bishop, N. A., Lu, T., and Yankner, B. A. (2010). Neural mechanisms of ageing and cognitive decline. Nature 464, 529–535. doi: 10.1038/nature08983
Brockmole, J. R., and Logie, R. H. (2013). Age-related change in visual working memory: a study of 55,753 participants aged 8–75. Front. Psychol. 4:12. doi: 10.3389/fpsyg.2013.00012
Brown, S. B., and Ridderinkhof, K. R. (2009). Aging and the neuroeconomics of decision making: a review. Cogn. Affect. Behav. Neurosci. 9, 365–379. doi: 10.3758/cabn.9.4.365
Bruine de Bruin, W., Strough, J., and Parker, A. M. (2014). Getting older isn’t all that bad: better decisions and coping when facing “sunk costs”. Psychol. Aging 29, 642–647. doi: 10.1037/a0036308
Canessa, N., Crespi, C., Motterlini, M., Baud-Bovy, G., Chierchia, G., Pantaleo, G., et al. (2013). The functional and structural neural basis of individual differences in loss aversion. J. Neurosci. 33, 14307–14317. doi: 10.1523/jneurosci.0497-13.2013
Carpenter, B., Gelman, A., Hoffman, M. D., Lee, D., Goodrich, B., Betancourt, M., et al. (2017). Stan: a probabilistic programming language. J. Stat. Softw. 76, 1–32. doi: 10.1017/9781108770750.002
Carstensen, L. L. (2006). The influence of a sense of time on human development. Science 312, 1913–1915. doi: 10.1126/science.1127488
Carstensen, L. L., and Turk-Charles, S. (1994). The salience of emotion across the adult life span. Psychol. Aging 9, 259–264. doi: 10.1037/0882-7974.9.2.259
Cassotti, M., Aïte, A., Osmont, A., Houdé, O., and Borst, G. (2014). What have we learned about the processes involved in the Iowa gambling task from developmental studies? Front. Psychol. 5:915. doi: 10.3389/fpsyg.2014.00915
Chen, M. K., Lakshminarayanan, V., and Santos, L. (2005). The evolution of Our Preferences: Evidence from Capuchin Monkey Trading Behavior. Cowles Foundation Discussion Papers 1524. New Haven, CT: Cowles Foundation for Research in Economics, Yale University.
Chen, Y., and Sun, Y. (2003). Age differences in financial decision-making: using simple heuristics. Educ. Gerontol. 29, 627–635. doi: 10.1080/713844418
Dale, A. M., Fischl, B., and Sereno, M. I. (1999). Cortical surface-based analysis: I. Segmentation and surface reconstruction. Neuroimage 9, 179–194.
Dean, A. C., Kohno, M., Morales, A. M., Ghahremani, D. G., and London, E. D. (2015). Denial in methamphetamine users: associations with cognition and functional connectivity in brain. Drug Alcohol Depend. 151, 84–91. doi: 10.1016/j.drugalcdep.2015.03.004
Dean, A. C., Morales, A. M., Hellemann, G., and London, E. D. (2018). Cognitive deficit in methamphetamine users relative to childhood academic performance: link to cortical thickness. Neuropsychopharmacology 43, 1745–1752. doi: 10.1038/s41386-018-0065-1
Dean, A. C., Nurmi, E. L., Morales, A. M., Cho, A. K., Seaman, L. C., and London, E. D. (2020). CYP2D6 genotype may moderate measures of brain structure in methamphetamine users. Addict. Biol. 26:e12950.
Dean, A. C., Sevak, R. J., Monterosso, J. R., Hellemann, G., Sugar, C. A., and London, E. D. (2011). Acute modafinil effects on attention and inhibitory control in methamphetamine-dependent humans. J. Stud. Alcohol Drugs 72, 943–953.
Depping, M. K., and Freund, A. M. (2011). Normal aging and decision making: the role of motivation. Hum. Dev. 54, 349–367. doi: 10.1159/000334396
Desikan, R. S., Ségonne, F., Fischl, B., Quinn, B. T., Dickerson, B. C., Blacker, D., et al. (2006). An automated labeling system for subdividing the human cerebral cortex on MRI scans into gyral based regions of interest. Neuroimage 31, 968–980. doi: 10.1016/j.neuroimage.2006.01.021
Di Rosa, E., Mapelli, D., Arcara, G., Amodio, P., Tamburin, S., and Schiff, S. (2017). Aging and risky decision-making: new ERP evidence from the Iowa gambling task. Neurosci. Lett. 640, 93–98. doi: 10.1016/j.neulet.2017.01.021
Ebner, N. C., Freund, A. M., and Baltes, P. B. (2006). Developmental changes in personal goal orientation from young to late adulthood: from striving for gains to maintenance and prevention of losses. Psychol. Aging 21, 664–678. doi: 10.1037/0882-7974.21.4.664
First, M., Spitzer, R., Gibbon, M., and Williams, J. (1998). Structured Clinical Interview for DSM-IV Patient Edition (SCID-I/PV and SCID-I/NP Version 2.0). New York, NY: Biometric Research Department.
Fischl, B., Van Der Kouwe, A., Destrieux, C., Halgren, E., Ségonne, F., Salat, D. H., et al. (2004). Automatically parcellating the human cerebral cortex. Cereb. Cortex 14, 11–22. doi: 10.1093/cercor/bhg087
Fortin, J.-P., Cullen, N., Sheline, Y. I., Taylor, W. D., Aselcioglu, I., Cook, P. A., et al. (2018). Harmonization of cortical thickness measurements across scanners and sites. Neuroimage 167, 104–120. doi: 10.1016/j.neuroimage.2017.11.024
Fung, H. H., and Carstensen, L. L. (2003). Sending memorable messages to the old: age differences in preferences and memory for advertisements. J. Pers. Soc. Psychol. 85, 163–178. doi: 10.1037/0022-3514.85.1.163
Gächter, S., Johnson, E. J., and Herrmann, A. (2010). Individual-Level Loss Aversion in Riskless and Risky Choices”. Discussion Papers 2010-20. Nottingham: The Centre for Decision Research and Experimental Economics, School of Economics, University of Nottingham.
Ghahremani, D. G., Lee, B., Robertson, C. L., Tabibnia, G., Morgan, A. T., De Shetler, N., et al. (2012). Striatal dopamine D2/D3 receptors mediate response inhibition and related activity in frontostriatal neural circuitry in humans. J. Neurosci. 32, 7316–7324. doi: 10.1523/jneurosci.4284-11.2012
Ghahremani, D. G., Tabibnia, G., Monterosso, J., Hellemann, G., Poldrack, R. A., and London, E. D. (2011). Effect of modafinil on learning and task-related brain activity in methamphetamine-dependent and healthy individuals. Neuropsychopharmacology 36, 950–959. doi: 10.1038/npp.2010.233
Gutchess, A. (2014). Plasticity of the aging brain: new directions in cognitive neuroscience. Science 346, 579–582. doi: 10.1126/science.1254604
Hanoch, Y., Wood, S., and Rice, T. (2007). Bounded rationality, emotions and older adult decision making: not so fast and yet so frugal. Hum. Dev. 50, 333–358. doi: 10.1159/000109835
Hartshorne, J. K., and Germine, L. T. (2015). When does cognitive functioning peak? The asynchronous rise and fall of different cognitive abilities across the life span. Psychol. Sci. 26, 433–443. doi: 10.1177/0956797614567339
Herweg, F., and Mierendorff, K. (2013). Uncertain demand, consumer loss aversion, and flat-rate tariffs. J. Eur. Econ. Assoc. 11, 399–432. doi: 10.1111/jeea.12004
Hess, T. M. (2014). Selective engagement of cognitive resources: motivational influences on older adults’ cognitive functioning. Perspect. Psychol. Sci. 9, 388–407. doi: 10.1177/1745691614527465
Hintze, A., Olson, R. S., Adami, C., and Hertwig, R. (2015). Risk sensitivity as an evolutionary adaptation. Sci. Rep. 5:8242.
Imai, K., Keele, L., and Yamamoto, T. (2010). Identification, inference and sensitivity analysis for causal mediation effects. Stat. Sci. 25, 51–71.
Imai, K., Tingley, D., and Yamamoto, T. (2013). Experimental designs for identifying causal mechanisms. J. R. Stat. Soc. Ser. A (Stat. Soc.) 176, 5–51. doi: 10.1111/j.1467-985x.2012.01032.x
Johnson, E. J., and Goldstein, D. (2003). Do defaults save lives? Science 302, 1338–1339. doi: 10.1126/science.1091721
Jones, H. W., Dean, A. C., Price, K. A., and London, E. D. (2016). Increased self-reported impulsivity in methamphetamine users maintaining drug abstinence. Am. J. Drug Alcohol Abuse 42, 500–506. doi: 10.1080/00952990.2016.1192639
Kable, J. W., and Glimcher, P. W. (2007). The neural correlates of subjective value during intertemporal choice. Nat. Neurosci. 10, 1625–1633. doi: 10.1038/nn2007
Kahneman, D., and Tversky, A. (1979). Prospect theory: an analysis of decision under risk. Econometrica 47, 263–292. doi: 10.2307/1914185
Klapwijk, E. T., Van De Kamp, F., Van Der Meulen, M., Peters, S., and Wierenga, L. M. (2019). Qoala-T: a supervised-learning tool for quality control of FreeSurfer segmented MRI data. Neuroimage 189, 116–129. doi: 10.1016/j.neuroimage.2019.01.014
Kleckner, I. R., Zhang, J., Touroutoglou, A., Chanes, L., Xia, C., Simmons, W. K., et al. (2017). Evidence for a large-scale brain system supporting allostasis and interoception in humans. Nat. Hum. Behav. 1:0069.
Köbberling, V., and Wakker, P. P. (2005). An index of loss aversion. J. Econ. Theory 122, 119–131. doi: 10.1093/acprof:oso/9780199972050.003.0006
Kohno, M., Morales, A. M., Ghahremani, D. G., Hellemann, G., and London, E. D. (2014). Risky decision making, prefrontal cortex, and mesocorticolimbic functional connectivity in methamphetamine dependence. JAMA Psychiatry 71, 812–820. doi: 10.1001/jamapsychiatry.2014.399
Kurnianingsih, Y. A., Sim, S. K. Y., Chee, M. W. L., and Mullette-Gillman, O. D. A. (2015). Aging and loss decision making: increased risk aversion and decreased use of maximizing information, with correlated rationality and value maximization. Front. Hum. Neurosci. 9:280. doi: 10.3389/fnhum.2015.00280
Leech, R., and Sharp, D. J. (2013). The role of the posterior cingulate cortex in cognition and disease. Brain 137, 12–32. doi: 10.1093/brain/awt162
Lemaitre, H., Goldman, A. L., Sambataro, F., Verchinski, B. A., Meyer-Lindenberg, A., Weinberger, D. R., et al. (2012). Normal age-related brain morphometric changes: nonuniformity across cortical thickness, surface area and gray matter volume? Neurobiol. Aging 33, 617.e611–617.e619.
Levy, I., Snell, J., Nelson, A. J., Rustichini, A., and Glimcher, P. W. (2010). Neural representation of subjective value under risk and ambiguity. J. Neurophysiol. 103, 1036–1047. doi: 10.1152/jn.00853.2009
Li, Y., Baldassi, M., Johnson, E. J., and Weber, E. U. (2013). Complementary cognitive capabilities, economic decision making, and aging. Psychol. Aging 28, 595–613. doi: 10.1037/a0034172
Li, Y., Gao, J., Enkavi, A. Z., Zaval, L., Weber, E. U., and Johnson, E. J. (2015). Sound credit scores and financial decisions despite cognitive aging. Proc. Natl. Acad. Sci. U.S.A. 112, 65–69. doi: 10.1073/pnas.1413570112
Lighthall, N. R. (2020). Neural mechanisms of decision-making in aging. Wiley Interdiscip. Rev. Cogn. Sci. 11:e1519.
Löckenhoff, C. E. (2011). Age, time, and decision making: from processing speed to global time horizons. Ann. N. Y. Acad. Sci. 1235, 44–56. doi: 10.1111/j.1749-6632.2011.06209.x
Löckenhoff, C. E. (2018). Aging and decision-making: a conceptual framework for future research–a mini-review. Gerontology 64, 140–148. doi: 10.1159/000485247
Loewenstein, G. (1996). Out of control: visceral influences on behavior. Organ. Behav. Hum. Decis. Process. 65, 272–292. doi: 10.1006/obhd.1996.0028
London, E. D., Okita, K., Kinney, K. R., Dean, A. C., Mcclintick, M. N., Rizor, E. J., et al. (2020). No significant elevation of translocator protein binding in the brains of recently abstinent methamphetamine users. Drug Alcohol Depend. 213:108104. doi: 10.1016/j.drugalcdep.2020.108104
MacLeod, S., Musich, S., Hawkins, K., and Armstrong, D. G. (2017). The growing need for resources to help older adults manage their financial and healthcare choices. BMC Geriatr. 17:84. doi: 10.1186/s12877-017-0477-5
Maddock, R. J., Garrett, A. S., and Buonocore, M. H. (2003). Posterior cingulate cortex activation by emotional words: fMRI evidence from a valence decision task. Hum. Brain Mapp. 18, 30–41. doi: 10.1002/hbm.10075
Markett, S., Heeren, G., Montag, C., Weber, B., and Reuter, M. (2016). Loss aversion is associated with bilateral insula volume. A voxel based morphometry study. Neurosci. Lett. 619, 172–176. doi: 10.1016/j.neulet.2016.03.029
Mata, R., and Hertwig, R. (2011). How to model age-related motivational reorientations in risky choice. Hum. Dev. 54, 368–375. doi: 10.1159/000334943
Mata, R., Schooler, L. J., and Rieskamp, J. (2007). The aging decision maker: cognitive aging and the adaptive selection of decision strategies. Psychol. Aging 22, 796–810. doi: 10.1037/0882-7974.22.4.796
McCoy, A. N., Crowley, J. C., Haghighian, G., Dean, H. L., and Platt, M. L. (2003). Saccade reward signals in posterior cingulate cortex. Neuron 40, 1031–1040. doi: 10.1016/s0896-6273(03)00719-0
Mellers, B., Schwartz, A., and Ritov, I. (1999). Emotion-based choice. J. Exp. Psychol. Gen. 128, 332–345.
Mishina, Y., Dykes, B. J., Block, E. S., and Pollock, T. G. (2010). Why “good” firms do bad things: The effects of high aspirations, high expectations, and prominence on the incidence of corporate illegality. Acad. Manag. J. 53, 701–722. doi: 10.5465/amj.2010.52814578
Moeller, S. J., Okita, K., Robertson, C. L., Ballard, M. E., Konova, A. B., Goldstein, R. Z., et al. (2018). Low striatal dopamine D2-type receptor availability is linked to simulated drug choice in methamphetamine users. Neuropsychopharmacology 43, 751–760. doi: 10.1038/npp.2017.138
Morales, A. M., Kohno, M., Robertson, C. L., Dean, A. C., Mandelkern, M. A., and London, E. D. (2015a). Gray-matter volume, midbrain dopamine D2/D3 receptors and drug craving in methamphetamine users. Mol. Psychiatry 20, 764–771. doi: 10.1038/mp.2015.47
Morales, A. M., Kohno, M., Robertson, C. L., Dean, A. C., Mandelkern, M. A., and London, E. D. (2015b). Midbrain dopamine D2/D3 receptor availability and drug craving are associated with mesocorticolimbic gray matter volume in methamphetamine users. Mol. Psychiatry 20, 658–658. doi: 10.1038/mp.2015.59
Morales, A. M., Lee, B., Hellemann, G., O’neill, J., and London, E. D. (2012). Gray-matter volume in methamphetamine dependence: cigarette smoking and changes with abstinence from methamphetamine. Drug Alcohol Depend. 125, 230–238. doi: 10.1016/j.drugalcdep.2012.02.017
O’Brien, E. L., and Hess, T. M. (2020). Differential focus on probability and losses between young and older adults in risky decision-making. Aging Neuropsychol. Cogn. 27, 532–552. doi: 10.1080/13825585.2019.1642442
Okita, K., Ghahremani, D. G., Payer, D. E., Robertson, C. L., Dean, A. C., Mandelkern, M. A., et al. (2016a). Emotion dysregulation and amygdala dopamine D2-type receptor availability in methamphetamine users. Drug Alcohol Depend. 161, 163–170. doi: 10.1016/j.drugalcdep.2016.01.029
Okita, K., Ghahremani, D. G., Payer, D. E., Robertson, C. L., Mandelkern, M. A., and London, E. D. (2016b). Relationship of alexithymia ratings to dopamine D2-type receptors in anterior cingulate and insula of healthy control subjects but not methamphetamine-dependent individuals. Int. J. Neuropsychopharmacol. 19:yv129.
Okita, K., Mandelkern, M. A., and London, E. D. (2016c). Cigarette use and striatal dopamine D2/3 receptors: possible role in the link between smoking and nicotine dependence. Int. J. Neuropsychopharmacol. 19:yw074.
Okita, K., Morales, A. M., Dean, A. C., Johnson, M. C., Lu, V., Farahi, J., et al. (2018). Striatal dopamine D1-type receptor availability: no difference from control but association with cortical thickness in methamphetamine users. Mol. Psychiatry 23, 1320–1327. doi: 10.1038/mp.2017.172
Pachur, T., Mata, R., and Hertwig, R. (2017). Who dares, who errs? Disentangling cognitive and motivational roots of age differences in decisions under risk. Psychol. Sci. 28, 504–518. doi: 10.1177/0956797616687729
Payer, D., Nurmi, E., Wilson, S., Mccracken, J., and London, E. D. (2012). Effects of methamphetamine abuse and serotonin transporter gene variants on aggression and emotion-processing neurocircuitry. Transl. Psychiatry 2:e80. doi: 10.1038/tp.2011.73
Pearson, J. M., Heilbronner, S. R., Barack, D. L., Hayden, B. Y., and Platt, M. L. (2011). Posterior cingulate cortex: adapting behavior to a changing world. Trends Cogn. Sci. 15, 143–151. doi: 10.1016/j.tics.2011.02.002
Peters, E., Hess, T. M., Västfjäll, D., and Auman, C. (2007). Adult age differences in dual information processes: implications for the role of affective and deliberative processes in older adults’ decision making. Perspect. Psychol. Sci. 2, 1–23. doi: 10.1111/j.1745-6916.2007.00025.x
Phelps, E. A. (2009). “The study of emotion in neuroeconomics,” in Neuroeconomics: Decision Making and the Brain, eds P. W. Glimcher, C. F. Camerer, E. Fehr, and R. A. Poldrack (Amsterdam: Elsevier Academic Press), 233–250. doi: 10.1016/b978-0-12-374176-9.00016-6
Read, D., and Read, N. L. (2004). Time discounting over the lifespan. Organ. Behav. Hum. Decis. Process. 94, 22–32. doi: 10.1016/j.obhdp.2004.01.002
Robson, A. J. (1996). A biological basis for expected and non-expected utility. J. Econ. Theory 68, 397–424. doi: 10.1006/jeth.1996.0023
Rutledge, R. B., Smittenaar, P., Zeidman, P., Brown, H. R., Adams, R. A., Lindenberger, U., et al. (2016). Risk taking for potential reward decreases across the lifespan. Curr. Biol. CB 26, 1634–1639. doi: 10.1016/j.cub.2016.05.017
Salat, D. H., Buckner, R. L., Snyder, A. Z., Greve, D. N., Desikan, R. S. R., Busa, E., et al. (2004). Thinning of the cerebral cortex in aging. Cereb. Cortex 14, 721–730. doi: 10.1093/cercor/bhh032
Salthouse, T. A. (2019). Trajectories of normal cognitive aging. Psychol. Aging 34, 17–24. doi: 10.1037/pag0000288
Schleich, J., Gassmann, X., Meissner, T., and Faure, C. (2019). A large-scale test of the effects of time discounting, risk aversion, loss aversion, and present bias on household adoption of energy-efficient technologies. Energy Econ. 80, 377–393. doi: 10.1016/j.eneco.2018.12.018
Schunk, D., and Winter, J. (2009). The relationship between risk attitudes and heuristics in search tasks: a laboratory experiment. J. Econ. Behav. Organ. 71, 347–360. doi: 10.1016/j.jebo.2008.12.010
Seaman, K. L., Green, M. A., Shu, S., and Samanez-Larkin, G. R. (2018). Individual differences in loss aversion and preferences for skewed risks across adulthood. Psychol. Aging 33, 654–659. doi: 10.1037/pag0000261
Sokol-Hessner, P., Camerer, C. F., and Phelps, E. A. (2012). Emotion regulation reduces loss aversion and decreases amygdala responses to losses. Soc. Cogn. Affect. Neurosci. 8, 341–350. doi: 10.1093/scan/nss002
Sokol-Hessner, P., Hartley, C. A., Hamilton, J. R., and Phelps, E. A. (2015). Interoceptive ability predicts aversion to losses. Cogn. Emotion 29, 695–701. doi: 10.1080/02699931.2014.925426
Sokol-Hessner, P., Hsu, M., Curley, N. G., Delgado, M. R., Camerer, C. F., and Phelps, E. A. (2009). Thinking like a trader selectively reduces individuals’ loss aversion. Proc. Natl. Acad. Sci. 106, 5035–5040. doi: 10.1073/pnas.0806761106
Stamatis, C. A., Puccetti, N. A., Charpentier, C. J., Heller, A. S., and Timpano, K. R. (2020). Repetitive negative thinking following exposure to a natural stressor prospectively predicts altered stress responding and decision-making in the laboratory. Behav. Res. Ther. 129:103609. doi: 10.1016/j.brat.2020.103609
Steelandt, S., Broihanne, M.-H., Romain, A., Thierry, B., and Dufour, V. (2013). Decision-making under risk of loss in children. PLoS One 8:e52316. doi: 10.1371/journal.pone.0052316
Stern, E. R., Grimaldi, S. J., Muratore, A., Murrough, J., Leibu, E., Fleysher, L., et al. (2017). Neural correlates of interoception: effects of interoceptive focus and relationship to dimensional measures of body awareness. Hum. Brain Mapp. 38, 6068–6082. doi: 10.1002/hbm.23811
Storsve, A. B., Fjell, A. M., Tamnes, C. K., Westlye, L. T., Overbye, K., Aasland, H. W., et al. (2014). Differential longitudinal changes in cortical thickness, surface area and volume across the adult life span: regions of accelerating and decelerating change. J. Neurosci. 34, 8488–8498. doi: 10.1523/jneurosci.0391-14.2014
Tamnes, C. K., Østby, Y., Fjell, A. M., Westlye, L. T., Due-Tønnessen, P., and Walhovd, K. B. (2009). Brain maturation in adolescence and young adulthood: regional age-related changes in cortical thickness and white matter volume and microstructure. Cereb. Cortex 20, 534–548. doi: 10.1093/cercor/bhp118
Terasawa, Y., Fukushima, H., and Umeda, S. (2013). How does interoceptive awareness interact with the subjective experience of emotion? An fMRI Study. Hum. Brain Mapp. 34, 598–612.
Tom, S. M., Fox, C. R., Trepel, C., and Poldrack, R. A. (2007). The neural basis of loss aversion in decision-making under risk. Science 315, 515–518. doi: 10.1126/science.1134239
Tversky, A., and Kahneman, D. (1992). Advances in prospect theory: cumulative representation of uncertainty. J. Risk Uncertain. 5, 297–323. doi: 10.1007/bf00122574
Tymula, A., Rosenberg Belmaker, L. A., Ruderman, L., Glimcher, P. W., and Levy, I. (2013). Like cognitive function, decision making across the life span shows profound age-related changes. Proc. Natl. Acad. Sci. U.S.A. 110, 17143–17148. doi: 10.1073/pnas.1309909110
United Nations (2019). World Population Prospects 2019, Online Edition. Rev. 1. U.N. Department of Economic and Social Affairs–Population Division. New York, NY: United Nations.
Verhaeghen, P., and Salthouse, T. A. (1997). Meta-analyses of age–cognition relations in adulthood: Estimates of linear and nonlinear age effects and structural models. Psychol. Bull. 122, 231–249. doi: 10.1037/0033-2909.122.3.231
Vidal-Pineiro, D., Parker, N., Shin, J., French, L., Grydeland, H., Jackowski, A. P., et al. (2020). Cellular correlates of cortical thinning throughout the lifespan. Sci. Rep. 10:21803.
Wechsler, D. (2001). Wechsler Test of Adult Reading: WTAR. San Antonio, TX: Psychological Corporation.
Weller, J. A., Levin, I. P., and Denburg, N. L. (2011). Trajectory of risky decision making for potential gains and losses from ages 5 to 85. J. Behav. Decis. Mak. 24, 331–344. doi: 10.1002/bdm.690
Wood, S., Busemeyer, J., Koling, A., Cox, C. R., and Davis, H. (2005). Older adults as adaptive decision makers: evidence from the Iowa gambling task. Psychol. Aging 20, 220–225. doi: 10.1037/0882-7974.20.2.220
Xu, P., Van Dam, N. T., Van Tol, M.-J., Shen, X., Cui, Z., Gu, R., et al. (2020). Amygdala–prefrontal connectivity modulates loss aversion bias in anxious individuals. Neuroimage 218:116957. doi: 10.1016/j.neuroimage.2020.116957
Zaval, L., Li, Y., Johnson, E. J., and Weber, E. U. (2015). “Chapter 8–Complementary contributions of fluid and crystallized intelligence to decision making across the life span,” in Aging and Decision Making, eds T. M. Hess, J. Strough, and C. E. Löckenhoff (San Diego, CA: Academic Press), 149–168. doi: 10.1016/b978-0-12-417148-0.00008-x
Zhang, R., Brennan, T. J., and Lo, A. W. (2014). The origin of risk aversion. Proc. Natl. Acad. Sci. U.S.A. 111, 17777–17782.
Keywords: decision-making, loss aversion, aging, cortical thickness, posterior cingulate, neuroimaging
Citation: Guttman ZR, Ghahremani DG, Pochon J-B, Dean AC and London ED (2021) Age Influences Loss Aversion Through Effects on Posterior Cingulate Cortical Thickness. Front. Neurosci. 15:673106. doi: 10.3389/fnins.2021.673106
Received: 26 February 2021; Accepted: 15 June 2021;
Published: 12 July 2021.
Edited by:
N. Hinvest, University of Bath, United KingdomReviewed by:
Dieter J. Meyerhoff, University of California, San Francisco, United StatesLucy F. Ackert, Kennesaw State University, United States
Copyright © 2021 Guttman, Ghahremani, Pochon, Dean and London. This is an open-access article distributed under the terms of the Creative Commons Attribution License (CC BY). The use, distribution or reproduction in other forums is permitted, provided the original author(s) and the copyright owner(s) are credited and that the original publication in this journal is cited, in accordance with accepted academic practice. No use, distribution or reproduction is permitted which does not comply with these terms.
*Correspondence: Edythe D. London, elondon@mednet.ucla.edu