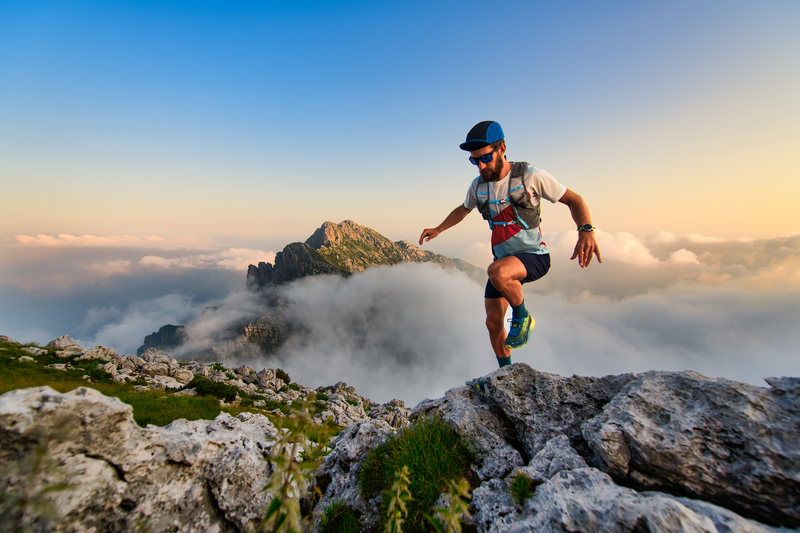
94% of researchers rate our articles as excellent or good
Learn more about the work of our research integrity team to safeguard the quality of each article we publish.
Find out more
ORIGINAL RESEARCH article
Front. Neurosci. , 11 January 2021
Sec. Decision Neuroscience
Volume 14 - 2020 | https://doi.org/10.3389/fnins.2020.607912
Openness to experience (OTE) has relatively stable and heritable characteristics. Previous studies have used candidate gene approaches to explore the genetic mechanisms of OTE, but genome-wide polygenic scores have a greater genetic effect than other genetic analysis methods, and previous studies have never examined the potential effect of OTE on this cumulative effect at the level of the brain mechanism. In the present study, we aim to explore the associations between polygenic scores (PGSs) of OTE and brain structure and functions. First, the results of PGSs of OTE at seven different thresholds were calculated in a large Chinese sample (N = 586). Then, we determined the associations between PGSs of OTE and cortical thickness and functional connectivity. The results showed that PGSs of OTE was negatively correlated with the thickness of the fusiform gyrus, and PGSs of OTE were negatively associated with the functional connectivity between the left intraparietal sulcus (IPS) and the right posterior occipital lobe. These findings may suggest that the brain structure of fusiform gyrus and brain functions of IPS and posterior occipital lobe are partly regulated by OTE-related genetic factors.
Openness to experience (OTE) is a dimension derived from personality that reflects individual differences in cognitive exploration of reasoning and perception (DeYoung, 2014). Previous studies have usually regarded OTE as an element of the Big Five Personality Model (McCrae, 1993). Individuals with high OTE generally show a greater curiosity for new things, a desire to explore, a sense of satisfaction derived from new knowledge and experiences, and a high tolerance for strange situations (McCrae and Costa, 1985). OTE is primarily related to individual intelligence and cognitive style, such as imagination and creative cognition (DeYoung et al., 2008; DeYoung and Allen, 2019). Artists and intellectuals scored higher on OTE in previous study (DeYoung, 2014). Some studies demonstrated that differences in OTE and intelligence significantly predict creative achievements in art and science (Kaufman et al., 2016). Moreover, OTE has been showed to be associated with positive coping strategies, such as seeking social support (Pereira-Morales et al., 2018). Thus, OTE is demonstrably conducive not only to the promotion of art and creativity but also to health-related quality of life.
OTE has been reported to be a heritable personality trait. A meta-analysis of 100,000 pairs of twins and families showed that 41% of participants had higher levels of OTE than in other dimensions of personality (Vukasović and Bratko, 2015). Candidate gene studies have shown that OTE is associated with genes such as 5-hydroxy tryptamine transporter (5-HTT), the dopamine D4 receptor gene (DRD4) and the catechol-O-methyltransferase gene (COMT) (Kalbitzer et al., 2009; DeYoung et al., 2011). With the development of gene sequencing and analysis methods, genome-wide association studies (GWASs) and genome-wide polygenic scores (GPSs) have been used to explore the relationship between genes and phenotypes (Dudbridge, 2013). GWASs are based on a larger sample size, and, as the sample size increases, GWASs can detect more risk-related loci (International Schizophrenia Consortium, 2009). The GWAS for OTE conducted by the Genetics of Personality Consortium (GPC) identified 2 genetic loci achieving genome-wide statistical significance (De Moor et al., 2012). However, the effect size of phenotypic variation explained by SNPs obtained by GWAS is very small (Visscher et al., 2012). The locus can only explain 0.22% of the variation for OTE, with odds ratios generally between 0.92 and 1 (De Moor et al., 2012). GPSs have allowed us to use large GWAS power in small-sample applications (Dima and Breen, 2015). GPSs can be used to estimate the cumulative effects of SNPs associated with certain phenotypes in each individual, thereby exploring the genetic basis that may affect the individual phenotype (Plomin and von Stumm, 2018). GPSs of OTE have been reported to be correlated with symptoms of diseases (Amare et al., 2018) and cognitive traits (Abdellaoui et al., 2018). For example, researchers using a polygenic score of personality traits (including OTE) in depressive patients found a genetic association between the five personality traits and the outcomes of serotonin reuptake inhibitors (SSRIs) (Amare et al., 2018). GPSs of OTE can provide greater effects and avoid the loss of genetic effects.
There is a large body of research demonstrating that the brain structure and functions of psychological and personality factors. In structural magnetic resonance imaging (sMRI) studies, OTE has been reported to be related to the prefrontal cortex (PFC) (DeYoung et al., 2005), the gray matter volume of the inferior parietal lobule, the middle temporal gyrus and the lingual gyrus (DeYoung et al., 2010). Kapogiannis further revealed a negative correlation between OTE and the bilateral fusiform gyrus, right medial prefrontal cortex (OFC) and left insular lobe (Kapogiannis et al., 2013). In functional magnetic resonance imaging (fMRI) studies, most studies examine the resting-state functional connectivity. Beaty et al. (2016) found that OTE was significantly correlated with the functional connectivity of default network. Beaty and colleagues further explore the dynamic functional connectivity of OTE, and they found a significant association between OTE and the dynamic functional connectivity of default network and the cognitive control network (Beaty et al., 2018).
Above all, OTE research has always been a hot topic in gene and neuroimaging studies. Previous studies used candidate gene approaches to explore the genetic mechanism of OTE. However, OTE has been shown to be polygenic, and GPSs can provide greater genetic effects than other genetic analysis methods (Middeldorp et al., 2011). Moreover, numerous studies focused on behavioral genetics have directly explored the relationship between OTE and genes. As far as we know, however, previous studies have never examined the potential effects of OTE on this cumulative effect at the level of brain mechanisms. This model has been used primarily to examine the personality traits of normal people. We hypothesize that the neural phenotypes are related to brain structure and functions in some specific regions, such as the PFC, OFC, fusiform gyrus (Jauk et al., 2015), and in default network (Beaty et al., 2016); additionally, these regions had been reported previously in studies on OTE behavior research networks (Beaty et al., 2016). In the present study, we combined GPSs, resting-state fMRI and sMRI to determine the neural phenotypes influenced by OTE genetic variants.
In the present study, 586 college students were recruited from Southwest University in Chongqing (mean ± SD = 19.5 ± 1.5), including 180 males and 406 females. All participants were right-handed and healthy, with no history of neurological or psychological disorders and provided written informed consent. All participants were required to complete brain imaging data and genotyping data acquisition. This study was approved by the Southwest University Brain Imaging Center Institutional Review Board.
All structural and functional MRI scans were performed on a 3T Trio scanner (Siemens Medical Systems, Erlangen, Germany) at the Brain Imaging Center, Southwest University.
The resting-state fMRI images were acquired using a gradient echo-planar imaging sequence: repetition time (TR)/echo time (TE) = 2,000 ms/30 ms, flip angle = 90°, slices = 32, thickness = 3 mm, field of view (FOV) = 240 × 240 mm2, resolution matrix = 64 × 64, voxel size = 3.4 × 3.4 × 3 mm3. High-resolution T1 structure images were acquired using a magnetization-prepared rapid gradient echo (MPRAGE) sequences: TR/TE = 1,900 ms/2.52 ms, inversion time = 900 ms, flip angle = 9°, slices = 176, thickness = l mm, FOV = 256 × 256 mm2, voxel size = 1 × l × l mm3.
The DPARSF software1 and the REST toolkit (Song et al., 2011) were based on MATLAB (Math Works, Natick, MA, United States), and SPM12 was used to preprocess the resting-state data. First, the first 10 volumes were discarded to allow the signal to reach equilibrium. Then, the remaining images were preprocessed by slice-time correction; head motion correction, removing the data of subjects whose head moved more than 2 mm on the X, Y, and Z axis or whose rotation angle exceeded 2°; spatial standardization and image registration to the Montreal Neurological Institute (MNI) standard template; resampling into 3 × 3 × 3 mm3; using a 6 mm FWHM Gaussian kernel for spatial smoothing to improve the signal-to-noise ratio of the image; removing linear drift; and using 0.01–0.08 Hz bandpass filtering to extract the low-frequency oscillation signalpart, eliminating physiological noise.
We used ROIs based on the templates from Dosenbach to construct whole-brain network (Dosenbach et al., 2007). 160-region network definition has been shown to perform better than the 90-parcel AAL atlas and voxel-based graph in representing some aspects of the functional organization of the brain with fMRI data (Dosenbach et al., 2010). The time series of 160 regions were extracted by averaging the time series of each ROI, and Pearson correlation between each pair of ROIs was calculated, generating 12,720 FC edges.
Cortical surface reconstruction and cortical thickness measurements were obtained using the FreeSurfer toolkit2. This set of methods was originally proposed by Dale in the 1990s (Dale et al., 1999; Fischl et al., 1999a), and improvements over the years finally resulted in an automated process (Fischl et al., 1999b, 2001, 2002; Ségonne et al., 2004; Fischl, 2012). First, a spatial adaptive non-local mean filter was used to denoise structural images (T1 volume) and start skull stripping. Next, cerebrospinal fluid, white matter and gray matter are automatically segmented, and the cortical surface is reconstructed to produce a smooth representation of the GM-WM interface (white surface) and the GM-CSF (cortical surface). After normalized estimation of triangular mesh space, the mesh is transformed over the GM-WM boundary. The surface patch curvature is calculated; then, the surface is modified to ensure that the curvature of the local surface is at the most appropriate scale. Then, the first 5 EPI volumes of each participant are discarded, and the slice timing is corrected. To map the brains of all subjects into a common space, the reconstructed surfaces are recorded onto an average cortical surface map using a non-linear program that optimizes the cross-boundary alignment of sulcal and gyral features (Fischer and Getis, 1999).
Approximately 2 μg genome DNA (gDNA) was extracted from 250 μl whole blood using an Axypre Blood Genomic DNA Kit (Corning Life Sciences, cat. no. 11313KC3). The concentration of all gDNA was quantified with the Qubit2.0 Fluorometer (Life Technologies, cat. no. Q32866) and the Qubit dsDNA HS Assay Kit (Life Technologies, cat. no. Q32854). gDNA samples were genotyped on the Illumina HumanExome v1.1 BeadChip (Illumina, San Diego, CA, United States) according to the manufacturer’s specifications. The genotyping module of Genomestudio v3.0 (Illumina, San Diego, CA, United States) was used to determine the genotypes based on the fluorescent signal with standard cluster algorithm.
Quality control of the genomic data was performed using PLINK v1.93 (Purcell, 2009; Chang et al., 2015). First, individuals with discordant sex information, outlying heterozygosity rate (± 3 SD from the mean) and SNPs call rate < 0.98 were removed. Duplicated and high-relatedness (pi-hat > 0.185) individuals were also excluded. Then, SNPs were filtered out with the following criteria: missingness > 0.02, Hardy-Weinberg equilibrium p < 10–4, and minor allele frequency (MAF) < 0.05. To control for population stratification, a principal component analysis (PCA) was performed using EIGENSTRAT version 6.1.3 and the HapMap phase 3 reference data set (Price et al., 2006). After 10 principal components (PCs) were obtained, individuals with PC1 or PC2 ± 6 SD from the mean were removed. After quality control, the SNP data was imputed from the EAS population of the 1,000 Genomes Phase3 reference dataset using the Michigan Imputation Server4 (Das et al., 2016). Phasing and imputation 1,000 were conducted using Eagle v2.3 and Minimac4 (Loh et al., 2016). Imputed SNPs were excluded with info score (R2 < 0.6) and MAF < 0.01.
GPSs of OTE were calculated using the “score” command in the PLINK software with reference to Purcell (2009). The reference GWAS statistics were derived from a Big Five personality GWAS meta-analysis published by the Genetics of Personality Consortium (De Moor et al., 2012)5. A total of 2.4 M single-nucleotide polymorphisms was found in 10 samples (17,375 adults) and five replicated samples (3,294 adults). From this result, we obtained the effect size of SNP risk alleles (obtained by logarithmic transformation of Odds Ratio value) and the correlation significance (p-value). Then, representative SNPs were selected from each linkage disequilibrium (LD) block, which made the loci included in the calculation of GPSs relatively independent. Finally, different GWAS association significance (p = 0.0001, 0.001, 0.01, 0.05, 0.25, 0.5, 1) was selected, and the risk effects of the corresponding reference sites were multiplied by the number of risk alleles (0, 1, or 2) carried by the subjects to obtain the GPSs under the seven threshold values. Previous studies have shown that a polygenic risk score (PRS) with a threshold of 0.05 generated the highest risk score prediction accuracy (Ripke et al., 2014). Another study also found that PRS6 (p = 0.05) constructed from schizophrenia-related alleles produced the highest risk score with prediction accuracy for hippocampal activity (Chen et al., 2018). Therefore, the GPSs with a threshold of 0.05 were selected for the main results. Other threshold results are shown in Supplementary Materials.
For each hemisphere, a multivariate general linear model (GLM) was used to test the correlation between each subject’s surface-based morphometry measures at each vertex and OTE GPSs. Age and gender were treated as the independent covariates of the GLM. A Monte Carlo simulation was used to control multiple comparisons (vertex-wise threshold of p < 0.001, cluster-wise threshold of p < 0.05) (Ledberg et al., 1998). Next, the cortical thickness values of brain regions significantly correlated with GPSs of OTE were extracted. Then, the cortical thickness and GPSs were then Z-transformed. Finally, correlation analysis was used to calculate the relationship between cortical thickness and GPSs.
Firstly, the Pearson correlation was calculated between each of the 12,720 (in the160 nodes network) edges and the GPSs of OTE after been transformed fisher Z across all subjects, and age and gender were treated as the independent covariates of the GLM. Afterward, the false discovery rate (FDR) (p < 0.05) in GRETNA software was used to control multiple comparisons.
Multivariate regression was used to establish a statistical model in 586 subjects. Sex and age were used as covariates to calculate the correlation between cortical thickness and GPSs (p = 0.05 for example, other thresholds in Supplementary Material). After performing Monte Carlo simulation (vertex-wise threshold of p < 0.001, cluster-wise threshold of p < 0.05), a cluster survived—fusiform [size (mm2) = 281.81, X = −37, Y = −17, Z = −25.30, NVtxs = 579] (Figure 1).
Figure 1. Associations between GPSs and cortical thickness. Statistical parametric maps from multiple linear regression analysis, after controlling for age and sex, demonstrated the correlation of GPSs and cortical thickness of the left fusiform [smooth = 10, Size (mm2) = 281.81, X = –37, Y = –17, Z = –25.30, NVtxs = 579]. The red bar denotes increased correlation between the cortical thickness of the left fusiform and GPSs after controlling for age and sex. The blue bar denotes decreased correlation between the cortical thickness of the left fusiform and GPSs after controlling for age and sex (A). Scatter plots of the correlations between the significant regions and the GPSs (B).
We then examined the associations between GPSs and the strength of functional connectivity. Age and gender were treated as the covariates, the Pearson correlation analysis was performed between GPSs and functional connectivity. The results showed a negative association between GPSs of OTE and the functional connectivity between the left IPS (x = −36, y = −69, z = 40) and the right posterior occipital lobe (x = 33, y = −81, z = −2) (p < 0.001, r = −0.20) (Figure 2).
Figure 2. The functional connectivity between the left IPS (x = –36, y = –69, z = 40) and the right posterior occipital lobe (x = 33, y = –81, z = v2). Participants with high GPSs showed significantly lower RSFC in these regions (A). Scatter plots of the correlations between GPSs and the functional connectivity (B).
The present study examined the relationship between the GPSs of OTE and cortical thickness as well as for intrinsic functional connectivity. The higher GPSs were significantly associated with a thinner fusiform gyrus and a weaker functional connectivity between IPS and the right posterior occipital lobe. Studies of behavioral genetics have indicated that a single gene directly acts on OTE, such as DRD4 and 5-HTT (Kalbitzer et al., 2009; DeYoung et al., 2011). The present results suggested that OTE, a complex personality trait, was influenced by multiple genes and mediated by the structure and function of the brain. The present study firstly combined neuroimaging data and gene data to identify two neural phenotypes in a region shown in previous studies to be related to OTE. Stimulating these phenotypes may influence the relationship between genes and OTE, thereby achieving the goal of improving OTE. The current findings could deepen our understanding of the internal mechanism of the formation of the OTE personality trait and can also help with the intervention in and cultivation of an open personality.
In the present study, a negative correlation between the GPSs of OTE and the cortical thickness of the fusiform gyrus were observed. The fusiform gyrus, located at the middle and bottom of the visual syncortex, is generally considered to be involved in face recognition and secondary classification of objects (McCarthy et al., 1997; Joseph and Gathers, 2002; Ramírez et al., 2014). Kapogiannis et al. (2013) found a negative correlation between OTE and the bilateral fusiform gyrus, right medial prefrontal cortex (OFC) and left insular lobe. Vivid visual stimuli have been associated with the activation of fusiform and temporal lobe (Wheeler et al., 2000). Moreover, individuals with high OTE tend to seek novel experiences and feelings through their senses (Aluja et al., 2003). The thinning fusiform gyrus may not be a biomarker of cognitive impairment but, rather, of the cognitive disinhibition of flexible cognition (Vartanian et al., 2018). Therefore, the present findings may suggest that people with higher GPSs of OTE have thicker spindle gyrus pruning, which allows for faster information flows, higher cognitive flexibility, and enhanced sensitivity to new stimuli. Furthermore, an important characteristic of the OTE population has been noted as high creativity (McCrae, 1987). Diminishing cortical thickness in areas including fusiform gyrus, left frontal lobe, lingual, and cuneus is associated with increased creativity (Jung et al., 2010). The mechanism of cortical thinning has been thought to involve more centralized functional activation in skill acquisition to improve information efficiency (Durston and Casey, 2006). In brief, the present study suggests that reduced cortical thickness in the fusiform gyrus may associated with fewer information barriers and increased cognitive flexibility, and the thinner fusiform gyrus is a gene-mediated intermediate phenotype for high OTE.
Importantly, the present study has shown that GPSs of OTE were negatively associated with the functional connectivity between the IPS and the right posterior occipital lobe. IPS is related mainly to visual perception, cognitive flexibility, guidance of attention, inhibitory control and working memory (Duhamel et al., 1998; Rottschy et al., 2012; Cieslik et al., 2013; Müller et al., 2015; Christopoulos et al., 2018). Higher OTE is associated with higher cognitive flexibility (DeYoung et al., 2005). Previous studies have shown that OTE is related to IPS. For example, Wright and colleagues reported a negative correlation between OTE/intelligence and inferior parietal cortical thickness in healthy elderly participants (Wainwright et al., 2008). Vartanian et al. (2018) also found that left hemispheric cortical thickness was negatively correlated with OTE, which was shown to be true for the left MTG, superior temporal gyrus, inferior parietal lobule and middle frontal gyrus (). Therefore, the present findings suggest that IPS influences GPSs of OTE through cognitive flexibility. Furthermore, IPS is located in the default network (Dosenbach et al., 2010). Previous study showed that the default network (DN) was related to OTE with more effective information processing (Patel et al., 2012). DN activity has been associated with spontaneous cognitive processes, such as autobiographical memory retrieval, future imagery, mental reasoning theory, moral decision-making judgment, creative cognition, and meditation, which are consistent with OTE (Beaty et al., 2016). Variations in the default network may be crucial to understanding individual differences in OTE (DeYoung and Allen, 2019). The occipital lobe is responsible for processing language, motion sense, abstract concepts and visual information (Kato et al., 1993; Bedny et al., 2011). An event-related potential (ERP) study showed greater theta event-related synchronization in the parietal and occipital regions, particularly on the right side on a sample of high OTE (Aftanas et al., 2001). Zhiyan and Singer (1997) demonstrated that people with high OTE scores were more likely to enter into mind wandering in visual tasks and demonstrate less attention. Individuals with high GPSs of OTE were more likely to diverge from outside visual stimuli through visual areas such as the occipital lobe to generate novel ideas. Furthermore, the parietal and occipital regions tend to be disconnected from the right temporal region when highly open groups engage in fantasies or generating new ideas (Camfield, 2008). It has also been demonstrated that the lower functional connectivity between the occipital and parietal is associated with, the lower exogenous visual top-down attention effect (Tsubomi et al., 2009). Previous findings suggest that the connections between the IPS and the occipital posterior lobe is partly regulated by PGSs of OTE. The weaker connections between IPS and posterior occipital lobe may be associated with lower attention and better access to mind wandering and generation of new ideas.
Finally, the present study has several limitations. First, the GWAS data used in this study to construct the polygenic score was obtained from the GPC, which is a database of European samples. However, the genetic data involved in the current study are from Chinese sample, and the calculation of polygenic score may be biased by race and culture (Liu et al., 2017). However, to our knowledge, there is no large gene database of Asian personality traits. Future research could establish Chinese genetic database to improve the statistical power of polygenic scores. Furthermore, the participants involved in the present study have high homogeneity. The age of participants is concentrated in the 18–20 range. Therefore, the funding of the present study is difficult to generalize to other age groups. Future research could examine individual differences in genetic and brain mechanisms on a wide age range sample.
In summary, our study suggested that the GPSs of OTE were negatively correlated with the cortical thickness of the fusiform gyrus and with the functional connectivity between the IPS and the posterior occipital lobe. These findings provide new insights that improve our understanding of the relationship between the intermediate phenotype of the brain biomarkers and genetic architecture of OTE.
The datasets presented in this article are not readily available because: This article contains genetic data and is not transferable. Requests to access the datasets should be directed to cWl1ajMxOEBzd3UuZWR1LmNu.
The studies involving human participants were reviewed and approved by the Southwest University Brain Imaging Center Institutional Review Board. The patients/participants provided their written informed consent to participate in this study.
ZR, CL, and JM analyzed the data and wrote the manuscript. ZR revised the manuscript and responsed to reviewers. JQ proposed the idea of the study and drafted the outline of the manuscript. QL and LS offered technical assistance in analyzing data. XW and LS proposed the idea of data analysis. All authors contributed to data acquisition.
This research was supported by the National Natural Science Foundation of China (31771231 and 32071070), the Natural Science Foundation of Chongqing (cstc2019jcyj-msxmX0520 and cstc2020jcyj-msxmX0299), the planned project of Chongqing humanities and Social Sciences (2018PY80 and 2019PY51), and the Fundamental Research Funds for the Central Universities (SWU119007), Chang Jiang Scholars Program, National Outstanding Young People Plan, Chongqing Talent Program. We also extend our gratitude to all participants.
The authors declare that the research was conducted in the absence of any commercial or financial relationships that could be construed as a potential conflict of interest.
The Supplementary Material for this article can be found online at: https://www.frontiersin.org/articles/10.3389/fnins.2020.607912/full#supplementary-material
Abdellaoui, A., Nivard, M. G., Hottenga, J. J., Fedko, I., Verweij, K. J. H., Baselmans, B. M. L., et al. (2018). Predicting loneliness with polygenic scores of social, psychological and psychiatric traits. Genes Brain Behav. 17:e12472. doi: 10.1111/gbb.12472
Aftanas, L., Varlamov, A., Pavlov, S., Makhnev, V., and Reva, N. (2001). Event-related synchronization and desynchronization during affective processing: emergence of valence-related time-dependent hemispheric asymmetries in theta and upper alpha band. Int. J. Neurosci. 110, 197–219. doi: 10.3109/00207450108986547
Aluja, A., Garcıa, O., and Garcıa, L. F. (2003). Relationships among extraversion, openness to experience, and sensation seeking. Pers. Individ. Dif. 35, 671–680. doi: 10.1016/s0191-8869(02)00244-1
Amare, A. T., Schubert, K. O., Tekola-Ayele, F., Hsu, Y.-H., Sangkuhl, K., Jenkins, G., et al. (2018). Association of the polygenic scores for personality traits and response to selective serotonin reuptake inhibitors in patients with major depressive disorder. Front. Psychiatry 9:65. doi: 10.3389/fpsyt.2018.00065
Beaty, R. E., Chen, Q., Christensen, A. P., Qiu, J., Silvia, P. J., and Schacter, D. L. (2018). Brain networks of the imaginative mind: dynamic functional connectivity of default and cognitive control networks relates to openness to experience. Hum. Brain Mapp. 39, 811–821. doi: 10.1002/hbm.23884
Beaty, R. E., Kaufman, S. B., Benedek, M., Jung, R. E., Kenett, Y. N., Jauk, E., et al. (2016). Personality and complex brain networks: the role of openness to experience in default network efficiency. Hum. Brain Mapp. 37, 773–779. doi: 10.1002/hbm.23065
Bedny, M., Pascual-Leone, A., Dodell-Feder, D., Fedorenko, E., and Saxe, R. (2011). Language processing in the occipital cortex of congenitally blind adults. Proc. Natl. Acad. Sci. U.S.A. 108, 4429–4434. doi: 10.1073/pnas.1014818108
Camfield, D. A. (2008). The Biological Basis of Openness to Experience. Melbourne, VIC: Swinburne University of Technology, Brain Sciences Institute.
Chang, C. C., Chow, C. C., Tellier, L. C., Vattikuti, S., Purcell, S. M., and Lee, J. J. (2015). Second-generation PLINK: rising to the challenge of larger and richer datasets. Gigascience 4:7.
Chen, Q., Ursini, G., Romer, A. L., Knodt, A. R., Mezeivtch, K., Xiao, E., et al. (2018). Schizophrenia polygenic risk score predicts mnemonic hippocampal activity. Brain 141, 1218–1228. doi: 10.1093/brain/awy004
Christopoulos, V. N., Kagan, I., and Andersen, R. A. (2018). Lateral intraparietal area (LIP) is largely effector-specific in free-choice decisions. Sci. Rep. 8:8611.
Cieslik, E. C., Bamberger, K., Rottschy, C., and Eickhoff, S. B. (2013). Unterschiedliche neuronale netzwerkes für die verarbeitung kognitiver interferenz–eine ALE-meta-analyse. Klinische Neurophysiol. 44, 72.
Dale, A. M., Fischl, B., and Sereno, M. I. (1999). Cortical surface-based analysis: I. Segmentation and surface reconstruction. Neuroimage 9, 179–194.
Das, S., Forer, L., Schönherr, S., Sidore, C., Locke, A. E., Kwong, A., et al. (2016). Next-generation genotype imputation service and methods. Nat. Genet. 48, 1284–1287. doi: 10.1038/ng.3656
De Moor, M. H. M., Costa, P. T., Terracciano, A., Krueger, R. F., De Geus, E. J. C., Toshiko, T., et al. (2012). Meta-analysis of genome-wide association studies for personality. Mol. Psychiatry 17, 337–349.
DeYoung, C. G. (2014). “Openness/Intellect: a dimension of personality reflecting cognitive exploration,” in APA Handbook of Personality and Social Psychology: Personality Processes and Individual Differences, Vol. 4, eds M. Mikulincer, P. R. Shaver, M. L. Cooper, and R. J. Larsen (Washington, WA: American Psychological Association), 369–399. doi: 10.1037/14343-017
DeYoung, C. G., and Allen, T. A. (2019). “Personality neuroscience,” in Handbook of Personality Development, eds D. P. McAdams, R. L. Shiner, and J. L. Tackett (New York, NY: Guilford Press), 79.
DeYoung, C. G., Cicchetti, D., Rogosch, F. A., Gray, J. R., Eastman, M., and Grigorenko, E. L. (2011). Sources of cognitive exploration: genetic variation in the prefrontal dopamine system predicts openness/intellect. J. Res. Pers. 45, 364–371. doi: 10.1016/j.jrp.2011.04.002
DeYoung, C. G., Hirsh, J. B., Shane, M. S., Papademetris, X., Rajeevan, N., and Gray, J. R. (2010). Testing predictions from personality neuroscience: brain structure and the big five. Psychol. Sci. 21, 820–828. doi: 10.1177/0956797610370159
DeYoung, C. G., Peterson, J. B., and Higgins, D. M. (2005). Sources of openness/intellect: cognitive and neuropsychological correlates of the fifth factor of personality. J. Pers. 73, 825–858. doi: 10.1111/j.1467-6494.2005.00330.x
DeYoung, C. G., Peterson, J. B., Séguin, J. R., and Tremblay, R. E. (2008). Externalizing behavior and the higher order factors of the Big Five. J. Abnorm. Psychol. 117, 947–953. doi: 10.1037/a0013742
Dima, D., and Breen, G. (2015). Polygenic risk scores in imaging genetics: usefulness and applications. J. Psychopharmacol. 29, 867–871. doi: 10.1177/0269881115584470
Dosenbach, N. U. F., Fair, D. A., Miezin, F. M., Cohen, A. L., Wenger, K. K., Dosenbach, R. A. T., et al. (2007). Distinct brain networks for adaptive and stable task control in humans. Proc. Natl. Acad. Sci. U.S.A. 104, 11073–11078. doi: 10.1073/pnas.0704320104
Dosenbach, N. U. F., Nardos, B., Cohen, A. L., Fair, D. A., Power, J. D., Church, J. A., et al. (2010). Prediction of individual brain maturity using fMRI. Science 329, 1358–1361. doi: 10.1126/science.1194144
Dudbridge, F. (2013). Power and predictive accuracy of polygenic risk scores. PLoS Genet. 9:e1003348. doi: 10.1371/journal.pgen.1003348
Duhamel, J. R., Colby, C. L., and Goldberg, M. E. (1998). Ventral intraparietal area of the macaque: congruent visual and somatic response properties. J. Neurophysiol. 79, 126–136. doi: 10.1152/jn.1998.79.1.126
Durston, S., and Casey, B. J. (2006). What have we learned about cognitive development from neuroimaging? Neuropsychologia 44, 2149–2157. doi: 10.1016/j.neuropsychologia.2005.10.010
Fischer, M. M., and Getis, A. (1999). New advances in spatial interaction theory. Pap. Reg. Sci. 78, 117–118. doi: 10.1111/j.1435-5597.1999.tb00735.x
Fischl, B., Liu, A., and Dale, A. M. (2001). Automated manifold surgery: constructing geometrically accurate and topologically correct models of the human cerebral cortex. IEEE Trans. Med. Imaging 20, 70–80. doi: 10.1109/42.906426
Fischl, B., Salat, D. H., Busa, E., Albert, M., Dieterich, M., Haselgrove, C., et al. (2002). Whole brain segmentation: automated labeling of neuroanatomical structures in the human brain. Neuron 33, 341–355.
Fischl, B., Sereno, M. I, and Dale, A. M. (1999a). Cortical surface-based analysis: II: inflation, flattening, and a surface-based coordinate system. Neuroimage 9, 195–207. doi: 10.1006/nimg.1998.0396
Fischl, B., Sereno, M. I, Tootell, R. B. H., and Dale, A. M. (1999b). High−resolution intersubject averaging and a coordinate system for the cortical surface. Hum. Brain Mapp. 8, 272–284. doi: 10.1002/(sici)1097-0193(1999)8:4<272::aid-hbm10>3.0.co;2-4
International Schizophrenia Consortium (2009). Common polygenic variation contributes to risk of schizophrenia and bipolar disorder. Nature 460, 748–752. doi: 10.1038/nature08185
Jauk, E., Neubauer, A. C., Dunst, B., Fink, A., and Benedek, M. (2015). Gray matter correlates of creative potential: a latent variable voxel-based morphometry study. Neuroimage 111, 312–320. doi: 10.1016/j.neuroimage.2015.02.002
Joseph, J. E., and Gathers, A. D. (2002). Natural and manufactured objects activate the fusiform face area. Neuroreport 13:935. doi: 10.1097/00001756-200205240-00007
Jung, R. E., Segall, J. M., Jeremy, B. H., Flores, R. A., Smith, S. M., Chavez, R. S., et al. (2010). Neuroanatomy of creativity. Hum. Brain Mapp. 31, 398–409.
Kalbitzer, J., Frokjaer, V. G., Erritzoe, D., Svarer, C., Cumming, P., and Nielsen, F. Å, et al. (2009). The personality trait openness is related to cerebral 5-HTT levels. Neuroimage 45, 280–285. doi: 10.1016/j.neuroimage.2008.12.001
Kapogiannis, D., Sutin, A., Davatzikos, C., Costa, P. Jr., and Resnick, S. (2013). The five factors of personality and regional cortical variability in the Baltimore longitudinal study of aging. Hum. Brain Mapp. 34, 2829–2840. doi: 10.1002/hbm.22108
Kato, T., Kamei, A., Takashima, S., and Ozaki, T. (1993). Human visual cortical function during photic stimulation monitoring by means of near-infrared spectroscopy. J. Cereb. Blood Flow Metab. 13, 516–520. doi: 10.1038/jcbfm.1993.66
Kaufman, S. B., Quilty, L. C., Grazioplene, R. G., Hirsh, J. B., Gray, J. R., Peterson, J. B., et al. (2016). Openness to experience and intellect differentially predict creative achievement in the arts and sciences. J. Pers. 84, 248–258. doi: 10.1111/jopy.12156
Ledberg, A., Åkerman, S., and Roland, P. E. (1998). Estimation of the probabilities of 3D clusters in functional brain images. Neuroimage 8, 113–128. doi: 10.1006/nimg.1998.0336
Liu, B., Zhang, X., Cui, Y., Qin, W., Tao, Y., Li, J., et al. (2017). Polygenic risk for schizophrenia influences cortical gyrification in 2 independent general populations. Schizophrenia Bull. 43, 673–680.
Loh, P. R., Danecek, P., Palamara, P. F., Fuchsberger, C., Reshef, Y. A., Finucane, H. K., et al. (2016). Reference-based phasing using the haplotype reference Consortium panel. Nat. Genet. 48, 1443–1448. doi: 10.1038/ng.3679
McCarthy, G., Puce, A., Gore, J. C., and Allison, T. (1997). Face-specific processing in the human fusiform gyrus. J. Cogn. Neurosci. 9, 605–610. doi: 10.1162/jocn.1997.9.5.605
McCrae, R. R. (1987). Creativity, divergent thinking, and openness to experience. J. Pers. Soc. Psychol. 52, 1258–1265. doi: 10.1037/0022-3514.52.6.1258
McCrae, R. R. (1993). Openness to experience as a basic dimension of personality. Imagin. Cogn. Pers. 13, 39–55. doi: 10.2190/h8h6-qykr-keu8-gaq0
Middeldorp, C. M., De Moor, M. H. M., McGrath, L. M., Gordon, S. D., Blackwood, D. H., Costa, P. T., et al. (2011). The genetic association between personality and major depression or bipolar disorder. A polygenic score analysis using genome-wide association data. Transl. Psychiatry 1:e50.
Müller, V. I., Langner, R., Cieslik, E. C., Rottschy, C., and Eickhoff, S. B. (2015). Interindividual differences in cognitive flexibility: influence of gray matter volume, functional connectivity and trait impulsivity. Brain Struct. Funct. 220, 2401–2414. doi: 10.1007/s00429-014-0797-6
Patel, R., Spreng, R. N., Shin, L. M., and Girard, T. A. (2012). Neurocircuitry models of posttraumatic stress disorder and beyond: a meta-analysis of functional neuroimaging studies. Neurosci. Biobehav. Rev. 36, 2130–2142. doi: 10.1016/j.neubiorev.2012.06.003
Pereira-Morales, A. J., Adan, A., Lopez-Leon, S., and Forero, D. A. (2018). Personality traits and health-related quality of life: the mediator role of coping strategies and psychological distress. Ann. Gen. Psychiatry 17:25.
Plomin, R., and von Stumm, S. (2018). The new genetics of intelligence. Nat. Rev. Genet. 19:148. doi: 10.1038/nrg.2017.104
Price, A. L., Patterson, N. J., Plenge, R. M., Weinblatt, M. E., Shadick, N. A., and David, R. (2006). Principal components analysis corrects for stratification in genome-wide association studies. Nat. Genet. 38, 904–909. doi: 10.1038/ng1847
Ramírez, F. M., Cichy, R. M., Allefeld, C., and Haynes, J. D. (2014). The neural code for face orientation in the human fusiform face area. J. Neurosci. 34, 12155–12167. doi: 10.1523/jneurosci.3156-13.2014
Ripke, S., Neale, B. M., Corvin, A., Walters, J. T. R., Farh, K.-H., Holmans, P. A., et al. (2014). Biological insights from 108 schizophrenia-associated genetic loci. Nature 511:421. doi: 10.1038/nature13595
Rottschy, C., Langner, R., Dogan, I., Reetz, K., Laird, A. R., Schulz, J. B., et al. (2012). Modelling neural correlates of working memory: a coordinate-based meta-analysis. Neuroimage 60, 830–846. doi: 10.1016/j.neuroimage.2011.11.050
Ségonne, F., Dale, A. M., Busa, E., Glessner, M., Salat, D., Hahn, H. K., et al. (2004). A hybrid approach to the skull stripping problem in MRI. Neuroimage 22, 1060–1075. doi: 10.1016/j.neuroimage.2004.03.032
Song, X.-W., Dong, Z.-Y., Long, X.-Y., Li, S.-F., Zuo, X.-N., Zhu, C.-Z., et al. (2011). REST: a toolkit for resting-state functional magnetic resonance imaging data processing. PLoS One 6:e25031. doi: 10.1371/journal.pone.0025031
Tsubomi, H., Ikeda, T., Hanakawa, T., Hirose, N., Fukuyama, H., and Osaka, N. (2009). Connectivity and signal intensity in the parieto-occipital cortex predicts top-down attentional effect in visual masking: an fMRI study based on individual differences. Neuroimage 45, 587–597. doi: 10.1016/j.neuroimage.2008.11.028
Vartanian, O., Wertz, C. J., Flores, R. A., Beatty, E. L., Smith, I., Blackler, K., et al. (2018). Structural correlates of openness and intellect: implications for the contribution of personality to creativity. Hum. Brain Mapp. 39, 2987–2996. doi: 10.1002/hbm.24054
Visscher, P. M., Brown, M. A., and McCarthy, M. I, and Yang, J. (2012). Five years of GWAS discovery. Am. J. Hum. Genet. 90, 7–24. doi: 10.1016/j.ajhg.2011.11.029
Vukasović, T., and Bratko, D. (2015). Heritability of personality: a meta-analysis of behavior genetic studies. Psychol. Bull. 141, 769–785. doi: 10.1037/bul0000017
Wainwright, M. A., Wright, M. J., Luciano, M., Geffen, G. M., and Martin, N. G. (2008). Genetic covariation among facets of openness to experience and general cognitive ability. Twin Res. Hum. Genet. 11, 275–286. doi: 10.1375/twin.11.3.275
Wheeler, M. E., Petersen, S. E., and Buckner, R. L. (2000). Memory’s echo: vivid remembering reactivates sensory-specific cortex. Proc. Natl. Acad. Sci. U.S.A. 97, 11125–11129. doi: 10.1073/pnas.97.20.11125
Keywords: openness to experience, genome-wide polygenic scores, cortical thickness, functional connectivity, brain structure
Citation: Ren Z, Liu C, Meng J, Liu Q, Shi L, Wu X, Song L and Qiu J (2021) Effects of the Openness to Experience Polygenic Score on Cortical Thickness and Functional Connectivity. Front. Neurosci. 14:607912. doi: 10.3389/fnins.2020.607912
Received: 18 September 2020; Accepted: 09 December 2020;
Published: 11 January 2021.
Edited by:
Yanjie Su, Peking University, ChinaReviewed by:
Zhi Yang, Shanghai Mental Health Center, ChinaCopyright © 2021 Ren, Liu, Meng, Liu, Shi, Wu, Song and Qiu. This is an open-access article distributed under the terms of the Creative Commons Attribution License (CC BY). The use, distribution or reproduction in other forums is permitted, provided the original author(s) and the copyright owner(s) are credited and that the original publication in this journal is cited, in accordance with accepted academic practice. No use, distribution or reproduction is permitted which does not comply with these terms.
*Correspondence: Jiang Qiu, cWl1ajMxOEBzd3UuZWR1LmNu
†These authors have contributed equally to this work
Disclaimer: All claims expressed in this article are solely those of the authors and do not necessarily represent those of their affiliated organizations, or those of the publisher, the editors and the reviewers. Any product that may be evaluated in this article or claim that may be made by its manufacturer is not guaranteed or endorsed by the publisher.
Research integrity at Frontiers
Learn more about the work of our research integrity team to safeguard the quality of each article we publish.