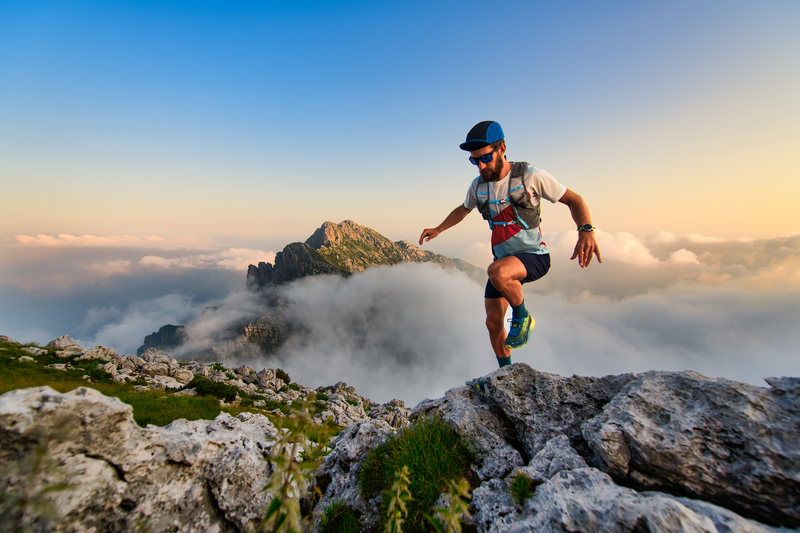
95% of researchers rate our articles as excellent or good
Learn more about the work of our research integrity team to safeguard the quality of each article we publish.
Find out more
ORIGINAL RESEARCH article
Front. Neurosci. , 04 September 2020
Sec. Systems Biology Archive
Volume 14 - 2020 | https://doi.org/10.3389/fnins.2020.00876
Traumatic brain injury (TBI) is a complex and serious disease as its multifaceted pathophysiological mechanisms remain vague. The molecular changes of hippocampal and cortical dysfunction in the process of TBI are poorly understood, especially their chronic effects on metabolic profiles. Here we utilize metabolomics-based liquid chromatography coupled with tandem mass spectrometry coupled with bioinformatics method to assess the perturbation of brain metabolism in rat hippocampus and cortex on day 7. The results revealed a signature panel which consisted of 13 identified metabolites to facilitate targeted interventions for subacute TBI discrimination. Purine metabolism change in cortical tissue and taurine and hypotaurine metabolism change in hippocampal tissue were detected. Furthermore, the associations between the metabolite markers and the perturbed pathways were analyzed based on databases: 64 enzyme and one pathway were evolved in TBI. The findings represented significant profiling changes and provided unique metabolite–protein information in a rat model of TBI following the subacute phase. This study may inspire scientists and doctors to further their studies and provide potential therapy targets for clinical interventions.
Traumatic brain injury (TBI) is defined as brain tissue damage caused by a mechanical force. This worldwide health problem causes high mortality and disability in all ages and countries. It has been estimated that the cases afflicted by TBI exceed 50 million and cost 400 billion US dollars annually (Maas et al., 2017). Unfortunately, specific TBI treatments failed because of very complicated pathophysiology (Berger et al., 2007; Zetterberg et al., 2013; Lichtman et al., 2014). This motivates studies to identify the possible potential pathogenic mechanisms of TBI in search for the underlying targets in brain sites.
To overcome difficulties in the exploration of pathogenic mechanisms following TBI (Maas et al., 1999), omics approaches including transcriptomics, proteomics, and metabolomics have become an emerging technology to discover biomarkers and biological processes by using a global untargeted approach (Lommen et al., 2007). Of omics platforms, metabolomics has become a powerful tool with the aid of quantifiable systems biology research in high-throughput metabolic data sets (Vasilopoulou et al., 2016). This method has been carried out for non-targeted metabolic profiling to reveal novel biomarkers and biochemical pathways to gain new insights into the potential pathogenic mechanisms of TBI (Zhang et al., 2010; Feala et al., 2013; Wolahan et al., 2015). Previous studies showed that the metabolomic differences induced by TBI were distinguished (Chitturi et al., 2018; Dickens et al., 2018; McGuire et al., 2019), and the metabolic signatures and progressions might be beneficial to clinical TBI diagnosis or therapeutic possibilities (Chitturi et al., 2018; Dickens et al., 2018; McGuire et al., 2019).
The characteristics of metabolism profiles during TBI refer to neurologic function alteration and cognitive impairment (Werner and Engelhard, 2007; Chauhan, 2014; Barkhoudarian et al., 2016). Moreover, evidence suggests that disorders (including glutamate excitotoxicity, neuroplasticity, metabolic disruption, and mitochondrial dysfunction) existed for long-lasting periods after TBI (Maas et al., 2008; Faden, 2011; Turner et al., 2013). The hippocampus and the cortex especially show significant aberrations in neural pathways that are associated with spatial learning memory and executive function (Boone et al., 2017). Therefore, investigating the pathogenesis of the hippocampus and the cortex may provide useful information about the potential mechanisms. Previous studies have obtained metabolic fingerprints of the cortex and the hippocampus of TBI, including energy metabolism disorder, oxidative stress, excitotoxic damage, and neuronal damage (Girgis et al., 2016). A non-targeted nuclear magnetic resonance (NMR) metabolomics study examined their metabolic profiles in five brain regions of chronic post-TBI injury (Viant et al., 2005). Although cortical and hippocampal metabolomics studies of acute and chronic TBI were well documented (Pierce et al., 1998; Casey et al., 2008; McGuire et al., 2019), we believe that a description on metabolomics of subacute TBI remains urgent. Because as a transition period, the subacute phase-induced metabolic disturbance may last long, and the pathological cascades may determine the prognosis and the development of TBI.
In this study, we focused on the metabolic changes of subacute TBI in a rat model. Alterations in brain metabolism in the cortex and the hippocampus after subacute TBI in rats were observed and analyzed by non-targeted liquid chromatography coupled with tandem mass spectrometry (LC-MS/MS) metabolomics method. The results tend to provide a more comprehensive understanding of TBI pathophysiology and the therapeutic strategies in the subacute phase. The workflow is illustrated in Figure 1.
All animal experiments were performed according to the relevant guidelines for animal research established by Xiangya Hospital Central South University. All procedures involved in the experiments were approved in compliance with the regulations of the Medical Ethics Committee at Xiangya Hospital Central South University.
Forty male Sprague–Dawley rats (weight: 200–250 g) were obtained from the Laboratory Animal Research Center of Central South University. All rats were between 6 and 8 weeks of age. They were bred with free access to food and water for at least 1 week and housed with 12-h circulation. The rats were randomly divided into two groups: (1) sham group (n = 10): operated rats that underwent the surgical procedures except for an impact-experienced trauma and (2) TBI group (n = 10): rats that experienced controlled cortical impact (CCI). The CCI model was performed following a previous description (Xing et al., 2016). Each rat was administered with 3% pentobarbital (50 mg/kg) prior to surgery. A craniotomy (5 mm in diameter), located at the center of the bregma and the lambda suture lines, was performed with a dental drill at the right portion of the skull. The hammer was made of an automated, controlled-pneumatic-impact device (PSI TBI-0310 Impactor, Precision Systems & Instrumentation, Fairfax Station, VA, United States). The parameters of the brain injury consisted of a 6.0 m/s impact velocity, 5 mm impact depth, and 500 ms dwell time. Then, the incision was closed with sutures and the animal was placed on a heating cushion to maintain the normal core temperature after the impact.
On the 7th day after CCI, the animals were deeply injected with intraperitoneal anesthetic pentobarbital and then perfused with 200 ml of 0.9% normal ice-cold saline. The hippocampal and the cortical tissues surrounding the injured side were rapidly dissected and placed in cryopreservation tubes. Then, the samples were stored in liquid nitrogen and stored at −80°C until LC-MS/MS analysis.
To assess the neurological functions, we used the modified Neurological Severity Score (mNSS) to evaluate the neurological impairments of CCI rats on the 7th day (Xing et al., 2016). The mNSS includes 18 points comprising motor (six points), sensory (two points), beam balance (six points), absent reflexes, and abnormal movements (four points). One point refers to the failure of one task; no points are given for success. Higher scores suggest more severity in such rats (normal score: 0, maximal deficit score: 18).
The LC-MS/MS method was done for quantitative analysis. Mobile phase A (methanol) was comprised of 25 mM ammonium acetate and 25 mM ammonium hydroxide with water. Mobile phase B (Acetonitrile) was applied at a flow rate of 0.4 ml/min by using a 4 l injection volume. Generic high-performance liquid chromatography (HPLC) gradient was used to determine the metabolites in terms of time (0.0, 1.0, 11.0, 14.0, 16.5, 18.5, 20.5, 25.0, 25.1, and 34.0 min): A (10, 10, 13, 20, 30, 50, 80, 80, 10, and 10%) and B (90, 90, 87, 80, 70, 50, 20, 20, 90, and 90%). Agilent 1260 Infinity HPLC (Agilent J&W Scientific, Folsom, CA, United States) with a Waters amide column (2.1 × 100 mm, 3.5 μm) was carried out for the HPLC analysis.
Subsequently, MS/MS analysis was carried out on the Q-Exactive MS/MS (Thermo) in both positive and negative ion modes. The MS parameters for the probe were set based on optimized conditions (auxiliary gas: 13, sheath gas: 40, aux gas heater temperature: 400°C, capillary temperature: 350°C, S-lens: 55, spray voltage: 3.5 kV for positive mode and negative mode). Data-dependent acquisition method was built based on the following parameters: full scan range: 60–900 m/z; normalized collision energies: 10, 17, 25 or 30, 40, 50; automatic gain control MS1: 3e6; ddMS2: 2e5; isolation window: 1.6 m/z; resolution MS1: 70,000; ddMS2: 17,500; maximum injection time MS1: 100 ms; and ddMS2: 45 ms. The full-scan method was set as follows: full scan range: 60–900 m/z, automatic gain control: 3 × 106 ions, resolution: 140,000, and maximum injection time: 100 ms.
Data (Supplementary Material 1, 2) were analyzed by multivariate statistical analysis, including principal component analysis (PCA), partial least-square discrimination analysis (PLS-DA), and orthogonal partial least-square discriminant analysis (OPLS-DA) using SIMCA-P software (version 11.0; Umetrics AB, Umea, Sweden) with log10 transferred and pareto-scale scaling. A PCA model of data set was visualized by clustering trend. PLS-DA and OPLS-DA models were employed for predicted probabilities and supervised discrimination. The values of R2X and Q2Y as goodness-of-fit were performed to estimate how accurate is the validated model and to describe the predictive ability. A total of 100 iterations of the permutation test in the PLS-DA model was subsequently used for reliability validation. According to the OPLS-DA analysis, the potentially different metabolites were selected by variable importance in the projection (VIP) values (VIP>1). Additionally, the potential metabolic signatures with false discovery rate (FDR) ≤ 0.05 and fold change (FC) > 1.5 or < 0.67 were identified. To illustrate the relevant pathways enriched by metabolites, different identifications were investigated using Kyoto Encyclopedia of Genes and Genomes (KEGG) in Metaboanalyst 3.01 with P < 0.05.
We identified the metabolites by mzCloud and ChemSpider. The exacted mass of each feature was submitted to ChemSpider with selected databases of BioCyc, Human Metabolome Database, KEGG, and LipidMAPS. The relative standard deviation of the different metabolites was calculated. We extracted the proteins which are related to significantly different metabolites via the Human Metabolome Database (HMDB). Then, we collected conserved gene-based proteins among these proteins via BLAST-NCBI2 per protein whose mRNA identification >80% was selected. Furthermore, we searched for traumatic brain injury-associated proteins via GeneCard. Finally, we analyzed metabolite–protein associations through the KEGG pathway. The biological pathway, biological process, and their correlation networks were drawn by Gingo package in cytoscape (version 3.5) software based on the KEGG pathway database.
Data for the mNSS tests were expressed as mean ± SD. Prism Graph Pad (version 8.0) software was used for statistical analysis and graphing. Statistical differences were determined using Student’s t-test with P set to <0.05 considered as statistically significant.
Before the surgical operation, the rats were evaluated by mNSS measurement, and each rat had 0 score. On the 7th day after the operation, the mNSS scores of the sham group were less than three points. When compared with the sham group, the TBI group showed significantly higher scores (p < 0.01, more than nine points). The results indicated that TBI led to marked neurological impairments (Figure 2).
Metabolomic profiling was performed on the 7th day after the operation. The metabolic features in the cortex and the hippocampus were detected. A PCA indicated that the TBI-induced cortex or hippocampus tissue group was clearly distinguished from the sham group. The first two principal components in their PCA score plot showed a clear classification between the TBI and the sham groups (Figures 3A, 4A). The PCA loading scatter profiled differences in the direction of the first predictive principal component during the progress of TBI as the most distinct separation features (Figures 3B, 4B). Furthermore, to minimize the effects between groups, we embedded a group separation trend for the TBI and the sham groups into the PLS-DA model. The PLS-DA model with R2Y and Q2Y [R2Y (cum) = 0.965 and Q2Y (cum) = 0.895 for the cortex and R2Y (cum) = 0.997 and Q2Y (cum) = 0.964 for the hippocampus] was obtained to indicate perfect fitting and reliable prediction (Figures 3C, 4C). In addition, a repeated permutation test (consisting of 100 random permutation texts) validated the model without overfitting (R2 was 0.778 for the cortex and 0.934 for the hippocampus; Q2 was −0.129 for the cortex and 0.48 for the hippocampus) (Figures 3D, 4D).
Figure 3. Metabolomic fingerprint analysis for the cortex in principal component analysis (PCA) and partial least-square discrimination analysis (PLS-DA). (A) PCA plots in cortical traumatic brain injury (TBI) vs. sham. (B) Loading plot corresponding to the PCA score scatter plot for cortical TBI. (C) PLS-DA results for cortical TBI [R2Y (cum) = 0.965 and Q2Y (cum) = 0.895]. (D) Validation plot obtained from 100 permutation tests for the cortex.
Figure 4. Metabolomic fingerprint analysis for the hippocampus in principal component analysis (PCA) and partial least-square discrimination analysis (PLS-DA). (A) PCA plots in hippocampal traumatic brain injury (TBI) versus sham. (B) Loading plot corresponding to the PCA score scatter plot for hippocampal TBI. (C) PLS-DA results for hippocampal TBI [R2Y (cum) = 0.997 and Q2Y (cum) = 0.964]. (D) Validation plot obtained from 100 permutation tests for the hippocampus.
The characteristic metabolites related to TBI-induced cortical or hippocampal tissues were detected by OPLS-DA. In the OPLS-DA plot, the reliable potential signatures were visible by maximizing the metabolite pattern between the TBI and the sham groups (Figures 5A,B). Potential metabolites were filtered by selecting the variable projected in an OPLS-DA model with high contributions according to the values of VIP shown in S-plot (Figures 5C,D). The potential metabolic results are summarized in Tables 1, 2. The characteristic metabolites were considered significant according to the following processes: (1) metabolites with VIP > 1, (2) Student’s t-test with P-value < 0.05, (3) FDR < 0.05, and (4) FC > 1.5 or < 0.67. Among these metabolites, eight metabolites for the cortex (betaine, trigonelline, palmitoylcarnitine, uric acid, guanidinoethyl sulfonate, ecgonine, 2′-deoxyinosine, and cholecalciferol) and seven metabolites for the hippocampus (L-norleucine, adenine, ascorbic acid, hypotaurine, propionylcarnitine, betaine, and palmitoylcarnitine) were selected for further bioinformatic analysis.
Figure 5. Orthogonal partial least-squares discriminant analysis (OPLS-DA) mode and S-plot of the subacute traumatic brain injury (TBI) vs. sham group. (A) OPLS-DA models for cortical TBI vs. sham. (B) OPLS-DA models for TBI of the hippocampus vs. sham. (C) OPLS-DA model for TBI of the cortex shown in the S-plot. (D) OPLS-DA model for TBI of the hippocampus is shown in the S-plot, which illustrates the relationship between correlation and covariance.
Table 1. List of significant metabolites identified from the orthogonal partial least-squares discriminant analysis (OPLS-DA) mode for cortical traumatic brain injury (TBI).
Table 2. List of significant metabolites identified from the orthogonal partial least-squares discriminant analysis (OPLS-DA) mode for the hippocampus.
Of these metabolites, six metabolites in the cortex of the TBI group were downregulated in comparison with those of the sham group, and six other metabolites were decreased in the hippocampus. These metabolic profiles, shown in heat maps, suggested a decreased alteration in the metabolic tissue of TBI during the subacute phase (Figures 6A,B). A pathway analysis by a metaboanalyst revealed significantly different metabolites in the cortex or the hippocampus. As shown in Figures 6C,D, the identified metabolites were involved in purine metabolism for the cortical tissue and in taurine and hypotaurine metabolism for the hippocampal tissue, respectively. The results of Venn graphics (Figure 7) showed 13 metabolites in two categories for the cortex and the hippocampus. The areas of overlap in the Venn graphics suggested that betaine and palmitoylcarnitine were both detected in the cortex and the hippocampus, but they changed in opposite trends. Moreover, betaine and palmitoylcarnitine were significantly enriched in glycine serine and threonine metabolism and fatty acid metabolism through analysis with a metaboanalyst following the subacute TBI. The results demonstrated that these metabolite alterations may characterize the variable metabolomics response to subacute TBI.
Figure 6. Results of heat maps and enrichment analysis of pathways. In the heat maps, the green color represents downregulation and the red color represents upregulation. For a metabolome view of the pathways, the colors of the nodes are determined based on the P-value of the pathway, and the radii are based on the impact values of their pathways. (A) Heat map of different metabolites in traumatic brain injury (TBI) of the cortex. (B) Heat map of different metabolites in TBI of the hippocampus. (C) Disturbed metabolic pathway associated with TBI of the cortex on day 7. (D) Disturbed metabolic pathway associated with TBI of the cortex on day 7.
Figure 7. Venn diagram describing the different VIP metabolites in two distinctive brain tissues after traumatic brain injury (TBI) on day 7. (A) A Venn diagram indicates the different metabolites corresponding to two brain tissues, showing with blue arrows the upregulated or downregulated cortical metabolites and red ones for those of the hippocampus. The overlap display shows that the two metabolites are both in response to subacute TBI in the two brain tissues, with different trends. (B) Pathway topology analysis associated with betaine in glycine, serine, and threonine metabolism was carried out by a metaboanalyst. (C) Pathway topology analysis associated with palmitoylcarnitine in fatty acid metabolism was carried out by a metaboanalyst.
To identify the metabolite-related proteins in the cortex and the hippocampus at the subacute period, we employed a metabolite–protein association network based on HMDB analysis (Figures 8, 9). Tables 3, 4 showed that a total of 21 proteins relevant to eight metabolites for the cortex and 43 proteins for seven metabolites for the hippocampus were extracted from the HMDB. For the underlying mechanism of the metabolite-related proteins, 64 proteins were analyzed to elucidate the biological processes and pathways by Cytoscape.
Figure 8. Metabolite-related proteins identified from the database and their associated analysis were visualized by the Cytoscape tool. (A) Representative metabolite–protein interaction network of the cortex, extracted from the database during the subacute phase. The network was constructed with metabolite compounds (yellow and rectangle) and protein enzymes (green and triangle) as nodes. (B) A pie chart provides a visualization of the three biological processes enriched by the proteins. (C) The protein pathway network provides a view of the functional relationships among proteins.
Figure 9. Metabolite-related proteins identified from the database and their associated analysis were visualized by the Cytoscape tool. (A) Representative metabolite–protein interaction network of the hippocampus, extracted from the database following the subacute phase. The network was constructed with metabolite compounds (yellow and rectangle) and protein enzymes (green and triangle) as nodes. (B) A pie chart provides a visualization of the seven biological processes enriched by the proteins. (C) The protein pathway network provides a view of the functional relationships among proteins.
Table 3. Different metabolites in cortical traumatic brain injury and related proteins revealed by the Human Metabolome Database analysis.
Table 4. Different metabolites in hippocampal traumatic brain injury and related proteins revealed by the Human Metabolome Database analysis.
Within the classification of the pie chart (Figures 8B, 9B), three biological processes related to 21 proteins and their related metabolites in the cortex on day 7, including amino acid betaine metabolic process followed by purine nucleotide catabolic process, and seven classifications of biological processes were mainly involved in amino acid betaine metabolic process and cellular amino acid metabolic process for the hippocampus. Moreover, to gain protein profiling, they were imported into Cytoscape for visualization of their corresponding enriched pathways by mapping these proteins into the KEGG reference. The relationship networks with their pathway associations were built by Cytoscape (Figures 8C, 9C). Our results revealed that 21 related proteins were involved in the two main series of pathways including lipid metabolism (fatty acid degradation) and endocrine system (PPAR signaling pathway, adipocytokine signaling pathway, and ovarian steroidogenesis) in the cortex on day 7, and there were four main series of pathways including lipid metabolism (fatty acid degradation and fatty acid metabolism), environmental adaptation (thermogenesis), endocrine system (PPAR signaling pathway), and amino acid metabolism (lysine degradation). The findings indicated that the 43 related proteins were involved in hippocampal TBI on day 7.
To investigate the underlying pathological mechanisms of TBI, 12 TBI-related proteins from 64 metabolite-associated proteins were performed based on GeneCards database. The results showed TBI-induced protein distribution networks with five proteins and their related four metabolites for the cortex and seven proteins and their related four metabolites for the hippocampus, respectively (Figures 10, 11A). In addition, we performed a pathway enrichment analysis using pathway databases in Cytoscape (Figures 11B,C). There are no biological processes and pathways related with cortical metabolites. Three proteins (CRAT, CPT1B, and CPT2) and two hippocampal metabolites (palmitoylcarnitine and propionylcarnitine) were involved in the fatty acid beta degradation pathway (Figure 12).
Figure 10. Perspective of traumatic brain injury (TBI) perturbation on integrated proteins, cortical metabolites: a network of TBI-causing candidate proteins (green and triangle) and cortical metabolites (blue and diamond).
Figure 11. Perspective of traumatic brain injury (TBI) perturbation on integrated proteins, hippocampal metabolites, and pathway: (A) network of TBI-causing candidate proteins (green and triangle) and hippocampal metabolites (blue and diamond). (B) Gene Ontology analysis of seven hippocampal metabolite-related proteins. (C) A subnetwork of three proteins enriched in fatty acid beta oxidation.
Figure 12. Kyoto Encyclopedia of Genes and Genomes (KEGG) pathway from an original KEGG pathway. Fatty acid metabolism KEGG pathway shows the interactions of proteins and metabolites with red circles.
This study uses metabolomics to explore the candidate metabolic signatures of the cortex and the hippocampus after TBI in the subacute phase. We identified the metabolite profile for the hippocampus and for the cortex. A total of 13 differentially expressed genes (DEGs) were screened compared with the sham group. The DEGs’ function was demonstrated by KEGG analysis, which showed two metabolism-related pathways including purine metabolism for the hippocampus and taurine and hypotaurine metabolism for the cortex. Based on the HMDB and the GENECARD database, 12 of the identified proteins related to the metabolites respond to subacute TBI; seven proteins were clustered to one pathway (fatty acid beta oxidation). The results suggest that the metabolic alterations, including the identified metabolites and their enriched pathways, tend to be the potential targets of TBI diagnostics and prognosis. Furthermore, the networks of metabolite-related proteins and their interactions provide an in-depth understanding of the molecular mechanisms in the process of TBI. Additionally, demarcations between the hippocampus and the cortex following subacute TBI demonstrate that different brain regions lead to dysfunctional metabolic perturbation responses.
Recently, the hippocampus and the cortex are the key functional locations in the brain (Yin et al., 2018; Han et al., 2019; Terranova et al., 2019; Vijayakumar et al., 2019). Long-term neurodegeneration results from deficits in the cortex and the hippocampus, which induces brain damage (Arulsamy et al., 2019; Kempuraj et al., 2019). The disorders by TBI-related damage implicates chronic dysregulations with involvements of neuroinflammation (Kempuraj et al., 2019; Needham et al., 2019), immune system (Sharma et al., 2019), neuroplasticity (Yi et al., 2016) and cell survival (Hanlon et al., 2019). The roles of the hippocampus and the cortex are region-dependent alterations occurring in the brain (Yang et al., 2014; Lieblein-Boff et al., 2015; Sapiurka et al., 2016). Therefore, we identified candidate metabolites and their enriched pathways to help reveal the molecular mechanisms of TBI. Our results reveal that these metabolomic characteristics of TBI-induced effects in the hippocampus and the cortex are correlated with many metabolic disturbances.
The consequences of TBI cause changes of neuronal function, especially in the cortex (Carron et al., 2016). Increased cholecalciferol is observed in brain tissues, which is associated with the regulation of calcium homeostasis (Bivona et al., 2018), restoration of autophagy flux (Campbell and Spector, 2012; Cui et al., 2017), and decrease in apoptosis (Li et al., 2019; Molinari et al., 2019). Our results also show increased cholecalciferol in the cortex of subacute TBI, which is related to neuroprotection exertion (Mechanick et al., 1997; Van de Kerkhof et al., 2002). Differently from previous studies (Tayag et al., 1996; Liu et al., 2018), our data indicate that the levels of uric acid (UA) are significantly lower in the cortex of TBI rats. UA is a major antioxidant in the central nervous system and/or a prediction that might support good clinical recovery following TBI (Dash et al., 2016). Therefore, uric acid may play its neuroprotective role for attenuating neurological deficits. Additionally, lower betaine, trigonelline, and palmitoylcarnitine levels are detected in our results. They can regulate cellular function (Dash et al., 2016), inflammation (Farkhondeh et al., 2018; Mallah et al., 2019), and energy metabolism disorder (Ojuka et al., 2016). To date, no studies have addressed the alterations of guanidinoethyl sulfonate, ecgonine, and 2′-deoxyinosinea during TBI. Herein our results provide evidence on perturbations in the cortical metabolites of subacute TBI.
In contrast to the cortex, palmitoylcarnitine and betaine change conversely in the brain region of the hippocampus in response to subacute TBI. The evidence in previous studies suggests that betaine treatment plays a vital role in synaptic plasticity associated with learning and memory (Kunisawa et al., 2017). An increase in palmitoylcarnitine plays an important role in the decrease of fatty acid beta oxidation at the acute time point, which is consistent with our study (Ojuka et al., 2016). This overlap results suggest that the same metabolite with brain-region-dependent functions causes different alterations in brain disease. Meantime, ascorbic acid performs cerebral antioxidant effects (Prieto et al., 2011). Hypotaurine produces taurine and may be involved in age-related cognitive GABAergic system alterations in taurine and hypotaurine metabolisms (Wu et al., 2008). The hypotaurine levels are elevated in response to hypoxia to exert antioxidant protection upon reperfusion (Sakuragawa et al., 2010; Liao et al., 2018), and propionylcarnitine function is reported to be the marker of oxidative stress in the hippocampal CA1 region 7 days after ischemia (Al-Majed et al., 2006). Adenine decreases in the periods of anoxia or ischemia in myocardial ATP (Esposito et al., 1999). Overall, our findings propose hippocampal regional variations that are relevant to synaptic plasticity dysregulation and antioxidant defenses in the subacute TBI brain.
Moreover, another finding that we observed in our present results is that seven subacute hippocampal metabolites, also identified in TBI when compared with our pervious study, were related to metabolic profiles during the acute phase (Zheng et al., 2020). Our results show that these hippocampal metabolites all increased in the subacute period of TBI except L-norleucine that keeps the same level in the subacute phase when compared with that in the acute phase of TBI. According to the analysis above, the increased levels of the six metabolites, along with the same levels of L-norleucine, further illustrate the sustainability of the biological dysregulation status in the rat brain with TBI compared to the sham subjects. The increased levels of six hippocampal metabolites may be explained by the higher variations relevant to synaptic plasticity dysregulation and antioxidant defenses from the acute phase to the subacute phase in TBI. This means that a link exists between the metabolic fluctuation in TBI and the biological progression in synaptic plasticity dysregulation and antioxidant defenses before post-period in TBI. In another perspective, there is a necessity to focus on synaptic plasticity and antioxidants in the acute hippocampal TBI.
In our study, metabolites enriched in purine metabolism pathway are identified in the cortex. Purine biology developments refer to the pathomechanisms of secondary injury and the therapies for brain injury (Chaikuad and Brady, 2009; Liu et al., 2018). Our study shows altered uric acid in this pathway, which is a waste product of purine metabolism (Johnson et al., 2009). In the subacute hippocampal TBI, taurine and hypotaurine metabolism is an enriched pathway in our study. Previous metabolomics studies have reported the involvement of taurine and hypotaurine metabolism in brain diseases such as Parkinson’s disease (Shao and Le, 2019). Taurine and hypotaurine metabolism is also correlated with inflammatory responses (Shui et al., 2018). Increasing evidence in this study suggests that the metabolic changes in the pathophysiology of the cortex and the hippocampus after subacute TBI are under different states.
Our database analysis shows an accumulation of 21 different proteins and two main pathways assigned in the cortex. There are 43 different proteins and three main pathways in the hippocampus. Purine nucleoside phosphorylase may form a key enzyme in the recycling of predominantly host-derived purines and produces the major purine precursor when catalyzing the phosphorolysis of inosine (Chaikuad and Brady, 2009). Adenosine deaminase (ADA) deficiency may have a deep impact on cellular physiology by elevating the levels of ADA substrates, including adenosine and deoxyadenosine (Dolezal et al., 2005). Uric acid is the final product of xanthine dehydrogenase as well as ADA in purine metabolism in humans (Yun et al., 2017). These protein maps may be used to identify the dysfunctional metabolic enzymes when considering purine metabolism disorder in subacute cortical TBI. The action of glutamate decarboxylases (GADs) such as GAD1 and GAD2 can synthesize gamma-aminobutyric acid, which is a neurotransmitter from amino acid glutamate (Grone and Maruska, 2016). Hypotaurine could be produced involving oxidative stress and membrane damage via 2-aminoethanethiol dioxygenase oxidation (Liu et al., 2017). Hypotaurine can be converted by cysteine sulfinic acid decarboxylase for taurine production (Wang et al., 2018). These novel results lead to new insights of effective diagnosis and treatment.
Our protein–disease network pattern, coupled with their interaction in pathways, shows that TBI may be regulated and predicted by proteins. Fatty acid synthesis is a multistep process involving several critical enzymes (Janßen and Steinbüchel, 2014). For example, CPT1 and CPT2 mediate palmitoylcarnitine or acetyl-CoA, which are the primary and the final products of fatty acid oxidation in the mitochondria (Kerner et al., 2014; Fontaine et al., 2018; Lin et al., 2018). These results provide a global view of the interactions between metabolites and connected proteins. The revelations of metabolite–protein interactions may help to guide the treatment of TBI.
Several limitations should be addressed. We only employed LC-MS/MS technology to detect the metabolic profiles. More metabolomics technologies such as gas chromatography–mass spectrometry and NMR are needed to confirm our findings. Additionally, the sample size was relatively small. Finally, the interactions of protein expression and their related metabolites should be further investigated to broaden their therapeutic and clinical potential.
In conclusion, our results represent significant profiling change and provide unique metabolite–protein information in a rat model of TBI following the subacute phase. This study may inspire scientists and doctors to further their studies and provide potential therapy targets for clinical interventions.
The datasets generated for this study are available on request to the corresponding author.
All procedures involving in the experiments were approved in compliance with regulations of Medical Ethics Committee at Xiangya Hospital of Central South University.
YW, WZ, and C-SD designed the study. FZ, Y-TZ, P-FL, EH, TL, TT, and J-KL performed the experiments. FZ and TL analyzed the data. FZ visualized the figures. FZ and YW drafted the manuscript. YW, WZ, and C-SD revised the manuscript. WZ and C-SD funded the study. All authors read and approved the final manuscript.
This work was supported by the National Natural Science Foundation of China (Nos. 81673719 and 61472126), the Hunan Provincial Natural Science Foundation of China (No. 2019JJ30042), the Outstanding Youth Fund of Hunan Natural Science (No. 2020JJ2024), and Natural Science Foundation of Hunan Province (No. 2018JJ2301).
The authors declare that the research was conducted in the absence of any commercial or financial relationships that could be construed as a potential conflict of interest.
The Supplementary Material for this article can be found online at: https://www.frontiersin.org/articles/10.3389/fnins.2020.00876/full#supplementary-material
Al-Majed, A. A., Sayed-Ahmed, M. M., Al-Omar, F. A., Al-Yahya, A. A., Aleisa, A. M., and Al-Shabanah, O. A. (2006). Carnitine esters prevent oxidative stress damage and energy depletion following transient forebrain ischaemia in the rat hippocampus. Clin. Exp. Pharmacol. Physiol. 33, 725–733. doi: 10.1111/j.1440-1681.2006.04425.x
Arulsamy, A., Corrigan, F., and Collins-Praino, L. E. (2019). Cognitive and neuropsychiatric impairments vary as a function of injury severity at 12 months post-experimental diffuse traumatic brain injury: implications for dementia development. Behav. Brain Res. 3, 66–76. doi: 10.1016/j.bbr.2019.02.045
Barkhoudarian, G., Hovda, D. A., and Giza, C. C. (2016). The molecularpath-ophysiology of concussive brain injury - an update. Phys. Med. Rehabil. Clin. North Am. 27, 373–393.
Berger, R. P., Beers, S. R., Richichi, R., Wiesman, D., and Adelson, P. D. (2007). Serum biomarker concentrations and outcome after pediatric traumatic brain injury. J. Neurotrauma 24, 1793–1801. doi: 10.1089/neu.2007.0316
Bivona, G., Agnello, L., and Ciaccio, M. (2018). The immunological implication of the new vitamin D metabolism. Central-Eur. J. Immunol. 43, 331–334. doi: 10.5114/ceji.2018.80053
Boone, D. K., Weisz, H. A., Bi, M., Falduto, M. T., Torres, K. E. O., Willey, H. E., et al. (2017). Evidence linking microRNA suppression of essential prosurvival genes with hippocampal cell death after traumatic brain injury. Sci. Rep. 7:6645.
Campbell, G. R., and Spector, S. A. (2012). Vitamin D inhibits human immunodeficiency virus type 1 and Mycobacterium tuberculosis infection in macrophages through the induction of autophagy. PLoS Pathogens 8:e1002689. doi: 10.1371/journal.ppat.1002689
Carron, S. F., Alwis, D. S., and Rajan, R. (2016). Traumatic brain injury and neuronal functionality changes in sensory cortex. Front. Sys. Neurosci. 10:47. doi: 10.3389/fnsys.2016.00047
Casey, P. A., McKenna, M. C., Fiskum, G., Saraswati, M., and Robertson, C. L. (2008). Early and sustained alterations in cerebral metabolism after traumatic brain injury in immature rats. J. Neurotrauma 25, 603–614. doi: 10.1089/neu.2007.0481
Chaikuad, A., and Brady, R. L. (2009). Conservation of structure and activity in Plasmodium purine nucleoside phosphorylases. BMC Struct. Biol. 9:42. doi: 10.1186/1472-6807-9-42
Chauhan, N. B. (2014). Chronic neurodegenerative consequences of traumatic brain injury. Restorat. Neurol. Neurosci. 32, 337–365. doi: 10.3233/rnn-130354
Chitturi, J., Li, Y., Santhakumar, V., and Kannurpatti, S. S. (2018). Early behavioral and metabolomic change after mild to moderate traumatic brain injury in the developing brain. Neurochem. Int. 120, 75–86. doi: 10.1016/j.neuint.2018.08.003
Cui, C., Cui, J., Jin, F., Cui, Y., Li, R., Jiang, X., et al. (2017). Induction of the Vitamin D receptor attenuates autophagy dysfunction-mediated cell death following traumatic brain injury. Cell. Physiol. Biochem. 42, 1888–1896. doi: 10.1159/000479571
Dash, P. K., Hergenroeder, G. W., Jeter, C. B., Choi, H. A., Kobori, N., and Moore, A. N. (2016). Traumatic brain injury alters methionine metabolism: implications for pathophysiology. Front. Sys. Neurosci. 29:36. doi: 10.3389/fnsys.2016.00036
Dickens, A. M., Posti, J. P., Takala, R. S. K., Ala-Seppälä, H., Mattila, I., Coles, J. P., et al. (2018). Serum metabolites associated with computed tomography findings after traumatic brain injury. J. Neurotrauma 35, 2673–2683. doi: 10.1089/neu.2017.5272
Dolezal, T., Dolezelova, E., Zurovec, M., and Bryant, P. J. (2005). A role for adenosine deaminase in drosophila larval development. PLoS Biol. 3:e201. doi: 10.1371/journal.pbio.0030201
Esposito, L. A., Melov, S., Panov, A., Cottrell, B. A., and Wallace, D. C. (1999). Mitochondrial disease in mouse results in increased oxidative stress. Proc. Natl. Acad. Sci. U.S.A. 96, 4820–4825. doi: 10.1073/pnas.96.9.4820
Faden, A. I. (2011). Microglial activation and traumatic brain injury. Ann. Neurol. 70, 345. doi: 10.1002/ana.22555
Farkhondeh, T., Samarghandian, S., Shahri, A. M. P., and Samini, F. (2018). The neuroprotective effects of thymoquinone: a review. Dose Response 16:1559325818761455.
Feala, J. D., Abdulhameed, M. D., Yu, C., Dutta, B., Yu, X., Schmid, K., et al. (2013). Systems biology approaches for discovering biomarkers for traumatic brain injury. J. Neurotrauma 30, 1101–1116.
Fontaine, M., Kim, I., Dessein, A. F., Mention-Mulliez, K., Dobbelaere, D., Douillard, C., et al. (2018). Fluxomic assay-assisted diagnosis orientation in a cohort of 11 patients with myopathic form of CPT2 deficiency. Mol. Genet. Metabol. 123, 441–448. doi: 10.1016/j.ymgme.2018.02.005
Girgis, F., Pace, J., Sweet, J., and Miller, J. P. (2016). Hippocampal neurophysiologic changes after mild traumatic brain injury and potential neuromodulation treatment approaches. Front. Sys. Neurosci. 10:8. doi: 10.3389/fnsys.2016.00008
Grone, B. P., and Maruska, K. P. (2016). Three distinct glutamate decarboxylase genes in vertebrates. Sci. Rep. 6:30507.
Han, S., Wang, X., He, Z., Sheng, W., Zou, Q., Li, L., et al. (2019). Decreased static and increased dynamic global signal topography in major depressive disorder. Prog. Neuro-Psychopharmacol. Biol. Psychiatry 13:109665. doi: 10.1016/j.pnpbp.2019.109665
Hanlon, L. A., Raghupathi, R., and Huh, J. W. (2019). Depletion of microglia immediately following traumatic brain injury in the pediatric rat: implications for cellular and behavioral pathology. Exp. Neurol. 316, 39–51. doi: 10.1016/j.expneurol.2019.04.004
Janßen, H. J., and Steinbüchel, A. (2014). Fatty acid synthesis in Escherichia coli and its applications towards the production of fatty acid based biofuels. Biotechnol. Biofuels 7:7. doi: 10.1186/1754-6834-7-7
Johnson, R. J., Sautin, Y. Y., Oliver, W. J., Roncal, C., Mu, W., and Gabriela et al., (2009). Lessons from comparative physiology: could uric acid represent a physiologic alarm signal gone awry in western society? J. Comp. Physiol. B. 179, 67–76. doi: 10.1007/s00360-008-0291-7
Kempuraj, D., Ahmed, M. E., Selvakumar, G. P., Thangavel, R., Dhaliwal, A. S., Dubova, I., et al. (2019). Brain injury-mediated neuroinflammatory response and Alzheimer’s disease.”. Neuroscientist 16:1073858419848293.
Kerner, J., Minkler, P. E., Lesnefsky, E. J., and Hoppel, C. L. (2014). Fatty acid chain elongation in palmitate-perfused working rat heart: mitochondrial acetyl-CoA is the source of two-carbon units for chain elongation. J. Biol. Chem. 289, 10223–10234. doi: 10.1074/jbc.m113.524314
Kunisawa, K., Kido, K., Nakashima, N., Matsukura, T., Nabeshima, T., and Hiramatsu, M. (2017). Betaine attenuates memory impairment after water-immersion restraint stress and is regulated by the GABAergic neuronal system in the hippocampus. Eur. J. Pharmacol. 5, 122–130. doi: 10.1016/j.ejphar.2016.12.007
Li, J., Xu, S., Zhu, J. B., Song, J., Luo, B., Song, Y. P., et al. (2019). Pretreatment with cholecalciferol alleviates renal cellular stress response during ischemia/reperfusion-induced acute kidney injury. Oxid. Med. Cell. Longev. 25:1897316.
Liao, W. T., Liu, J., Zhou, S. M., Xu, G., Gao, Y. Q., and Liu, W. Y. (2018). UHPLC-QTOFMS-based metabolomic analysis of the hippocampus in hypoxia preconditioned mouse. Front. Physiol. 9:1950. doi: 10.3389/fphys.2018.01950
Lichtman, J. W., Pfister, H., and Shavit, N. (2014). The big data challenges of connectomics. Nat. Neurosci. 17, 1448–1454. doi: 10.1038/nn.3837
Lieblein-Boff, J. C., Johnson, E. J., Kennedy, A. D., Lai, C. S., and Kuchan, M. J. (2015). Exploratory metabolomic analyses reveal compounds correlated with lutein concentration in frontal cortex. hippocampus, and occipital cortex of human infant brain. PLoS One 10:e0136904. doi: 10.1371/journal.pone.0136904
Lin, Z., Liu, F., Shi, P., Song, A., Huang, Z., Zou, D., et al. (2018). Fatty acid oxidation promotes reprogramming by enhancing oxidative phosphorylation and inhibiting protein kinase C. Stem Cell Res. Ther. 9:47.
Liu, C. L., Watson, A. M., Place, A. R., and Jagus, R. (2017). Taurine biosynthesis in a fish liver cell line (ZFL) adapted to a serum-free medium. Mar. Drugs 15:147. doi: 10.3390/md15060147
Liu, H., He, J., Zhong, J., Zhang, H., Zhang, Z., Liu, L., et al. (2018). Clinical and basic evaluation of the prognostic value of uric acid in traumatic brain injury. Int. J. Med. Sci. 15, 1072–1082. doi: 10.7150/ijms.25799
Lommen, A., van, der, Weg, G., van, Engelen, M. C., et al. (2007). An untargeted metabolomics approach to contaminant analysis: pinpointing potential unknown compounds. Anal. Chim. Acta 584, 43–49. doi: 10.1016/j.aca.2006.11.018
Maas, A. I., Steyerberg, E. W., Murray, G. D., Bullock, R., Baethmann, A., Marshall, L. F., et al. (1999). Why have recent trials of neuroprotective agents in head injury failed to show convincing efficacy? a pragmatic analysis and theoretical considerations. Neurosurgery 44, 1286–1298. doi: 10.1227/00006123-199906000-00076
Maas, A. I., Stocchetti, N., and Bullock, R. (2008). Moderate and severe traumatic brain injury in adults. Lancet Neurol. 7, 28–741.
Maas, A. I. R., Menon, D. K., Adelson, P. D., Andelic, N., Bell, M. J., Belli, A., et al. (2017). Traumatic brain injury: integrated approaches to improve prevention, clinical care, and research. Lancet Neurol. 16, 987–1048.
Mallah, K., Quanico, J., Raffo-Romero, A., Cardon, T., Aboulouard, S., Devos, D., et al. (2019). Mapping spatiotemporal microproteomics landscape in experimental model of traumatic brain injury unveils a link to Parkinson’s disease. Mol. Cell. Proteom. 18, 1669–1682. doi: 10.1074/mcp.ra119.001604
McGuire, J. L., DePasquale, E. A. K., Watanabe, M., Anwar, F., Ngwenya, L. B., Atluri, G., et al. (2019). Chronic dysregulation of cortical and subcortical metabolism after experimental traumatic brain injury. Mol. Neurobiol. 56, 2908–2921. doi: 10.1007/s12035-018-1276-5
Mechanick, J. I., Pomerantz, F., Flanagan, S., Stein, A., Gordon, W. A., and Ragnarsson, K. T. (1997). Parathyroid hormone suppression in spinal cord injury patients is associated with the degree of neurologic impairment and not the level of injury. Arch. Phys. Med. Rehabil. 78, 692–696. doi: 10.1016/s0003-9993(97)90075-7
Molinari, C., Morsanuto, V., Ghirlanda, S., Ruga, S., Notte, F., Gaetano, L., et al. (2019). Role of combined lipoic acid and vitamin d3 on astrocytes as a way to prevent brain ageing by induced oxidative stress and iron accumulation. Oxid. Med. Cell. Longev. 2019, 1–16. doi: 10.1155/2019/2843121
Needham, E. J., Helmy, A., Zanier, E. R., Jones, J. L., Coles, A. J., and Menon, D. K. (2019). The immunological response to traumatic brain injury. J. Neuroimmunol. 15, 112–125.
Ojuka, E., Andrew, B., Bezuidenhout, N., George, S., Maarman, G., Madlala, H. P., et al. (2016). Measurement of β-oxidation capacity of biological samples by respirometry: a review of principles and substrates. Am. J. Physiol. Endocrinol. Metab. 310, E715–E723.
Pierce, J. E., Smith, D. H., Trojanowski, J. Q., and McIntosh, T. K. (1998). Enduring cognitive, neurobehavioral and histopathological changes persist for up to one year following severe experimental brain injury in rats. Neuroscience 87, 359–369. doi: 10.1016/s0306-4522(98)00142-0
Prieto, R., Tavazzi, B., Taya, K., Barrios, L., Amorini, A. M., and Di et al., (2011). Brain energy depletion in a rodent model of diffuse traumatic brain injury is not prevented with administration of sodium lactate. Brain Res. 2, 39–49. doi: 10.1016/j.brainres.2011.06.006
Sakuragawa, T., Hishiki, T., Ueno, Y., Ikeda, S., Soga, T., Yachie-Kinoshita, A., et al. (2010). Hypotaurine is an energy-saving hepatoprotective compound against ischemia-reperfusion injury of the rat liver. J. Clin. Biochem. Nutr. 46, 126–134. doi: 10.3164/jcbn.09-91
Sapiurka, M., Squire, L. R., and Clark, R. E. (2016). Distinct roles of hippocampus and medial prefrontal cortex in spatial and nonspatial memory. Hippocampus 26, 1515–1524. doi: 10.1002/hipo.22652
Shao, Y., and Le, W. (2019). Recent advances and perspectives of metabolomics-based investigations in Parkinson’s disease. Mol. Neurodegener. 14:3.
Sharma, R., Shultz, S. R., Robinson, M. J., Belli, A., Hibbs, M. L., O’Brien, T. J., et al. (2019). Infections after a traumatic brain injury: the complex interplay between the immune and neurological systems. Brain Behav. Immun. 79, 63–74. doi: 10.1016/j.bbi.2019.04.034
Shui, S., Cai, X., Huang, R., Xiao, B., and Yang, J. (2018). The investigation of anti-inflammatory activity of Yi Guanjian decoction by serum metabonomics approach. J. Pharm. Biomed. Anal. 156, 221–231.
Tayag, E. C., Nair, S. N., Wahhab, S., Katsetos, C. D., Lighthall, J. W., and Lehmann, J. C. (1996). Cerebral uric acid increases following experimental traumatic brain injury in rat. Brain Res. 733, 287–291. doi: 10.1016/0006-8993(96)00669-5
Terranova, J. I., Ogawa, S. K., and Kitamura, T. (2019). Adult hippocampal neurogenesis for systems consolidation of memory. Behav. Brain Res. 372:112035. doi: 10.1016/j.bbr.2019.112035
Turner, R. C., Lucke-Wold, B. P., Robson, M. J., Omalu, B. I., Petraglia, A. L., and Bailes, J. E. (2013). Repetitive traumatic brain injury and development of chronic traumatic encephalopathy: a potential role for biomarkers in diagnosis, prognosis, and treatment? Front. Neurol. 3:186. doi: 10.3389/fneur.2012.00186
Van de Kerkhof, P. C., Berth-Jones, J., Griffiths, C. E., Harrison, P. V., Hönigsmann, H., Marks, R., et al. (2002). Long-term efficacy and safety of tacalcitol ointment in patients with chronic plaque psoriasis. Br. J. Dermatol. 146, 414–422. doi: 10.1046/j.1365-2133.2002.04567.x
Vasilopoulou, C. G., Margarity, M., and Klapa, M. I. (2016). Metabolomic analysis in brain research: opportunities and challenges. Front. Physiol. 7:183. doi: 10.3389/fphys.2016.00183
Viant, M. R., Lyeth, B. G., Miller, M. G., and Berman, R. F. (2005). An NMR metabolomic investigation of early metabolic disturbances following traumatic brain injury in a mammalian model. NMR Biomed. 18, 507–516. doi: 10.1002/nbm.980
Vijayakumar, N., Pfeifer, J. H., Flournoy, J. C., Hernandez, L. M., and Dapretto, M. (2019). Affective reactivity during adolescence: associations with age, puberty and testosterone. Cortex 117, 336–350. doi: 10.1016/j.cortex.2019.04.024
Wang, Y., Matye, D., Nguyen, N., Zhang, Y., and Li, T. (2018). HNF4α Regulates CSAD to couple hepatic taurine production to bile acid synthesis in mice. Gene Exp. 18, 187–196. doi: 10.3727/105221618x15277685544442
Werner, C., and Engelhard, K. (2007). Pathophysiology of traumatic brain injury. Br. J. Anaesth. 99, 4–9.
Wolahan, S. M., Hirt, D., and Glenn, T. C. (2015). “Translational metabolomics of head injury: exploring dysfunctional cerebral metabolism with ex vivo nmr spectroscopy-based metabolite quantification,” in Brain Neurotrauma: Molecular, Neuropsychological, and Rehabilitation Aspects Frontiers in Neuroengineering, ed F. H. Kobeissy (Boca Raton, FL: CRC Press).
Wu, J., Kohno, T., Georgiev, S. K., Ikoma, M., Ishii, H., Petrenko, A. B., et al. (2008). Taurine activates glycine and γ-aminobutyric acid a receptors in rat substantia gelatinosa neurons. Neuroreport 19, 333–337. doi: 10.1097/wnr.0b013e3282f50c90
Xing, Z., Xia, Z., Peng, W., Li, J., Zhang, C., Fu, C., et al. (2016). Xuefu Zhuyu decoction, a traditional Chinese medicine, provides neuroprotection in a rat model of traumatic brain injury via an anti-inflammatory pathway. Sci. Rep. 6:20040.
Yang, T., Bavley, R. L., Fomalont, K., Blomstrom, K. J., Mitz, A. R., Turchi, J., et al. (2014). Contributions of the hippocampus and entorhinal cortex to rapid visuomotor learning in rhesus monkeys. Hippocampus 24, 1102–1111. doi: 10.1002/hipo.22294
Yi, L., Shi, S., Wang, Y., Huang, W., Xia, Z. A., Xing, Z., et al. (2016). Serum metabolic profiling reveals altered metabolic pathways in patients with post-traumatic cognitive impairments. Sci. Rep. 17:21320.
Yin, Y., Li, E., Sun, G., Yan, H. Q., Foley, L. M., Andrzejczuk, L. A., et al. (2018). Effects of DHA on hippocampal autophagy and lysosome function after traumatic brain injury. Mol. Neurobiol. 55, 2454–2470. doi: 10.1007/s12035-017-0504-8
Yun, Y., Yin, H., Gao, Z., Li, Y., Gao, T., Duan, J., et al. (2017). Intestinal tract is an important organ for lowering serum uric acid in rats. PLoS One. 12:e0190194. doi: 10.1371/journal.pone.0190194
Zetterberg, H., Smith, D. H., and Blennow, K. (2013). Biomarkers of mild traumatic brain injury in cerebrospinal fluid and blood. Nat. Rev. Neurol. 9, 201–210. doi: 10.1038/nrneurol.2013.9
Zhang, Z., Larner, S. F., Kobeissy, F., Hayes, R. L., and Wang, K. K. (2010). Systems biology and theranostic approach to drug discovery and development to treat traumatic brain injury. Methods Mol. Biol. 662, 317–329. doi: 10.1007/978-1-60761-800-3_16
Keywords: traumatic brain injury, hippocampus and cortex, metabolomics, LC-MS/MS, subacute phase
Citation: Zheng F, Zhou Y-T, Li P-F, Hu E, Li T, Tang T, Luo J-K, Zhang W, Ding C-S and Wang Y (2020) Metabolomics Analysis of Hippocampus and Cortex in a Rat Model of Traumatic Brain Injury in the Subacute Phase. Front. Neurosci. 14:876. doi: 10.3389/fnins.2020.00876
Received: 02 February 2020; Accepted: 28 July 2020;
Published: 04 September 2020.
Edited by:
Firas H. Kobeissy, University of Florida, United StatesReviewed by:
Sheng Wei, Shandong University of Traditional Chinese Medicine, ChinaCopyright © 2020 Zheng, Zhou, Li, Hu, Li, Tang, Luo, Zhang, Ding and Wang. This is an open-access article distributed under the terms of the Creative Commons Attribution License (CC BY). The use, distribution or reproduction in other forums is permitted, provided the original author(s) and the copyright owner(s) are credited and that the original publication in this journal is cited, in accordance with accepted academic practice. No use, distribution or reproduction is permitted which does not comply with these terms.
*Correspondence: Wei Zhang, emhhbmd3ZWkxOTc5QGhudWNtLmVkdS5jbg==; Chang-Song Ding, ZGluZ2NzMTk3NUBobnVjbS5lZHUuY24=; Yang Wang, d2FuZ3lhbmdfeHk4N0Bjc3UuZWR1LmNu
†These authors have contributed equally to this work
Disclaimer: All claims expressed in this article are solely those of the authors and do not necessarily represent those of their affiliated organizations, or those of the publisher, the editors and the reviewers. Any product that may be evaluated in this article or claim that may be made by its manufacturer is not guaranteed or endorsed by the publisher.
Research integrity at Frontiers
Learn more about the work of our research integrity team to safeguard the quality of each article we publish.