- 1McLean Imaging Center, McLean Hospital, Belmont, MA, United States
- 2Harvard Medical School, Boston, MA, United States
- 3Department of Psychology, Rutgers University, Newark, NJ, United States
The ability to perceive and exercise control is a major contributor to our mental and physical wellbeing. When faced with uncontrollable aversive stimuli, organisms develop heightened anxiety and become unwilling to exert effort to avoid the stimuli. In contrast, when faced with controllable aversive stimuli, organisms demonstrate behavioral vigor via avoidance attempts toward trying to seek and exercise control over the environment. As such, controllability confers protective effects against reduced avoidance motivation trigged by aversive environments. These observations beg the question of whether controllability can be potent enough to reverse passivity following repeated exposure to uncontrollable aversive stimuli and how this protective effect is encoded neurally. Human participants performed a Control in Aversive Domain (CAD) task where they were first subjected to a series of repeated uncontrollable aversive stimuli (i.e., aversive tones) across several contexts that were followed by a series of controllable aversive stimuli in a novel context. Faced with persistent uncontrollability, participants significantly reduced their avoidance attempts over time and biased toward giving up. However, the subsequent presence of controllability rescued participants’ avoidance behavior. Strikingly, participants who responded more strongly to the protective effects of control also had greater ventromedial prefrontal cortical (vmPFC) activation—a region previously observed to be associated with encoding the subjective value of control. Taken together, these findings highlighted the protective effect conferred by perceived control against passivity and offered insights into the potential role of the vmPFC in controllable environments, with implications for understanding the beneficial influence of perceived control on adaptive behavior.
Introduction
Our sense of control is governed by our perceived ability to influence the environment. This ability to perceive and exercise control—henceforth referred to as perceived control—serves an important role to help maintain and support a healthy psychological and physical state. As such, when an organism is faced with a situation where controllability is diminished or altogether absent, as represented by the dissociation of behavior and outcome, it is prone to develop passivity and heightened anxiety (Rodin, 1986; Wallston et al., 1987; Ryan and Deci, 2000). Passivity can translate into the action of giving up where the organism exhibits no overt avoidance or escape behaviors. This passivity and the associated reduction in avoidance motivation is often exacerbated in aversive environments, particularly when the organism is made to repeatedly endure uncontrollable aversive stimuli (for review see Maier and Seligman, 2016).
Prior work exploring the behavioral effects of perceived control have found that both animals and humans work harder and longer to obtain rewards or to avoid aversive outcomes when they perceive the environment to be controllable (Bongard, 1995; Bhanji et al., 2016; Wang et al., 2020). Moreover, when given the ability to do something about the external environment either in the form of performing an avoidance behavior (Hiroto, 1974) or granted the opportunity to exercise a choice (Rodin and Langer, 1977), human participants report stronger positive emotions and enhanced self-competence (Rodin, 1986; Deci and Ryan, 1987; Ly et al., 2019). In the same vein, having this perception of control is often also accompanied by stronger intrinsic motivation to learn to avoid or escape from aversive stimuli (Holmes and Jackson, 1975; Taub and Dollinger, 1975; Maier and Seligman, 1976; Quaglieri, 1980; Feather and Volkmer, 1988; Trusty and Macan, 1995). Given these findings, it is argued that perceived control over the environment has a protective effect on an organism’s avoidance motivation, particularly in an aversive environment that otherwise tends to induce passivity. When an organism believes that its behavior can reliably bring about desired outcomes, the organism—endowed with perceived control—is protected from feeling helpless in an aversive environment and maintains avoidance motivation. One open question that follows is whether the protective effect conferred by perceived control can reverse passivity associated with repeated exposure to uncontrollable aversive environment.
Here, we examined the protective effects of perceived control on human participants’ avoidance behavior and studied how such induced behavioral changes are subserved neurally. Previous research has largely implicated the ventromedial prefrontal cortex (vmPFC; Amat et al., 2005; Christianson et al., 2009) and the striatum (Leotti and Delgado, 2011, 2014) as critical regions associated with perceiving a sense of control. The vmPFC in particular, is important in encoding the subjective value of perceived control—how much people prefer to exert vs. give up control in order to attain a reward (Wang and Delgado, 2019). As such, we hypothesize that perceived control can not only engender avoidance behavior changes via its protective effects, it is likely that the vmPFC is involved in mediating such behavioral changes.
To test our hypotheses, we adapted a prior aversive behavioral paradigm using auditory tones (Hiroto, 1974) to design the Control in Aversive Domain (CAD) task. Briefly, the CAD task consisted of three different phases (i.e., exposure, uncontrollable, and controllable). The objective of the task was to first expose participants to uncontrollability (in the exposure phase), followed by testing for decreases in avoidance behavior (i.e., passivity) in a novel aversive but uncontrollable context (in the uncontrollable phase), and finally study the protective effects of controllability in rescuing avoidance behavior in a novel aversive context (in the controllable phase). To capture avoidance behavior and passivity, we asked participants to choose between an AVOID and a GIVE-UP option. Specifically, participants were asked to either make an active attempt to try and avoid the aversive stimulus (AVOID option) or make an active decision to give up trying to avoid the stimulus and accept it (GIVE-UP option). We predicted that the protective effects of controllability would be potent enough to rescue participants’ avoidance behavior and this behavioral change would be subserved by the vmPFC.
Materials and Methods
Participants
Thirty-one right-handed individuals (11 Males and 20 Females) between the ages of 18 and 37 [Mean (M) = 23.3, standard deviation (SD) = 5.1] were recruited from the Rutgers University community to perform two separate functional tasks: a Value of Control paradigm (published in Wang and Delgado, 2019) and the Control in Aversive Domain task (included here). Participants were prescreened for any history of psychiatric and neurological illness. They were given monetary compensation for their voluntary participation in the experiment. All participants provided written informed consent in accordance with the experimental protocol approved by the Rutgers University Institutional Review Board. Three participants did not complete the experiment due to equipment failure, while two participants were excluded from subsequent analyses due to complications (e.g., experienced phobia during the scanning session). Four participants were excluded based on the criteria that they had >50% missed trials in at least one experimental run. The final participant count was 22 (8 Males and 14 Females; M = 23.3, SD = 4.58), consistent with the desired sample size of 19, obtained from a power analysis for paired t-test conducted using G∗Power (version 3.1; Faul et al., 2007) according to the guidelines established by Cohen, 1992 (alpha = 0.05, power = 0.9, effect size = 0.8).
Experimental Task and Design
We adapted a behavioral paradigm implemented in both animals (Maier and Seligman, 1976) and humans (Hiroto, 1974) to design the Control in Aversive Domain (CAD) neuroimaging task and tested the overarching hypothesis that vmPFC subserves the protective effects of controllability on avoidance behavior in an aversive context. This task was conceived with four factors in mind: (a) demonstrate behavioral differences in response to uncontrollable aversive and neutral contexts in a within-subject design; (b) repeated and uninterrupted exposure to uncontrollability across several contexts to examine its behavioral effect; (c) test for behavioral changes in response to controllability in a novel context after the exposure to repeatedly uncontrollability; (d) require participants to choose between AVOID or GIVE-UP options with a button press in order to balance motor- and effort- related confounds for neuroimaging analyses.
The CAD task comprised three sequential phases: exposure, uncontrollable, and controllable. In the exposure phase, we tested participants’ avoidance responses toward two cues (i.e., colored shapes) paired respectively with an aversive (i.e., 4,000 Hz) and a neutral (i.e., 500 Hz) tone. Both cues were presented in an uncontrollable context where participants had no real behavioral control and could never successfully avoid the tones. In the ensuing uncontrollable and controllable phases, we paired two novel cues respectively with a controllable and an uncontrollable aversive tone to investigate changes in participants’ avoidance behavior toward these novel contexts. Each phase will be described in more details in subsequent sections.
At the beginning of the experiment, participants were first asked to hear and rate the aversiveness [i.e., “How aversive (unpleasant) is the tone?”] of two tones on a Likert scale of 1–7. The aversive and neutral tones were designed to match in terms of their amplitude at 75 decibels (i.e., loudness), but differed in their frequency (i.e., pitch). The presentation of the tones was counterbalanced across participants.
Run Structure
The experiment was divided into four sequential runs each lasting 202 s: two exposure phase runs, one uncontrollable phase run and one controllable phase run (Figure 1A). We had quick verbal check-ins with participants after each run to ensure that they were doing okay in the scanner and was ready to continue onto the next run. We did not explicitly inform participants of the structure of the task (e.g., sequence and nature of the different phases) other than the trial structure. Specifically, all four runs featured identical instructions with no explicit mentioning of controllability or uncontrollability. Each phase also featured a novel cue (i.e., colored shape)—that participants were aware of—paired with either an aversive or a neutral tone (see section “Trial Structure” for further details), which make each phase a novel environment to probe for changes in participants’ avoidance behavior across the runs.
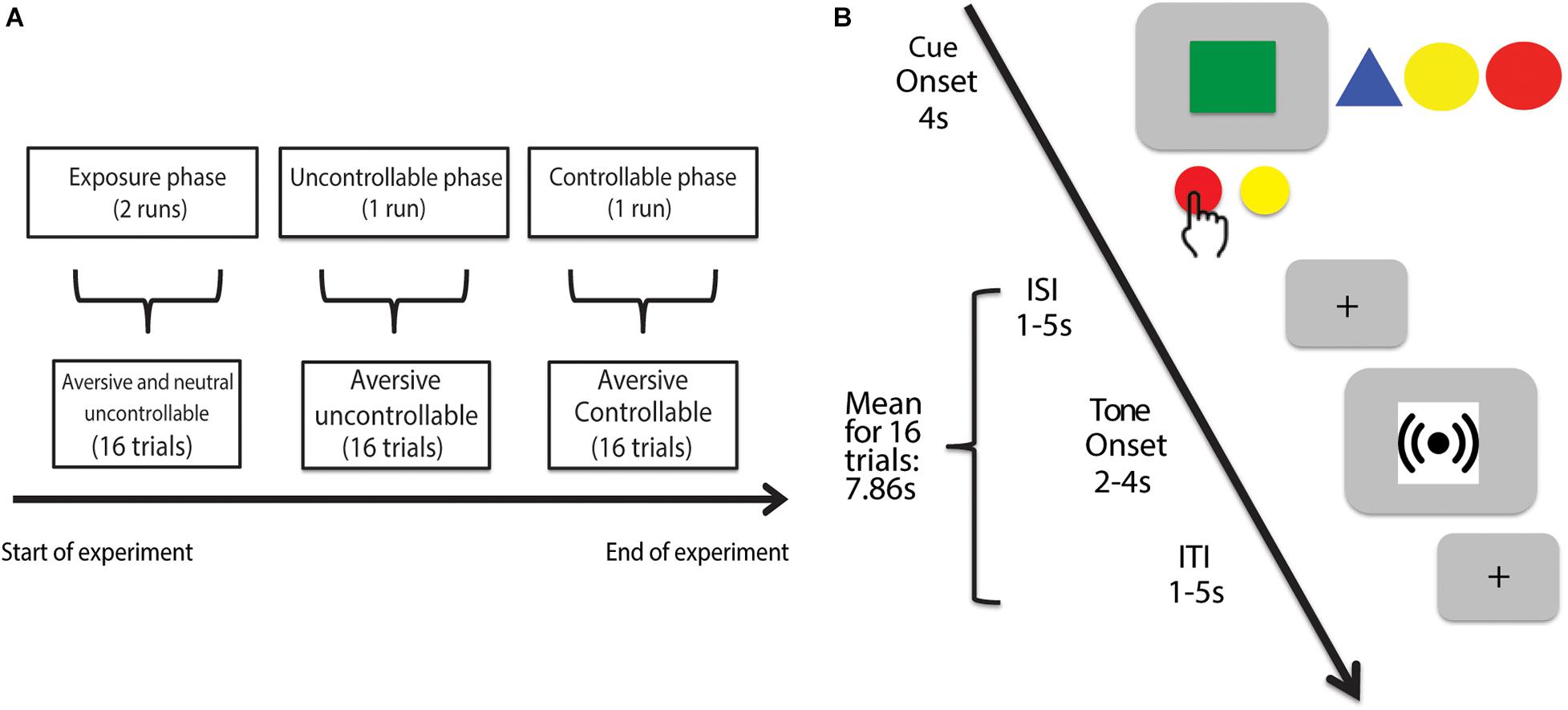
Figure 1. Experimental timeline of Control in Aversive Domain (CAD) task. (A) The CAD task consisted of three phases. The first phase comprised the exposure trials where participants responded to uncontrollable cues paired with either an aversive (4,000 Hz) or neutral (500 Hz) tone. The second phase comprised the uncontrollable trials where participants experienced a series of uncontrollable aversive tones (4,000 Hz) represented by a different cue compared to the exposure trials. The last phase comprised the controllable trials where participants were given a series of controllable aversive tones (4,000 Hz) paired with yet another novel cue. (B) Example trial. In each trial, regardless of the experimental phase, participants were presented a cue (all experimental cues shown for completeness) displayed for 4 s. During the cue presentation for each trial, participants had the option to either press the AVOID or GIVE-UP button. By choosing to press the AVOID button, participants could try to control and avoid the associated tone. A successful AVOID button press yielded no tone presentation whereas a failed AVOID button press yielded 4 s of tone. By choosing the GIVE-UP button, participants would receive a guaranteed 2 s of the associated tone. The cue and tone periods respectively ended with a jittered interstimulus and intertrial interval signaled by a crosshair.
Exposure Phase
The exposure phase runs each featured one block of 8 aversive trials and one block of 8 neutral trials. The block presentation order was counterbalanced across participants. All trials in the exposure phase were uncontrollable where participants never received correct feedback (no tone) for any AVOID button presses made in the cue presentation period despite being instructed that they can try to avoid the tone with the AVOID button. Participants received negative feedback (4s tone) to signal that they had no effective behavioral control over the tone presentation. All participants underwent two consecutive exposure phase runs (i.e., early and late runs) with the same set of aversive and neutral cue-tone pairings.
Uncontrollable and Controllable Phases
The uncontrollable and controllable phases each comprised 16 aversive trials and introduced new cues that were different from those that the participants experienced in the exposure phase. The uncontrollable phase featured uncontrollable aversive trials where participants always received the incorrect feedback (i.e., 4s tone) for AVOID button presses but never the correct feedback (0s tone). In contrast, the controllable phase provided participants with correct feedback with a deterministic feedback schedule (that was not revealed to participants and does not depend on the timing of AVOID button press)—the first AVOID button press always yielded an incorrect feedback and each subsequent AVOID button press was on an interleaved 50% correct/incorrect schedule. We set the outcome for the first AVOID press to be incorrect to account for participants making an AVOID press by accident. In addition, we chose this schedule to ensure that all participants received the same order of positive and negative feedback regardless of which trial number they made an initial AVOID button press.
Trial Structure
A trial (Figure 1B) consisted of a 4s cue period and a 4s tone period, separated by a jittered 1–5 s inter-stimulus interval (ISI) and concluded with a jittered 1–5 s inter-trial interval (ITI). All trial structures were consistent across experimental phases.
Within the cue period, participants were shown one of four different visual cues represented by shapes of different color (e.g., green square). These cues were individually paired with either the aversive or neutral tone. During cue presentation, participants were instructed to make a choice between pressing the AVOID or the GIVE-UP button. The AVOID button was described to participants as having a correct response for each cue—they had to figure out which specific 1 s interval was the correct timing to press the AVOID button in order to successfully avoid the tone. In reality, the feedback for their AVOID button presses was deterministic so as to permit us to manipulate whether the cue represented a controllable or uncontrollable context. For instance, in an uncontrollable context, participants were never given correct feedback (no tone) for any AVOID button presses, hence inducing them to perceive no behavioral control over the tone outcome.
In comparison, the GIVE-UP button did not have an associated correct/incorrect feedback. Instead, participants were informed that pressing it anytime during the cue period would always lead to the expected presentation of an abbreviated 2s-tone. This abbreviated tone associated with the GIVE-UP button was designed to best mimic animal studies where rodents, in response to uncontrollable contexts, chose the lesser of two evils by becoming behaviorally passive (i.e., no longer exerting effort) and conserving energy to accept the impending aversive outcome. This parallels our task where the act of pressing the GIVE-UP button translates into participants knowingly accept that they would get a shortened but guaranteed tone with no chance of avoiding it.
Participants were instructed that only one button press (the first one) was allowed to register for each cue period. To disincentivize participants from choosing the GIVE-UP button for a shorter experimental duration, 2 s was added to the inter-trial interval (ITI) whenever a GIVE-UP button press was made so that all trials had the same length. Any missed response during the 4s-window cue period was registered as a missed trial that resulted in 4s of tone presentation and carried a $1 monetary penalty on the experimental compensation.
Neuroimaging Data Acquisition
Images were collected using a 3T Siemens MAGNETOM Trio scanner with the 12-channel head at the Rutgers University Brain Imaging Center (RUBIC). High-resolution structural images encompassing the whole brain were acquired using a T1-weighted magnetization-prepared rapid gradient echo (MPRAGE) sequence (repetition time (TR): 1,900 ms; echo time (TE): 2.52 ms; matrix 256 × 256; field of view (FOV): 256 mm; voxel size 1.0 × 1.0 × 1.0 mm; 176 slices; flip angle: 9°). The blood-oxygenation-level-dependent (BOLD) functional images were obtained using a single-shot T2∗-weighted echo-planar imaging (EPI) sequence (TR: 2,000 ms; TE: 25 ms; matrix 64 × 64; FOV: 192 mm; voxel size 3.0 × 3.0 × 3.0 mm; 35 slices (0% gap); flip angle: 90°). In addition, B0 field maps (TR: 400 ms; TE1: 5.19 ms; TE2: 7.65 ms; matrix 64 × 64; FOV: 192 mm; voxel size 3.0 × 3.0 × 3.0 mm; 35 slices (0% gap); flip angle: 60°) were collected prior to the functional images to correct for geometric distortion in the functional images.
FMRI Preprocessing
The neuroimaging data were preprocessed using SPM12 (Ashburner, 2012)1. First, we defined the origin of each image to align with the anterior and posterior commissure plane (Ardekani and Bachman, 2009). After we motion-corrected each time series to its first volume, we then performed spatial unwarping to minimize geometric distortions due to susceptibility artifacts (Andersson et al., 2001; Hutton et al., 2002). Next, we coregistered the mean functional image to the anatomical scan and normalized the anatomical using the unified segmentation model (Ashburner and Friston, 2005). The normalized anatomical was subsequently used to reslice the functional data to standard stereotaxic space defined by the Montreal Neurological Institute (MNI). We applied a spatial smoothing at full-width half-maximize of 6 mm to the normalized functional data.
To minimize the impact of head motion on the neuroimaging data, we applied additional preprocessing steps using tools from FSL (FMRIB Software Library version 5.0.4; Smith et al., 2004)2. We detected motion spikes using the FSL tools fsl_motion_outliers. The motion spikes were evaluated with two metrics: (1) root-mean-square (RMS) intensity difference of each volume relative to the reference volume obtained from the first time point; and (2) frame-wise displacements calculated as the mean RMS change in rotation/translation parameters relative to the same reference volume. We subjected the metric values within a run to a boxplot threshold (75th percentile plus 1.5 times the interquartile range) and labeled volumes as spikes, which were subsequently removed via regression (Satterthwaite et al., 2013; Power et al., 2015). Across all participants, this method removed 6.2% of volumes (range: 0.99–13.6%). After the removal of motion spikes, no participants exhibited extreme average volume-to-volume head motion (M = 0.058 mm; range: 0.027–0.10 mm) or maximum volume-to-volume head motion (M = 0.13mm; range: 0.060–0.26 mm). Following the removal of motion spikes, we extracted brain material from the functional images (Smith, 2002) and normalized the entire 4D dataset using a single scaling factor (grand-mean intensity scaling). Images were then processed through the SUSAN (Smallest Univalue Segment Assimilating Nucleus) noise reduction filter, part of the FSL software package, using a 2 mm kernel (Smith and Brady, 1997). This step allowed us to achieve greater signal-to-noise ratio while preserving the image structure. Lastly, we applied a high-pass temporal filter with a 100 s cutoff (Gaussian-weighted least-squares straight line fitting, with sigma = 50 s) to remove low frequency drift in the MR signal. Applying the temporal filter after the removal of motion spikes helps to minimize ringing artifacts (Weissenbacher et al., 2009; Carp, 2013; Satterthwaite et al., 2013).
Data Analysis
Behavioral Analysis of Choices in the CAD Task
We were primarily interested in participants’ avoidance behavior toward the aversive tone across the different experimental phases (i.e., exposure, uncontrollable, and controllable). First, in the exposure phase, we tested for differences in both total avoidance attempts and changes in avoidance behavior over time associated with the aversive and neutral tones. Second, using a novel cue in the uncontrollable phase, we studied the development of passivity and their reduced avoidance motivation in participants exposed to aversive contexts. And finally, by presenting participants with a controllable but aversive context in the last controllable phase, we investigated the protective effects of controllability on avoidance behavior.
Exposure Phase
We first established any potential differences in participants subjective tone ratings and their AVOID button presses between the aversive and neutral trials using paired t-tests. We then conducted a repeated-measure two-way ANOVA examining the effect of time (i.e., early vs. late run) and cue type (i.e., aversive vs. neutral) on the number of AVOID button presses. Overall, we hypothesized that participants would rate the 4,000 Hz tone to be more aversive and show more avoidance behavior in the aversive compared to the neutral trials, particularly in the early aversive trials.
Uncontrollable and Controllable Phases
We first compared the aversive trials in the exposure and uncontrollable phases utilizing a paired t-test to examine changes in avoidance behavior. We hypothesized that participants would show more avoidance behavior in the exposure compared to the uncontrollable phase. Furthermore, we modeled participants’ proportion of AVOID button presses in the exposure phase into a probit regression to investigate whether the aversive or neutral exposure avoidance behavior predicted participants’ avoidance behavior in the uncontrollable phase. We hypothesized that the aversive exposure compared to the neutral trials would better predict participants’ avoidance behavior in the uncontrollable phase.
For the controllable phase, we implemented a paired t-test to probe any differences in the proportion of AVOID button presses made in the uncontrollable compared to controllable phase. We predicted that participants would demonstrate more avoidance behavior in the controllable compared to the uncontrollable trials. Finally, to examine the avoidance behavior across all three aversive phases, we analyzed the proportion of AVOID button presses using time as the factor (i.e., early exposure, late exposure, uncontrollable, controllable) in a repeated-measure one-way ANOVA model to test for behavioral differences.
Neuroimaging Analysis
Neuroimaging analyses were carried out with FSL FEAT (FMRI Expert Analysis Tool) Version 6.0 (Smith et al., 2004). All of the general linear models (GLM) described below included a reaction time (RT) regressor of no-interest for the cue period with the duration set to the RT of a button press in each cue period and an intensity of one. We regressed out the RTs for each cue period in order to remove RT-related confounds that were unrelated to participants’ choices between AVOID and GIVE-UP presses. All GLMs described below also included regressors of no-interest for any missed trials for the cue period with the duration set to 4 s and an intensity of one. For the first-level analysis, the regressors in all the general linear models (GLM) were convolved with the canonical hemodynamic response function and incorporated temporal derivatives and temporal filtering.
At the group-level analysis, we performed a mixed-effects one-sample t-tests using FEAT’s FLAME 1 + 2, which first fits the model using Bayesian modeling for mixed-effects variance estimation before processing all voxels that were close to threshold using the Metropolis-Hastings Markov Chain Monte Carlo sampling to obtain a more precise estimation of the mixed-effect variance (Woolrich et al., 2004). Unless stated otherwise, for all z-statistics images discussed, we thresholded and corrected for multiple comparisons across the whole brain using a false-discovery rate-corrected voxel-extent threshold of p < 0.05 (Worsley, 2001; Lieberman and Cunningham, 2009). We used MRIcroN and MRIcroGL to create the statistical overlay images (Rorden et al., 2007)3.
Exposure Phase
For the exposure phase, we were interested in differences in neural responses toward aversive and neutral cues in the early and late trials. To investigate this question, we performed a 2 (aversive vs. neutral) × 2 (early vs. late) ANOVA. This ANOVA allowed us to probe whether the context (i.e., aversive or neutral) influenced participants to exhibit different cue responses to the initial and latter stages of learning to avoid uncontrollable tones. Building on our behavioral predictions, we hypothesized that participants would react to the aversive cue more unfavorably, particularly in the late trials when they have learned that the cue was unavoidable, due to the 2-fold setbacks of aversive context coupled with uncontrollability. Based on previous studies implicating the amygdala and ventral striatum in aversive learning (Schoenbaum and Setlow, 2003; Kienast et al., 2008), we hypothesized that participants would show greater activation in these regions when we examine the contrast of aversive – neutral × late – early interaction.
For the first-level GLM analysis, we modeled participant-specific design matrices for each run with the following regressors: (1) a linear regressor encoding the aversive cue period with duration corresponding to 4 s and intensity set to one; (2) a linear regressor encoding the neutral cue period with duration corresponding to 4 s and intensity set to one; (3) a linear regressor encoding the aversive tone period with duration corresponding to 4 s and intensity set to one; (4) a linear regressor encoding the neutral tone period with duration corresponding to 4 s and intensity set to one. This model also included RT regressors of no-interest with duration set to the RT for the button press during the cue period and intensity of one. In addition, we also added nuisance regressors for any missed trials occurring in the cue and tone periods with the duration set to 4 s and an intensity of one. For the first-level model, we created the following contrasts: (1) aversive – neutral cue period; (2) aversive – neutral tone period; (3) aversive + neutral cue period; (4) aversive + neutral tone period. Accordingly, the first-level contrasts allowed us to model the aversive and neutral trials separately.
In the second-level analysis, we used a fixed-effects model to either combine the data across the two runs or contrasted the early and late trials. This setup resulted in three second-level contrasts: (1) early + late; (2) early – late; (3) late – early. In effect, the second level contrasts permitted us to model the temporal element of the task.
In the group-level analysis, we added a participant-specific covariate corresponding to their subjective rating difference between the aversive and neutral tones. This covariate was included to account for the subjective differences in tone perception. We performed a mixed-effects ANOVA to test the main effects of time (late – early) and cue type (aversive – neutral) as well as the interaction between these two factors.
Uncontrollable and Controllable Phases
For the uncontrollable and controllable phases, we wanted to examine differential neural responses toward uncontrollable and controllable cues in the uncontrollable and controllable phases respectively. We hypothesized that in the uncontrollable—controllable contrast, participants would recruit amygdala and insula. This hypothesis was grounded on previous work suggesting that the loss or lack of perceived control in an aversive context (e.g., receiving painful stimuli) is associated with increased activity in regions related to negative emotion arousal and the anticipation of aversive events (Salomons et al., 2004; Tanaka et al., 2006; Mohr et al., 2008; Alvarez et al., 2015; Bräscher et al., 2016). In addition, based on our prediction that participants would develop passivity in the uncontrollable phase, previous animal research has suggested that regions such as the dorsal striatum and amygdala might be involved in the neural mechanism subserving passivity (Thierry et al., 1976; Maier et al., 1993; Strong et al., 2011; Clark et al., 2014). On the other hand, in the controllable—uncontrollable contrast, we anticipated that participants would have greater activity in the ventral striatum (i.e., nucleus accumbens) and vmPFC. This prediction was based on our previous finding (Wang and Delgado, 2019) and others (e.g., Maier and Watkins, 2010; Leotti and Delgado, 2011) showing that the nucleus accumbens and vmPFC served as key nodes in the neural circuitry for perceived control. To test these hypotheses, we performed a GLM contrasting the uncontrollable and controllable trials.
For the first-level analysis, we modeled participant-specific design matrices with the following regressors: (1) a linear regressor encoding the cue period with duration corresponding to 4 s and intensity set to one; (2) a linear regressor encoding the tone period with duration corresponding to 4 s and intensity set to one. This model also included RT regressors of no-interest with duration set to the RT for the button press in the cue period and intensity of one as well as nuisance regressors for any missed trials occurring in the cue and tone periods with the duration set to 4 s and an intensity of one.
In the second-level analysis, using a fixed-effects model, we compared the two experimental phases by creating two contrasts: (1) uncontrollable—controllable; (2) controllable—uncontrollable. Finally, in the group-level analysis, we added a participant-specific covariate accounting for their subjective tone rating for the aversive tone. We carried out mixed-effects t-tests to examine differences in neural activation in cue period between the two experimental conditions (i.e., controllable and uncontrollable phases). In addition, we also applied a vmPFC ROI based on our previous finding (Wang and Delgado, 2019) to examine whether vmPFC activity exhibited a significant relationship with participants’ changes in avoidance behavior between the controllable and uncontrollable phases.
Results
Behavioral Results
Prior to the experiment, participants rated the aversiveness of each tone on a Likert scale of 1–7. Participants on average rated the aversive tone (M = 5.55, SD = 1.41) significantly higher than the neutral tone (M = 2.09, SD = 1.02); [t(21) = 9.80, p < 0.0001, Cohen’s d = 2.08].
Aversive vs. Neutral Exposure Trials
In the exposure phase, participants made more AVOID than GIVE-UP presses during both the aversive (AVOID: M = 12.09, SD = 2.94; GIVE-UP: M = 3.64, SD = 3.05) and neutral (AVOID: M = 12.09, SD = 2.79; GIVE-UP: M = 3.64, SD = 2.84) trials, without any difference in AVOID button presses between the two trial types [t(21) = 0.00, p = 1.00]. We ran a 2 × 2 ANOVA to examine the effects of trial type (aversive vs. neutral) and time (early vs. late) on the proportion of AVOID button presses. We did not find a significant interaction between trial type and time [F(1, 66) = 0.19, p = 0.67] or a main effect of trial type [F(1, 66) = 0.02, p = 0.89]. However, there was a significant main effect of time [Figure 2A; F(1, 66) = 6.31, p = 0.014] and post hoc pairwise comparisons of mean revealed a difference between the early and late aversive trials (p = 0.041, Cohen’s d = 2.04) but not the neutral trials (p = 0.15) where participants made more avoidance responses in the early trials.
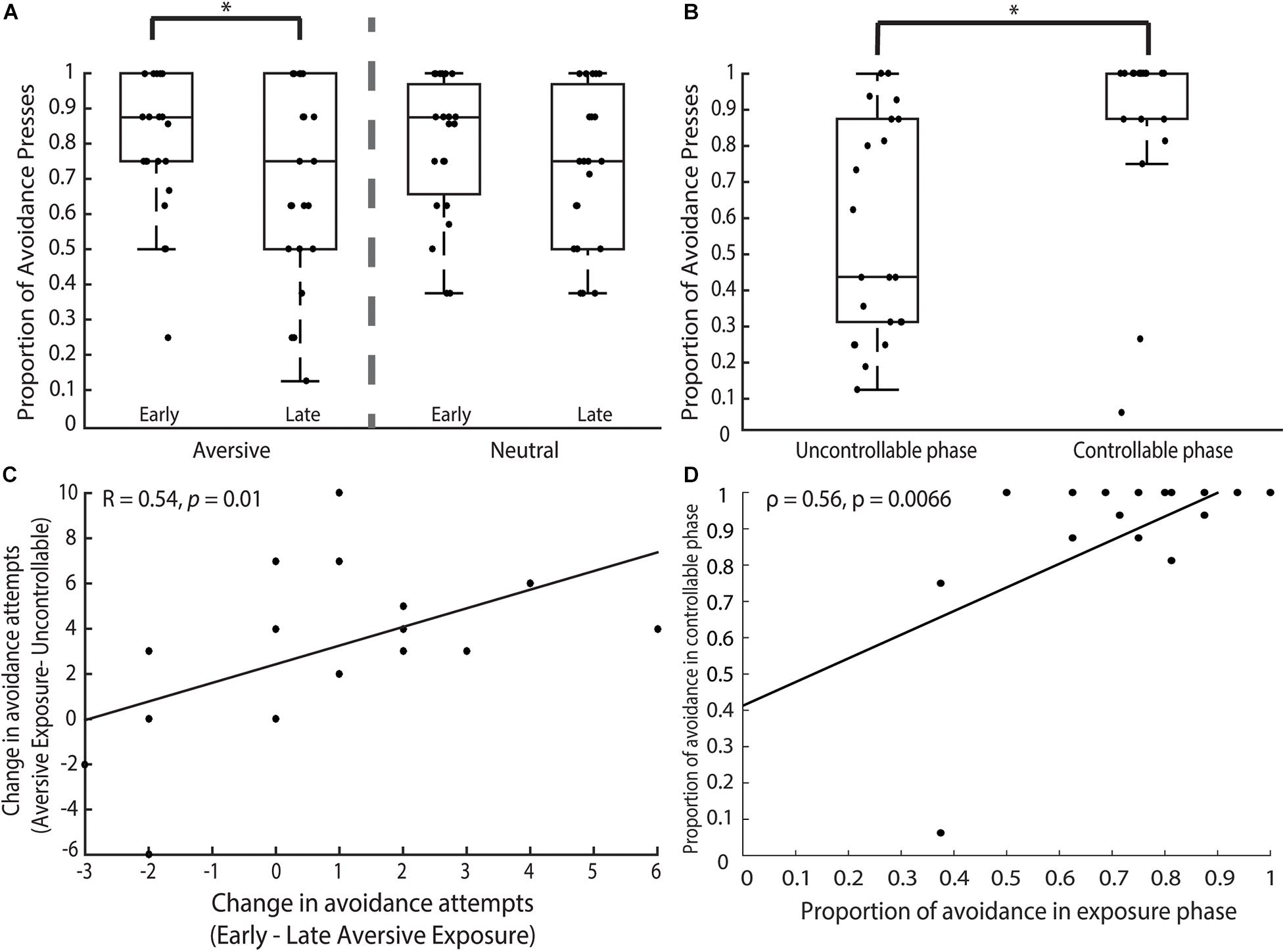
Figure 2. Behavioral findings. (A) Exposure phase. Participants’ avoidance behavior revealed a significant main effect of time. Pairwise comparisons showed that this significant effect was driven by the marked decrease in AVOID button presses unilaterally present in the aversive but not neutral trials. (B) Uncontrollable and controllable phases. Participants significantly increased their avoidance behavior in the controllable phase when compared to the uncontrollable phase. (C) We examined participants’ changes in avoidance behavior between the early and late aversive exposure trials compared to the same changes in behavior across exposure (combined trials) and uncontrollable trials. We found that participants who showed greater decrease in their avoidance behavior from the early to late aversive exposure trials (i.e., larger x-axis) also showed greater decrease in their avoidance behavior from the aversive exposure to uncontrollable trials (i.e., larger y-axis). (D) We investigated the relationship between the proportion of avoidance behavior in the exposure and controllable phases and found that participants who made more avoidance presses in the exposure phase also subsequently made more avoidance presses in the controllable phase. Result remained significant even after removing outllier. *p < 0.05.
Uncontrollable vs. Controllable Phases
In the uncontrollable phase, participants made more AVOID (M = 9.14, SD = 4.75) than GIVE-UP presses (M = 6.59, SD = 4.84) in a novel aversive context. In the controllable phase, the participants similarly made more AVOID (M = 14.64, SD = 3.26) than GIVE-UP presses (M = 1.27, SD = 3.28). Using a non-parametric Mann-Whitney test, we found that participants had a higher proportion of AVOID button presses in the controllable compared to the uncontrollable phase (Figure 2B; z = 4.17, p < 0.0001), alluding to increases in avoidance behavior that was driven by the presence of controllability in an otherwise aversive but novel context. Note that two participants could be classified as outliers based on the criterion of less than 3 SD from mean, but importantly, removing them from analysis does not affect the reported results.
Observations Across Time
Examining the avoidance behavior across the three experimental phases (i.e., early and late exposure, uncontrollable, controllable), we used a Kruskal-Wallis rank test and found a significant effect of time [X 2(3) = 21.2, p = 0.0001]. We conducted post hoc pairwise comparisons using Dunn’s test (Dunn, 1964) and found a significant difference between early exposure and uncontrollable (z = 2.38, p = 0.0086) as well as late exposure and controllable (z = −2.96, p = 0.0016) phases. We also observed significant difference between early exposure and controllable (z = −2.13, p = 0.017) and a marginally significant difference between late exposure and uncontrollable (z = 1.55, p = 0.06) phases.
Looking specifically at the aversive exposure and uncontrollable phases, we found that participants made significantly fewer proportion of AVOID button presses in the uncontrollable compared to the exposure trials (z = −1.99, p = 0.047), suggesting that participants showed the development of passivity marked by less avoidance and more giving-up behavior in response to uncontrollable aversive cues over time. Interestingly, we also found that the total proportion of AVOID button presses during the exposure phase predicted avoidance behavior in the uncontrollable phase, but only for aversive exposure trials (β = 2.43; z = 3.96; p < 0.0001) but not neutral trials (β = 0.79; z = 1.22; p = 0.22). To investigate further, we examined changes in avoidance behavior during aversive trials in the exposure and uncontrollable phases. Strikingly, we found that participants’ change in avoidance attempts from the early to late aversive exposure trials, but not the neutral trials (r = 0.33, p = 0.14), showed a strong positive relationship with their change in avoidance attempts from the aversive exposure to uncontrollable trials (Figure 2C; r = 0.54, p = 0.01), suggesting that those with greater decrease in avoidance behavior between the early and late aversive exposure trials also showed greater decrease in avoidance behavior in the uncontrollable compared to exposure trials. That is, an initial decrease in avoidance predicted increased passivity as the task progressed.
In addition, we investigated the relationship between participants’ avoidance behavior in the exposure and controllable phases. We found that a significant relationship (Figure 2D; ρ = 0.56, p = 0.0066)—that remained significant even after removing the outlier (ρ = 0.48, p = 0.026)—where individuals who made more avoidance behavior in the exposure phase also made more avoidance behavior in the subsequent controllable phase. This suggested a negative relationship between passivity and controllability where individuals who were less passive during the exposure phase were more likely to make avoidance behavior in the controllable phase, supporting the overarching notion that early passive behavior in the experiment was related to less protection from controllability on avoidance motivation.
These results collectively depicted a behavioral pattern where participants showed a significant decrease in avoidance behavior from the early exposure to uncontrollable trials, all of which were uncontrollable but novel aversive contexts. However, participants increased their avoidance behavior in the controllable phase when controllability was present in a novel aversive context and their avoidance behavior was on par with what they showed in the early exposure trials, suggesting that controllability served protective effects to rescue participants’ avoidance behavior.
Neuroimaging Results
Exposure Phase
In the exposure phase, we were interested in neural activation due to a sustained aversive context. In our 2 (aversive vs. neutral) × 2 (early vs. late) ANOVA, we did not find any regions that survived multiple comparisons for the interaction or main effects of trial type and time.
Uncontrollable and Controllable Phases
In our behavioral findings, we showed that participants made significantly more avoidance behaviors in the controllable compared to the uncontrollable phase, suggesting that participants recognized the difference in controllability between the two contexts. We performed a GLM to examine whether there exist differences in neural activation toward the controllable and uncontrollable cues. In the contrast of uncontrollable—controllable, we found neural activation in the amygdala (Figure 3; peak z-stats = 3.4 at MNIx, y, z = 19, −4, −20, pFDR voxel–corrected < 0.05), insula (Figure 3; peak z-stats = 4.0 at MNIx, y, z = 42, 0, 7, pFDR voxel–corrected < 0.05), cingulate cortex (Figure 3; peak z-stats = 4.6 at MNIx, y, z = -12, 12, 44, pFDR voxel–corrected < 0.05) and caudate nucleus (Figure 3; peak z-stats = 5.0 at MNIx, y, z = −10, 11, 7, pFDR voxel–corrected < 0.05). On the other hand, in the contrast of controllable—uncontrollable, we did not find any region that survived correction for multiple comparisons.
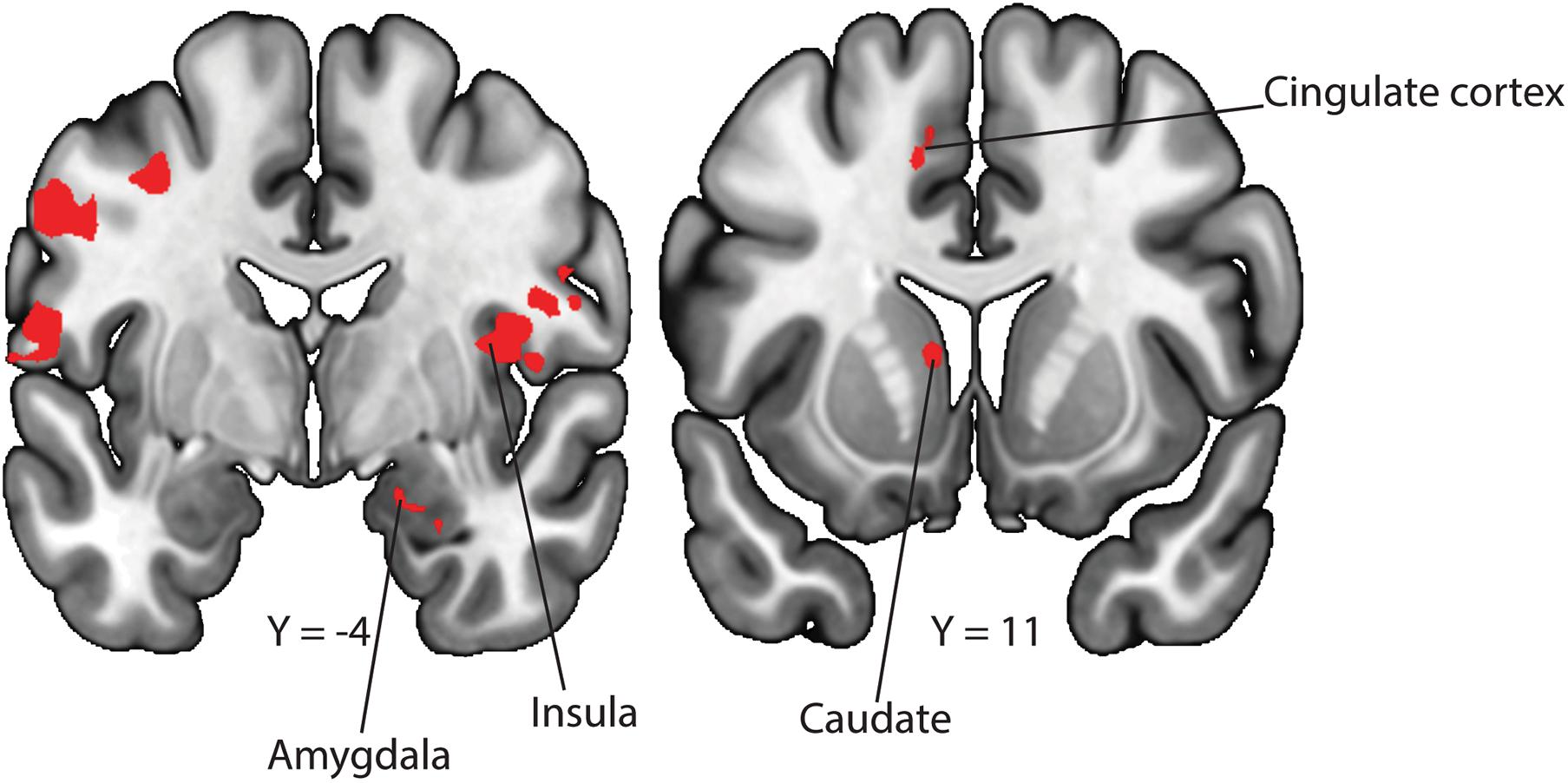
Figure 3. Neural correlates for uncontrollable cues. To examine differences in neural activation between the uncontrollable and controllable phases, we conducted a GLM contrasting the uncontrollable—controllable trials. We observed activity in the amygdala, insula, cingulate cortex and caudate after correcting for multiple comparisons.
As we did not observe significant activation in the contrast of controllable—uncontrollable, we conducted an exploratory follow-up analysis with a specific vmPFC ROI. In a previous study (Wang and Delgado, 2019), we reported that the subjective value of control in the appetitive domain was tracked in the vmPFC. Given that our current experiment investigated how controllability influenced avoidance behavior, we were interested in examining whether vmPFC activity in the controllable context showed any relationship with participants’ avoidance behavior. Specifically, in the contrast between controllable and uncontrollable cues, we used the peak vmPFC coordinate reported by Wang and Delgado (2019; MNIx, y, z = −6, 32, −14) and created a 3 mm region-of-interest functional mask. With this vmPFC mask, we extracted the peak activation (M = 47.07, bootstrap bias-corrected and accelerated 95% confidence interval = [36.80, 61.46]) and correlated this activation with participants’ change in avoidance behavior between the controllable—uncontrollable trials using a spearman’s correlation (Rousselet and Pernet, 2012). We found that participants who had higher vmPFC peak activation to controllable cues also had greater increase in avoidance behavior in the controllable compared to uncontrollable trials (Figure 4; ρ = 0.43, p = 0.04). To investigate whether vmPFC activity in the controllable phase can predict participants’ behavior change, we also performed a robust regression to reveal an association between vmPFC activity and behavioral changes (t = 4.45, p < 0.0001). These findings suggest that participants with stronger vmPFC activation to controllable cues had a correspondingly larger increase in avoidance behavior when presented with a controllable compared to an uncontrollable context.
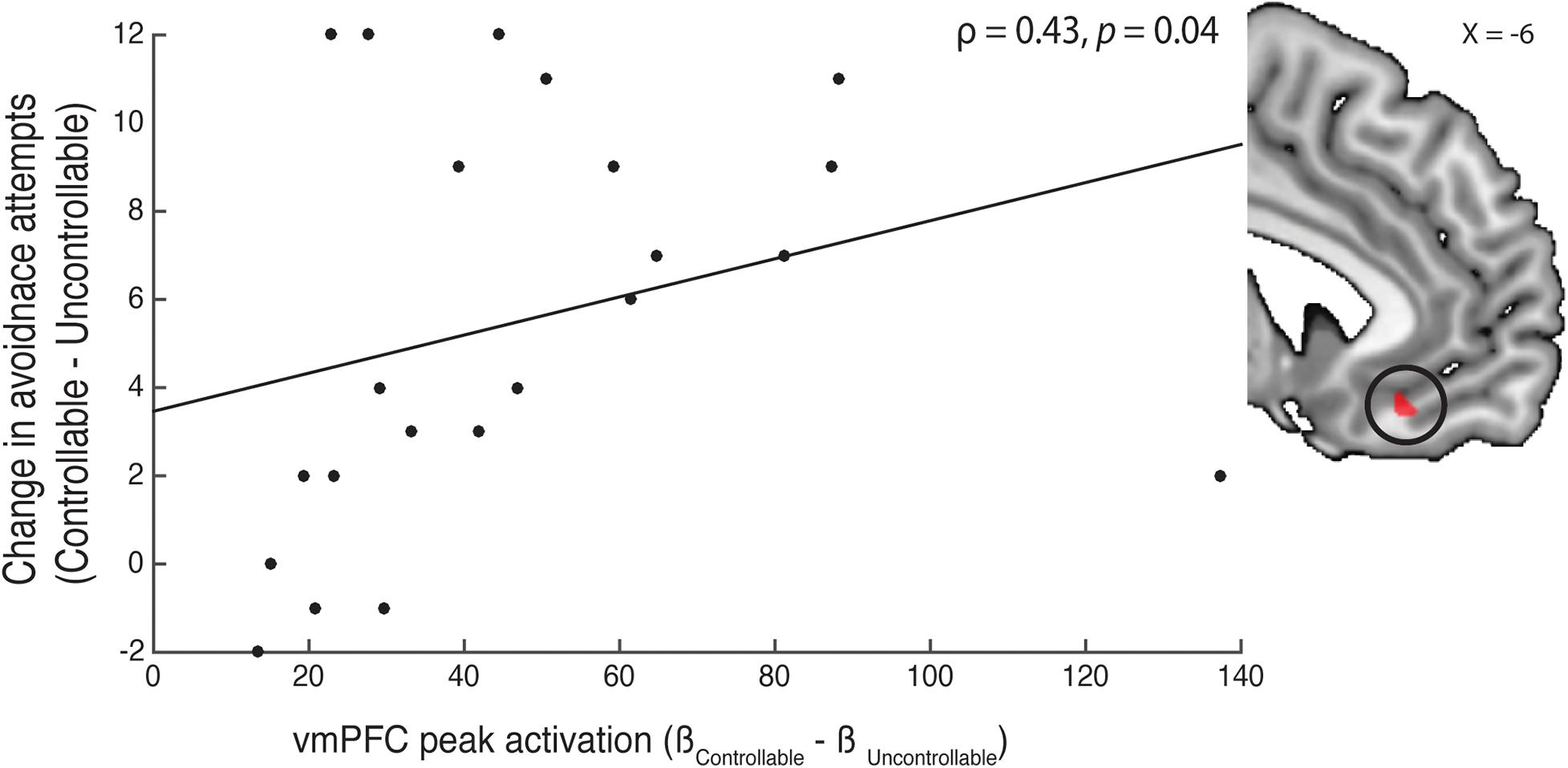
Figure 4. Correlation of avoidance behavior and neural activity. We examined the relationship between vmPFC activity in the controllable—uncontrollable cue contrast and participants’ changes in avoidance behavior between the two phases. Using a functional mask (shown in the right insert) created from the peak coordinate (peak MNIx, y, z = −6, 32, −14) reported in Wang and Delgado (2019), we extracted the peak activation and correlated them with participants’ change in avoidance attempts from the controllable to uncontrollable phase. We found that participants with a larger vmPFC peak activation also had a bigger increase in avoidance behavior in the controllable trials.
Discussion
We investigated the influence of perceived control on rescuing avoidance behavior in aversive contexts and probed the neural basis underlying this behavioral change. We found that after repeated exposure to uncontrollability, participants developed passivity as demonstrated by their decreased avoidance behavior toward a novel aversive cue that was subserved by neural activation in the insula, amygdala and caudate nucleus. However, the presence of control in a subsequent novel aversive context was able to rescue participants’ avoidance behavior and reverse passivity. Importantly, greater change in avoidance behavior in the controllable context was related to stronger activity in the vmPFC.
In the exposure phase, we specifically tested for behavioral and neural differences in response to aversive and neutral cues that were both uncontrollable. While participants rated the aversive tone as significantly more aversive compared to the neutral tone, we did not correspondingly find a significant behavioral or neural main effect of cue aversiveness. However, we did find a behavioral main effect of time (early vs. late) that was driven by reduced avoidance behavior in the late compared to early aversive exposure trials. Given that our experimental design deviates from prior work using aversive tones (e.g., Büchel et al., 1998), we reason that the uncontrollability nature of the exposure trials rendered both cues (associated with either aversive or neutral tones) to be perceived as aversive. Indeed, previous research have reported that uncontrollability was regarded as both aversive and undesirable (Rodin, 1986; Grillon et al., 2008; Kim et al., 2017) and served in and of itself as an aversive stimulus to trigger cortisol release (Weiner, 1992; Peters et al., 1998). As such, in our CAD task, participants might have regarded both types of cues as equally aversive over time due to their inherent uncontrollability. A potential way to start probing this presumption would have been to ask participants to subjectively rate the aversiveness of the two cues at the conclusion of each exposure experimental run. Their subjective ratings would have offered some insights into participants’ subjective perception of the two types of cues.
During the exposure phase, we reported a significant decrease in avoidance behavior that was unilaterally present in the aversive but not the neutral trials. Coupled to this finding was the observation that participants made significantly fewer avoidance attempts in the ensuing uncontrollable compared to the exposure aversive trials. Together, these results suggest that participants’ avoidance behavior reduced over time as they endured persistent uncontrollability across different aversive contexts. Importantly, this reduction in avoidance behavior was observed in participants’ responses to novel cues (in the uncontrollable phase), suggesting that they were in some state of passivity where they showed reduced avoidance motivation and behavior (Maier and Seligman, 2016), in line with previous studies examining learned helplessness in both rodents (Seligman et al., 1975; Anisman and Merali, 2001), dogs (Seligman et al., 1979) and humans (Hiroto, 1974; Hiroto and Seligman, 1975).
Our current experimental design allowed us to show that participants’ behavioral change from the early to late aversive exposure trials significantly predicted their subsequent behavioral changes from the aversive exposure to uncontrollable phase. Notably, only the behavioral change in aversive, rather than the neutral exposure trials, predicted participants’ ensuing behavioral change in the uncontrollable phase. This finding suggests that participants’ behavioral responses toward aversive cues in the late stages of experiencing uncontrollability mimicked their behavior in the early stages where those who gave up quicker also gave up more over time. That is, those whose avoidance motivation sagged early on in the face of uncontrollable aversiveness were also those whose avoidance motivation showed greater decline in latter stages, potentially hinting at the importance of understanding avoidance motivation in the initial/early stages of experiencing an uncontrollable aversive context. Future studies should aim to replicate and extend our current findings to investigate possible explanations for this observation. We hypothesize that this finding could be partially reconciled if participants who gave up quicker were also less persistent in general, hence alluding to a potential interaction of susceptibility to passivity and the behavioral trait of persistence (Cloninger et al., 1998; Duckworth et al., 2007; Bhanji and Delgado, 2014; Lucas et al., 2015).
In addition to the aforementioned behavioral findings, we also observed that the uncontrollable phase elicited activation in neural regions such as the insula, amygdala, cingulate cortex and caudate nucleus. This is in line with prior work showing that uncontrollability is undesirable and induces negative emotions (Chorpita and Barlow, 1998; Robbins, 2005; Sanjuán and Magallares, 2009; Pryce et al., 2012). Indeed, uncontrollability can be aversive in and of itself to drive activity in neural regions subserving aversive processing (Hayes et al., 2014). Moreover, the amygdala and caudate nucleus observations are consistent with prior animal studies reporting that these regions contribute to the neural circuitry underlying the behavioral consequences of uncontrollable aversive stimulus, particularly the role of serotonergic activity within these regions in mediating the learning deficits induced by uncontrollability (Amat et al., 1998; Strong et al., 2011). Furthermore, our current findings also support the notion that these regions serve important roles in avoidance learning (Delgado et al., 2009; Choi et al., 2010; Palminteri et al., 2012; Lewis et al., 2013; Atlas et al., 2016).
Across the course of the experiment, participants showed progressively fewer avoidance behaviors, reaching the lowest proportion of avoidance behavior in the uncontrollable phase before significantly rebounding in the controllable phase. This suggests that presence of control was potent enough to reverse passivity and rescue avoidance behavior even after exposure to persistent uncontrollability in an aversive domain. However, we note that there was one participant whose behavior did not rebound in the controllable phase. Instead, this participant exhibited the classic learned helplessness behavior where they demonstrated passivity even when control was present. Although this participant was a behavioral outlier in our current study, their behavior points to the individual differences that exist where presence of control does not exert the same protective effects for everyone across the board. This is an interesting question for a future study with a larger sample to probe personality traits and behavioral tendencies that could make an individual more resistant to the protective effects of control.
In the controllable phase, participants who rebounded the most in terms of avoidance behavior were the ones who responded the strongest to the protective effects of controllability. We inferred that these individuals have a stronger desire for control and would accordingly tend to seek out control in the environment, perhaps driven by a stronger coping and resilient tendency (Maier and Watkins, 2010). However, we did not, as hypothesized, observe significant activation in the ventral striatum and vmPFC when in the contrast of controllable—uncontrollable. As we did not observe significant activation, in our follow-up exploratory analysis with a functional vmPFC ROI from a previous study (Wang and Delgado, 2019), we considered differences in participants’ avoidance between the uncontrollable and controllable phases. Specifically in the controllable phase, we found that vmPFC activity, which was shown in our previous work to encode participants’ subjective value of control (Wang and Delgado, 2019), positively predicted participants’ avoidance behavioral change in the controllable phase compared to the uncontrollable phase. This finding, which would benefit from replication using a larger sample size, supports the hypothesis that a neural region (i.e., vmPFC) associated with encoding perceived control could predict participants’ changes in avoidance behavior when control is present. However, this does not preclude the possibility that, specifically within an aversive domain, perceived control could recruit specific subregions within the vmPFC or cortical areas such as the ACC—a hypothesis that will benefit from future work. In addition, future studies could also consider the possibility that perceived control could alter the functional connectivity between regions that might respond to the opportunity to exert control in an aversive domain.
Regardless, inferring from our current finding, we reason that how much individuals subjectively value control could help to predict the protective effect of control on their avoidance motivation, an effect that could persist—pending future investigations—even if the environment becomes uncontrollable again. Based on prior work on learned helplessness (Maier and Seligman, 2016), it is possible that successful avoidance in the controllable phase would increase the amount of persistence to avoid in a subsequent uncontrollable run where individuals who performed more avoidance behavior in the controllable run would be more protected in subsequent uncontrollable environments. Appreciating the protective effect of perceived control could have implications on understanding an individual’s vulnerability toward developing psychopathologies associated with control deficits such as depression (Li et al., 2011; Cheng et al., 2013; Vollmayr and Gass, 2013; Romaniuk et al., 2019) and treatment strategies that aim to enhance subjective perception of control (Kadden and Litt, 2011). In conclusion, we found that an enhanced perception of control influences behavioral passivity and encourages avoidance behavior in an aversive context, a potentially protective effect that is subserved by activity in the vmPFC.
Data Availability Statement
The raw data supporting the conclusions of this article will be made available by the authors, without undue reservation.
Ethics Statement
The studies involving human participants were reviewed and approved by the Rutgers University Institutional Review Board. The patients/participants provided their written informed consent to participate in this study.
Author Contributions
KW and MD contributed to conception and design of the study. KW collected the data, performed the statistical analysis, and wrote the first draft of the manuscript. Both authors contributed to manuscript revision, read, and approved the submitted version.
Funding
This work was supported by funding from the National Institutes of Health (DA027764) and the McKnight Foundation.
Conflict of Interest
The authors declare that the research was conducted in the absence of any commercial or financial relationships that could be construed as a potential conflict of interest.
Footnotes
- ^ http://www.fil.ion.ucl.ac.uk/spm/software/spm12
- ^ http://www.fmrib.ox.ac.uk/fsl
- ^ https://www.mccauslandcenter.sc.edu/crnl/tools
References
Alvarez, R., Kirlic, N., Misaki, M., Bodurka, J., Rhudy, J., Paulus, M., et al. (2015). Increased anterior insula activity in anxious individuals is linked to diminished perceived control. Transl. Psychiatry 5:e591. doi: 10.1038/tp.2015.84
Amat, J., Baratta, M. V., Paul, E., Bland, S. T., Watkins, L. R., and Maier, S. F. (2005). Medial prefrontal cortex determines how stressor controllability affects behavior and dorsal raphe nucleus. Nat. Neurosci. 8, 365–371. doi: 10.1038/nn1399
Amat, J., Matus-Amat, P., Watkins, L. R., and Maier, S. F. (1998). Escapable and inescapable stress differentially alter extracellular levels of 5-HT in the basolateral amygdala of the rat. Brain Res. 812, 113–120. doi: 10.1016/s0006-8993(98)00960-3
Andersson, J. L., Hutton, C., Ashburner, J., Turner, R., and Friston, K. (2001). Modeling geometric deformations in EPI time series. Neuroimage 13, 903–919. doi: 10.1006/nimg.2001.0746
Anisman, H., and Merali, Z. (2001). Rodent models of depression: learned helplessness induced in mice. Curr. Prot. Neurosci. Chapter 8, Unit 8.10C.
Ardekani, B. A., and Bachman, A. H. (2009). Model-based automatic detection of the anterior and posterior commissures on MRI scans. Neuroimage 46, 677–682. doi: 10.1016/j.neuroimage.2009.02.030
Ashburner, J., and Friston, K. J. (2005). Unified segmentation. Neuroimage 26, 839–851. doi: 10.1016/j.neuroimage.2005.02.018
Atlas, L. Y., Doll, B. B., Li, J., Daw, N. D., and Phelps, E. A. (2016). Instructed knowledge shapes feedback-driven aversive learning in striatum and orbitofrontal cortex, but not the amygdala. Elife 5:e15192.
Bhanji, J. P., and Delgado, M. R. (2014). Perceived control influences neural responses to setbacks and promotes persistence. Neuron 83, 1369–1375. doi: 10.1016/j.neuron.2014.08.012
Bhanji, J. P., Kim, E. S., and Delgado, M. R. (2016). Perceived control alters the effect of acute stress on persistence. J. Exp. Psychol. 145:356. doi: 10.1037/xge0000137
Bongard, S. (1995). Mental effort during active and passive coping: a dual−task analysis. Psychophysiology 32, 242–248. doi: 10.1111/j.1469-8986.1995.tb02960.x
Bräscher, A.-K., Becker, S., Hoeppli, M.-E., and Schweinhardt, P. (2016). Different brain circuitries mediating controllable and uncontrollable pain. J. Neurosci. 36, 5013–5025. doi: 10.1523/jneurosci.1954-15.2016
Büchel, C., Morris, J., Dolan, R. J., and Friston, K. J. (1998). Brain systems mediating aversive conditioning: an event-related fMRI study. Neuron 20, 947–957. doi: 10.1016/s0896-6273(00)80476-6
Carp, J. (2013). Optimizing the order of operations for movement scrubbing: comment on power et al. Neuroimage 76, 436–438. doi: 10.1016/j.neuroimage.2011.12.061
Cheng, C., Cheung, S. F., Chio, J. H.-M., and Chan, M.-P. S. (2013). Cultural meaning of perceived control: a meta-analysis of locus of control and psychological symptoms across 18 cultural regions. Psychol. Bull. 139:152. doi: 10.1037/a0028596
Choi, J.-S., Cain, C. K., and Ledoux, J. E. (2010). The role of amygdala nuclei in the expression of auditory signaled two-way active avoidance in rats. Learn. Mem. 17, 139–147. doi: 10.1101/lm.1676610
Chorpita, B. F., and Barlow, D. H. (1998). The development of anxiety: the role of control in the early environment. Psychol. Bull. 124:3. doi: 10.1037/0033-2909.124.1.3
Christianson, J. P., Thompson, B. M., Watkins, L. R., and Maier, S. F. (2009). Medial prefrontal cortical activation modulates the impact of controllable and uncontrollable stressor exposure on a social exploration test of anxiety in the rat. Stress 12, 445–450. doi: 10.1080/10253890802510302
Clark, P. J., Ghasem, P. R., Mika, A., Day, H. E., Herrera, J. J., Greenwood, B. N., et al. (2014). Wheel running alters patterns of uncontrollable stress-induced cfos mRNA expression in rat dorsal striatum direct and indirect pathways: a possible role for plasticity in adenosine receptors. Behav. Brain Res. 272, 252–263. doi: 10.1016/j.bbr.2014.07.006
Cloninger, C., Svrakic, D., and Przybeck, T. (1998). A psychobiological model of temperament and character. Dev. Psychiatry Complex. 50, 975–990. doi: 10.1001/archpsyc.1993.01820240059008
Deci, E. L., and Ryan, R. M. (1987). The support of autonomy and the control of behavior. J. Pers. Soc. Psychol. 53:1024. doi: 10.1037/0022-3514.53.6.1024
Delgado, M. R., Jou, R. L., Ledoux, J. E., and Phelps, E. A. (2009). Avoiding negative outcomes: tracking the mechanisms of avoidance learning in humans during fear conditioning. Front. Behav. Neurosci. 3:33. doi: 10.3389/neuro.08.033.2009
Duckworth, A. L., Peterson, C., Matthews, M. D., and Kelly, D. R. (2007). Grit: perseverance and passion for long-term goals. J. Pers. Soc. Psychol. 92:1087. doi: 10.1037/0022-3514.92.6.1087
Dunn, O. J. (1964). Multiple comparisons using rank sums. Technometrics 6, 241–252. doi: 10.1080/00401706.1964.10490181
Faul, F., Erdfelder, E., Lang, A.-G., and Buchner, A. (2007). G∗ Power 3: a flexible statistical power analysis program for the social, behavioral, and biomedical sciences. Behav. Res. Methods 39, 175–191. doi: 10.3758/bf03193146
Feather, N., and Volkmer, R. (1988). Preference for situations involving effort, time pressure, and feedback in relation to Type A behavior, locus of control, and test anxiety. J. Pers. Soc. Psychol. 55:266. doi: 10.1037/0022-3514.55.2.266
Grillon, C., Lissek, S., Rabin, S., Mcdowell, D., Dvir, S., and Pine, D. S. (2008). Increased anxiety during anticipation of unpredictable but not predictable aversive stimuli as a psychophysiologic marker of panic disorder. Am. J. Psychiatry 165, 898–904. doi: 10.1176/appi.ajp.2007.07101581
Hayes, D. J., Duncan, N. W., Xu, J., and Northoff, G. (2014). A comparison of neural responses to appetitive and aversive stimuli in humans and other mammals. Neurosci. Biobehav. Rev. 45, 350–368. doi: 10.1016/j.neubiorev.2014.06.018
Hiroto, D. S. (1974). Locus of control and learned helplessness. J. Exp. Psychol. 102:187. doi: 10.1037/h0035910
Hiroto, D. S., and Seligman, M. E. (1975). Generality of learned helplessness in man. J. Pers. Soc. Psychol. 31:311. doi: 10.1037/h0076270
Holmes, D. S., and Jackson, T. H. (1975). Influence of locus of control on interpersonal attraction and affective reactions in situations involving reward and punishment. J. Pers. Soc. Psychol. 31:132. doi: 10.1037/h0076242
Hutton, C., Bork, A., Josephs, O., Deichmann, R., Ashburner, J., and Turner, R. (2002). Image distortion correction in fMRI: a quantitative evaluation. Neuroimage 16, 217–240. doi: 10.1006/nimg.2001.1054
Kadden, R. M., and Litt, M. D. (2011). The role of self-efficacy in the treatment of substance use disorders. Addict. Behav. 36, 1120–1126. doi: 10.1016/j.addbeh.2011.07.032
Kienast, T., Hariri, A. R., Schlagenhauf, F., Wrase, J., Sterzer, P., Buchholz, H. G., et al. (2008). Dopamine in amygdala gates limbic processing of aversive stimuli in humans. Nat. Neurosci. 11:1381. doi: 10.1038/nn.2222
Kim, J. Y., Yang, S. H., Kwon, J., Lee, H. W., and Kim, H. (2017). Mice subjected to uncontrollable electric shocks show depression-like behaviors irrespective of their state of helplessness. Behav. Brain Res. 322, 138–144. doi: 10.1016/j.bbr.2017.01.008
Leotti, L. A., and Delgado, M. R. (2011). The inherent reward of choice. Psychol. Sci. 22, 1310–1318. doi: 10.1177/0956797611417005
Leotti, L. A., and Delgado, M. R. (2014). The value of exercising control over monetary gains and losses. Psychol. Sci. 25, 596–604. doi: 10.1177/0956797613514589
Lewis, A. H., Niznikiewicz, M. A., Delamater, A. R., and Delgado, M. R. (2013). Avoidance-based human Pavlovian-to-instrumental transfer. Eur. J. Neurosci. 38, 3740–3748. doi: 10.1111/ejn.12377
Li, B., Piriz, J., Mirrione, M., Chung, C., Proulx, C. D., Schulz, D., et al. (2011). Synaptic potentiation onto habenula neurons in the learned helplessness model of depression. Nature 470:535. doi: 10.1038/nature09742
Lieberman, M. D., and Cunningham, W. A. (2009). Type I and Type II error concerns in fMRI research: re-balancing the scale. Soc. Cogn. Affect. Neurosci. 4, 423–428. doi: 10.1093/scan/nsp052
Lucas, G. M., Gratch, J., Cheng, L., and Marsella, S. (2015). When the going gets tough: grit predicts costly perseverance. J. Res. Pers. 59, 15–22. doi: 10.1016/j.jrp.2015.08.004
Ly, V., Wang, K. S., Bhanji, J., and Delgado, M. R. (2019). A reward-based framework of perceived control. Front. Neurosci. 13:65. doi: 10.3389/fnins.2019.00065
Maier, S. F., Grahn, R. E., Kalman, B. A., Sutton, L. C., Wiertelak, E. P., and Watkins, L. R. (1993). The role of the amygdala and dorsal raphe nucleus in mediating the behavioral consequences of inescapable shock. Behav. Neurosci. 107:377. doi: 10.1037/0735-7044.107.2.377
Maier, S. F., and Seligman, M. E. (1976). Learned helplessness: theory and evidence. J. Exp. Psychol. 105:3.
Maier, S. F., and Seligman, M. E. (2016). Learned helplessness at fifty: Insights from neuroscience. Psychol. Rev. 123, 349. doi: 10.1037/rev0000033
Maier, S. F., and Watkins, L. R. (2010). Role of the medial prefrontal cortex in coping and resilience. Brain Res. 1355, 52–60. doi: 10.1016/j.brainres.2010.08.039
Mohr, C., Leyendecker, S., and Helmchen, C. (2008). Dissociable neural activity to self-vs. externally administered thermal hyperalgesia: a parametric fMRI study. Eur. J. Neurosci. 27, 739–749. doi: 10.1111/j.1460-9568.2008.06036.x
Palminteri, S., Justo, D., Jauffret, C., Pavlicek, B., Dauta, A., Delmaire, C., et al. (2012). Critical roles for anterior insula and dorsal striatum in punishment-based avoidance learning. Neuron 76, 998–1009. doi: 10.1016/j.neuron.2012.10.017
Peters, M. L., Godaert, G. L., Ballieux, R. E., Van Vliet, M., Willemsen, J. J., Sweep, F. C., et al. (1998). Cardiovascular and endocrine responses to experimental stress: effects of mental effort and controllability. Psychoneuroendocrinology 23, 1–17. doi: 10.1016/s0306-4530(97)00082-6
Power, J. D., Schlaggar, B. L., and Petersen, S. E. (2015). Recent progress and outstanding issues in motion correction in resting state fMRI. Neuroimage 105, 536–551. doi: 10.1016/j.neuroimage.2014.10.044
Pryce, C. R., Azzinnari, D., Sigrist, H., Gschwind, T., Lesch, K.-P., and Seifritz, E. (2012). Establishing a learned-helplessness effect paradigm in C57BL/6 mice: behavioural evidence for emotional, motivational and cognitive effects of aversive uncontrollability per se. Neuropharmacology 62, 358–372. doi: 10.1016/j.neuropharm.2011.08.012
Quaglieri, P. L. (1980). Locus of control and perceived utility of feedback. Psychol. Rep. 46, 859–862. doi: 10.2466/pr0.1980.46.3.859
Robbins, T. W. (2005). Controlling stress: how the brain protects itself from depression. Nat. Neurosci. 8:261. doi: 10.1038/nn0305-261
Rodin, J. (1986). Aging and health: effects of the sense of control. Science 233, 1271–1276. doi: 10.1126/science.3749877
Rodin, J., and Langer, E. J. (1977). Long-term effects of a control-relevant intervention with the institutionalized aged. J. Pers. Soc. Psychol. 35:897. doi: 10.1037/0022-3514.35.12.897
Romaniuk, L., Sandu, A.-L., Waiter, G. D., Mcneil, C. J., Xueyi, S., Harris, M. A., et al. (2019). The neurobiology of personal control during reward learning and its relationship to mood. Biol. Psychiatry 4, 190–199. doi: 10.1016/j.bpsc.2018.09.015
Rorden, C., Karnath, H.-O., and Bonilha, L. (2007). Improving lesion-symptom mapping. J. Cogn. Neurosci. 19, 1081–1088. doi: 10.1162/jocn.2007.19.7.1081
Rousselet, G. A., and Pernet, C. R. (2012). Improving standards in brain-behavior correlation analyses. Front. Hum. Neurosci. 6:119.
Ryan, R. M., and Deci, E. L. (2000). Self-determination theory and the facilitation of intrinsic motivation, social development, and well-being. Am. Psychol. 55:68. doi: 10.1037/0003-066x.55.1.68
Salomons, T. V., Johnstone, T., Backonja, M.-M., and Davidson, R. J. (2004). Perceived controllability modulates the neural response to pain. J. Neurosci. 24, 7199–7203. doi: 10.1523/jneurosci.1315-04.2004
Sanjuán, P., and Magallares, A. (2009). A longitudinal study of the negative explanatory style and attributions of uncontrollability as predictors of depressive symptoms. Pers. Individ. Differ. 46, 714–718. doi: 10.1016/j.paid.2009.01.030
Satterthwaite, T. D., Elliott, M. A., Gerraty, R. T., Ruparel, K., Loughead, J., Calkins, M. E., et al. (2013). An improved framework for confound regression and filtering for control of motion artifact in the preprocessing of resting-state functional connectivity data. Neuroimage 64, 240–256. doi: 10.1016/j.neuroimage.2012.08.052
Schoenbaum, G., and Setlow, B. (2003). Lesions of nucleus accumbens disrupt learning about aversive outcomes. J. Neurosci. 23, 9833–9841. doi: 10.1523/jneurosci.23-30-09833.2003
Seligman, M. E., Maier, S. F., and Geer, J. (1979). “Alleviation of learned helplessness in the dog,” in Origins of Madness, ed J. D. Keehn (Elmsford, NY: Elsevier), 401–409. doi: 10.1016/b978-0-08-023725-1.50049-5
Seligman, M. E., Rosellini, R. A., and Kozak, M. J. (1975). Learned helplessness in the rat: time course, immunization, and reversibility. J. Compar. Physiol. Psychol. 88:542. doi: 10.1037/h0076431
Smith, S. M. (2002). Fast robust automated brain extraction. Hum. Brain Mapp. 17, 143–155. doi: 10.1002/hbm.10062
Smith, S. M., and Brady, J. M. (1997). SUSAN—A new approach to low level image processing. Int. J. Comput. Vis. 23, 45–78.
Smith, S. M., Jenkinson, M., Woolrich, M. W., Beckmann, C. F., Behrens, T. E., Johansen-Berg, H., et al. (2004). Advances in functional and structural MR image analysis and implementation as FSL. Neuroimage 23, S208–S219.
Strong, P. V., Christianson, J. P., Loughridge, A. B., Amat, J., Maier, S. F., Fleshner, M., et al. (2011). 5-hydroxytryptamine 2C receptors in the dorsal striatum mediate stress-induced interference with negatively reinforced instrumental escape behavior. Neuroscience 197, 132–144. doi: 10.1016/j.neuroscience.2011.09.041
Tanaka, S. C., Samejima, K., Okada, G., Ueda, K., Okamoto, Y., Yamawaki, S., et al. (2006). Brain mechanism of reward prediction under predictable and unpredictable environmental dynamics. Neural Netw. 19, 1233–1241. doi: 10.1016/j.neunet.2006.05.039
Taub, S. I., and Dollinger, S. J. (1975). Reward and purpose as incentives for children differing in locus of control expectancies. J. Pers. 43, 179–195. doi: 10.1111/j.1467-6494.1975.tb00701.x
Thierry, A., Tassin, J., Blanc, G., and Glowinski, J. (1976). Selective activation of the mesocortical DA system by stress. Nature 263, 242. doi: 10.1038/263242a0
Trusty, M. L., and Macan, T. H. (1995). Personal control: effects of reward contingency and locus of control. J. Soc. Behav. Pers. 10:201.
Vollmayr, B., and Gass, P. (2013). Learned helplessness: unique features and translational value of a cognitive depression model. Cell Tissue Res. 354, 175–178. doi: 10.1007/s00441-013-1654-2
Wallston, K. A., Wallston, B. S., Smith, S., and Dobbins, C. J. (1987). Perceived control and health. Curr. Psychol. 6, 5–25.
Wang, K. S., and Delgado, M. R. (2019). Corticostriatal circuits encode the subjective value of perceived control. Cereb. Cortex 29, 5049–5060. doi: 10.1093/cercor/bhz045
Wang, K. S., Kashyap, M., and Delgado, M. R. (2020). The influence of contextual factors on the subjective value of control. Emotion. In press.
Weiner, B. (1992). Attributional Theories of Human Motivation. Human Motivation: Metaphors, Theories, and Research. Newbury Park, CA: Sage.
Weissenbacher, A., Kasess, C., Gerstl, F., Lanzenberger, R., Moser, E., and Windischberger, C. (2009). Correlations and anticorrelations in resting-state functional connectivity MRI: a quantitative comparison of preprocessing strategies. Neuroimage 47, 1408–1416. doi: 10.1016/j.neuroimage.2009.05.005
Woolrich, M. W., Behrens, T. E., Beckmann, C. F., Jenkinson, M., and Smith, S. M. J. N. (2004). Multilevel linear modelling for FMRI group analysis using Bayesian inference. Neuroimage 21, 1732–1747. doi: 10.1016/j.neuroimage.2003.12.023
Keywords: perceived control, ventromedial prefrontal cortex, learned helplessness, passivity, avoidance behavior
Citation: Wang KS and Delgado MR (2021) The Protective Effects of Perceived Control During Repeated Exposure to Aversive Stimuli. Front. Neurosci. 15:625816. doi: 10.3389/fnins.2021.625816
Received: 04 November 2020; Accepted: 11 January 2021;
Published: 03 February 2021.
Edited by:
Rutsuko Ito, University of Toronto, CanadaReviewed by:
Michael V. Baratta, University of Colorado Boulder, United StatesMasataka Watanabe, Tokyo Metropolitan Institute of Medical Science, Japan
Copyright © 2021 Wang and Delgado. This is an open-access article distributed under the terms of the Creative Commons Attribution License (CC BY). The use, distribution or reproduction in other forums is permitted, provided the original author(s) and the copyright owner(s) are credited and that the original publication in this journal is cited, in accordance with accepted academic practice. No use, distribution or reproduction is permitted which does not comply with these terms.
*Correspondence: Kainan S. Wang, a3dhbmcyM0BtY2xlYW4uaGFydmFyZC5lZHU=; Mauricio R. Delgado, ZGVsZ2Fkb0Bwc3ljaG9sb2d5LnJ1dGdlcnMuZWR1