- 1Graduate Program in Neuroscience, University of Washington, Seattle, WA, United States
- 2Center for Neurobiology of Addiction, Pain, and Emotion, University of Washington, Seattle, WA, United States
- 3Department of Psychiatry and Behavioral Sciences, University of Washington, Seattle, WA, United States
- 4Center for Integrative Brain Research, Seattle Children’s Research Institute, Seattle, WA, United States
- 5Alcohol and Drug Abuse Institute, University of Washington, Seattle, WA, United States
Substance use disorder (SUD) is a chronic, relapsing disease with a highly multifaceted pathology that includes (but is not limited to) sensitivity to drug-associated cues, negative affect, and motivation to maintain drug consumption. SUDs are highly prevalent, with 35 million people meeting criteria for SUD. While drug use and addiction are highly studied, most investigations of SUDs examine drug use in isolation, rather than in the more prevalent context of comorbid substance histories. Indeed, 11.3% of individuals diagnosed with a SUD have concurrent alcohol and illicit drug use disorders. Furthermore, having a SUD with one substance increases susceptibility to developing dependence on additional substances. For example, the increased risk of developing heroin dependence is twofold for alcohol misusers, threefold for cannabis users, 15-fold for cocaine users, and 40-fold for prescription misusers. Given the prevalence and risk associated with polysubstance use and current public health crises, examining these disorders through the lens of co-use is essential for translatability and improved treatment efficacy. The escalating economic and social costs and continued rise in drug use has spurred interest in developing preclinical models that effectively model this phenomenon. Here, we review the current state of the field in understanding the behavioral and neural circuitry in the context of co-use with common pairings of alcohol, nicotine, cannabis, and other addictive substances. Moreover, we outline key considerations when developing polysubstance models, including challenges to developing preclinical models to provide insights and improve treatment outcomes.
Introduction
Drug addiction is a heterogeneous disorder characterized by cyclic periods of drug use, withdrawal and abstinence, and drug-craving and recurrence of use (Koob and Volkow, 2016). Addiction is highly prevalent in our society, with an estimated 35 million people world-wide and 19.3 million people in the United States (US) currently meeting diagnostic criteria for a substance use disorder (SUD) (Figure 1A; Substance Abuse and Mental Health Services Administration, 2019; United Nations Office on Drugs and Crime, 2019). Additionally, epidemiological surveys suggest that, in a person’s lifetime, there is a ∼10% prevalence of a SUD (Grant et al., 2016; Substance Abuse and Mental Health Services Administration, 2019). Drug addiction is also one of the largest public health problems in the US, with an annual financial burden of $740 billion in costs related to treatment, lost work productivity, healthcare, and crime (National Institute on Drug Abuse, 2020). These numbers are likely to increase as illicit drug use is rising, with a quarter of a billion people worldwide reporting use in the past year (United Nations Office on Drugs and Crime, 2019). Within the US, over 17 million people aged 12 and above are estimated to initiate drug use annually. Rates of opioid use, in particular, are continuing to climb, with 53 million past-year opioid users worldwide and ∼11 million people in the US reporting opioid misuse within the past year (United Nations Office on Drugs and Crime, 2019). This is especially alarming, as the number of deaths in the US involving opioids has increased 6-fold from 1999 to 2017, with ∼130 Americans dying from use per day (Centers for Disease Control, 2018).
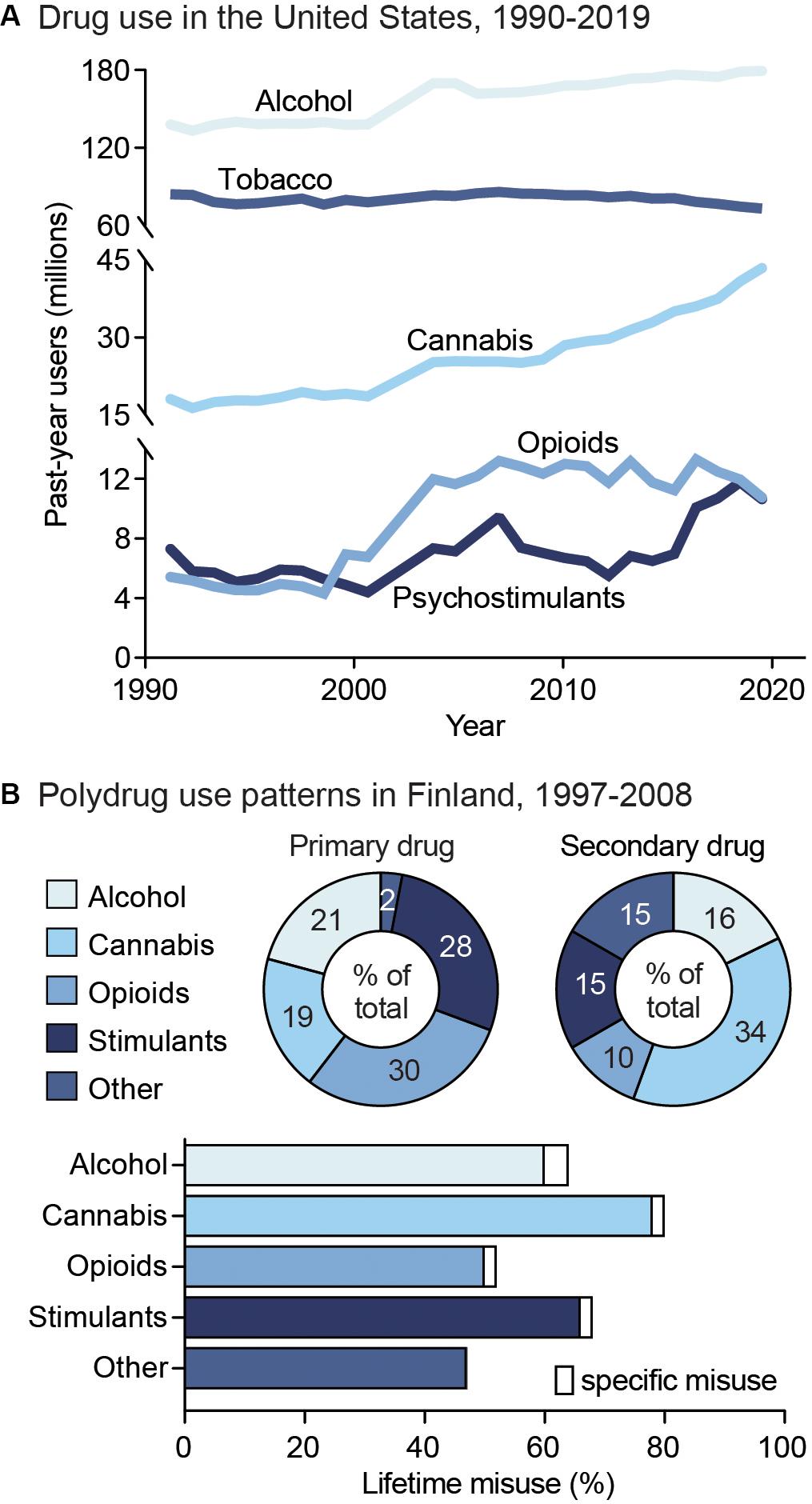
Figure 1. Public health trends in drug use. (A) Drug use in the United States from 1990 to 2019. Data from the National Household Survey on Drug Abuse (Substance Abuse and Mental Health Services Administration, 1993; Substance Abuse and Mental Health Services Administration, 1995; Substance Abuse and Mental Health Services Administration, 1997; Substance Abuse and Mental Health Services Administration, 2003) and the National Survey on Drug Use and Health (Substance Abuse and Mental Health Services Administration, 2005; Substance Abuse and Mental Health Services Administration, 2007; Substance Abuse and Mental Health Services Administration, 2009; Substance Abuse and Mental Health Services Administration, 2011; Substance Abuse and Mental Health Services Administration, 2013; Substance Abuse and Mental Health Services Administration, 2015; Substance Abuse and Mental Health Services Administration, 2017; Substance Abuse and Mental Health Services Administration, 2019). (B) Unspecified polysubstance use in treatment-seeking drug users in Finland from 1997 to 2008. Top: Primary (left) and secondary (right) drugs used by treatment-seeking drug users, shown as percent of total users. Bottom: Percent of users reporting exclusive misuse of one drug (white bars) or misuse of a given drug along with polysubstance use of another (colored bars). Data from Onyeka et al. (2012).
Although the majority of research on SUDs has focused on individual substances in isolation, with a multiple drug use history often considered an exclusion criterion for clinical studies, it is important to recognize that many drug users engage in polysubstance use. For instance, 30–80% of heroin users have been reported to also use cocaine (Leri et al., 2003a), and deaths involving both cocaine and opioids in the US more than doubled between 2010 and 2015 (Centers for Disease Control, 2018). A person is considered a polysubstance user if they use more than one substance, including use of multiple drugs on separate occasions (sequential use) or at the same time (concurrent/simultaneous). Limiting studies to individual drugs risks overlooking interactions between substances, decreases translatability of preclinical research, and can impede the efficacy of identified treatments for SUDs. Indeed, polysubstance use has consistently been associated with worse treatment outcomes, including poorer treatment retention, higher rates of relapse, and a three-fold higher mortality rate compared to mono-substance use (Williamson et al., 2006; Staiger et al., 2013; de la Fuente et al., 2014). This review seeks to combine the current knowledge of the mechanisms and consequences of individual drug use with the most up-to-date research on polysubstance use, making sure to note, when possible, if polysubstance use is concurrent/simultaneous, sequential, or a combination of these patterns. We will first provide an overview of public health trends regarding single and polysubstance use, as well as the impact of polysubstance history on metrics of substance use severity. This will be followed by a discussion of findings from preclinical studies, and their translatability to real-world substance use, outlining considerations to be made when designing polysubstance studies. We then detail the pharmacology of individual substances and some of their effects on the cortical-basal ganglia-thalamic (C-BG-T) circuitry, which sets the groundwork for understanding how polysubstance use may change the neuropathology of addiction. Care will be given to discussing the differences between brain alterations in single versus polysubstance use, highlighting the most common combinations of polysubstance use. For clarity and in order to avoid duplication in our discussions, sections are organized by primary used substance, with consideration for the consequences that result when additional drugs are combined with a primary drug. Finally, we offer suggestions and highlight potential methods to move forward with the important task of examining polysubstance disorders.
Public Health Trends in Substance Use
Drug addiction is both pervasive and deadly, with ∼585,000 drug use-related deaths occurring each year worldwide (United Nations Office on Drugs and Crime, 2019). Nonetheless, although drug addiction and its impacts are often centered around individual drugs, drug misuse is largely found to involve multiple substances (Gjersing et al., 2013; Roy et al., 2013; Substance Abuse and Mental Health Services Administration, 2016). Indeed, drug-dependent individuals report an average use of 3.5 substances (Onyeka et al., 2012), including both simultaneous and sequential polydrug use (Figure 1B). In addition, the likelihood of developing comorbid substance dependencies is high in clinical populations (Leri et al., 2004; Lorvick et al., 2018). Although combinations of co-used substances vary, primary drug dependencies are typically found for alcohol, opioids, amphetamine, and methamphetamine, while cannabis and cocaine are more often reported as secondary-or tertiary-used substances (Substance Abuse and Mental Health Services Administration, 2016). The high prevalence of polysubstance use is particularly concerning given the impact it can have on both SUD severity and treatment outcomes. For example, a polysubstance history is associated with greater unmet physical and mental health care needs, increased risk behavior, violence, and increased overdose and mortality risk compared to single substance use (Pennings et al., 2002; Gilmore et al., 2018; Lorvick et al., 2018). In this section, we will discuss the public health consequences surrounding single substance use, as well as polysubstance use in relation to secondary substance combinations. This overview will aim to address overarching patterns of polydrug use, including the substance combinations and patterns of use that most commonly occur. However, given that data is limited for specific drug use patterns, types of classification, and differences in definition of polydrug combinations across studies, it is unlikely to capture all combinations, histories, and patterns of use.
Psychostimulants
Psychostimulants are the second-most widely used class of drugs, with 18 million current cocaine users and 29 million current prescription stimulant users worldwide (United Nations Office on Drugs and Crime, 2019). Worldwide prevalence of psychostimulant use has remained relatively stable from 1990 to 2017, with 7.38 million reported to meet criteria for an amphetamine use disorder and 5.02 million reported to meet criteria for a cocaine use disorder (Degenhardt et al., 2018). However, the number of drug-related overdose deaths involving psychostimulants has continued to climb, especially in the US, with a 2.6-fold increase in the cocaine overdose death rate and 3.6-fold increase in the methamphetamine overdose death rate from 2000 to 2017 (Degenhardt et al., 2018).
Notably, cocaine and amphetamine users are predominantly polysubstance users, with one study reporting 74 and 80% incidence of polysubstance history, respectively (Kedia et al., 2007). Specifically, cocaine use and developing a cocaine use disorder is associated with concurrent heroin, cannabis, tobacco, and alcohol use (Kedia et al., 2007; Roy et al., 2013; John and Wu, 2017). Similarly, amphetamine users exhibit several types of polysubstance use, with high probabilities of alcohol, tobacco, and cannabis use. In addition, other classes of amphetamine polysubstance users exhibit higher probabilities of heroin and other opioid use. Across groups, lower probabilities of cocaine use with amphetamine compared to other drug classes used with amphetamine are reported as well (Darke and Hall, 1995; Kelly et al., 2017). Polysubstance use is common among stimulant users with both concurrent and sequential drug consumption patterns. For instance, simultaneous use of psychostimulants and opioids is seen with both cocaine (“speedball”) and methamphetamine (“bombita”). Sequential use of psychostimulants and opioids is also common, including the use of cocaine or amphetamine to avoid opioid-related somatic withdrawal symptoms (Hunt et al., 1984; Ellis et al., 2018) and the use of opioids to reduce overexcitation following cocaine use (Kreek, 1997). Additionally, there is an increased likelihood of same-day methamphetamine use with alcohol consumption (Bujarski et al., 2014).
Though these studies did not specify the patterns of polydrug use, a meta-analysis of reports on concurrent versus simultaneous cocaine use found a 24–98% range of simultaneous cocaine and alcohol use and 12–76% incidence of simultaneous cannabis use (Liu et al., 2018). Rates of concurrent use were 37–96% for cocaine and alcohol use, 43–94% for cocaine and cannabis use (Liu et al., 2018), 70–80% for cocaine and nicotine use (Budney et al., 1993; Weinberger and Sofuoglu, 2009), and 85–95% for amphetamine and nicotine use (Brecht et al., 2007; Grant et al., 2007). The high variability in reported frequencies highlights the complexity in identifying drug use patterns, which can vary across demographics, study periods, study structure, and definitions of concurrent and simultaneous use.
Polydrug use involving psychostimulants poses significant public health risks. For example, one study showed that amphetamine users were 21 times more likely to have a concurrent cannabis use disorder and 7 times more likely to have past-year concurrent cocaine use, compared to those with no prior history of amphetamine use (Massaro et al., 2017). In addition, nearly one-third of overdose deaths involved both psychostimulants and opioids, such as heroin and fentanyl (Kariisa et al., 2019). The hazards of psychostimulant co-use also extend to other substances, with combined cocaine and cannabis use resulting in higher standardized death rates in emergency department (ED) visits, suggesting elevated mortality risks with this combination (Gilmore et al., 2018). Additionally, combining cocaine and alcohol use increases the risk for cardiotoxicity compared to either drug alone (Pennings et al., 2002).
Nicotine
Although the use of tobacco (i.e. the dried leaves of the tobacco plant containing nicotine) has declined since the early 2000s, nicotine is still one of the most commonly used drugs, with 58.8 million people aged 12 or above reporting past-month nicotine use in the US (Substance Abuse and Mental Health Services Administration, 2019). It is also commonly used with many other substances, as 17% of nicotine users also used cannabis, 4.7% also used opioids, 2.6% also used cocaine, and 1.4% also used psychostimulants in the past month. In contrast, nonsmokers had much lower percentages of past-month substance use (3.7, 1.2, 0.2, and 0.3% for the aforementioned substances, respectively), (Moeller et al., 2018). This difference is notable, given that people with a nicotine use disorder are 3–4 times more likely to have a second SUD (Chou et al., 2016). In addition, it was found that past-year tobacco use was significantly associated with opioid use disorders, as well as comorbidities for cannabis and alcohol use and use disorders, and cocaine use in samples of primary care patients (John et al., 2019). Tobacco use severity (i.e. frequency of use and number of cigarettes smoked) has also been significantly correlated with onset of heroin and cocaine use (Frosch et al., 2000). Historically, nicotine has primaryily been used by smoking tobacco cigarettes. However, new advances in technology have led to the development of electronic (e-) cigarettes, designed to deliver nicotine in a toxin-free manner. The marketing of e-cigarettes as a safer alternative to traditional tobacco cigarettes is concerning, as it has led to an increase in the probability of nicotine use and a resurgence in the potential for nicotine addiction. For example, e-cigarette use among middle and high school students has increased from 2012 to 2016 (Gentzke et al., 2019), and a spike in use was observed among young adults (18–24 years old) around 2013 to 2014, when e-cigarette products were introduced (Gentzke et al., 2019). Despite delivery of lower doses of nicotine, the safety of commercial e-cigarettes has been debated, since compensatory “puffing” behaviors or high voltage settings leads to the production of carcinogenic agents (Jensen et al., 2015). The potential danger of use is further compounded by the variable amounts of nicotine provided across e-cigarette manufacturers (Goniewicz et al., 2013). The unique influence of vaping on the development of nicotine dependence and how this differentially contributes to polysubstance use disorders remains largely unknown and should be studied in the coming years.
Opioids
The prevalence of opioid misuse (i.e. use outside of prescribed use) has risen dramatically in recent years, with ∼53 million adults (1.1% of global population) reporting past-year non-medical use of an opioid (United Nations Office on Drugs and Crime, 2019). In the US alone, 11 million people reported past-year opioid misuse in 2016 (Substance Abuse and Mental Health Services Administration, 2017); however, this estimate is conservative as it does not include homeless or incarcerated individuals with disproportionately higher levels of opioid use. In addition, the rate of first-time heroin users rose in parallel with non-medical use of prescription opioids from 2002 to 2011 (United Nations Office on Drugs and Crime, 2019), a reflection that suggests individuals with past-year prescription opioid misuse are 19 times more likely to initiate heroin use than those without such a history (Muhuri et al., 2013; Cicero et al., 2018). Studies have investigated polydrug use among heroin and prescription opioid misusers and found higher frequencies of opioid use in people that also use cocaine (>33%) or methamphetamine (>20%) (Wang et al., 2017; Hedegaard et al., 2018), but reduced prevelance for primary opioid use in those that have secondary alcohol or cannabis use (Wang et al., 2017; but see Cicero et al., 2020). In addition, first-time methamphetamine use is more prevalent following past-month opioid use (Cicero et al., 2020). Of those entering treatment for heroin use, it has been found that 91% of people reported a lifetime history of cocaine use (Williamson et al., 2006). Additionally, a study in the United Kingdom found that 54% of opioid users in treatment between 2017 and 2018 also had a comorbid crack cocaine use disorder (Public Health England, 2018). With respect to patterns of multi-drug use, simultaneous use of heroin with alcohol and/or cannabis is more common than with psychostimulants (Kelly et al., 2017; Bobashev et al., 2018), and a sequential pattern of drug use is preferred for opioids and psychostimulants.
In the US, opioid use is a national public health emergency responsible for more than 1.6 million years of life lost from 2001 to 2016 (Gomes et al., 2018). Moreover, opioid overdose deaths are currently the leading cause of accidental death among US adults, with 68% of all drug overdose deaths involving an opioid (United Nations Office on Drugs and Crime, 2019). Given that nearly 80% of fatal opioid overdoses also involved another substance, it appears that there is a greater risk of death when opioids are used in combination with other opioids and/or other drugs (Jones et al., 2018). Specifically, of these deaths, 78% involved another opioid, 21.6% involved cocaine, 11.1% involved alcohol, and 5.4% involved a psychostimulant other than cocaine (Jones et al., 2018). Furthermore, opioid-related ED visits also involved tobacco (51.1%), cocaine (36.9%), other stimulants (22.6%), cannabis (25.1%), or alcohol (16.9%). Substantial polysubstance use of three or more of these substances has also been reported for opioid-related ED visits (Liu and Vivolo-Kantor, 2020), and the likelihood of these visits has been associated with the degree of severity of other SUDs (Zale et al., 2015; John et al., 2019). Taken together, these reports suggest that combining opioid use with use of other substances can exacerbate the deleterious consequences of opioid use. In addition to overdose risk, opioid users experience very high rates of relapse, with 59% of individuals relapsing in the first week and 80% relapsing in the first month of abstinence (Smyth et al., 2010). Past use of other substances, including the degree of cocaine use, increases relapse susceptibility (Williamson et al., 2006). Methamphetamine use among those seeking treatment for opioid use has also been on the rise (United Nations Office on Drugs and Crime, 2019), and recent reports indicate that methamphetamine use is associated with a discontinuation of buprenorphine treatment in people with an opioid use disorder (Tsui et al., 2020). Thus, a better understanding of the impact of polysubstance use in the context of opioids is crucial for more successful emergency responses and long-term treatment outcomes.
Cannabinoids
It is estimated that 188 million individuals 12 years or older use cannabis worldwide (United Nations Office on Drugs and Crime, 2019), including 43.5 million individuals in the US (Substance Abuse and Mental Health Services Administration, 2019). Beginning in 2012 with Washington and Colorado, 11 states and the District of Columbia have legalized recreational cannabis, making it legally accessible to ∼328 million people. The number of cannabis users in the US has risen with its gradual decriminalization and legalization, from 4.1% in 2002, to 9.9% in 2007, to 15.9% in 2018 (Hasin et al., 2015; Substance Abuse and Mental Health Services Administration, 2019). Frequency of cannabis use is also high, with reports of 40% of individuals being daily or near-daily users (Substance Abuse and Mental Health Services Administration, 2019).
Cannabis users are reported to have high rates of past month tobacco, alcohol, and/or amphetamine use (Connor et al., 2013). One of the most common combinations is simultaneous use of alcohol and cannabis (McCabe et al., 2006), along with simultaneous alcohol, cocaine, and cannabis use (Liu et al., 2018). The impact of concurrent cannabis is notable, as this pattern of use is associated with more alcoholic drinks per day, suggesting facilitation of alcohol use with coexisting cannabis consumption (Aharonovich et al., 2005; Subbaraman et al., 2017). Polydrug use is particularly prevalent in younger populations. Among adolescent cannabis users, 27.5% reported additional drug use within the same year of starting cannabis use, and nearly 67% use two or more other drugs (Subbaraman and Kerr, 2015). Cannabis is frequently used during treatment for other SUDs (Connor et al., 2013; Subbaraman et al., 2017), and this has been associated with reduced treatment efficacy. For example, cannabis use has been found to result in shorter periods of alcohol abstinence (Subbaraman et al., 2017), as well as greater incidence of relapse to cocaine (Aharonovich et al., 2005; Mojarrad et al., 2014). In addition, polydrug use among cannabis users has been correlated with reduced socioeconomic mobility, financial instability, and relationship difficulties (Aharonovich et al., 2005; Cerdá et al., 2016; Subbaraman et al., 2017), a heightened degree of mood disorder symptom severity, decision-making deficits, social difficulties, and self-harm (Subbaraman and Kerr, 2015; Lopez-Quintero et al., 2018). Although these data suggest that the consequences of drug use are enhanced by concurrent cannabis use, it should be noted that clinical outcomes can vary for studies examining polydrug use among cannabis users. For example, some studies suggest a nuanced impact of polysubstance use that is dose-dependent, with no synergistic effects of cannabis and alcohol at low doses of either drug (Ballard and De Wit, 2011), and a lack of association of cannabis use in heroin relapse (Aharonovich et al., 2005).
Alcohol
Alcohol is one of the most commonly used drugs, with up to 290 million people diagnosed with an alcohol use disorder worldwide (United Nations Office on Drugs and Crime, 2019), including 15 million people in the US (Substance Abuse and Mental Health Services Administration, 2019). Alcohol is frequently used with other substances, with reports indicating that 5.6% of US adults have used both alcohol and another illicit drug within the past year, and 1.1% have met diagnostic criteria for both an alcohol use disorder and another SUD (Falk et al., 2006). The most commonly reported substance co-used with alcohol is cannabis (10%), with less common comorbidities found with opioids (2.4%), cocaine (2.5%), and amphetamine (1.2%) (Falk et al., 2006). Although simultaneous use of alcohol and cannabis or alcohol and prescription opioids is most common (McCabe et al., 2006), simultaneous use is also seen with cocaine (Liu et al., 2018).
Polydrug use increases the risk of developing an alcohol use disorder (Grant et al., 2015, 2016), particularly in young adults, men, and American Indians/Alaskan Natives (Falk et al., 2006). Polydrug use that includes alcohol is associated with additional comorbidities, including higher prevalence of mood disorders, anxiety disorders, more intense drinking, and more intense drug consumption and drug-craving (Preston et al., 2016; Saha et al., 2018). The negative consequences of alcohol polydrug use are also highlighted by data indicating that 21% of ED visits for patients 12–24 years old involved both alcohol and drugs. These visits were also more likely to require treatment for injuries, and had higher rates of inpatient admittance (Naeger, 2017). In addition, 17% of substance treatment admissions were related to both alcohol and drug use, representing 45% of primary alcohol admissions and 33% of drug misuse admissions (White et al., 2011; Substance Abuse and Mental Health Services Administration, 2016; Naeger, 2017). The rate of hospitalizations involving alcohol polydrug use has been increasing, particularly in young adults, with reports suggesting a 76% rise in inpatient admittance between 1998 and 2008, compared to either drug or alcohol overdoses alone (White et al., 2011). While the polysubstance users in these surveys were primarily white and male, recent trends indicate a rise in ED visits relating to alcohol and drug combinations in females (Naeger, 2017), suggesting a change in the demographics of polysubstance combinations that include alcohol.
Behavioral Models of Addiction in Polydrug Studies
Behavioral models of drug addiction are used to examine the neurobiological underpinnings of the development, maintenance and relapse to drug use. The most commonly used models are locomotor sensitization (a progressive and persistent increase in locomotor responses to the same dose of a drug), conditioned place preference (CPP; a test of drug reward measured as an increase in time spent in a drug-paired chamber) and drug self-administration (response-contingent intake of drug) (see Panlilio and Goldberg, 2007; Spanagel, 2017; Kuhn et al., 2019 for review). Experimental designs using these models vary across a number of pharmacological and non-pharmacological parameters including contingency of drug use, amount of access to drug, context associated with drug use, and routes of administration. Here, we describe how these models have been used with polydrug combinations, and how this work has informed our understanding of polydrug use and addiction.
Initial polysubstance studies largely used noncontingent models of drug administration, particularly CPP and cross-sensitization models, whereby the impact of priming doses of one drug on side preference or motor activity, respectively, of another drug are determined (Shippenberg et al., 1998; Lu et al., 2002; Cole et al., 2003; Leri et al., 2003b; Liang et al., 2006). More recently, studies have been examining how drug self-administration history impacts subsequent drug choice preference and/or drug-craving via responding to drug-associated cues following extinction and/or withdrawal (Leri and Stewart, 2001; De Luca et al., 2019; Rubio et al., 2019; Crummy et al., 2020). Preclinical polysubstance models involving simultaneous administration of multiple drugs, such as alcohol and nicotine or cocaine and heroin combinations, have also been used frequently (Mello and Newman, 2011; Mello et al., 2013; Sentir et al., 2020). In clinical models, both concurrent and sequential polysubstance use is assessed in subjects via scoring of affective measures to drug-taking, drug-craving following visual cues, and autonomic response measures such as blood pressure and heart rate (Foltin et al., 1993; Greenwald et al., 2010; Giasson-Gariépy et al., 2017).
More recently, studies are comparing single versus polysubstance self-administration to determine the effect of drug history on drug-induced molecular and circuit alterations (Briggs et al., 2018; Stennett et al., 2020; Zhu et al., 2020). Additionally, paradigms based on behavioral economic principles can determine the preferred level of drug intake (i.e. no-cost intake; Q0), as well as the amount of effort an animal is willing to exert to defend Q0 before consumption and responding begins to decline (i.e. price; Pmax) (Oleson and Roberts, 2009). These paradigms are especially powerful in that they can use Q0 and Pmax to generate normalized measures of value (i.e. essential value; α) and price (nPmax), which have been used to compare price sensitivity, effort, and value across different drug and non-drug rewards in polysubstance models in several species (e.g. in rats, rhesus monkeys, and human participants) (Petry and Bickel, 1998; Ward et al., 2006; Wade-Galuska et al., 2007, 2011; Crummy et al., 2020). In particular, these studies permit examination of the relative reinforcing properties of different doses and classes of drugs (Wade-Galuska et al., 2007; Cooper et al., 2010; Huskinson et al., 2015), as well as alterations in cost valuation for a drug following pre-exposure to another drug (Cooper et al., 2010; Hofford et al., 2016; Morris et al., 2018). Direct quantification of the assigned value of these drugs across different polysubstance histories and drug doses is very useful for assessing the impact of polysubstance history on relative reinforcer value. Furthermore, these measures can be used to compare how polysubstance users value drug rewards across different experimental parameters (e.g. differences in priming dose of one drug, environmental context, pattern of drug use). Finally, clinical studies are using questionnaires or controlled laboratory environments to investigate the behavioral effects of a polysubstance history. Specifically, these studies use monetary choice procedures that compare assigned value of drugs at different doses (Greenwald et al., 2010), how assigned value changes for one drug with a change in price of another (Petry and Bickel, 1998; Petry, 2001; Sumnall et al., 2004; Chalmers et al., 2010), or how relative value of one drug changes with perceived change in subjective quality of another available drug (Cole et al., 2008). Additionally, progressive ratio tests for a single drug or drug combinations to study motivation (Greenwald et al., 2010), and delay-discounting rates for money and drug rewards to study decision-making, (Strickland et al., 2019) have also been performed. These studies permit comparison of perceived value across multiple drugs in participants with histories of single or polysubstance use (for further review, see Heinz et al., 2012).
Effects of Polydrug Use on Addictive Behaviors
Given the unique neurobiological alterations that can occur with exposure to multiple drugs, along with the high prevalence of polysubstance use disorders, there is a strong need to develop polydrug paradigms that have high translational value. These paradigms are critical for fully understanding the behavioral changes and addiction-related phenotypes that develop following polydrug use. However, given the vast number of potential substance combinations and the variability in methodologies that exist across studies, there are currently mixed results and interpretations regarding the impact of polydrug history on addiction-related behaviors. Nonetheless, some general trends in drug consumption, drug preference and drug-seeking have been demonstrated in commonly investigated substance combinations (Figure 2).
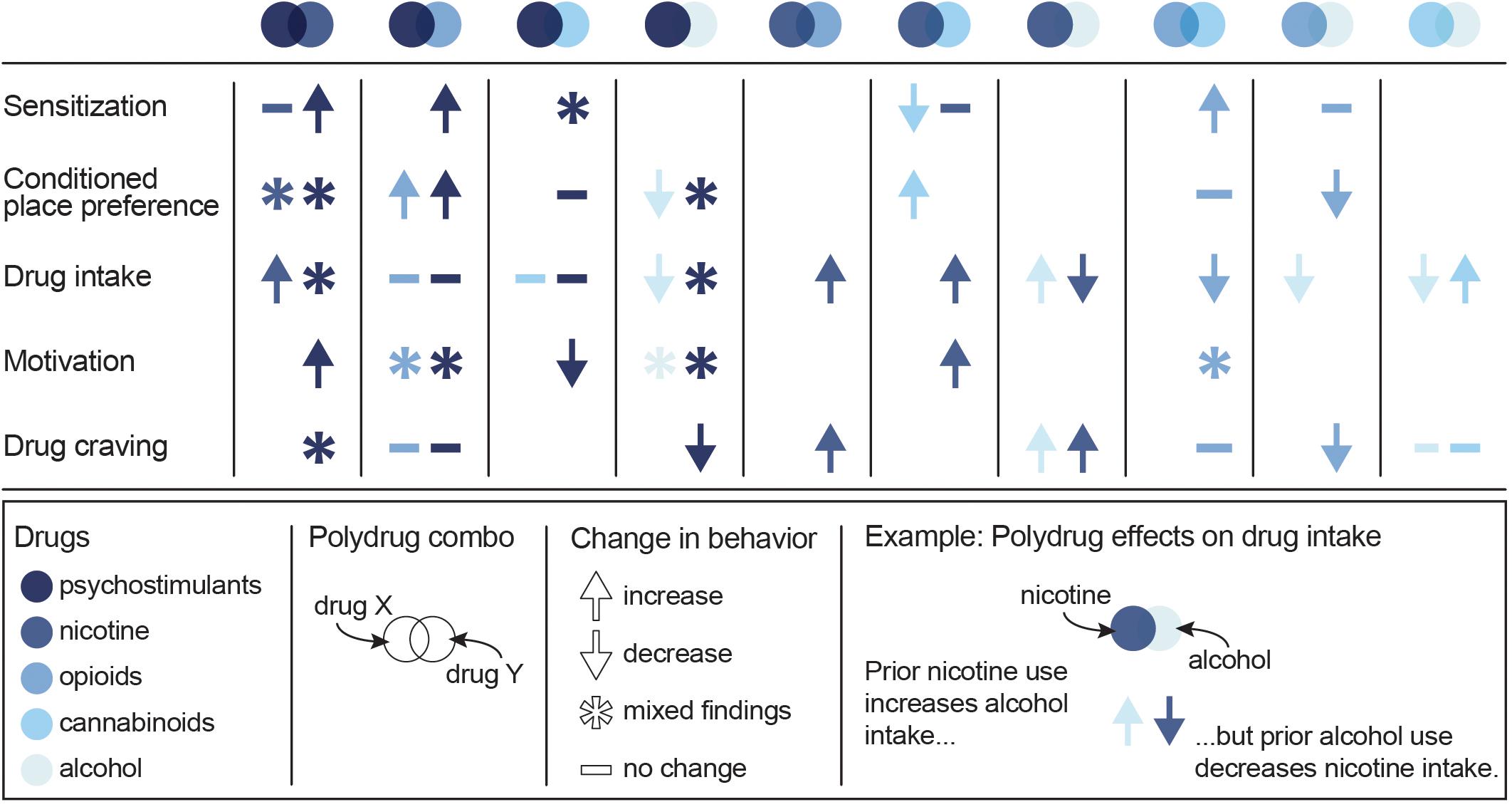
Figure 2. Summary of the effects of specific polydrug combinations on assays of addiction-like behaviors. Studies are organized into X/Y polydrug combos (columns) and behavioral assays (rows), with subcolumns for the effect of drug Y on drug X (left subcolumn) and the effect of drug X on drug Y (right subcolumn). Symbols represent the net effect of the X/Y polydrug combo on a given behavior, with color depicting the specific drugs tested. Sensitization: locomotor sensitization; Conditioned place preference: acquisition, expression; Drug intake: self-administration; Motivation: progressive ratio, behavioral economics; Drug craving: reinstatement, cue reactivity. Data from Mello and Mendelson (1978), Mello et al. (1980), Mello et al. (2014), Huston-Lyons et al. (1993), Foltin et al. (1993), Aspen and Winger (1997), Ranaldi and Wise (2000), Valverde et al. (2001); De Vries et al. (2001), Parker et al. (2004); Solinas et al. (2005), Liang et al. (2006); Biala and Budzynska (2006), Ward et al. (2006); Panlilio et al. (2007), Panlilio et al. (2013), Winger et al. (2007), Lê et al. (2010), Lê et al. (2014), Levine et al. (2011), Cortright et al. (2011), Pomfrey et al. (2015), Maguire and France (2016); Mahmud et al. (2017), Fredriksson et al. (2017); Giasson-Gariépy et al. (2017), Griffin et al. (2017); Schwartz et al. (2018), Winkler et al. (2018); Manwell et al. (2019), Ponzoni et al. (2019), Crummy et al. (2020), and Stennett et al. (2020).
Psychostimulants
Some of the more commonly studied polydrug combinations include administration of cocaine with other drugs (Francesco et al., 2003; Leri et al., 2003b; Substance Abuse and Mental Health Services Administration, 2016). However, in contrast to human reports (Heil et al., 2001; Williamson et al., 2006; Staiger et al., 2013; Preston et al., 2016; Lorvick et al., 2018; Kariisa et al., 2019), an increase in addiction-like severity has not been observed in preclinical studies, suggesting a need for models with greater translational relevance that can capture the enhanced severity seen in human polysubstance users. Specifically, animal studies of sequential cocaine and alcohol or cocaine and heroin use have not found differences in drug intake or reinstatement of drug-seeking as a function of single versus polydrug use (Pattison et al., 2014; Fredriksson et al., 2017; Crummy et al., 2020; Stennett et al., 2020). These effects were observed despite variance in the use of contingent and non-contingent drug administration, drug doses, and species, including rats (Crummy et al., 2020; Stennett et al., 2020) and rhesus monkeys (Aspen and Winger, 1997). Consistent with this work, intermittent alcohol exposure has not been shown to affect cocaine self-administration (Aspen and Winger, 1997; Fredriksson et al., 2017) or the reinforcing properties of cocaine measured via demand curves in rhesus monkeys (Winger et al., 2007). In addition, intermittent alcohol exposure has not been shown to affect progressive ratio tests of motivation for cocaine (Mateos-García et al., 2015), or the long-term reconsolidation of preference for cocaine in drug-paired contexts (Zhu et al., 2020) in rats. In contrast, adolescent alcohol exposure has been shown to have long-lasting effects on cocaine self-administration and reward, suggesting that this population is particularly susceptible to the effects of polysubstance use. For example, adolescent alcohol exposure increases motivation for cocaine (Mateos-García et al., 2015), enhances the development of a cocaine CPP in both mice (Molet et al., 2013) and rats (Hutchison and Riley, 2012; Mateos-García et al., 2015), and weakens cocaine-induced taste aversion (Busse et al., 2005). In addition, simultaneous heroin and psychostimulant administration increases the motivation to self-administer cocaine (Ward et al., 2006) and methamphetamine (Ranaldi and Wise, 2000), and both simultaneous and sequential administration of morphine and methamphetamine have been shown to be rewarding, as measured by the development of a CPP (Briggs et al., 2018). Pretreatment with an opioid also enhances methamphetamine-induced psychomotor sensitization (Liang et al., 2006). These findings suggest that opioids can enhance the rewarding and motivational properties of psychostimulants, particularly when administered simultaneously.
Polydrug studies with cocaine and nicotine have largely reported additive and/or synergistic effects of the two drugs. In particular, co-administration of cocaine and nicotine increases drug intake in rhesus monkeys (Mello et al., 2014) and rats (Bechtholt and Mark, 2002), enhances locomotor sensitization and the development of a CPP in mice (Levine et al., 2011), and induces a cross-sensitized drug-craving (Reid et al., 1998; Weinberger and Sofuoglu, 2009; Cortright et al., 2012). Additionally, chronic nicotine pretreatment facilitates the acquisition of cocaine self-administration (Horger et al., 1992; Bechtholt and Mark, 2002; Linker et al., 2020), increases motivation under a progressive ratio schedule, impairs extinction learning, and enhances drug-primed reinstatement for amphetamine following amphetamine self-administration (Cortright et al., 2012). Conversely, prior nicotine treatment history reduces demand elasticity for cocaine (Schwartz et al., 2018). Notably, the effects of nicotine and psychostimulant polydrug use are largely dose-dependent as pretreatment with a smaller dose of nicotine [0.3 mg/kg, subcutaneous (sc)] increases motivation to take cocaine under a progressive ratio schedule in rats, whereas a larger dose of nicotine (0.6 mg/kg, sc) has the opposite effect (Bechtholt and Mark, 2002). Additionally, simultaneous administration of methamphetamine [2.0 mg/kg, intraperitoneal (ip)] and nicotine (1.0 mg/kg ip) induces a conditioned place aversion in mice, while sequential administration of the same dose induces a CPP (Briggs et al., 2018). Together, these studies demonstrate that the effects of nicotine on psychostimulant motivation, intake, and reward are heavily impacted by the parameters surrounding nicotine delivery (e.g. dose, route, pattern, etc.), which should be carefully considered when comparing study results and developing preclinical polydrug paradigms. Notably, adolescent exposure to nicotine has no effect on the subsequent development of a cocaine CPP, cocaine-induced taste aversion, cocaine self-administration, extinction, or reinstatement of cocaine-seeking in adulthood (Pomfrey et al., 2015), although it has been shown to enhance cocaine self-administration in adolescent rats (Linker et al., 2020). These data suggest that, unlike alcohol, early exposure to nicotine does not lead to increases in addiction-like behavior to cocaine in animals.
In humans, simultaneous cocaine and cannabis use produces feelings of “stimulated” and “high” that last longer than either drug alone (Foltin et al., 1993), and cue-induced drug-craving in individuals who co-use cocaine and cannabis lasts longer than for those who only use cocaine (Giasson-Gariépy et al., 2017). In contrast, THC reduces the motivation to self-administer cocaine in rodents (Panlilio et al., 2007). Although this suggests a differential regulation of cocaine’s effects in humans and rodents, further work is necessary to ensure that animal models of increased addiction severity cannot, in fact, be developed. Notably, however, neither cocaine and cannabis nor cocaine and alcohol co-administration in humans produces subjective effects that are different from cocaine, cannabis, or alcohol alone (Foltin et al., 1993). Similarly, THC pretreatment in rodents has no effect on psychostimulant reward or self-administration, nor does it potentiate the development of a CPP to amphetamine (Panlilio et al., 2007; Cortright et al., 2011; Keeley et al., 2018), indicating a unique effect of cocaine and cannabis on drug-craving. Interestingly, CBD has no effect on cocaine self-administration, motivation, or cue-induced reinstatement of cocaine-seeking (Mahmud et al., 2017), suggesting the effects of cannabis on cocaine craving are likely due to THC, rather than CBD. However, CBD treatment has been found to reduce motivation to self-administer methamphetamine on a progressive ratio schedule and to reduce methamphetamine-primed reinstatement of drug-seeking (Hay et al., 2018). The additive effects of cannabinoid and psychostimulant polydrug use appear to be dependent on both the amount of drug consumed and the age range during use. For example, acute THC weakens psychomotor sensitization, but repeated THC administration promotes tolerance to the acute effects, increasing amphetamine-induced stereotypy and locomotor activity (Gorriti et al., 1999; Cortright et al., 2011). Additionally, adolescent THC exposure accelerates acquisition of cocaine self-administration and increases intake of low doses of cocaine (Friedman et al., 2019), indicating long-lasting changes in reward circuitry following adolescent THC use, similar to alcohol.
Nicotine
Limited work has focused on the effects of polydrug use on nicotine-induced addiction behaviors. However, it has been found that THC pretreatment can enhance nicotine consumption and price inelasticity (measured by α) in behavioral economic tests in rats (Panlilio et al., 2013), and heroin intake has been found to increase cigarette consumption in people (Mello et al., 1980). In addition, pre-exposure to alcohol or simultaneous access to both alcohol and nicotine decreases nicotine self-administration in rodent studies (Lê et al., 2010, 2014). Although access to alcohol has no effect on responding for nicotine under extinction conditions, a priming dose of alcohol does reinstate nicotine-seeking (Lê et al., 2010). Finally, systemic co-administration of methamphetamine and nicotine produces a conditioned place aversion in rats (Briggs et al., 2018), whereas pretreatment with either amphetamine or morphine increases the rewarding properties of nicotine as shown with lowered intracranial self-stimulation thresholds (Huston-Lyons et al., 1993). These studies further emphasize the need to consider use patterns and dose in interpretation of polydrug use effects.
Opioids
Similar to psychostimulant polydrug studies, sequential use of heroin and cocaine has not been found to alter heroin self-administration or reinstatement of heroin-seeking (Crummy et al., 2020). Although alcohol pretreatment can prevent the long-term reconsolidation of preference for morphine in drug-paired contexts (Zhu et al., 2020), adolescent alcohol exposure enhances the development of a morphine CPP (Molet et al., 2013). This finding indicates that the long-term effects of adolescent alcohol exposure are generalizable to multiple drug classes. Interestingly, co-administration of morphine and THC prevents the development of the analgesic tolerance that normally accompanies long-term exposure to either drug alone (Cichewicz and McCarthy, 2003; Cox et al., 2007; Smith et al., 2007). In addition, the analgesic effects of THC and oxycodone co-administration are additive to oxycodone alone (Nguyen et al., 2019). Moreover, administration of either THC or both THC and CBD attenuates naloxone-precipitated withdrawal without impacting the development of a morphine CPP (Lichtman et al., 2001; Valverde et al., 2001). These data suggest a potential role for cannabinoids in regulating a physical dependence to opioids without altering their reinforcing properties. In support of this, repeated THC administration has no effect on breakpoint during a PR test of heroin self-administration (Solinas et al., 2004, but see Nguyen et al., 2019) or relapse to heroin-seeking, although it produces a small reduction in both heroin (Maguire and France, 2016) and oxycodone (Nguyen et al., 2019) intake in fixed-ratio self-administration sessions. The effects of opioid and cannabis polydrug use, however, appear to be dose-dependent as systemic administration of THC prior to heroin self-administration reduces responding for large doses of heroin, but has no effect on responding for lower doses in both monkeys and rats (Solinas et al., 2004; Maguire and France, 2016).
Cannabinoids
As with psychostimulants and opioids, administration of nicotine with THC augments the effects of either drug alone when measured in tests of locomotion, analgesia, and hypothermia (Valjent et al., 2002). In addition, THC and nicotine co-administration exacerbates the somatic symptoms of THC withdrawal (Valjent et al., 2002). However, after repeated nicotine treatment and 2 weeks of drug abstinence, nicotine re-administration attenuates THC-induced decreases in locomotor activity, increases in anxiety measures (when assessed in the elevated-plus maze), and changes in social interaction (Manwell et al., 2019). These findings suggest that nicotine enhances the negative symptoms of THC when administered concurrently or in close temporal proximity. Although nicotine pretreatment enhances the rewarding effects of subthreshold doses of THC (Ponzoni et al., 2019), cocaine pretreatment heightens THC-induced anxiogenic behaviors (Panlilio et al., 2007). Cannabis and alcohol polydrug use is relatively common in humans, and individuals report reduced alcohol consumption when cannabis is available (Mello and Mendelson, 1978), suggesting a role for cannabinoids in alcohol intake. However, drug-induced cognitive and physical impairments in humans, as assessed in a driving simulation, were found to be more severe after use of THC and alcohol compared to either drug alone (Downey et al., 2013). Conducting polydrug studies of combinations of THC or CBD with other drug classes is therefore necessary to understand the differential effects resulting from these drugs.
Unfortunately, due to long-term restrictions on cannabis research in the US and past difficulties in modeling cannabis use with self-administration models in rodents (Panlilio et al., 2015), much less is known about the impact of cannabis relative to other drugs. The development of novel methods of cannabis self-administration in animals, including oral self-administration of Δ9-tetrahydrocannabinol (THC)-containing gelatin (Kruse et al., 2019), self-administration of vaporized THC and cannabidiol (CBD) (Freels et al., 2020), and intravenous self-administration of THC and CBD (Neuhofer et al., 2019) will help facilitate the preclinical study of cannabis use disorder, as well as enable us to better understand the consequences of polydrug use involving cannabis.
Alcohol
Polydrug use of alcohol and nicotine produce mixed phenotypes in relation to addiction behaviors. For example, pre-exposure to alcohol or simultaneous access to both alcohol and nicotine increases alcohol self-administration, but not when nicotine is administered prior to alcohol each day (Lê et al., 2010, 2014). Chronic nicotine treatment also enhances alcohol preference, an effect that persists through nicotine withdrawal (Blomqvist et al., 1996). However, although access to nicotine impairs extinction learning to alcohol responding, it has no effect on reinstatement of drug-seeking, as rats respond similarly on alcohol and nicotine-associated levers following a priming injection of nicotine (Lê et al., 2010). Nonetheless, another study found that priming doses of alcohol, but not nicotine, were capable of reinstating alcohol-seeking following self-administration of both nicotine and alcohol (Sentir et al., 2020). Studies have not systematically examined the effects of other drugs on alcohol use and addiction.
Neurobiology of Addiction
The development and maintenance of addiction behaviors arises in part from maladaptive neuroplasticity within the neural circuits responsible for decision-making, learning, motivation, and reward processing. In particular, alterations in the cortico-basal ganglia-thalamic (C-BG-T) network are known to contribute to drug-taking and drug-seeking behaviors, as well as the persistence of SUDs (Koob and Volkow, 2016). The C-BG-T is a heavily interconnected network that integrates sensory and interoceptive cues to drive motivated behavioral output. The striatum, which serves as an interface of the C-BG-T, receives extensive glutamatergic input from cortical (e.g. prefrontal) and subcortical (e.g. amygdala, hippocampus, thalamus) regions, along with dopaminergic input from the midbrain [substantia nigra (SN)/ventral tegmental area (VTA)] (Gerfen and Surmeier, 2011; Calabresi et al., 2014). Integration of glutamatergic and dopaminergic inputs with local inhibition in the striatum contributes to the initiation or suppression of behavioral output, and imbalanced signaling between the two striatal output pathways (i.e. the direct and indirect) can drive addictive behaviors (Kravitz et al., 2010; Ferguson et al., 2011; O’Neal et al., 2019). It is beyond the scope of the current review to fully explore all of the neurobiological changes that occur in the C-BG-T with drug use. We will instead focus on one microcircuit within the C-BG-T that is central to the acute effects of drugs with addictive potential, and in some of the persistent changes that develop following long-term drug use: The prefrontal cortex (PFC) – nucleus accumbens (NAc) – VTA network (Figure 3). Following a review of the microcircuitry and connectivity of these regions, we will discuss disruptions that occur within this network following both acute and long-term exposure to different classes of drugs, with emphasis on the similarities and/or differences of effects relative to polydrug combinations.
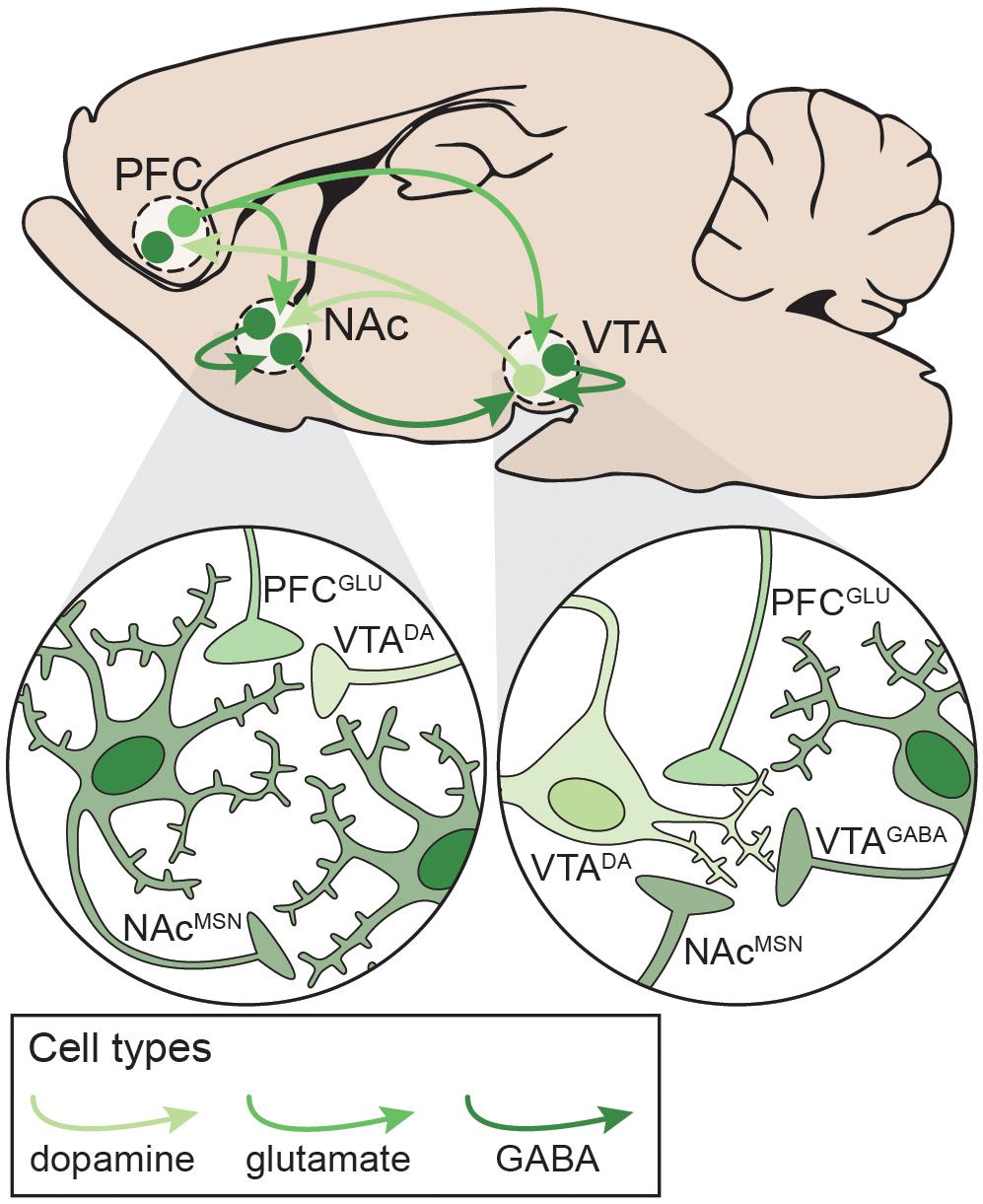
Figure 3. Neural circuitry targeted by potentially addictive drugs. Simplified schematic emphasizing local and distal connections between the PFC, NAc, and VTA that are targeted by potentially addictive drugs. Left: NAcMSNs receive excitatory glutamatergic inputs from PFCGLU neurons, dopaminergic inputs from VTADA neurons, and inhibitory GABAergic inputs from other NAcMSNs. Right: VTADA neurons are maintained under tonic inhibition by local VTAGABA interneurons and NAcMSNs and receive excitatory inputs from PFCGLU neurons. DA: dopamine; GLU: glutamate; MSN: medium spiny neuron; NAc: nucleus accumbens; PFC: prefrontal cortex; VTA: ventral tegmental area.
Prefrontal Cortex
The PFC is centrally involved in reward learning, decision-making, and outcome valuation (Garcia et al., 2018). It is a highly heterogenous structure, which adds to the complexity in understanding its role in cognition, as well as how its dysregulation contributes to drug use and addiction. In general, the medial prefrontal cortex (mPFC) – encompassing the anterior cingulate (ACC), prelimbic (PrL), and infralimbic (IL) cortices – regulates motivation and seeking of both natural and drug rewards via excitatory glutamatergic projections to the NAc and VTA (Koob and Volkow, 2016). Notably, projections from the mPFC to the NAc are topographically organized, with the PrL innervating the NAc core and the IL innervating the NAc shell (Brog et al., 1993). In contrast, the orbitofrontal cortex projects more heavily to the dorsal striatum and SN and is primarily involved in outcome and probability valuation (Padoa-Schioppa and Conen, 2017). Hypoactivity in the PFC contributes to drug craving and seeking despite negative consequences in preoccupation stages of addiction, with dysregulated connectivity to the striatum and VTA contributing to cue sensitivity and motivated drug-taking (Volkow and Boyle, 2018). The PFC is comprised of six layers, each with unique connectivity patterns and distinct cell types. Specifically, a majority of PFC neurons are large pyramidal output cells (75%), as well as several subtypes of interneurons (∼25%) (Santana and Artigas, 2017). Pyramidal cells in layers II/III send local projections within cortex, while those in layers V-VI send projections throughout the C-BG-T, including to the striatum, midbrain, amygdala, hippocampus, and thalamus (Gabbott et al., 2005; Santana and Artigas, 2017). Pyramidal cells can be further subdivided based on physiology and connectivity (Morishima and Kawaguchi, 2006; Brown and Hestrin, 2009; Reiner et al., 2010; Shepherd, 2013; Kim E. J. et al., 2015). Recent studies have begun to characterize the anatomical, electrophysiological, and molecular profiles of each of these cell types (Kalmbach et al., 2015; Kim E. J. et al., 2015; Saiki et al., 2018; Chen et al., 2019; Winnubst et al., 2019), though how they each regulate behavior remains poorly understood. Finally, the PFC contains multiple populations of interneurons that heavily regulate cortical output via projections to both pyramidal neurons and interneurons (van Versendaal and Levelt, 2016; Batista-Brito et al., 2017).
Nucleus Accumbens
The striatum is a heterogeneous structure comprised primarily of two interspersed populations of GABAergic medium spiny neurons (MSNs) that can bidirectionally regulate behavioral output. Direct pathway MSNs (dMSNs) express dopamine D1-like (D1) receptors and the neuropeptides dynorphin and substance P, project directly to the midbrain, and can promote behavioral output by serving as a “go” signal. Conversely, indirect pathway MSNs (iMSNs) express dopamine D2-like (D2) receptors and the neuropeptide enkephalin, project indirectly to the midbrain via the pallidum (GPe and VP), and can suppress behavioral actions by serving as a “stop” signal (Kravitz et al., 2010; Gerfen and Surmeier, 2011). Drug use promotes increased phasic dopamine from D1 activation, prompting reward attribution to drug use during binge/intoxication phases of the addiction cycle, conditioning, and incentive salience attribution to drug-taking contexts (Volkow et al., 2011; Koob and Volkow, 2016). The striatum contains dorsal and ventral compartments, with further subdivisions based on connectivity and function. The ventral striatum – comprised of the olfactory tubercule, NAc core, and NAc shell – receives dopaminergic modulation from the VTA and glutamatergic input from the PFC, as well as thalamic, hippocampal, and amygdala nuclei (Li et al., 2018). In general, the ventral striatum regulates motivated behavior and reward learning. However, it has been hypothesized that an ascending loop between the ventral and the dorsal striatum facilitates information consolidation during learning, whereby habitual behaviors transition from the ventral striatum to the dorsal striatum, contributing to compulsive drug-seeking (Dobbs et al., 2016; Koob and Volkow, 2016; Burke et al., 2017). Importantly, while dMSNs and iMSNs have historically been differentiated by downstream targets and expression of dopamine receptors, ventral striatal dMSNs send collaterals to the VP (Cazorla et al., 2014; Kupchik et al., 2015), and D1 and D2 receptors are co-expressed to some degree in the NAc core (6–7%), and NAc shell (12–15%) (Bertran-Gonzalez et al., 2008; Gagnon et al., 2017). In addition to MSNs, the striatum contains large, tonically active cholinergic interneurons and multiple subtypes of GABAergic interneurons with distinct electrophysiological properties and peptide expression patterns (Burke et al., 2017). MSNs also receive cholinergic modulation from other projections (Dautan et al., 2014), though the relevance of these inputs to local or network dynamics and the role of cholinergic striatal neurons in the addiction cycle remains uncertain. Interestingly, each MSN receives ∼5000–15000 excitatory inputs in addition to ∼1200–1800 GABAergic inputs from other MSNs, therefore, modulation of MSN activity via cholinergic and dopaminergic inputs appears necessary for signal integration and effective synaptic plasticity (Moyer et al., 2007; Burke et al., 2017). Indeed, MSNs exhibit bi-stability, residing almost exclusively in either a down-state (−80 mV) or an up-state (−50 mV, near threshold) in the absence of external input. In addition, the maintenance of bi-stability and intrinsic excitability relies on the activity of cation channels that are under robust dopaminergic and cholinergic modulation (Plenz and Kitai, 1998; Moyer et al., 2007). Maintenance of intrinsic excitability within MSNs is critical for normal regulation of behavioral output, and dysregulation of striatal microcircuitry contributes to the development and expression of addiction behaviors (Bock et al., 2013; Stefanik et al., 2013; O’Neal et al., 2019).
Ventral Tegmental Area
The VTA sends dopaminergic projections to cortical, striatal, and subcortical (e.g. hippocampus, amygdala, thalamus) areas to modulate C-BG-T network activity (Koob and Volkow, 2010). Dopaminergic projections from the VTA to the NAc regulate goal-directed behaviors and have been heavily implicated in the binge/intoxication phase of SUDs (Koob and Volkow, 2016). Recently identified subtypes of VTADA neurons with unique molecular profiles preferentially project to the NAc core or NAc shell, and regulate reward learning or motivation, respectively. However, co-activation of both populations appears to be necessary for robust reinforcement (Heymann et al., 2019). VTADA neurons receive dense glutamatergic input from the PFC and several midbrain structures, as well as GABAergic input from a variety of sources (reviewed in Morales and Margolis, 2017), including the NAc and local VTAGABA neurons. VTAGABA neurons maintain tonic DA levels via inhibition of VTADA neurons, and brief disinhibition of VTADA neurons results in phasic DA release into the NAc. VTAGABA neurons receive glutamatergic input from the PFC and a number of subcortical nuclei, as well as GABAergic input from throughout the brain, including the NAc (Morales and Margolis, 2017). The VTA also contains glutamatergic neurons that project to striatal interneurons (Brown et al., 2012; Qi et al., 2016), though the inputs to and behavioral relevance of these neurons is unknown. Notably, the VTA contains subpopulations of DA neurons that can release glutamate and/or GABA (Kim J. I. et al., 2015; Berrios et al., 2016; Morales and Margolis, 2017), allowing the VTA to modulate local C-BG-T activity at multiple levels and time scales. Within the NAc, the activity of VTADA neurons is modulated by dopamine D2 autoreceptors on VTADA terminals and cholinergic interneurons (Morales and Margolis, 2017).
Mechanisms of Addiction: Acute Drug Effects
A unique feature of all potentially addictive drugs is the ability to reinforce the binge/intoxication phase of the addiction cycle via evoked phasic DA release into the NAc, yet the underlying mechanisms vary across drugs (Figure 4; Volkow and Boyle, 2018). Psychostimulants disrupt DA reuptake into VTADA terminals (Pontieri et al., 1995), nicotine and alcohol directly activate VTADA neurons (Pidoplichko et al., 1997; Brodie et al., 1999), and opioids and cannabinoids disinhibit VTADA neurons (Pidoplichko et al., 1997; Cheer et al., 2000). The synergistic and antagonistic interactions between different drugs within the C-BG-T lends complexity to the study of polydrug use, and little is known about the mechanisms underlying the acute effects of specific polydrug combinations. However, in vivo extracellular recordings in rats that alternatively self-administered cocaine and heroin in the same session found that only ∼20% of PFC and NAc neurons responded similarly to both drugs (Chang et al., 1998), indicating divergent engagement of the C-BG-T by these drugs. Thus, examining the synergistic and antagonistic mechanisms of different drugs can guide our understanding of how specific polydrug combinations may disrupt C-BG-T network dynamics and contribute to the manifestation of addiction behaviors.
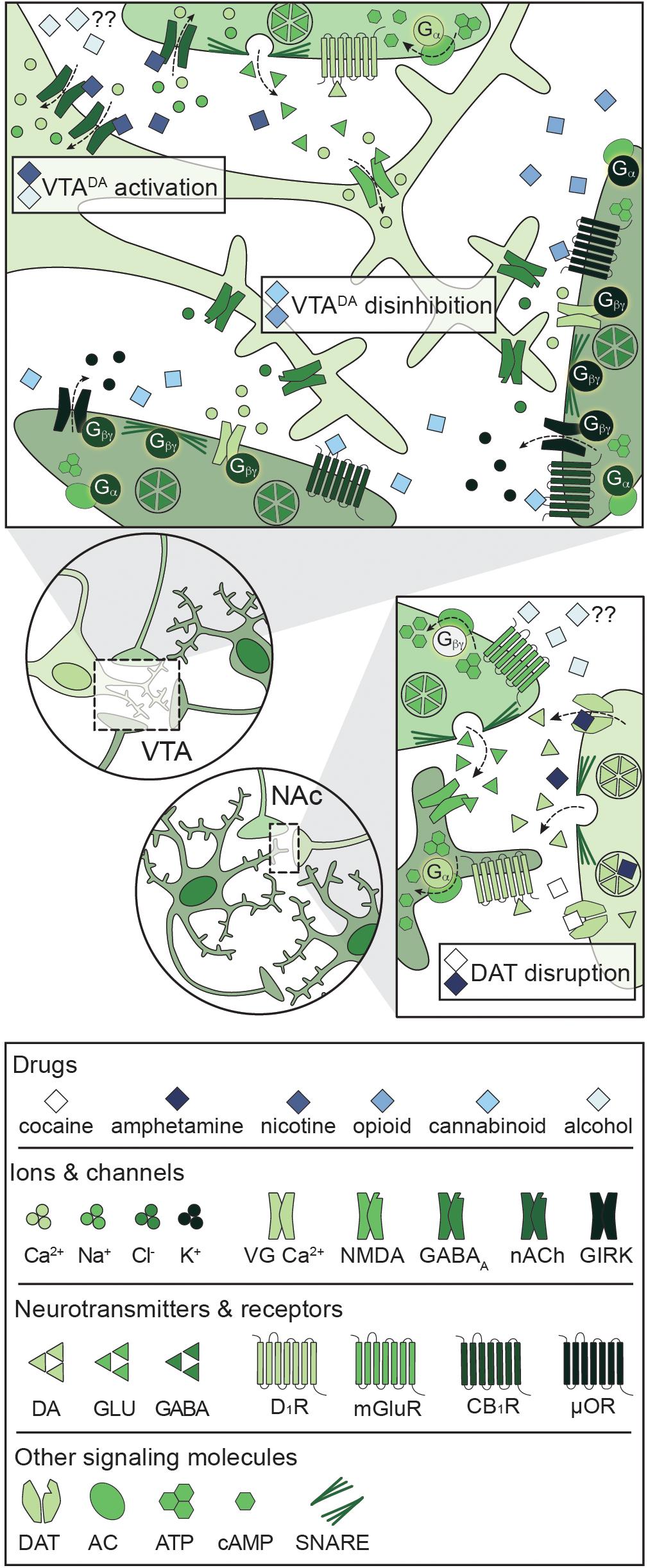
Figure 4. Primary mechanisms of action of potentially addictive drugs. Potentially addictive drugs increase DA release into the NAc, but different drugs act via distinct mechanisms. Top: Opioids and cannabinoids disinhibit VTADA neurons via presynaptic inhibition of VTAGABA and NAcMSN inputs through four notable mechanisms: Inhibition of VG Ca2+ channels, activation of GIRKs, inactivation of AC, and inhibition of GABA release. Nicotine activates VTADA neurons via direct activation of somatodendritic nAChRs and activation of presynaptic PFCGLU inputs. Alcohol directly activates VTADA cell bodies, but the mechanism is not understood. Bottom: Psychostimulants impair DA reuptake by blocking DAT (cocaine) or reversing the activity of DAT and facilitating DA release (amphetamine), leading to increased dopaminergic tone in the NAc. Alcohol also targets PFCGLU inputs to the NAc, but the net effect on PFCGLU activity is unknown. AC: adenylyl cyclase; ATP: adenosine triphosphate; cAMP: cyclic adenosine monophosphate; CB1R: cannabinoid 1 receptor; DA: dopamine; DAT: dopamine transporter; D1R: dopamine D1 receptor; GABA: gamma- aminobutyric acid; GIRK: G protein-coupled inwardly rectifying K+ channel; GLU: glutamate; mGluR: metabotropic glutamate receptor; μOR: mu opioid receptor; NAc: nucleus accumbens; nACh: nicotinic acetylcholine receptor; NMDA: N-methyl-D-aspartate receptor; PFC: prefrontal cortex; SNARE: soluble N-ethylmaleimide-sensitive factor attachment protein receptor; VG Ca2+: voltage-gated Ca2+ channel; VTA: ventral tegmental area.
Psychostimulants
All psychostimulants directly enhance striatal DA release via disruption of dopamine transporter (DAT) activity (Pontieri et al., 1995), though they do so via distinct mechanisms. Cocaine blocks DAT-mediated reuptake of DA, while amphetamine reverses DAT activity and induces DA release from VTADA terminals (Hyman et al., 2006; Kelly et al., 2008). Psychostimulants also acutely increase glutamate transmission in the PFC, NAc, and VTA (Reid and Berger, 1996; You et al., 2007; Shin et al., 2016), indicating broad increases in activity throughout the C-BG-T network following psychostimulant use. The combination of enhanced glutamatergic and dopaminergic input to the NAc facilitates the transition of MSNs to the up- state, and activation of DA-dependent signaling cascades (McFarland and Kalivas, 2001; Feltenstein and See, 2008). For example, psychostimulants acutely increase activation of the immediate early gene Fos in striatal dMSNs and iMSNs (Badiani et al., 1999; Uslaner et al., 2001; Ferguson and Robinson, 2004). Fos encodes a number of proteins, including ΔFosB, that have been widely implicated in addiction pathology, and enhanced activation in the NAc is thought to contribute to long-term disruptions in normal C-BG-T activity (Nestler et al., 2001). Co-administration of nicotine, alcohol, or heroin enhances psychostimulant-induced DA release into the NAc (Bunney et al., 2001; Mello et al., 2014; Pattison et al., 2014), though specific combinations do so via divergent mechanisms. For example, administration of cocaine and nicotine simultaneously activates VTADA neurons and disrupts DA reuptake (Mello et al., 2014; De Moura et al., 2019), resulting in a greater magnitude of DA release into the NAc than that evoked from either drug alone. Notably, some polydrug combinations that include psychostimulants have divergent and opposing effects on the C-BG-T circuit. Co-administration of alcohol with cocaine induces hepatic production of cocaethylene, which can target DAT and presynaptic D2 autoreceptors to amplify DA release (Bunney et al., 2001). However, alcohol also prevents cocaine-induced glutamate transmission in the NAc core (Stennett et al., 2020). Lastly, polydrug studies with psychostimulants have identified an exacerbation of drug-induced cellular toxicity compared to psychostimulant use alone. Specifically, co-administration of heroin with cocaine decreases metabolic activity, increases intracellular Ca2+ signaling, and decreases mitochondrial membrane potential (Cunha-Oliveira et al., 2008). Collectively, these effects contribute to enhanced caspase 3-dependent apoptotic activity and subsequent cell death compared to either drug alone (Cunha-Oliveira et al., 2008).
Nicotine
Unlike psychostimulants, nicotine enhances DA release via activation of nicotinic acetylcholine (nACh) receptors within the VTA. nACh receptors are non-selective cation channels, with permeability to Na+, K+, and Ca2+, and their activation leads to depolarization and enhanced neurotransmitter release (Benowitz, 2009). The reinforcing effects of nicotine are primarily due to nicotine’s activity on somatodendritic nACh receptors located on VTADA neurons and on presynaptic nACh receptors located on PFCGLU inputs (Nisell et al., 1994). Acute nicotine exposure activates both presynaptic PFC inputs (Fu et al., 2000; Picciotto and Kenny, 2013) and VTADA cell bodies (Calabresi et al., 1989; Pidoplichko et al., 1997), triggering phasic DA release into the NAc (Dani and De Biasi, 2001; Picciotto and Mineur, 2014). Notably, nicotine-evoked DA release is occluded with blockade of glutamate receptors or activation of GABAB receptors (Fadda et al., 2003; Kosowski et al., 2004), highlighting the extensive regulation of VTADA neuron activity by local VTA microcircuitry. Studies have not examined how the acute effects of nicotine are changed by polydrug use.
Opioids
Although opioids are differentiated by their origin, potency, and receptor bias factor (Schmid et al., 2017), all opioids exert their rewarding effects via activation of mu opioid (μO) receptors. μO receptors are expressed both somatodendritically and axonally (Arvidsson et al., 1995), but the primary mechanism of opioid-induced DA release relies on presynaptic inhibition of VTAGABA neurons. μO receptors are inhibitory G protein-coupled receptors (GPCRs), and their activation reduces neuronal excitability via four mechanisms: (1) Gα-mediated inhibition of cAMP-dependent signaling cascades (e.g. PKA, CREB), (2) Gβγ-mediated activation of G protein-coupled inwardly rectifying K+ (GIRK) channels, (3) Gβγ-mediated inactivation of voltage-gated Ca2+ channels, and (4) Gβγ-mediated inhibition of SNARE-dependent vesicle release (Bourinet et al., 1996; Blanchet and Lüscher, 2002; Blackmer et al., 2005; Al-Hasani and Bruchas, 2011; Zamponi and Currie, 2013). Acute exposure to opioids inhibits VTAGABA neurons (Johnson and North, 1992; Corre et al., 2018), resulting in subsequent disinhibition of VTADA neurons and phasic DA release into the NAc (Hemby et al., 1995; Pontieri et al., 1995). Nonetheless, although opioids facilitate DA release into the striatum, whether this DA transmission is necessary for opioid reward remains a point of debate (Badiani et al., 2011). Opioids activate VTADA neurons in vivo and increase DA in the NAc (Di Chiara and Imperato, 1988; Johnson and North, 1992), but neither lesions of the NAc nor systemic antagonism of DA receptor blockades have an effect on opioid reward (Ettenberg et al., 1982; Van Ree and Ramsey, 1987; Olmstead and Franklin, 1997; Sellings and Clarke, 2003). Given the divergent mechanism of action for opioids compared to psychostimulants and nicotine, it is not surprising that co-administration of these drugs augments the acute effects of opioids. Indeed, simultaneous administration of opioids and psychostimulants produces an additive increase in DA release in the NAc, and prolongs elevated levels of DA and its metabolites, DOPAC and HVA (Zernig et al., 1997). Similarly, simultaneous administration of opioids and nicotine enhances opioid-evoked DA release in the NAc and dorsal striatum (Vihavainen et al., 2008). Cross-tolerance to opioid-mediated analgesia has also been shown following pre-exposure to nicotine and cannabis (Schmidt et al., 2001), and chronic nicotine treatment dose-dependently reduces analgesic tolerance to opioids in a nACh receptor-dependent manner (Haghparast et al., 2008; De Moura et al., 2019). Interestingly, pretreatment with Ca2+ channel blockers or naloxone prevents this tolerance, suggesting a complex pharmacological interaction between opioids and nicotine (Biala and Weglinska, 2006). Notably, cross-tolerance to opioid analgesia is mediated via divergent mechanisms for different polydrug combinations. Nicotine and opioid cross-tolerance is mediated by μO and nACh receptors (Haghparast et al., 2008; De Moura et al., 2019) while cannabinoid and opioid cross-tolerance is mediated by μO receptors and cannabinoid 1 (CB1) receptors (Pugh et al., 1994, 1996).
Cannabinoids
Cannabis contains two principal cannabinoids with varying affinity for cannabinoid (CB) receptors: THC is a partial agonist with moderate affinity for both CB1 and CB2 receptors whereas CBD has extremely low affinity for CB1 and CB2 receptors and signals through an unknown mechanism (Pertwee, 2008). Interestingly, pretreatment with a range of CBD doses has no effect on THC self-administration (Wakeford et al., 2017), indicating non-overlapping signaling pathways for each cannabinoid. CB1 receptors are expressed on presynaptic terminals throughout the CNS and are responsible for the psychoactive effects of cannabis, while CB2 receptors are primarily expressed on immune cells of the CNS and PNS and are primarily responsible for the antinociceptive and anti-inflammatory effects of cannabis (Pertwee, 2008). Both subtypes signal through inhibitory GPCR signaling pathways (similar to opioids), and activation of the receptors results in presynaptic inhibition (via activation of GIRK channels and inhibition of VG Ca2+ channels) and downregulation of cAMP-dependent signaling cascades. CB1 receptors are heavily expressed on presynaptic terminals of VTAGABA neurons as well as NAc dMSNs that target VTADA neurons (Szabo et al., 2002; Lupica et al., 2004), and activation of CB1 receptors reduces GABA-mediated inhibitory postsynaptic currents in VTA slices (Cheer et al., 2000). CB1-mediated disinhibition of VTADA neurons results in an increase in burst firing (French et al., 1997; Diana et al., 1998) and subsequent release of DA into the NAc (Ton et al., 1988; Chen et al., 1990; Fadda et al., 2006), and these effects are blocked by systemic or intra-VTA naloxone (Chen et al., 1990; Tanda et al., 1997). Similar to other drugs, the acute effects of cannabinoids on C-BG-T network activity are broad and engage multiple neurotransmitter systems. For example, acute THC increases both DA and glutamate signaling in the NAc and PFC (Pistis et al., 2002a, b), but decreases GABA signaling in the VTA and PFC (Cheer et al., 2000; Pistis et al., 2002a). Collectively, these alterations in signaling reduce inhibitory feedback within the C-BG-T and facilitate behavioral output. Alcohol consumption prior to cannabis use enhances plasma THC levels and increases self-reported euphoria in humans (Lukas and Orozco, 2001), indicating synergistic effects between the two drugs. Moreover, simultaneous administration of cannabinoids with psychostimulants or opioids enhances activation of VTADA neurons (Pistis et al., 2004), and simultaneous administration of THC and nicotine increases cFos activation throughout the C-BG-T (Valjent et al., 2002).
Alcohol
Despite its widespread use, much less is known about the mechanisms underlying the acute effects of alcohol. Alcohol activates dissociated VTADA neurons (Brodie et al., 1999) and induces DA release into the NAc (Weiss et al., 1993; Pontieri et al., 1996; Lecca et al., 2006), similar to other potentially addictive drugs. However, GABAA receptors are known to play a central role in the effects of alcohol (Hyytiä and Koob, 1995; Lobo and Harris, 2008). For example, alcohol potentiates GABAA signaling both in cortical slices and neuronal cultures (Aguayo, 1990; Reynolds and Prasad, 1991; Reynolds et al., 1992; Tatebayashi et al., 1998). Given that NAc dMSNs selectively inhibit VTAGABA neurons via GABAA-mediated signaling (Edwards et al., 2017), it is possible that alcohol facilitates phasic DA release from VTADA neurons via inactivation of local VTAGABA neurons. In support of this hypothesis, alcohol inhibits VTAGABA neurons (Steffensen et al., 2009), and intra-VTA infusion of GABAA agonists dose-dependently increase DA release (Kalivas et al., 1990). In addition to GABA, the acute effects of alcohol are dependent on glutamatergic signaling within the C-BG-T (Grant and Colombo, 1993; Krystal et al., 1994). Alcohol increases glutamate release in the NAc and VTA via activation of presynaptic D1 receptors (Nie et al., 1994; Xiao et al., 2009), suggesting that alcohol engages a feedforward loop for activation of VTADA neurons. Polydrug use with alcohol produces synergistic effects throughout the C-BG-T, likely as a result of alcohol’s unique pharmacological profile. For example, chronic pretreatment with nicotine enhances acute alcohol-induced DA release in the NAc (Johnson et al., 1995; Blomqvist et al., 1996), and elevated levels of DA, DOPAC, and HVA persist for over an hour (Tizabi et al., 2002, 2007; Ding et al., 2012). Additionally, alcohol and nicotine co-administration acutely increase production of BDNF and GDNF in the NAc (Truitt et al., 2015), along with increases in glutamatergic signaling in the VTA and PFC (Deehan et al., 2015; Engle et al., 2015). Notably, this wide activation of the C-BG-T network is absent following administration of either drug alone, demonstrating a unique mechanism of action for alcohol and nicotine polydrug use.
Mechanisms of Addiction: Long-Term Alterations
Long-term use of psychostimulants, nicotine, opioids, cannabinoids, and alcohol results in widespread and disparate changes throughout the C-BG-T network, yet there are notable alterations that are shared across drugs (Figure 5). These long-term adaptations contribute to transitions from binge/intoxication phases to withdrawal and negative affect, followed by preoccupation and compulsive drug-seeking (Koob and Volkow, 2016). For example, acute withdrawal produces a transient reduction in tonic DA levels in the NAc (Weiss et al., 1992; Diana et al., 1993, 1998; Hildebrand et al., 1998). This is followed by a persistent increase in excitability of VTADA neurons (Mansvelder and McGehee, 2000; Bloomfield et al., 2016; Creed et al., 2016; Langlois and Nugent, 2017; You et al., 2018), which contributes to enhanced cue-evoked phasic DA release during abstinence (Volkow et al., 2011). Conversely, withdrawal also produces a persistent reduction in long-term depression (LTD) and intrinsic excitability in NAc MSNs, as well as a reduction in striatal D2 receptor binding (Trifilieff and Martinez, 2013). As described earlier, striatal D2 receptors are primarily expressed on iMSNs, can serve as a “stop” signal on the C-BG-T circuit, and reduced D2 availability has been ubiquitously linked to a range of addictive diseases, including drug addiction and obesity (Volkow and Morales, 2015; Kravitz et al., 2016; Friend D.M. et al., 2017). Finally, these drugs all drive a persistent increase in ΔFosB expression in cortical pyramidal cells and NAc dMSNs (Lobo et al., 2013), which has been linked to drug-seeking during abstinence (Nestler et al., 2001). Importantly, although chronic use of any of these drugs results in a multitude of other transient and/or persistent changes across the C-BG-T network, it is beyond the scope of the current review to provide an exhaustive summary. Rather, the focus will be on changes in plasticity, morphology, and connectivity within the VTA, NAc, and PFC following both single and polydrug use, with an emphasis on how the antagonistic and synergistic effects of these drugs can differentially disrupt C-BG-T network dynamics.
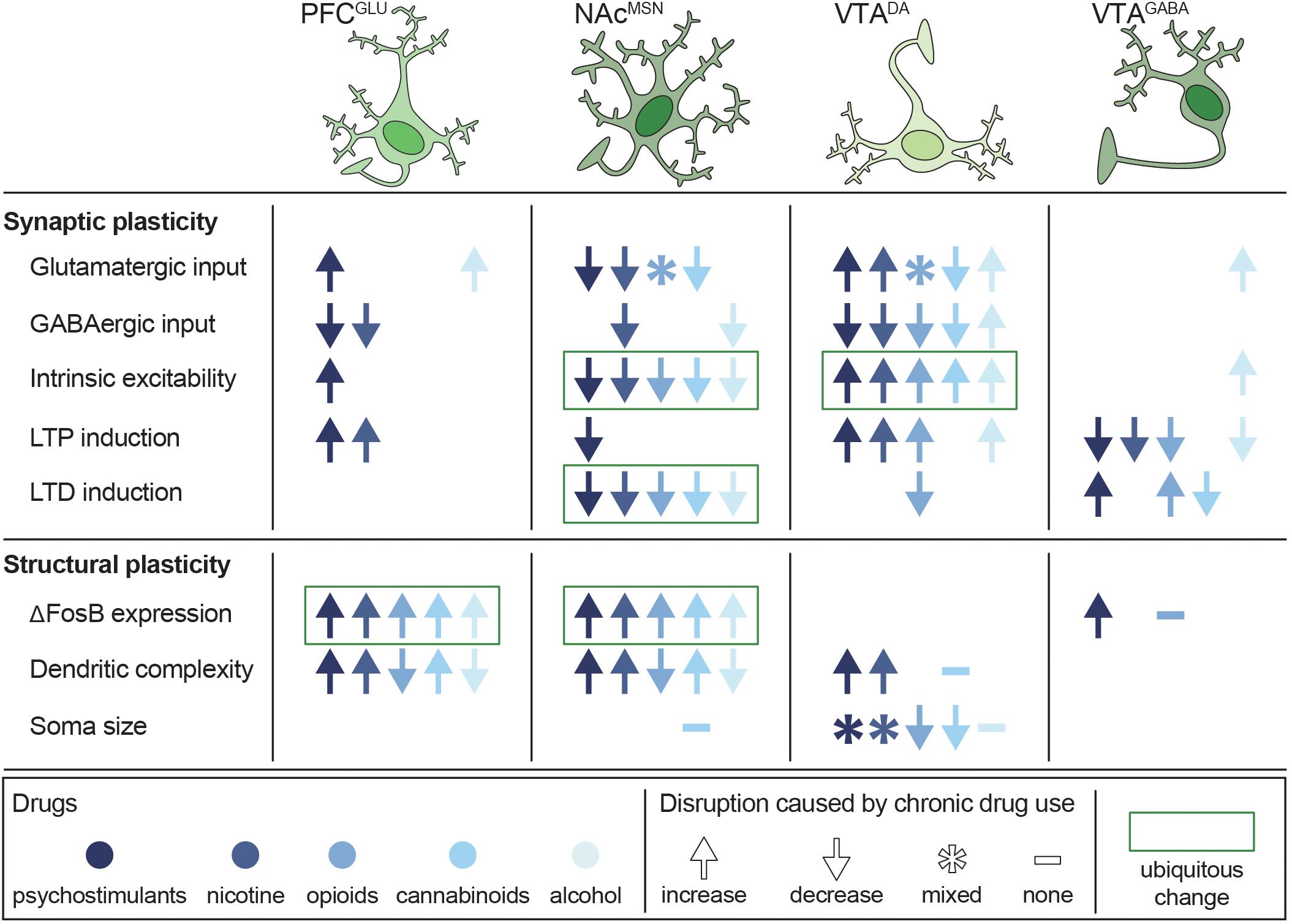
Figure 5. Persistent disruptions in synaptic and structural plasticity caused by long-term use of potentially addictive drugs. Studies are organized by cell type (columns) and type of disruption (rows), with symbols depicting the net change in plasticity and color depicting which drug was tested. Data from Bonci and Williams (1996), Kang et al. (1996, 1998), Robinson and Kolb (1997), Robinson and Kolb (2004), Badiani et al. (1999), Mansvelder and McGehee (2000), Dahchour and De Witte (2000), Uslaner et al. (2001), Brown and Kolb (2001), Robinson et al. (2002), Saal et al. (2003), Amantea et al. (2004), Hamilton and Kolb (2005), Nasif et al. (2005), Kolb et al. (2006), Kolb et al. (2018), Huang et al. (2007), Zhou et al. (2007), Van Den Oever et al. (2008), Kalivas et al. (2009), Niehaus et al. (2010), Russo et al. (2010), Bowers et al. (2010), Spiga et al. (2010), Levine et al. (2011), Dacher and Nugent (2011), Kroener et al. (2012), Lobo et al. (2013), Trifilieff and Martinez (2013), Mello et al. (2014), Peterson et al. (2015), Bloomfield et al. (2016), Creed et al. (2016), Ehlinger et al. (2016), Hearing et al. (2016), Morud et al. (2016), Friend L. et al., 2017), Langlois and Nugent (2017), Edwards et al. (2017), Spencer et al. (2018), You et al. (2018), Hwang and Lupica (2019), Kruse et al. (2019), McDevitt et al. (2019), Neuhofer et al. (2019), Pickel et al. (2019), and Ponzoni et al. (2019).
Psychostimulants
Psychostimulants produce long-term disruptions in glutamate homeostasis and alterations in neuronal morphology throughout the C-BG-T network (Kalivas, 2009; Badiani et al., 2011). Repeated cocaine administration weakens GABAA-mediated inhibition of prelimbic (PrL) pyramidal neurons, increasing their excitability and augmenting excitatory drive to the NAc (Nasif et al., 2005; Huang et al., 2007). Similarly, chronic cocaine increases glutamatergic input to the VTA (Saal et al., 2003; Bowers et al., 2010) and weakens GABAB-mediated inhibition of VTADA neurons (Bonci and Williams, 1996; Edwards et al., 2017), leading to a facilitation of VTADA neuron activity. Additionally, psychostimulants generate silent synapses on dMSNs via synaptogenesis (Boudreau et al., 2007; Graziane et al., 2016) and depress glutamate release from PrL inputs to the NAc core (Bamford and Wang, 2019), weakening striatal output. Importantly, cocaine-silenced synapses on dMSNs can be unsilenced during withdrawal via recruitment of AMPA receptors (Boudreau et al., 2007; Graziane et al., 2016), and a low dose psychostimulant challenge restores glutamate release into the NAc core (Boudreau et al., 2007; Bamford and Wang, 2019), suggesting the promotion of allostasis. Finally, repeated psychostimulant exposure increases expression of ΔFosB in PFC pyramidal neurons and NAc dMSNs (Perrotti et al., 2005), which contributes to an increase in dendritic branching that persists for at least 30 days of abstinence (Robinson and Kolb, 1997, 2004; Russo et al., 2010). Studies have not examined how these effects of psychostimulants are changed by polydrug use.
Nicotine
Similar to psychostimulants, long-term nicotine administration reduces GABAB-mediated signaling in the mPFC and NAc (Amantea et al., 2004), dampening inhibitory drive onto the C- BG-T network. Additionally, nicotine enhances glutamatergic input to VTADA neurons (Mansvelder and McGehee, 2000; Saal et al., 2003), and sensitizes evoked DA release into the NAc (Benwell et al., 1995). Repeated nicotine use also leads to an upregulation of nicotinic acetylcholine receptors throughout the C-BG-T, including the PFC and midbrain (Marks et al., 1983; Benwell et al., 1988; Breese et al., 1997). Conversely, chronic nicotine exposure weakens both glutamatergic and GABAergic inputs to the NAc during abstinence, potentially via enhanced sensitivity of NAc D2 receptors (Morud et al., 2016). Because NAc output is heavily regulated via local GABAergic microcircuitry, increased activity of iMSNs would likely dampen overall NAc output. This is supported by the upregulation of Ca2+-permeable AMPA receptors in the NAc that are capable of conducting Ca2+ in the absence of NMDA receptor activation (Ponzoni et al., 2019). Long-term nicotine use also produces a persistent increase in mPFC pyramidal cell and NAc MSN dendritic branching (Brown and Kolb, 2001; Hamilton and Kolb, 2005; Ehlinger et al., 2016), similar to psychostimulants. Moreover, adolescent nicotine use enhances synaptic pruning, microglial activation, and inflammatory cytokine expression throughout the C-BG-T via a D2 receptor-mediated mechanism (Linker et al., 2020). Repeated co-administration of psychostimulants and nicotine augments long-term potentiation (LTP) induction and ΔFosB in the NAc core (Levine et al., 2011; Mello et al., 2014). Interestingly, these effects are absent following sequential administration of these drugs (Levine et al., 2011), indicating a unique pharmacological profile for concurrent nicotine and psychostimulant administration.
Opioids
Similar to psychostimulants and nicotine, long-term exposure to opioids strengthens glutamatergic input to VTADA neurons (Bonci and Williams, 1996; Saal et al., 2003). They also weaken GABAergic inhibition of VTADA neurons (Mansvelder and McGehee, 2000; Niehaus et al., 2010; Dacher and Nugent, 2011) via a reduction of dMSN-mediated GABAB inhibition (Bonci and Williams, 1996). This effect is largely driven by MSNs in the NAc (Yang et al., 2018), indicating a persistent disruption in NAc output. Unlike psychostimulants and nicotine, however, long-term exposure to opioids decreases dendritic branching and spine density in NAc MSNs and mPFC pyramidal cells (Badiani et al., 1999; Robinson et al., 2002; Van Den Oever et al., 2008). Within the NAc, long-term opioid exposure weakens glutamatergic input to NAc shell iMSNs (Hearing et al., 2016; McDevitt et al., 2019) and induces silent synapses on iMSNs via AMPA internalization (Graziane et al., 2016). Moreover, during withdrawal, opioid-generated silent synapses on iMSNs are eliminated (Graziane et al., 2016), and the intrinsic excitability of iMSNs is weakened (McDevitt et al., 2019). Given that MSNs create a dense network of lateral inhibition within the NAc, with ∼30% of iMSNs synapsing onto other iMSNs or dMSNs (Taverna et al., 2008), it is possible that opioid-induced disruptions in iMSN signaling could disrupt local NAc microcircuitry and facilitate aberrant C-BG-T network dynamics. Studies have not examined how these effects of opioids are changed by polydrug use.
Cannabinoids
Repeated THC administration weakens glutamatergic signaling from the mPFC to the NAc (Spencer et al., 2018; Hwang and Lupica, 2019; Neuhofer et al., 2019) but strengthens input from the basolateral amygdala and ventral hippocampus to the NAc shell (Hwang and Lupica, 2019), suggesting a rewiring of excitatory input to the NAc following long-term cannabinoid exposure. Notably, repeated THC administration also occludes CB1-mediated LTD on VTAGABA neurons (Friend L. et al., 2017), indicating a loss of local inhibitory drive on VTADA neurons. Additionally, adolescent THC administration followed by abstinence reduces intrinsic excitability of PrL neurons (Pickel et al., 2019) and weakens glutamatergic input to the VTA (Kruse et al., 2019), indicating the presence of a hypoglutamatergic state induced by cannabinoids. THC exposure also produces long-lasting changes in morphology throughout the C-BG-T, increasing dendritic spine length and branching in the mPFC and NAc shell (Kolb et al., 2006) and dendritic spine density in the NAc shell (Kolb et al., 2018), but reducing the size of VTADA neurons (Spiga et al., 2010; Behan et al., 2012). Repeated co-administration of THC and nicotine enhances expression of ΔFosB in the NAc, and acute withdrawal dysregulates glutamatergic input to the NAc and PFC (Ponzoni et al., 2019).
Alcohol
Similar to opioids, extended alcohol exposure decreases dendritic branching and spine density in the NAc shell and mPFC (Zhou et al., 2007; Peterson et al., 2015), and withdrawal from alcohol reduces tonic DA levels in the NAc (Rossetti et al., 1992; Diana et al., 1993). However, alcohol withdrawal also causes distinct changes throughout the C-BG-T network, including reduced GABAergic signaling in the NAc and hippocampus (Kang et al., 1996, 1998; Dahchour and De Witte, 2000) and increased glutamatergic signaling in the NAc and PFC (Dahchour and De Witte, 2000; Kroener et al., 2012). Notably, this increased glutamatergic signaling is due to disrupted glutamate reuptake, rather than enhanced glutamate release (Pati et al., 2016). In vitro polydrug exposure to alcohol and nicotine induces a 2.5-fold increase in caspase-3 activation, elevating apoptotic cascades and driving cell death (Ramlochansingh et al., 2011). Interestingly, however, alcohol or nicotine withdrawal-induced neurodegeneration is less severe following co-administration of both drugs (Oliveira-da-Silva et al., 2010), indicating a unique molecular pathology following nicotine and alcohol polydrug use.
Conclusion
Improving the translatability and mapping of behavioral measures in preclinical models to accurately reflect polysubstance history and dependency in clinical populations is essential. This is particularly true, as human imaging studies are limited by an inability to control for intake history, making behavioral models in other species advantageous for assessing polydrug history under controlled intake conditions. However, these models are limited in their capacity to fully encompass the complex social and environmental contexts that contribute to the unique use patterns for multiple substances with addiction potential. Nevertheless, when designing experiments in clinical or preclinical populations, factors such as time of day of intake, temporal proximity of intake, and environmental preferences for administration must be consideredfor each substance class. For instance, cocaine use is predominantly favored outside of home environments, whereas heroin use is greater in “home” contexts in both humans and rodents (Caprioli et al., 2009; Badiani and Spagnolo, 2013; De Pirro et al., 2018; De Luca et al., 2019). In addition, route of drug administration (e.g. oral ingestion, injection, and inhalation) is especially important to factor into studies, as it leads to unique patterns of polysubstance history that may impact the developmentand severity of addiction behaviors (Roy et al., 2013).
Accounting for temporal patterns of drug use is also essential. Although many studies have focused on simultaneous drug combinations, sequential patterns of polydrug consumption are more frequently reported (Leri et al., 2004; Roy et al., 2013) and produce unique circuit adaptations following acute and repeated drug exposure (Cunha-Oliveira et al., 2008). Understanding sex differences in frequency and pattern of polydrug use (McClure et al., 2017), drug discrimination (Spence et al., 2016), and circuit alterations (Canterberry et al., 2016; Manwell et al., 2019) is also necessary to fully understand the interactions and impacts of polydrug use in clinical populations. Furthermore, although powerful behavioral economic models allow comparisons across drug classes, these experiments must be designed with consideration of different scales of intake and indifference points for drug valuation in order to accurately model parameters and interpret data (Newman and Ferrario, 2019). As these factors, coupled with the interplay of socioeconomic background, social environment, and access strategies, affect frequency and susceptibility to polydrug use (Gjersing et al., 2013; Hernández-Serrano et al., 2018), encompassing these influences in preclinical models is crucial for translational relevance of findings.
It is important to appreciate that novel preclinical paradigms are continuously being developed to model different routes of drug administration and to study relapse under clinically relevant conditions. For instance, recently established models allow for voluntary control over self-administration of vaporized ethanol (de Guglielmo et al., 2017; Kimbrough et al., 2017), cannabis (McLaughlin, 2018; Freels et al., 2020), and nicotine (Marusich et al., 2019). In addition, multiple models for inducing drug abstinence are being introduced. For instance, voluntary abstinence is achieved via pairing of drug-taking with adverse consequences such as shock-lever pairings or electrified barriers placed in front of levers (Krasnova et al., 2014; Venniro et al., 2016), andthrough choice procedures involving a drug versus an alternative reinforcer (e.g. palatable food or social interaction) (Venniro et al., 2016, 2017, 2019). These paradigms are notable for their high translational value and may be powerful for understanding the neural basis of therapies such as contingency management, in which abstinence from drug use results in a monetary reward. Combining these models with behavioral economic paradigms could clarify how the relative value of drugs and alternative reinforcers changes with polydrug use to improve treatment efficacy. Addiction is a complex disease with multiple, highly variable factors contributing to the initiation and maintenance of drug use, as well as relapse. Given the widespread prevalence of polydrug use among drug users, it is critical that we incorporate this variablility into human studies and animal models. This will help to determine if polysubstance use exacerbates SUD severity, if increased SUD severity drives polysubstance use, or if there is a bidirectional relationship between the two. Though challenging, understanding the behavioral, genetic, and environmental contributions to polysubstance use and addiction, as well as the mechanisms that underlie addiction-severity and relapse, will aid in developing efficacious treatment and policy strategies to combat this ongoing public health crisis.
Author Contributions
All authors wrote and revised the manuscript, as well as read and approved the submitted version.
Funding
Financial support for this work was provided by the National Science Foundation (GRFP DGE-1762114 to EC) and the National Institute on Drug Abuse (F31DA047012 to TO, T32DA007278 to BB, and R01DA036582 to SF). Any opinions, findings, and conclusions, or recommendations expressed in this material are those of the author(s) and do not necessarily reflect the views of the National Science Foundation.
Conflict of Interest
The authors declare that the research was conducted in the absence of any commercial or financial relationships that could be construed as a potential conflict of interest.
References
Aguayo, L. G. (1990). Ethanol potentiates the GABAA-activated Cl- current in mouse hippocampal and cortical neurons. Eur. J. Pharmacol. 187, 127–130. doi: 10.1016/0014-2999(90)90349-B
Aharonovich, E., Liu, X., Samet, S., Nunes, E., Waxman, R., and Hasin, D. (2005). Postdischarge cannabis use and its relationship to cocaine, alcohol, and heroin use: a prospective study. Am. J. Psychiatry 162, 1507–1514. doi: 10.1176/appi.ajp.162.8.1507
Al-Hasani, R., and Bruchas, M. R. (2011). Molecular mechanisms of opioid receptor-dependent signaling and behavior. Anesthesiology 115, 1363–1381. doi: 10.1097/ALN.0b013e318238bba6
Amantea, D., Tessari, M., and Bowery, N. G. (2004). Reduced G-protein coupling to the GABAB receptor in the nucleus accumbens and the medial prefrontal cortex of the rat after chronic treatment with nicotine. Neurosci. Lett. 355, 161–164. doi: 10.1016/j.neulet.2003.10.060
Arvidsson, U., Riedl, M., Chakrabarti, S., Lee, J. H., Nakano, A. H., Dado, R. J., et al. (1995). Distribution and targeting of a μ-opioid receptor (MOR1) in brain and spinal cord. J. Neurosci. 15, 3328–3341. doi: 10.1523/jneurosci.15-05-03328.1995
Aspen, J. M., and Winger, G. (1997). Ethanol effects on self-administration of alfentanil, cocaine, and nomifensine in rhesus monkeys. Psychopharmacology 130, 222–227. doi: 10.1007/s002130050232
Badiani, A., Belin, D., Epstein, D., Calu, D., and Shaham, Y. (2011). Opiate versus psychostimulant addiction: the differences do matter. Nat. Rev. Neurosci. 12, 685–700. doi: 10.1038/nrn3104
Badiani, A., Oates, M. M., Day, H. E. W., Watson, S. J., Akil, H., and Robinson, T. E. (1999). Environmental modulation of amphetamine-induced c-fos expression in D1 versus D2 striatal neurons. Behav. Brain Res. 103, 203–209. doi: 10.1016/S0166-4328(99)00041-48
Badiani, A., and Spagnolo, P. (2013). Role of environmental factors in cocaine addiction. Curr. Pharm. Des. 19, 6996–7008. doi: 10.2174/1381612819999131125221238
Ballard, M. E., and De Wit, H. (2011). Combined effects of acute, very-low-dose ethanol and delta(9)-tetrahydrocannabinol in healthy human volunteers. Pharmacol. Biochem. Behav. 97, 627–631. doi: 10.1016/j.pbb.2010.11.013
Bamford, N. S., and Wang, W. (2019). Corticostriatal plasticity in the nucleus accumbens core. J. Neurosci. Res. 97, 1559–1578. doi: 10.1002/jnr.24494
Batista-Brito, R., Vinck, M., Ferguson, K. A., Chang, J. T., Laubender, D., Lur, G., et al. (2017). Developmental dysfunction of VIP interneurons impairs cortical circuits. Neuron 95, 884–895. doi: 10.1016/j.neuron.2017.07.034
Bechtholt, A. J., and Mark, G. P. (2002). Enhancement of cocaine-seeking behavior by repeated nicotine exposure in rats. Psychopharmacology 162, 178–185. doi: 10.1007/s00213-002-1079-1071
Behan, ÁT., Hryniewiecka, M., O’Tuathaigh, C. M. P., Kinsella, A., Cannon, M., Karayiorgou, M., et al. (2012). Chronic adolescent exposure to delta-9-tetrahydrocannabinol in COMT mutant mice: impact on indices of dopaminergic, endocannabinoid and GABAergic pathways. Neuropsychopharmacology 37, 1773–1783. doi: 10.1038/npp.2012.24
Benowitz, N. L. (2009). Pharmacology of Nicotine: addiction, smoking-induced disease, and therapeutics. Annu. Rev. Pharmacol. Toxicol. 49, 57–71. doi: 10.1146/annurev.pharmtox.48.113006.094742
Benwell, M. E. M., Balfour, D. J. K., and Anderson, J. M. (1988). Evidence that tobacco smoking increases the density of (-)-[3H]Nicotine binding sites in human brain. J. Neurochem. 50, 1243–1247. doi: 10.1111/j.1471-4159.1988.tb10600.x
Benwell, M. E. M., Balfour, D. J. K., and Birrell, C. E. (1995). Desensitization of the nicotine-induced mesolimbic dopamine responses during constant infusion with nicotine. Br. J. Pharmacol. 114, 454–460. doi: 10.1111/j.1476-5381.1995.tb13248.x
Berrios, J., Stamatakis, A. M., Kantak, P. A., McElligott, Z. A., Judson, M. C., Aita, M., et al. (2016). Loss of UBE3A from TH-expressing neurons suppresses GABA co-release and enhances VTA-NAc optical self-stimulation. Nat. Commun. 7:10702. doi: 10.1038/ncomms10702
Bertran-Gonzalez, J., Bosch, C., Maroteaux, M., Matamales, M., Hervé, D., Valjent, E., et al. (2008). Opposing patterns of signaling activation in dopamine D1 and D2 receptor-expressing striatal neurons in response to cocaine and haloperidol. J. Neurosci. 28, 5671–5685. doi: 10.1523/JNEUROSCI.1039-08.2008
Biala, G., and Budzynska, B. (2006). Reinstatement of nicotine-conditioned place preference by drug priming: effects of calcium channel antagonists. Eur. J. Pharmacol. 537, 85–93. doi: 10.1016/j.ejphar.2006.03.017
Biala, G., and Weglinska, B. (2006). On the mechanism of cross-tolerance between morphine-and nicotine-induced antinociception: involvement of calcium channels. Prog. Neuro Psychopharmacol. Biol. Psychiatry 30, 15–21. doi: 10.1016/j.pnpbp.2005.04.021
Blackmer, T., Larsen, E. C., Bartleson, C., Kowalchyk, J. A., Yoon, E. J., Preininger, A. M., et al. (2005). G protein βγ directly regulates SNARE protein fusion machinery for secretory granule exocytosis. Nat. Neurosci. 8, 421–425. doi: 10.1038/nn1423
Blanchet, C., and Lüscher, C. (2002). Desensitization of μ-opioid receptor-evoked potassium currents: initiation at the receptor, expression at the effector. Proc. Natl. Acad. Sci. U.S.A. 99, 4674–4679. doi: 10.1073/pnas.072075399
Blomqvist, O., Ericson, M., Johnson, D. H., Engel, J. A., and Söderpalm, B. (1996). Voluntary ethanol intake in the rat: effects of nicotinic acetylcholine receptor blockade or subchronic nicotine treatment. Eur. J. Pharmacol. 314, 257–267. doi: 10.1016/S0014-2999(96)00583-583
Bloomfield, M. A. P., Ashok, A. H., Volkow, N. D., and Howes, O. D. (2016). The effects of Δ9-tetrahydrocannabinol on the dopamine system. Nature 539, 369–377. doi: 10.1038/nature20153
Bobashev, G., Tebbe, K., Peiper, N., and Hoffer, L. (2018). Polydrug use among heroin users in Cleveland, OH. Drug Alcohol Depend. 192, 80–87. doi: 10.1016/j.drugalcdep.2018.06.039
Bock, R., Hoon Shin, J., Kaplan, A. R., Dobi, A., Markey, E., Kramer, P. F., et al. (2013). Strengthening the accumbal indirect pathway promotes resilience to compulsive cocaine use. Nat. Neurosci. 16, 632–638. doi: 10.1038/nn.3369
Bonci, A., and Williams, J. T. (1996). A common mechanism mediates long-term changes in synaptic transmission after chronic cocaine and morphine. Neuron 16, 631–639. doi: 10.1016/S0896-6273(00)80082-80083
Boudreau, A. C., Reimers, J. M., Milovanovic, M., and Wolf, M. E. (2007). Cell surface AMPA receptors in the rat nucleus accumbens increase during cocaine withdrawal but internalize after cocaine challenge in association with altered activation of mitogen-activated protein kinases. J. Neurosci. 27, 10621–10635. doi: 10.1523/JNEUROSCI.2163-07.2007
Bourinet, E., Soong, T. W., Stea, A., and Snutch, T. P. (1996). Determinants of the G protein-dependent opioid modulation of neuronal calcium channels. Proc. Natl. Acad. Sci. U.S.A. 93, 1486–1491. doi: 10.1073/pnas.93.4.1486
Bowers, M. S., Chen, B. T., and Bonci, A. (2010). AMPA receptor synaptic plasticity induced by psychostimulants: the past, present, and therapeutic future. Neuron 67, 11–24. doi: 10.1016/j.neuron.2010.06.004
Brecht, M. L., Greenwell, L., and Anglin, M. D. (2007). Substance use pathways to methamphetamine use among treated users. Addict. Behav. 32, 24–38. doi: 10.1016/j.addbeh.2006.03.017
Breese, C. R., Marks, M. J., Logel, J., Adams, C. E., Sullivan, B., Collins, A. C., et al. (1997). Effect of smoking history on [3H]nicotine binding in human postmortem brain. J. Pharmacol. Exp. Ther. 282, 7–13.
Briggs, S. B., Hafenbreidel, M., Young, E. J., Rumbaugh, G., and Miller, C. A. (2018). The role of nonmuscle myosin II in polydrug memories and memory reconsolidation. Learn. Mem. 25, 391–398. doi: 10.1101/lm.046763.117
Brodie, M. S., Pesold, C., and Appel, S. B. (1999). Ethanol directly excites dopaminergic ventral tegmental area reward neurons. Alcohol. Clin. Exp. Res. 23, 1848–1852. doi: 10.1111/j.1530-0277.1999.tb04082.x
Brog, J. S., Salyapongse, A., Deutch, A. Y., and Zahm, D. S. (1993). The patterns of afferent innervation of the core and shell in the “Accumbens” part of the rat ventral striatum: immunohistochemical detection of retrogradely transported fluoro-gold. J. Comp. Neurol. 338, 255–278. doi: 10.1002/cne.903380209
Brown, M. T. C., Tan, K. R., O’Connor, E. C., Nikonenko, I., Muller, D., and Lüscher, C. (2012). Ventral tegmental area GABA projections pause accumbal cholinergic interneurons to enhance associative learning. Nature 492, 452–456. doi: 10.1038/nature11657
Brown, R. W., and Kolb, B. (2001). Nicotine sensitization increases dendritic length and spine density in the nucleus accumbens and cingulate cortex. Brain Res. 899, 94–100. doi: 10.1016/S0006-8993(01)02201-2206
Brown, S. P., and Hestrin, S. (2009). Intracortical circuits of pyramidal neurons reflect their long-range axonal targets. Nature 457, 1133–1136. doi: 10.1038/nature07658
Budney, A. J., Higgins, S. T., Hughes, J. R., and Bickel, W. K. (1993). Nicotine and caffeine use in cocaine-dependent individuals. J. Subst. Abuse 5, 117–130. doi: 10.1016/0899-3289(93)90056-H
Bujarski, S., Roche, D. J. O., Lunny, K., Moallem, N. R., Courtney, K. E., Allen, V., et al. (2014). The relationship between methamphetamine and alcohol use in a community sample of methamphetamine users. Drug Alcohol Depend. 142, 127–132. doi: 10.1016/j.drugalcdep.2014.06.004
Bunney, E. B., Appel, S. B., and Brodie, M. S. (2001). Electrophysiological effects of cocaethylene, cocaine, and ethanol on dopaminergic neurons of the ventral tegmental area. J. Pharmacol. Exp. Ther. 297, 696–703.
Burke, D. A., Rotstein, H. G., and Alvarez, V. A. (2017). Striatal local circuitry: a new framework for lateral inhibition. Neuron 96, 267–284. doi: 10.1016/j.neuron.2017.09.019
Busse, G. D., Verendeev, A., Jones, J., and Riley, A. L. (2005). The effects of cocaine, alcohol and cocaine/alcohol combinations in conditioned taste aversion learning. Pharmacol. Biochem. Behav. 82, 207–214. doi: 10.1016/j.pbb.2005.08.013
Calabresi, P., Lacey, M. G., and North, R. A. (1989). Nicotinic excitation of rat ventral tegmental neurones in vitro studied by intracellular recording. Br. J. Pharmacol. 98, 135–140. doi: 10.1111/j.1476-5381.1989.tb16873.x
Calabresi, P., Picconi, B., Tozzi, A., Ghiglieri, V., and Di Filippo, M. (2014). Direct and indirect pathways of basal ganglia: a critical reappraisal. Nat. Neurosci. 17, 1022–1030. doi: 10.1038/nn.3743
Canterberry, M., Peltier, M. R., Brady, K. T., and Hanlon, C. A. (2016). Attenuated neural response to emotional cues in cocaine-dependence: a preliminary analysis of gender differences. Am. J. Drug Alcohol Abuse 42, 577–586. doi: 10.1080/00952990.2016.1192183
Caprioli, D., Celentano, M., Dubla, A., Lucantonio, F., Nencini, P., and Badiani, A. (2009). Ambience and drug choice: cocaine-and heroin-taking as a function of environmental context in humans and rats. Biol. Psychiatry 65, 893–899. doi: 10.1016/j.biopsych.2008.12.009
Cazorla, M., DeCarvalho, F. D., Chohan, M. O., Shegda, M., Chuhma, N., Rayport, S., et al. (2014). Dopamine d2 receptors regulate the anatomical and functional balance of basal ganglia circuitry. Neuron 81, 153–164. doi: 10.1016/j.neuron.2013.10.041
Centers for Disease Control (2018). Multiple Cause of Death 1999-2016. CDC WONDER Online Database. Atlanta: Centers for Disease Control.
Cerdá, M., Moffitt, T. E., Meier, M. H., Harrington, H. L., Houts, R., Ramrakha, S., et al. (2016). Persistent Cannabis dependence and alcohol dependence represent risks for midlife economic and social problems: a longitudinal cohort study. Clin. Psychol. Sci. 4, 1028–1046. doi: 10.1177/2167702616630958
Chalmers, J., Bradford, D., and Jones, C. (2010). The effect of methamphetamine and heroin price on polydrug use: a behavioural economics analysis in Sydney, Australia. Int. J. Drug Policy 21, 381–389. doi: 10.1016/j.drugpo.2010.06.002
Chang, J. Y., Janak, P. H., and Woodward, D. J. (1998). Comparison of mesocorticolimbic neuronal responses during cocaine and heroin self-administration in freely moving rats. J. Neurosci. 18, 3098–3115. doi: 10.1523/jneurosci.18-08-03098.1998
Cheer, J. F., Marsden, C. A., Kendall, D. A., and Mason, R. (2000). Lack of response suppression follows repeated ventral tegmental cannabinoid administration: an in vitro electrophysiological study. Neuroscience 99, 661–667. doi: 10.1016/S0306-4522(00)00241-244
Chen, J., Paredes, W., Li, J., Smith, D., Lowinson, J., and Gardner, E. L. (1990). Δ9-Tetrahydrocannabinol produces naloxone-blockable enhancement of presynaptic basal dopamine efflux in nucleus accumbens of conscious, freely-moving rats as measured by intracerebral microdialysis. Psychopharmacology 102, 156–162. doi: 10.1007/BF02245916
Chen, X., Sun, Y. C., Zhan, H., Kebschull, J. M., Fischer, S., Matho, K., et al. (2019). High-throughput mapping of long-range neuronal projection using in situ sequencing. Cell 179:772-786.e19. doi: 10.1016/j.cell.2019.09.023
Chou, S. P., Goldstein, R. B., Smith, S. M., Huang, B., Ruan, W. J., Zhang, H., et al. (2016). The epidemiology of DSM-5 nicotine use disorder: results from the national epidemiologic survey on alcohol and related conditions-III. J. Clin. Psychiatry 77, 1404–1412. doi: 10.4088/JCP.15m10114
Cicero, T. J., Ellis, M. S., and Kasper, Z. A. (2020). Polysubstance use: a broader understanding of substance use during the opioid crisis. Am. J. Public Health 110, 244–250. doi: 10.2105/AJPH.2019.305412
Cicero, T. J., Kasper, Z. A., and Ellis, M. S. (2018). Increased use of heroin as an initiating opioid of abuse: further considerations and policy implications. Addict. Behav. 87, 267–271. doi: 10.1016/j.addbeh.2018.05.030
Cichewicz, D. L., and McCarthy, E. A. (2003). Antinociceptive synergy between Δ9-tetrahydrocannabinol and opioids after oral administration. J. Pharmacol. Exp. Ther. 304, 1010–1015. doi: 10.1124/jpet.102.045575
Cole, J. C., Goudie, A. J., Field, M., Loverseed, A.-C., Charlton, S., and Sumnall, H. R. (2008). The effects of perceived quality on the behavioural economics of alcohol, amphetamine, cannabis, cocaine, and ecstasy purchases. Drug Alcohol Depend. 94, 183–190. doi: 10.1016/j.drugalcdep.2007.11.014
Cole, J. C., Sumnall, H. R., O’Shea, E., and Marsden, C. A. (2003). Effects of MDMA exposure on the conditioned place preference produced by other drugs of abuse. Psychopharmacology 166, 383–390. doi: 10.1007/s00213-002-1374-x
Connor, J. P., Gullo, M. J., Chan, G., Young, R. M. D., Hall, W. D., and Feeney, G. F. X. (2013). Polysubstance use in cannabis users referred for treatment: drug use profiles, psychiatric comorbidity and cannabis-related beliefs. Front. Psychiatry 4:79. doi: 10.3389/fpsyt.2013.00079
Cooper, Z. D., Shi, Y. G., and Woods, J. H. (2010). Reinforcer-dependent enhancement of operant responding in opioid-withdrawn rats. Psychopharmacology 212, 369–378. doi: 10.1007/s00213-010-1966-1969
Corre, J., van Zessen, R., Loureiro, M., Patriarchi, T., Tian, L., Pascoli, V., et al. (2018). Dopamine neurons projecting to medial shell of the nucleus accumbens drive heroin reinforcement. eLife 7:e39945. doi: 10.7554/eLife.39945
Cortright, J. J., Lorrain, D. S., Beeler, J. A., Tang, W. J., and Vezina, P. (2011). Previous exposure to Δ9-tetrahydrocannibinol enhances locomotor responding to but not self-administration of amphetamine. J. Pharmacol. Exp. Ther. 337, 724–733. doi: 10.1124/jpet.111.180208
Cortright, J. J., Sampedro, G. R., Neugebauer, N. M., and Vezina, P. (2012). Previous exposure to nicotine enhances the incentive motivational effects of amphetamine via nicotine-associated contextual stimuli. Neuropsychopharmacology 37, 2277–2284. doi: 10.1038/npp.2012.80
Cox, M. L., Haller, V. L., and Welch, S. P. (2007). Synergy between Δ9-tetrahydrocannabinol and morphine in the arthritic rat. Eur. J. Pharmacol. 567, 125–130. doi: 10.1016/j.ejphar.2007.04.010
Creed, M., Kaufling, J., Fois, G. R., Jalabert, M., Yuan, T., Lüscher, C., et al. (2016). Cocaine exposure enhances the activity of ventral tegmental area dopamine neurons via calcium-impermeable NMDARs. J. Neurosci. 36, 10759–10768. doi: 10.1523/JNEUROSCI.1703-16.2016
Crummy, E. A., Donckels, E. A., Baskin, B. M., Bentzley, B. S., and Ferguson, S. M. (2020). The impact of cocaine and heroin drug history on motivation and cue sensitivity in a rat model of polydrug abuse. Psychopharmacology 237, 55–68. doi: 10.1007/s00213-019-05349-5342
Cunha-Oliveira, T., Rego, A. C., and Oliveira, C. R. (2008). Cellular and molecular mechanisms involved in the neurotoxicity of opioid and psychostimulant drugs. Brain Res. Rev. 58, 192–208. doi: 10.1016/j.brainresrev.2008.03.002
Dacher, M., and Nugent, F. S. (2011). Morphine-induced modulation of LTD at GABAergic synapses in the ventral tegmental area. Neuropharmacology 61, 1166–1171. doi: 10.1016/j.neuropharm.2010.11.012
Dahchour, A., and De Witte, P. (2000). Taurine blocks the glutamate increase in the nucleus accumbens microdialysate of ethanol-dependent rats. Pharmacol. Biochem. Behav. 65, 345–350. doi: 10.1016/S0091-3057(99)00197-195
Dani, J. A., and De Biasi, M. (2001). Cellular mechanisms of nicotine addiction. Pharmacol. Biochem. Behav. 70, 439–446. doi: 10.1016/S0091-3057(01)00652-9
Darke, S., and Hall, W. (1995). Levels and correlates of polydrug use among heroin users and regular amphetamine users. Drug Alcohol Depend. 39, 231–235. doi: 10.1016/0376-8716(95)01171-1179
Dautan, D., Huerta-Ocampo, I., Witten, I. B., Deisseroth, K., Paul Bolam, J., Gerdjikov, T., et al. (2014). A major external source of cholinergic innervation of the striatum and nucleus accumbens originates in the brainstem. J. Neurosci. 34, 4509–4518. doi: 10.1523/JNEUROSCI.5071-13.2014
de Guglielmo, G., Kallupi, M., Cole, M. D., and George, O. (2017). Voluntary induction and maintenance of alcohol dependence in rats using alcohol vapor self-administration. Psychopharmacology 234, 2009–2018. doi: 10.1007/s00213-017-4608-4607
de la Fuente, L., Molist, G., Espelt, A., Barrio, G., Guitart, A., Bravo, M. J., et al. (2014). Mortality risk factors and excess mortality in a cohort of cocaine users admitted to drug treatment in Spain. J. Subst. Abuse Treat. 46, 219–226. doi: 10.1016/j.jsat.2013.07.001
De Luca, M. T., Montanari, C., Meringolo, M., Contu, L., Celentano, M., and Badiani, A. (2019). Heroin versus cocaine: opposite choice as a function of context but not of drug history in the rat. Psychopharmacology 236, 787–798. doi: 10.1007/s00213-018-5115-5111
De Moura, F. B., Withey, S. L., and Bergman, J. (2019). Enhancement of opioid antinociception by nicotine. J. Pharmacol. Exp. Ther. 371, 624–632. doi: 10.1124/jpet.119.261438
De Pirro, S., Galati, G., Pizzamiglio, L., and Badiani, A. (2018). The affective and neural correlates of heroin versus cocaine use in addiction are influenced by environmental setting but in opposite directions. J. Neurosci. 38, 5182–5195. doi: 10.1523/JNEUROSCI.0019-18.2018
De Vries, T. J., Shaham, Y., Homberg, J. R., Crombag, H., Schuurman, K., Dieben, J., et al. (2001). A cannabinoid mechanism in relapse to cocaine seeking. Nat. Med. 7, 1151–1154. doi: 10.1038/nm1001-1151
Deehan, G. A., Hauser, S. R., Waeiss, R. A., Knight, C. P., Toalston, J. E., Truitt, W. A., et al. (2015). Co-administration of ethanol and nicotine: the enduring alterations in the rewarding properties of nicotine and glutamate activity within the mesocorticolimbic system of female alcohol-preferring (P) rats. Psychopharmacology 232, 4293–4302. doi: 10.1007/s00213-015-4056-4051
Degenhardt, L., Charlson, F., Ferrari, A., Santomauro, D., Erskine, H., Mantilla-Herrara, A., et al. (2018). The global burden of disease attributable to alcohol and drug use in 195 countries and territories, 1990–2016: a systematic analysis for the Global Burden of Disease Study 2016. Lancet Psychiatry 5, 987–1012. doi: 10.1016/S2215-0366(18)30337-30337
Di Chiara, G., and Imperato, A. (1988). Drugs abused by humans preferentially increase synaptic dopamine concentrations in the mesolimbic system of freely moving rats. Proc. Natl. Acad. Sci. U.S.A. 85, 5274–5278. doi: 10.1073/pnas.85.14.5274
Diana, M., Melis, M., and Gessa, G. L. (1998). Increase in meso-prefrontal dopaminergic activity after stimulation of CB1 receptors by cannabinoids. Eur. J. Neurosci. 10, 2825–2830. doi: 10.1111/j.1460-9568.1998.00292.x
Diana, M., Pistis, M., Carboni, S., Gessa, G. L., and Rossetti, Z. L. (1993). Profound decrement of mesolimbic dopaminergic neuronal activity during ethanol withdrawal syndrome in rats: electrophysiological and biochemical evidence. Proc. Natl. Acad. Sci. U.S.A. 90, 7966–7969. doi: 10.1073/pnas.90.17.7966
Ding, Z. M., Katner, S. N., Rodd, Z. A., Truitt, W., Hauser, S. R., Deehan, G. A., et al. (2012). Repeated exposure of the posterior ventral tegmental area to nicotine increases the sensitivity of local dopamine neurons to the stimulating effects of ethanol. Alcohol 46, 217–223. doi: 10.1016/j.alcohol.2011.11.007
Dobbs, L. K. K., Kaplan, A. R. R., Lemos, J. C. C., Matsui, A., Rubinstein, M., and Alvarez, V. A. A. (2016). Dopamine regulation of lateral inhibition between striatal neurons gates the stimulant actions of Cocaine. Neuron 90, 1100–1113. doi: 10.1016/j.neuron.2016.04.031
Downey, L. A., King, R., Papafotiou, K., Swann, P., Ogden, E., Boorman, M., et al. (2013). The effects of cannabis and alcohol on simulated driving: influences of dose and experience. Accid. Anal. Prev. 50, 879–886. doi: 10.1016/j.aap.2012.07.016
Edwards, N. J., Tejeda, H. A., Pignatelli, M., Zhang, S., McDevitt, R. A., Wu, J., et al. (2017). Circuit specificity in the inhibitory architecture of the VTA regulates cocaine-induced behavior. Nat. Neurosci. 20, 438–448. doi: 10.1038/nn.4482
Ehlinger, D. G., Bergstrom, H. C., Burke, J. C., Fernandez, G. M., McDonald, C. G., and Smith, R. F. (2016). Adolescent nicotine-induced dendrite remodeling in the nucleus accumbens is rapid, persistent, and D1-dopamine receptor dependent. Brain Struct. Funct. 221, 133–145. doi: 10.1007/s00429-014-0897-893
Ellis, M. S., Kasper, Z. A., and Cicero, T. J. (2018). Twin epidemics: the surging rise of methamphetamine use in chronic opioid users. Drug Alcohol Depend. 193, 14–20. doi: 10.1016/j.drugalcdep.2018.08.029
Engle, S. E., McIntosh, J. M., and Drenan, R. M. (2015). Nicotine and ethanol cooperate to enhance ventral tegmental area AMPA receptor function via α6-containing nicotinic receptors. Neuropharmacology 91, 13–22. doi: 10.1016/j.neuropharm.2014.11.014
Ettenberg, A., Pettit, H. O., Bloom, F. E., and Koob, G. F. (1982). Heroin and cocaine intravenous self-administration in rats: mediation by separate neural systems. Psychopharmacology 78, 204–209. doi: 10.1007/BF00428151
Fadda, P., Scherma, M., Fresu, A., Collu, M., and Fratta, W. (2003). Baclofen antagonizes nicotine-, cocaine-, and morphine-induced dopamine release in the nucleus accumbens of rat. Synapse 50, 1–6. doi: 10.1002/syn.10238
Fadda, P., Scherma, M., Spano, M. S., Salis, P., Melis, V., Fattore, L., et al. (2006). Cannabinoid self-administration increases dopamine release in the nucleus accumbens. Neuroreport 17, 1629–1632. doi: 10.1097/01.wnr.0000236853.40221.8e
Falk, D. E., Yi, H. Y., and Hiller-Sturmhöfel, S. (2006). An epidemiologic analysis of co-occurring alcohol and tobacco use and disorders: findings from the National Epidemiologic Survey on Alcohol and Related Conditions. Alcohol Res. Heal. 29, 162–171.
Feltenstein, M. W., and See, R. E. (2008). The neurocircuitry of addiction: an overview. Br. J. Pharmacol. 154, 261–274. doi: 10.1038/bjp.2008.51
Ferguson, S. M., Eskenazi, D., Ishikawa, M., Wanat, M. J., Phillips, P. E. M., Dong, Y., et al. (2011). Transient neuronal inhibition reveals opposing roles of indirect and direct pathways in sensitization. Nat. Neurosci. 14, 22–24. doi: 10.1038/nn.2703
Ferguson, S. M., and Robinson, T. E. (2004). Amphetamine-evoked gene expression in striatopallidal neurons: regulation by corticostriatal afferents and the ERK/MAPK signaling cascade. J. Neurochem. 91, 337–348. doi: 10.1111/j.1471-4159.2004.02712.x
Foltin, R. W., Fischman, M. W., Pippen, P. A., and Kelly, T. H. (1993). Behavioral effects of cocaine alone and in combination with ethanol or marijuana in humans. Drug Alcohol Depend. 32, 93–106. doi: 10.1016/0376-8716(93)80001-U
Francesco, L., Julie, B., and Jane, S. (2003). Understanding polydrug use: review of heroin and cocaine co-use. Addiction 98, 7–22. doi: 10.1046/j.1360-0443.2003.00236.x
Fredriksson, I., Adhikary, S., Steensland, P., Vendruscolo, L. F., Bonci, A., Shaham, Y., et al. (2017). Prior Exposure to Alcohol Has No Effect on Cocaine Self-Administration and Relapse in Rats: evidence from a Rat Model that Does Not Support the Gateway Hypothesis. Neuropsychopharmacology 42, 1001–1011. doi: 10.1038/npp.2016.209
Freels, T. G., Baxter-Potter, L. N., Lugo, J. M., Glodosky, N. C., Wright, H. R., Baglot, S. L., et al. (2020). Vaporized cannabis extracts have reinforcing properties and support conditioned drug-seeking behavior in rats. J. Neurosci. 40, 2416–2419. doi: 10.1523/jneurosci.2416-19.2020
French, E. D., Dillon, K., and Wu, X. (1997). Cannabinoids excite dopamine neurons in the ventral tegmentum and substantia nigra. Neuroreport 8, 649–652. doi: 10.1097/00001756-199702100-199702114
Friedman, A. L., Meurice, C., and Jutkiewicz, E. M. (2019). Effects of adolescent Δ9-tetrahydrocannabinol exposure on the behavioral effects of cocaine in adult Sprague-Dawley rats. Exp. Clin. Psychopharmacol. 27, 326–337. doi: 10.1037/pha0000276
Friend, D. M., Devarakonda, K., O’Neal, T. J., Skirzewski, M., Papazoglou, I., Kaplan, A. R., et al. (2017). Basal Ganglia dysfunction contributes to physical inactivity in obesity. Cell Metab. 25, 312–321. doi: 10.1016/j.cmet.2016.12.001
Friend, L., Weed, J., Sandoval, P., Nufer, T., Ostlund, I., and Edwards, J. G. (2017). CB1-dependent long-term depression in ventral tegmental area GABA neurons: A novel target for marijuana. J. Neurosci. 37, 10943–10954. doi: 10.1523/JNEUROSCI.0190-17.2017
Frosch, D. L., Nahom, D., Shoptaw, S., and Jarvik, M. E. (2000). Associations between tobacco smoking and illicit drug use among methadone-maintained opiate-dependent individuals. Exp. Clin. Psychopharmacol. 8, 97–103. doi: 10.1037/1064-1297.8.1.97
Fu, Y., Matta, S. G., Gao, W., Brower, V. G., and Sharp, B. M. (2000). Systemic nicotine stimulates dopamine release in nucleus accumbens: Re-evaluation of the role of N-methyl-D-aspartate receptors in the ventral tegmental area. J. Pharmacol. Exp. Ther. 294, 458–465.
Gabbott, P. L. A., Warner, T. A., Jays, P. R. L., Salway, P., and Busby, S. J. (2005). Prefrontal cortex in the rat: projections to subcortical autonomic, motor, and limbic centers. J. Comp. Neurol. 492, 145–177. doi: 10.1002/cne.20738
Gagnon, D., Petryszyn, S., Sanchez, M. G., Bories, C., Beaulieu, J. M., De Koninck, Y., et al. (2017). Striatal neurons expressing D1 and D2 receptors are morphologically distinct and differently affected by dopamine denervation in mice. Sci. Rep. 7:41432. doi: 10.1038/srep41432
Garcia, A. F., Nakata, K. G., and Ferguson, S. M. (2018). Viral strategies for targeting cortical circuits that control cocaine-taking and cocaine-seeking in rodents. Pharmacol. Biochem. Behav. 174, 33–41. doi: 10.1016/j.pbb.2017.05.009
Gentzke, A. S., Creamer, M. L., Cullen, K. A., Ambrose, B. K., Willis, G., Jamal, A., et al. (2019). Vital signs: tobacco product use among middle and high school students -United States, 2011-2018. MMWR. Morb. Mortal. Wkly. Rep. 68, 157–164. doi: 10.15585/mmwr.mm6806e1
Gerfen, C. R., and Surmeier, D. J. (2011). Modulation of striatal projection systems by dopamine. Annu. Rev. Neurosci. 34, 441–466. doi: 10.1146/annurev-neuro-061010-113641
Giasson-Gariépy, K., Potvin, S., Ghabrash, M., Bruneau, J., and Jutras-Aswad, D. (2017). Cannabis and cue-induced craving in cocaine-dependent individuals: a pilot study. Addict. Behav. 73, 4–8. doi: 10.1016/j.addbeh.2017.03.025
Gilmore, D., Zorland, J., Akin, J., Johnson, J. A., Emshoff, J. G., and Kuperminc, G. P. (2018). Mortality risk in a sample of emergency department patients who use cocaine with alcohol and/or cannabis. Subst. Abus. 39, 266–270. doi: 10.1080/08897077.2017.1389799
Gjersing, L., Jonassen, K. V., Ravndal, E., Waal, H., Clausen, T., Biong, S., et al. (2013). Diversity in causes and characteristics of drug-induced deaths in an urban setting. Scand. J. Public Health 41, 119–125. doi: 10.1177/1403494812472007
Gomes, T., Tadrous, M., Mamdani, M. M., Paterson, J. M., and Juurlink, D. N. (2018). The burden of opioid-related mortality in the United States. JAMA Netw. Open 1:e180217. doi: 10.1001/jamanetworkopen.2018.0217
Goniewicz, M. L., Kuma, T., Gawron, M., Knysak, J., and Kosmider, L. (2013). Nicotine levels in electronic cigarettes. Nicotine Tob. Res. 15, 158–166. doi: 10.1093/ntr/nts103
Gorriti, M. A., Rodríguez De Fonseca, F., Navarro, M., and Palomo, T. (1999). Chronic (-)-Δ9-tetrahydrocannabinol treatment induces sensitization to the psychomotor effects of amphetamine in rats. Eur. J. Pharmacol. 365, 133–142. doi: 10.1016/S0014-2999(98)00851-856
Grant, B. F., Goldstein, R. B., Saha, T. D., Patricia Chou, S., Jung, J., Zhang, H., et al. (2015). Epidemiology of DSM-5 alcohol use disorder results from the national epidemiologic survey on alcohol and related conditions III. JAMA Psychiatry 72, 757–766. doi: 10.1001/jamapsychiatry.2015.0584
Grant, B. F., Saha, T. D., June Ruan, W., Goldstein, R. B., Patricia Chou, S., Jung, J., et al. (2016). Epidemiology of DSM-5 drug use disorder results from the national epidemiologic survey on alcohol and related conditions-III. JAMA Psychiatry 73, 39–47. doi: 10.1001/jamapsychiatry.2015.2132
Grant, K. A., and Colombo, G. (1993). Discriminative stimulus effects of ethanol: effect of training dose on the substitution of N-methyl-D-aspartate antagonists. J. Pharmacol. Exp. Ther. 264, 1241–1247.
Grant, K. M., Kelley, S. S., Agrawal, S., Meza, J. L., Meyer, J. R., and Romberger, D. J. (2007). Methamphetamine use in rural Midwesterners. Am. J. Addict. 16, 79–84. doi: 10.1080/10550490601184159
Graziane, N. M., Sun, S., Wright, W. J., Jang, D., Liu, Z., Huang, Y. H., et al. (2016). Opposing mechanisms mediate morphine-and cocaine-induced generation of silent synapses. Nat. Neurosci. 19, 912–925. doi: 10.1038/nn.4313
Greenwald, M. K., Lundahl, L. H., and Steinmiller, C. L. (2010). Sustained release d-amphetamine reduces cocaine but not speedball-seeking in buprenorphine-maintained volunteers: a test of dual-agonist pharmacotherapy for cocaine/heroin polydrug abusers. Neuropsychopharmacology 35, 2624–2637. doi: 10.1038/npp.2010.175
Griffin, E. A., Melas, P. A., Zhou, R., Li, Y., Mercado, P., Kempadoo, K. A., et al. (2017). Prior alcohol use enhances vulnerability to compulsive cocaine self-administration by promoting degradation of HDAC4 and HDAC5. Sci. Adv. 3:e1701682. doi: 10.1126/sciadv.1701682
Haghparast, A., Khani, A., Naderi, N., Alizadeh, A. M., and Motamedi, F. (2008). Repeated administration of nicotine attenuates the development of morphine tolerance and dependence in mice. Pharmacol. Biochem. Behav. 88, 385–392. doi: 10.1016/j.pbb.2007.09.010
Hamilton, D. A., and Kolb, B. (2005). Differential effects of nicotine and complex housing on subsequent experience-dependent structural plasticity in the nucleus accumbens. Behav. Neurosci. 119, 355–365. doi: 10.1037/0735-7044.119.2.355
Hasin, D. S., Saha, T. D., Kerridge, B. T., Goldstein, R. B., Chou, S. P., Zhang, H., et al. (2015). Prevalence of marijuana use disorders in the United States between 2001-2002 and 2012-2013. JAMA Psychiatry 72, 1235–1242. doi: 10.1001/jamapsychiatry.2015.1858
Hay, G. L., Baracz, S. J., Everett, N. A., Roberts, J., Costa, P. A., Arnold, J. C., et al. (2018). Cannabidiol treatment reduces the motivation to self-administer methamphetamine and methamphetamine-primed relapse in rats. J. Psychopharmacol. 32, 1369–1378. doi: 10.1177/0269881118799954
Hearing, M. C., Jedynak, J., Ebner, S. R., Ingebretson, A., Asp, A. J., Fischer, R. A., et al. (2016). Reversal of morphine-induced cell-type-specific synaptic plasticity in the nucleus accumbens shell blocks reinstatement. Proc. Natl. Acad. Sci. U.S.A. 113, 757–762. doi: 10.1073/pnas.1519248113
Hedegaard, H., Bastian, B. A., Trinidad, J. P., Spencer, M., and Warner, M. (2018). Drugs most frequently involved in drug overdose deaths: United states, 2011–2016. Natl. Vital Stat. Rep. 67, 1–15.
Heil, S. H., Badger, G. J., and Higgins, S. T. (2001). Alcohol dependence among Cocaine-dependent outpatients: demographics, drug use, treatment outcome and other characteristics. J. Stud. Alcohol. 62, 14–22. doi: 10.15288/jsa.2001.62.14
Heinz, A. J., Lilje, T. C., Kassel, J. D., and de Wit, H. (2012). Quantifying reinforcement value and demand for psychoactive substances in humans. Curr. Drug Abuse Rev. 5, 257–272. doi: 10.2174/1874473711205040002
Hemby, S. E., Martin, T. J., Co, C., Dworkin, S. I., and Smith, J. E. (1995). The effects of intravenous heroin administration on extracellular nucleus accumbens dopamine concentrations as determined by in vivo microdialysis. J. Pharmacol. Exp. Ther. 273, 591–598.
Hernández-Serrano, O., Gras, M. E., and Font-Mayolas, S. (2018). Concurrent and simultaneous use of cannabis and tobacco and its relationship with academic achievement amongst university students. Behav. Sci. 8:31. doi: 10.3390/bs8030031
Heymann, G., Jo, Y. S., Reichard, K. L., McFarland, N., Chavkin, C., Palmiter, R. D., et al. (2019). Synergy of distinct dopamine projection populations in behavioral reinforcement. Neuron 105:909-920.e5. doi: 10.1016/j.neuron.2019.11.024
Hildebrand, B. E., Nomikos, G. G., Hertel, P., Schilstrom, B., and Svensson, T. H. (1998). Reduced dopamine output in the nucleus accumbens but not in the medial prefrontal cortex in rats displaying a mecamylamine-precipitated nicotine withdrawal syndrome. Brain Res. 779, 214–225. doi: 10.1016/S0006-8993(97)01135-1139
Hofford, R. S., Beckmann, J. S., and Bardo, M. T. (2016). Rearing environment differentially modulates cocaine self-administration after opioid pretreatment: a behavioral economic analysis. Drug Alcohol Depend. 167, 89–94. doi: 10.1016/j.drugalcdep.2016.07.026
Horger, B. A., Giles, M. K., and Schenk, S. (1992). Preexposure to amphetamine and nicotine predisposes rats to self-administer a low dose of cocaine. Psychopharmacology 107, 271–276. doi: 10.1007/BF02245147
Huang, C. C., Lin, H. J., and Hsu, K. S. (2007). Repeated cocaine administration promotes long-term potentiation induction in rat medial prefrontal cortex. Cereb. Cortex 17, 1877–1888. doi: 10.1093/cercor/bhl096
Hunt, D. E., Lipton, D. S., Goldsmith, D., and Strug, D. (1984). Street pharmacology: uses of cocaine and heroin in the treatment of addiction. Drug Alcohol Depend. 13, 375–387. doi: 10.1016/0376-8716(84)90005-X
Huskinson, S. L., Freeman, K. B., and Woolverton, W. L. (2015). Self-administration of cocaine and remifentanil by monkeys under concurrent-access conditions. Psychopharmacology 232, 321–330. doi: 10.1007/s00213-014-3661-3668
Huston-Lyons, D., Sarkar, M., and Kornetsky, C. (1993). Nicotine and brain-stimulation reward: interactions with morphine, amphetamine and pimozide. Pharmacol. Biochem. Behav. 46, 453–457. doi: 10.1016/0091-3057(93)90378-90377
Hutchison, M. A., and Riley, A. L. (2012). Ethanol exposure during either adolescence or adulthood alters the rewarding effects of cocaine in adult rats. Pharmacol. Biochem. Behav. 101, 458–464. doi: 10.1016/j.pbb.2012.02.007
Hwang, E. K., and Lupica, C. R. (2019). Altered Corticolimbic control of the nucleus accumbens by long-term Δ 9-Tetrahydrocannabinol exposure. Biol. Psychiatry 87, 619–631. doi: 10.1016/j.biopsych.2019.07.024
Hyman, S. E., Malenka, R. C., and Nestler, E. J. (2006). Neural mechanisms of addiction: the role of reward-related learning and memory. Annu. Rev. Neurosci. 29, 565–598. doi: 10.1146/annurev.neuro.29.051605.113009
Hyytiä, P., and Koob, G. F. (1995). GABAA receptor antagonism in the extended amygdala decreases ethanol self-administration in rats. Eur. J. Pharmacol. 283, 151–159. doi: 10.1016/0014-2999(95)00314-B
Jensen, R. P., Luo, W., Pankow, J. F., Strongin, R. M., and Peyton, D. H. (2015). Hidden formaldehyde in e-cigarette aerosols. N. Engl. J. Med. 372, 392–394. doi: 10.1056/NEJMc1413069
John, W. S., and Wu, L. T. (2017). Trends and correlates of cocaine use and cocaine use disorder in the United States from 2011 to 2015. Drug Alcohol Depend. 180, 376–384. doi: 10.1016/j.drugalcdep.2017.08.031
John, W. S., Zhu, H., Mannelli, P., Subramaniam, G. A., Schwartz, R. P., McNeely, J., et al. (2019). Prevalence and patterns of opioid misuse and opioid use disorder among primary care patients who use tobacco. Drug Alcohol Depend. 194, 468–475. doi: 10.1016/j.drugalcdep.2018.11.011
Johnson, D. H., Blomqvist, O., Engel, J. A., and Söderpalm, B. (1995). Subchronic intermittent nicotine treatment enhances ethanol-induced locomotor stimulation and dopamine turnover in mice. Behav. Pharmacol. 6, 203–207.
Johnson, S. W., and North, R. A. (1992). Opioids excite dopamine neurons by hyperpolarization of local interneurons. J. Neurosci. 12, 483–488. doi: 10.1523/jneurosci.12-02-00483.1992
Jones, C. M., Einstein, E. B., and Compton, W. M. (2018). Changes in synthetic opioid involvement in drug overdose deaths in the United States, 2010-2016. JAMA J. Am. Med. Assoc. 319, 1819–1821. doi: 10.1001/jama.2018.2844
Kalivas, P. W. (2009). The glutamate homeostasis hypothesis of addiction. Nat. Rev. Neurosci. 10, 561–572. doi: 10.1038/nrn2515
Kalivas, P. W., Duffy, P., and Eberhardt, H. (1990). Modulation of A10 dopamine neurons by γ-aminobutyric acid agonists. J. Pharmacol. Exp. Ther. 253, 858–866.
Kalivas, P. W., LaLumiere, R. T., Knackstedt, L., and Shen, H. (2009). Glutamate transmission in addiction. Neuropharmacology 56, 169–173. doi: 10.1016/j.neuropharm.2008.07.011
Kalmbach, B. E., Johnston, D., and Brager, D. H. (2015). Cell-type specific channelopathies in the prefrontal cortex of the fmr1-/y mouse model of fragile X syndrome. eNeuro 2:ENEURO.0114-15. doi: 10.1523/ENEURO.0114-15.2015
Kang, M., Spigelman, I., Sapp, D. W., and Olsen, R. W. (1996). Persistent reduction of GABAA receptor-mediated inhibition in rat hippocampus after chronic intermittent ethanol treatment. Brain Res. 709, 221–228. doi: 10.1016/0006-8993(95)01274-1275
Kang, M.-H., Spigelman, I., and Olsen, R. W. (1998). Alteration in the sensitivity of GABA a receptors to Allosteric modulatory drugs in rat hippocampus after chronic intermittent ethanol treatment. Alcohol. Clin. Exp. Res. 22, 2165–2173. doi: 10.1111/j.1530-0277.1998.tb05930.x
Kariisa, M., Scholl, L., Wilson, N., Seth, P., and Hoots, B. (2019). Drug overdose deaths involving cocaine and psychostimulants with abuse potential-United States, 2003-2017. MMWR. Morb. Mortal. Wkly. Rep. 68, 388–395. doi: 10.15585/mmwr.mm6817a3
Kedia, S., Sell, M. A., and Relyea, G. (2007). Mono-versus polydrug abuse patterns among publicly funded clients. Subst. Abus. Treat. Prev. Policy 2:33. doi: 10.1186/1747-597X-2-33
Keeley, R. J., Bye, C., Trow, J., and McDonald, R. J. (2018). Adolescent THC exposure does not sensitize conditioned place preferences to subthreshold d-amphetamine in male and female rats. F1000Research 7:342. doi: 10.12688/f1000research.14029.2
Kelly, M. A., Low, M. J., Rubinstein, M., and Phillips, T. J. (2008). Role of dopamine D1-like receptors in methamphetamine locomotor responses of D2 receptor knockout mice. Genes, Brain Behav. 7, 568–577. doi: 10.1111/j.1601-183X.2008.00392.x
Kelly, P. J., Robinson, L. D., Baker, A. L., Deane, F. P., McKetin, R., Hudson, S., et al. (2017). Polysubstance use in treatment seekers who inject amphetamine: drug use profiles, injecting practices and quality of life. Addict. Behav. 71, 25–30. doi: 10.1016/j.addbeh.2017.02.006
Kim, E. J., Juavinett, A. L., Kyubwa, E. M., Jacobs, M. W., and Callaway, E. M. (2015). Three types of cortical layer 5 neurons that differ in brain-wide connectivity and function. Neuron 88, 1253–1267. doi: 10.1016/j.neuron.2015.11.002
Kim, J. I., Ganesan, S., Luo, S. X., Wu, Y. W., Park, E., Huang, E. J., et al. (2015). Aldehyde dehydrogenase 1a1 mediates a GABA synthesis pathway in midbrain dopaminergic neurons. Science 350, 102–106. doi: 10.1126/science.aac4690
Kimbrough, A., Kim, S., Cole, M., Brennan, M., and George, O. (2017). Intermittent Access to Ethanol drinking facilitates the transition to excessive drinking after chronic intermittent ethanol vapor exposure. Alcohol. Clin. Exp. Res. 41, 1502–1509. doi: 10.1111/acer.13434
Kolb, B., Gorny, G., Limebeer, C. L., and Parker, L. A. (2006). Chronic treatment with Δ-9-tetrahydrocannabinol alters the structure of neurons in the nucleus accumbens shell and medial prefrontal cortex of rats. Synapse 60, 429–436. doi: 10.1002/syn.20313
Kolb, B., Li, Y., Robinson, T., and Parker, L. A. (2018). THC alters alters morphology of neurons in medial prefrontal cortex, orbital prefrontal cortex, and nucleus accumbens and alters the ability of later experience to promote structural plasticity. Synapse 72:e22020. doi: 10.1002/syn.22020
Koob, G. F., and Volkow, N. D. (2010). Neurocircuitry of addiction. Neuropsychopharmacology 35, 217–238. doi: 10.1038/npp.2009.110
Koob, G. F., and Volkow, N. D. (2016). Neurobiology of addiction: a neurocircuitry analysis. Lancet Psychiatry 3, 760–773. doi: 10.1016/S2215-0366(16)00104-108
Kosowski, A. R., Cebers, G., Cebere, A., Swanhagen, A. C., and Liljequist, S. (2004). Nicotine-induced dopamine release in the nucleus accumbens is inhibited by the novel AMPA antagonist ZK200775 and the NMDA antagonist CGP39551. Psychopharmacology 175, 114–123. doi: 10.1007/s00213-004-1797-1797
Krasnova, I. N., Marchant, N. J., Ladenheim, B., McCoy, M. T., Panlilio, L. V., Bossert, J. M., et al. (2014). Incubation of methamphetamine and palatable food craving after punishment-induced abstinence. Neuropsychopharmacology 39, 2008–2016. doi: 10.1038/npp.2014.50
Kravitz, A. V., Freeze, B. S., Parker, P. R. L., Kay, K., Thwin, M. T., Deisseroth, K., et al. (2010). Regulation of parkinsonian motor behaviours by optogenetic control of basal ganglia circuitry. Nature 466, 622–626. doi: 10.1038/nature09159
Kravitz, A. V., O’Neal, T. J., and Friend, D. M. (2016). Do dopaminergic impairments underlie physical inactivity in people with obesity? Front. Hum. Neurosci. 10:514. doi: 10.3389/fnhum.2016.00514
Kreek, M. J. (1997). Opiate and cocaine addictions: challenge for pharmacotherapies. Pharmacol. Biochem. Behav. 57, 551–569. doi: 10.1016/s0091-3057(96)00440-6
Kroener, S., Mulholland, P. J., New, N. N., Gass, J. T., Becker, H. C., and Chandler, L. J. (2012). Chronic alcohol exposure alters behavioral and synaptic plasticity of the rodent prefrontal cortex. PLoS One 7:e37541. doi: 10.1371/journal.pone.0037541
Kruse, L. C., Cao, J. K., Viray, K., Stella, N., and Clark, J. J. (2019). Voluntary oral consumption of Δ9-tetrahydrocannabinol by adolescent rats impairs reward-predictive cue behaviors in adulthood. Neuropsychopharmacology 44, 1406–1414. doi: 10.1038/s41386-019-0387-387
Krystal, J. H., Karper, L. P., Seibyl, J. P., Freeman, G. K., Delaney, R., Bremner, J. D., et al. (1994). Subanesthetic effects of the noncompetitive NMDA antagonist, ketamine, in humans: psychotomimetic, perceptual, cognitive, and neuroendocrine responses. Arch. Gen. Psychiatry 51, 199–214. doi: 10.1001/archpsyc.1994.03950030035004
Kuhn, B. N., Kalivas, P. W., and Bobadilla, A. C. (2019). Understanding addiction using animal models. Front. Behav. Neurosci. 13:262. doi: 10.3389/fnbeh.2019.00262
Kupchik, Y. M., Brown, R. M., Heinsbroek, J. A., Lobo, M. K., Schwartz, D. J., and Kalivas, P. W. (2015). Coding the direct/indirect pathways by D1 and D2 receptors is not valid for accumbens projections. Nat. Neurosci. 18, 1230–1232. doi: 10.1038/nn.4068
Langlois, L. D., and Nugent, F. S. (2017). Opiates and plasticity in the ventral tegmental area. ACS Chem. Neurosci. 8, 1830–1838. doi: 10.1021/acschemneuro.7b00281
Lê, A. D., Funk, D., Lo, S., and Coen, K. (2014). Operant self-administration of alcohol and nicotine in a preclinical model of co-abuse. Psychopharmacology 231, 4019–4029. doi: 10.1007/s00213-014-3541-3542
Lê, A. D., Lo, S., Harding, S., Juzytsch, W., Marinelli, P. W., and Funk, D. (2010). Coadministration of intravenous nicotine and oral alcohol in rats. Psychopharmacology 208, 475–486. doi: 10.1007/s00213-009-1746-1746
Lecca, D., Cacciapaglia, F., Valentini, V., Gronli, J., Spiga, S., and Di Chiara, G. (2006). Preferential increase of extracellular dopamine in the rat nucleus accumbens shell as compared to that in the core during acquisition and maintenance of intravenous nicotine self-administration. Psychopharmacology 184, 435–446. doi: 10.1007/s00213-005-0280-284
Leri, F., Bruneau, J., and Stewart, J. (2003a). Understanding polydrug use: review of heroin and cocaine co-use. Addiction 98, 7–22.
Leri, F., Flores, J., Rajabi, H., and Stewart, J. (2003b). Effects of Cocaine in rats exposed to heroin. Neuropsychopharmacology 28, 2102–2116. doi: 10.1038/sj.npp.1300284
Leri, F., and Stewart, J. (2001). Drug-induced reinstatement to heroin and cocaine seeking: a rodent model of relapse in polydrug use. Exp. Clin. Psychopharmacol. 9, 297–306. doi: 10.1037/1064-1297.9.3.297
Leri, F., Stewart, J., Tremblay, A., and Bruneau, J. (2004). Heroin and cocaine co-use in a group of injection drug users in Montréal. J. Psychiatry Neurosci. 29, 40–47.
Levine, A., Huang, Y. Y., Drisaldi, B., Griffin, E. A., Pollak, D. D., Xu, S., et al. (2011). Molecular mechanism for a gateway drug: epigenetic changes initiated by nicotine prime gene expression by cocaine. Sci. Transl. Med. 3:107ra109. doi: 10.1126/scitranslmed.3003062
Li, Z., Chen, Z., Fan, G., Li, A., Yuan, J., and Xu, T. (2018). Cell-type-specific afferent innervation of the nucleus accumbens core and shell. Front. Neuroanat. 12:84. doi: 10.3389/fnana.2018.00084
Liang, J. H., Wang, K., Sun, H. L., and Han, R. (2006). Potentiating effect of tramadol on methamphetamine-induced behavioral sensitization in mice. Psychopharmacology 185, 1–10. doi: 10.1007/s00213-005-0260-268
Lichtman, A. H., Sheikh, S. M., Loh, H. H., and Martin, B. R. (2001). Opioid and cannabinoid modulation of precipitated withdrawal in δ9-tetrahydrocannabinol and morphine-dependent mice. J. Pharmacol. Exp. Ther. 298, 1007–1014.
Linker, K. E., Elabd, M. G., Tawadrous, P., Cano, M., Green, K. N., Wood, M. A., et al. (2020). Microglial activation increases cocaine self-administration following adolescent nicotine exposure. Nat. Commun. 11:306. doi: 10.1038/s41467-019-14173-14173
Liu, S., and Vivolo-Kantor, A. (2020). A latent class analysis of drug and substance use patterns among patients treated in emergency departments for suspected drug overdose. Addict. Behav. 101:106142. doi: 10.1016/j.addbeh.2019.106142
Liu, Y., Williamson, V., Setlow, B., Cottler, L. B., and Knackstedt, L. A. (2018). The importance of considering polysubstance use: lessons from cocaine research. Drug Alcohol Depend. 192, 16–28. doi: 10.1016/j.drugalcdep.2018.07.025
Lobo, I. A., and Harris, R. A. (2008). GABAA receptors and alcohol. Pharmacol. Biochem. Behav. 90, 90–94. doi: 10.1016/j.pbb.2008.03.006
Lobo, M. K., Zaman, S., Damez-Werno, D. M., Koo, J. W., Bagot, R. C., DiNieri, J. A., et al. (2013). ΔFosB induction in striatal medium spiny neuron subtypes in response to chronic pharmacological, emotional, and optogenetic stimuli. J. Neurosci. 33, 18381–18395. doi: 10.1523/JNEUROSCI.1875-13.2013
Lopez-Quintero, C., Granja, K., Hawes, S., Duperrouzel, J. C., Pacheco-Colón, I., and Gonzalez, R. (2018). Transition to drug co-use among adolescent cannabis users: the role of decision-making and mental health. Addict. Behav. 85, 43–50. doi: 10.1016/j.addbeh.2018.05.010
Lorvick, J., Browne, E. N., Lambdin, B. H., and Comfort, M. (2018). Polydrug use patterns, risk behavior and unmet healthcare need in a community-based sample of women who use cocaine, heroin or methamphetamine. Addict. Behav. 85, 94–99. doi: 10.1016/j.addbeh.2018.05.013
Lu, L., Xu, N. J., Ge, X., Yue, W., Su, W. J., Pei, G., et al. (2002). Reactivation of morphine conditioned place preference by drug priming: role of environmental cues and sensitization. Psychopharmacology 159, 125–132. doi: 10.1007/s002130100885
Lukas, S. E., and Orozco, S. (2001). Ethanol increases plasma Δ9-tetrahydrocannabinol (THC) levels and subjective effects after marihuana smoking in human volunteers. Drug Alcohol Depend. 64, 143–149. doi: 10.1016/S0376-8716(01)00118-111
Lupica, C. R., Riegel, A. C., and Hoffman, A. F. (2004). Marijuana and cannabinoid regulation of brain reward circuits. Br. J. Pharmacol. 143, 227–234. doi: 10.1038/sj.bjp.0705931
Maguire, D. R., and France, C. P. (2016). Effects of daily delta-9-tetrahydrocannabinol treatment on heroin self-administration in rhesus monkeys. Behav. Pharmacol. 27, 249–257. doi: 10.1097/FBP.0000000000000192
Mahmud, A., Gallant, S., Sedki, F., D’Cunha, T., and Shalev, U. (2017). Effects of an acute cannabidiol treatment on cocaine self-administration and cue-induced cocaine seeking in male rats. J. Psychopharmacol. 31, 96–104. doi: 10.1177/0269881116667706
Mansvelder, H. D., and McGehee, D. S. (2000). Long-term potentiation of excitatory inputs to brain reward areas by nicotine. Neuron 27, 349–357. doi: 10.1016/S0896-6273(00)00042-48
Manwell, L. A., Miladinovic, T., Raaphorst, E., Rana, S., Malecki, S., and Mallet, P. E. (2019). Chronic nicotine exposure attenuates the effects of Δ9-tetrahydrocannabinol on anxiety-related behavior and social interaction in adult male and female rats. Brain Behav. 9:e01375. doi: 10.1002/brb3.1375
Marks, M. J., Burch, J. B., and Collins, A. C. (1983). Effects of chronic nicotinic infusion on tolerance development and nicotinic receptors. J. Pharmacol. Exp. Ther. 226, 817–825.
Marusich, J. A., Wiley, J. L., Silinski, M. A. R., Thomas, B. F., Meredith, S. E., Gahl, R. F., et al. (2019). Comparison of cigarette, little cigar, and waterpipe tobacco smoke condensate and e-cigarette aerosol condensate in a self-administration model. Behav. Brain Res. 372:112061. doi: 10.1016/j.bbr.2019.112061
Massaro, L. T. S., Abdalla, R. R., Laranjeira, R., Caetano, R., Pinsky, I., and Madruga, C. S. (2017). Amphetamine-type stimulant use and conditional paths of consumption: data from the second Brazilian national alcohol and drugs survey. Rev. Bras. Psiquiatr. 39, 201–207. doi: 10.1590/1516-4446-2015-1894
Mateos-García, A., Manzanedo, C., Rodríguez-Arias, M., Aguilar, M. A., Reig-Sanchis, E., Navarro-Francés, C. I., et al. (2015). Sex differences in the long-lasting consequences of adolescent ethanol exposure for the rewarding effects of cocaine in mice. Psychopharmacology 232, 2995–3007. doi: 10.1007/s00213-015-3937-3937
McCabe, S. E., Cranford, J. A., Morales, M., and Young, A. (2006). Simultaneous and concurrent polydrug use of alcohol and prescription drugs: prevalence, correlates, and consequences. J. Stud. Alcohol 67, 529–537. doi: 10.15288/jsa.2006.67.529
McClure, F. L., Niles, J. K., Kaufman, H. W., and Gudin, J. (2017). Concurrent use of Opioids and benzodiazepines: evaluation of prescription drug monitoring by a United States Laboratory. J. Addict. Med. 11, 420–426. doi: 10.1097/ADM.0000000000000354
McDevitt, D. S., Jonik, B., and Graziane, N. M. (2019). Morphine differentially alters the synaptic and Intrinsic properties of D1R-and D2R-expressing medium spiny neurons in the nucleus accumbens. Front. Synaptic Neurosci. 11:35. doi: 10.3389/fnsyn.2019.00035
McFarland, K., and Kalivas, P. W. (2001). The circuitry mediating cocaine-induced reinstatement of drug-seeking behavior. J. Neurosci. 21, 8655–8663. doi: 10.1523/jneurosci.21-21-08655.2001
McLaughlin, R. J. (2018). Toward a translationally relevant preclinical model of Cannabis use. Neuropsychopharmacol 431:213. doi: 10.1038/npp.2017.191
Mello, N. K., Fivel, P. A., and Kohut, S. J. (2013). Effects of chronic buspirone treatment on nicotine and concurrent nicotine+cocaine self-administration. Neuropsychopharmacology 38, 1264–1275. doi: 10.1038/npp.2013.25
Mello, N. K., Fivel, P. A., Kohut, S. J., and Carroll, F. I. (2014). Effects of chronic varenicline treatment on nicotine, cocaine, and concurrent Nicotine+Cocaine self-administration. Neuropsychopharmacology 39, 1222–1231. doi: 10.1038/npp.2013.325
Mello, N. K., and Mendelson, J. H. (1978). Marihuana, alcohol, and polydrug use: human self-administration studies. NIDA Res. Monogr. 20, 93–127.
Mello, N. K., Mendelson, J. H., Sellers, M. L., and Kuehnle, J. C. (1980). Effects of heroin self-administration on cigarette smoking. Psychopharmacology 67, 45–52. doi: 10.1007/BF00427594
Mello, N. K., and Newman, J. L. (2011). Discriminative and reinforcing stimulus effects of Nicotine, Cocaine, and Cocaine + Nicotine combinations in rhesus monkeys. Exp. Clin. Psychopharmacol. 19, 203–214. doi: 10.1037/a0023373
Moeller, S. J., Fink, D. S., Gbedemah, M., Hasin, D. S., Galea, S., Zvolensky, M. J., et al. (2018). Trends in illicit drug use among smokers and nonsmokers in the United States, 2002-2014. J. Clin. Psychiatry 79:17m11718. doi: 10.4088/JCP.17m11718
Mojarrad, M., Samet, J. H., Cheng, D. M., Winter, M. R., and Saitz, R. (2014). Marijuana use and achievement of abstinence from alcohol and other drugs among people with substance dependence: a prospective cohort study. Drug Alcohol Depend. 142, 91–97. doi: 10.1016/j.drugalcdep.2014.06.006
Molet, J., Hervé, D., Thiébot, M. H., Hamon, M., and Lanfumey, L. (2013). Juvenile ethanol exposure increases rewarding properties of cocaine and morphine in adult DBA/2J mice. Eur. Neuropsychopharmacol. 23, 1816–1825. doi: 10.1016/j.euroneuro.2013.03.010
Morales, M., and Margolis, E. B. (2017). Ventral tegmental area: cellular heterogeneity, connectivity and behaviour. Nat. Rev. Neurosci. 18, 73–85. doi: 10.1038/nrn.2016.165
Morishima, M., and Kawaguchi, Y. (2006). Recurrent connection patterns of corticostriatal pyramidal cells in frontal cortex. J. Neurosci. 26, 4394–4405. doi: 10.1523/JNEUROSCI.0252-06.2006
Morris, V., Patel, H., Vedelago, L., Reed, D. D., Metrik, J., Aston, E., et al. (2018). Elevated behavioral economic demand for alcohol in co-users of alcohol and cannabis. J. Stud. Alcohol Drugs 79, 929–934. doi: 10.15288/jsad.2018.79.929
Morud, J., Adermark, L., Perez-Alcazar, M., Ericson, M., and Söderpalm, B. (2016). Nicotine produces chronic behavioral sensitization with changes in accumbal neurotransmission and increased sensitivity to re-exposure. Addict. Biol. 21, 397–406. doi: 10.1111/adb.12219
Moyer, J. T., Wolf, J. A., and Finkel, L. H. (2007). Effects of dopaminergic modulation on the integrative properties of the ventral striatal medium spiny neuron. J. Neurophysiol. 98, 3731–3748. doi: 10.1152/jn.00335.2007
Muhuri, P. K., Gfroerer, J. C., and Davies, C. (2013). Associations of Nonmedical Pain Reliever Use and Initiation of Heroin Use in the United States. Rockville, MD: SAMHSA.
Naeger, S. (2017). Emergency Department Visits Involving Underage Alcohol Misuse: 2010 to 2013. Rockville, MD: Substance Abuse and Mental Health Services Administration.
Nasif, F. J., Sidiropoulou, K., Hu, X. T., and White, F. J. (2005). Repeated cocaine administration increases membrane excitability of pyramidal neurons in the rat medial prefrontal cortex. J. Pharmacol. Exp. Ther. 312, 1305–1313. doi: 10.1124/jpet.104.075184
National Institute on Drug Abuse (2020). Trends & Statistics. Bethesda, MA: National Institute on Drug Abuse.
Nestler, E. J., Barrot, M., and Self, D. W. (2001). ΔFosB: a sustained molecular switch for addiction. Proc. Natl. Acad. Sci. U.S.A. 98, 11042–11046. doi: 10.1073/pnas.191352698
Neuhofer, D., Spencer, S. M., Chioma, V. C., Beloate, L. N., Schwartz, D., and Kalivas, P. W. (2019). The loss of NMDAR-dependent LTD following cannabinoid self-administration is restored by positive allosteric modulation of CB1 receptors. Addict. Biol. doi: 10.1111/adb.12843 [Online ahead of print]
Newman, M., and Ferrario, C. R. (2019). An improved demand curve for analysis of food or drug consumption in animal experiments. bioRxiv [Prepint] doi: 10.1101/765461
Nguyen, J. D., Grant, Y., Creehan, K. M., Hwang, C. S., Vandewater, S. A., Janda, K. D., et al. (2019). Δ 9 -tetrahydrocannabinol attenuates oxycodone self-administration under extended access conditions. Neuropharmacology 151, 127–135. doi: 10.1016/j.neuropharm.2019.04.010
Nie, Z., Madamba, S. G., and Siggins, G. R. (1994). Ethanol inhibits glutamatergic neurotransmission in nucleus accumbens neurons by multiple mechanisms. J. Pharmacol. Exp. Ther. 271, 1566–1573.
Niehaus, J. L., Murali, M., and Kauer, J. A. (2010). Drugs of abuse and stress impair LTP at inhibitory synapses in the ventral tegmental area. Eur. J. Neurosci. 32, 108–117. doi: 10.1111/j.1460-9568.2010.07256.x
Nisell, M., Nomikos, G. G., and Svensson, T. H. (1994). Systemic nicotine-induced dopamine release in the rat nucleus accumbens is regulated by nicotinic receptors in the ventral tegmental area. Synapse 16, 36–44. doi: 10.1002/syn.890160105
Oleson, E. B., and Roberts, D. C. S. (2009). Behavioral economic assessment of price and cocaine consumption following self-administration histories that produce escalation of either final ratios or intake. Neuropsychopharmacology 34, 796–804. doi: 10.1038/npp.2008.195
Oliveira-da-Silva, A., Manhães, A. C., Cristina-Rodrigues, F., Filgueiras, C. C., and Abreu-Villaça, Y. (2010). Hippocampal increased cell death and decreased cell density elicited by nicotine and/or ethanol during adolescence are reversed during drug withdrawal. Neuroscience 167, 163–173. doi: 10.1016/j.neuroscience.2010.01.060
Olmstead, M. C., and Franklin, K. B. J. (1997). The development of a conditioned place preference to morphine: effects of lesions of various CNS sites. Behav. Neurosci. 111, 1313–1323. doi: 10.1037/0735-7044.111.6.1313
O’Neal, T. J., Nooney, M. N., Thien, K., and Ferguson, S. M. (2019). Chemogenetic modulation of accumbens direct or indirect pathways bidirectionally alters reinstatement of heroin-seeking in high-but not low-risk rats. Neuropsychopharmacology doi: 10.1038/s41386-019-0571-579 [Online ahead of print]
Onyeka, I. N., Uosukainen, H., Korhonen, M. J., Beynon, C., Bell, J. S., Ronkainen, K., et al. (2012). Sociodemographic characteristics and drug abuse patterns of treatment-seeking illicit drug abusers in finland, 1997-2008: the huuti study. J. Addict. Dis. 31, 350–362. doi: 10.1080/10550887.2012.735563
Padoa-Schioppa, C., and Conen, K. E. (2017). Orbitofrontal cortex: a neural circuit for economic decisions. Neuron 96, 736–754. doi: 10.1016/j.neuron.2017.09.031
Panlilio, L. V., and Goldberg, S. R. (2007). Self-administration of drugs in animals and humans as a model and an investigative tool. Addiction 102, 1863–1870. doi: 10.1111/j.1360-0443.2007.02011.x
Panlilio, L. V., Goldberg, S. R., and Justinova, Z. (2015). Cannabinoid abuse and addiction: clinical and preclinical findings. Clin. Pharmacol. Ther. 97, 616–627. doi: 10.1002/cpt.118
Panlilio, L. V., Solinas, M., Matthews, S. A., and Goldberg, S. R. (2007). Previous exposure to THC alters the reinforcing efficacy and anxiety-related effects of cocaine in rats. Neuropsychopharmacology 32, 646–657. doi: 10.1038/sj.npp.1301109
Panlilio, L. V., Zanettini, C., Barnes, C., Solinas, M., and Goldberg, S. R. (2013). Prior exposure to THC increases the addictive effects of nicotine in rats. Neuropsychopharmacology 38, 1198–1208. doi: 10.1038/npp.2013.16
Parker, L. A., Burton, P., Sorge, R. E., Yakiwchuk, C., and Mechoulam, R. (2004). Effect of low doses of Δ9-tetrahydrocannabinol and cannabidiol on the extinction of cocaine-induced and amphetamine-induced conditioned place preference learning in rats. Psychopharmacology 175, 360–366. doi: 10.1007/s00213-004-1825-1827
Pati, D., Kelly, K., Stennett, B., Frazier, C. J., and Knackstedt, L. A. (2016). Alcohol consumption increases basal extracellular glutamate in the nucleus accumbens core of Sprague–Dawley rats without increasing spontaneous glutamate release. Eur. J. Neurosci. 44, 1896–1905. doi: 10.1111/ejn.13284
Pattison, L. P., Mcintosh, S., Sexton, T., Childers, S. R., and Hemby, S. E. (2014). Changes in dopamine transporter binding in nucleus accumbens following chronic self-administration cocaine: heroin combinations. Synapse 68, 437–444. doi: 10.1002/syn.21755
Pennings, E. J. M., Leccese, A. P., and De Wolff, F. A. (2002). Effects of concurrent use of alcohol and cocaine. Addiction 97, 773–783. doi: 10.1046/j.1360-0443.2002.00158.x
Perrotti, L. I., Bolaños, C. A., Choi, K. H., Russo, S. J., Edwards, S., Ulery, P. G., et al. (2005). ΔFosB accumulates in a GABAergic cell population in the posterior tail of the ventral tegmental area after psychostimulant treatment. Eur. J. Neurosci. 21, 2817–2824. doi: 10.1111/j.1460-9568.2005.04110.x
Pertwee, R. G. (2008). The diverse CB 1 and CB 2 receptor pharmacology of three plant cannabinoids: Δ 9-tetrahydrocannabinol, cannabidiol and Δ 9-tetrahydrocannabivarin. Br. J. Pharmacol. 153, 199–215. doi: 10.1038/sj.bjp.0707442
Peterson, V. L., McCool, B. A., and Hamilton, D. A. (2015). Effects of ethanol exposure and withdrawal on dendritic morphology and spine density in the nucleus accumbens core and shell. Brain Res. 1594, 125–135. doi: 10.1016/j.brainres.2014.10.036
Petry, N. M. (2001). A behavioral economic analysis of polydrug abuse in alcoholics: asymmetrical substitution of alcohol and cocaine. Drug Alcohol Depend. 62, 31–39. doi: 10.1016/s0376-8716(00)00157-5
Petry, N. M., and Bickel, W. K. (1998). Polydrug abuse in heroin addicts: A behavioral economic analysis. Addiction 93, 321–335. doi: 10.1046/j.1360-0443.1998.9333212.x
Picciotto, M. R., and Kenny, P. J. (2013). Molecular mechanisms underlying behaviors related to nicotine addiction. Cold Spring Harb. Perspect. Med. 3:a012112. doi: 10.1101/cshperspect.a012112
Picciotto, M. R., and Mineur, Y. S. (2014). Molecules and circuits involved in nicotine addiction: the many faces of smoking. Neuropharmacology 76, 545–553. doi: 10.1016/j.neuropharm.2013.04.028
Pickel, V. M., Bourie, F., Chan, J., Mackie, K., Lane, D. A., and Wang, G. (2019). Chronic adolescent exposure to Δ9-tetrahydrocannabinol decreases NMDA current and extrasynaptic plasmalemmal density of NMDA GluN1 subunits in the prelimbic cortex of adult male mice. Neuropsychopharmacology 45, 374–383. doi: 10.1038/s41386-019-0466-469
Pidoplichko, V. I., DeBiasi, M., Wiliams, J. T., and Dani, J. A. (1997). Nicotine activates and desensitizes midbrain dopamine neurons. Nature 390, 401–404. doi: 10.1038/37120
Pistis, M., Ferraro, L., Pira, L., Flore, G., Tanganelli, S., Gessa, G. L., et al. (2002a). Δ9-Tetrahydrocannabinol decreases extracellular GABA and increases extracellular glutamate and dopamine levels in the rat prefrontal cortex: an in vivo microdialysis study. Brain Res. 948, 155–158. doi: 10.1016/S0006-8993(02)03055-X
Pistis, M., Muntoni, A. L., Pillolla, G., and Gessa, G. L. (2002b). Cannabinoids inhibit excitatory inputs to neurons in the shell of the nucleus accumbens: an in vivo electrophysiological study. Eur. J. Neurosci. 15, 1795–1802. doi: 10.1046/j.1460-9568.2002.02019.x
Pistis, M., Perra, S., Pillolla, G., Melis, M., Muntoni, A. L., and Gessa, G. L. (2004). Adolescent exposure to cannabinoids induces long-lasting changes in the response to drugs of abuse of rat midbrain dopamine neurons. Biol. Psychiatry 56, 86–94. doi: 10.1016/j.biopsych.2004.05.006
Plenz, D., and Kitai, S. T. (1998). Up and down states in striatal medium spiny neurons simultaneously recorded with spontaneous activity in fast-spiking interneurons studied in cortex-striatum-substantia nigra organotypic cultures. J. Neurosci. 18, 266–283. doi: 10.1523/jneurosci.18-01-00266.1998
Pomfrey, R. L., Bostwick, T. A., Wetzell, B. B., and Riley, A. L. (2015). Adolescent nicotine exposure fails to impact cocaine reward, aversion and self-administration in adult male rats. Pharmacol. Biochem. Behav. 137, 30–37. doi: 10.1016/j.pbb.2015.08.004
Pontieri, F. E., Tanda, G., and Di Chiara, G. (1995). Intravenous cocaine, morphine, and amphetamine preferentially increase extracellular dopamine in the “shell” as compared with the “core” of the rat nucleus accumbens. Proc. Natl. Acad. Sci. U.S.A. 92, 12304–12308. doi: 10.1073/pnas.92.26.12304
Pontieri, F. E., Tanda, G., Orzi, F., and Di Chiara, G. (1996). Effects of nicotine on the nucleus accumbens and similarity to those of addictive drugs. Nature 382, 255–257. doi: 10.1038/382255a0
Ponzoni, L., Moretti, M., Braida, D., Zoli, M., Clementi, F., Viani, P., et al. (2019). Increased sensitivity to Δ 9 -THC-induced rewarding effects after seven-week exposure to electronic and tobacco cigarettes in mice. Eur. Neuropsychopharmacol. 29, 566–576. doi: 10.1016/j.euroneuro.2019.02.001
Preston, K. L., Jobes, M. L., Phillips, K. A., and Epstein, D. H. (2016). Real-time assessment of alcohol drinking and drug use in opioid-dependent polydrug users. Behav. Pharmacol. 27, 579–584. doi: 10.1097/FBP.0000000000000250
Public Health England (2018). Drug Statistics from the National Drug Treatment Monitoring System (NDTMS). London: Public Health England, doi: 10.1038/1811181a0
Pugh, G., Smith, P. B., Dombrowski, D. S., and Welch, S. P. (1996). The role of endogenous opioids in enhancing the antinociception produced by the combination of Δ9-tetrahydrocannabinol and morphine in the spinal cord. J. Pharmacol. Exp. Ther. 279, 608–616.
Pugh, G., Welch, S. P., and Bass, P. P. (1994). Modulation of free intracellular calcium and cAMP by morphine and cannabinoids, alone and in combination in mouse brain and spinal cord synaptosomes. Pharmacol. Biochem. Behav. 49, 1093–1100. doi: 10.1016/0091-3057(94)90270-90274
Qi, J., Zhang, S., Wang, H. L., Barker, D. J., Miranda-Barrientos, J., and Morales, M. (2016). VTA glutamatergic inputs to nucleus accumbens drive aversion by acting on GABAergic interneurons. Nat. Neurosci. 19, 725–733. doi: 10.1038/nn.4281
Ramlochansingh, C., Taylor, R. E., and Tizabi, Y. (2011). Toxic effects of low alcohol and nicotine combinations in SH-SY5Y cells are apoptotically mediated. Neurotox. Res. 20, 263–269. doi: 10.1007/s12640-011-9239-x
Ranaldi, R., and Wise, R. A. (2000). Intravenous self-administration of methamphetamine-heroin (speedball) combinations under a progressive-ratio schedule of reinforcement in rats. Neuroreport 11, 2621–2623. doi: 10.1097/00001756-200008210-200008213
Reid, M. S., and Berger, S. P. (1996). Evidence for sensitization of cocaine-induced nucleus accumbens glutamate release. Neuroreport 7, 1325–1329. doi: 10.1097/00001756-199605170-199605122
Reid, M. S., Mickalian, J. D., Delucchi, K. L., Hall, S. M., and Berger, S. P. (1998). An acute dose of nicotine enhances cue-induced cocaine craving. Drug Alcohol Depend. 49, 95–104. doi: 10.1016/S0376-8716(97)00144-140
Reiner, A., Hart, N. M., Lei, W., and Deng, Y. (2010). Corticostriatal projection neurons-Dichotomous types and dichotomous functions. Front. Neuroanat. 4:142. doi: 10.3389/fnana.2010.00142
Reynolds, J. N., and Prasad, A. (1991). Ethanol enhances GABAA receptor-activated chloride currents in chick cerebral cortical neurons. Brain Res. 564, 138–142. doi: 10.1016/0006-8993(91)91363-91366
Reynolds, J. N., Prasad, A., and MacDonald, J. F. (1992). Ethanol modulation of GABA receptor-activated Cl-currents in neurons of the chick, rat and mouse central nervous system. Eur. J. Pharmacol. 224, 173–181. doi: 10.1016/0014-2999(92)90802-B
Robinson, T. E., Gorny, G., Savage, V. R., and Kolb, B. (2002). Widespread but regionally specific effects of experimenter-versus self-administered morphine on dendritic spines in the nucleus accumbens, hippocampus, and neocortex of adult rats. Synapse 46, 271–279. doi: 10.1002/syn.10146
Robinson, T. E., and Kolb, B. (1997). Persistent structural modifications in nucleus accumbens and prefrontal cortex neurons produced by previous experience with amphetamine. J. Neurosci. 17, 8491–8497. doi: 10.1523/jneurosci.17-21-08491.1997
Robinson, T. E., and Kolb, B. (2004). Structural plasticity associated with exposure to drugs of abuse. Neuropharmacology 47, 33–46. doi: 10.1016/j.neuropharm.2004.06.025
Rossetti, Z. L., Melis, F., Carboni, S., Diana, M., and Gessa, G. L. (1992). Alcohol Withdrawal in Rats Is Associated with a Marked Fall in Extraneuronal Dopamine. Alcohol. Clin. Exp. Res. 16, 529–532. doi: 10.1111/j.1530-0277.1992.tb01411.x
Roy, É, Richer, I., Arruda, N., Vandermeerschen, J., and Bruneau, J. (2013). Patterns of cocaine and opioid co-use and polyroutes of administration among street-based cocaine users in Montréal, Canada. Int. J. Drug Policy 24, 142–149. doi: 10.1016/j.drugpo.2012.10.004
Rubio, F. J., Quintana-Feliciano, R., Warren, B. L., Li, X., Witonsky, K. F. R., del Valle, F. S., et al. (2019). Prelimbic cortex is a common brain area activated during cue-induced reinstatement of cocaine and heroin seeking in a polydrug self-administration rat model. Eur. J. Neurosci. 49, 165–178. doi: 10.1111/ejn.14203
Russo, S. J., Dietz, D. M., Dumitriu, D., Morrison, J. H., Malenka, R. C., and Nestler, E. J. (2010). The addicted synapse: mechanisms of synaptic and structural plasticity in nucleus accumbens. Trends Neurosci. 33, 267–276. doi: 10.1016/j.tins.2010.02.002
Saal, D., Dong, Y., Bonci, A., and Malenka, R. C. (2003). Drugs of abuse and stress trigger a common synaptic adaptation in dopamine neurons. Neuron 37, 577–582. doi: 10.1016/S0896-6273(03)00021-27
Saha, T. D., Grant, B. F., Chou, S. P., Kerridge, B. T., Pickering, R. P., and Ruan, W. J. (2018). Concurrent use of alcohol with other drugs and DSM-5 alcohol use disorder comorbid with other drug use disorders: sociodemographic characteristics, severity, and psychopathology. Drug Alcohol Depend. 187, 261–269. doi: 10.1016/j.drugalcdep.2018.03.006
Saiki, A., Sakai, Y., Fukabori, R., Soma, S., Yoshida, J., Kawabata, M., et al. (2018). In Vivo Spiking Dynamics of Intra-and extratelencephalic projection neurons in rat motor cortex. Cereb. Cortex 28, 1024–1038. doi: 10.1093/cercor/bhx012
Santana, N., and Artigas, F. (2017). Laminar and cellular distribution of monoamine receptors in rat medial prefrontal cortex. Front. Neuroanat. 11:87. doi: 10.3389/fnana.2017.00087
Schmid, C. L., Kennedy, N. M., Ross, N. C., Lovell, K. M., Yue, Z., Morgenweck, J., et al. (2017). Bias factor and therapeutic window correlate to predict safer opioid analgesics. Cell 171, 1165–1175. doi: 10.1016/j.cell.2017.10.035
Schmidt, B. L., Tambeli, C. H., Gear, R. W., and Levine, J. D. (2001). Nicotine withdrawal hyperalgesia and opioid-mediated analgesia depend on nicotine receptors in nucleus accumbens. Neuroscience 106, 129–136. doi: 10.1016/S0306-4522(01)00264-260
Schwartz, L. P., Kearns, D. N., and Silberberg, A. (2018). The effect of nicotine pre-exposure on demand for cocaine and sucrose in male rats. Behav. Pharmacol. 29, 316–326. doi: 10.1097/FBP.0000000000000357
Sellings, L. H. L., and Clarke, P. B. S. (2003). Segregation of amphetamine reward and locomotor stimulation between nucleus accumbens medial shell and core. J. Neurosci. 23, 6295–6303. doi: 10.1523/jneurosci.23-15-06295.2003
Sentir, A. M., Bell, R. L., Engleman, E. A., and Chambers, R. A. (2020). Polysubstance addiction vulnerability in mental illness: concurrent alcohol and nicotine self-administration in the neurodevelopmental hippocampal lesion rat model of schizophrenia. Addict. Biol. 25:e12704. doi: 10.1111/adb.12704
Shin, C. B., Serchia, M. M., Shahin, J. R., Ruppert-Majer, M. A., Kippin, T. E., and Szumlinski, K. K. (2016). Incubation of cocaine-craving relates to glutamate over-flow within ventromedial prefrontal cortex. Neuropharmacology 102, 103–110. doi: 10.1016/j.neuropharm.2015.10.038
Shippenberg, T. S., Lefevour, A., and Thompson, A. C. (1998). Sensitization to the conditioned rewarding effects of morphine and cocaine: differential effects of the κ-opioid receptor agonist U69593. Eur. J. Pharmacol. 345, 27–34. doi: 10.1016/S0014-2999(97)01614-1612
Smith, P. A., Selley, D. E., Sim-Selley, L. J., and Welch, S. P. (2007). Low dose combination of morphine and Δ9-tetrahydrocannabinol circumvents antinociceptive tolerance and apparent desensitization of receptors. Eur. J. Pharmacol. 571, 129–137. doi: 10.1016/j.ejphar.2007.06.001
Smyth, B. P., Barry, J., Keenan, E., and Ducray, K. (2010). Lapse and relapse following inpatient treatment of opiate dependence. Ir. Med. J. 103, 176–179.
Solinas, M., Panlilio, L. V., and Goldberg, S. R. (2004). Exposure to Δ-9-Tetrahydrocannabinol (THC) increases subsequent heroin taking but not heroin’s reinforcing efficacy: a self-administration study in rats. Neuropsychopharmacology 29, 1301–1311. doi: 10.1038/sj.npp.1300431
Solinas, M., Panlilio, L. V., Tanda, G., Makriyannis, A., Matthews, S. A., and Goldberg, S. R. (2005). Cannabinoid agonists but not inhibitors of endogenous cannabinoid transport or metabolism enhance the reinforcing efficacy of heroin in rats. Neuropsychopharmacology 30, 2046–2057. doi: 10.1038/sj.npp.1300754
Spanagel, R. (2017). Animal models of addiction. Dialogues Clin. Neurosci. 19, 247–258. doi: 10.1093/med/9780199934959.003.0050
Spence, A. L., Guerin, G. F., and Goeders, N. E. (2016). Differential modulation of the discriminative stimulus effects of methamphetamine and cocaine by alprazolam and oxazepam in male and female rats. Neuropharmacology 102, 146–157. doi: 10.1016/j.neuropharm.2015.10.041
Spencer, S., Neuhofer, D., Chioma, V. C., Garcia-Keller, C., Schwartz, D. J., Allen, N., et al. (2018). A Model of Δ9-Tetrahydrocannabinol self-administration and reinstatement that alters synaptic plasticity in nucleus accumbens. Biol. Psychiatry 84, 601–610. doi: 10.1016/j.biopsych.2018.04.016
Spiga, S., Lintas, A., Migliore, M., and Diana, M. (2010). Altered architecture and functional consequences of the mesolimbic dopamine system in cannabis dependence. Addict. Biol. 15, 266–276. doi: 10.1111/j.1369-1600.2010.00218.x
Staiger, P. K., Richardson, B., Long, C. M., Carr, V., and Marlatt, G. A. (2013). Overlooked and underestimated? Problematic alcohol use in clients recovering from drug dependence. Addiction 108, 1188–1193. doi: 10.1111/j.1360-0443.2012.04075.x
Stefanik, M. T., Moussawi, K., Kupchik, Y. M., Smith, K. C., Miller, R. L., Huff, M. L., et al. (2013). Optogenetic inhibition of cocaine seeking in rats. Addict. Biol. 18, 50–53. doi: 10.1111/j.1369-1600.2012.00479.x
Steffensen, S. C., Walton, C. H., Hansen, D. M., Yorgason, J. T., Gallegos, R. A., and Criado, J. R. (2009). Contingent and non-contingent effects of low-dose ethanol on GABA neuron activity in the ventral tegmental area. Pharmacol. Biochem. Behav. 92, 68–75. doi: 10.1016/j.pbb.2008.10.012
Stennett, B. A., Padovan-Hernandez, Y., and Knackstedt, L. A. (2020). Sequential cocaine-alcohol self-administration produces adaptations in rat nucleus accumbens core glutamate homeostasis that are distinct from those produced by cocaine self-administration alone. Neuropsychopharmacology 45, 441–450. doi: 10.1038/s41386-019-0452-452
Shepherd, G. M. G. (2013). Corticostriatal connectivity and its role in disease. Nat. Rev. Neurosci. 14, 278–291. doi: 10.1038/nrn3469
Strickland, J. C., Lile, J. A., and Stoops, W. W. (2019). Evaluating non-medical prescription opioid demand using commodity purchase tasks: test-retest reliability and incremental validity. Psychopharmacology 236, 2641–2652. doi: 10.1007/s00213-019-05234-y
Subbaraman, M. S., and Kerr, W. C. (2015). Simultaneous versus concurrent use of alcohol and cannabis in the national alcohol survey. Alcohol. Clin. Exp. Res. 39, 872–879. doi: 10.1111/acer.12698
Subbaraman, M. S., Metrik, J., Patterson, D., and Swift, R. (2017). Cannabis use during treatment for alcohol use disorders predicts alcohol treatment outcomes. Addiction 112, 685–694. doi: 10.1111/add.13693
Substance Abuse, and Mental Health Services Administration (1993). National Household Survey on Drug Abuse: Population estimates, 1992. Rockville, MA: Substance Abuse, and Mental Health Services Administration.
Substance Abuse, and Mental Health Services Administration (1995). National Household Survey on Drug Abuse: Population estimates, 1994. Rockville, MA: Substance Abuse, and Mental Health Services Administration.
Substance Abuse, and Mental Health Services Administration (1997). National Household Survey on Drug Abuse: Population estimates, 1996. Rockville, MA: Substance Abuse, and Mental Health Services Administration.
Substance Abuse, and Mental Health Services Administration (2003). Results from the 2002 National Household Survey on Drug Abuse: National findings. Rockville, MA: Substance Abuse, and Mental Health Services Administration.
Substance Abuse, and Mental Health Services Administration (2005). Results from the 2004 National Survey on Drug Use and Health: National findings. Rockville, MA: Substance Abuse, and Mental Health Services Administration.
Substance Abuse, and Mental Health Services Administration (2007). Results from the 2006 National Survey on Drug Use and Health: National findings. Rockville, MA: Substance Abuse, and Mental Health Services Administration.
Substance Abuse, and Mental Health Services Administration (2009). Results from the 2008 National Survey on Drug Use and Health: National findings. Rockville, MA: Substance Abuse, and Mental Health Services Administration.
Substance Abuse, and Mental Health Services Administration (2011). Results from the 2010 National Survey on Drug Use and Health: Summary of National Findings. Rockville, MA: Substance Abuse, and Mental Health Services Administration.
Substance Abuse, and Mental Health Services Administration (2013). Results from the 2012 National Survey on Drug Use and Health: Summary of National Findings. Rockville, MA: Substance Abuse, and Mental Health Services Administration.
Substance Abuse, and Mental Health Services Administration (2015). Results from the 2014 National Survey on Drug Use and Health: Summary of National Findings. Rockville, MA: Substance Abuse, and Mental Health Services Administration.
Substance Abuse, and Mental Health Services Administration (2016). Treatment Episode Data Set (TEDS): 2004-2014. National Admissions to Substance Abuse Treatment. Rockville, MA: Substance Abuse, and Mental Health Services Administration.
Substance Abuse, and Mental Health Services Administration (2017). Key substance use and mental health indicators in the United States: Results from the 2016 National Survey on Drug Use and Health. Rockville, MA: Substance Abuse, and Mental Health Services Administration.
Substance Abuse, and Mental Health Services Administration (2019). Key substance use and mental health indicators in the United States: Results from the 2018 National Survey on Drug Use and Health. Rockville, MA: Substance Abuse, and Mental Health Services Administration.
Sumnall, H. R., Tyler, E., Wagstaff, G. F., and Cole, J. C. (2004). A behavioural economic analysis of alcohol, amphetamine, cocaine and ecstasy purchases by polysubstance misusers. Drug Alcohol Depend. 76, 93–99. doi: 10.1016/j.drugalcdep.2004.04.006
Szabo, B., Siemes, S., and Wallmichrath, I. (2002). Inhibition of GABAergic neurotransmission in the ventral tegmental area by cannabinoids. Eur. J. Neurosci. 15, 2057–2061. doi: 10.1046/j.1460-9568.2002.02041.x
Tanda, G., Pontieri, F. E., and Di Chiara, G. (1997). Cannabinoid and heroin activation of mesolimbic dopamine transmission by a common μ1 opioid receptor mechanism. Science 276, 2048–2050. doi: 10.1126/science.276.5321.2048
Tatebayashi, H., Motomura, H., and Narahashi, T. (1998). Alcohol modulation of single GABA(A) receptor-channel kinetics. Neuroreport 9, 1769–1775. doi: 10.1097/00001756-199806010-199806018
Taverna, S., Ilijic, E., and Surmeier, D. J. (2008). Recurrent collateral connections of striatal medium spiny neurons are disrupted in models of Parkinson’s disease. J. Neurosci. 28, 5504–5512. doi: 10.1523/JNEUROSCI.5493-07.2008
Tizabi, Y., Bai, L., Copeland, R. I., and Taylor, R. E. (2007). Combined effects of systemic alcohol and nicotine on dopamine release in the nucleus accumbens shell. Alcohol Alcohol. 42, 413–416. doi: 10.1093/alcalc/agm057
Tizabi, Y., Copeland, R. L., Louis, V. A., and Taylor, R. E. (2002). Effects of combined systemic alcohol and central nicotine administration into ventral tegmental area on dopamine release in the nucleus accumbens. Alcohol. Clin. Exp. Res. 26, 394–399. doi: 10.1111/j.1530-0277.2002.tb02551.x
Ton, J. M. N. C., Gerhardt, G. A., Friedemann, M., Etgen, A. M., Rose, G. M., Sharpless, N. S., et al. (1988). The effects of Δ9-tetrahydrocannabinol on potassium-evoked release of dopamine in the rat caudate nucleus: an in vivo electrochemical and in vivo microdialysis study. Brain Res. 451, 59–68. doi: 10.1016/0006-8993(88)90749-90744
Trifilieff, P., and Martinez, D. (2013). “Cocaine: mechanism and effects in the human brain,” in The Effects of Drug Abuse on the Human Nervous System, eds M. J. Kuhar and B. Madras (Amsterdam: Elsevier), doi: 10.1016/B978-0-12-418679-8.00005-8
Truitt, W. A., Hauser, S. R., Deehan, G. A., Toalston, J. E., Wilden, J. A., Bell, R. L., et al. (2015). Ethanol and nicotine interaction within the posterior ventral tegmental area in male and female alcohol-preferring rats: evidence of synergy and differential gene activation in the nucleus accumbens shell. Psychopharmacology 232, 639–649. doi: 10.1007/s00213-014-3702-3703
Tsui, J. I., Mayfield, J., Speaker, E. C., Yakup, S., Ries, R., Funai, H., et al. (2020). Association between methamphetamine use and retention among patients with opioid use disorders treated with buprenorphine. J. Subst. Abuse Treat. 109, 80–85. doi: 10.1016/j.jsat.2019.10.005
United Nations Office on Drugs and Crime (2019). World Drug Report 2019. Vienna: United Nations Office on Drugs and Crime.
Uslaner, J., Badiani, A., Norton, C. S., Day, H. E. W., Watson, S. J., Akil, H., et al. (2001). Amphetamine and cocaine induce different patterns of c-fos mRNA expression in the striatum and subthalamic nucleus depending on environmental context. Eur. J. Neurosci. 13, 1977–1983. doi: 10.1046/j.0953-816X.2001.01574.x
Valjent, E., Mitchell, J. M., Besson, M.-J., Caboche, J., and Maldonado, R. (2002). Behavioural and biochemical evidence for interactions between Δ9-tetrahydrocannabinol and nicotine. Br. J. Pharmacol. 135, 564–578. doi: 10.1038/sj.bjp.0704479
Valverde, O., Noble, F., Beslot, F., Daugé, V., Fournié-Zaluski, M. C., and Roques, B. P. (2001). Δ9-tetrahydrocannabinol releases and facilitates the effects of endogenous enkephalins: reduction in morphine withdrawal syndrome without change in rewarding effect. Eur. J. Neurosci. 13, 1816–1824. doi: 10.1046/j.0953-816X.2001.01558.x
Van Den Oever, M. C., Goriounova, N. A., Wan, Li, K., Van Der Schors, R. C., Binnekade, R., et al. (2008). Prefrontal cortex AMPA receptor plasticity is crucial for cue-induced relapse to heroin-seeking. Nat. Neurosci. 11, 1053–1058. doi: 10.1038/nn.2165
Van Ree, J. M., and Ramsey, N. (1987). The dopamine hypothesis of opiate reward challenged. Eur. J. Pharmacol. 134, 239–243. doi: 10.1016/0014-2999(87)90172-5
van Versendaal, D., and Levelt, C. N. (2016). Inhibitory interneurons in visual cortical plasticity. Cell. Mol. Life Sci. 73, 3677–3691. doi: 10.1007/s00018-016-2264-2264
Venniro, M., Caprioli, D., and Shaham, Y. (2016). Animal models of drug relapse and craving: from drug priming-induced reinstatement to incubation of craving after voluntary abstinence. Prog. Brain Res. 224, 25–52. doi: 10.1016/bs.pbr.2015.08.004
Venniro, M., Caprioli, D., and Shaham, Y. (2019). Novel models of drug relapse and craving after voluntary abstinence. Neuropsychopharmacology 44, 234–235. doi: 10.1038/s41386-018-0196-4
Venniro, M., Zhang, M., Shaham, Y., and Caprioli, D. (2017). Incubation of Methamphetamine but not heroin craving after voluntary abstinence in male and female rats. Neuropsychopharmacology 42, 1126–1135. doi: 10.1038/npp.2016.287
Vihavainen, T., Relander, T. R. A., Leiviskä, R., Airavaara, M., Tuominen, R. K., Ahtee, L., et al. (2008). Chronic nicotine modifies the effects of morphine on extracellular striatal dopamine and ventral tegmental GABA. J. Neurochem. 107, 844–854. doi: 10.1111/j.1471-4159.2008.05676.x
Volkow, N. D., and Boyle, M. (2018). Neuroscience of addiction: relevance to prevention and treatment. Am. J. Psychiatry 175, 729–740. doi: 10.1176/appi.ajp.2018.17101174
Volkow, N. D., and Morales, M. (2015). The brain on drugs: from reward to addiction. Cell 162, 712–725. doi: 10.1016/j.cell.2015.07.046
Volkow, N. D., Wang, G. J., Fowler, J. S., Tomasi, D., and Telang, F. (2011). Addiction: Beyond dopamine reward circuitry. Proc. Natl. Acad. Sci. U.S.A. 108, 15037–15042. doi: 10.1073/pnas.1010654108
Wade-Galuska, T., Galuska, C. M., and Winger, G. (2011). Effects of daily morphine administration and deprivation on choice and demand for remifentanil and cocaine in rhesus monkeys. J. Exp. Anal. Behav. 95, 75–89. doi: 10.1901/jeab.2011.95-75
Wade-Galuska, T., Galuska, C. M., Winger, G., and Woods, J. H. (2007). Aspartame demand in rhesus monkeys: effects of volume and concentration manipulations. Behav. Process. 74, 71–78. doi: 10.1016/j.beproc.2006.09.015
Wakeford, A. G. P., Wetzell, B. B., Pomfrey, R. L., Clasen, M. M., Taylor, W. W., Hempel, B. J., et al. (2017). The effects of cannabidiol (CBD) on Δ9-tetrahydrocannabinol (THC) self-administration in male and female Long-Evans rats. Exp. Clin. Psychopharmacol. 25, 242–248. doi: 10.1037/pha0000135
Wang, L., Min, J. E., Krebs, E., Evans, E., Huang, D., Liu, L., et al. (2017). Polydrug use and its association with drug treatment outcomes among primary heroin, methamphetamine, and cocaine users. Int. J. Drug Policy 49, 32–40. doi: 10.1016/j.drugpo.2017.07.009
Ward, S. J., Läck, C., Morgan, D., and Roberts, D. C. S. (2006). Discrete-trials heroin self-administration produces sensitization to the reinforcing effects of cocaine in rats. Psychopharmacology 185, 150–159. doi: 10.1007/s00213-005-0288-289
Weinberger, A. H., and Sofuoglu, M. (2009). The impact of cigarette smoking on stimulant addiction. Am. J. Drug Alcohol Abuse 35, 12–17. doi: 10.1080/00952990802326280
Weiss, F., Lorang, M. T., Bloom, F. E., and Koob, G. F. (1993). Oral alcohol self-administration stimulates dopamine release in the rat nucleus accumbens: genetic and motivational determinants. J. Pharmacol. Exp. Ther. 267, 250–258.
Weiss, F., Markou, A., Lorang, M. T., and Koob, G. F. (1992). Basal extracellular dopamine levels in the nucleus accumbens are decreased during cocaine withdrawal after unlimited-access self-administration. Brain Res. 593, 314–318. doi: 10.1016/0006-8993(92)91327-B
White, A. M., Hingson, R. W., I-jen, P., and Hsiao-ye, Y. (2011). Hospitalizations for alcohol and drug overdoses in young adults ages 18-24 in the United States, 1999-2008: results from the Nationwide inpatient sample. J. Stud. Alcohol Drugs 72, 774–786. doi: 10.15288/jsad.2011.72.774
Williamson, A., Darke, S., Ross, J., and Teesson, M. (2006). The effect of persistence of cocaine use on 12-month outcomes for the treatment of heroin dependence. Drug Alcohol Depend. 81, 293–300. doi: 10.1016/j.drugalcdep.2005.08.010
Winger, G., Galuska, C. M., and Hursh, S. R. (2007). Modification of ethanol’s reinforcing effectiveness in rhesus monkeys by cocaine, flunitrazepam, or gamma-hydroxybutyrate. Psychopharmacology 193, 587–598. doi: 10.1007/s00213-007-0809-809
Winkler, M. C., Greager, E. M., Stafford, J., and Bachtell, R. K. (2018). Methamphetamine self-administration reduces alcohol consumption and preference in alcohol-preferring P rats. Addict. Biol. 23, 90–101. doi: 10.1111/adb.12476
Winnubst, J., Bas, E., Ferreira, T. A., Wu, Z., Economo, M. N., Edson, P., et al. (2019). Reconstruction of 1,000 projection neurons reveals new cell types and organization of long-range connectivity in the mouse brain. Cell 179, 268–281. doi: 10.1016/j.cell.2019.07.042
Xiao, C., Shao, X. M., Olive, M. F., Griffin, W. C., Li, K. Y., Krnjević, K., et al. (2009). Ethanol facilitates glutamatergic transmission to dopamine neurons in the ventral tegmental area. Neuropsychopharmacology 34, 307–318. doi: 10.1038/npp.2008.99
Yang, H., de Jong, J. W., Tak, Y. E., Peck, J., Bateup, H. S., and Lammel, S. (2018). Nucleus Accumbens Subnuclei regulate motivated behavior via direct inhibition and disinhibition of VTA dopamine subpopulations. Neuron 97, 434–449. doi: 10.1016/j.neuron.2017.12.022
You, C., Vandegrift, B., and Brodie, M. S. (2018). Ethanol actions on the ventral tegmental area: novel potential targets on reward pathway neurons. Psychopharmacology 235, 1711–1726. doi: 10.1007/s00213-018-4875-y
You, Z. B., Wang, B., Zitzman, D., Azari, S., and Wise, R. A. (2007). A role for conditioned ventral tegmental glutamate release in cocaine seeking. J. Neurosci. 27, 10546–10555. doi: 10.1523/JNEUROSCI.2967-07.2007
Zale, E. L., Dorfman, M. L., Hooten, W. M., Warner, D. O., Zvolensky, M. J., and Ditre, J. W. (2015). Tobacco smoking, nicotine dependence, and patterns of prescription opioid misuse: results from a nationally representative sample. Nicotine Tob. Res. 17, 1096–1103. doi: 10.1093/ntr/ntu227
Zamponi, G. W., and Currie, K. P. M. (2013). Regulation of CaV2 calcium channels by G protein coupled receptors. Biochim. Biophys. Acta Biomembr. 1828, 1629–1643. doi: 10.1016/j.bbamem.2012.10.004
Zernig, G., O’Laughlin, I. A., and Fibiger, H. C. (1997). Nicotine and heroin augment cocaine-induced dopamine overflow in nucleus accumbens. Eur. J. Pharmacol. 337, 1–10. doi: 10.1016/S0014-2999(97)01184-1189
Zhou, F. C., Anthony, B., Dunn, K. W., Lindquist, W. B., Xu, Z. C., and Deng, P. (2007). Chronic alcohol drinking alters neuronal dendritic spines in the brain reward center nucleus accumbens. Brain Res. 1134, 148–161. doi: 10.1016/j.brainres.2006.11.046
Keywords: polydrug, substance use, addiction, reward circuitry, preclinical models, neuronal signaling and behavior, review, neurobiology of addiction
Citation: Crummy EA, O’Neal TJ, Baskin BM and Ferguson SM (2020) One Is Not Enough: Understanding and Modeling Polysubstance Use. Front. Neurosci. 14:569. doi: 10.3389/fnins.2020.00569
Received: 10 March 2020; Accepted: 08 May 2020;
Published: 16 June 2020.
Edited by:
Paul Meyer, University at Buffalo, United StatesReviewed by:
Gregory Carl Loney, University at Buffalo, United StatesGiordano de Guglielmo, University of California, San Diego, United States
Copyright © 2020 Crummy, O’Neal, Baskin and Ferguson. This is an open-access article distributed under the terms of the Creative Commons Attribution License (CC BY). The use, distribution or reproduction in other forums is permitted, provided the original author(s) and the copyright owner(s) are credited and that the original publication in this journal is cited, in accordance with accepted academic practice. No use, distribution or reproduction is permitted which does not comply with these terms.
*Correspondence: Susan M. Ferguson, smfergus@uw.edu