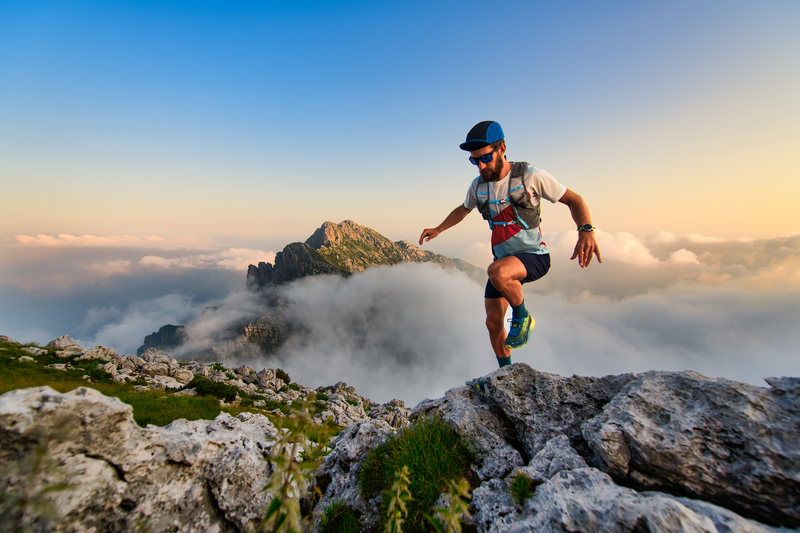
94% of researchers rate our articles as excellent or good
Learn more about the work of our research integrity team to safeguard the quality of each article we publish.
Find out more
ORIGINAL RESEARCH article
Front. Neurosci. , 20 November 2020
Sec. Brain Imaging Methods
Volume 14 - 2020 | https://doi.org/10.3389/fnins.2020.575538
Schizophrenia is a complex disorder about which much is still unknown. Potential treatments, such as transcranial magnetic stimulation (TMS), have not been exploited, in part because of the variability in behavioral response. This can be overcome with the use of response biomarkers. It has been however shown that repetitive transcranial magnetic stimulation (rTMS) can the relieve positive and negative symptoms of schizophrenia, particularly auditory verbal hallucinations (AVH). This exploratory work aims to establish a quantitative methodological tool, based on high-density electroencephalogram (HD-EEG) data analysis, to assess the effect of rTMS on patients with schizophrenia and AVH. Ten schizophrenia patients with drug-resistant AVH were divided into two groups: the treatment group (TG) received 1 Hz rTMS treatment during 10 daily sessions (900 pulses/session) over the left T3-P3 International 10-20 location. The control group (CG) received rTMS treatment over the Cz (vertex) EEG location. We used the P300 oddball auditory paradigm, known for its reduced amplitude in schizophrenia with AVH, and recorded high-density electroencephalography (HD-EEG, 256 channels), twice for each patient: pre-rTMS and 1 week post-rTMS treatment. The use of HD-EEG enabled the analysis of the data in the time domain, but also in the frequency and source-space connectivity domains. The HD-EEG data were linked with the clinical outcome derived from the auditory hallucinations subscale (AHS) of the Psychotic Symptom Rating Scale (PSYRATS), the Quality of Life Scale (QoLS), and the Depression, Anxiety and Stress Scale (DASS). The general results show a variability between subjects, independent of the group they belong to. The time domain showed a higher N1-P3 amplitude post-rTMS, the frequency domain a higher power spectral density (PSD) in the alpha and beta bands, and the connectivity analysis revealed a higher brain network integration (quantified using the participation coefficient) in the beta band. Despite the small number of subjects and the high variability of the results, this work shows a robust data analysis and an interplay between morphology, spectral, and connectivity data. The identification of a trend post-rTMS for each domain in our results is a first step toward the definition of quantitative neurophysiological parameters to assess rTMS treatment.
Hallucinations are sensory perceptions occurring in the absence of an external stimulus. Auditory verbal hallucinations (AVH) are positive psychotic symptoms of schizophrenia and a diagnostic feature in the pathology, occurring in an estimated 60–70% of people with this disorder. An increased interaction among the auditory-language and striatal brain regions occurs while patients hallucinate (Ćurčić Blake et al., 2017). Patients with AVH present evidence of structural brain alterations associated with these perceptions, such as reduced gray matter volume in the superior temporal gyrus (Kasai et al., 2003), including the primary auditory cortex, and abnormal connectivity among the temporal, prefrontal, and anterior cingulate regions (Homan, 2013; Ćurčić Blake et al., 2017). Among the empirically supported theories of the origin of AVH, are a misinterpretation of inner speech (Frith and Done, 1988) and aberrant activation of the auditory cortex (Dierks et al., 1999). Almost one-third of patients with positive psychotic schizophrenia present treatment resistant symptoms (Howes et al., 2009) and there is a compelling need for novel treatments.
Transcranial magnetic stimulation (TMS) is a non-invasive method used over the past 25 years in the treatment of neurobehavioral disorders (Stanford et al., 2008). It uses an alternating magnetic field to induce an electrical current in the brain, depolarizing neurons and generating action potentials. Wassermann et al. (1996) and Chen et al. (1997) reported that 1 Hz repetitive TMS (rTMS) reduces the excitability of cortical neurons in healthy individuals. Based on these effects, Hoffman et al. (1999) hypothesized that 1 Hz rTMS delivered to the left temporoparietal cortex reduced activity in receptive language areas associated with AVH in patients with schizophrenia. Neuroimaging studies of AVH showed an increased activation in the absence of an external stimulus in the left primary auditory cortex of subjects with this symptom (Kompus et al., 2011).
Our goal was to establish a methodological tool to quantitatively assess the cognitive processes of people suffering with AVH. We aimed to develop an hypothesis that can validate psychometric results with event related potential (ERP) morphology (time domain), power spectral density (frequency domain), and brain connectivity in patients undergoing 10 sessions of low-frequency rTMS.
To date, the mechanism of the effect of rTMS on AVH has only been inferred from the hypothesis of left temporoparietal cortex dysfunction and the behavioral response is variable from patient to patient. Dozens of studies have used inhibitory low frequency rTMS over the T3-P3 EEG location as a treatment for pharmaco-resistant AVH with the effects measured mainly with psychometric scales (Lefaucheur et al., 2014; Slotema et al., 2014). Physiological measures linked to specific brain areas and biomarkers of target engagement and response are needed to optimize treatment. Indeed, response biomarkers are essential as predictors of treatment where behavioral outcomes can be variable. They may also be very useful for rTMS treatments, where multiple parameters, including frequency, train length, intensity, duration, and treatment schedule can all influence effectiveness and should be optimized before full-scale clinical trials are attempted. Past studies indicate a relation between different frequency bands and cognitive processes (Klimesch et al., 1998). The power spectral density (PSD) changes observed in response to attentional demands can be of interest to monitor patients with schizophrenia behavior. Electroencephalographic (EEG) measures, including spectral density and evoked potentials (Barr et al., 2011), have been used as measures of the physiological response to TMS treatment. For instance, it has been observed that rTMS to the dorsolateral prefrontal area increased the P300 response in patients with schizophrenia, but not healthy controls (Lin et al., 2018).
The P300 first described by Sutton et al. (1965), mostly studied as a parameter of voluntary attention (Mazaheri and Picton, 2005), is the leading Event Related Potential (ERP) correlate of target discrimination (Mazaheri and Picton, 2005) and it has been largely employed to characterize schizophrenia (Jeon and Polich, 2003). Previous studies have found that patients with auditory hallucinations exhibit reduced P300 amplitudes (Jeon and Polich, 2003; Bramon et al., 2004; Fisher et al., 2014). Many works based on P300 also analyzed N100, the negative deflection that occurs approximately 100 ms after the auditory stimulus, noting a relation with working memory (Lijffijt et al., 2009). The mean amplitudes of the auditory N100 and P300 responses are decreased in patients with schizophrenia in comparison to healthy participants (Ogura et al., 1991; Ford et al., 2001; Earls et al., 2016).
Here, we compared alterations in the P300 response after left temporal and vertex [used as a control in previous studies with schizophrenia and AVH (Nyffeler et al., 2006; Nowak et al., 2008; Loo et al., 2010)] TMS in patients with schizophrenia using three different approaches : time, frequency, and source-space connectivity. Patients also underwent a battery of neurobehavioral and tests before and after treatment.
We remained descriptive in our analysis before and after treatment, at group and single levels.
The patients were recruited from the psychiatric wards and outpatient clinics of the National Hospital of Iceland. They were diagnosed with schizophrenia, following the ICD-10 (International Classification of Diseases, Tenth Revision, Clinical Modification) schizophrenia classification (F20). Only those still experiencing persistent AVH after finishing at least two 6–8 week drug treatments were selected. Patients were excluded if they had history of seizures, were using cannabis or drinking more than three units of alcohol daily, were using any other illegal drugs within 1 month prior to the beginning of the study, or showing TMS contraindications during the pre-treatment interview (Rossi et al., 2011). Permission from the Health Research Ethics Committee at the University Hospital of Iceland was obtained (approval no. 21.2018). Ten patients (7 men and 3 women, mean age = 32, SD = 6.41) were selected for the study. All of them were taking medications. Table 1 sums up the patient information. The patients were randomly assigned into two groups. Five patients (four men and one woman, mean age 35.2, SD = 5.12,range 30–48) were included in the active treatment group (TG). They received ten daily sessions of 15 min 1 Hz frequency rTMS (900 pulses/session) at 100% of abductor pollicis brevis resting motor threshold (RMT) applied at T3-P3 location. Five patients (three men and two women, mean age 29.6, SD = 3.92, range 26–39) were included in the control group (CG) and received rTMS at 100% RMT to the vertex of CG 10-20 location. The EEG and psychometric data were acquired twice in each patient group; before the rTMS treatment (pre-treatment) and within 1 week after completing ten sessions of rTMS treatment (post-treatment). This produced 20 datasets: five pre-TMS TG, five post-TMS TG, five pre-TMS CG, and five post-TMS CG. Figure 1 shows the experimental set-up and workflow designed for this study. Figure 2 shows the pre-processing and data analysis pipeline used for this study.
Three scales were used to collect clinical information pre- and post-treatment.
Psychotic Symptom Rating Scales (PSYRATS) auditory hallucinations subscale (AHS) is an interview measuring auditory hallucinations using 11 items rated on a five-point ordinal scale (0–4). The scale measures the severity of AVH for the past week in 11 dimensions which are: frequency, duration, location, loudness, beliefs about origin, negative content, intensity of negative content, amount of distress, intensity of distress, disruption of life, and control. PSYRATS has shown excellent inter-rater reliability and good discriminant and convergent validity for both chronic and first episode psychosis (Haddock et al., 1999; Drake et al., 2007).
Quality of life was assessed with a 16 item self-report scale, consisting of five conceptual domains of quality of life: material and physical well-being, relationships with other people, social community and civic activities, personal development and fulfillment, and recreation. The scale has been shown to have good test-retest reliability and good convergent and discriminant validity (Flanagan, 1978).
The DASS is a measure of mental health focusing on the three traits of depression, anxiety, and stress. It consists of 42 items, rated on a four point Likert type scale of how much that symptom occurred in the last week. In clinical samples the scale has shown excellent internal consistency and temporal stability as well as excellent discriminant validity and good convergent validity (Brown et al., 1997).
P300 was measured with an auditory oddball paradigm attention task. The recordings took place between 11h00 and 14h00 for a duration of 1 h. The subjects sat with their eyes closed. The frequent (F) and the rare (R) auditory stimuli were presented binaurally through headphones at an interstimulus interval between tones of constant 1.1 s. The loudness was adjusted for each participant. For each subject, there was one trial of 200 tones, comporting a random tone occurrence with a probability of 0.2, leading to 160 frequent tones and 40 rare (Marcu et al., 2020). We required the participants to focus on the rare stimuli without counting or moving a finger.
The EEG was recorded using a 256 channel system (ANT Neuro, Netherlands) with an electrooculogram (EOG) electrode placed below the right eye and a ground electrode placed on the left side of the neck. Data pre-processing and analysis were performed with Brainstorm (Tadel et al., 2011) and MATLAB 2018b (MathWorks, Inc., Natick, 158 Massachusetts, USA).
The data were sampled at 1,024 Hz and re-referenced to the average of left and right mastoid electrodes (R19R, L19L). A bandpass filter was set between 0.5 and 70 Hz and notch filter from 49 to 51 Hz was used to remove undesired monomorphic artifacts from 50 Hz mains electricity. Bad channels were manually removed when EEG voltage was higher than ±80 μV; if more than 10% of the channels showed too much noise or incorrect signal, the whole trial was rejected. The signals were digitized in epochs of 1,200 ms, starting 500 ms before the presentation of each auditory stimulus (–500 to +700 ms). Baseline correction was performed using pre-stimulus 500 ms to pre-stimulus 100 ms window and channels marked as bad were removed and interpolated. Individual trials were visually inspected and rejected when indicative of excessive muscle activity, eye movements, or other artifacts.
N100-P300 complex values of both frequent and rare stimuli were calculated and plotted via MATLAB 2018b for each subject (pre- and post-treatment for patients groups).
The scalp was divided into 5 regions of interest (ROI), see Figure 2. The 254 electrodes were partitioned as follows: 80 channels for the Frontal region (F), 59 for the Parietal region (P), 69 for the Occipital region (O), 23 for Right Temporal lobe (RT), and 23 for Left Temporal lobe (LT) (Schartner et al., 2015).
N1-P3 wave signals were calculated for the entire N100-P300 complex from the average of channels of every ROI as the difference between the most negative voltage value within time range of 80–150 ms (N100) and the most positive voltage value within time range of 250–500 ms (P300).
The differences between frequent and rare stimulus and pre- and post-treatment were also computed and plotted.
The power spectral density (PSD) was computed for each epoch with Welch's method, using Brainstorm, with the following frequency bands: delta (0.5–4 Hz), theta (4–8 Hz), alpha (8–13 Hz), beta (13–30 Hz), gamma (30–70 Hz). The PSD has been divided by the associated bandwidth for each frequency band.
Using the same scalp division as that of the time analysis, the PSD of electrodes within the same ROI were averaged for frequent and rare stimuli, pre- and post-treatment for each subject.
The PSD difference post-pre treatment and frequent—rare were computed for each subject.
The connectivity has been computed at the cortical level using the "EEG source connectivity" method. It consists of estimating the brain sources (over 68 regions of interest—ROI—) and then computing the statistical coupling between these reconstructed sources. The weighted minimum norm estimate (wMNE) and the Phase Locking Value (PLV) were used to solve the inverse problem and compute the functional connectivity, respectively. This choice was based on previous comparative studies showing good performance of this combination on simulated and real data. (Hassan et al., 2014, 2017; Hassan and Wendling, 2018). The analysis has been performed only on the beta and gamma bands, due to window length constraints (here 700 ms). The source-space networks were estimated for each trial, subject and conditions. To compare between conditions, the networks were quantified using network measures that allow the extraction of the topological properties of the networks. We made the choice here to focus on network integration as it is the most consistent network feature that changes due to electric/magnetic stimulation (Modolo et al., 2020) or brain disorders (Stam, 2014). The network integration reflects the ability of the brain network to integrate information form different and distant brain regions, a key feature of efficient information processing. To quantify the network integration, we used the participation coefficient (PC), to calculate the interactions between brain modules (distant sub-networks), on the thresholded connectivity matrices (here 20%). We used the brain connectivity toolbox (BCT) (Rubinov and Sporns, 2010) to compute the PC (http://www.brain-connectivity-toolbox.net/).
The analysis for each individual revealed general consistent results. The results picturing the evolution (increase or decrease) of the neurophysiological and psychometric data and are detailed in Table 2 for the TG, and in Table 3 for the CG. The associated numerical values are detailed in Table 4 for the TG and in Table 5 for the CG. The analysis of the psychometric tests revealed that four out of five subjects in TG (Tables 2, 4) and three out of five subjects in CG (Tables 3, 5) felt improved condition after the treatment, whereas the other subjects remained neutral or reported worse psychometric scores. In the time domain analysis, the N1-P3 amplitude was globally higher post-treatment than pre-treatment, for six subjects, two in TG (Tables 2, 4) and four in CG (Tables 3, 5). The PSD increased post-treatment mainly for the alpha band and beta band globally, for six subjects as well, two in TG (Tables 2, 4) and four in CG (Tables 3, 5). No trends were detectable for the gamma and theta bands. In several subjects, the right temporal area showed an opposite behavior compared to the other regions. The connectivity results showed an increased network integration (increase in participation coefficient) during post-treatment for frequent, for the beta band especially, for seven subjects, four in CG (Tables 3, 5), three in TG (Tables 2, 4). Due to the small sample size and high variability of the results, we will discuss selected study cases individually. The following four patients were selected due to their interplay between psychometric score and neurophysiological results, independant of treatment. Two subjects (T2, in TG and C3, in CG) presented an improvement in the psychometric score post-TMS, and the two others presented a stagnation in the psychometric (C2, in CG) or a decrement (T5, in TG). The rest of the data are provided in the Supplementary Material. There were no significant changes on AVH severity measured with PSYRATS AHS, in QoL and DASS global scores after rTMS between TG and CG.
Table 2. Treated Group: Increase (↑), decrease (↓), or constancy (–) of the value after treatment of N1-P3, Connectivity, Psychometric and Power spectral density (PSD) of the frequent () and rare () stimuli (F, frontal; P, parietal; O, occipital; LT, left temporal; RT, right temporal).
Table 3. Control Group: Increase (↑), decrease (↓) or constancy (-) of the value after treatment of N1-P3, Connectivity, Psychometric and Power Spectral Density (PSD) of the frequent () and rare () stimuli(F, frontal; P, parietal; O, occipital; LT, left temporal; RT, right temporal).
Table 4. Treatment Group: Values pre and post treatment of N1-P3, Connectivity, Psychometric and Power spectral density of the frequent () and rare () stimuli(F, frontal; P, parietal; O, occipital; LT, left temporal; RT, right temporal).
Table 5. Control Group: Values pre and post treatment of N1-P3, Connectivity, Psychometric and Power spectral density of the frequent () and rare () stimuli(F, frontal; P, parietal; O, occipital; LT, left temporal; RT, right temporal).
The two patients detailed in this section presented an improvement in their psychometric score post-treatment. We chose to describe them in this section due to their higher values post treatment in the neurophysiological data, (Figures 3A, 4A) in order to find a potential correlation between those two outcomes.
Figure 3. Results of patient T2 : (A) psychometric; (B) Scalp-level frequency analysis; (C) Source-space connectivity; (D) Scalp-level time analysis. The yellow areas in frequency analysis are related to a higher Power Spectral Density (PSD) post-treatment, whereas the blue ones are related to a higher PSD pre-treatment. The size of the node in the connectivity is related to the amount of increase (green) or decrease (orange) participation coefficient (PC) values. The positive bars in time analysis are related to a higher N1-P3 amplitude post-treatment. (QoLS, Quality of Life Scale; DASS, Depression Anxiety Stress Scale; PSYRATS, Psychotic Symptom Rating Scales).
Figure 4. Results of patient C3 : (A) psychometric; (B) Scalp-level frequency analysis; (C) Source-space connectivity; (D) Scalp-level time analysis. The yellow areas in frequency analysis are related to a higher Power Spectral Density (PSD) post-treatment, whereas the blue ones are related to a higher PSD pre-treatment. The size of the node in the connectivity is related to the amount of increase (green) or decrease (orange) participation coefficient (PC) values. The positive bars in time analysis are related to a higher N1-P3 amplitude post-treatment. (QoLS, Quality of Life Scale; DASS, Depression Anxiety Stress Scale; PSYRATS, Psychotic Symptom Rating Scales).
Patient T2 (Figure 3) is a man with paranoid schizophrenia, in the TG, who took part in the study while taking : clozapine, olanzapine, perphenazine, alprazolam, levomepromazine, oxazepam, and melatonin. The psychometric tests (Figure 3A) show an improvement of the quality of life post-treatment, a decreased DASS after TMS and decreased PSYRATS post-treatment. The temporal analysis (Figure 3D) showed a lower N1-P3 amplitude post-treatment, except for the parietal and left temporal parts. The PSD (Figure 3B) showed higher alpha power post-TMS. However, the beta power is lower post-TMS. The connectivity (Figure 3C) revealed a clear higher participation coefficient (represented by the larger green nodes), especially in the left central, left orbito-frontal and the right occipital brain regions. The frontal area showed a relatively lower participation coefficient.
Patient C3 (Figure 4) is a woman with paranoid schizophrenia, in the CG, who tool part in the study while taking : aripriprazole, olanzapine, chloroprothixene, and pregabalin. The psychometric outcome (Figure 4A) revealed an improvement after the treatment. The quality of life increased, the DASS decreased, while the PSYRATS did not change. The time domain analysis (Figure 4D) showed a higher amplitude of the N1-P3 complex after the treatment, except on the temporal regions for the rare stimulus. The PSD (Figure 4B) showed higher alpha power post-TMS, except from the right temporal region for both frequent and rare stimuli. The beta band also showed a higher PSD post-TMS. Finally, the connectivity study (Figure 4C) displayed a globally improved participation coefficient, principally in the frontal, occipital, and central areas of the brain.
The patient detailed in this section presented a stagnation in her psychometric score post treatment (Figure 5A). We chose to describe her in this section in order to find a potential correlation with the neurophysiological data.
Figure 5. Results of patient C2 : (A) psychometric; (B) Scalp-level frequency analysis; (C) Source-space connectivity; (D) Scalp-level time analysis. The yellow areas in frequency analysis are related to a higher Power Spectral Density (PSD) post-treatment, whereas the blue ones are related to a higher PSD pre-treatment. The size of the node in the connectivity is related to the amount of increase (green) or decrease (orange) participation coefficient (PC) values. The positive bars in time analysis are related to a higher N1-P3 amplitude post-treatment. (QoLS, Quality of Life Scale; DASS, Depression Anxiety Stress Scale; PSYRATS, Psychotic Symptom Rating Scales).
Patient C2 (Figure 5) is a woman with paranoid schizophrenia, in the CG, who took part in the study while taking : clozapine, flupenthixol, zopiclone, mirtazapine, escitalopram, metoprolol, and chlorpomazine. The psychometric data (Figure 5A) showed that the treatment did not have a lot of impact on this scale. The quality of life, the DASS and the PSYRATS remained more or less the same. The time domain (Figure 5D) showed a global increase of N1-P3 amplitude post-TMS, except for the parietal region for both stimuli and the frontal region for the frequent stimulus. The PSD analysis (Figure 5B) showed higher alpha and beta power post-TMS (with the exception of frontal frequent stimulus responses in the alpha band). The connectivity analysis (Figure 5C) revealed a balanced participation evolution. Globally the left hemisphere (mainly the entorhinal and frontal) showed a decreased participation coefficient, and the right areas (mainly the frontal and occipital) showed an increased participation coefficients.
The patient detailed in this section presented a decrease in their psychometric score post treatment. We chose to describe him due to his lower values in the neurophysiological data post treatment (Figure 6A), in order to find a potential correlation between those two outcomes.
Figure 6. Results of patient T5 : (A) psychometric; (B) Scalp-level frequency analysis; (C) Source-space connectivity; (D) Scalp-level time analysis. The yellow areas in frequency analysis are related to a higher Power Spectral Density (PSD) post-treatment, whereas the blue ones are related to a higher PSD pre-treatment. The size of the node in the connectivity is related to the amount of increase (green) or decrease (orange) participation coefficient (PC) values. The positive bars in time analysis are related to a higher N1-P3 amplitude post-treatment. (QoLS, Quality of Life Scale; DASS, Depression Anxiety Stress Scale; PSYRATS, Psychotic Symptom Rating Scales).
Patient T5 (Figure 6) is a man with paranoid schizophrenia, in the TG, who took part in the study while taking : clozapine, flupenthixol, zopiclone, mirtazapine, escitalopram, metoprolol, and chlorpomazine. The psychometric data (Figure 6A) showed very little effect of treatment on this scale. The quality of life remained the same, the DASS increased and the PSYRATS slightly increased. The time domain (Figure 6D) showed a global decrease of N1-P3 amplitude post-TMS, except for the parietal region for the rare stimulus. The PSD analysis (Figure 6B) showed a lower alpha power post-TMS, except for the right temporal region. The beta power decreased as well, except for the right temporal region for the frequent stimulus. The connectivity analysis (Figure 6C) showed a globally higher participation coefficient in the right frontal, left central, and occipital brain regions.
The present work aimed to develop hypothesis to assess the effects of TMS in schizophrenia with AVH, analysing EEG data and psychometric outcome. This was based on three different approaches : temporal size (with the calculation of N1P3 complex amplitude), spectral [with the evaluation of the PSD in several frequency bands (theta, alpha, beta, and gamma)] and connectivity, (with the calculation of the participation coefficient in beta and gamma band).
The general results from our study revealed a high variability between individuals, in both groups. This can be explained in several ways : Firstly, subjects were taking a range of medications all of which can interfere with the background neural activity and the generation of ERPs (Javitt et al., 2008). Secondly, the long and tiring recording procedure (around 1 h), and the different states of the patients during the protocol could also have led to varying data quality. Indeed, Polich (1997) highlights the fact that background EEG variation contributes significantly to a high P300 individual variability.
However, there were some indications of improvement in the psychometric results, in four out of five subjects in TG and three out of five in CG. Half of our subjects (six out of 10, four CG, two TG) showed an increase of the N1-P3 amplitude after the treatment, especially for the rare stimulus. Bramon et al. (2004) and Jeon and Polich (2003) established that patients with schizophrenia and AVH presented an inhibition to the P300 experiment. Thus, the clearer presence of N100 and P300 waves post-TMS, which is a known response to the auditory oddball task (Patel and Azzam, 2005), suggested a response to TMS. Likewise, the spectral analysis displayed an increase of the PSD in alpha and beta bands for six subjects (4 in CG, 2 in TG). Ray and Cole (1985) demonstrated that those bands were directly linked to attention, focus, emotional and cognitive processes. A higher power in these bands could be indicative of a change in those mechanisms. Finally, our brain connectivity results showed a global increased participation coefficient in the beta band after treatment, for six subjects (3 in TG, 4 in CG). These results lead us to the conclusion that TMS seemed to have a positive impact on the patients, in both groups. However, it is not possible to assume that the location where the treatment was applied had a different impact on the brain function. The results in connectivity analysis show indeed an improved participation coefficient thus a better network integration independently from the group. Therefore, we discussed the results without regard to the patients' group. We chose to underline in the results section some patients that showed an agreement between the psychometric scores and our neurophysiological data.
It is interesting to note that for patient C3, where the psychometric results were better post-TMS, all three neurophysiological components used for this study revealed a higher value post-TMS. For this patient, there seems to be a clear link between clinical and neurophysiological outcomes. For T2, the trend is there, but is less obvious, with half of the neurophysiological results being in concordance with the improved psychometric score. Conversely, patient T5 had a deterioration in their psychometric post-TMS, and the same tendency is visible in their neurophysiological data.
Although there was significant dissociation between clinical and neurophysiological outcome, the participation coefficient from the connectivity analysis was the parameter that seemed to interact most closely with the psychometric results, followed by alpha power. Concerning the connectivity analysis, our results showed an increased network integration in some brain regions and a slight decrease in other regions, different for every patient. This reconfiguration of the brain network has been widely reported in the literature when stimulating the brain using electrical and magnetic means, and is also present in several brain disorders (Fornito et al., 2015). The increased network integration may be related to better information processing in the human brain and more efficient networks. This increase in network integration was associated with a decrease in this same integration in other brain regions, reflecting the inter-subject variability. Although the small sample size did not allow us to test statistical significance, we showed a clear “trend” of reshaping of the functional brain network between the different conditions.The frequency analysis revealed the most interesting changes in power spectrum, mainly in the alpha and beta bands. There was higher alpha power post-TMS, which is less prominent but still visible in the beta power. The gamma and theta power did not show any clear trends. The fact that alpha and beta bands are directly linked to attention, focus and emotional tasks (Ray and Cole, 1985) is interesting. A higher power post-TMS linked with a better score in the psychometric scale could indicate that the improvement of these cognitive mechanisms was directly linked to the progress of the patient's clinical condition. Due to our small sample size, this has to be put in perspective, and nothing definitive can be assessed. However, there was a “trend” of an increase in alpha and beta powers post-TMS that is linked with an improvement in the clinical outcome. Finally, the time domain was the area of analysis presenting the most variability. Half of the patients presented a higher N1-P3, especially in the rare stimuli, but no clear trend was established between this outcome and the clinical outcome. However, due to its conclusive results in studies related to schizophrenia with AVH (Ogura et al., 1991; Ford et al., 2001; Earls et al., 2016), our small number of subjects, as well as its subject inter-variability (Polich, 1997), it is a paradigm that should be taken in account in further studies.
Considering the fact that psychometric tests are a semi-self assessment evaluation of patients condition, this work is a first step toward a development of a hypothesis to correlate and validate psychometrics with quantitative neurophysiological data. We suggest further investigation of any link between psychometrics and neurophysiological data under the umbrella of TMS, focusing mainly on the participation coefficient in the beta band and the power spectral density in alpha band. The beta power as well as the N1-P3 amplitude should also be considered of interest.
The study has many limitations. Firstly, due to the very small sample size, it was not possible to assess our results definitively. Rather, we aimed to discover trends in order to generate hypotheses for further study. Secondly, the patients were also undergoing their usual treatment, including antipsychotic and sedative medications. This did not change between pre- and post-rTMS conditions but might have influenced the background neural activity and the generation of the ERPs (Javitt et al., 2008). Thirdly, the experimental procedure was long (1 h) and tiring and some patients had difficulty cooperating and maintaining task engagement, which may have affected data quality. Muscle and movement artifacts added noise to the EEG signal, requiring a thorough pre-processing and the exclusion of many trials. We encourage similar experiments with patients in the supine position to reduce the noise and improve the data quality, making them easier to process and analyze. Finally, in this study we only analyzed the oddball auditory paradigm. We recommend pursuing this work using other procedures as well, in order to have a more complete overview of the results.
Future studies of rTMS and neurophysiological markers in schizophrenia should recruit larger number of participants than the present study. The possible association of AVH and other symptoms of schizophrenia with variations in P300, PSD and other EEG markers should be studied further. This may help to establish whether the PSD in the alpha and beta bands, the N1-P3 complex and the participation coefficient in beta bands are reliable biomarkers of the neural response to TMS in patients with schizophrenia and AVH.
After conducting TMS, most patients showed an evolution in psychometric data as well as on the neurophysiological quantitative data, independent of the stimulation site. We examined the interplay between the psychometric and the neurophysiological data. When the psychometric improved post-TMS, we could observe an increased network integration mainly, through the participation coefficient in the beta bands, a higher alpha and beta band power, and sometimes a higher N1-P3 amplitude. Due to the small sample size, it is not possible to assess definitively the impact of TMS on the brain function in schizophrenia, nor the correlation between psychometric and neurophysiological data. However, our results suggest that brain connectivity, through the participation coefficient, alpha and beta power bands, were highly related with the psychometric score, and that N1-P3, despite his variability, should be investigated. This hypothesis will have to be verified in further studies, with a larger sample size, and an improved recording procedure, leading to a better data quality. This is a first step toward the definition of quantitative neurophysiological parameters to assess TMS treatment.
The raw data supporting the conclusions of this article will be made available by the authors, without undue reservation.
The studies involving human participants were reviewed and approved by Health Research Ethics Committee at the University Hospital of Iceland (approval no. 21.2018). The patients/participants provided their written informed consent to participate in this study.
RA and RS wrote the manuscript and performed temporal and spectral group analyses with support from PG. OB, SS, AJ, and EÍ carried out the rTMS treatment. RA, VJ, AJ, EÍ, and OB performed the oddball auditory experiments and HD-EEG acquisitions. OB and EW designed the TMS protocol and made additions to the manuscript. MHas and SY performed the brain connectivity analysis and made additions to the manuscript. MHar and BM designed the psychometrics study. VJ conducted the psychometrics interview. OB and VJ performed psychometrics analysis. DJ reviewed and made additions to the manuscript. OB and PG conceived the original idea and PG coordinated the work. All authors contributed to the article and approved the submitted version.
EW was received support from the Intramural Research Program of the National Institute of Neurological Disorders and Stroke. Landspitali Scientific funds supported this work.
The authors declare that the research was conducted in the absence of any commercial or financial relationships that could be construed as a potential conflict of interest.
The Supplementary Material for this article can be found online at: https://www.frontiersin.org/articles/10.3389/fnins.2020.575538/full#supplementary-material
AHS, Auditory Hallucinations Subscale; AVH, Auditory Verbal Hallucinations; CG, Control Group; DASS, Depression Anxiety Stress Scale; ERP, Event-related Potential; HD EEG, High Density Electroencephalogram; PC, Participation coefficient; PSD, Power Spectral Density; PSYRATS, Psychotic Symptom Rating Scales; QoLS, Quality of Life Scale; rTMS, Repetitive Transcranial Magnetic Stimulation; TG, Treatment Group; TMS, Transcranial Magnetic Stimulation.
Barr, M. S., Farzan, F., Arenovich, T., Chen, R., Fitzgerald, P. B., and Daskalakis, Z. J. (2011). The effect of repetitive transcranial magnetic stimulation on gamma oscillatory activity in schizophrenia. PLoS ONE 6:e22627. doi: 10.1371/journal.pone.0022627
Bramon, E., Rabe-Hesketh, S., Sham, P., Murray, R. M., and Frangou, S. (2004). Meta-analysis of the p300 and p50 waveforms in schizophrenia. Schizophr. Res. 70, 315–329. doi: 10.1016/j.schres.2004.01.004
Brown, T. A., Chorpita, B. F., Korotitsch, W., and Barlow, D. H. (1997). Psychometric properties of the depression anxiety stress scales (dass) in clinical samples. Behav. Res. Ther. 35, 79–89. doi: 10.1016/S0005-7967(96)00068-X
Chen, R., Classen, J., Gerloff, C., Celnik, P., Wassermann, E. M., Hallett, M., et al. (1997). Depression of motor cortex excitability by low-frequency transcranial magnetic stimulation. Neurology 48, 1398–1403. doi: 10.1212/WNL.48.5.1398
Ćurčić Blake, B., Ford, J. M., Hubl, D., Orlov, N. D., Sommer, I. E., Waters, F., et al. (2017). Interaction of language, auditory and memory brain networks in auditory verbal hallucinations. Prog. Neurobiol. 148, 1–20. doi: 10.1016/j.pneurobio.2016.11.002
Dierks, T., Linden, D. E., Jandl, M., Formisano, E., Goebel, R., Lanfermann, H., et al. (1999). Activation of heschl's gyrus during auditory hallucinations. Neuron 22, 615–621. doi: 10.1016/S0896-6273(00)80715-1
Drake, R., Haddock, G., Tarrier, N., Bentall, R., and Lewis, S. (2007). The psychotic symptom rating scales (psyrats): their usefulness and properties in first episode psychosis. Schizophr. Res. 89, 119–122. doi: 10.1016/j.schres.2006.04.024
Earls, H. A., Curran, T., and Mittal, V. (2016). A meta-analytic review of auditory event-related potential components as endophenotypes for schizophrenia: perspectives from first-degree relatives. Schizophr. Bull. 42, 1504–1516. doi: 10.1093/schbul/sbw047
Fisher, D. J., Smith, D. M., Labelle, A., and Knott, V. J. (2014). Attenuation of mismatch negativity (mmn) and novelty p300 in schizophrenia patients with auditory hallucinations experiencing acute exacerbation of illness. Biol. Psychol. 100, 43–49. doi: 10.1016/j.biopsycho.2014.05.005
Flanagan, J. C. (1978). A research approach to improving our quality of life. Am. Psychol. 33, 138–147. doi: 10.1037/0003-066X.33.2.138
Ford, J. M., Mathalon, D. H., Kalba, S., Marsh, L., and Pfefferbaum, A. (2001). N1 and p300 abnormalities in patients with schizophrenia, epilepsy, and epilepsy with schizophrenialike features. Biol. Psychiatry 49, 848–860. doi: 10.1016/S0006-3223(00)01051-9
Fornito, A., Zalesky, A., and Breakspear, M. (2015). The connectomics of brain disorders. Nat. Rev. Neurosci. 16, 159–172. doi: 10.1038/nrn3901
Frith, C. D., and Done, D. J. (1988). Towards a neuropsychology of schizophrenia. Br. J. Psychiatry 153, 437–443. doi: 10.1192/bjp.153.4.437
Haddock, G., McCarron, J., Tarrier, N., and Faragher, E. B. (1999). Scales to measure dimensions of hallucinations and delusions: the psychotic symptom rating scales (psyrats). Psychol. Med. 29, 879–889. doi: 10.1017/S0033291799008661
Hassan, M., Dufor, O., Merlet, I., Berrou, C., and Wendling, F. (2014). EEG source connectivity analysis: from dense array recordings to brain networks. PLoS ONE 9:e105041. doi: 10.1371/journal.pone.0105041
Hassan, M., Merlet, I., Mheich, A., Kabbara, A., Biraben, A., Nica, A., et al. (2017). Identification of interictal epileptic networks from dense-EEG. Brain Topogr. 30, 60–76. doi: 10.1007/s10548-016-0517-z
Hassan, M., and Wendling, F. (2018). Electroencephalography source connectivity: aiming for high resolution of brain networks in time and space. IEEE Signal Process. Magaz. 35, 81–96. doi: 10.1109/MSP.2017.2777518
Hoffman, R. E., Boutros, N. N., Berman, R. M., Roessler, E., Belger, A., Krystal, J. H., et al. (1999). Transcranial magnetic stimulation of left temporoparietal cortex in three patients reporting hallucinated voices. Biol. Psychiatry 46, 130–132. doi: 10.1016/S0006-3223(98)00358-8
Homan, P. (2013). On the neurobiology of hallucinations. J. Psychiatry Neurosci. (2009) 34, 260–262.
Howes, O., Egerton, A., Allan, V., McGuire, P., Stokes, P., and Kapur, S. (2009). Mechanisms underlying psychosis and antipsychotic treatment response in schizophrenia: insights from pet and spect imaging. Curr. Pharm. Design 15, 2550–2559. doi: 10.2174/138161209788957528
Javitt, D. C., Spencer, K. M., Thaker, G. K., Winterer, G., and Hajós, M. (2008). Neurophysiological biomarkers for drug development in schizophrenia. Nat. Rev. Drug Discov. 7, 68–83. doi: 10.1038/nrd2463
Jeon, Y.-W., and Polich, J. (2003). Meta-analysis of p300 and schizophrenia: patients, paradigms, and practical implications. Psychophysiology 40, 684–701. doi: 10.1111/1469-8986.00070
Kasai, K., Shenton, M. E., Salisbury, D. F., Hirayasu, Y., Lee, C.-U., Ciszewski, A. A., et al. (2003). Progressive decrease of left superior temporal gyrus gray matter volume in patients with first-episode schizophrenia. Am. J. Psychiatry 160, 156–164. doi: 10.1176/appi.ajp.160.1.156
Klimesch, W., Doppelmayr, M., Russegger, H., Pachinger, T., and Schwaiger, J. (1998). Induced alpha band power changes in the human EEG and attention. Neurosci. Lett. 244, 73–76. doi: 10.1016/S0304-3940(98)00122-0
Kompus, K., Westerhausen, R., and Hugdahl, K. (2011). The paradoxical engagement of the primary auditory cortex in patients with auditory verbal hallucinations: a meta-analysis of functional neuroimaging studies. Neuropsychologia 49, 3361–3369. doi: 10.1016/j.neuropsychologia.2011.08.010
Lefaucheur, J.-P., André-Obadia, N., Antal, A., Ayache, S. S., Baeken, C., Benninger, D. H., et al. (2014). Evidence-based guidelines on the therapeutic use of repetitive transcranial magnetic stimulation (rtms). Clin. Neurophysiol. 125, 2150–2206. doi: 10.1016/j.clinph.2014.05.021
Lijffijt, M., Lane, S. D., Meier, S. L., Boutros, N. N., Burroughs, S., Steinberg, J. L., et al. (2009). P50, n100, and p200 sensory gating: relationships with behavioral inhibition, attention, and working memory. Psychophysiology 46, 1059–1068. doi: 10.1111/j.1469-8986.2009.00845.x
Lin, X.-D., Chen, X.-S., Chen, C., Zhang, L.-J., Xie, Z.-L., Huang, Z.-Y., et al. (2018). Effects of repetitive transcranial magnetic stimulation treatment on event-related potentials in schizophrenia. Chinese Med. J. 131:301. doi: 10.4103/0366-6999.223846
Loo, C. K., Sainsbury, K., Mitchell, P., Hadzi-Pavlovic, D., and Sachdev, P. S. (2010). A sham-controlled trial of left and right temporal rtms for the treatment of auditory hallucinations. Psychol. Med. 40, 541–546. doi: 10.1017/S0033291709990900
Marcu, S., Pegolo, E., Ívarsson, E., Jónasson, A. D., Jónasson, V. D., Aubonnet, R., et al. (2020). Using high density EEG to assess tms treatment in patients with schizophrenia. Eur. J. Transl. Myol. 30, 134–138. doi: 10.4081/ejtm.2019.8903
Mazaheri, A., and Picton, T. W. (2005). EEG spectral dynamics during discrimination of auditory and visual targets. Cogn. Brain Res. 24, 81–96. doi: 10.1016/j.cogbrainres.2004.12.013
Modolo, J., Hassan, M., Ruffini, G., and Legros, A. (2020). Probing the circuits of conscious perception with magnetophosphenes. J. Neural Eng. 17:036034. doi: 10.1088/1741-2552/ab97f7
Nowak, D. A., Grefkes, C., Dafotakis, M., Eickhoff, S., Küst, J., Karbe, H., et al. (2008). Effects of low-frequency repetitive transcranial magnetic stimulation of the contralesional primary motor cortex on movement kinematics and neural activity in subcortical stroke. Arch. Neurol. 65, 741–747. doi: 10.1001/archneur.65.6.741
Nyffeler, T., Wurtz, P., Lüscher, H.-R., Hess, C. W., Senn, W., Pflugshaupt, T., et al. (2006). Repetitive tms over the human oculomotor cortex: comparison of 1-hz and theta burst stimulation. Neurosci. Lett. 409, 57–60. doi: 10.1016/j.neulet.2006.09.011
Ogura, C., Nageishi, Y., Matsubayashi, M., Omura, F., Kishimoto, A., and Shimokochi, M. (1991). Abnormalities in event-related potentials, n100, p200, p300 and slow wave in schizophrenia. Psychiatry Clin Neurosci. 45, 57–65. doi: 10.1111/j.1440-1819.1991.tb00506.x
Patel, S. H., and Azzam, P. N. (2005). Characterization of n200 and p300: selected studies of the event-related potential. Int. J. Med. Sci. 2, 147–154. doi: 10.7150/ijms.2.147
Polich, J. (1997). On the relationship between EEG and p300: individual differences, aging, and ultradian rhythms. Int. J. Psychophysiol. 26, 299–317. doi: 10.1016/S0167-8760(97)00772-1
Ray, W., and Cole, H. (1985). EEG alpha activity reflects attentional demands, and beta activity reflects emotional and cognitive processes. Science 228, 750–752. doi: 10.1126/science.3992243
Rossi, S., Hallett, M., Rossini, P. M., and Pascual-Leone, A. (2011). Screening questionnaire before tms: an update. Clin. Neurophysiol. 122:1686. doi: 10.1016/j.clinph.2010.12.037
Rubinov, M., and Sporns, O. (2010). Complex network measures of brain connectivity: uses and interpretations. Neuroimage 52, 1059–1069. doi: 10.1016/j.neuroimage.2009.10.003
Schartner, M., Seth, A., Noirhomme, Q., Boly, M., Bruno, M.-A., Laureys, S., et al. (2015). Complexity of multi-dimensional spontaneous eeg decreases during propofol induced general anaesthesia. PLoS ONE 10:e0133532. doi: 10.1371/journal.pone.0133532
Slotema, C. W., Blom, J. D., [van Lutterveld], R., Hoek, H. W., and Sommer, I. E. (2014). Review of the efficacy of transcranial magnetic stimulation for auditory verbal hallucinations. Biol. Psychiatry 76, 101–110. doi: 10.1016/j.biopsych.2013.09.038
Stam, C. J. (2014). Modern network science of neurological disorders. Nat. Rev. Neurosci. 15, 683–695. doi: 10.1038/nrn3801
Stanford, A. D., Sharif, Z., Corcoran, C., Urban, N., Malaspina, D., and Lisanby, S. H. (2008). rTMS strategies for the study and treatment of schizophrenia: a review. Int. J. Neuropsychopharmacol. 11, 563–576. doi: 10.1017/S1461145707008309
Sutton, S., Braren, M., Zubin, J., and John, E. R. (1965). Evoked-potential correlates of stimulus uncertainty. Science 150, 1187–1188. doi: 10.1126/science.150.3700.1187
Tadel, F., Baillet, S., Mosher, J. C., Pantazis, D., and Leahy, R. M. (2011). Brainstorm: a user-friendly application for MEG/EEG analysis. Comput. Intell. Neurosci. 2011:879716. doi: 10.1155/2011/879716
Keywords: high-density EEG, TMS (repetitive transcranial magnetic stimulation), P300, schizophrenia, spectral analysis, temporal analysis, brain connectivity
Citation: Aubonnet R, Banea OC, Sirica R, Wassermann EM, Yassine S, Jacob D, Magnúsdóttir BB, Haraldsson M, Stefansson SB, Jónasson VD, Ívarsson E, Jónasson AD, Hassan M and Gargiulo P (2020) P300 Analysis Using High-Density EEG to Decipher Neural Response to rTMS in Patients With Schizophrenia and Auditory Verbal Hallucinations. Front. Neurosci. 14:575538. doi: 10.3389/fnins.2020.575538
Received: 23 June 2020; Accepted: 23 October 2020;
Published: 20 November 2020.
Edited by:
Silvia Comani, University of Studies G. d'Annunzio Chieti and Pescara, ItalyReviewed by:
Chunyan Liu, Capital Medical University, ChinaCopyright © 2020 Aubonnet, Banea, Sirica, Wassermann, Yassine, Jacob, Magnúsdóttir, Haraldsson, Stefansson, Jónasson, Ívarsson, Jónasson, Hassan and Gargiulo. This is an open-access article distributed under the terms of the Creative Commons Attribution License (CC BY). The use, distribution or reproduction in other forums is permitted, provided the original author(s) and the copyright owner(s) are credited and that the original publication in this journal is cited, in accordance with accepted academic practice. No use, distribution or reproduction is permitted which does not comply with these terms.
*Correspondence: Paolo Gargiulo, cGFvbG9AcnUuaXM=
Disclaimer: All claims expressed in this article are solely those of the authors and do not necessarily represent those of their affiliated organizations, or those of the publisher, the editors and the reviewers. Any product that may be evaluated in this article or claim that may be made by its manufacturer is not guaranteed or endorsed by the publisher.
Research integrity at Frontiers
Learn more about the work of our research integrity team to safeguard the quality of each article we publish.